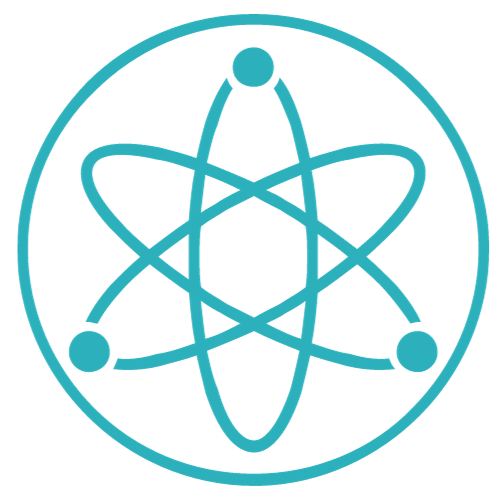
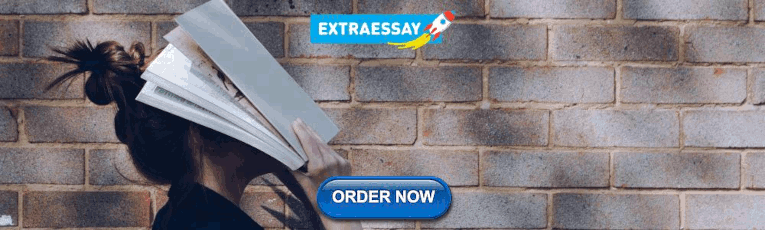
10 Unique Data Science Capstone Project Ideas
A capstone project is a culminating assignment that allows students to demonstrate the skills and knowledge they’ve acquired throughout their degree program. For data science students, it’s a chance to tackle a substantial real-world data problem.
If you’re short on time, here’s a quick answer to your question: Some great data science capstone ideas include analyzing health trends, building a predictive movie recommendation system, optimizing traffic patterns, forecasting cryptocurrency prices, and more .
In this comprehensive guide, we will explore 10 unique capstone project ideas for data science students. We’ll overview potential data sources, analysis methods, and practical applications for each idea.
Whether you want to work with social media datasets, geospatial data, or anything in between, you’re sure to find an interesting capstone topic.
Project Idea #1: Analyzing Health Trends
When it comes to data science capstone projects, analyzing health trends is an intriguing idea that can have a significant impact on public health. By leveraging data from various sources, data scientists can uncover valuable insights that can help improve healthcare outcomes and inform policy decisions.
Data Sources
There are several data sources that can be used to analyze health trends. One of the most common sources is electronic health records (EHRs), which contain a wealth of information about patient demographics, medical history, and treatment outcomes.
Other sources include health surveys, wearable devices, social media, and even environmental data.
Analysis Approaches
When analyzing health trends, data scientists can employ a variety of analysis approaches. Descriptive analysis can provide a snapshot of current health trends, such as the prevalence of certain diseases or the distribution of risk factors.
Predictive analysis can be used to forecast future health outcomes, such as predicting disease outbreaks or identifying individuals at high risk for certain conditions. Machine learning algorithms can be trained to identify patterns and make accurate predictions based on large datasets.
Applications
The applications of analyzing health trends are vast and far-reaching. By understanding patterns and trends in health data, policymakers can make informed decisions about resource allocation and public health initiatives.
Healthcare providers can use these insights to develop personalized treatment plans and interventions. Researchers can uncover new insights into disease progression and identify potential targets for intervention.
Ultimately, analyzing health trends has the potential to improve overall population health and reduce healthcare costs.
Project Idea #2: Movie Recommendation System
When developing a movie recommendation system, there are several data sources that can be used to gather information about movies and user preferences. One popular data source is the MovieLens dataset, which contains a large collection of movie ratings provided by users.
Another source is IMDb, a trusted website that provides comprehensive information about movies, including user ratings and reviews. Additionally, streaming platforms like Netflix and Amazon Prime also provide access to user ratings and viewing history, which can be valuable for building an accurate recommendation system.
There are several analysis approaches that can be employed to build a movie recommendation system. One common approach is collaborative filtering, which uses user ratings and preferences to identify patterns and make recommendations based on similar users’ preferences.
Another approach is content-based filtering, which analyzes the characteristics of movies (such as genre, director, and actors) to recommend similar movies to users. Hybrid approaches that combine both collaborative and content-based filtering techniques are also popular, as they can provide more accurate and diverse recommendations.
A movie recommendation system has numerous applications in the entertainment industry. One application is to enhance the user experience on streaming platforms by providing personalized movie recommendations based on individual preferences.
This can help users discover new movies they might enjoy and improve overall satisfaction with the platform. Additionally, movie recommendation systems can be used by movie production companies to analyze user preferences and trends, aiding in the decision-making process for creating new movies.
Finally, movie recommendation systems can also be utilized by movie critics and reviewers to identify movies that are likely to be well-received by audiences.
For more information on movie recommendation systems, you can visit https://www.kaggle.com/rounakbanik/movie-recommender-systems or https://www.researchgate.net/publication/221364567_A_new_movie_recommendation_system_for_large-scale_data .
Project Idea #3: Optimizing Traffic Patterns
When it comes to optimizing traffic patterns, there are several data sources that can be utilized. One of the most prominent sources is real-time traffic data collected from various sources such as GPS devices, traffic cameras, and mobile applications.
This data provides valuable insights into the current traffic conditions, including congestion, accidents, and road closures. Additionally, historical traffic data can also be used to identify recurring patterns and trends in traffic flow.
Other data sources that can be used include weather data, which can help in understanding how weather conditions impact traffic patterns, and social media data, which can provide information about events or incidents that may affect traffic.
Optimizing traffic patterns requires the use of advanced data analysis techniques. One approach is to use machine learning algorithms to predict traffic patterns based on historical and real-time data.
These algorithms can analyze various factors such as time of day, day of the week, weather conditions, and events to predict traffic congestion and suggest alternative routes.
Another approach is to use network analysis to identify bottlenecks and areas of congestion in the road network. By analyzing the flow of traffic and identifying areas where traffic slows down or comes to a halt, transportation authorities can make informed decisions on how to optimize traffic flow.
The optimization of traffic patterns has numerous applications and benefits. One of the main benefits is the reduction of traffic congestion, which can lead to significant time and fuel savings for commuters.
By optimizing traffic patterns, transportation authorities can also improve road safety by reducing the likelihood of accidents caused by congestion.
Additionally, optimizing traffic patterns can have positive environmental impacts by reducing greenhouse gas emissions. By minimizing the time spent idling in traffic, vehicles can operate more efficiently and emit fewer pollutants.
Furthermore, optimizing traffic patterns can have economic benefits by improving the flow of goods and services. Efficient traffic patterns can reduce delivery times and increase productivity for businesses.
Project Idea #4: Forecasting Cryptocurrency Prices
With the growing popularity of cryptocurrencies like Bitcoin and Ethereum, forecasting their prices has become an exciting and challenging task for data scientists. This project idea involves using historical data to predict future price movements and trends in the cryptocurrency market.
When working on this project, data scientists can gather cryptocurrency price data from various sources such as cryptocurrency exchanges, financial websites, or APIs. Websites like CoinMarketCap (https://coinmarketcap.com/) provide comprehensive data on various cryptocurrencies, including historical price data.
Additionally, platforms like CryptoCompare (https://www.cryptocompare.com/) offer real-time and historical data for different cryptocurrencies.
To forecast cryptocurrency prices, data scientists can employ various analysis approaches. Some common techniques include:
- Time Series Analysis: This approach involves analyzing historical price data to identify patterns, trends, and seasonality in cryptocurrency prices. Techniques like moving averages, autoregressive integrated moving average (ARIMA), or exponential smoothing can be used to make predictions.
- Machine Learning: Machine learning algorithms, such as random forests, support vector machines, or neural networks, can be trained on historical cryptocurrency data to predict future price movements. These algorithms can consider multiple variables, such as trading volume, market sentiment, or external factors, to make accurate predictions.
- Sentiment Analysis: This approach involves analyzing social media sentiment and news articles related to cryptocurrencies to gauge market sentiment. By considering the collective sentiment, data scientists can predict how positive or negative sentiment can impact cryptocurrency prices.
Forecasting cryptocurrency prices can have several practical applications:
- Investment Decision Making: Accurate price forecasts can help investors make informed decisions when buying or selling cryptocurrencies. By considering the predicted price movements, investors can optimize their investment strategies and potentially maximize their returns.
- Trading Strategies: Traders can use price forecasts to develop trading strategies, such as trend following or mean reversion. By leveraging predicted price movements, traders can make profitable trades in the volatile cryptocurrency market.
- Risk Management: Cryptocurrency price forecasts can help individuals and organizations manage their risk exposure. By understanding potential price fluctuations, risk management strategies can be implemented to mitigate losses.
Project Idea #5: Predicting Flight Delays
One interesting and practical data science capstone project idea is to create a model that can predict flight delays. Flight delays can cause a lot of inconvenience for passengers and can have a significant impact on travel plans.
By developing a predictive model, airlines and travelers can be better prepared for potential delays and take appropriate actions.
To create a flight delay prediction model, you would need to gather relevant data from various sources. Some potential data sources include:
- Flight data from airlines or aviation organizations
- Weather data from meteorological agencies
- Historical flight delay data from airports
By combining these different data sources, you can build a comprehensive dataset that captures the factors contributing to flight delays.
Once you have collected the necessary data, you can employ different analysis approaches to predict flight delays. Some common approaches include:
- Machine learning algorithms such as decision trees, random forests, or neural networks
- Time series analysis to identify patterns and trends in flight delay data
- Feature engineering to extract relevant features from the dataset
By applying these analysis techniques, you can develop a model that can accurately predict flight delays based on the available data.
The applications of a flight delay prediction model are numerous. Airlines can use the model to optimize their operations, improve scheduling, and minimize disruptions caused by delays. Travelers can benefit from the model by being alerted in advance about potential delays and making necessary adjustments to their travel plans.
Additionally, airports can use the model to improve resource allocation and manage passenger flow during periods of high delay probability. Overall, a flight delay prediction model can significantly enhance the efficiency and customer satisfaction in the aviation industry.
Project Idea #6: Fighting Fake News
With the rise of social media and the easy access to information, the spread of fake news has become a significant concern. Data science can play a crucial role in combating this issue by developing innovative solutions.
Here are some aspects to consider when working on a project that aims to fight fake news.
When it comes to fighting fake news, having reliable data sources is essential. There are several trustworthy platforms that provide access to credible news articles and fact-checking databases. Websites like Snopes and FactCheck.org are good starting points for obtaining accurate information.
Additionally, social media platforms such as Twitter and Facebook can be valuable sources for analyzing the spread of misinformation.
One approach to analyzing fake news is by utilizing natural language processing (NLP) techniques. NLP can help identify patterns and linguistic cues that indicate the presence of misleading information.
Sentiment analysis can also be employed to determine the emotional tone of news articles or social media posts, which can be an indicator of potential bias or misinformation.
Another approach is network analysis, which focuses on understanding how information spreads through social networks. By analyzing the connections between users and the content they share, it becomes possible to identify patterns of misinformation dissemination.
Network analysis can also help in identifying influential sources and detecting coordinated efforts to spread fake news.
The applications of a project aiming to fight fake news are numerous. One possible application is the development of a browser extension or a mobile application that provides users with real-time fact-checking information.
This tool could flag potentially misleading articles or social media posts and provide users with accurate information to help them make informed decisions.
Another application could be the creation of an algorithm that automatically identifies fake news articles and separates them from reliable sources. This algorithm could be integrated into news aggregation platforms to help users distinguish between credible and non-credible information.
Project Idea #7: Analyzing Social Media Sentiment
Social media platforms have become a treasure trove of valuable data for businesses and researchers alike. When analyzing social media sentiment, there are several data sources that can be tapped into. The most popular ones include:
- Twitter: With its vast user base and real-time nature, Twitter is often the go-to platform for sentiment analysis. Researchers can gather tweets containing specific keywords or hashtags to analyze the sentiment of a particular topic.
- Facebook: Facebook offers rich data for sentiment analysis, including posts, comments, and reactions. Analyzing the sentiment of Facebook posts can provide valuable insights into user opinions and preferences.
- Instagram: Instagram’s visual nature makes it an interesting platform for sentiment analysis. By analyzing the comments and captions on Instagram posts, researchers can gain insights into the sentiment associated with different images or topics.
- Reddit: Reddit is a popular platform for discussions on various topics. By analyzing the sentiment of comments and posts on specific subreddits, researchers can gain insights into the sentiment of different communities.
These are just a few examples of the data sources that can be used for analyzing social media sentiment. Depending on the research goals, other platforms such as LinkedIn, YouTube, and TikTok can also be explored.
When it comes to analyzing social media sentiment, there are various approaches that can be employed. Some commonly used analysis techniques include:
- Lexicon-based analysis: This approach involves using predefined sentiment lexicons to assign sentiment scores to words or phrases in social media posts. By aggregating these scores, researchers can determine the overall sentiment of a post or a collection of posts.
- Machine learning: Machine learning algorithms can be trained to classify social media posts into positive, negative, or neutral sentiment categories. These algorithms learn from labeled data and can make predictions on new, unlabeled data.
- Deep learning: Deep learning techniques, such as recurrent neural networks (RNNs) or convolutional neural networks (CNNs), can be used to capture the complex patterns and dependencies in social media data. These models can learn to extract sentiment information from textual or visual content.
It is important to note that the choice of analysis approach depends on the specific research objectives, available resources, and the nature of the social media data being analyzed.
Analyzing social media sentiment has a wide range of applications across different industries. Here are a few examples:
- Brand reputation management: By analyzing social media sentiment, businesses can monitor and manage their brand reputation. They can identify potential issues, respond to customer feedback, and take proactive measures to maintain a positive image.
- Market research: Social media sentiment analysis can provide valuable insights into consumer opinions and preferences. Businesses can use this information to understand market trends, identify customer needs, and develop targeted marketing strategies.
- Customer feedback analysis: Social media sentiment analysis can help businesses understand customer satisfaction levels and identify areas for improvement. By analyzing sentiment in customer feedback, companies can make data-driven decisions to enhance their products or services.
- Public opinion analysis: Researchers can analyze social media sentiment to study public opinion on various topics, such as political events, social issues, or product launches. This information can be used to understand public sentiment, predict trends, and inform decision-making.
These are just a few examples of how analyzing social media sentiment can be applied in real-world scenarios. The insights gained from sentiment analysis can help businesses and researchers make informed decisions, improve customer experience, and drive innovation.
Project Idea #8: Improving Online Ad Targeting
Improving online ad targeting involves analyzing various data sources to gain insights into users’ preferences and behaviors. These data sources may include:
- Website analytics: Gathering data from websites to understand user engagement, page views, and click-through rates.
- Demographic data: Utilizing information such as age, gender, location, and income to create targeted ad campaigns.
- Social media data: Extracting data from platforms like Facebook, Twitter, and Instagram to understand users’ interests and online behavior.
- Search engine data: Analyzing search queries and user behavior on search engines to identify intent and preferences.
By combining and analyzing these diverse data sources, data scientists can gain a comprehensive understanding of users and their ad preferences.
To improve online ad targeting, data scientists can employ various analysis approaches:
- Segmentation analysis: Dividing users into distinct groups based on shared characteristics and preferences.
- Collaborative filtering: Recommending ads based on users with similar preferences and behaviors.
- Predictive modeling: Developing algorithms to predict users’ likelihood of engaging with specific ads.
- Machine learning: Utilizing algorithms that can continuously learn from user interactions to optimize ad targeting.
These analysis approaches help data scientists uncover patterns and insights that can enhance the effectiveness of online ad campaigns.
Improved online ad targeting has numerous applications:
- Increased ad revenue: By delivering more relevant ads to users, advertisers can expect higher click-through rates and conversions.
- Better user experience: Users are more likely to engage with ads that align with their interests, leading to a more positive browsing experience.
- Reduced ad fatigue: By targeting ads more effectively, users are less likely to feel overwhelmed by irrelevant or repetitive advertisements.
- Maximized ad budget: Advertisers can optimize their budget by focusing on the most promising target audiences.
Project Idea #9: Enhancing Customer Segmentation
Enhancing customer segmentation involves gathering relevant data from various sources to gain insights into customer behavior, preferences, and demographics. Some common data sources include:
- Customer transaction data
- Customer surveys and feedback
- Social media data
- Website analytics
- Customer support interactions
By combining data from these sources, businesses can create a comprehensive profile of their customers and identify patterns and trends that will help in improving their segmentation strategies.
There are several analysis approaches that can be used to enhance customer segmentation:
- Clustering: Using clustering algorithms to group customers based on similar characteristics or behaviors.
- Classification: Building predictive models to assign customers to different segments based on their attributes.
- Association Rule Mining: Identifying relationships and patterns in customer data to uncover hidden insights.
- Sentiment Analysis: Analyzing customer feedback and social media data to understand customer sentiment and preferences.
These analysis approaches can be used individually or in combination to enhance customer segmentation and create more targeted marketing strategies.
Enhancing customer segmentation can have numerous applications across industries:
- Personalized marketing campaigns: By understanding customer preferences and behaviors, businesses can tailor their marketing messages to individual customers, increasing the likelihood of engagement and conversion.
- Product recommendations: By segmenting customers based on their purchase history and preferences, businesses can provide personalized product recommendations, leading to higher customer satisfaction and sales.
- Customer retention: By identifying at-risk customers and understanding their needs, businesses can implement targeted retention strategies to reduce churn and improve customer loyalty.
- Market segmentation: By identifying distinct customer segments, businesses can develop tailored product offerings and marketing strategies for each segment, maximizing the effectiveness of their marketing efforts.
Project Idea #10: Building a Chatbot
A chatbot is a computer program that uses artificial intelligence to simulate human conversation. It can interact with users in a natural language through text or voice. Building a chatbot can be an exciting and challenging data science capstone project.
It requires a combination of natural language processing, machine learning, and programming skills.
When building a chatbot, data sources play a crucial role in training and improving its performance. There are various data sources that can be used:
- Chat logs: Analyzing existing chat logs can help in understanding common user queries, responses, and patterns. This data can be used to train the chatbot on how to respond to different types of questions and scenarios.
- Knowledge bases: Integrating a knowledge base can provide the chatbot with a wide range of information and facts. This can be useful in answering specific questions or providing detailed explanations on certain topics.
- APIs: Utilizing APIs from different platforms can enhance the chatbot’s capabilities. For example, integrating a weather API can allow the chatbot to provide real-time weather information based on user queries.
There are several analysis approaches that can be used to build an efficient and effective chatbot:
- Natural Language Processing (NLP): NLP techniques enable the chatbot to understand and interpret user queries. This involves tasks such as tokenization, part-of-speech tagging, named entity recognition, and sentiment analysis.
- Intent recognition: Identifying the intent behind user queries is crucial for providing accurate responses. Machine learning algorithms can be trained to classify user intents based on the input text.
- Contextual understanding: Chatbots need to understand the context of the conversation to provide relevant and meaningful responses. Techniques such as sequence-to-sequence models or attention mechanisms can be used to capture contextual information.
Chatbots have a wide range of applications in various industries:
- Customer support: Chatbots can be used to handle customer queries and provide instant support. They can assist with common troubleshooting issues, answer frequently asked questions, and escalate complex queries to human agents when necessary.
- E-commerce: Chatbots can enhance the shopping experience by assisting users in finding products, providing recommendations, and answering product-related queries.
- Healthcare: Chatbots can be deployed in healthcare settings to provide preliminary medical advice, answer general health-related questions, and assist with appointment scheduling.
Building a chatbot as a data science capstone project not only showcases your technical skills but also allows you to explore the exciting field of artificial intelligence and natural language processing.
It can be a great opportunity to create a practical and useful tool that can benefit users in various domains.
Completing an in-depth capstone project is the perfect way for data science students to demonstrate their technical skills and business acumen. This guide outlined 10 unique project ideas spanning industries like healthcare, transportation, finance, and more.
By identifying the ideal data sources, analysis techniques, and practical applications for their chosen project, students can produce an impressive capstone that solves real-world problems and showcases their abilities.
Similar Posts
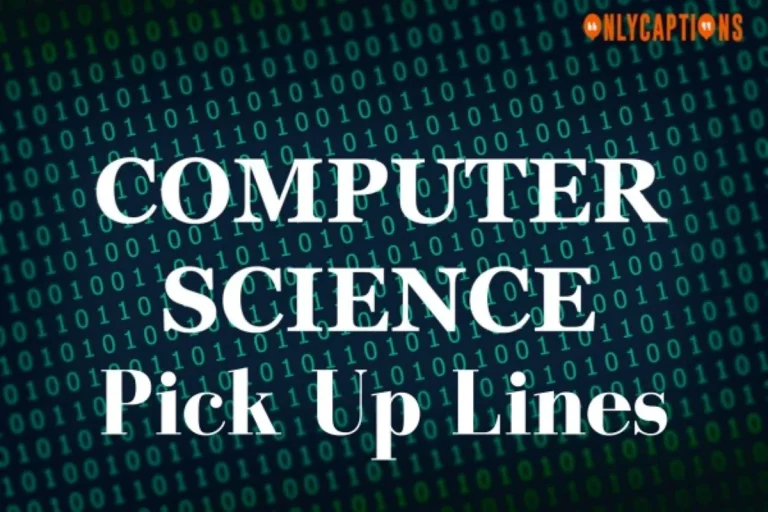
The Ultimate List Of Computer Science Pick Up Lines
Looking for some geeky ways to break the ice with your crush in the computer lab or woo fellow techies at a hackathon? Computer science pick up lines combine romantic intent with programming humor, offering a fun and flirty way to connect. Whether you’re a coding pro or total newbie, these pick up lines are…
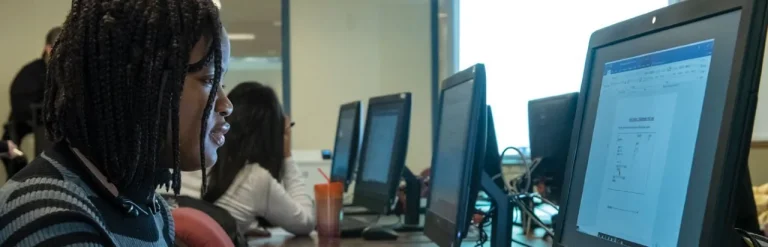
The Top 10 Community Colleges For Computer Science Degrees
For many students, community college provides an affordable path to launch a technology career. With associate’s degrees and transfer programs in computer science, you can gain foundational coding skills and professional development for as little as $3,000 per year at some CC’s. If you’re short on time, here’s a quick answer: Santa Monica College, Mesa…
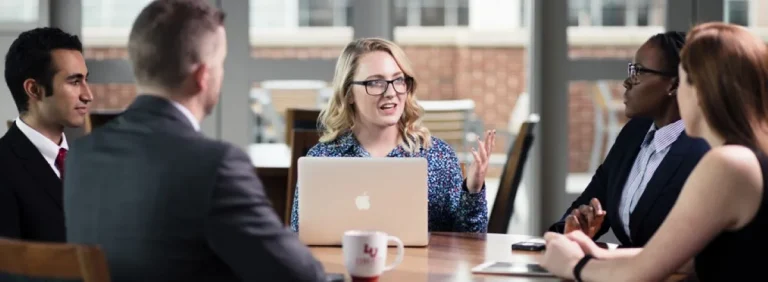
Is A Business Degree Considered A Bachelor Of Science?
When pursuing a business degree, you’ll have to choose between a Bachelor of Science (BS) or a Bachelor of Arts (BA). What’s the difference? If you’re short on time, here’s a quick answer: Business degrees can be either a BS or BA, depending on the program’s focus. BS programs emphasize technical skills like analytics, while…
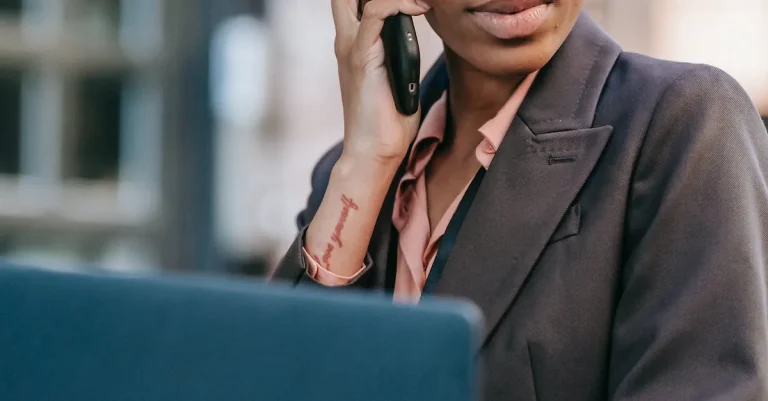
What Is A Call In Computer Science?
In computer programming, a call is an instruction that tells a program to execute a certain function or procedure. Understanding calls is key to grasping how programs operate behind the scenes. If you’re short on time, here’s a quick answer to your question: A call in computer science is an instruction that activates a function…
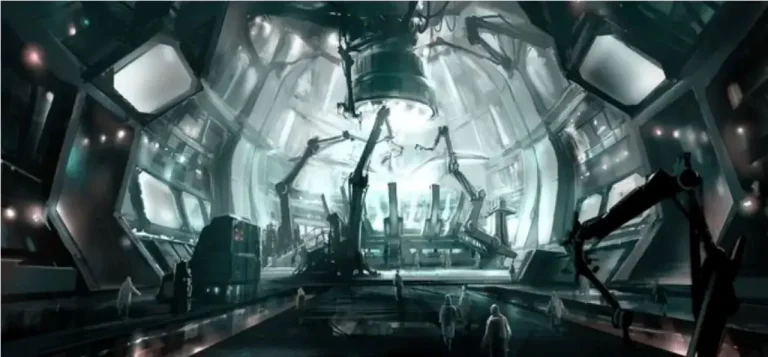
What Is One Main Purpose Of Science Fiction?
Science fiction is a genre that allows us to explore imaginative futures, worlds, and technologies through storytelling. If you’re short on time, here’s a quick answer: One of the main purposes of science fiction is to use speculation and imagination to examine the impact of science and technology on humanity. In this comprehensive guide, we…
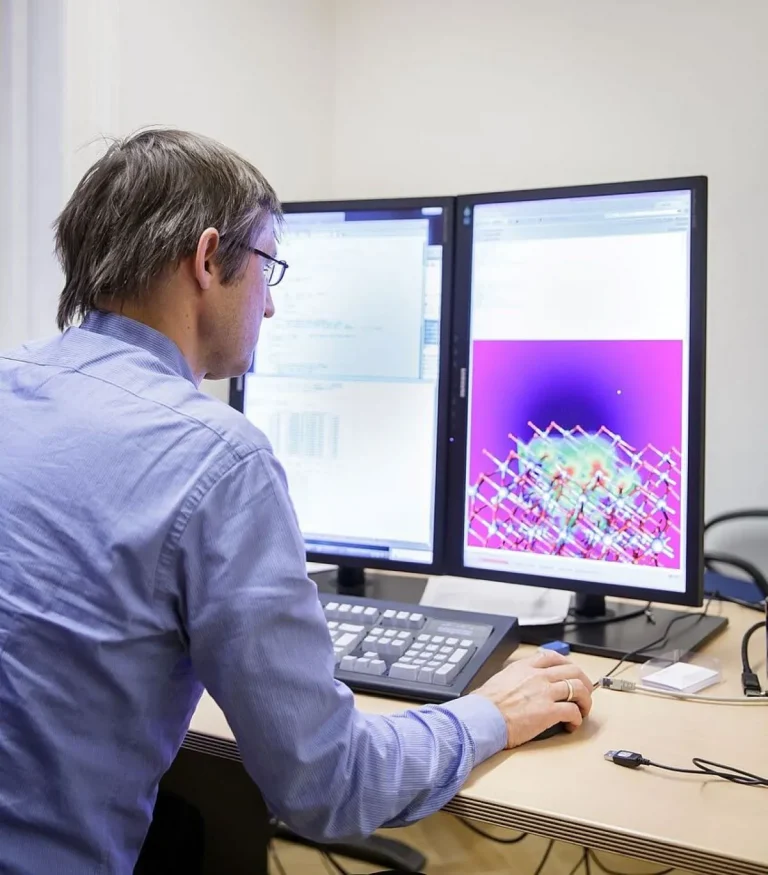
Computational Science Vs Computer Science: Understanding The Key Differences
In today’s digital world, both computational science and computer science are appealing fields of study for students interested in technology and programming. But what exactly is the difference between the two disciplines? If you’re short on time, here’s a quick answer to your question: While computational science focuses on using computers to analyze and solve…

21 Interesting Data Science Capstone Project Ideas [2024]
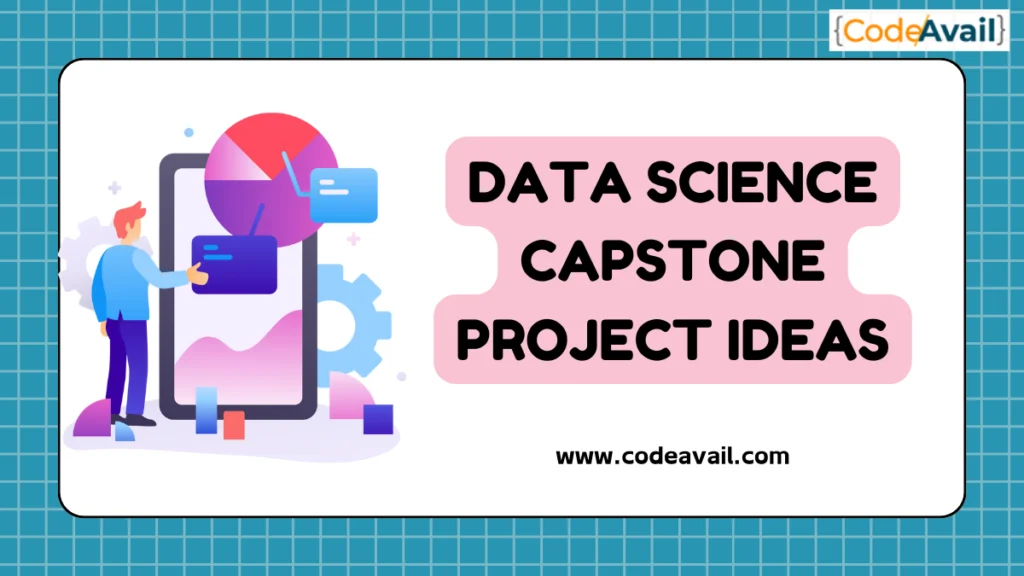
Data science, encompassing the analysis and interpretation of data, stands as a cornerstone of modern innovation.
Capstone projects in data science education play a pivotal role, offering students hands-on experience to apply theoretical concepts in practical settings.
These projects serve as a culmination of their learning journey, providing invaluable opportunities for skill development and problem-solving.
Our blog is dedicated to guiding prospective students through the selection process of data science capstone project ideas. It offers curated ideas and insights to help them embark on a fulfilling educational experience.
Join us as we navigate the dynamic world of data science, empowering students to thrive in this exciting field.
Data Science Capstone Project: A Comprehensive Overview
Table of Contents
Data science capstone projects are an essential component of data science education, providing students with the opportunity to apply their knowledge and skills to real-world problems.
Capstone projects challenge students to acquire and analyze data to solve real-world problems. These projects are designed to test students’ skills in data visualization, probability, inference and modeling, data wrangling, data organization, regression, and machine learning.
In addition, capstone projects are conducted with industry, government, and academic partners, and most projects are sponsored by an organization.
The projects are drawn from real-world problems, and students work in teams consisting of two to four students and a faculty advisor.
However, the goal of the capstone project is to create a usable/public data product that can be used to show students’ skills to potential employers.
Best Data Science Capstone Project Ideas – According to Skill Level
Data science capstone projects are a great way to showcase your skills and apply what you’ve learned in a real-world context. Here are some project ideas categorized by skill level:
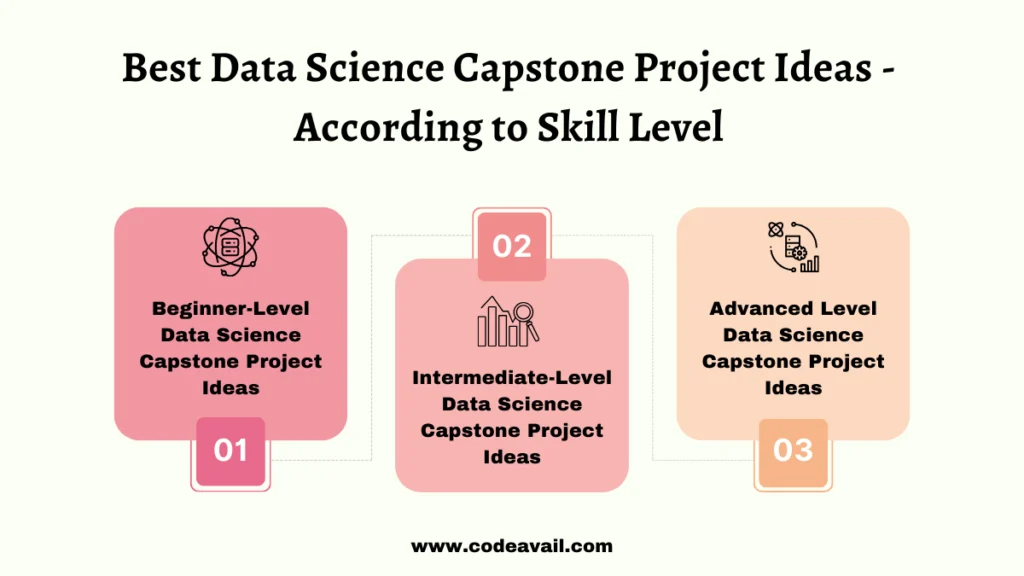
Beginner-Level Data Science Capstone Project Ideas
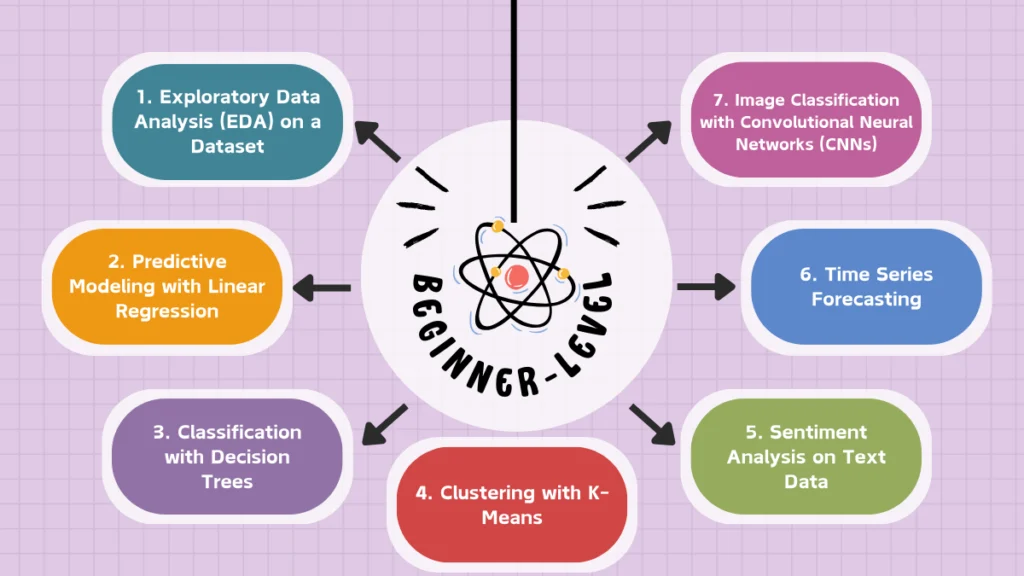
1. Exploratory Data Analysis (EDA) on a Dataset
Start by analyzing a dataset of your choice and exploring its characteristics, trends, and relationships. Practice using basic statistical techniques and visualization tools to gain insights and present your findings clearly and understandably.
2. Predictive Modeling with Linear Regression
Build a simple linear regression model to predict a target variable based on one or more input features. Learn about model evaluation techniques such as mean squared error and R-squared, and interpret the results to make meaningful predictions.
3. Classification with Decision Trees
Use decision tree algorithms to classify data into distinct categories. Learn how to preprocess data, train a decision tree model, and evaluate its performance using metrics like accuracy, precision, and recall. Apply your model to practical scenarios like predicting customer churn or classifying spam emails.
4. Clustering with K-Means
Explore unsupervised learning by applying the K-Means algorithm to group similar data points together. Practice feature scaling and model evaluation to identify meaningful clusters within your dataset. Apply your clustering model to segment customers or analyze patterns in market data.
5. Sentiment Analysis on Text Data
Dive into natural language processing (NLP) by analyzing text data to determine sentiment polarity (positive, negative, or neutral).
Learn about tokenization, text preprocessing, and sentiment analysis techniques using libraries like NLTK or spaCy. Apply your skills to analyze product reviews or social media comments.
6. Time Series Forecasting
Predict future trends or values based on historical time series data. Learn about time series decomposition, trend analysis, and seasonal patterns using methods like ARIMA or exponential smoothing. Apply your forecasting skills to predict stock prices, weather patterns, or sales trends.
7. Image Classification with Convolutional Neural Networks (CNNs)
Explore deep learning concepts by building a basic CNN model to classify images into different categories.
Learn about convolutional layers, pooling, and fully connected layers, and experiment with different architectures to improve model performance. Apply your CNN model to tasks like recognizing handwritten digits or classifying images of animals.
Intermediate-Level Data Science Capstone Project Ideas
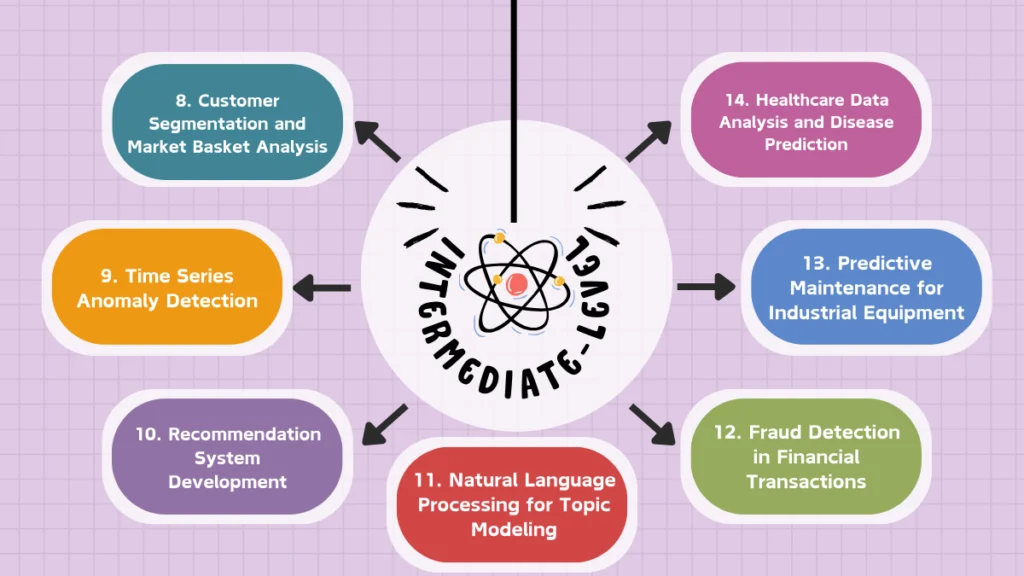
8. Customer Segmentation and Market Basket Analysis
Utilize advanced clustering techniques to segment customers based on their purchasing behavior. Conduct market basket analysis to identify frequent item associations and recommend personalized product suggestions.
Implement techniques like the Apriori algorithm or association rules mining to uncover valuable insights for targeted marketing strategies.
9. Time Series Anomaly Detection
Apply anomaly detection algorithms to identify unusual patterns or outliers in time series data. Utilize techniques such as moving average, Z-score, or autoencoders to detect anomalies in various domains, including finance, IoT sensors, or network traffic.
Develop robust anomaly detection models to enhance data security and predictive maintenance.
10. Recommendation System Development
Build a recommendation engine to suggest personalized items or content to users based on their preferences and behavior. Implement collaborative filtering, content-based filtering, or hybrid recommendation approaches to improve user engagement and satisfaction.
Evaluate the performance of your recommendation system using metrics like precision, recall, and mean average precision.
11. Natural Language Processing for Topic Modeling
Dive deeper into NLP by exploring topic modeling techniques to extract meaningful topics from text data.
Implement algorithms like Latent Dirichlet Allocation (LDA) or Non-Negative Matrix Factorization (NMF) to identify hidden themes or subjects within large text corpora. Apply topic modeling to analyze customer feedback, news articles, or academic papers.
12. Fraud Detection in Financial Transactions
Develop a fraud detection system using machine learning algorithms to identify suspicious activities in financial transactions. Utilize supervised learning techniques such as logistic regression, random forests, or gradient boosting to classify transactions as fraudulent or legitimate.
Employ feature engineering and model evaluation to improve fraud detection accuracy and minimize false positives.
13. Predictive Maintenance for Industrial Equipment
Implement predictive maintenance techniques to anticipate equipment failures and prevent costly downtime.
Analyze sensor data from machinery using machine learning algorithms like support vector machines or recurrent neural networks to predict when maintenance is required. Optimize maintenance schedules to minimize downtime and maximize operational efficiency.
14. Healthcare Data Analysis and Disease Prediction
Utilize healthcare datasets to analyze patient demographics, medical history, and diagnostic tests to predict the likelihood of disease occurrence or progression.
Apply machine learning algorithms such as logistic regression, decision trees, or support vector machines to develop predictive models for diseases like diabetes, cancer, or heart disease. Evaluate model performance using metrics like sensitivity, specificity, and area under the ROC curve.
Advanced Level Data Science Capstone Project Ideas
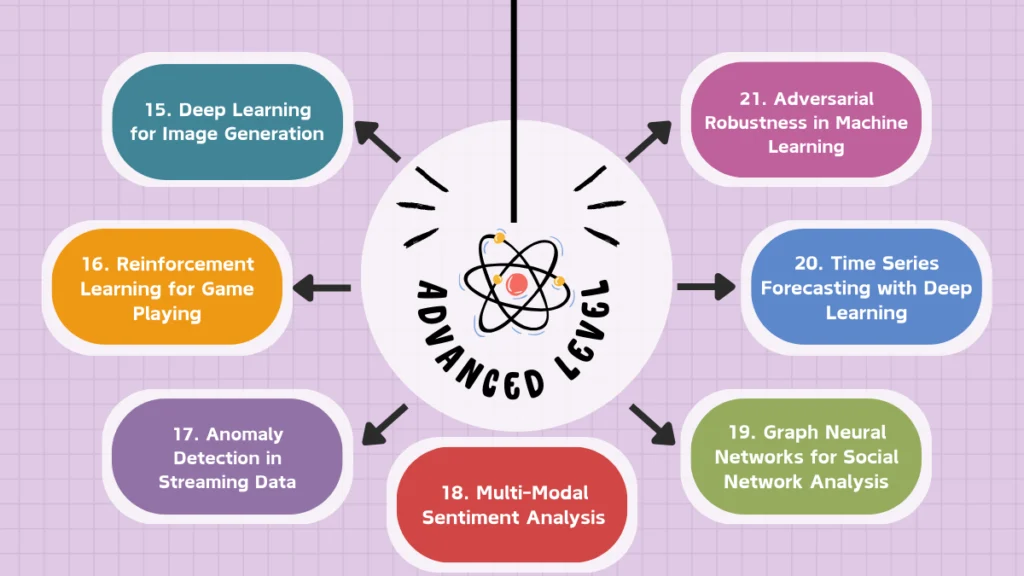
15. Deep Learning for Image Generation
Explore generative adversarial networks (GANs) or variational autoencoders (VAEs) to generate realistic images from scratch. Experiment with architectures like DCGAN or StyleGAN to create high-resolution images of faces, landscapes, or artwork.
Evaluate image quality and diversity using perceptual metrics and human judgment.
16. Reinforcement Learning for Game Playing
Implement reinforcement learning algorithms like deep Q-learning or policy gradients to train agents to play complex games like Atari or board games.
Experiment with exploration-exploitation strategies and reward-shaping techniques to improve agent performance and achieve superhuman levels of gameplay.
17. Anomaly Detection in Streaming Data
Develop real-time anomaly detection systems to identify abnormal behavior in streaming data streams such as network traffic, sensor readings, or financial transactions.
Utilize online learning algorithms like streaming k-means or Isolation Forest to detect anomalies and trigger timely alerts for intervention.
18. Multi-Modal Sentiment Analysis
Extend sentiment analysis to incorporate multiple modalities such as text, images, and audio to capture rich emotional expressions.
However, utilize deep learning architectures like multimodal transformers or fusion models to analyze sentiment across different modalities and improve understanding of complex human emotions.
19. Graph Neural Networks for Social Network Analysis
Apply graph neural networks (GNNs) to model and analyze complex relational data in social networks. Use techniques like graph convolutional networks (GCNs) or graph attention networks (GATs) to learn node embeddings and predict node properties such as community detection or influential users.
20. Time Series Forecasting with Deep Learning
Explore advanced deep learning architectures like long short-term memory (LSTM) networks or transformer-based models for time series forecasting.
Utilize attention mechanisms and multi-horizon forecasting to capture long-term dependencies and improve prediction accuracy in dynamic and volatile environments.
21. Adversarial Robustness in Machine Learning
Investigate techniques to improve the robustness of machine learning models against adversarial attacks.
Explore methods like adversarial training, defensive distillation, or certified robustness to mitigate vulnerabilities and ensure model reliability in adversarial perturbations, particularly in critical applications like autonomous vehicles or healthcare.
These project ideas cater to various skill levels in data science, ranging from beginners to experts. Choose a project that aligns with your interests and skill level, and don’t hesitate to experiment and learn along the way!
Factors to Consider When Choosing a Data Science Capstone Project
Choosing the right data science capstone project is crucial for your learning experience and effectively showcasing your skills. Here are some factors to consider when selecting a data science capstone project:
Personal Interest
Select a project that aligns with your passions and career goals to stay motivated and engaged throughout the process.
Data Availability
Ensure access to relevant and sufficient data to complete the project and draw meaningful insights effectively.
Complexity Level
Consider your current skill level and choose a project that challenges you without overwhelming you, allowing for growth and learning.
Real-World Impact
Aim for projects with practical applications or societal relevance to showcase your ability to solve tangible problems.
Resource Requirements
Evaluate the availability of resources such as time, computing power, and software tools needed to execute the project successfully.
Mentorship and Support
Seek projects with opportunities for guidance and feedback from mentors or peers to enhance your learning experience.
Novelty and Innovation
Explore projects that push boundaries and explore new techniques or approaches to demonstrate creativity and originality in your work.
Tips for Successfully Completing a Data Science Capstone Project
Successfully completing a data science capstone project requires careful planning, effective execution, and strong communication skills. Here are some tips to help you navigate through the process:
- Plan and Prioritize: Break down the project into manageable tasks and create a timeline to stay organized and focused.
- Understand the Problem: Clearly define the project objectives, requirements, and expected outcomes before analyzing.
- Explore and Experiment: Experiment with different methodologies, algorithms, and techniques to find the most suitable approach.
- Document and Iterate: Document your process, results, and insights thoroughly, and iterate on your analyses based on feedback and new findings.
- Collaborate and Seek Feedback: Collaborate with peers, mentors, and stakeholders, actively seeking feedback to improve your work and decision-making.
- Practice Communication: Communicate your findings effectively through clear visualizations, reports, and presentations tailored to your audience’s understanding.
- Reflect and Learn: Reflect on your challenges, successes, and lessons learned throughout the project to inform your future endeavors and continuous improvement.
By following these tips, you can successfully navigate the data science capstone project and demonstrate your skills and expertise in the field.
Wrapping Up
In wrapping up, data science capstone project ideas are invaluable in bridging the gap between theory and practice, offering students a chance to apply their knowledge in real-world scenarios.
They are a cornerstone of data science education, fostering critical thinking, problem-solving, and practical skills development.
As you embark on your journey, don’t hesitate to explore diverse and challenging project ideas. Embrace the opportunity to push boundaries, innovate, and make meaningful contributions to the field.
Share your insights, challenges, and successes with others, and invite fellow enthusiasts to exchange ideas and experiences.
1. What is the purpose of a data science capstone project?
A data science capstone project serves as a culmination of a student’s learning experience, allowing them to apply their knowledge and skills to solve real-world problems in the field of data science. It provides hands-on experience and showcases their ability to analyze data, derive insights, and communicate findings effectively.
2. What are some examples of data science capstone projects?
Data science capstone projects can cover a wide range of topics and domains, including predictive modeling, natural language processing, image classification, recommendation systems, and more. Examples may include analyzing customer behavior, predicting stock prices, sentiment analysis on social media data, or detecting anomalies in financial transactions.
3. How long does it typically take to complete a data science capstone project?
The duration of a data science capstone project can vary depending on factors such as project complexity, available resources, and individual pace. Generally, it may take several weeks to several months to complete a project, including tasks such as data collection, preprocessing, analysis, modeling, and presentation of findings.
Related Posts

Science Fair Project Ideas For 6th Graders
When it comes to Science Fair Project Ideas For 6th Graders, the possibilities are endless! These projects not only help students develop essential skills, such…
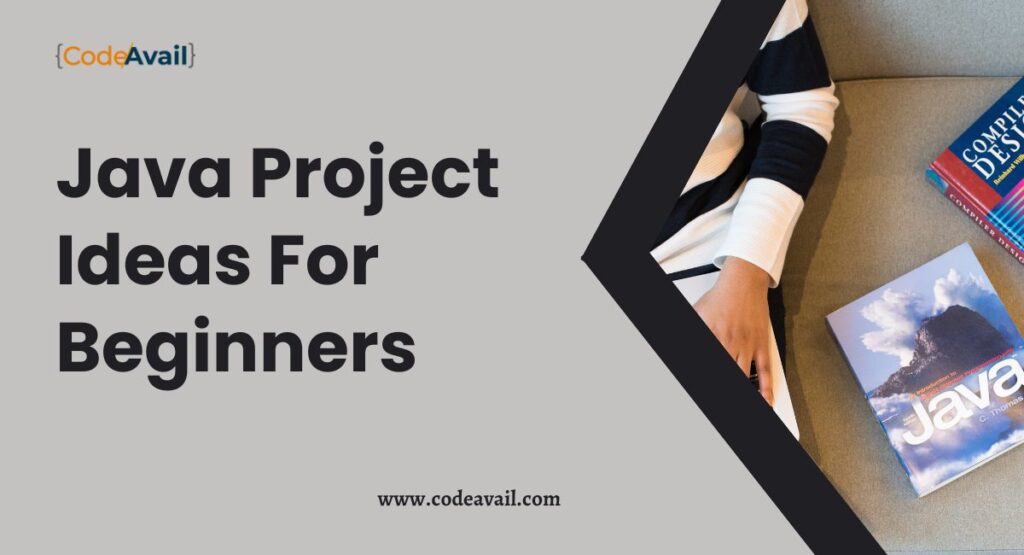
Java Project Ideas for Beginners
Java is one of the most popular programming languages. It is used for many applications, from laptops to data centers, gaming consoles, scientific supercomputers, and…
Capstone Projects
Education is one of the pillars of the data science institute..
Through educational activities, we strive to create a community in Data Science at Columbia. The capstone project is one of the most lauded elements of our MS in Data Science program. As a final step during their study at Columbia, our MS students work on a project sponsored by a DSI industry affiliate or a faculty member over the course of a semester.
Faculty-Sponsored Capstone Projects
A DSI faculty member proposes a research project and advises a team of students working on this project. This is a great way to run a research project with enthusiastic students, eager to try out their newly acquired data science skills in a research setting. This is especially a good opportunity for developing and accelerating interdisciplinary collaboration.
2024-2025 Academic Year: July 15, 2024 via this form
Project Archive
- Spring 2022
- Spring 2020
- Spring 2019
- Spring 2018
- Spring 2016
Data Science: Capstone
To become an expert you need practice and experience..
Show what you’ve learned from the Professional Certificate Program in Data Science.
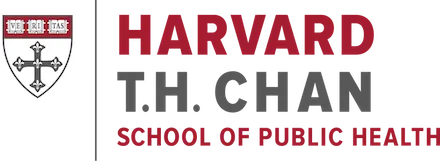
What You'll Learn
To become an expert data scientist you need practice and experience. By completing this capstone project you will get an opportunity to apply the knowledge and skills in R data analysis that you have gained throughout the series. This final project will test your skills in data visualization, probability, inference and modeling, data wrangling, data organization, regression, and machine learning.
Unlike the rest of our Professional Certificate Program in Data Science , in this course, you will receive much less guidance from the instructors. When you complete the project you will have a data product to show off to potential employers or educational programs, a strong indicator of your expertise in the field of data science.
The course will be delivered via edX and connect learners around the world. By the end of the course, participants will understand the following concepts:
- How to apply the knowledge base and skills learned throughout the series to a real-world problem
- How to independently work on a data analysis project
Your Instructors
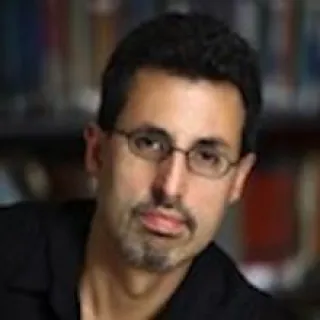
Rafael Irizarry
Professor of Biostatistics at Harvard University Read full bio.
Ways to take this course
When you enroll in this course, you will have the option of pursuing a Verified Certificate or Auditing the Course.
A Verified Certificate costs $149 and provides unlimited access to full course materials, activities, tests, and forums. At the end of the course, learners who earn a passing grade can receive a certificate.
Alternatively, learners can Audit the course for free and have access to select course material, activities, tests, and forums. Please note that this track does not offer a certificate for learners who earn a passing grade.
Introduction to Linear Models and Matrix Algebra
Learn to use R programming to apply linear models to analyze data in life sciences.
High-Dimensional Data Analysis
A focus on several techniques that are widely used in the analysis of high-dimensional data.
Introduction to Bioconductor
Join Harvard faculty in this online course to learn the structure, annotation, normalization, and interpretation of genome scale assays.
Data Science: Capstone
Show what you’ve learned from the Professional Certificate Program in Data Science.
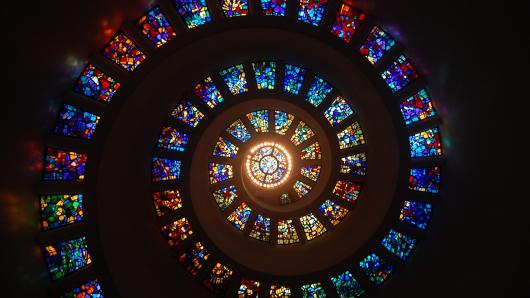
Associated Schools
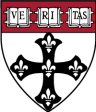
Harvard T.H. Chan School of Public Health
What you'll learn.
How to apply the knowledge base and skills learned throughout the series to a real-world problem
Independently work on a data analysis project
Course description
To become an expert data scientist you need practice and experience. By completing this capstone project you will get an opportunity to apply the knowledge and skills in R data analysis that you have gained throughout the series. This final project will test your skills in data visualization, probability, inference and modeling, data wrangling, data organization, regression, and machine learning.
Unlike the rest of our Professional Certificate Program in Data Science, in this course, you will receive much less guidance from the instructors. When you complete the project you will have a data product to show off to potential employers or educational programs, a strong indicator of your expertise in the field of data science.
Instructors
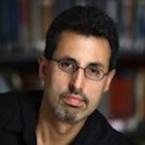
Rafael Irizarry
You may also like.

High-Dimensional Data Analysis
A focus on several techniques that are widely used in the analysis of high-dimensional data.
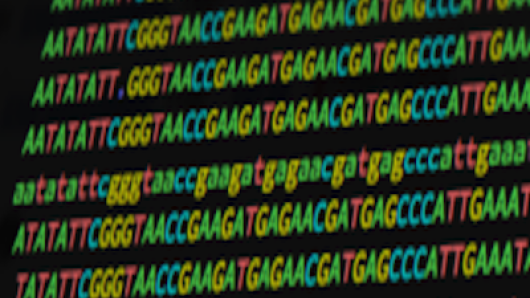
Advanced Bioconductor
Learn advanced approaches to genomic visualization, reproducible analysis, data architecture, and exploration of cloud-scale consortium-generated genomic data.
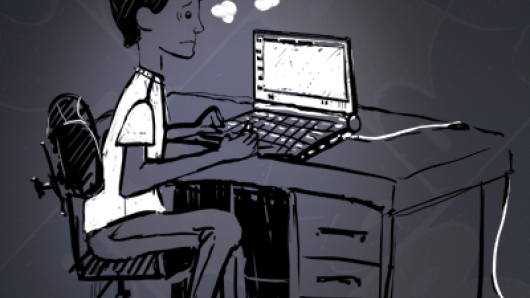
Principles, Statistical and Computational Tools for Reproducible Data Science
Learn skills and tools that support data science and reproducible research, to ensure you can trust your own research results, reproduce them yourself, and communicate them to others.
Join our list to learn more
Capstone Projects
The culminating experience in the Master’s in Applied Data Science program is a Capstone Project where you’ll put your knowledge and skills into practice . You will immerse yourself in a real business problem and will gain valuable, data driven insights using authentic data. Together with project sponsors, you will develop a data science solution to address organization problems, enhance analytics capabilities, and expand talent pools and employment opportunities. Leveraging the university’s rich research portfolio, you also have the option to join a research-focused team .
Selected Capstone Projects
Copd readmission and cost reduction assessment, an nfl ticket pricing study: optimizing revenue using variable and dynamic pricing methods, using image recognition to identify yoga poses, using image recognition to measure the speed of a pitch, real-time credit card fraud detection, interested in becoming a capstone sponsor.
The Master’s in Applied Data Science program accepts projects year-round for placement at the beginning of every quarter, with the Spring quarter being the largest cohort. All projects must be submitted no later than one month prior to the beginning of the preferred starting quarter based on the UChicago academic calendar .
Capstone Sponsor Incentives
Sponsors derive measurable benefits from this unique opportunity to support higher education. Partner organizations propose real-world problems, untested ideas or research queries. Students review them from the perspective of data scientists trained to generate actionable insights that provide long-term value. Through the project, Capstone partners gain access to a symbiotic pool of world-class students, highly accomplished instructors, and cited researchers, resulting in optimized utilization of modern data science-based methods, using your data. Further, for many sponsors, the project becomes a meaningful source of recruitment through the excellent pool of students who work on your project.
Capstone Sponsor Obligations
While there is no monetary cost or contract necessary to sponsor a project, we do consider this a partnership. Teams comprised of four students and guided by an instructor and subject matter expert are provided with expectations from the capstone sponsor and learning objectives, assignments, and evaluation requirements from instructors. In turn, Capstone partners should be prepared to provide the following:
- A detailed problem statement with a description of the data and expected results
- Two or more points of contact
- Access to data relevant to the project by the first week of the applicable quarter
- Engagement through regular meetings (typically bi-weekly) while classes are in session
- If requested, a non-disclosure agreement that may be completed by the student team
Interested in Becoming a Capstone or Industry Research Partner?
Get in touch with us to submit your idea for a collaboration or ask us questions about how the partnership process works.
_.jpg)
Wednesdays @ 12:45pm - 3:00pm SEC LL2.223 (Allston Campus)
Capstone research project course, ac297r, fall 2022 weiwei pan, founded by the institute for applied computational science (iacs)'s scientific program director, pavlos protopapas , the capstone research course is a group-based research experience where students work directly with a partner from industry, government, academia, or an ngo to solve a real-world data science/ computation problem. students will create a solution in the form of a software package, which will require varying levels of research. upon completion of this challenging project, students will be better equipped to conduct research and enter the professional world. every class session includes a guest lecture concerning various essential skills for one's career -- from public speaking, reading and writing research papers, how to work remotely on a team, everything about start-ups, and more..

Snake Classification using Neural-Backed Decision Trees
- Group members: Rui Zheng, Weihua Zhao, Nikolas Racelis-Russell
Abstract: Many advanced algorithms, specifically deep learning models, are considered “black box” to human understanding. Transparency to intrprete such models has become a key obstacle which prevents such algorithms from being put into practical use. Although algorithms, such as GradCam, are invented to provide visual explanations from deep networks via gradient-based localization, they do not provide details of how the models reached their final decision step by step in detail. The goal of this project is to provide more interpretability to Convolutional Neural Networks (CNN) models by combining Grad-CAM with Neural Backed Decision Trees (NBDTs), and provide visual explanations with detailed decision making process of CNN models. This project demonstrates the potential and limitations of jointly applying Grad-CAM and NBDTs on snake classification.
Autonomous Vehicles
Autoware and lgsvl.
- Group members: Andres Bernal, Amir Uqdah, Jie Wang
Abstract: We were able to replicate the ThunderHill race track using the Unity 3D game engine and integrated Unity with the track and robot into the LGSVL simulator. Once the integration was complete we were able to see our robot with the Thunderhill Track as our map in the simulator. We were then able to virtualize the functions of the IMU, odometry and lidar sensors and RGB-D cameras to better visualize what our robot perceives in the simulation. Finally we were able to fully visualize what our robot sees with the virtual sensors using Autoware Rviz which displays the location and point cloud map of the vehicle and its surroundings.
Computer Vision and Lane Segmentation in Autonomous Vehicles
- Group members: Evan Kim, Joseph Fallon, Ka Chan
Abstract: Perception is absolutely vital to navigation. Without perception, any corporeal entity cannot localize itself in its environment and move around obstacles. To create an autonomous vehicle (AV) capable of racing on the Thunderhill Raceway track in Berkeley, California, the team must supplement a stereo camera capable of supporting image perception and computer vision. The team was given two cameras, the Intel RealSense D455 and the ZED camera. In this analysis, the team will compare the capabilities of the two cameras and responsibly select a camera capable of supporting the object detection, and will develop a lane segmentation algorithm that will help extract lanes from the camera feed.
Autonomous Mapping, Localization and Navigation using Computer Vision as well as Tuning of Camera
- Group members: Youngseo Do, Jay Chong, Siddharth Saha
Abstract: One of the main tools used in autonomous mapping and navigation is a 3D Lidar. A 3D Lidar provides various advantages. It is not sensitive to light conditions, it can detect color through reflective channels, it has a complete 360 degree view of the environment and does not require any ”learning” to detect obstacles. One can use the reflective channel to detect the color of lanes as well as avoid obstacles. The pointcloud information from the Lidar can also easily enable mapping and localization as the vehicle will know where it is at all points. It is easy to see why so many large scale autonomous vehicle units invest in expensive and bulky Lidars. However, this is not accessible to all due to it’s price. A camera (even depth) is much more affordable. However it comes with it’s own slew of disadvantages. It can see color but programming for the color is hard due to varying light conditions. Unless you use multiple cameras you often can’t see all around you. These factors together are a hindrance to autonomous navigation. We thus aim to demonstrate 3 goals: 1) Mapping and Localization with a single camera and other sensory information using RTABMAP SLAM algorithm 2) Obstacle avoidance and lane following with a single camera using Facebook AI Detectron2 Deep Learning and ROS 3) Tuning of the camera to be less sensitive to varying light conditions using ROS rqt_reconfigure
Data Visualizations and Interface For Autonomous Robots
- Group members: Jia Shi, Seokmin Hong, Yuxi Luo
Abstract: Autonomous navigation requires a wide-range of engineering expertise and a well-developed technological architecture in order to operate. The focus of this project and report is to illustrate the significance of data visualizations and an interactive interface with regards to autonomous navigation in a racing environment. In order to yield the best results in an autonomous navigation race, the users must be able to understand the behavior of the vehicle when training navigation models and during the live race. In order to address these concerns, teams working on autonomous navigation must be able to visualize and interact with the robot. In this project, different algorithms such as A* search and RRT* (Rapidly-exploring random tree) are implemented to create path planning and obstacle avoidance. Visualizations of these respective algorithms and a user interface to send/receive commands will help to enhance model testing, debug unexpected behavior, and improve upon existing autonomous navigation models. Simulations with the most optimal navigation algorithm will also be run to demonstrate the functionality of the interactive interface. Results, implications of the interface, and further improvements will also be discussed.
GPS Based Autonomous Navigation on the 1/5th Scale
- Group members: Shiyin Liang, Garrett Gibo, Neghena Faizyar
Abstract: Self-driving vehicles are revolutionizing the automotive industry with companies like Tesla, Toyota, Audi and many more pouring a substantial amount of money into research and development. While many of these self-driving systems use a combination of cameras, lidars, and radars for local perception and navigation, the fundamental global localization system that they use relies upon a GPS. The challenge in building a navigation system around a GPS derives from the inherent issues of the sensor itself. In general, GPS’s tend to suffer from issues of signal interference that lead to infrequent positional updates and lower precision. On the 1/5th car scale, positional inaccuracies are magnified, so it is crucial that we know the location of our vehicle with speed and precision. In this project, we compare the performance of different GPS’s in order to determine what level of performance is best suited at the 1/5th scale. Using the best-suited GPS, we design a navigation system that can mitigate the shortcomings of the GPS and provide both a reliable autonomous vehicle.
Autonomous: Odometry and IMU
- Group members: Pranav Deshmane, Sally Poon
Abstract: For a vehicle to successfully navigate istelf and even race autonomously, it is essential for the vehicle to be able localize itself within its environment. This is where Odometry and IMU data can greatly support the robot’s navigational ability. Wheel Odometry provides useful measurements to estimate the position of the car through the use of wheel’s circumference and rotations per second. IMU, which stands for Interial Measurement Unit, is 9 axis sensor that can sense linear acceleration, angular velocity, and magnetic fields. Together, these data sources can provide us crucial information in deriving a Position Estimate (how far our robot has traveled) and a Compass Heading (orientation of the robot/where it’s headed). While most navigation stacks rely on GPS or Computer Vision to achieve successful navigation, this leaves the robot vulnerable to unfavorable scenarios. For example, GPS is prone to lag and may be infeasible in unfamiliar terrain. Computer Vision approaches often depend heavily on training data and cannot always provide continouos and accurate orientation. Odometry and IMU readings are thus invaluable sources of sensing information that can easily complement and enhance navigational stacks in place to build more robust and accurate autonomous navigation models.
Malware and Graph Learning
Malware detection.
- Group members: Yu-Chieh Chen, Ruoyu Liu, Yikai Hao
Abstract: As the technology grows fast in recent years, more and more people cannot live without cell phones. It is important to protect users’ data for cell phone companies and operating system providers. Therefore, detecting malwares based on the code they have can avoid publishing of malwares and prohibiting them from the source. This report aims at finding a model which can detect malwares accurately and with a small computational cost. It uses different matrices and graphs to search the relationships between applications and detecting malwares based on the similarity. As a result, the best model can achieve a test accuracy around 99%.
Potential Improvement of MAMADROID System
- Group members: Zihan Qin, Jian Jiao
Abstract: Nowadays, smartphone is an indispensable part of people's daily life. Android System is the most popular system running on smartphone. Due to this popularity, malware detection on Android becomes on of the most significant task for research community. In this project, we are mainly focusing on one called MAMADROID System. Instead of previous work which highly relied on the permissions requested by apps, MAMADROID relied on the sequences of abstracted API calls performed by apps. We are very interested in finding ways to improve this model. To achieve this, we've been trying to find some new features to fit into the model. We made three basic model and take the one with the highest accuracy and made two more advanced model based on this model with the best performance.
Exploring the Language of Malware
- Group members: Neel Shah, Mandy Ma
Abstract: The Android app store and its open-source features make it extremely vulnerable to malicious software, known as Malware. The current state of the art encompasses the use of advanced code analysis and corresponding machine learning models. Although along with our initial research we found that the Applications in the Android app store along with their corresponding API calls behave a lot like a language. They have their comparable own syntax, structure, and grammar. This inspired us to use techniques from Natural Language Processing(NLP) and use the same idea of creating graphical relationships between applications and APIs. Additionally, we also show that the use of these graphical embeddings maintains the integrity of classification metrics to even correctly identify and differentiate Malware and Benign applications.
CoCoDroid: Detecting Malware By Building Common Graph Using Control Flow Graph
- Group members: Edwin Huang, Sabrina Ho
Abstract: In today's world, malware has grown so much. In 2020, there are more than 129 millions of Android users around the world. With Android applications dominating the devices, we hope to produce a detection tool that is accessible to the general public. We present a structure that analyze apps in the form of control flow graph. With that, we build a common graph to capture how close the apps are to each other and classify whether they are malicious or not. We compare our work with other methods and show that using control flow graph is a good choice as a representation of Android applications (APKs) and can outperform other models. We built features using Metapath2Vec and Doc2Vec, and trained Random Forest, 1-Nearest Neighbors, and 3-Nearest Neighbors Models.
Attacking the HinDroid Malware Detector
- Group members: Ruben Gonzalez, Amar Joea
Abstract: Over the past decade, malware has established itself as a constant issue for the Android operating system. In 2018, Symantec reported that they blocked more than 10 thousand malicious Android apps per day, while nearly 3 quarters of Android devices remained on older versions of Android. With billions of active Android devices, millions of users are only a swipe away from becoming victims. Naturally, automated machine learning-based detection systems have become commonplace solutions as they can drastically speed up the labeling process. However, it has been shown that many of these models are vulnerable to adversarial attacks, notably attacks that add redundant code to malware to consfuse detectors. First, we introduce a new model that extends the Hindroid detection system by employing node embeddings using metapath2vec. We believe that the introduction of node embeddings will improve the performance of the model beyond the capabilities of HinDroid. Second, we intend to break these two models using a method similar to that proposed in the Android HIV paper. That is we train an adversarial model that perturbs malware such that a detector mislabels it as a benign app. We then measure the performance of each model after recursively feeding adversarial examples back into them. We believe that by doing so, our model will be able to outperform the Hindroid implementation in its ability to label malware even after adversarial examples have been added.
Text Mining and NLP
Autophrase application web.
- Group members: Tiange Wan, Yicen Ma, Anant Gandhi
Abstract: We propose the creation of a full-stack website as an extension of the AutoPhrase algorithm and text analysis to help the non-tech users understand their text efficiently. Also, we provide a notebook with one specific dataset with text analysis to the users.
Analyzing Movies Using Phrase Mining
- Group members: Daniel Lee, Yuxuan Fan, Huilai Miao
Abstract: Movies are a rich source of human culture from which we can derive insight. Previous work addresses either a textual analysis of movie plots or the use of phrase mining for natural language processing, but not both. Here, we propose a novel analysis of movies by extracting key phrases from movie plot summaries using AutoPhrase, a phrase mining framework. Using these phrases, we analyze movies through 1) an exploratory data analysis that examines the progression of human culture over time, 2) the development and interpretation of a classification model that predicts movie genre, and 3) the development and interpretation of a clustering model that clusters movies. We see that this application of phrase mining to movie plots provides a unique and valuable insight into human culture while remaining accessible to a general audience, e.g., history and anthropology non-experts.
AutoPhrase for Financial Documents Interpretation
- Group members: Joey Hou, Shaoqing Yi, Zachary Ling
Abstract: The stock market is one of the most popular markets that the investors like to put their money in. There are millions of investors who participate in the stock market investment directly or indirectly, such as by mutual fund, defined-benefit plan. The performance of the stock price is highly related to the latest news information, such as the 8-K reports, the annual or quarter report. These reports reflect the operating performance of the companies, which are the important fundaments for the stock price. However, there are numerous news to the market for each day, and we want to build a model to extract the features from the news and use them to predict the price trend. In this project, we apply the AutoPhrase model from Professor Jingbo Shang to extract the high-quality phrases from the news documents and to predict stock price trends. We aim to explore if certain words or phrases correlate to higher or lower stock prices after a release of an 8-K report.
Text Classification with Named-Entity Recognition and AutoPhrase
- Group members: Siyu Deng, Rachel Ung, Yang Li
Abstract: Text Classification (TC) and Named-Entity Recognition (NER) are two fundamental tasks for many Natural Language Processing (NLP) applications, which involve understanding, extracting information, and categorizing the text. In order to achieve these goals, we utilized AutoPhrase and a pre-trained language NER model to extract quality phrases. Using these as part of our features, we are able to achieve very high performance for a five-class and a twenty-class text classification dataset. Our project will follow a similar setting as previous works with train, validation, and test datasets and comparing the results across different methods.
AutoLibrary - A Personal Digital Library to Find Related Works via Text Analyzer
- Group members: Bingqi Zhou, Jiayi Fan, Yichun Ren
Abstract: When encountering scientific papers, it is challenging for readers themselves to find other related works. First of all, it is hard to identify keywords that summarize the papers to search for similar papers. This dilemma is most common if readers are not familiar with the domains of papers that they are reading. Meanwhile, traditional recommendation models based on user profile and collection data are not applicable for recommending similar works. Some existing digital libraries’ recommender systems utilize phrase mining methods such as taxonomy construction and topic modeling, but such methods also fail to catch the specific topics of the paper. AutoLibrary is designed to address these difficulties, where users can input a scientific paper and get the most related papers. AutoLibrary solves the dilemma via a text analyzer method called AutoPhrase. AutoPhrase is a domain-independent phrase mining method developed by Jingbo Shang et al. (2018) that can automatically extract quality phrases from the input paper. After users upload the paper and select the fields of study of the paper, AutoLibrary utilizes AutoPhrase and our pre-trained domain datasets to return high-quality domain-specific keywords that could represent the paper. While AutoLibrary uses the top three keywords to search on Semantic Scholar for similar works at first, users could also customize the selection of the high-quality phrases or enter their own keywords to explore other related works. Based on the experiments and result analysis, AutoLibrary outperforms other similar text analyzer applications efficiently and effectively across different scientific fields. AutoLibrary is beneficial as it eases the pain point of manually extracting accurate, specific keywords from papers and provides a personalized user experience for finding related papers of various domains and subdomains.
Restaurant Recommender System
- Group members: Shenghan Liu, Catherine Hou, Vincent Le
Abstract: Over time, we rely more and more heavily on online platforms such as Netflix, Amazon, Spotify, which are embedded with the recommendation system in the applications. They know users’ preferences by collecting their ratings, recording the clicks, combing the reviews and then recommending more items. In building the recommender system, review texts can hold the same importance as the numerical statistics because they contain key phrases that characterize how they felt about the review. For this project, we propose to build the recommender system with primary focus on the text reviews analysis through TF-IDF (term frequency-inverse document frequency) and AutoPhrase and to add targeted segmented analysis on phrases to attach sentiments to aspects of a restaurant to rank those recommendations. The ultimate goal is designing a website for deploying our recommender system and showing its functionality.
Recommender Systems
Forumrec - a question recommender for the super user community.
- Group members: Yo Jeremijenko-Conley, Jack Lin, Jasraj Johl
Abstract: The Super User forum exists on the internet as a medium for users to exchange information. In particular, the information shared here primarily related to questions pertaining to operating systems. The system we developed, ForumRec, aims to increase usability for the forum’s participants by specifically recommending questions that may be more suitable for a user in particular to answer. The model we built uses a combination of technique content-based and collaborative filtering from the LightFM package to identify how well a novel question would fit for the desired user. In comparison to baseline models of how Super User already recommends questions, the model attains better performance for more recent data, scoring 0.0014, 0.0033, and 0.5160 on precision at 100, recall at 100, and AUC, which is markedly better than the baselines.
OnSight: Outdoor Rock Climbing Recommendations
- Group members: Brent Min, Brian Cheng, Eric Liu
Abstract: Recommendations for outdoor rock climbing has historically been limited to word of mouth, guide books, and most popular climbs. We aim to change that with our project OnSight, offering personalized recommendations for outdoor rock climbers.
Bridging the Gap: Solving Music Disputes with Recommendation Systems
- Group members: Nayoung Park, Sarat Sreepathy, Duncan Carlmark
Abstract: Many have probably found themselves in an uncomfortable conversation in which a parent is questioning why the song playing over a bedroom speaker is so loud, repetitive, or profane. If someone has never had such a conversation, at the very least they have probably made a conscious decision to refrain from playing a certain genre or artist when their parents are around. Knowing what music to play in these situations does not have to be an elaborate, stressful process. In fact, finding appropriate songs can be made quite simple with the help of recommendation systems. Our solution to this issue actually consists of two recommendation systems that function in similar ways. The first takes music that parents enjoy and recommends it to their children. The second takes music that children enjoy and recommends it to their parents. Both these recommendation systems create their own individual Spotify playlists that try to “bridge the gap” between the music tastes of parents and their children. Through user testing and user interviews we found that our recommenders had mixed success in creating playlists that could be listened to by children and their parents. The success of our recommendations seemed to be largely correlated with the degree of inherent similarity between the music tastes of children and their parents. So while our solution is not perfect, in situations where overlap between parents and children exist, our recommender can successfully “bridge the gap”.
Asnapp - Workout Video Recommender
- Group members: Peter Peng, Najeem Kanishka, Amanda Shu
Abstract: For those who work out at home, finding a good workout routine is difficult. Many of the workout options you may find online are non-personalized and do not take into account your time and equipment constraints, as well as your workout preferences. Asnapp is a web application that provides personalized recommendations of workout videos by Fitness Blender, a company that provides free online workout videos. Our website displays several lists of recommendations (similar to Netflix’s user interface), such as “top upper body workouts for you”. Users can login into our website, choose between several models to generate their recommendations, browse through personalized recommendations lists, and choose a workout to do, saving them the time and effort needed to build a good workout routine.
- Group members: Zachary Nguyen, Alex Pham, Anthony Fong
Abstract: Existing options for recipe recommendations are less than satisfactory. We sought to solve this problem by creating our own recommendation system hosted on a website. We used Food.com recipe data to create a classifier to identify cuisines of recipes, a popularity based recommender, and a content-based filtering recommender using cosine similarity. In the future, we would like to improve upon this recommender by exploring alternative ways to model ingredients, try to start tracking implicit/explicit data of a user, and try to create a hybrid recommender using collaborative techniques.
Makeup Recommender
- Group members: Justin Lee, Shayal Singh, Alexandria Kim
Abstract: Although product recommenders are conventional in the world of machine learning based recommender systems, cosmetics are an overlooked field. By providing a complete set of cosmetic recommendations, we can reduce the time and effort required for users to find the best products for a user’s personalized needs. Our goal is to create a recommender that will provide a one-stop shop experience where a user will get recommended an array of products to create an entire makeup look based on similar products that the user enjoys, products that similar users have purchased, as well as products that are personalized to the user including skin type, skin tone, ingredient preferences, and budget. The website recommends a complete makeup set personalized to the user. The user inputs their skin type, skin tone, budget, and any ingredient preferences so that we can suggest the best products for their personalized needs. The user also inputs a product of their choice from one of the four categories to aid with further personalization. Using this preference and knowledge about the user, we will suggest a complete set of products to complete a look. Our recommender provides four categories of products: face, cheeks, eyes, and lips. Our project aims to utilize collaborative filtering recommendations to ensure user satisfaction and success when creating their desired look.
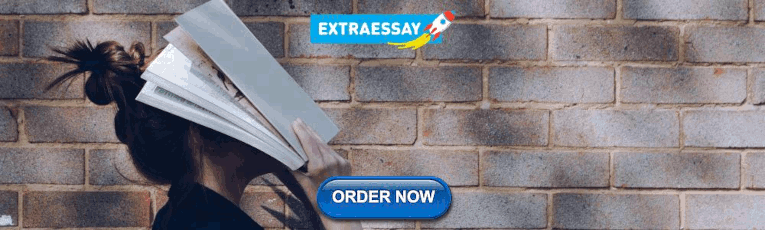
Opioid Overdose Prevalence Analysis
- Group members: Gunther Schwartz, Flory Huang, Hanbyul Ryu
Abstract: Substance abuse is not only a significant health hazard of epidemic proportions, it is also a large marketplace where addictive substances, abuse and co-abuse patterns, supply and demand patterns, Governmental regulations enforcement -- all play a role. The interplay of these factors change significantly when exceptional events like the COVID-19 pandemic strikes. The proposed Capstone research will develop a knowledge graph based approach to compare pre-pandemic and in-pandemic dynamics. It will combine Information Integration, Natural Language Processing and Machine Learning techniques to automatically construct a Knowledge Graph by fusing information from Governmental, News and Social Media data.
Large-scale Multiple Testing
Multiple testing method with empirical null distribution in leukemia studies.
- Group members: Raymond Wang
Abstract: In genomics we are often faced with the task to identify genes correlated with a specific disease among a large number of candidate gene pools. A naive approach is to apply a hypothesis test to every individual gene. This method ignores confounding factors in the data and does not adjust for the additional variance. In this paper we will introduce a much more robust method primarily using estimations of the empirical null distribution and the false discovery rate (FDR). A leukemia dataset is used to demonstrate that the empirical null distribution, one estimated from observing the data first, provides a better fit of the theoretical null distribution. Furthermore, we will compare and contrast the result with unsupervised classification methods such as k-Means and the Gaussian Mixture Model.
Large-scale Multiple Testing with Empirical Null Distribution in Predicting Cardiovascular Disease
- Group members: Zimin Dai, Leyang Zhang, Wentao Chen
Abstract: According to the World Health Organization, cardiovascular disease, such as ischemic heart disease and stroke, is the leading cause of deaths globally. We use features and some health conditions of a person to determine the signals and the probability of whether he/she has cardiovascular disease. To achieve such a goal, we implemented logistic regression and large-scale multiple testing methods on a dataset with ample information. Empirical Null is applied to find outliers and filter the dataset, we successfully removed about 10000 outliers out of the 70000 observations by applying the FDR and FPR method to find the upper and lower bound. Finally, our product was a Logistic Regression model that predicts whether a person has Cardiovasculardisease with an accuracy score of .7220 on the test set.
Spatial-temporal Analyses of Infectious Disease Dynamics
Spatial-temporal prediction of covid-19 case counts through epidemiology model.
- Group members: Shuyuan Wang
Abstract: Epidemiology Model alone is not sufficient to account for the complexity of COVID-19 and thus when implemented alone, often gives very inaccurate predictions. One reason is that the model itself does not take into consideration of the spatial aspect of the region. It assumes the region to be isolated from any other regions. In reality, there is a lot of traffic going back and forth among countries at the boundaries. In this project, my team adjusts the Epidemiology Model to account for the spatial aspect of the disease in order to predict case counts of Californian counties. We included the adjacency, the distance between counties, and the mobility. After fitting the original Epidemiology Model through Gradient Approach with learning rates adjusted by Hessain Matrix to found the infection duration and infection rate for each county, we implemented geographical information, including adjacency and distance among the Californian counties. The spatial model also takes into account the mobility score of each county, that is, how fast people are moving around. To test out the model, we performed predictions on 3/2/2021 based on the previous day's (3/1/2021) case counts with dt as 5. The infection duration and infection rate are based on previous 40 days. Most of the counties yield less than 1% error. However, 5 counties have inaccurate predictions because of the missing data on its neighbors due to being on the edge of California, or low population. The model currently has not been tested out on the entire United States due to a lack of computing speed and missing data which will result in many counties without any neighbors. In the future, the model can be extended to predict 3 days in advance or more days to generate more value. Furthermore, the infection duration and infection rate are derived still based on the original model. In the future, we wish to use Gradient Descent to acquire infection duration and infection rate dynamically from the new model.
Graph Data Analysis
A graph ml analysis of senatorial twitter accounts.
- Group members: Yueting Wu, Yimei Zhao, Anurag Pamuru
Abstract: This project’s main inquiry is into whether there is a tangible difference in the way that Democrat members of Congress speak and interact on social media in comparison to Republican members of Congress. If there are such differences, this project will leverage them to train a suitable ML model on this data for node classification. That is to say, this project aims to determine a Senator’s political affiliation based off of a) their Twitter relationships to other Senators b) their speech patterns, and c) other mine-able features on Twitter. In order to truly utilize the complex implicit relationships hidden in the Twitter graph, we can use models such as Graph Convolutional Networks, which apply the concept of “convolutions” from CNNs to a graph network-oriented framework. These GCNs learn feature representations for each node in the Twitter graph and utilize those representations to fuel the aforementioned node classification task. However useful the GCN may be, there is no shortage of other graph ML techniques that could lend themselves to the prediction task at hand. Of particular interest are inductive graph ML techniques; inductive Graph Networks are a new assortment of Graph Networks that no longer need to be trained on a whole graph to get feature representations for all nodes in the dataset (transductive). Instead, inductive techniques like GraphSage peer into the structural composition of all the nodes in a graph by building neighborhood embeddings for each node. By using a medley of networks on this dataset, we gain deeper insight into what kind of graph we are working with. In other words, if more complex techniques like GraphSage outrank vanilla GCNs, it would point to an equally complex structural composition within the graph that only an inductive technique like GraphSage would be able to pinpoint. However, it is harder to train any network without features. In the case of our analysis, these features will be some text embedding of a politician's tweets. Solutions like word2vec or even a sentiment analysis metric that aggregate across the hundreds of thousands of tweets posted by the 116th Congress could prove quite useful as features for the training of the aforementioned models.
GCN on 3d Points
- Group members: Shang Li, Xinrui Zhan
Abstract: This research focuses on 3D shape classification. Our goal is to predict the category of shapes consisting of 3D data points. We aim to implement Graph Neural Network models and compare the performances with the PointNet, a popular architecture for 3d points cloud classification tasks. Not only will we compare standard metrics such as accuracy and confusion matrix, we will also explore the model's resilience on data transformation. What’s more, we tried combining PointNet with graph pooling layers. Our experiment shows that even though PointNet has a higher accuracy overall, GCN has much more reasonable misclassification and is much more robust to data augmentation.
Graph-Based Product Recommendation
- Group members: Abdullatif Jarkas, Nathan Tsai
Abstract: Recommender systems are important, revenue-generating technologies in many of the services today, providing recommendations for social, product, and other networks. However, the majority of existing recommender system methods use metrics of similarity to recommend other nodes through content-based and collaborative filtering approaches, which do not take into account the graph structure of the relationships between the nodes. A graph-based recommender system then is able to utilize graph relationships to improve node embeddings for recommendation in a way that conventional recommender systems cannot. Inspired by PinSage, we explore an unsupervised graph-based recommendation method that can take advantage of the relationships between nodes, in addition to the text and image features, and generate more accurate and robust embeddings for Amazon product recommendation.
NBA Game Prediction
- Group members: Austin Le, Mengyuan Shi
Abstract: When working with an NBA dataset, we wanted to figure out the best way to represent a network like structure amongst the teams and figured that the amount of time that each player spends on the court with one another would prove useful. By extracting this network and projecting player statistics upon each node, we will utilize GraphSage, a framework that will embed node features onto each player and aggregate each team to predict whether or not they can make the playoffs.
NBA Seeds with Graph Neural Networks
- Group members: Steven Liu, Aurelio Barrios
Abstract: The NBA contains many challenges when attempting to make predictions. The performance of a team in the NBA is difficult because many things can happen over the course of 81 games. Our analysis attempts to produce accurate results by exploiting the natural structure of the NBA league and data of previous player stats. Our analysis begins with identifying the players on each roster to create an aggregated stat for each team, then we will take advantage of the schedules of each team to learn the unique performance of a team against every other team. Leveraging the features and the schedule of the teams, we expect to be able to make decent predictions of NBA seedings before a season starts.
Stock Market Sentiment Predictor
- Group members: Jason Chau, Sung-Lin Chang, Dylan Loe
Abstract: In this project, we aim to produce a tool that will be able to predict the stock movement of a company. The output will be a binary output where it will indicated whether we are bullish or bearish on a stock. In our pursuit of making this tool, we will incorporate graph convolutional networks to take advantage of the interconnected features of stocks.
The Spread of Misinformation
Political popularity of misinformation.
- Group members: Catherine Tao, Aaron Chan, Matthew Sao
Abstract: For our research on Political Popularity of Misinformation, we want to research the influence politicians have on Twitter, a well known social media platform for users to voice their opinions to a wider audience. The information shared on Twitter that we are interested in will be grouped into scientific information or misinformation. Politicians can easily sway public opinion with a simple tweet, therefore we wanted to analyze how much they influence other Twitter users. We gathered ten politicians who we considered to spread scientific information on Twitter and ten politicians who we considered to spread misinformation on Twitter. We analyze the two groups to show how controversial a tweet appears. We do this by looking at tweet engagement as well as a popularity metrics to see growth over time. The results of our investigation showed that politicians who spread misinformation have a higher ratio value on average and have less overall likes over their tweets. Our permutation tests shows that our scientific group has been consistently growing and increasing in growth over time. In contrast, our misinformation group has grown significantly, but only in the more recent years. Overall, our results show that a politician can experience the most growth through spreading non-controversial, scientific information.
The Sentiment of U.S. Presidential Elections on Twitter
- Group members: Zahra Masood, Sravya Voleti, Hannah Peterson
Abstract: Political tensions in the United States came to a head in 2020 as the public responded to various major events such as the onset of the COVID-19 pandemic and the murder of George Floyd, as well as the 2020 presidential election. Here we investigate if there is evidence of increasing polarization and negativity in regards to politics among the American public on social media by analyzing Twitter data related to the 2016 and 2020 presidential elections. Using publicly available datasets of tweets for each election, we perform sentiment analysis on the text of tweets to quantify their degrees of negativity and subjectivity. We also identify political leanings of tweets by analyzing their hashtag usage and identify “dialogue” occurring between and amongst left- and right-leaning users by analyzing the tweets’ user mentions. We then conduct permutation testing on these various groupings of tweets between the two years to determine if there is statistical evidence of increased polarization and negativity on social media surrounding the U.S. presidential election from 2016 to 2020, both generally and between and within political parties. We find that election-related tweets in 2020 generally used less neutral language than in 2016 but were not conclusively more positive or negative in sentiment.
Community Effects From Misinformation Flags on Twitter
- Group members: Nigel Doering, Tanuj Pankaj, Raechel Walker
Abstract: Recent events including the 2016 election, COVID-19 pandemic, the 2020 election, and the development of a COVID-19 vaccine has laid bare the essential need to prevent misinformation from spreading uncontrollably on social networks. Social media companies have developed systems for preventing the further spread of misinformation. Most notably, some companies have begun placing flags that warn a user of the misinformative content of the post. Research has addressed a way to analyze Twitter users on how conservative versus liberal, moderate versus extreme, and pro-science versus anti-science they are based on their tweet history. We detail a novel machine learning approach to classify users based on three similar dimensions. We then conduct an analysis comparing Twitter users who retweeted flagged tweets versus those who retweeted unflagged tweets, with the tweets coming from high profile conservative Twitter users, such as Eric Trump. Results from the analysis suggest that users who are sharing these flagged tweets tend to be slightly more liberal and more moderate than the users who are sharing unflagged tweets. We propose possible explanations, as well as future work to better understand the impact of misinformation flags.
Political Polarization of Major News Networks on Twitter
- Group members: Christopher Ly, Shutong Li, Mark Chang
Abstract: We will construct a geometric definition of the political spectrum of major US news outlets through an unsupervised approach. We model the political alignments of the outlets in terms of pairwise political similarity among pairs of outlets using graphs and embed the graph onto a Euclidean space for result. We will be collecting hashtags used in the users' own timelines and cross reference it with the hashtags used during election period to classify their political stance as well as create a graph analysis between the news networks as a whole. Through this, we demonstrate the location where each news network lies on the U.S. political spectrum and how they lie relative in hashtag vector space to one another.
Twitter’s Impact on Elections
- Group members: Prem Pathuri, Zhi Lin
Abstract: The rise of social media has dominated every aspect of our daily lives. In the height of the 2020 presidential election and as COVID-19 rampaged throughout the world, it facilitated increased online discussion, as well asthe spread of information and misinformation. This project investigates the relationship that discussion on social media has with election outcomes. It finds that in comparing two distinct presidential elections, both of whichtook place as Twitter usage grew steadily, increased discussion levels were present in a Democratic win of the election.
Analyzing the the Diffusions of Various Forms of Misinformation on Reddit
- Group members: Cindy Huynh, Hasan Liou, Helen Chung
Abstract: Misinformation has taken social media by storm. It reaches every corner of these kinds of platforms, from topics like the existence of aliens or even contesting the outcomes of a presidential election. The consequences of such viral misleading content is disruptive, and we are just beginning to see how devastating these effects can be in real time. We look into the diffusion of misinformation on Reddit, specifically how users within specific subreddits behave and interact with one another. We look at three categories of subreddits that we had selected: ones regarding scientific information, political misinformation, and urban myth misinformation. We analyze these three categories, analyzing how they intersect with each other or the lack thereof. We utilize user polarities, which is defined as how “loyal” a user may be to one category of subreddits compared to the other two categories on a number scale of 0 to 1. We conclude that there is the existence of echo chambers in the categories that we had looked at, and that the users within these respective categories behave differently from one another.
COVID-19 Sentiment and Daily ases Analysis on Social Media
- Group members: Jiawei Zheng, Yunlin Tang, Zhou Li
Abstract: With the unexpected impact of Covid-19, drastic changes were induced to people’s health, lifestyle, and mentality. During the research last quarter, we noticed that the majority of posts in our Twitter dataset have strong emotions and sentiments. In this project, we trained our SVC tweet sentiment model using a dataset that contains 1.6 million data with text and sentiment labels from Kaggle. The trained model is used to predict sentiment scores on the daily tweets sampled from the Panacea Lab dataset. After that, we detrended the daily case data and performed multiple analyses including correlation, cointegration test, and Fourier transformation to study its relationship with the sentiment score.
Conflict and Collaboration in Online Communities
Controversy in wikipedia articles.
- Group members: Hengyu Liu, Xiangchen Zhao, Xingyu Jiang
Abstract: There are “wars” going on every day online, but instead of cities, they are defending their options and perspects. This phenomenon is especially common on the Wikipedia platform where users are free to edit others' revisions. In fact, there are “about 12% of discussions are devoted to reverts and vandalism, suggesting that the WP development process is highly contentious.” (Robert 1) As Wikipedia has become a trusted source of information and knowledge which is freely accessible, It is important to investigate how editors collaborate and controvert each other in such a platform. This paper will discuss a new method of measuring controvisality in Wikipedia articles. We have found out that controversiality is highly related to the number of revert edits, the sentiment level among one article comments, and the view counts of that article. Thus we developed a weighted sum formula, which combines those three factors to accurately measure the controversy level within articles in Wikipedia.
The Large-Scale Collaborative Presence of Online Fandoms
- Group members: Casey Duong, Kylee Peng, Darren Liu
Abstract: Fan communities exist within every industry, and there has been little study on understanding their scale and how they influence the media and their industries. As technology and social media have made it easier than ever for fans to connect with their favorite influencers and find like-minded fans, we’ve seen a rise in fan culture or “fandom”. These individuals form fan groups and communities, which have become increasingly popular online and have rallied behind their favorite artists for different causes. In recent years, K-pop has taken the music industry by storm, quickly rising to global significance and gathering some of the most dedicated fanbases in the world. We explore the similarities and differences in collaboration efforts among fans of three popular artists, BTS, Taylor Swift, and Justin Bieber on two primary online social platforms, Twitter and Wikipedia. We present a new method to quantify the strength and influence of online fan communities—with a focus on the BTS fanbase—and how this online collaboration affects outside audiences.
Wikipedia’s Response to the COVID-19 Pandemic
- Group members: Michael Lam, Gabrielle Avila, Yiheng Ye
Abstract: Through collaborative efforts online, Wikipedia has always been at the forefront of providing information to the public on almost any topic, including a pandemic. Covid-19 has been one of the most relevant topics of 2020 and still remains so as of right now, therefore gathering as much information as possible is essential for the world to combat such a virus. Many official health sources online provide such knowledge with the resources that they have, but false or outdated information can spread quickly. In this article, we perform EDA and LDA on different Wikipedia articles related to coronavirus and compare the results to the word clouds of traditional sources to explore how Wikipedia can provide reliable and updated details and data about Covid-19.
A Study of LGBTQ+ Wikipedia Articles Sentiment over Time
- Group members: Henry Lozada, Emma Logomasini, Parth Patel, Yuanbo Shi
Abstract: We detail a specific method that determines how, if at all, sentiment changes over time for a category of Wikipedia articles, which, in our study, are articles categorized by Wikipedia as LGBT articles. This method uses three different sentiment analyzers, one for each of the three different language editions of Wikipedia we are analyzing, to calculate the sentiment of a Wikipedia article, doing so for all edits in the article's revision history and for all articles in each language's LGBT category. This enables us to calculate a fixed effects regression for each language's sentiment scores, allowing us to determine whether or not time has a positive effect on the articles' sentiment scores, as well as to compare these trends across languages.
Politics on Wikipedia
- Group members: Cameron Thomas, Iakov Vasilyev, Joseph Del-Val
Abstract: This paper seeks to analyze the degree and prevalence of political bias and controversy in Wikipedia. Using pre-trained models from Rheault and Cochrane (2019) and Shapiro and Gentzkow (2019) we validate our methods for generalizability on the ideological books corpus (Sim et al., 2013) with sub-sentential annotations (Iyyer et al., 2014) and attempt to apply these methods to receive insight into political bias in Wikipedia. We attempt to combat overlap in political slants and avoid labeling political bias whose detection is unavoidable due to the topic of the article in question. With insight into political bias on Wikipedia gained we hope it will be able to prove useful in combating counterproductive activity on Wikipedia and allow for more precise and targeted activity by Wikipedia monitors.
Genetic Basis of Mental Health
Differential gene expression analysis of human opioid abusers.
- Group members: Cathleen Pena, Dennis Wu, Zhaoyi Guo
Abstract: Opioid abuse is a serious national crisis. The opioid epidemic is unique and important because 21-29% of patients who are prescribed opioids end up misusing them [16]. Opioids increase the amount of dopamine made in the brain by increasing dopamine-synthesizing neurons- making them very easy to become addicted to. This study explores the long-lasting changes in gene expression that may contribute to addiction, cravings, and relapse by studying subjects who continuously used opioids. Using DESeq2 [7] and WGCNA [8], our analyses identified differentially expressed genes by finding which genes were up-regulated and down-regualted. We also found distinct gene networks associated with opioid abuse. Overall, 28 genes were found to be down-regualted and 16 genes were up-regulated. The opioid-regulated genes identified from our project could possibly serve as new therapeutic targets to help combat opioid addiction.
The Genetic Basis of Antibiotic Resistance in E. Coli
- Group members: Jiayi Wu, Alan Chen, Myra Haider
Abstract: One of the greatest challenges in public health is the growing number of bacterial species that have developed resistance to antibiotics through point mutations in the genome. Our project aims to identify these genetic markers of antibiotic resistance through a genome wide association study between 36 antibiotic resistant E.Coli samples and 36 controls. Variants were identified in both groups, checked for statistical significance, and analyzed for any functional effects.
Blood-based Analysis of Alzheimer's Disease from miRNA Data
- Group members: Gregory Thein, Justin Kang, Ryan Cummings
Abstract: Alzheimer’s Disease (AD) is an irreversible, progressive neurodegenerative disorder that slowly destroys a person's cognitive and physical abilities. The cause of AD is unclear, but is believed to be a combination of genetic, environmental and lifestyle factors. Because the only way to definitely diagnose AD is post mortem, the search for earlier definitive detection is crucial. One way of doing this is by analyzing blood samples to detect biomarkers and microRNAs. A biomarker is defined as a characteristic that is objectively measured as an indicator of normal biological processes, while microRNAs (miRNAs) are non-coding RNA molecules that are involved in the regulation of gene expression. Recent studies show miRNAs and biomarkers as possible tools for AD diagnosis, thus, leading us to analyze blood miRNA data for our study. Utilizing influences from various other studies, we examined 70 blood samples of AD and controlled patients through our custom genetics pipeline in hopes of a breakthrough in understanding the pathology of the disease. We then implemented two different statistical tests, a non-parametric hypothesis test (Wilcoxon-Mann-Whitney Test) and a parametric hypothesis t-test (DESeq2). From these tests we were able to isolate nine significant samples to perform further analysis on its relationship and effect to AD.
Comparison of Differential Gene Expression Analysis Tools
- Group members: Brandon Tsui, Joseph Bui, Weijie Cheng
Abstract: RNA-Seq (named as an abbreviation of "RNA sequencing") is a technology-based sequencing technique which uses next-generation sequencing (NGS) to reveal the presence and quantity of RNA in a biological sample at a given moment, analyzing the continuously changing cellular transcriptome. Differential expression analysis takes the normalized read count data and performs statistical analysis to discover quantitative changes in expression levels between experimental groups. As technology progresses by each year passing, there are now a lot of technological tools available in the Internet that can perform such differential expression analysis. The purpose of our project is to take a closer look at some of these tools, and compare their performances to understand which tools are optimal to utilize. Specifically, the softwares that we are going to focus on are: ABSSeq1, voom.limma2, PoissonSeq3, DESeq24, NOISeq5, ttest6 and edgeR7. We are going to compare their performances by looking at parameters such as Area Under the Curve (AOC), False Discovery Rate (FDR), Type I error rate, and Sensitivity.
Genetic Overlap between Alzheimer's, Parkinson’s, and healthy patients
- Group members: Xuanyu Wu, Justin Lu, Saroop Samra
Abstract: Our research compares overlapping patterns in miRNA between patients with Alzheimer's and Parkinson’s across two biofluids, cerebrospinal fluid and serum, by pinpointing significant transcription factors that the diseases share. We hope the results of our gene analysis can be leveraged by researchers to help alleviate the effects of the disorders and potentially develop medicines and therapies that target these genes.
Live vs. Video on Demand Inside VPN Detection
- Group members: Da Gong, Mariam Qader, Andrey Pristinsky, Tianran Qiu, Zishun Jin
Abstract: Due to the variety, affordability and convenience of online video streaming, there are more subscribers than ever to video streaming platforms. Moreover, the decreased operation of non-essential businesses and increase in the number of people working from home in this past year has further compounded this effect. More people are streaming live lectures, sports, news, and video calls via the internet at home today than we have ever seen before. In March 2020, Youtube saw a 2.5x increase in the amount of time people spent streaming live video [1]. Twitch more than doubled their hours of content in three months after the start of the pandemic [1]. There is a huge boom in the video content world, and it does not seem to be slowing down anytime soon. Internet Service Providers, such as Viasat, are tasked with optimizing internet connections and tailoring their allocation of resources to fit each unique customer’s needs. With this increase in internet activity, it would be especially beneficial for Viasat to understand what issues arise when customers stream various forms of video. When a user has difficulties with their internet connections, ISP’s want to be able to understand their activity to give potential reasons to why the problem occurred and a quick solution.
DANE: Data Automation and Network Emulation Tool
- Group members: Danial Yaseen, Sahil Altekar, Parker Addison
Abstract: In the field of network traffic research, datasets are often manually generated with in-house methods using fast internet connections. This creates a data representation issue, as we can’t expect all internet users to have great network conditions. How can we make sure network research is taking diverse network conditions into account? Is there a better way to generate traffic datasets with representative network conditions? DANE is a hackable and automated dataset generation tool which collects traffic data in a variety of configurable network environments. In our talk we introduce the tool, the purpose it serves, and how it works. Finally, we dive into an example of real-world analysis using data collected by our tool.
Res Recovery: Classifying Video Resolutions Through a VPN Tunnel
- Group members: Samson Qian, Shrimant Singh, Soon Shin, Iman Nematollahi, Stephen Doan
Abstract: Virtual private networks, or VPNs, have seen a growth in popularity as more of the general population has come to realize the importance of maintaining data privacy and security while browsing the Internet. In previous works, our domain developed robust classifiers that could identify when a user was streaming video. As an extension, our group has developed a Random Forest model that determines the resolution at the time of video streaming.
- Group members: Arely Vasquez, Chang Yuan, Raimundo Castro, Jerry Qian, Molly Rowland
Abstract: Whether to access another country's Netflix library or for privacy, more people are using Virtual Private Networks (VPN) to stream videos than ever before. However, many of the different service providers offer different user experiences that can lead to differences in the network transmissions. In this project we will discuss the methods in which we made a classifying model to determine what streaming service provider was being used over a VPN. The streaming providers that the model identifies are Amazon Prime, Youtube, Netflix, Youtube Live and Twitch. This is valuable in understanding the differences in the network work patterns for the different streaming service providers. Across all providers, our Random Forest model achieves a 96.5% accuracy in provider classification.
Particle Physics
Interpreting higgs boson interaction network with layerwise relevance propagation.
- Group members: Alex Luo, Cecilia Xiao
Abstract: While graph interaction networks achieve exceptional results in Higgs boson identification, GNN explainer methodology is still in its infancy. To introduce GNN interpretation to the particle physics domain, we apply layerwise relevance propagation (LRP) to our existing Higgs boson interaction network (HIN) to calculate relevance scores and reveal what features, nodes, and connections are most influential in prediction. We call this application HIN-LRP. The synergy between the LRP interpretation and the inherent structure of the HIN is such that HIN-LRP is able to illuminate which particles and particle features in a given jet are most significant in Higgs boson identification. The resulting interpretations are ultimately congruent with extant particle physics theory, with the model demonstrably learning the importance of concepts like the presence of muons, characteristics of secondary decay, and salient features such as impact parameter and momentum.
Deep Learning for Particle Jet Multiclassification
- Group members: Nathan Roberts, Darren Chang, Sharmi Mathur
Abstract: As data scientists, we are often driven toward those domains which generate vast amounts of data. High-energy physics is no exception. The Large Hadron Collider (LHC) alone produces around 90 petabytes of data per year (roughly 240 terabytes per day). As such, there are thousands upon thousands of researchers combing through the LHC’s particle interactions to draw conclusions. But, there exists one major difficulty in doing so: the colliders themselves only have instruments that can detect physical quantities (energies, momentums, and the like). The LHC simulates particle collisions that result in a spray of subatomic particles called jets. Considering the many categories of jets (Higgs boson, singly charmed quarks, etc.), classification of jets must be conducted outside of the LHC by researchers and their algorithms. We implement multiple multiclass classifiers (CNN, GNN, ENN) to discriminate between six types of jets which may occur. While a similar classifier exists at the LHC, the ceiling for improvement extends higher with each advancement in machine learning- deep neural network architecture being the most recent. In implementing our own neural network, we strive to achieve a higher level of model performance.
COVID-19 & Microbiome
Rtl automation.
- Group members: Richard Duong, Nick Lin, Yijian Zong
Abstract: The RTL Automation aims to build data pipelines, automate the major workflow of the RTL, and free researchers from doing manual chores like updating Google Sheet or drop in csv files. Simply put, we make researchers' lives easier and help them more efficiently end this pandemic.
Cyber-Physical Systems (CPS) using IOT Devices
Autobrick: a system for end-to-end automation of building point labels to brick turtle files.
- Group members: Advitya Gemawat, Devanshu Desai
Abstract: BRICK is a schema for representing various building equipment, including but not limited to, HVAC air handling units and carbon dioxide sensors in different rooms. While the schema is a clear step up over the current state-of-the-art, its potential is severely hindered because it is not backwards compatible. This means that converting CSV files storing building data to a BRICK-compatible data format is a cumbersome and imperfect process as different systems use different conventions to denote the same systems. This conversion usually required human involvement until now. AUTOBRICK is a software tool that automates this conversion with minimal human intervention and provides an order of magnitude greater speed up (90x) over the current state of the art.
Airborne Infection Risk Estimator for COVID-19
- Group members: Etienne Doidic, Zhexu Li, Nicholas Kho
Abstract: The global pandemic of COVID-19 has demonstrated the exceptional transmissibility of the SARS-CoV-2 virus and has highlighted the vulnerability of our built environments to similar airborne pathogens. The traditional process for retrieving information in the target area and making estimations is quite complicated and involves a lot of manual work. In order to bring a convenient and comprehensive view of important information and estimations in interested zones, our team has developed an open source app which could be easy enough to be used by the everyday consumer or as detailed as a building manager needs it to be.
System Usage Reporting (SUR, a.k.a. DCA)
Mouse wait classification.
- Group members: Pan Yeung, Sijie Mei, Yingyin Xiao
Abstract: This thesis describes an study of machine learning and its application to mouse wait time in computers. Specifically, we are building a classification model of mouse wait time based on dynamic and static system information within the 2020 time interval to classify if a mouse wait event would last within 0-5 secs, 5-10 secs, or 10+ secs. Dynamic system information, such as CPU utilization, is subject to the configuration of each system. Therefore, by incorporating static system information which includes the computer configuration of each system into the model, we could significantly improve the accuracy of the prediction. Currently, the model reaches an accuracy of 70\% with Decision Tree Classifier.
Predicting Battery Remaining Minutes based Related Features
- Group members: Jinzong Que, Yijun Liu, Kaixin Huang
Abstract: Our goal for this project is to understand and discover features that affect the battery’s estimated remaining time. Through our exploratory data analysis, we have discovered eight features, namely the number of devices, number of processes, average memory, average page faults, designed capacity, cpu percentage, cpu seconds, and cpu tempera- ture. Using these eight features, we decided to come up with several different models, Linear Regression, Decision Tree Regressor, SVM, Random Forest Regressor, Adaboost Regressor, Gradient Boosting Regressor and Bagging Re- gressor. To understand which model performs the best given these features, we performed hypothesis testing. In the end, our results show that Gradient Boosting Regressor performs the best out of all in that the maes generated on the train and test set are quite low and very similar. This indicates that Gradient Boosting Regressor has less of an overfitting issue than the other two models. Another indication is that through our hypothesis testing, our P-values indicate that Gradient Boosting Regressor performs the best among all others.
Persona Analysis
- Group members: Ruotian Gao, Xin Yu, Weihang Gao
Abstract: In this project, our goal is to find the relationship between the Persona and their PC system. We are trying to compare the performances of different models and use the features in the system to predict the type of a user. To achieve this, we collect data from the user end, clean the data, explore the data using hypothesis tests and fit our data into some classification machine learning models, and also test the performance and optimizing parameters of our models.
Predicting a User’s Persona Using Computer’s Specifications, CPU Utilization, CPU Temperature & Application Usage Time
- Group members: Jon Zhang, Keshan Chen, Vince Wong
Abstract: During the first half of this project, we learned about Intel’s telemetry framework. The framework allows remote data collection from devices with Windows operating systems. Two important components of the telemetry framework are the Input Library (IL) and Analyzer Task Library (ATL). The IL exposes metrics from a device and the ATL generates on-device statistics from the data collected by the IL. In the second half of the project, we used pre-collected data provided by Intel that used their telemetry framework to create a classification model. Our goal with the model was to predict the persona of a user using their computer’s specifications, CPU utilization, CPU temperature, and time spent on certain types of applications. User personas were provided by Intel which classified if users were casual web users, gamers, communication, etc.. The classifications of these personas were done by Intel based on the amount of time users spent on certain applications based on their usage of different types of .exe files. For example, if a majority of a device’s time is spent on an application like Skype, they are most likely classified as a communication user. Similarly, if a user spends a majority of their time on the League of Legends .exe file, they are most likely classified as a gamer. After training multiple classification models, we were able to predict user personas with 64% accuracy using a gradient boosting classifier. In the following paper, we will discuss our hypotheses, processes, methodologies, and results.
Spatial Agent-based Modeling for School Reopening
Geographically assisted agent-based model for covid-19 transmission (geoact).
- Group members: Johnny Lei, Akshay Bhide, Evan Price, Kaushik Ganapathy
Abstract: As schools attempt to reopen amid the COVID-19 pandemic, there is an increasing need to detect and quantify potentially risky activities in schools to help schools test out their individual reopening plans in order to prevent an outbreak. In this paper, we describe the development of a spatially explicit agent-based model to help detect risky activities and assess reopening plans for individual schools by incorporating elements such as behavioral factors, environmental factors, and effects from pharmaceutical and non-pharmaceutical interventions. Following this we describe the development of a gateway infrastructure powered by Apache Airavata to allow general-purpose users to run model simulations with user-defined parameters. Finally, we use the aforementioned model to estimate COVID-19 case counts and the effectiveness of proposed interventions over a two week period for a real school to demonstrate model usability.
Modelling COVID-19 Transmission in San Diego School Buses
- Group members: Ziqian Cui, Bernard Wong, Farhood Ensan, Areeb Syed
Abstract: Using Agent Based Modelling, we model the spread of COVID-19 in San Diego school buses, by running simulations of school trips, with the goal of providing guidlines on the key factors that impact the spread of the virus in the case of schools reopening for in-person education.
COVID-19 Spatial Agent-based Modeling: Single Room Infection
- Group members: Eric Yu, Bailey Man, Songling Lu, Michael Kusnadi
Abstract: Several models exist for the transmission of SARS-CoV-2 (severe acute respiratory syndrome coronavirus 2) based on varying assumptions and parameters. The Chu and Chen models investigate coronavirus transmission and infection as functions of nonpharmaceutical interventions (physical distances, masks) and respiratory droplets, respectively. The results of the Chu model suggest guidelines for social distancing (1 meter or more) between individuals and public usage of facial and eye protection, while the Chen model shows the relationship between droplet size and transmission range. The two models both attempt to examine coronavirus transmission, but they report results that are not necessarily conflicting, but rather, incomplete on their own. The significance of this problem is that because models vary depending on the parameters and underlying assumptions, there is uncertainty on how to filter out the valid and optimal inputs. In this replication study, we develop a simple infection rate model based on the results and parameters reported by the Chu and Chen models, the MIT COVID-19 Indoor Safety Tool, and the airborne.cam tool by Cambridge. The output of this experiment will be primarily a simulation where a user will be able to set parameters to see the resulting risks and infections caused by in person instructions. This report will be a secondary output along with the website and visual presentation and will be used as a guide to explain methods as well as theory behind the work.
FACULTY OPENING: The school of Engineering invites applicants for a Teaching Faculty position. →

Data Science Capstone
Interested in working with Rice student and faculty teams to solve real-world data science challenges? We are now accepting project proposals for Fall 2024. Submissions are due May 3, 2024.
D2K Capstone Timeline
DATE | EVENT |
---|---|
Jan. 8, 2024 | Spring 2024: First Day of Class |
Jan. 12, 2024 | Spring 2024: (Sponsors) Data transfer due and access granted to Faculty |
Jan. 22, 2024 | Spring 2024: Sponsor and Student Orientation in class |
Jan. 29, 2024 | Spring 2024: (Sponsors) Invoice due to Rice University |
April 17, 2024 | Spring 2024: D2K Showcase Event |
April 29, 2024 | Spring 2024: (Students) Final Report and Code due |
May 3, 2024 | Fall 2024: Call for Capstone Project Proposal due |
July 26, 2024 | Fall 2024: Last date for announcing accepted projects |
August 12, 2024 | Fall 2024: Signed Sponsored Research Agreement due |
August 26, 2024 | Fall 2024: First day of Class |
The First-of-its-kind Capstone Program
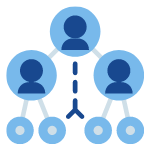
Experiential Learning
Innovative education in applied machine learning and data science.
Interdisciplinary student teams in the D2K Capstone program have the opportunity to utilize their machine learning and advanced computational skills by working on real-world data science challenges.

Industrial and Social Impact
Solutions to real-world data science challenges.
D2K Capstone students work on industry-sponsored (finance, energy, healthcare, tech) projects, community impact (government and non-profit) projects. At the end of the semester, students will present their work, compete for prizes and showcase their impact in the D2K Showcase. Learn more about our impact >>
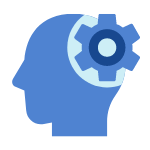
The Future of Data Science
We need people who can transform data into actionable knowledge..
The D2K Capstone helps students gain technical and practical skills, and prepares them to become the next generation of data scientists, analysts, and computational engineers. Many of our students continue working with clients through an internship or a full-time position.
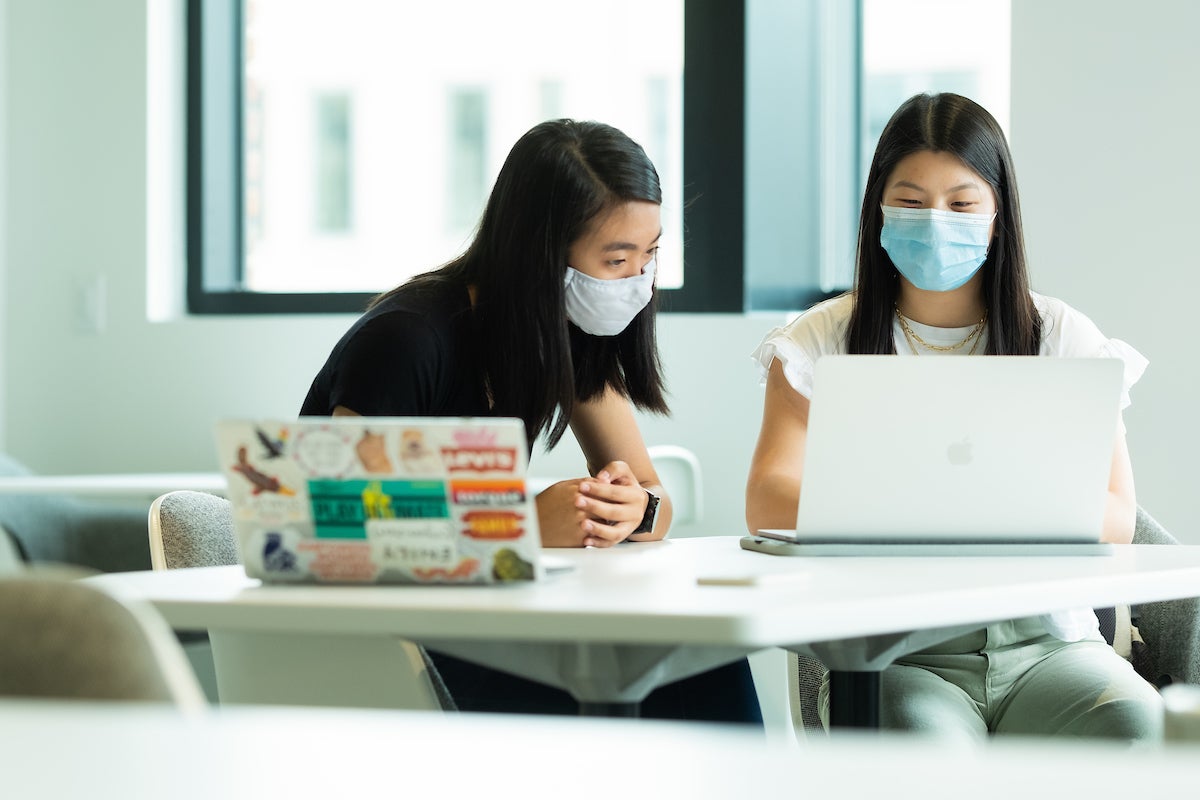
How It Works
Teams of 4-6 students come from a variety of disciplines and experience levels (advanced undergraduates and graduate students). Teams are co-mentored by a sponsor mentor, D2K Fellows, and Rice data science faculty.
A non-disclosure agreement for the students and faculty mentor is built into the sponsored research agreement. Rice will educate students and faculty as to the particular clauses of this agreement.
At the end of the semester, student teams produce a data science report and deliver all software and scripts used in the report.
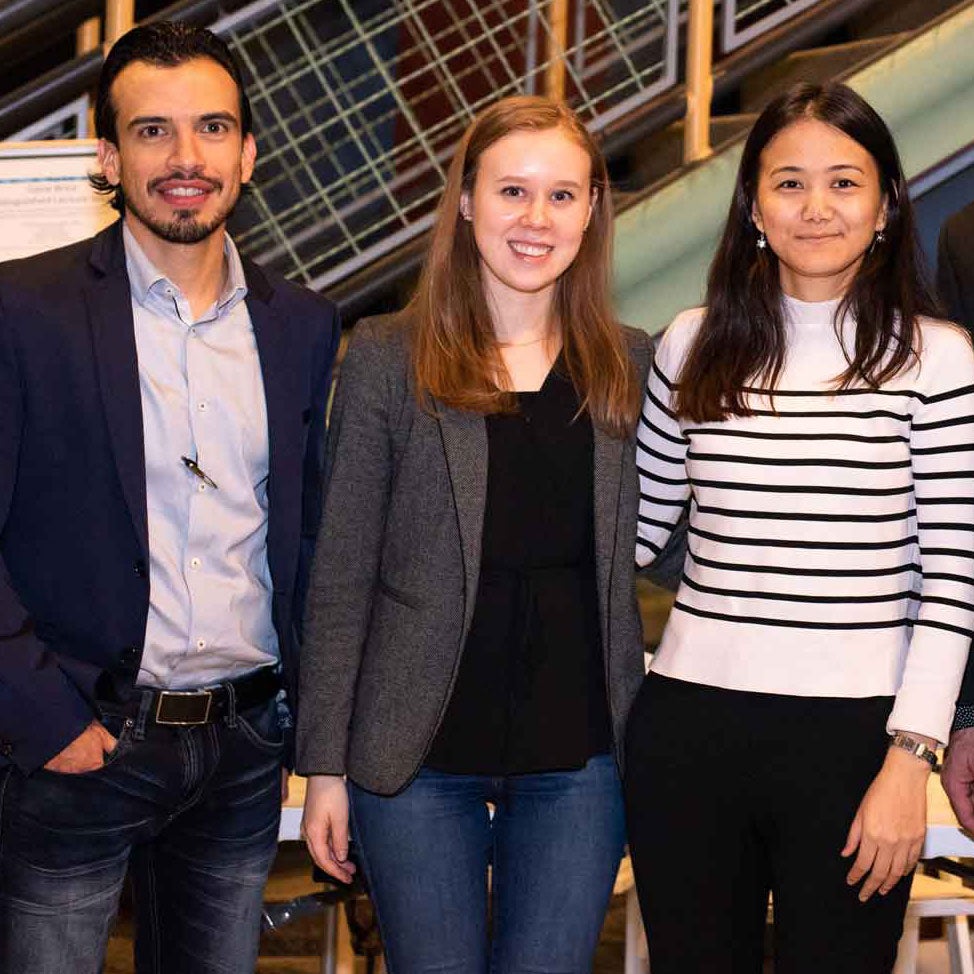
Partnerships
As a D2K Lab member, you have the opportunity to sponsor a data science capstone project. Our sponsors are paired with student-faculty teams to solve complex, real-world problems with the power of data science in an experiential learning environment like no other. With a sponsored research agreement, sponsors will own any resulting Intellectual Property (IP). Learn More about our Sponsor Research Agreement and the D2K Capstone Project Timeline >>
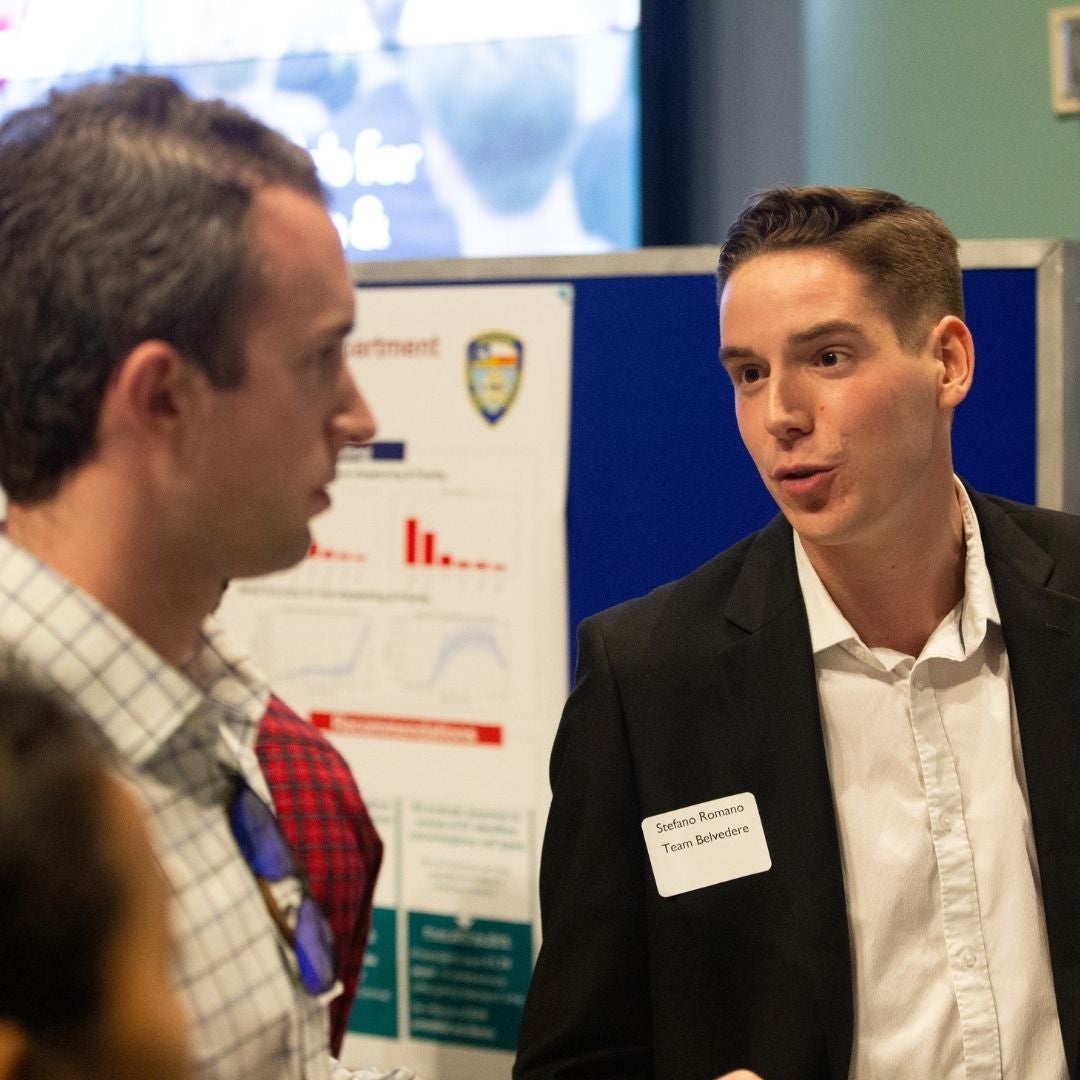
Student and Client Testimonials
“The cool thing about the Capstone program is that we actually help produce something useful, especially something like predicting cardiac signals, that I never would have thought I would be doing as a computer science and electrical engineering major.”
Read more about what our students and clients say about the D2K Capstone program >>
D2K Showcase Highlights
Vision zero houston: reducing traffic deaths to zero by 2030, using deep learning to predict stroke risk, using computer vision and ai to enhance on-field sports analytics, analyzing texas higher education data for financial reporting, recent capstone news.
Thursday, Apr. 21, 2022
Rice CS' Treangen Lab collaborated with D2K students, the Houston Zoo, and Baylor College of Medicine on the project.
Tuesday, Dec. 7, 2021
Monday, Dec. 6, 2021
Team’s computer vision system tracks waterfowl, counts them from the air
Monday, Jun. 7, 2021
Rice D2K Lab students build a predictive maintenance model to improve operations with machine learning.
Thank you to our partners and Spring 2023 project sponsors:
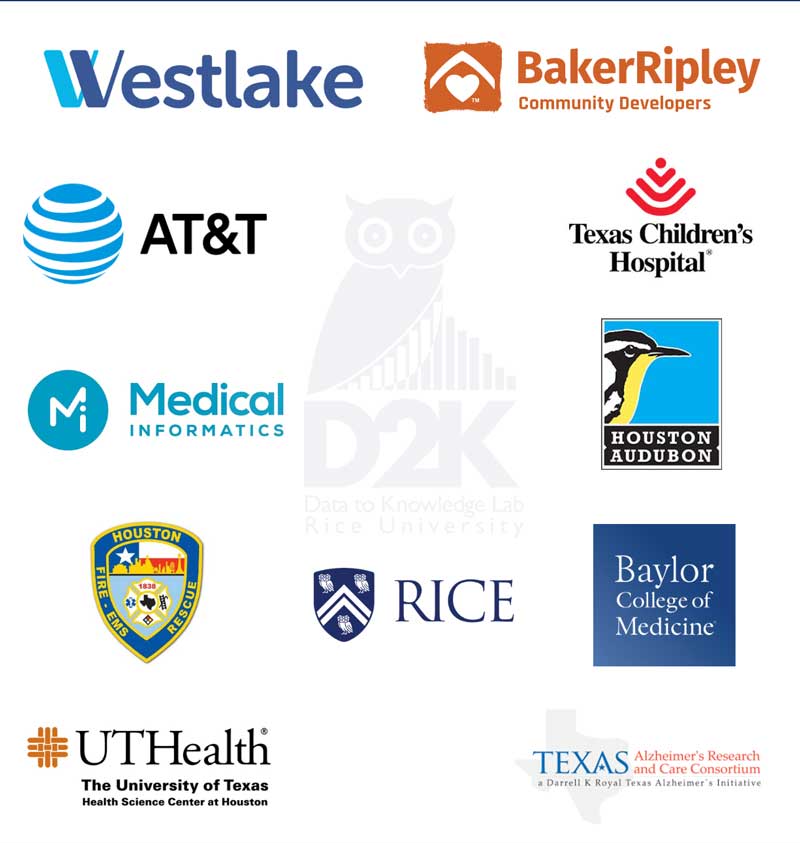
Helpful Links
Capstone Project in Data Science
(Fall 2020, Winter 2021, Spring 2021)
The course will study data science from the systems engineering perspective, introduce and address a variety of ethical issues that arise in data science projects, and engage students in project-based learning through a series of carefully selected and curated data science studies. A major overarching goal is to prepare students to make a positive impact on the world with data- intensive methodologies. In line with this, we will study and discuss a number of case studies in “ethics in data science” which emphasize responsible data practice. Another major focus will be on correctly interpreting, explaining, and communicating the results of analyses. This component of the course will focus on decision making under uncertainty, the role of correlation and causation, and drawing attention to common statistical traps and paradoxes that drive erroneous conclusions.
The Fall course is a lecture-based course with projects and papers. The capstone projects (pursued in Winter and Spring) will be interdisciplinary, will have outside customers, and will require students to apply skills or investigate issues across different subject areas or domains of knowledge. Students will work with leaders from the industry and research labs. See the sponsoring institutions at https://centralcoastdatascience.org/industry . Examples of projects include quantifying insect-plant network interactions, risk prediction, energy efficiency, inferring health from personal fitness devices, call tracking/analytics, and modeling of COVID-19.
Upon completing the course sequence, students will be able to understand the data science process and the structure and the role of each of its constituent steps; engineer the appropriate data science process for a given data analytical problem; design and implement evaluation studies to compare the quality of performed data analysis; understand technical trade-offs associated with working with “Big Data”; understand ethical implications of data science work, and be able to apply ethical reasoning to specific data science projects; visualize the results of data analytical studies, and convey them to customers.
- Classroom instruction in Fall 2020: focus on the process of discovering knowledge from data, public policy, ethics, fairness, and statistical traps.
- Followed by two quarters of faculty-mentored experiential project work.
- Synthesize course materials from individual machine learning, statistics, and data engineering courses, and place them in the context of concrete problems and datasets.
- Culminates in an end-of-year showcase of projects to the local data science community
- Oral communication and public speaking
- Time management
- Data analysis and informed decision making
Enrollment details: 4 units each quarter
- Fall 2020: CMPSC 190DD, MW 5-6:30.
- Winter 2021: CMPSC 190DE, times TBD.
- Spring 2021: CMPSC 190DF, times TBD.
If you are interested, please fill out this course survey.
Staff: Tim Robinson, [email protected] Faculty: Ambuj Singh, [email protected]
UCSB Contact
- +1-805-893-3510
- [email protected]
- Office 2118 Harold Frank Hall
Cal Poly Contact
- +1-805-756-5624
- [email protected]
- Office 14-227
- Information
- Terms of Use
Capstone Projects
The capstone project experience.
In the final two quarters of the program, students gain real world experience working in small groups on a data science challenge facing a company or not-for-profit. At the conclusion of the capstone project, sponsoring organizations are invited to attend a formal Capstone Event where students showcase their work. Capstone projects typically span a wide range of interests, including energy, agriculture, retail, urban planning, healthcare, marketing, and education.
Examples of Previous Capstone Sponsors
- Biblioteca Italiana Seattle
- Civil & Environmental Engineering, WSU
- Equal Opportunity Schools
- iSchool, UW
- Kids on 45th
- Seattle Children’s Hospital
- Urban Planning, UW
Capstone 2020-22 Archives (Gather.Town)

Due to the pandemic, our Capstone 2021 was held entirely online in the Gather.Town platform , to which we added galleries of our 2020 and 2022 Capstone projects for an archive you can digitally wander and browse.
Gather presents a map-based, interactive platform where you can wander among projects, see media like posters, infographics, and video, and do video/audio chat with others who are logged into the space. You can read some basics about using this platform at the Gather site. One of the other benefits of Gather is that it created a persistent archive of our Capstone 2020-2022 projects, which you can view and digitally wander among here:
https://tinyurl.com/msdsfair
Other examples of past projects.

Visualizing Gentrification in Seattle
MSDS students Deepa Agrawal, Angel Wang, and Erin Orbits created an interactive mapping tool to visualize gentrification in Seattle.
Sponsor: Urban Planning, University of Washington

Using Artificial Intelligence to Monitor Inventory in Real Time
Capstone researchers Havan Agrawal, Toan Luong, Vishnu Nandakumar, and Tejas Hosangadi explored new methods for optimizing supply chains and product placements to improve sales.
Sponsor: Clobotics

Predicting Soil Moisture with Machine Learning
MSDS students Samir Patel, Rex Thompson, Michael Grant, and Dane Jordan developed machine learning models to accurately estimate soil moisture using satellite imagery.
Sponsor: Civil & Environmental Engineering, Washington State University
Admissions Timelines
Applications for Autumn 2024 admissions are now closed.
Information about Autumn 2025 applications will be available in October.
Admissions Updates
Be boundless, connect with us:.
© 2024 University of Washington | Seattle, WA
Navigation Menu
Search code, repositories, users, issues, pull requests..., provide feedback.
We read every piece of feedback, and take your input very seriously.
Saved searches
Use saved searches to filter your results more quickly.
To see all available qualifiers, see our documentation .
- Notifications You must be signed in to change notification settings
maroofc/Applied-Data-Science-Capstone-Project
Folders and files.
Name | Name | |||
---|---|---|---|---|
17 Commits | ||||
Repository files navigation
Applied-data-science-capstone-project.
This will be my 1st file to initialize this repo as part of the course!
- Jupyter Notebook 99.6%
- Python 0.4%
Capstone Projects
Online M.S. in Data Science students are required to complete a capstone project. Capstone projects challenge students to acquire and analyze data to solve real-world problems. Project teams consist of two to four students and a faculty advisor. Teams select their capstone project in term 4 and work on the project in term 5, which is their final term.
Most projects are sponsored by an organization—academic, commercial, non-profit, and government—seeking valuable recommendations to address strategic and operational issues. Depending on the needs of the sponsor, teams may develop web-based applications that can support ongoing decision-making. The capstone project concludes with a paper and presentation.
Key takeaways:
- Synthesizing the concepts you have learned throughout the program in various courses (this requires that the question posed by the project be complex enough to require the application of appropriate analytical approaches learned in the program and that the available data be of sufficient size to qualify as ‘big’)
- Experience working with ‘raw’ data exposing you to the data pipeline process you are likely to encounter in the ‘real world’
- Demonstrating oral and written communication skills through a formal paper and presentation of project outcomes
- Acquisition of team building skills on a long-term, complex, data science project
- Addressing an actual client's need by building a data product that can be shared with the client
Capstone projects have been sponsors by a variety of organizations and industries, including: Capital One, City of Charlottesville, Deloitte Consulting LLP, Metropolitan Museum of Art, MITRE Corporation, a multinational banking firm, The Public Library of Science, S&P Global Market Intelligence, UVA Brain Institute, UVA Center for Diabetes Technology, UVA Health System, U.S. Army Research Laboratory, Virginia Department of Health, Virginia Department of Motor Vehicles, Virginia Office of the Governor, Wikipedia, and more.
Sponsor a Capstone Project
View previous examples of capstone projects and check out answers to frequently asked questions.
What does the process look like?
- The School of Data Science periodically puts out a Call for Proposals . Prospective project sponsors submit official proposals, vetted by the Associate Director for Research Development, Capstone Director, and faculty.
- Sponsors present their projects to students at “Pitch Day” during Semester 4, where students have the opportunity to ask questions.
- Students individually rank their top project choices. An algorithm sorts students into capstone groups of approximately 3 to 4 students per group.
- Adjustments are made by hand as necessary to finalize groups.
- Each group is assigned a faculty mentor, who will meet groups each week in a seminar-style format.
What is the seminar approach to mentoring capstones?
We utilize a seminar approach to managing capstones to provide faculty mentorship and streamlined logistics. This approach involves one mentor supervising three to four loosely related projects and meeting with these groups on a regular basis. Project teams often encounter similar roadblocks and issues so meeting together to share information and report on progress toward key milestones is highly beneficial.
Do all capstone projects have corporate sponsors?
Not necessarily. Generally, each group works with a sponsor from outside the School of Data Science. Some sponsors are corporations, some are from nonprofit and governmental organizations, and some are from in other departments at UVA.
One of the challenges we continue to encounter when curating capstone projects with external sponsors is appropriately scoping and defining a question that is of sufficient depth for our students, obtaining data of sufficient size, obtaining access to the data in sufficient time for adequate analysis to be performed and navigating a myriad of legal issues (including conflicts of interest). While we continue to strive to use sponsored projects and work to solve these issues, we also look for ways to leverage openly available data to solve interesting societal problems which allow students to apply the skills learned throughout the program. While not all capstones have sponsors, all capstones have clients. That is, the work is being done for someone who cares and has investment in the outcome.
Why do we have to work in groups?
Because data science is a team sport!
All capstone projects are completed by group work. While this requires additional coordination , this collaborative component of the program reflects the way companies expect their employees to work. Building this skill is one of our core learning objectives for the program.
I didn’t get my first choice of capstone project from the algorithm matching. What can I do?
Remember that the point of the capstone projects isn’t the subject matter; it’s the data science. Professional data scientists may find themselves in positions in which they work on topics assigned to them, but they use methods they enjoy and still learn much through the process. That said, there are many ways to tackle a subject, and we are more than happy to work with you to find an approach to the work that most aligns with your interests.
Why don’t we have a say in the capstone topics?
Your ability to influence which project you work on is in the ranking process after “pitch day” and in encouraging your company or department to submit a proposal during the Call for Proposal process. At a minimum it takes several months to work with a sponsor to adequately scope a project, confirm access to the data and put the appropriate legal agreements into place. Before you ever see a project presented on pitch day, a lot of work has taken place to get it to that point!
Can I work on a project for my current employer?
Each spring, we put forward a public call for capstone projects. You are encouraged to share this call widely with your community, including your employer, non-profit organizations, or any entity that might have a big data problem that we can help solve. As a reminder, capstone projects are group projects so the project would require sufficient student interest after ‘pitch day’. In addition, you (the student) cannot serve as the project sponsor (someone else within your employer organization must serve in that capacity).
If my project doesn’t have a corporate sponsor, am I losing out on a career opportunity?
The capstone project will provide you with the opportunity to do relevant, high-quality work which can be included on a resume and discussed during job interviews. The project paper and your code on Github will provide more career opportunities than the sponsor of the project. Although it does happen from time to time, it is rare that capstones lead to a direct job offer with the capstone sponsor's company. Capstone projects are just one networking opportunity available to you in the program.
Capstone Project Reflections From Alumni
For my Capstone project, I used Python to train machine learning models for visual analysis – also known as computer vision. Computer vision helped my Capstone team analyze the ergonomic posture of workers at risk of developing musculoskeletal injuries. We automated the process, and hope our work further protects the health and safety of American workers.” — Theophilus Braimoh, MSDS Online Program 2023, Admissions Student Ambassador
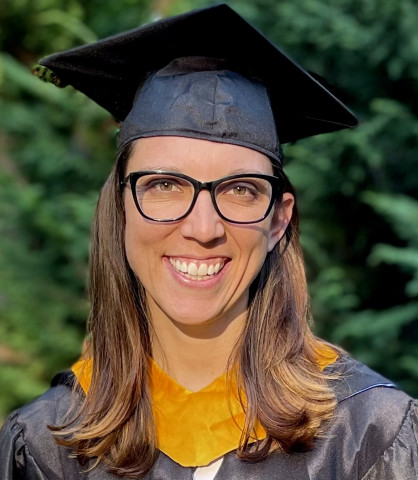
“My Capstone experience with the ALMA Observatory and NRAO was a pivotal chapter in my UVA Master’s in Data Science journey. It fostered profound growth in my data science expertise and instilled a confidence that I'm ready to make meaningful contributions in the professional realm.” — Haley Egan, MSDS Online Program 2023, Admissions Student Ambassador
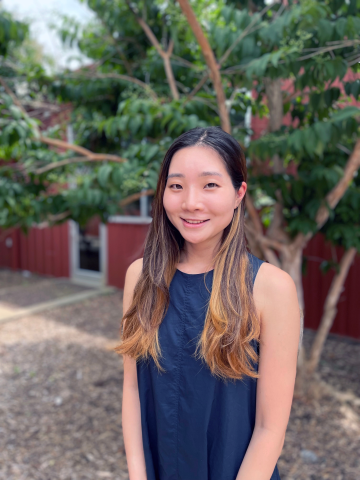
“Our Capstone projects gave us the opportunity to gain new domain knowledge and answer big data questions beyond the classroom setting.” — Mina Kim, MSDS Residential Program 2023, Ph.D. in Psychology Candidate
Capstone Project Reflections From Sponsors
“For us, the level of expertise, and special expertise, of the capstone students gives us ‘extra legs’ and an extra push to move a project forward. The team was asked to provide a replicable prototype air quality sensor that connected to the Cville Things Network, a free and community supported IoT network in Charlottesville. Their final product was a fantastic example that included clear circuit diagrams for replication by citizen scientists.” — Lucas Ames, Founder, Smart Cville
“Working with students on an exploratory project allowed us to focus on the data part of the problem rather than the business part, while testing with little risk. If our hypothesis falls flat, we gain valuable information; if it is validated or exceeded, we gain valuable information and are a few steps closer to a new product offering than when we started.” — Ellen Loeshelle, Senior Director of Product Management, Clarabridge
Get the latest news
Subscribe to receive updates from the School of Data Science.
- Prospective Student
- School of Data Science Alumnus
- UVA Affiliate
- Industry Member
- Industrial and Management Systems Engineering Home
- Undergraduate
Senior Design Capstone Project
The WVU Industrial Engineering Capstone Program aims to develop mutually-beneficial relationships with businesses in the community and senior-level Industrial Engineering students, by providing free-of-cost industrial engineering support. The WVU IECP supports and coordinates the senior design projects for graduating seniors from the ABET-accredited Bachelor of Science in Industrial Engineering program.
We are continuously looking for senior design projects to i) provide student learning experiences and ii) uphold the land-grant mission of the University by supporting our local community.
With the WVU IECP, at no monetary cost to you, we provide a team of 3-6 senior industrial engineering students, with the majority having at least one prior full-time internship or co-op experience, who will partner with you to resolve a complex problem using industrial engineering skills and tools. In exchange, our students will gain valuable “real-world” experience.
In a general sense, the WVU IECP supports any type of IE projects that are centered around process improvements for businesses with pre-existing processes and business practices. This can take on many forms, and the WVU IECP is happy to assist in identifying and defining the most meaningful/useful projects for your business. If you have been struggling with project/process/operational efficiencies and just can’t seem to narrow down the problem and/or right solution, we are here to help!
Benefits to you:
- Free of cost service to your facility
- Attention allotted to a project that may not have otherwise received attention
- A fresh perspective and a new take on a problem, often resulting in creative solutions not yet considered
- Development of a mutually beneficial relationship with the WVU IMSE Department
- Opportunity to learn about our students and potentially find your next hire!
Types of Projects:
- Production planning and control
- Productivity performance improvements
- Inventory improvements/optimization
- Industrial quality control
- Project scheduling
- Human factors: safety and ergonomics
- Plant layout and material handling
- Elimination of wastes
- Customer service improvements
- Optimization (with linear programming)
- SO MUCH MORE!
Deliverables:
- Implementable recommendation(s) with detailed engineering analysis
- Formal written report including all analyses, findings, and recommendations
- Presentation of project from start to finish, including recommendations by WVU student teams to your company
What we provide:
- A team of 3-6 eager and knowledgeable senior-level students
- Guidance and support for students in the classroom
- Options for Fall, Spring, or Full-Academic-Year projects
- Travel for site visit(s), if applicable
What we need from you:
- A complex engineering problem (we can help you identify/assess this!)
- A site visit, either in person (preferred) or virtual with student team members
- Commitment to our students (time and energy)
- Willingness and ability to support their data and information needs to fully assess the problem(s)
WVU Industrial Engineering Capstone Project Interest Form
Senior Capstone Perspective
Tommy Azinger
BSIE December 2023
The WVU IECP gave me great insight into the kinds of skills I need to be a successful industrial engineer. Being directly involved in a project like that, allowed me to utilize the tools that I have picked up along my academic path and gave me experience with professionally engaging with clients. The IMSE Department does a wonderful job of ensuring that the students learn the appropriate content and that they are actively applying what they are learning. I have grown a tremendous amount because of the WVU IECP and from the internship experiences I have had over my college career!
Piper Gaines
BSIE Graduating Class May 2024
My capstone project experience has given me many useful tools and the knowledge to have confidence as I go into my future career. My project is improving a production planning process, and this will impact my future greatly as it will give me experience in a field I have not really worked much with yet.
Kasmir Lauber
The capstone project during my final semester at WVU was a valuable experience that prepared me to hit the ground running in my first job after college. While there are numerous positive aspects I could highlight about collaborating with industry partners, one particular aspect stood out to me – the impact of working on real-world projects with authentic data on one's approach. It enhances communication skills and fosters a sense of ambition to deliver tangible results. Overall, I believe the capstone project experience is an excellent opportunity to facilitate a seamless transition from school to the industry.
Juan Marino
IMSE Class of December 2023, first ever class of the WVU IECP
My capstone project posed a significant challenge, requiring me to learn a completely unfamiliar software and tackle the tasks without a partner. Despite these hurdles, the project proved to be a valuable experience, fostering my skills in self-directed learning, effective communication, and project management.
Isabelle Nesbit
ABM IMSE grad 2025
My classmates and I have been working on a project for the new Clorox facility in Inwood, WV having a real industry project for my senior capstone course is so beneficial to me. It's really given me the opportunity to take the skills that I have been learning for the past four years in the classroom and apply them to real engineering problem in industry. I think my favorite part of working on this project so far is that it's helped me identify places in my engineering skillset that I really need to work on strengthening. I'm lucky to have professors and resources at WVU that can really help me improve these skills before I am in the workforce. Not many students get the opportunity to transfer their skills to the workforce before they even graduate I'm grateful that my industrial engineering Capstone program experience has helped prepare me to be a successful engineer after I graduate from WVU.
Jerry Finnegan
Manufacturing Engineering Manager - Industry Partner - Sensus
It was a pleasure to work with our WVU IECP partner this past Fall semester. This was a good first experience. Juan was eager to learn and get involved. We are currently partnered with a team for the Spring and look forward to continuing our relationship in the future.
Dave Pancake
Industry Partner - SJ Morse
Our Fall 2023 project team was immediately engaged in the goal definition, early investigations and analysis, applying their initial assumptions to new ideas and solutions. Their effort resulted in a comprehensive report with immediate actionable next steps toward improving production time efficiencies and methods to measure continuous improvement. Thank you for the wonderful opportunity to work with WVU IECP!
Department of Industrial and Management Systems Engineering
1306 Evansdale Drive | PO Box 6107 Morgantown, West Virginia 26506-6107 Phone: 304-293-9470 | Fax: 304-293-4970
Benjamin M. Statler College of Engineering and Mineral Resources
1374 Evansdale Drive | PO Box 6070 Morgantown, West Virginia 26506-6070
Phone: 304.293.4821 | Email: [email protected]
Driving Directions
Connect With Us
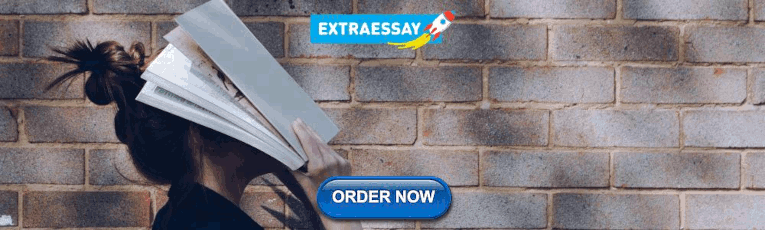
IMAGES
VIDEO
COMMENTS
Project Idea #10: Building a Chatbot. A chatbot is a computer program that uses artificial intelligence to simulate human conversation. It can interact with users in a natural language through text or voice. Building a chatbot can be an exciting and challenging data science capstone project.
Capstone projects in data science education play a pivotal role, offering students hands-on experience to apply theoretical concepts in practical settings. These projects serve as a culmination of their learning journey, providing invaluable opportunities for skill development and problem-solving.
There are 7 modules in this course. The capstone project class will allow students to create a usable/public data product that can be used to show your skills to potential employers. Projects will be drawn from real-world problems and will be conducted with industry, government, and academic partners.
Faculty-Sponsored Capstone Projects. A DSI faculty member proposes a research project and advises a team of students working on this project. This is a great way to run a research project with enthusiastic students, eager to try out their newly acquired data science skills in a research setting.
By completing this capstone project you will get an opportunity to apply the knowledge and skills in R data analysis that you have gained throughout the series. This final project will test your skills in data visualization, probability, inference and modeling, data wrangling, data organization, regression, and machine learning.
In this capstone course, you will apply various data science skills and techniques that you have learned as part of the previous courses in the IBM Data Science with R Specialization or IBM Data Analytics with Excel and R Professional Certificate. For this project, you will assume the role of a Data Scientist who has recently joined an ...
This is the final course in the IBM Data Science Professional Certificate as well as the Applied Data Science with Python Specialization. This capstone project course will give you the chance to practice the work that data scientists do in real life when working with datasets. In this course you will assume the role of a Data Scientist working ...
To become an expert data scientist you need practice and experience. By completing this capstone project you will get an opportunity to apply the knowledge and skills in R data analysis that you have gained throughout the series. This final project will test your skills in data visualization, probability, inference and modeling, data wrangling ...
Add this topic to your repo. To associate your repository with the data-science-capstone topic, visit your repo's landing page and select "manage topics." GitHub is where people build software. More than 100 million people use GitHub to discover, fork, and contribute to over 420 million projects.
This page contains the project materials for UCSD's Data Science Capstone sequence. Projects are grouped into subject-matter areas called domains of inquiry, led by the domain mentors listed below. Each project listing contains: The title and abstract, A link to the project's website. A link to the project's code repository.
Capstone Projects. You will stay at the cutting edge of what real clients need from data scientists through the Capstone experience. You will enhance your collaboration skills, benefit mentoring, and engage in networking opportunities. These faculty-mentored teams add value to top companies across multiple sectors—from finance to ...
Data science education for master's students at Harvard culminates in a semester-long capstone research project course where skills like machine learning, statistics, data management and visualization are used to solve real-world problems from partner companies and organizations.
Many websites and online courses focus on what beginners need to learn in order to become data scientists or on the importance of doing capstone projects to showcase one's skills.
This page contains the project materials for UCSD's Data Science Capstone sequence. Projects are grouped into subject-matter areas called domains of inquiry, led by the domain mentors listed below. Each project listing contains: The title and abstract, A link to the project's website; A link to the project's code repository
Capstone Projects. M.S. in Data Science students are required to complete a capstone project. Capstone projects challenge students to acquire and analyze data to solve real-world problems. Project teams consist of two to four students and a faculty advisor. Teams select their capstone project at the beginning of the year and work on the project ...
There are 4 modules in this course. Data science is a dynamic and growing career field that demands knowledge and skills-based in SQL to be successful. This course is designed to provide you with a solid foundation in applying SQL skills to analyze data and solve real business problems. Whether you have successfully completed the other courses ...
Rice University's D2K Lab offers cutting-edge data science courses and impact projects for students to work closely with companies and researchers to make sense of their data. By working on the capstone projects, students apply computing and data science skills to solve real-world data challenges. Fostering diversity and an intellectual environment, Rice University is a comprehensive research ...
Program Details and Capstone Projects. For people who are not aware - Praxis Business School offers a year-long program - PGP in Data Science with ML & AI at both its campuses - Kolkata and Bengaluru. The program is structured in a manner where the first 9 months are spent in the classroom with in-house and industry faculty and the last 3 ...
The capstone course will cement skills learned throughout the MIDS program — both core data science skills and "soft skills" like problem-solving, communication, influencing, and management — preparing students for success in the field. The centerpiece is a semester-long group project in which teams of students propose and select project ideas, conduct and communicate their
Capstone Project in Data Science (Fall 2020, Winter 2021, Spring 2021) The course will study data science from the systems engineering perspective, introduce and address a variety of ethical issues that arise in data science projects, and engage students in project-based learning through a series of carefully selected and curated data science ...
In the capstone, students will engage on a real world project requiring them to apply skills from the entire data science pipeline: preparing, organizing, and transforming data, constructing a model, and evaluating results.
The Capstone Project Experience. In the final two quarters of the program, students gain real world experience working in small groups on a data science challenge facing a company or not-for-profit. At the conclusion of the capstone project, sponsoring organizations are invited to attend a formal Capstone Event where students showcase their work.
Applied-Data-Science-Capstone-Project. This will be my 1st file to initialize this repo as part of the course! About. No description, website, or topics provided. Resources. Readme Activity. Stars. 0 stars Watchers. 1 watching Forks. 0 forks Report repository Releases No releases published. Packages 0.
Capstone Projects. Online M.S. in Data Science students are required to complete a capstone project. Capstone projects challenge students to acquire and analyze data to solve real-world problems. Project teams consist of two to four students and a faculty advisor. Teams select their capstone project in term 4 and work on the project in term 5 ...
Choose a specialism to match your career needs, from machine learning and AI, data science, web and mobile development, UX and more Specialise in 1 of 7 cutting-edge topics: ML and AI, data science, web and mobile development, physical computing and IoT, game development, VR, or UX. ... Learn how to tackle real-world problems with the capstone ...
The capstone project during my final semester at WVU was a valuable experience that prepared me to hit the ground running in my first job after college. While there are numerous positive aspects I could highlight about collaborating with industry partners, one particular aspect stood out to me - the impact of working on real-world projects ...