Multistep Worksheets
- Kindergarten
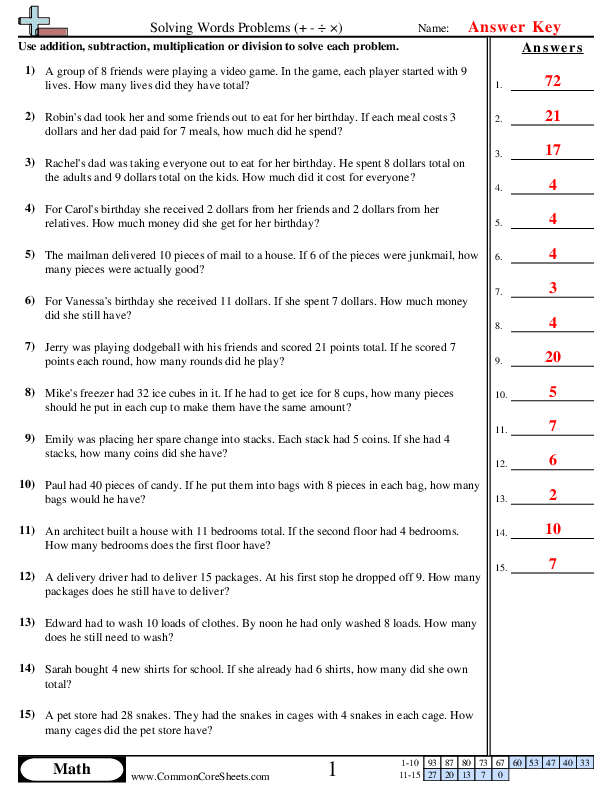

Addition (Basic)
Addition (Multi-Digit)
Algebra & Pre-Algebra
Comparing Numbers
Daily Math Review
Division (Basic)
Division (Long Division)
Hundreds Charts
Measurement
Multiplication (Basic)
Multiplication (Multi-Digit)
Order of Operations
Place Value
Probability
Skip Counting
Subtraction
Telling Time
Word Problems (Daily)
More Math Worksheets
Reading Comprehension
Reading Comprehension Gr. 1
Reading Comprehension Gr. 2
Reading Comprehension Gr. 3
Reading Comprehension Gr. 4
Reading Comprehension Gr. 5
Reading Comprehension Gr. 6
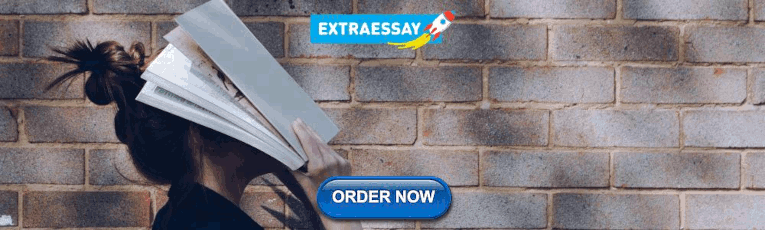
Reading & Writing
Reading Worksheets
Cause & Effect
Fact & Opinion
Fix the Sentences
Graphic Organizers
Synonyms & Antonyms
Writing Prompts
Writing Story Pictures
Writing Worksheets
More ELA Worksheets
Consonant Sounds
Vowel Sounds
Consonant Blends
Consonant Digraphs
Word Families
More Phonics Worksheets
Early Literacy
Build Sentences
Sight Word Units
Sight Words (Individual)
More Early Literacy
Punctuation
Subjects and Predicates
More Grammar Worksheets
Spelling Lists
Spelling Grade 1
Spelling Grade 2
Spelling Grade 3
Spelling Grade 4
Spelling Grade 5
Spelling Grade 6
More Spelling Worksheets
Chapter Books
Charlotte's Web
Magic Tree House #1
Boxcar Children
More Literacy Units
Animal (Vertebrate) Groups
Butterfly Life Cycle
Electricity
Matter (Solid, Liquid, Gas)
Simple Machines
Space - Solar System
More Science Worksheets
Social Studies
Maps (Geography)
Maps (Map Skills)
More Social Studies
Mother's Day
Father's Day
More Holiday Worksheets
Puzzles & Brain Teasers
Brain Teasers
Logic: Addition Squares
Mystery Graph Pictures
Number Detective
Lost in the USA
More Thinking Puzzles
Teacher Helpers
Teaching Tools
Award Certificates
More Teacher Helpers
Pre-K and Kindergarten
Alphabet (ABCs)
Numbers and Counting
Shapes (Basic)
More Kindergarten
Worksheet Generator
Word Search Generator
Multiple Choice Generator
Fill-in-the-Blanks Generator
More Generator Tools
Full Website Index
Multiple-Step Word Problems
These multiple-step word problems require students to use reasoning and critical thinking skills to determine how each problem can be solved.
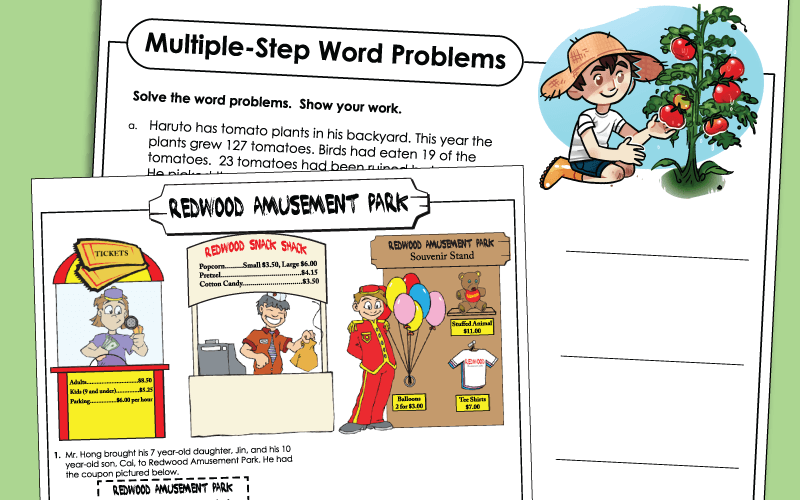
Basic (Grades 2 - 3)
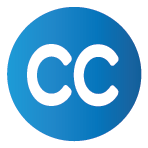
Logged in members can use the Super Teacher Worksheets filing cabinet to save their favorite worksheets.
Quickly access your most used files AND your custom generated worksheets!
Please login to your account or become a member and join our community today to utilize this helpful feature.
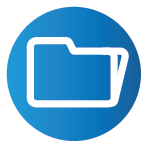
Intermediate (Grades 3 - 4)
Advanced (grades 4 - 5).
These word problems are sorted by type: addition, subtraction, multiplication, division, fractions and more.
These worksheets, sorted by grade level, cover a mix of skills from the curriculum.
S.T.W. has thousands of worksheets. Visit the full math index to find them all, sorted by topic.
Sample Images of Our Printables
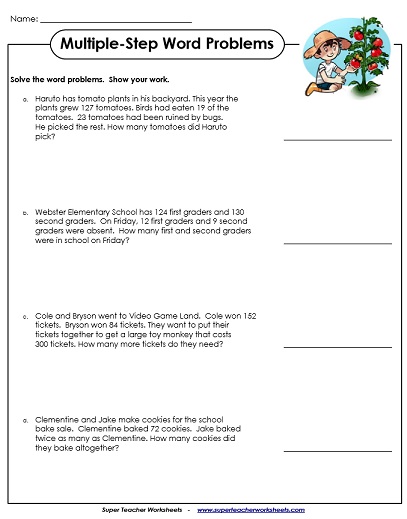
PDF with answer key:
PDF no answer key:
Transum Shop :: Laptops aid Learning :: School Books :: Tablets :: Educational Toys :: STEM Books
Menu Level 1 Level 2 Level 3 More Arithmetic
This is level 1; Addition and subtraction multi-step problems. You can earn a trophy if you get at least 7 questions correct.
This is Multi-step Problems level 1. You can also try: Level 2 Level 3
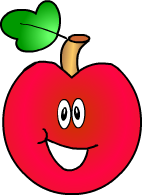
For Students:
- Times Tables
- TablesMaster
- Investigations
- Exam Questions
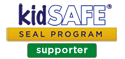
For Teachers:
- Starter of the Day
- Shine+Write
- Random Names
- Maths Videos
- Laptops in Lessons
- Maths On Display
- Class Admin
- Create An Account
- About Transum
- Privacy Policy
©1997-2024 WWW.TRANSUM.ORG
Description of Levels
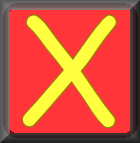
Level 1 - Addition and subtraction multi-step problems
Level 2 - Multiplication and division multi-step problems
Level 3 - Mixed multi-step problems
More Arithmetic including lesson Starters, visual aids, investigations and self-marking exercises.
Answers to this exercise are available lower down this page when you are logged in to your Transum account. If you don’t yet have a Transum subscription one can be very quickly set up if you are a teacher, tutor or parent.
Log in Sign up
Curriculum Reference
See the National Curriculum page for links to related online activities and resources.

Child Login
- Kindergarten
- Number charts
- Skip Counting
- Place Value
- Number Lines
- Subtraction
- Multiplication
- Word Problems
- Comparing Numbers
- Ordering Numbers
- Odd and Even
- Prime and Composite
- Roman Numerals
- Ordinal Numbers
- In and Out Boxes
- Number System Conversions
- More Number Sense Worksheets
- Size Comparison
- Measuring Length
- Metric Unit Conversion
- Customary Unit Conversion
- Temperature
- More Measurement Worksheets
- Writing Checks
- Profit and Loss
- Simple Interest
- Compound Interest
- Tally Marks
- Mean, Median, Mode, Range
- Mean Absolute Deviation
- Stem-and-leaf Plot
- Box-and-whisker Plot
- Permutation and Combination
- Probability
- Venn Diagram
- More Statistics Worksheets
- Shapes - 2D
- Shapes - 3D
- Lines, Rays and Line Segments
- Points, Lines and Planes
- Transformation
- Quadrilateral
- Ordered Pairs
- Midpoint Formula
- Distance Formula
- Parallel, Perpendicular and Intersecting Lines
- Scale Factor
- Surface Area
- Pythagorean Theorem
- More Geometry Worksheets
- Converting between Fractions and Decimals
- Significant Figures
- Convert between Fractions, Decimals, and Percents
- Proportions
- Direct and Inverse Variation
- Order of Operations
- Squaring Numbers
- Square Roots
- Scientific Notations
- Speed, Distance, and Time
- Absolute Value
- More Pre-Algebra Worksheets
- Translating Algebraic Phrases
- Evaluating Algebraic Expressions
- Simplifying Algebraic Expressions
- Algebraic Identities
- Quadratic Equations
- Systems of Equations
- Polynomials
- Inequalities
- Sequence and Series
- Complex Numbers
- More Algebra Worksheets
- Trigonometry
- Math Workbooks
- English Language Arts
- Summer Review Packets
- Social Studies
- Holidays and Events
- Worksheets >
- Number Sense >
- Multiplication >
Multiplication Word Problem Worksheets
This page hosts a vast collection of multiplication word problems for 3rd grade, 4th grade, and 5th grade kids, based on real-life scenarios, practical applications, interesting facts, and vibrant themes. Featured here are various word problems ranging from basic single-digit multiplication to two-digit and three-digit multiplication. Another set of printable worksheets hone children's multiplication skill by multiplying large numbers. Free worksheets are included.

Single-digit Multiplication Word Problems
The printable PDF worksheets presented here involve single-digit multiplication word problems. Each worksheet carries five word problems based on day-to-day scenarios.
- Download the set
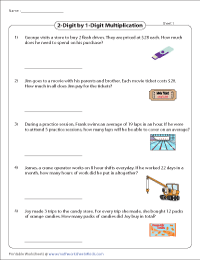
Multiplication Word Problems: Two-digit times Single-digit
The word problems featured here require a grade 3 learner to find the product by multiplying a two-digit number by a single-digit multiplier.

Multiplication Word Problems: Two-digit times Two-digit
The worksheets presented here involve multiplication of two-digit numbers. Read the word problems and find the product. Apply long multiplication (also known as column multiplication) method for easy calculation.

Theme Based Word Problems
Our engaging theme-based pdf worksheets help young minds understand the fundamentals of multiplication. Answer the word problems based on three fascinating themes - Winter Season, Ice rink and Library.

Multiplication Word Problems: Three-digit times Two-digit
Read the word problems featured in these printable worksheets for grade 4 and find the product of three-digit and two-digit numbers. Write down your answers and use the answer key below to check if they are right.

Three-digit Multiplication Word Problems
Solve these well-researched word problems that involve three-digit multiplication. Perform multiplication operation and carry over numbers carefully to find the product.

Multiplication: Three or Four-digit times Single-digit
The word problems featured here are based on practical applications and fact-based situations. Multiply a three or four-digit number by a single-digit multiplier to find the correct product.
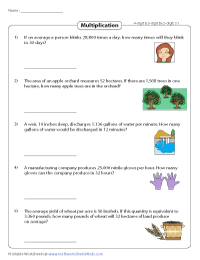
Multi-digit Word Problems: Multiplying Large Numbers
Sharpen your skills by solving these engaging multi-digit word problems for grade 5. Apply long multiplication method to solve the problems. Use the answer key to check your answers.
Related Worksheets
» Addition Word Problems
» Subtraction Word Problems
» Division Word Problems
» Word Problems
» Multiplication
Become a Member
Membership Information
Privacy Policy
What's New?
Printing Help
Testimonial

Copyright © 2024 - Math Worksheets 4 Kids
This is a members-only feature!

- Home |
- About |
- Contact Us |
- Privacy |
- Newsletter |
- Shop |
- 🔍 Search Site
- Easter Color By Number Sheets
- Printable Easter Dot to Dot
- Easter Worksheets for kids
- Kindergarten
- All Generated Sheets
- Place Value Generated Sheets
- Addition Generated Sheets
- Subtraction Generated Sheets
- Multiplication Generated Sheets
- Division Generated Sheets
- Money Generated Sheets
- Negative Numbers Generated Sheets
- Fraction Generated Sheets
- Place Value Zones
- Number Bonds
- Addition & Subtraction
- Times Tables
- Fraction & Percent Zones
- All Calculators
- Fraction Calculators
- Percent calculators
- Area & Volume Calculators
- Age Calculator
- Height Calculator
- Roman Numeral Calculator
- Coloring Pages
- Fun Math Sheets
- Math Puzzles
- Mental Math Sheets
- Online Times Tables
- Online Addition & Subtraction
- Math Grab Packs
- All Math Quizzes
- 1st Grade Quizzes
- 2nd Grade Quizzes
- 3rd Grade Quizzes
- 4th Grade Quizzes
- 5th Grade Quizzes
- 6th Grade Math Quizzes
- Place Value
- Rounding Numbers
- Comparing Numbers
- Number Lines
- Prime Numbers
- Negative Numbers
- Roman Numerals
- Subtraction
- Add & Subtract
- Multiplication
- Fraction Worksheets
- Learning Fractions
- Fraction Printables
- Percent Worksheets & Help
- All Geometry
- 2d Shapes Worksheets
- 3d Shapes Worksheets
- Shape Properties
- Geometry Cheat Sheets
- Printable Shapes
- Coordinates
- Measurement
- Math Conversion
- Statistics Worksheets
- Bar Graph Worksheets
- Venn Diagrams
- All Word Problems
- Finding all possibilities
- Logic Problems
- Ratio Word Problems
- All UK Maths Sheets
- Year 1 Maths Worksheets
- Year 2 Maths Worksheets
- Year 3 Maths Worksheets
- Year 4 Maths Worksheets
- Year 5 Maths Worksheets
- Year 6 Maths Worksheets
- All AU Maths Sheets
- Kindergarten Maths Australia
- Year 1 Maths Australia
- Year 2 Maths Australia
- Year 3 Maths Australia
- Year 4 Maths Australia
- Year 5 Maths Australia
- Meet the Sallies
- Certificates
Multiplication Word Problems 4th Grade
Welcome to our Multiplication Word Problems for 4th Grade. Here you will find our range of printable multiplication problems which will help your child apply and practice their multiplication and times tables skills to solve a range of 'real life' problems at a 4th grade level.
For full functionality of this site it is necessary to enable JavaScript.
Here are the instructions how to enable JavaScript in your web browser .
Quicklinks to ...
- 4th Grade Multiplication Problems Worksheets
- Easier & Harder Multiplication Worksheets
- More related resources
Multiplication Word Problems 4th Grade Online Quiz
Multiplication word problems, 4th grade multiplication problems.
Here you will find a range of problem solving worksheets involving multiplication.
Each sheet involves solving a range of written multiplication problems.
There are 3 levels of difficulty for each worksheet below: A,B and C.
Worksheet A is the easiest level, suitable for children at the beginning of their grade.
Worksheet B is a medium level worksheets for children who are working at the expected level in their grade.
Worksheet C is set at a harder level, suitable for children who are more able mathematicians.
The problems in each worksheet are similar in wording, but the numbers involved become trickier as the level gets harder.
To encourage careful checking and thinking skills, each sheet includes one 'trick' question which is not a multiplication problem. Children need to spot this word problem, and work out which operation they need to solve it.
Using these sheets will help your child to:
- apply their multiplication and times tables skills at a 4th grade level;
- apply their times table knowledge to work out related facts;
- recognise multiplication problems, and try to spot 'trick' problems;
- solve a range of 'real life' problems.
Some of the sheets have a UK version with spelling and currency symbols set for the UK.
4th Grade Multiplication Word Problem Sheets
Series 4 sheet 1 set.
- Series 4 Sheet 1A (easier)
- Series 4 Sheet 1B (medium)
- Series 4 Sheet 1C (hard)

- PDF Series 4.1 (6 sheets)
- PDF Series 4.1 UK version (6 sheets)
Series 4 Sheet 2 Set
- Series 4 Sheet 2A (easier)
- Series 4 Sheet 2B (medium)
- Series 4 Sheet 2C (hard)
- PDF Series 4.2 (6 sheets)
- PDF Series 4.2 UK version (6 sheets)
Series 4 Sheet 3 Set
- Series 4 Sheet 3A (easier)
- Series 4 Sheet 3B (medium)
- Series 4 Sheet 3C (hard)
- PDF version Series 4.3 (6 sheets)
- PDF version Series 4.3 UK version (6 sheets)
Multiplication Word Problems Walkthrough Video
This short video walkthrough shows several problems from our Multiplication Problems Worksheet 4.3A being solved and has been produced by the West Explains Best math channel.
If you would like some support in solving the problems on these sheets, check out the video!
Looking for some easier Multiplication Problems?
In our 3rd Grade Multiplication word problem area, you will find a range of multiplication problems aimed at 3rd graders.
The following areas are covered:
- basic multiplication fact sheets;
- multiplication facts to 10x10;
- 2 digits x 1 digit
- Multiplication Word Problem Worksheets 3rd Grade
Looking for some harder Multiplication Problems?
In our 5th Grade Multiplication word problem area, you will find a range of multiplication problems aimed at 5th graders.
- multiplication fact sheets;
- multiplication related facts to 10x10 e.g. 6 x 70, 8 x 0.6, etc;
- problems needing written multiplication methods to solve e.g. 2 digits x 2 digits, decimal multiplication
- Multiplication Problems Printable 5th Grade
More Recommended Math Worksheets
Take a look at some more of our worksheets similar to these.
Looking for more 4th Grade Word Problems?
Here is our set of 4th grade math problems to help your child with their problem solving skills.
Each problem sheet comes complete with answers, and is available in both standard and metric units where applicable.
Many of the problems are based around 'real-life' problems and data such as the world's heaviest animals.
- apply their addition, subtraction and problem solving skills;
- apply their knowledge of rounding and place value;
- solve a range of 'real life' problems;
- attempt more challenging longer problems.
Using the problems in this section will help your child develop their problem solving and reasoning skills.
- 4th Grade Math Word Problems
Multiplication Times Table Charts
Here you will find a selection of Multiplication Times Table Charts to 10x10 or 12x12 to support your child in learning their multiplication facts.
There is a wide selection of multiplication charts including both color and black and white, smaller charts, filled charts and blank charts.
Using these charts will help your child to:
- Learn their multiplication facts to 10x10 or 12x12;
- Practice their multiplication table.
All the free printable Math charts in this section are informed by the Elementary Math Benchmarks.
- Large Multiplication Chart
- Large Multiplication Charts Times Tables
- Multiplication Times Tables Chart to 10x10
- Times Table Grid to 12x12
- Blank Multiplication Charts to 10x10
- Blank Printable Charts to 12x12
- Multiplication Math Games
Here you will find a range of Free Printable Multiplication Games.
The following games develop the Math skill of multiplying in a fun and motivating way.
- learn their multiplication facts;
- practice and improve their multiplication table recall;
- develop their strategic thinking skills.
Our quizzes have been created using Google Forms.
At the end of the quiz, you will get the chance to see your results by clicking 'See Score'.
This will take you to a new webpage where your results will be shown. You can print a copy of your results from this page, either as a pdf or as a paper copy.
For incorrect responses, we have added some helpful learning points to explain which answer was correct and why.
We do not collect any personal data from our quizzes, except in the 'First Name' and 'Group/Class' fields which are both optional and only used for teachers to identify students within their educational setting.
We also collect the results from the quizzes which we use to help us to develop our resources and give us insight into future resources to create.
For more information on the information we collect, please take a look at our Privacy Policy
We would be grateful for any feedback on our quizzes, please let us know using our Contact Us link, or use the Facebook Comments form at the bottom of the page.
This quick quiz tests your knowledge and skill at solving multiplication word problems by tens and hundreds.
How to Print or Save these sheets 🖶
Need help with printing or saving? Follow these 3 steps to get your worksheets printed perfectly!
- How to Print support
Subscribe to Math Salamanders News
Sign up for our newsletter to get free math support delivered to your inbox each month. Plus, get a seasonal math grab pack included for free!
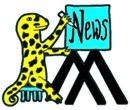
- Newsletter Signup
Return to 4th Grade Math Worksheets Hub
Return to Math Problem Worksheets Hub
Return from Multiplication Word Problems 4th Grade to Math Salamanders Homepage
Math-Salamanders.com
The Math Salamanders hope you enjoy using these free printable Math worksheets and all our other Math games and resources.
We welcome any comments about our site or worksheets on the Facebook comments box at the bottom of every page.
New! Comments
TOP OF PAGE

© 2010-2024 Math Salamanders Limited. All Rights Reserved.
- Privacy Policy
- Copyright Policy
Helping with Math
Multi-Step Math Word Problems
What to expect in this article.
After reading this article, you will be able to analyze, process, and solve multi-step word problems . This lesson will provide help and guidance in solving these types of problems as it includes tips on how to solve a multi-step problem . There are two given examples wherein you can practice and guide your children in honing their mathematical skills. You can also read the common errors and misconceptions of students in solving multi-step problems. Furthermore, this article consists of links directed to worksheets – which you can find at the bottom of the page.
What is a multi-step word problem?
Math word problems are a critical component of the mathematics curriculum because they help students develop their mental abilities , improve logical analysis , and stimulate creative thinking . Word problems are fun and challenging to solve because they represent actual situations that happen in our world. More so, having the ability to solve math word problems significantly benefits one’s career and personal life.
To be able to solve any math word problem , children must be familiar with the mathematics language associated with the mathematical symbols they are accustomed in order to comprehend the word problem.
A multi-step math word problem is a type of problem wherein you need to solve one or more problems first in order to get the necessary information to solve the question being asked. It usually involves multiple operations and may also involve more than one strand of the curriculum. Say, for example, a multi-step word problem involving area and perimeter may also require the application of ratio and multiplication .
How to solve multi-step word problems?
In any word problem, the true challenge is deciding which mathematical operation to use. In solving multi-step word problems, there may be two or more operations that you need to work on, and you must solve them in the correct order to be able to get the correct answer. Since word problems describe a real situation in detail, the question being asked can get lost in all the information, especially in a multi-step problem.
To solve multi-step word problems, you may follow these strategy:
- Analyze and understand the problem.
- Break down each sentence of the problem and identify the clues.
- List all the information.
- Identify the unknown in the problem.
- Devise a plan or identify the mathematical operations you are going to use.
- Carry out the plan.
- Label your final answer.
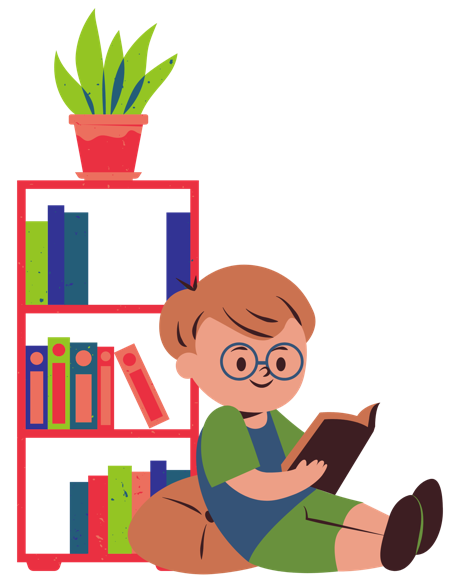
Multi-Step Word Problem #1
Step 1: Break down each sentence of the problem and identify the information needed to solve the problem.
- The first sentence states that “Steven is reading a book that has 260 pages.” Hence, the total number of pages of that particular book is 260 .
- The second statement says, “He read 35 pages on Monday night and 40 pages on Tuesday night.”
Step 2: Analyze the question of the problem and find the keyword for the unknown. The last sentence of the problem, “How many pages does he has left to read?” asks us how many more pages Steven needs to read. Hence, we are going to find the number of pages he still needs to read.
Step 3: Based on the second statement, Steven read 35 pages on a Monday night and 45 pages on a Tuesday night. Hence, we will use addition in getting the total number of pages he read for 2 nights. Thus,
35 + 40 = 75
Therefore, Steven read 75 pages in the span of two days. However, that is not the answer we are looking for.
Step 4: Since we are asked to get the number of pages he still needs to read, the first sentence on our problem shows us that there are 260 pages in the book. Hence, we need to subtract the number of pages Steven has read from the total number of pages of the book. Thus,
260 – 75 = 185
Therefore, Steven has 185 pages left to read.
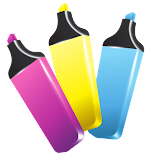
Multi-Step Word Problem #2
Jesy bought a dozen of boxes, each containing 24 highlighter pens inside. Each box costs \$8. Jesy repacked five of these boxes into packages of six highlighters each and sold them for \$3 per package. She sold the rest of the highlighters at the price of three pens for \$2. How much profit did Jesy make?
- The statement, “Jesy bought a dozen of boxes , each containing 24 highlighter pens inside,” tells us that there are a dozen of boxes that contains 24 highlighters. A dozen means there are 12 boxes .
- The second sentence, “Each box costs \$8”, means Jesy bought 12 boxes at \$8 each .
- “Jesy repacked five of these boxes into packages of six highlighters each and sold them for \ $3 per package ” means that Jesy separated 5 boxes from the original 12 boxes to be repacked at a package of six, which was sold at \$3 each.
- “She sold the rest of the highlighters at the price of three pens for \$2 ” means that Jesy sold the remaining highlighters and bundled it for 3 pens for \$2.
Step 2: Analyze the question of the problem and find the keyword. The last sentence of the problem, “How much profit did Jesy make?” asks us how much profit Jesy earned after repacking the highlighter pens. Profit is defined as the amount earned minus the amount spent to buy the highlighters.
Step 3: Based on the first statement, Jesy bought 12 boxes containing 24 highlighters. The follow-up statement that says, “Each box costs \$8” refers to the price of each box. In this particular statement, we can find the total expenditures of Jesy for the highlighter pens by simply multiplying the total number of boxes to \$8. Hence,
12 x \$8 = \$96
This means that Jesy spent \$96 to buy all the highlighters. However, that is not the question being asked. Hence, we need to work on the follow-up statements and find more clues to get Jesy’s profit in selling highlighters.
Step 4: The next statement says that “Jesy repacked five of these boxes into packages of six highlighters each and sold them for \$3 per package” means that Jesy separated 5 boxes from the dozen to be repacked at a package of six, which was sold at \$3 each. Based on this statement, we need to do three things:
- Find the total number of highlighters she got from separating 5 boxes;
- Find the total number of packages she made by repacking it by 6; and
- Find how much money she made by selling the sets of 6 at \$3.
Step 5: To find the total number of highlighters she got from separating 5 boxes, we simply multiply 5 by the number of highlighters inside the box. Based on the first statement, each box contains 24 highlighters. Hence,
5 x 24 = 120
This means Jesy repacked a total of 120 highlighter pens.
Step 6: To find the total number of packages she made by repacking 120 highlighter pens by 6, we will divide 120 by 6. Thus,
So, she was able to make 20 sets of 6 highlighter pens.
Step 7: The next thing we need to do is find how much money she made by selling the sets of 6 by \$3. This can be done by multiplying 20 sets by \$3. Hence,
20 x \$3 = \$60
Thus, Jesy made \$60 from the 20 sets of 6 highlighter pens.
Step 8: The third sentence, “She sold the rest of the highlighters at the price of three pens for \$2” means that Jesy sold the remaining highlighters and bundled it for 3 pens for \$2. From this statement, we need to work on four things first:
- Find the remaining number of boxes;
- Find the total number of highlighter pens she repacked;
- Find the number of sets she repacked by making sets of 3; and
- Find how much money Jesy made by selling packs of 3 at \$2.
Step 9: To find the remaining number of boxes, we need to go through some of the problem statements. Based on the first statement, we have 12 boxes, then 5 boxes were separated to make a highlighter set of 6. Hence, we will subtract 5 from 12.
So, we still have 7 remaining boxes.
Step 10: To find the total number of highlighter pens she repacked, we need to multiply the remaining 7 boxes to the number of highlighter pens inside the box. Going back to the information we already have, we know that there are 24 highlighter pens inside a box. Thus,
7 x 24 = 168
This means Jesy repacked a total of 168 highlighter pens.
Step 11: Find the number of sets Jesy made by repacking 168 highlighter pens by 3. This can be done by dividing 168 by 3. Hence,
Thus, Jesy was able to make 56 sets of 3 highlighter pens.
Step 12: Determine how much money Jesy made by selling each set for \$2. Hence,
56 x \$2 = \$112
This means Jesy made \$112 by selling 3 highlighter pens for \$2.
Step 13: The question asks us to determine the profit Jesy made by selling the highlighter pens. In order to find the profit, we need the information of:
- How much did Jesy spend on the highlighter. In Step 3, we found out that she paid \$96 on buying all the highlighter pens.
- How much money does Jesy make on selling packs of 6 highlighters for \$3. In Step 7, we already know that she made \$60; and
- How much money does Jesy make on selling sets of 3 highlighter pens for \$2. In Step 12, we found out that she made \$112.
Step 14: Before getting the profit Jesy made, we need to know the total money Jesy made in selling the highlighters. Hence, we will simply add the money of \$60 and \$112. Thus,
\$60 + \$112 = \$172
However, \$172 is not the profit Jesy made. This is just the money she was able to make in selling the highlighter pens.
Step 15: Lastly, we will subtract the money Jesy spent on buying the highlighters from the money she made by selling it to find the profit. Thus,
\ $172 – \$96 = \$76
Therefore, Jesy made a profit of \$76 by selling the highlighter pens.
You can tell that there are lots of things to remember with a multi-step word problem, even when the problem itself is relatively easy. But that’s what makes these problems challenging: you get to use both sides of your brain – your logical math skills and your verbal language skills. That’s why they are often more fun to do than problems that are just numbers without the details and context that word problems give you. The better you understand how to solve them, the more fun they are to solve.
What are the common errors in solving multi-step word problems?
Mathematical word problems can be challenging to solve. To obtain the correct answer, children must read the words and carefully analyze the problem, determine the appropriate math operation, and then perform the calculations correctly. An error in working on one of the steps may result in a wrong answer.
Here’s a list of some errors students make when solving multi-step word problems:
- The most common error of students is stopping at one process if they solve one problem. Consider the same word problem about Steven.
“Steven is reading a book that has 260 pages. He read 35 pages on Monday night and 40 pages on Tuesday night. How many pages does he has left to read?”
Most students recognize that they need to add 35 and 40 together to get the total number of pages Steven has read so far. Most errors occur when students stop at one process. Adding 35 + 40 will tell you that Steven has read 75 pages so far, but if we go back to the question you are being asked, you will notice that 75 pages are not the answer you are being asked. Thus, we still need to take another step to get there. Steven has read 75 pages so far, but the questions asked us to solve the number of pages he has left to read. Hence, subtracting 75 from the total number of pages of the book makes much more sense.
- Students get confused with the mathematical operation to use. Even if children are strong readers, they may struggle to pick up on clues in word problems. These clues are phrases that instruct children on how to solve a problem, such as adding or subtracting. The children are then required to convert these phrases into a number sentence in order to solve word problems.
How to teach multi-step problems to children?
There are certain activities or practices that you can try with your child in order to develop their skills in solving multi-step problems.
- The first and most important skill in working with multi-step is being able to understand the problem clearly. Hence, practicing your child in slowly reading and visualizing problems is the first step in implementing our effective reading comprehension strategies.
- Practice your child in recognizing mathematics terms and vocabulary that are used in word problems. There are keywords or clues that we can easily spot in a word problem if we familiarize ourselves with these mathematical terms.
Let’s look at the sample words related to addition, subtraction , multiplication, and division.
However, some English words can sometimes be confusing as they may mean differently depending on the context.
Let’s look at the table below:
Recommended Worksheets
Word Problems Involving Decimals (Market Themed) Math Worksheets Word Problems Involving Measurements (Restaurant Themed) Math Worksheets Word Problems Involving Perimeter and Area of Polygons (Carpentry Themed) Worksheets
Link/Reference Us
We spend a lot of time researching and compiling the information on this site. If you find this useful in your research, please use the tool below to properly link to or reference Helping with Math as the source. We appreciate your support!
<a href="https://helpingwithmath.com/multi-step-math-word-problems/">Multi-Step Math Word Problems</a>
"Multi-Step Math Word Problems". Helping with Math . Accessed on May 21, 2024. https://helpingwithmath.com/multi-step-math-word-problems/.
"Multi-Step Math Word Problems". Helping with Math , https://helpingwithmath.com/multi-step-math-word-problems/. Accessed 21 May, 2024.
Additional Operations of Numbers Theory:
Latest worksheets.
The worksheets below are the mostly recently added to the site.
Comparing Data Using “Less” and “More” 1st Grade Math Worksheets

Representing Data using Table and Charts 1st Grade Math Worksheets

Solving Word Problems involving Addition and Subtraction of numbers within 120 1st Grade Math Worksheets

Understanding Commutative and Associative Property of Addition 1st Grade Math Worksheets

Determining the Unknown Whole Number in an Addition or Subtraction Equation 1st Grade Math Worksheets
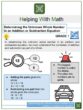
Translation of the Coordinate Plane (School Fair Themed) Math Worksheets
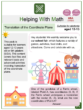
Classifying Shapes by Lines and Angles 4th Grade Math Worksheets
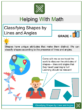
Understanding Number and Shape Patterns 4th Grade Math Worksheets

Prime and Composite Numbers 4th Grade Math Worksheets
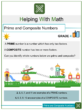
Comparing Multi-digit Numbers 4th Grade Math Worksheets
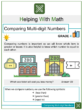
multistep multiplication word problems
All Formats
Resource types, all resource types.
- Rating Count
- Price (Ascending)
- Price (Descending)
- Most Recent
Multistep multiplication word problems
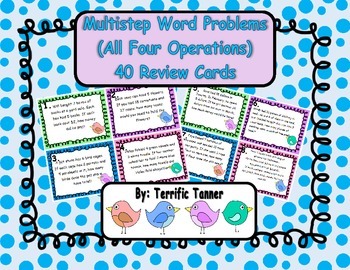
Multistep Word Problem Task Cards - Add., Subtract, Multiplication , and Division
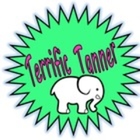
Fraction Word Problems ; Multiply , Divide, Add, Subtract and Multi-Step Fractions
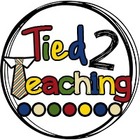
- Google Apps™
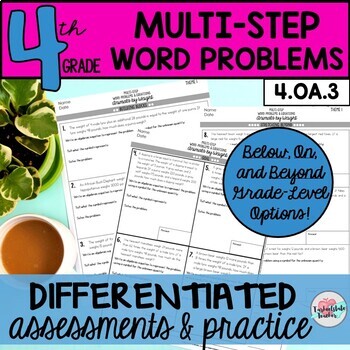
4th Grade Algebraic Thinking Multistep Multiplication Word Problems 4.OA.3
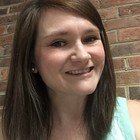
Multiplication & Division Multi-Step Word Problems
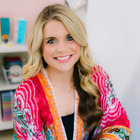
Two Step & Multi-Step Word Problems Add & Subtract, Multiply & Divide 4th Grade

Multiplication and Division Multi-Step Word Problems - Differentiated Task Cards
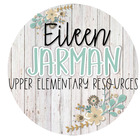
4.OA.3 Task Cards {Set 2}: Multi-Step Word Problems (Add, Subtract, & Multiply )
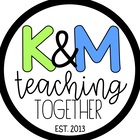
- Easel Activity
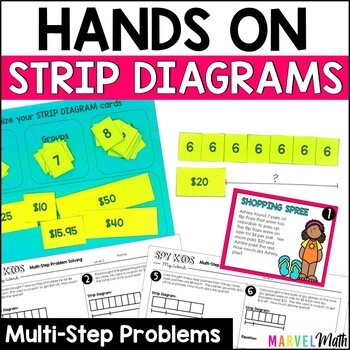
Hands On Strip Diagrams Multi Step Word Problems - Multiplication TEKS 3.5B 3.4K
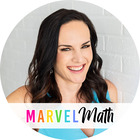
Two Step Multi-Step Word Problems Add Subtract Multiply & Divide 3rd Grade Math
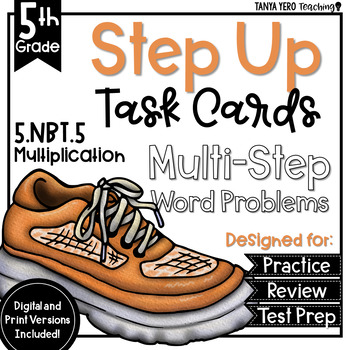
Multiplication Math Multi-Step Word Problems Task Cards 5th Grade 5.NBT.5 GOOGLE
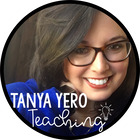
Decimal Word Problems ; Multiply , Divide, Add, Subtract and Multi-Step Decimals
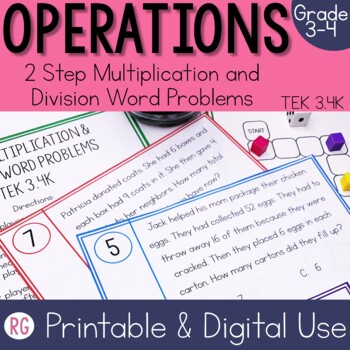
Two Step Word Word Problems Multi Step Word Problems | Multiplication & Division
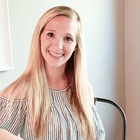
Multiplication Division Multistep Word Problems Task Cards Activities or Centers
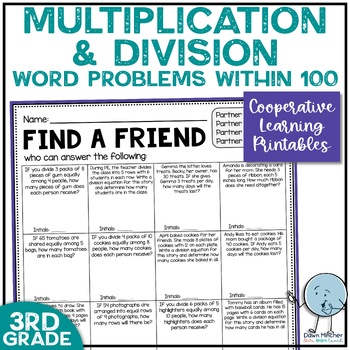
Multistep Word Problems One & Two Step Multiplication & Division 3rd Grade Math
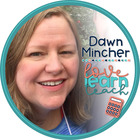
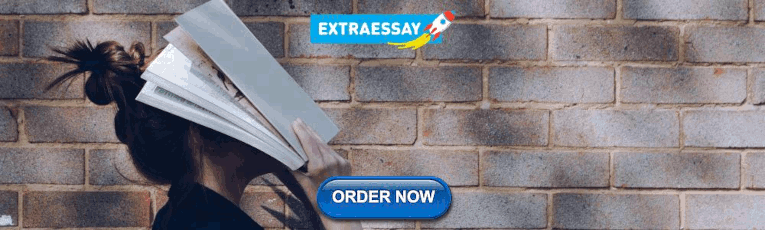
Multi Step Addition, Subtraction, & Multiplication Word Problems 4th Grade
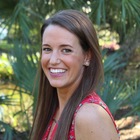
4th Grade Multi Step Word Problems Multiplication Division Task Cards DIGITAL
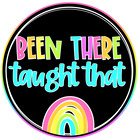
Multistep Multiplication And Division Word Problem , Fall Math Activity 5th Grade
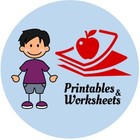
Multistep Multiplication Word Problem Task Cards
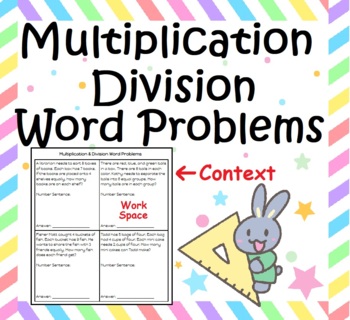
Mixed Multiplication and Division Multistep Word Problems Worksheet Printable
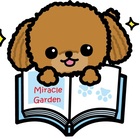
Multiplication And Division Multistep Word Problems Strategies Coloring Sheets
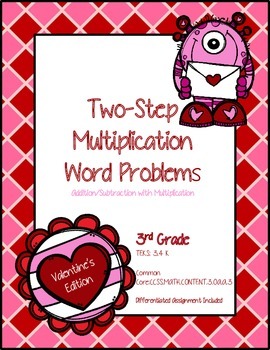
Valentine's Day Multistep Multiplication Word Problems *Freebie*

Multistep Multiplication and Division Word Problems Digital Google Forms
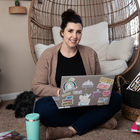
40 Math Multistep Word Problems | Addition Subtraction Multiplication Division
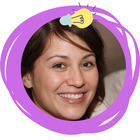
Taylor Swift Multi Step Operation Word Problems Math Multiply Divide Add May Fun
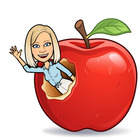
- We're hiring
- Help & FAQ
- Privacy policy
- Student privacy
- Terms of service
- Tell us what you think
Free Printable Multi-Step Word Problems worksheets
Multi-Step Word Problems: Discover a vast collection of free printable math worksheets, crafted by expert educators to help students develop problem-solving skills and master complex mathematical concepts. Ideal for teachers and learners alike!
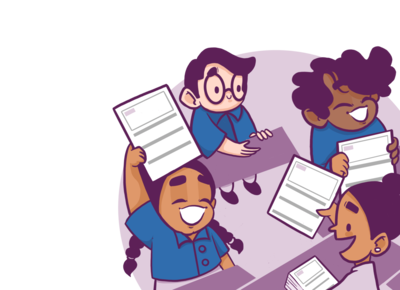
Explore Worksheets by Grade
- kindergarten
Explore Worksheets by Subjects
- Social studies
- Social emotional
- Foreign language
- Reading & Writing
Explore printable Multi-Step Word Problems worksheets
Multi-Step Word Problems worksheets are an essential tool for teachers looking to challenge their students in the realm of Math. These worksheets provide a variety of Math Word Problems that require students to use critical thinking and problem-solving skills to find the solution. By incorporating multiple steps, these worksheets help students develop a deeper understanding of mathematical concepts and improve their ability to apply these concepts in real-world situations. Teachers can use these worksheets to create engaging and interactive lessons, allowing students to work individually or in groups to solve the problems. With a wide range of topics and difficulty levels, Multi-Step Word Problems worksheets cater to the diverse needs of students and can be easily adapted to suit different grade levels.
Quizizz is an excellent platform for teachers to access a vast library of worksheets, including Multi-Step Word Problems worksheets, and other valuable resources for teaching Math. With Quizizz, teachers can create customized quizzes and interactive lessons that incorporate Math Word Problems, allowing students to practice and improve their problem-solving skills. The platform also offers various features such as real-time feedback, progress tracking, and gamification elements, making learning Math more engaging and enjoyable for students. Teachers can easily integrate Quizizz into their lesson plans, ensuring that students receive a comprehensive and well-rounded education in Math. By utilizing Quizizz and its extensive collection of worksheets, teachers can effectively teach Math Word Problems and help their students excel in this crucial subject.
Math Word Problems
Welcome to the math word problems worksheets page at Math-Drills.com! On this page, you will find Math word and story problems worksheets with single- and multi-step solutions on a variety of math topics including addition, multiplication, subtraction, division and other math topics. It is usually a good idea to ensure students already have a strategy or two in place to complete the math operations involved in a particular question. For example, students may need a way to figure out what 7 × 8 is or have previously memorized the answer before you give them a word problem that involves finding the answer to 7 × 8.
There are a number of strategies used in solving math word problems; if you don't have a favorite, try the Math-Drills.com problem-solving strategy:
- Question : Understand what the question is asking. What operation or operations do you need to use to solve this question? Ask for help to understand the question if you can't do it on your own.
- Estimate : Use an estimation strategy, so you can check your answer for reasonableness in the evaluate step. Try underestimating and overestimating, so you know what range the answer is supposed to be in. Be flexible in rounding numbers if it will make your estimate easier.
- Strategize : Choose a strategy to solve the problem. Will you use mental math, manipulatives, or pencil and paper? Use a strategy that works for you. Save the calculator until the evaluate stage.
- Calculate : Use your strategy to solve the problem.
- Evaluate : Compare your answer to your estimate. If you under and overestimated, is the answer in the correct range. If you rounded up or down, does the answer make sense (e.g. is it a little less or a little more than the estimate). Also check with a calculator.
Most Popular Math Word Problems this Week
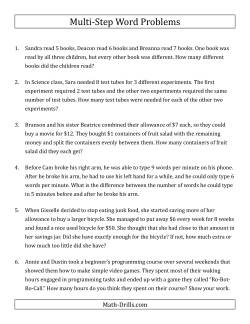
Arithmetic Word Problems
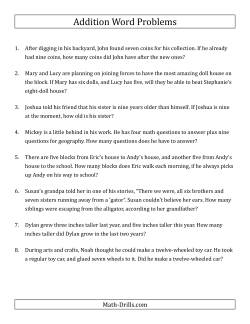
- Addition Word Problems One-Step Addition Word Problems Using Single-Digit Numbers One-Step Addition Word Problems Using Two-Digit Numbers
- Subtraction Word Problems Subtraction Facts Word Problems With Differences from 5 to 12
- Multiplication Word Problems One-Step Multiplication Word Problems up to 10 × 10
- Division Word Problems Division Facts Word Problems with Quotients from 5 to 12
- Multi-Step Word Problems Easy Multi-Step Word Problems
Copyright © 2005-2024 Math-Drills.com You may use the math worksheets on this website according to our Terms of Use to help students learn math.
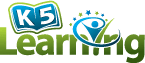
Reading & Math for K-5
- Kindergarten
- Learning numbers
- Comparing numbers
- Place Value
- Roman numerals
- Subtraction
- Multiplication
- Order of operations
- Drills & practice
- Measurement
- Factoring & prime factors
- Proportions
- Shape & geometry
- Data & graphing
- Word problems
- Children's stories
- Leveled Stories
- Context clues
- Cause & effect
- Compare & contrast
- Fact vs. fiction
- Fact vs. opinion
- Main idea & details
- Story elements
- Conclusions & inferences
- Sounds & phonics
- Words & vocabulary
- Reading comprehension
- Early writing
- Numbers & counting
- Simple math
- Social skills
- Other activities
- Dolch sight words
- Fry sight words
- Multiple meaning words
- Prefixes & suffixes
- Vocabulary cards
- Other parts of speech
- Punctuation
- Capitalization
- Narrative writing
- Opinion writing
- Informative writing
- Cursive alphabet
- Cursive letters
- Cursive letter joins
- Cursive words
- Cursive sentences
- Cursive passages
- Grammar & Writing
Breadcrumbs
- Word Problems
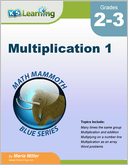
Download & Print Only $5.60
Multiplication word problems for grade 3
Simple multiplication.
These worksheets contain simple multiplication word problems. Students derive a multiplication equation from the word problem, solve the equation by mental multiplication and express the answer in appropriate units. Students should understand the meaning of multiplication before attempting these worksheets.

These worksheets are available to members only.
Join K5 to save time, skip ads and access more content. Learn More
More word problem worksheets
Explore all of our math word problem worksheets , from kindergarten through grade 5.
What is K5?
K5 Learning offers free worksheets , flashcards and inexpensive workbooks for kids in kindergarten to grade 5. Become a member to access additional content and skip ads.
Our members helped us give away millions of worksheets last year.
We provide free educational materials to parents and teachers in over 100 countries. If you can, please consider purchasing a membership ($24/year) to support our efforts.
Members skip ads and access exclusive features.
Learn about member benefits
This content is available to members only.
- Forgot Password?
EdSource is committed to bringing you the latest in education news.
But we can’t do this without readers like you.
Will you join our spring campaign as one of 50 new monthly supporters before May 22?
Student journalists on the frontlines of protest coverage
How can California teach more adults to read in English?
Hundreds of teachers in limbo after spike in pink slips
How earning a college degree put four California men on a path from prison to new lives | Documentary
Patrick Acuña’s journey from prison to UC Irvine | Video
Family reunited after four years separated by Trump-era immigration policy

Black teachers: How to recruit them and make them stay

Lessons in higher education: California and beyond

Keeping California public university options open
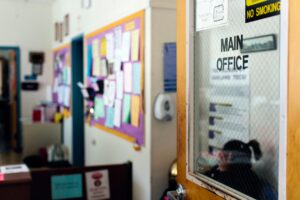
Superintendents: Well-paid and walking away
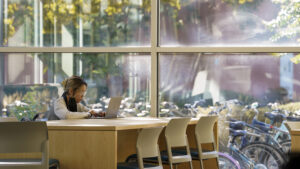
The debt to degree connection

College in prison: How earning a degree can lead to a new life

May 14, 2024
Getting California kids to read: What will it take?

April 24, 2024
Is dual admission a solution to California’s broken transfer system?

To teach math effectively, California must focus on deep, conceptual learning
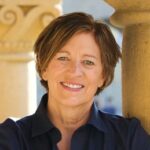
Cole Sampson
May 20, 2024.

EdSource’s journalism is always free for everyone — because we believe an informed public is necessary for a more equitable future for every student. Join our spring campaign as one of 50 new monthly supporters before May 22.
Fierce wars continue to rage around math instruction, but there are many practical changes we should make for mathematics students upon which most of us can probably agree, that could transform their ability to achieve.
A promising new initiative for California that we have both been involved with tackles two of the most pressing flaws of traditional math instruction with elegant solutions that should be appealing to many, no matter which camp they occupy in the debates. Ask any teacher of math what they wish they did not have to deal with, and they will tell you the excessive amount of content they need to teach, which leads to the second problem — the shallow coverage of hundreds of methods that students do not learn in meaningful ways.
U.S. math textbooks are massive and heavy tomes. By contrast, math textbooks in Japan and China are small and slim. The reason for this is that U.S. curriculum repeats content every year. In China and Japan, content is taught less frequently but more deeply and conceptually . As teachers in the U.S. are forced to “cover” an extensive amount of content in every year of school, students only gain a shallow experience of mathematical methods and rules.
The second problem, linked to the first, is that students are taught hundreds of methods as though they are all equally important, without experiencing the more foundational concepts deeply and conceptually. Some concepts are much more central than others because they link to other areas of content, and they deserve to be learned deeply, over multiple lessons, through applied tasks that relate to students’ lives. An example of a central concept in grade four is “factors and groups.” Instead of learning about these through short questions and answers, students can learn them through rich tasks in which they are more deeply engaged, as can be seen here .
Students can learn all foundational concepts, such as fractions or functions, by drawing, building and learning about them through real-world examples. Every important idea in mathematics can be learned visually, physically and conceptually, including algebra and calculus . Instead, most students work through pages of numerical calculations, absent of any connection to the world, and spend hours of algebra class manipulating X’s on a page.
A solution to both of these problems is to teach the “big ideas in mathematics” for every grade, as set out in the California Mathematics Framework , such as “being flexible within 10” (kindergarten) or “unit rates in the world” (grade seven), making sure that for each of the eight or so big ideas in every grade, students have a deep and rich experience of their underlying concepts: by drawing them, building them and talking about them. Even if it is only these eight or so ideas that are experienced in this way each year, they will serve as a foundation for everything else students learn as they progress.
Many California school districts are now waiting for funding to be devoted to the training of teachers to move to the approaches set out in the framework. But in Kern County, leaders have been sharing these ideas for the past three years. Semitropic Elementary school, which serves mainly Latinx, English learners and socioeconomically disadvantaged students, is one example of a school that has moved to the approach of the framework. In the 2018-19 school year, before Covid-19 and the implementation of the new framework, only 5.6% of Semitropic students met or exceeded standards on math Smarter Balanced tests in grades 3-8, with less than 5% in grades four and five, and no students in grades 6 or 8. After the leaders in Kern County supported teachers in learning and implementing the ideas of the framework, through a series of professional development sessions to build capacity, with classroom demonstration lessons to model the new strategies, in action with their students, and coaching to meet teachers where they were, proficiency levels shot up, increasing to 16.3% overall, with the fourth grade showing the most significant increase , to 36.8%. There is more work to be done in this and other districts, but the demonstrable positive changes already unfolding are impressive.
What changed in the classrooms of the schools in Kern County? The teachers focused on big ideas, such as “being flexible within 10” which starts in kindergarten and extends through the elementary grades. Instead of students learning 10 as a fixed number that they use to calculate, they now spend time learning how 10 is made up, and all the ways they can make 10. A powerful strategy teachers started to use was “ number talks ,” in which teachers pose a number problem and collect the different ways students approach the problem, representing them visually. They also started using richer, deeper tasks, encouraging students to discuss ideas and learn with visuals and manipulatives. The superintendent and county math coaches were thrilled with the high levels of engagement they saw in the classrooms, as well as the significant changes in state test scores.
There are several problems with the systems of mathematics education in many states, and proposed solutions often spark disagreement . But perhaps we should all agree on one thing: Students need to learn important mathematical concepts deeply and well. They should not be working through sets of procedural questions that mean nothing to them, but rather should experience rich applied mathematics that inspires them, helps them learn effectively, and shows them that mathematics is important to their lives.
Jo Boaler is a Stanford professor and author of “Math-ish: Finding Creativity, Diversity & Meaning in Mathematics .” She was one of the writers of California’s new mathematics framework .
Cole Sampson is the administrator of professional learning for the Kern County Superintendent of Schools Office .
The opinions in this commentary are those of the authors. If you would like to submit a commentary, please review our guidelines and contact us .
Share Article
Comments (9)
Leave a comment, your email address will not be published. required fields are marked * *.
Click here to cancel reply.
XHTML: You can use these tags: <a href="" title=""> <abbr title=""> <acronym title=""> <b> <blockquote cite=""> <cite> <code> <del datetime=""> <em> <i> <q cite=""> <s> <strike> <strong>
Comments Policy
We welcome your comments. All comments are moderated for civility, relevance and other considerations. Click here for EdSource's Comments Policy .
A. Nelson 6 hours ago 6 hours ago
Dr. Boaler and Dr. Simpson write: "In China and Japan, content is taught less frequently but more deeply and conceptually." With respect, this is simply not the case, or at most, a half-truth. In both China and Japan, students are almost always taught using explicit (direct) instruction first, are required to memorize their multiplication facts by their equivalent of grade 3, in both Arab-Indian numerals and traditional Chinese numbers or Japanese Kanji script, and they practice math … Read More
Dr. Boaler and Dr. Simpson write:
“In China and Japan, content is taught less frequently but more deeply and conceptually.”
With respect, this is simply not the case, or at most, a half-truth. In both China and Japan, students are almost always taught using explicit (direct) instruction first, are required to memorize their multiplication facts by their equivalent of grade 3, in both Arab-Indian numerals and traditional Chinese numbers or Japanese Kanji script, and they practice math skills – boy do they practice – often in addition to their normal school day in after-school programs.
While Chinese and Japanese students might be taught concepts – the ideas behind the algorithms – they are doing so after having stored a significant amount of math facts in their long-term memories. They have knowledge to work with when they “discover” or “inquire” about new problems.
It is important to stress that the actual way Chinese and Japanese students are taught is antithetical to how Dr. Boaler (et. al.) approach math learning. Chinese and Japanese students memorize times tables, they are explicitly taught, and they practice (for hours), in addition to conceptual work. Dr. Boaler (et. al.) are against memorizing times tables, explicit teaching, and a lot of practice. That’s what they tried to push on to SFUSD, with the result of math scores steadily declining over ten years.
EdSource, again with respect, you are an important source of information for teachers, administrators, and parents. Please, if you can, try to fact check the citations and links provided by academic education professors. You may discover some uncomfortable truths.
Simon P 9 hours ago 9 hours ago
We've experienced several years of the "deep, conceptual" approach in our schools. The results are saddening. One example is where our early elementary students are being taught that multiplication is merely repeated, serial addition. This pedagogical system, shared by Singapore math, when Implemented properly, it's not altogether bad. However, students must quickly un-learn this concept if they're going to grasp ratio, proportion, or anything other than positive integers. Rather than conceptualizing multiplication as scaling and … Read More
We’ve experienced several years of the “deep, conceptual” approach in our schools. The results are saddening.
One example is where our early elementary students are being taught that multiplication is merely repeated, serial addition. This pedagogical system, shared by Singapore math, when Implemented properly, it’s not altogether bad. However, students must quickly un-learn this concept if they’re going to grasp ratio, proportion, or anything other than positive integers. Rather than conceptualizing multiplication as scaling and identity, our 4th and 5th grade students are still fumbling around with tape and block diagrams. Fast forward to 9th and 10th grade, when we ask students to factorize a quadratic equation, and we’ve lost them.
Fact fluency/arithmetic automaticity has been so demonized in early elementary schools that a multiplication table can’t be found in a classroom – flashcards, smuggled in a backpack might be treated as contraband. We’ve robbed our kids of the simple joy of memorizing things.
Transmitting and acquiring maths competency follows many other developmental processes – particular problems are solved while gently abstractifying further problems into increasingly general (often more complex) cases.
Current “deep conceptual” learning implementations have failed miserably, leaving students to un-learn such concepts at best, or worse, hobbling them for higher level cognitive tasks.
Steve 12 hours ago 12 hours ago
Ironically you speak about deep and conceptual learning but you make no effort to describe what you propose in anything but the most shallow terms. As a child of poverty in a school serving mostly similar families, I had teachers who were able to use storytelling to illustrate concepts very well. This might be what you're trying to describe; however they, possibly unlike you, had years of practical application to draw on, not merely academics. … Read More
Ironically you speak about deep and conceptual learning but you make no effort to describe what you propose in anything but the most shallow terms. As a child of poverty in a school serving mostly similar families, I had teachers who were able to use storytelling to illustrate concepts very well. This might be what you’re trying to describe; however they, possibly unlike you, had years of practical application to draw on, not merely academics.
In addition, while your concepts are not unappealing on the surface, they need nuance. For example, flexibility within 10 as a concept can be taught within a few days but then memorization is still needed, and certainly doesn’t need more than one grade level. The same applies to the multiplication tables. The instant readiness of these basics in our minds remain the foundation for every next level.
Why can’t there be a nuanced approach? Yes, select conceptual methodologies to focus on, but don’t throw out the baby with the bathwater. Provide hands-on, visual, or multimedia workshops to get the concepts, but also require the memory work. And don’t take years to do it, either.
The best teachers have always intuitively used adaptations of what you seem to be proposing, but like so many so-called progressive ideas these days, you’re trying to make drastic changes instead of incremental improvements that build on realizations. You might argue that making small changes every year would be tantamount to experimenting on children, but massive change and discarding of current models is much more tenuous.
The problem is the academics get hold of an idea and spend years designing its implementation in a vacuum, rather than getting it into classrooms in a small way and adjusting it on the fly. This is what students will face in the real world, if they have to work and meet their responsibilities, and they are coming out singularly unprepared.
Brian 15 hours ago 15 hours ago
Oddly enough, the students in China, India, South Korea, Taiwan and Singapore master math (and by extension, STEM) without the convoluted nonsense underlying this mathematic framework. Further, the SFUSD has already shown us what an unmitigated disaster this is. This type of approach to math will ensure one, and only one, outcome: The CA public school system will churn out a generation of poorly qualified individuals who will get displaced by H1-B visa holders in the … Read More
Oddly enough, the students in China, India, South Korea, Taiwan and Singapore master math (and by extension, STEM) without the convoluted nonsense underlying this mathematic framework. Further, the SFUSD has already shown us what an unmitigated disaster this is.
This type of approach to math will ensure one, and only one, outcome: The CA public school system will churn out a generation of poorly qualified individuals who will get displaced by H1-B visa holders in the workforce because they will be utterly unemployable in STEM fields. Given the foundational nature of math to other skills such as reasoning, judgement & decision-making, this detrimental effect will likely generalize to fields other than STEM as well.
We already have the immigrant communities here in our midst in California already. They come from backgrounds with a strong foundation in math. Seek wisdom from them in shaping our math curriculum and jettison the well-intentioned but ultimately clueless leftist approach to mucking up the education system. While individuals like Jo Boaler experiment with our students education as part of her academic pursuits, nations like China and India will eat our lunch.
Phillip 19 hours ago 19 hours ago
I'm tired of experimenting on children. These foolish plans and frameworks do nothing but bring down the success of students. It's absurd that we could even call this experimentation, under normal circumstances, after seeing poor results or a lowering of performance from a change, you would revert back to the previous method that was working. Not embark on endless change with progressively worse results. What was the curriculum when students were … Read More
I’m tired of experimenting on children. These foolish plans and frameworks do nothing but bring down the success of students. It’s absurd that we could even call this experimentation, under normal circumstances, after seeing poor results or a lowering of performance from a change, you would revert back to the previous method that was working. Not embark on endless change with progressively worse results. What was the curriculum when students were at their most successful? Start there.
A. Nelson 4 hours ago 4 hours ago
Thank you. SFUSD, under the guidance of Dr. Boaler (et. al.) did just what you wrote. In 2014, they initiated an experiment on 50,000 students - with no controls for comparison. What followed was the greatest decline in SFUSD's standardized math test scores since we started collecting that data in the late 1970s. See the links provided by the commenter named Maya K. This curricular change was irresponsible, unethical, detrimental to student math learning, and … Read More
Thank you. SFUSD, under the guidance of Dr. Boaler (et. al.) did just what you wrote. In 2014, they initiated an experiment on 50,000 students – with no controls for comparison. What followed was the greatest decline in SFUSD’s standardized math test scores since we started collecting that data in the late 1970s. See the links provided by the commenter named Maya K.
This curricular change was irresponsible, unethical, detrimental to student math learning, and against basic academic principles. Why would SFUSD agree to such a radical change without proper structure and controls? It boggles the mind.
JudiAU 21 hours ago 21 hours ago
Our charter school used Everday Math and the elementary schools combined reported a 65% Pass rate on the CASSP. But the small campus scored twenty points higher for 3rd and 4th grades year after year. And when they moved to the 5th grade those kids stayed ahead. Those teacher taught the curriculum assigned by also assigned traditional warm up work, extra weekly homework, and absolutely made sure the kids memorized their times tables. … Read More
Our charter school used Everday Math and the elementary schools combined reported a 65% Pass rate on the CASSP. But the small campus scored twenty points higher for 3rd and 4th grades year after year. And when they moved to the 5th grade those kids stayed ahead. Those teacher taught the curriculum assigned by also assigned traditional warm up work, extra weekly homework, and absolutely made sure the kids memorized their times tables. Those kids were all more successful in middle school too.
The kids don’t need any more ducky arrays.
Barry Garelick 22 hours ago 22 hours ago
"Students need to learn important mathematical concepts deeply and well." Which concepts do you feel that students are not being taught? And what do you mean by "deep conceptual learning"? If a student is taught how a fractional division problem is similar to a whole number division problem, wouldn't the main concept of "division" suffice? Wouldn't the context of the problem dictate what the concept is? Do you need the student to draw a picture … Read More
“Students need to learn important mathematical concepts deeply and well.” Which concepts do you feel that students are not being taught? And what do you mean by “deep conceptual learning”? If a student is taught how a fractional division problem is similar to a whole number division problem, wouldn’t the main concept of “division” suffice? Wouldn’t the context of the problem dictate what the concept is? Do you need the student to draw a picture each time, or use a convoluted strategy to demonstrate that they “understand” the concept?
“They should not be working through sets of procedural questions that mean nothing to them, but rather should experience rich applied mathematics that inspires them, helps them learn effectively, and shows them that mathematics is important to their lives.”
If they can see what procedures are needed to solve a problem, why do you say that it means nothing to them? If students can see what is needed to solve a problem – like multi-step problems that incorporate multiple concepts and procedures – they really don’t care if the problem is “relevant” to them. They like to be able to be successful.
As far as algebraic manipulations and algorithmic reasoning, there are important reasons that what you think students find “trivial” and “irrelevant” should be mastered. If you are so intent on students being able to “think like mathematicians,” why do you prevent them from learning and using the tools they need to analyze and solve problems?
See: https://barrygarelick.substack.com/p/why-algorithmic-reasoning-is-a-form
Maya K 1 day ago 1 day ago
A similar pre K to 11 curriculum was implemented in San Francisco Unified School District for ten years with disastrous results. I realize the authors are disavowing all knowledge however contemporaneous articles at the time say this: https://www.edweek.org/teaching-learning/a-bold-effort-to-end-algebra-tracking-shows-promise/2018/06 " Heavily based on work by Jo Boaler, a Stanford University professor of math education, the curriculum emphasizes having groups of students work through a series of ambitious math tasks." The SFUSD core curriculum is here: "The SFUSD Math … Read More
A similar pre K to 11 curriculum was implemented in San Francisco Unified School District for ten years with disastrous results.
I realize the authors are disavowing all knowledge however contemporaneous articles at the time say this: https://www.edweek.org/teaching-learning/a-bold-effort-to-end-algebra-tracking-shows-promise/2018/06 ” Heavily based on work by Jo Boaler, a Stanford University professor of math education, the curriculum emphasizes having groups of students work through a series of ambitious math tasks.”
The SFUSD core curriculum is here: “The SFUSD Math Core Curriculum is a Common Core Math Curriculum for students from PK to Grade 11. The curriculum was developed and revised by SFUSD teachers with advice from partners such as Strategic Education Research Partnership (SERP) and the Silicon Valley Mathematics Initiative (SVMI). SFUSD began full implementation of the curriculum in 2014–2015.” https://web.archive.org/web/20240221224936/https://www.sfusd.edu/departments/mathematics-department-page/sfusd-math-core-curriculum
The recent audit results were dismal. Especially for middle school where this curriculum doesn’t meet standards at all. The district is piloting new curriculum to replace it. Audit results: https://drive.google.com/file/d/1EagtuK5d85k6O8IE5_vEvYdMNjWu9t7f/view?pli=1
Finally, reading something like this: “where they were, proficiency levels shot up, increasing to 16.3% overall, with the fourth grade showing the most significant increase, to 36.8%.” reminds me some of these folks claimed that 40% failed Algebra I in SFUSD the last year it was offered in 8th grade. When in reality 4% did.
I think people need to inspect the raw data before taking this at face value. San Francisco should be the warning sign. Fool me once and all.
EdSource Special Reports

Fewer than half of California students are reading by third grade, experts say. Worse still, far fewer Black and Latino students meet that standard. What needs to change?

For the four men whose stories are told in this documentary, just the chance to earn the degree made it possible for them to see themselves living a different life outside of prison.

Amid Israel-Hamas war, colleges draw lines on faculty free speech
The conflict in Gaza has rekindled efforts to control controversy and conversation on campuses. The UC system could be the latest to weigh in.

Dissent, no funding yet for statewide teacher training in math and reading
A bill sponsored by State Superintendent Tony Thurmond would provide math and reading training for all teachers. But money is scarce, and some English language advocates have problems with phonics.
EdSource in your inbox!
Stay ahead of the latest developments on education in California and nationally from early childhood to college and beyond. Sign up for EdSource’s no-cost daily email.
Stay informed with our daily newsletter
Multi-Step Problems Worksheet
Assess your math skills by solving multi-step problems in this worksheet..
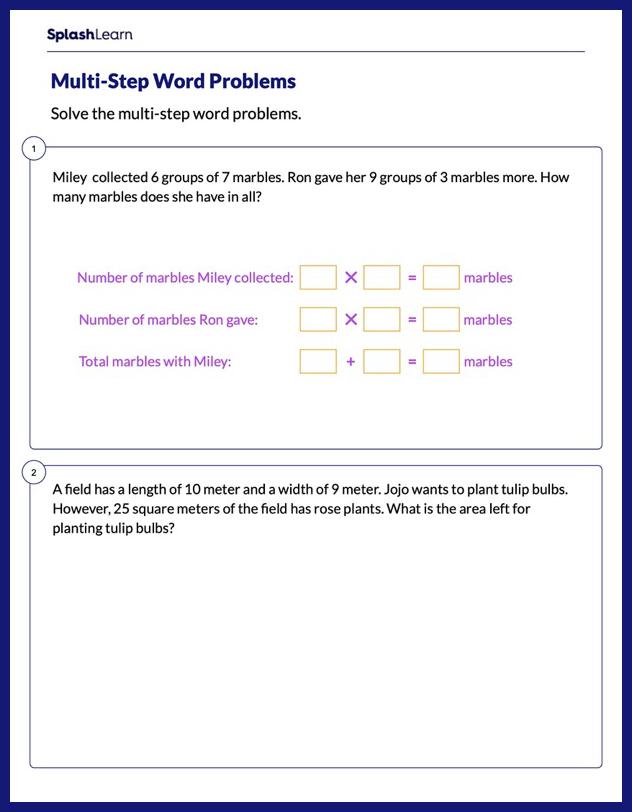
Know more about Multi-Step Problems Worksheet
Help your child become an expert in multiplication with this worksheet. Young learners will make connections between math and the real world as they solve a set of multi-step word problems involving a variety of scenarios. In these problems, they comprehend the scenarios and find the final answer. What better way is there for your young mathematician to learn than to have fun while learning?
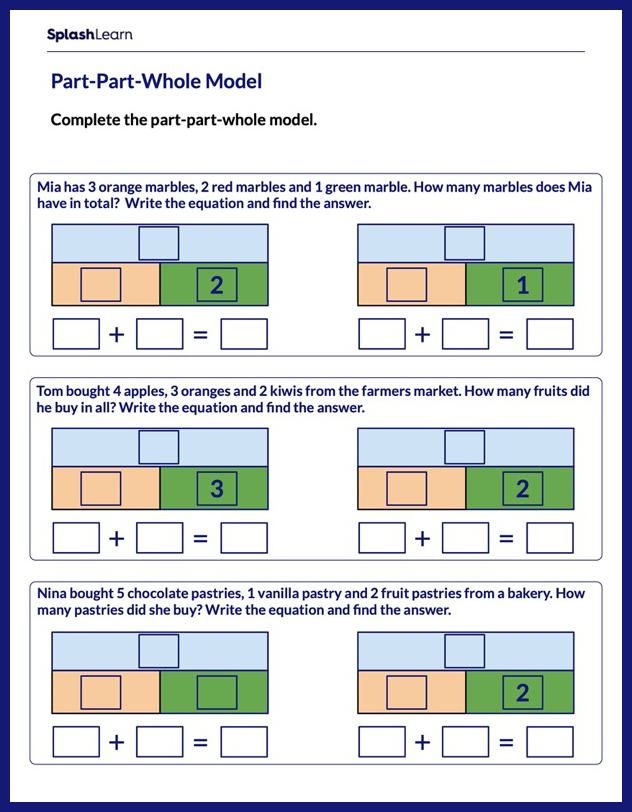
Your one stop solution for all grade learning needs.
Dynamic programming with meta-reinforcement learning: a novel approach for multi-objective optimization
- Original Article
- Open access
- Published: 18 May 2024
- Cite this article
You have full access to this open access article
- Qi Wang ORCID: orcid.org/0000-0003-3249-8459 1 ,
- Chengwei Zhang 1 &
51 Accesses
Explore all metrics
Multi-objective optimization (MOO) endeavors to identify optimal solutions from a finite array of possibilities. In recent years, deep reinforcement learning (RL) has exhibited promise through its well-crafted heuristics in tackling NP-hard combinatorial optimization (CO) problems. Nonetheless, current methodologies grapple with two key challenges: (1) They primarily concentrate on single-objective optimization quandaries, rendering them less adaptable to the more prevalent MOO scenarios encountered in real-world applications. (2) These approaches furnish an approximate solution by imbibing heuristics, lacking a systematic means to enhance or substantiate optimality. Given these challenges, this study introduces an overarching hybrid strategy, dynamic programming with meta-reinforcement learning (DPML), to resolve MOO predicaments. The approach melds meta-learning into an RL framework, addressing multiple subproblems inherent to MOO. Furthermore, the precision of solutions is elevated by endowing exact dynamic programming with the prowess of meta-graph neural networks. Empirical results substantiate the supremacy of our methodology over previous RL and heuristics approaches, bridging the chasm between theoretical underpinnings and real-world applicability within this domain.
Similar content being viewed by others
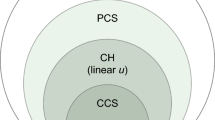
A practical guide to multi-objective reinforcement learning and planning
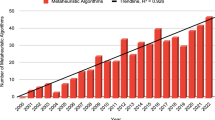
An exhaustive review of the metaheuristic algorithms for search and optimization: taxonomy, applications, and open challenges
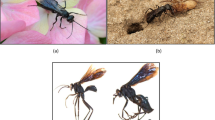
Spider wasp optimizer: a novel meta-heuristic optimization algorithm
Avoid common mistakes on your manuscript.
Introduction
Multi-objective optimization (MOO) problems [ 1 , 2 ] are common in the real world, where the optimization of two or more objectives simultaneously is required. These problems often arise in various domains, such as engineering, finance, and logistics, where decision-makers must balance competing objectives [ 3 , 4 ]. A MOO problem [ 5 ] can be formulated as follows, without any loss of generality:
where \(f\left(x\right)\) consists of \(m\) multiple objective functions, and \(x\subseteq {R}^{n}\) is the decision space. Because these \(m\) objectives are frequently in conflict, a collection of trade-off solutions known as Pareto optimal solutions is searched for MOO. For two purposes, \(u\) is shown to dominate \(v\) if and only if \({u}_{i}\le {v}_{i}\) for every \(i\in \{1, 2,\dots , m\}\) and \({u}_{j}\le {v}_{j}\) for at least one \(j\in \{1, 2,\dots , m\}\) . The solution is called a Pareto optimal solution when there is no solution \(x\) where \(f\left(x\right)\) dominates \(f\left({x}^{*}\right)\) . All of the Pareto optimal solutions construct a Pareto set. A Pareto front is formed by the appropriate objective vectors \(\{f\left({x}^{*}\right)|{x}^{*}\in \mathrm{Pareto set}\}\) .
Routing problems like multi-objective vehicle routing problems (MOVRPs) [ 6 ] and traveling salesman problems (MOTSPs) [ 7 ] are typical MOO problems. Finding all of the precise Pareto optimum solutions to MOO problem-solving is quite tricky. For many issues, it can be NP-hard to discover a single Pareto optimum solution [ 1 ], and the number of Pareto solutions may scale exponentially with the complexity of the problem [ 2 ]. It is frequently impossible to predict the decision-maker’s preference among several objectives, which makes it exceedingly challenging to simplify the problem into a single purpose. Several approaches have been developed to approximate Pareto sets in an acceptable amount of computing time for various MOO situations. For each challenge, these techniques frequently need carefully developed, customized heuristics. It may be highly labor-intensive, such as multi-objective evolutionary algorithms NSGA-II [ 8 ] and MOEA/D [ 9 ]. As the problem statement evolves, the traditional approaches typically necessitate a fresh search or adaptation of heuristic guidelines through iterative trial and error. However, it is worth noting that a substantial portion of CO predicaments encountered in analogous contexts exhibit akin intrinsic structures [ 10 ]. Also, the computational effort and complexity of this class of algorithms increase significantly with the size of the problems. Evolutionary methods can search for feasible solutions, but many iterations also significantly increase the computation time with the problem size [ 11 , 12 ].
Combinatorial optimization (CO) aims to select the best choice variables in a discrete decision space, aligning with RL’s fundamental sequential decision-making property [ 10 , 13 ]. Deep reinforcement Learning (RL), which enables both offline training and online inference of CO policies, is considered a promising approach for addressing CO problems [ 10 , 11 ]. However, most current learning-based methods focus on single-objective optimization problems, while real-world applications often involve MOO [ 14 ]. In the context of MOO, the effectiveness of existing methods heavily relies on RL’s comprehension and refinement of the weight vector. When RL encounters an unfamiliar weight vector, it may require training a new model or adapting an existing one to handle the relevant sub-problems. This necessity arises from the fact that the training and testing datasets need to adhere to similar distributions [ 13 , 14 , 15 , 16 ].
To overcome these limitations, there is a growing need for novel techniques that can successfully apply RL to the challenging domain of MOO. By developing innovative algorithms and strategies, we can enhance RL’s ability to handle diverse and complex objective functions, enabling more efficient and effective optimization in real-world scenarios [ 12 , 16 ]. These advancements not only expand the applicability of RL in MOO but also facilitate more comprehensive and reliable decision-making processes [ 11 ].
One promising avenue to address these challenges is integrating dynamic programming-enhanced meta-reinforcement learning. By leveraging the power of dynamic programming [ 2 ], we can effectively capture the inherent structure of CO problems and facilitate more efficient adaptation to shifting problem formulations. This integration expedites the adjustment process and establishes a more versatile problem-solving framework. Furthermore, the essence of meta-reinforcement learning enhances this paradigm by enabling the approach to learn and generalize from past experiences. This empowers it to swiftly adapt to novel instances of MOO, thereby reducing the overhead associated with heuristic reconfiguration [ 17 ].
Our approach to tackling MOO challenges revolves around meta-learning, which enables our model to dynamically adjust parameters and acquire new tasks based on prior knowledge. The core component of our approach is the meta-value network, which guides various policies in handling a multitude of potential sub-problems. In our framework (depicted in Fig. 1 ), the meta-value network is trained to adapt quickly to different sub-problems through a few fine-tuning steps. This allows our model to efficiently leverage prior knowledge and rapidly adjust its parameters to address specific instances of MOO. By integrating exact dynamic programming within the meta-value network, we aim to elevate the solution quality and tackle the challenges MOO poses.
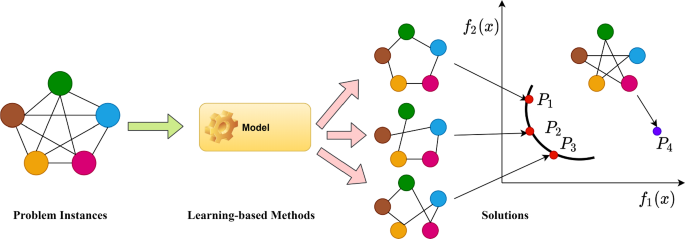
An example of introducing machine learning into MOO. MOTSP instances are optimized to generate different solutions; among them, \({P}_{1}\) , \({P}_{2}\) , \({P}_{3}\) are different optimal trade-offs between the two objectives \({f}_{1}\left(x\right)\) and \({f}_{2}\left(x\right)\) , and \({P}_{4}\) is a poor solution that should be avoided
Integrating exact dynamic programming within the meta-value network is a crucial aspect of our approach. Dynamic programming, known for its divide-and-conquer principles, allows us to decompose complex MOO problems into smaller sub-problems. By harnessing the power of dynamic programming, we can efficiently explore and exploit the decision space, optimizing the solution quality. To leverage the capabilities of neural networks, we approximate and enhance the dynamic programming principles within the meta-value network. The neural network component of our approach enables us to capture and generalize the inherent structure of the MOO problems, enhancing the adaptability and efficiency of the algorithm. By synthesizing the core principles of dynamic programming with the meta-learning paradigm, we create a robust methodology for addressing MOO challenges. The meta-learning component facilitates the rapid acquisition of new tasks and the adjustment of parameters while integrating dynamic programming principles that enhance the solution’s quality and efficiency.
Our comprehensive approach seamlessly combines the strengths of meta-learning, dynamic programming, and neural networks into a cohesive algorithm. At its core, the meta-value network serves as a guiding beacon, leveraging prior knowledge and dynamically adjusting parameters to adeptly handle the diverse sub-problems inherent in the MOO domain. This integration of dynamic programming principles within the neural network architecture elevates solution quality, enabling efficient exploration and exploitation of the decision space. By approximating and augmenting dynamic programming with neural networks, we forge a holistic methodology that unites the fundamental tenets of both paradigms, culminating in a robust and versatile solution for MOO challenges. Experimental results underscore its proficiency in enhancing solution quality, computation speed, and adaptability to a wide array of objective functions.
In summary, this paper makes the following contributions:
We incorporate meta-learning into the meta-critic network within the actor-critic framework. This allows us to learn various policies that tackle realistic MOO problems or tasks. The meta-learning component can serve as an adaptive plug-in that can be applied to reinforcement learning and supervised learning, enhancing their performance.
We propose a novel meta-attention network approximating the dynamic programming meta-value network to attain more precise policies. This meta-attention network balances accuracy, complexity, and runtime, enabling us to learn more exact policies that align with the given optimization tasks.
We design a Transformer-based policy network to address the challenges posed by realistic and complex routing optimization problems. This network is specifically tailored to handle such problems, providing contextual information during the encoder-decoder processing to aid in meta-learning, specifically the task-actor encoder.
The remaining sections of this paper are organized as follows: In Sect. “ Related work ”, we provide an introduction to multi-objective optimization, learning-based construction heuristic methods, learning-based improvement heuristic methods, dynamic programming, and the differences between the proposed method and existing methods. Sect. “ Preliminaries and overview ” presents the problem definition, the Markov decision process based on dynamic programming, and meta-learning background. Sect. “ Methodology ” provides a detailed description of the proposed method and its components, including the architecture of the policy network, the meta-learning optimization framework, and the overall optimization algorithm with its complexity analysis. In Sect. “ Evaluation ”, we validate the efficiency and effectiveness of the proposed method on multiple datasets and different tasks.
Related work
Multi-objective optimization.
The realm of MOO has garnered increasing attention from various research communities for several decades [ 2 ]. Researchers have predominantly pursued two principal avenues in addressing MOO challenges: exact methodologies and approximation strategies. The landscape of MOO often entails NP-hard problems with extensive dimensions, rendering exact techniques impractically resource-intensive. Consequently, a plethora of heuristics and approximation methods [ 8 , 9 ] have emerged to navigate this complexity, striving to yield a judicious number of Pareto-optimal approximations within reasonable computational constraints [ 18 ]. The intricacies of NP-hard MOO problems have propelled the development of heuristics and approximation methodologies. While exact approaches prove arduous due to computational infeasibility, these heuristics strive to balance solution quality and computational efficiency. They endeavor to unearth an array of approximated Pareto solutions that provide valuable insights into the trade-offs between competing objectives. Nevertheless, these heuristic paradigms often necessitate meticulous handcrafting tailored to each unique problem instance. This requirement for painstaking design constitutes a notable hurdle, particularly when transitioning to real-world scenarios where the intricacies of the problem are further compounded.
As the field evolves, there is a growing inclination toward seeking more adaptable and automated techniques. Relying on manually engineered heuristics for distinct problems becomes cumbersome and nontrivial in practical applications [ 19 ]. Researchers are exploring innovative avenues that leverage machine learning and optimization fusion prowess, thereby mitigating the need for bespoke heuristics [ 10 , 20 , 21 ]. These modern paradigms hold the potential to learn from past problem instances and generalize across a spectrum of scenarios, diminishing the burden of bespoke design efforts [ 3 ].
We broadly classify the current learning-based approaches into those based on the learning construction and improvement heuristic. An extensive survey of different methods at the intersection of deep RL and CO is given by Bengio et al. [ 13 ]; Mazyavkina et al. [ 22 ].
Construction heuristic
The essence of a construction heuristic lies in its ability to build solutions from scratch, meticulously exploring the realm of feasible solutions one step at a time. Vinyals et al. designed the pointer network [ 23 ] which uses an attention mechanism to learn the conditional probability of a sequence of permutations given the input. They applied supervised learning to train the model to solve TSP instances with 50 client nodes. The idea was extended by Bello et al. [ 24 ], who applied an actor-critic RL algorithm to train the pointer network and improved the performance by a fine-tuning approach based on supervised learning.
The utilization of graph neural networks [ 25 ] and attention mechanisms [ 26 ] to craft encoding–decoding policies has become widespread in contemporary times. Dai et al. [ 27 ] proposed a graph embedding network that learns greedy heuristics, and they applied the Q-learning algorithm to train the policy network. Li et al. [ 28 ] combined tree search and graph convolutional networks (GCN), training them in a supervised manner. Mittal et al. [ 29 ] employed supervised learning followed by RL to train policy networks modeled by GCN, enabling them to handle millions of instances through data preprocessing. Kool et al. [ 30 ] utilized a transformer [ 26 ] to model the encoder and decoder, employing the REINFORCE algorithm with a deterministic greedy rollout baseline to train the policy network. They also combined the greedy algorithm and beam search in decoding to generate a solution sequence. Joshi et al. [ 31 ] employed a GCN to generate an edge adjacency matrix representing the probability of edge occurrences in the solution, subsequently applying beam search to convert the edge probabilities into a reasonable solution. Fu et al. [ 32 ] integrated machine learning with heuristic techniques such as tree search, graph sampling, graph transformation, and heat map merging. Their approach extended a small pre-trained model to handle arbitrarily large instances of the Traveling Salesperson Problem (TSP).
Improvement heuristic
Approaches founded on improvement heuristics incorporate reinforcement learning (RL) into advanced search or aim to learn improvement operators directly [ 33 , 34 ]. These methods typically take longer to solve instances (due to the extensive number of iterative operations) than construction heuristic-based approaches, yet they often yield superior results. Chen and Tian et al. [ 35 ] proposed a method that iteratively modifies the local elements of a solution. A trainable region selection policy identifies the portions of the solution to be altered in each iteration. In contrast, a trainable rule selection policy selects an operation from a collection of feasible modification procedures in each iteration. Hottong and Tierney et al. [ 36 ] employ predetermined manual procedures to systematically alter sections of the solution, which are then rebuilt using learned repair processes. Wu et al. [ 2 ] suggested using RL to select better solutions from defined local neighborhoods (e.g., 2-opt neighborhoods) for solving routing issues. Hottong et al. [ 37 ] learned continuous representations of solutions to discrete routing problems using conditional variational autoencoders and searched for solutions with a generic continuous optimizer. Lu et al. [ 38 ] introduced a learning-based iterative technique for the vehicle routing problem (VRP) that focuses on controller selection. Their approach learns to optimize solutions iteratively using an improvement operator based on RL. To enhance result quality, Zhao et al. [ 39 ] merged a deep reinforcement learning (DRL) model with a local search strategy, and they tested their approach on VRPTW instances of various sizes. Zheng et al. [ 40 ] combined three RL strategies (Q-learning, Sarsa, and Monte Carlo) with the well-known Lin Kernighan-Helsgaun (LKH) algorithm for tackling the traveling salesperson problem (TSP). Similar methodologies based on improvement heuristics also encompass [ 41 , 42 , 43 , 44 , 45 , 46 ].
Dynamic programming
The construction and improvement heuristics mentioned above are powerful but limited in adaptability because particular procedures must be created for individual issues. Dynamic programming is a versatile and exact approach to finding an optimal solution. On the other hand, traditional dynamic programming techniques are confined to small-scale issue cases due to the curse of dimensionality; hence, they are not as popular as heuristics. For a complete examination of using classical dynamic programming to solve multiple objective challenges, see Mahmoud A. Abo-Sinna [ 47 ].
If an effective and computationally efficient strategy for selecting potentially viable solutions can be devised, surpassing the limitations of dynamic programming might be possible. Currently, employing neural networks to approximate or enhance dynamic programming techniques presents a promising avenue of exploration. For instance, Yang et al. introduced a method called neural network dynamic programming (NNDP) [ 48 ]
In contrast to NNDP, the approach proposed by Xu et al. [ 49 ] eliminates the need for training test samples. Instead of training a single neural network for each problem size, they train a series of neural networks sequentially. Similar methodologies that amalgamate neural networks with dynamic programming to tackle combinatorial optimization problems include works like [ 50 , 51 ], among others.
Differences from existing methods
Existing learning-based methodologies have predominantly focused on addressing single-objective combinatorial problems. Recent endeavors have sought to extend these techniques [ 14 , 15 , 52 ] to tackle the complexities of MOO. These approaches employ the MOEA/D [ 9 ] framework to decompose the MOO problem into a series of single-objective subproblems. Subsequently, a collection of models is established to address each subproblem independently.
Nonetheless, the exponential proliferation of Pareto solutions presents a formidable challenge, necessitating many models to identify the entire Pareto set comprehensively. In this paper, our proposed approach capitalizes on the power of meta-learning and meta-neural networks to construct versatile meta-models that exhibit superior adaptability and flexibility across diverse subproblems. By leveraging meta-learning, our models acquire the ability to adapt to new tasks based on prior knowledge swiftly, enabling efficient handling of a wide range of subproblems within the MOO.
Moreover, we integrate the principles of dynamic programming within the meta-model to facilitate the decomposition of MOO, ultimately enhancing the precision and accuracy of our solutions.
Preliminaries and overview
Problem setup.
This paper focuses on solving two MOO problems: the multi-objective traveler problem (MOTSP) and the multi-objective vehicle routing problem with a time window (MOVRPTW).
MOTSP [ 53 ] is defined on a graph \(G=(\overline{V }, \overline{E })\) with \(m\) cost matrices, where \(\overline{V }=\{0,\dots ,n\}\) is the node-set (node 0 denotes the depot, and other nodes denote customers), \(\overline{E }=\{{e}_{ij}|i,j\in V, i\ne j\}\) is the edge set. The \(k-th\) cost matrix \({[{c}_{i,j}^{k}]}_{n\times n}\) gives the cost from node \(i\) to node \(j\) . The objective is to find a permutation \(\pi (\pi \left(0\right), \pi \left(2\right),\dots ,\pi \left(i\right),\dots ,\pi (n))\) of \(n\) nodes that minimizes the \(m\) objective functions simultaneously. The \(k-th\) objective function can be calculated as follows:
MOVRPTW [ 54 ] comprises multiple routes corresponding to uniform vehicles with the same capacity. Each vehicle leaves the depot, serves the customers under capacity and time window constraints, and returns to the depot. We simultaneously optimize multiple conflicting objectives (for example, minimizing the total tour distance, total time, etc.):
The decomposition of the multi-objective problem into multiple single-objective problems (scalar optimization subproblems) can be done using the strategy of weight sums, i.e., linear combinations of different objectives:
where \(\uplambda \) is a uniformly distributed weight vector and \(f\left(\pi |{\lambda }^{j}\right)\) is the objective function of the \(j-th\) subproblem.
Dynamic programming and Markov decision processes
Dynamic programming was created to address a specific optimization problem [ 49 ]. When using it to solve a combinatorial optimization problem, we usually consider it a multi-step decision problem. Making an optimal decision at each step should lead to the optimal solution for the overall problem. Dynamic programming can be considered an improvement of the fundamental search strategy when the search space has the form of optimum substructures and overlapping subproblems.
We denote \((S, A\left(s\right), P\left({s}{^\prime}|s, a\right), R\left(s, a\right), \gamma )\) as a discounted Markov Decision Process (MDP), where \(S\) is the set of problem states, \(A\left(s\right)\) is the set of feasible actions when in a state \(s\in S\) , \(P\left({s}{^\prime}|s, a\right)\) is the transition probability of transitioning from state \(s\) to state \({s}{^\prime}\in {S}{^\prime}\subseteq S\) after taking an action \(a\in A(s)\) , \(R(s, a)\) is a direct reward when taking action \(a\) in state \(s\) , and \(\gamma \in [\mathrm{0,1})\) is the discount rate applied to the future reward. The Bellman equation determines the maximum value for each state:
where \(V\left(s\right)\) is the value function of state \(s\) . The Bellman equation can be solved by backward induction, and the final value function is produced by calculating the value function for smaller subproblems one at a time.
Background on meta-learning
Meta-learning aims to generalize across tasks rather than around data points [ 55 ]. Inputs \({x}_{t}\) , outputs \({a}_{t}\) , a loss function \({\mathcal{L}}_{i}({x}_{t}, {a}_{t})\) , a transition distribution \({P}_{i}({x}_{t}|{x}_{t-1}, {a}_{t-1})\) , and an episode length \({H}_{i}\) define each task \({\Gamma }_{i}\) . The distribution \(\pi ({a}_{t}|{x}_{1},...,{x}_{t};\theta )\) is modeled by a meta-learner with parameters \(\theta \) . The meta-learner’s goal is to minimize its expected loss concerning \(\theta \) , given a distribution over tasks \(\Gamma =P({\Gamma }_{i})\) .
The expected loss is optimized over tasks (or mini-batches) sampled from \(\Gamma \) to train a meta-learner. For testing, the meta-learner is evaluated on previously unseen tasks from a task distribution \(\widetilde{\Gamma }=P(\widetilde{{\Gamma }_{i}})\) , which is comparable to the training task distribution \(\Gamma \) .
Methodology
Policy network.
We use the transformer [ 26 , 30 , 56 ], the state-of-the-art modeling for routing optimization, as the infrastructure for policy networks. We also improve the original transformer to make it more suitable for complex routing problems.
Encoder : The encoder takes node features \({x}_{i}\) ( \(i\in \overline{V })\) including coordinates and demand as input and first applying a linear projection \({h}_{i}^{0}={W}_{0}{x}_{i}+{b}_{0}\) ( \({W}_{0}\) and \({b}_{0}\) are learnable parameters) to create an initial embedding \({h}_{i}^{0}\) . The central part of the encoder consists of three self-attentive blocks (SA) that generate the embedding.
where \({H}^{0}=({h}_{0}^{0},\dots ,{h}_{n}^{0})\) is the sequence of initial embeddings. Each block consists of a multi-head attention layer (MHA) and an element-wise fully connected layer (FF), each in turn then followed by a residual (res) connection and a batch normalization layer (BN).
where \({h}_{i}^{l}\) denotes the embedding of \(i-th\) node in layer \(l (l\in \{1,\dots ,N\})\) .
The general definition of the fully connected and residual layers is as follows:
We apply MHA to different aggregate types of information from other nodes, as follows:
where \(M\) denotes the number of heads in the MHA, which is based on SA, and its calculation process is described as follows:
Both W-series and b-series are learnable parameters (Fig. 2 .
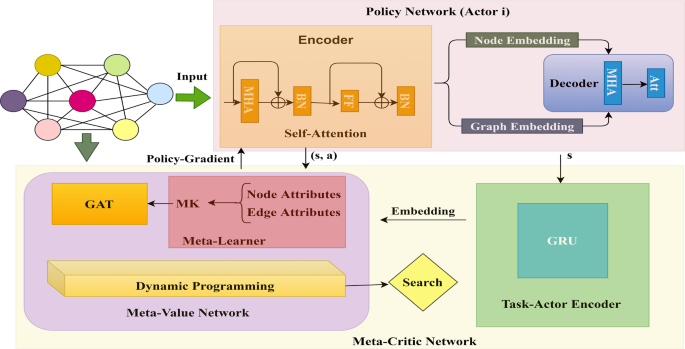
An overview of the proposed framework. DPML consists mainly of a policy network (actors) and a meta-critic network. The meta-critic consists of a meta-value network and a task-actor encoder, instructing actors to learn about different policies
Decoder : The decoder accepts a context \({C}_{t}\) and selects the next node to add to the current tour by looking over the sequence \(seq=({\widehat{h}}_{0}^{node}, {\widehat{h}}_{1}^{node}, \dots , {\widehat{h}}_{n}^{node})\) at decoding step \(t\in \{1, ..., T\}\) . The context \({C}_{t}\) is made up of the graph embedding \({\widehat{h}}_{i}^{graph}=\frac{1}{n+1}{\sum }_{i=0}^{n}{\widehat{h}}_{i}^{node}\) , the current vehicle’s remaining capacity \({Q}_{r}^{(t)}\) , and the preceding node’s embedding \({\widehat{h}}^{pre}={\widehat{h}}_{pre}^{node}\) :
The concatenation operator is represented by \([;]\) . A multi-head attention layer and a subsequent layer with just attention weights operate on a masked input sequence. Values of alternatives ruled out by hard constraints of the routing issues are set to \(-\infty \) and give zero weights. At step \(t\) of decoding, the decoder outputs the probability \({P}_{\theta }\) of choosing node \(i\) for the decision \({\pi }_{t}\) .
Meta-learning
We construct our policy network as an actor-network for each training task. We also construct a core policy-guiding network, the meta-critic network, consisting of a meta-value network and a task-actor encoder. We train this meta-critic network simultaneously using the actor-critic policy gradient algorithm with multiple tasks (problem instances). It is akin to constructing a new actor network when dealing with a new task (the probability distributions generated by the decoding policies of various subproblem instances are different) while the meta-critic network remains intact.
Task-actor encoder : In the task-actor encoder, we input the task’s historical trajectory and get a task representation. We concatenate it with the general value network’s inputs (states and actions) and feed it into the meta-value network. While the transformer has achieved groundbreaking results in modeling sequences for supervised learning tasks, there is a distinct lack of demonstration of the transformer as a valuable RL memory. We define the task-actor encoder as a gated recurrent unit (GRU) that inputs a learning trace as a sequence of states:
where \({W}_{s}\) and \({U}_{s}\) are weight matrices, \({b}_{s}\) are biases. GRU derives a vector representation of the hidden state, denoted as
where \(\circ \) is the element-wise multiplication.
Actor and Meta-critic network optimization : Policy networks for different tasks are optimized under the guidance of meta-critic networks. The policy network \({P}_{\theta }\) outputs a categorical distribution \({\pi }_{t}\) of available actions and then samples the actions from the generated distribution \({a}_{t}\sim {P}_{\theta }\) . Suppose there exist \({M}^{(T)}\) different training tasks (problem instances) and the updated rules for each actor (policy) \(i\) and meta-critic network \({Q}_{\phi }\) are as follows:
where \(l(,)\) is the cross-entropy loss function, \({C}_{t}^{\left(i\right)}\) is the contextual embedding (Eq. ( 14 )) generated by our policy network \({P}_{\theta }\) and \({a}_{t}^{\left(i\right)}={P}_{{\theta }^{(i)}}({s}_{t}^{(i)})\) .
Meta Graph Attention Networks : We incorporate meta-knowledge into the GAT [ 57 ] to make it MetaGAT, which shares parameters and inputs with the policy network. The score of the edge \({e}_{ij}\) is related to the hidden states of node \(i\) and node \(j\) and the meta-knowledge of nodes and edges learned from the graph. For edge \({e}_{ij}\) , we obtain the hidden states of the nodes, i.e., \({\widehat{h}}_{i}^{node}\) and \({\widehat{h}}_{j}^{node}\) by index, and the metaknowledge \({MK}_{ij}\) , which is a combination of node and edge metaknowledge:
Here \(\parallel \) denotes the concatenation operator, and the attention score is calculated as follows:
Here \({w}_{ij}\) is a vector indicating the importance of \({\widehat{h}}_{i}^{node}\) for \({\widehat{h}}_{j}^{node}\) .
Approximate dynamic programming meta-value network : Dynamic programming can be used to solve optimization problems in two ways. For changes based on the value function, solving the value function of the states and then selecting a policy based on maximizing the value function; the other approach is to derive the optimal policy for each state and perform the optimal action in each state. Since our meta-learning-based RL algorithm is to construct a meta-value network to guide policies to handle different tasks, this process is more in line with the former.
We implement dynamic programming using the above meta-neural networks to approximate the meta-value network. We approximate the value function \({V}_{\varphi }\) starting from the smallest problem or the state in the last decision step. MetaGAT is trained to minimize the mean square error (MSE) of the results of the value function estimation:
where \({T}_{a}^{s}\) is the total number of state and action combinations, \({\varphi }_{1}\) is the parameter of \({MetaGAT}_{1}\) , \({S}_{1}\) the set of all possible states of the subproblem, \(R({s}_{1}, a)\) is the instantaneous reward obtained by selecting the action \({a}_{1}\) at state \({s}_{1}\) . Then for subsequent neural networks corresponding to subproblems, we train the neural network \({MetaGAT}_{{\text{n}}}\) is to minimize the following MSE:
where \({s}_{i}\) is the state of subproblem \(i\) due to the following policies generated by the neural networks. The complete flow of the proposed method is shown in Algorithms 1, 2, and 3, where Algorithms 1 and 3 are meta-learning that can be used as a general framework. Algorithm 2 is a specific RL algorithm that can be replaced with other RL algorithms or supervised learning.
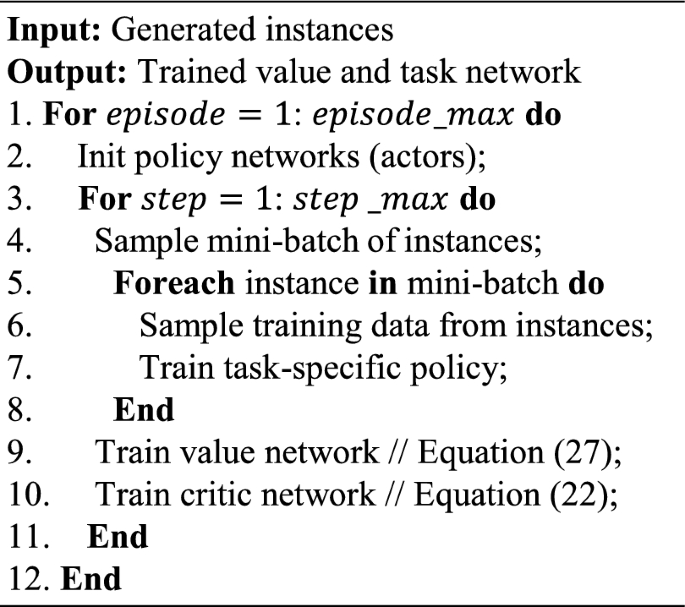
Actor-critic algorithm
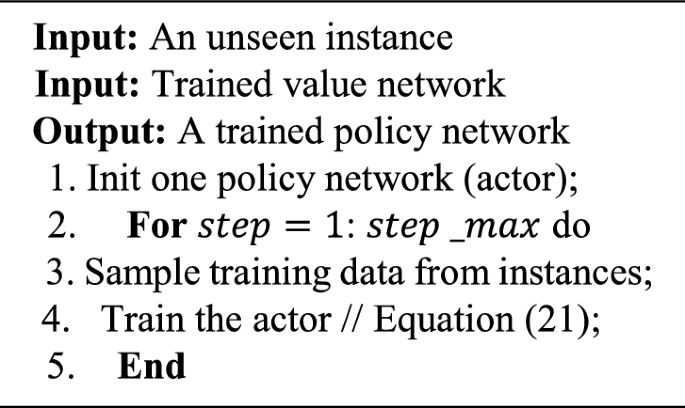
Meta-testing
Complexity analysis : Our transformer-based encoder has a time complexity of \(O\) ( \({n}^{2}*d\) ), where \(d\) is the number of dimensions and \(n\) is the number of nodes. The Transformer-based encoder involves processing data with a complexity that scales linearly with the number of dimensions and logarithmically with the dimensionality itself. This complexity arises from the operations performed on each node, such as feature extraction and dimension reduction. On the other hand, the decoder outputs a linear sequence of size \(n\) at a time, resulting in a computational complexity of \(O(n)\) . This complexity is primarily determined by the number of nodes in the output sequence and remains constant regardless of the dimensions or other factors.
Several factors influence the training algorithm’s complexity, including the number of \(episode\_max\) , \(mini-batch\) , batch \(B\) , and \(step\_max\) . Algorithm 1, consisting of a triple loop, has a time complexity of \(O\) ( \(step \_max*{\text{mini}}-{\text{batches}}*episode\_max\) ), where \(step\_max\) and \(episode\_max\) represent the maximum time steps and maximum episodes, and \(mini-batch\) denotes the number of sampled mini-batches. Algorithm 2, with double loops iterating over batch \(B\) and step_max, has a time complexity of \(O\) ( \(B* step \_max\) ), where \(B\) represents the number of batches. Algorithm 3, comprising a single loop, has a time complexity of \(O\) ( \(step \_max\) ). Finally, the time complexity of DPML is determined by the highest time complexity among the mentioned algorithms, which is \(O\) ( \(step \_max*{\text{mini}}-{\text{batches}}*episode\_max\) ).
Optimality analysis : This paper’s multi-objective routing optimization problems aim to minimize the cost of each sub-problem (sub-objective function), as shown in Eq. ( 4 ).
[ 58 ] A feasible solution \({\pi }^{*}\) is Pareto optimal if and only if there exists a weight vector \(\lambda >0\) such that \({\pi }^{*}\) is an optimal solution to the problem (4).
Lemma 1 states that every Pareto solution may be obtained by resolving subproblems with specific weights. According to Lemma 1 , in the best scenario, if every produced solution \(\pi \) is the optimal solution \({\pi }^{*}\) of the problem (4) with vector \(\lambda \) , our model may provide the complete Pareto set of the original MOO problem.
Assumption 1
A potentially robust approximation of the complete Pareto set for MOO problem can be achieved if the proposed method is capable of effectively solving subproblems with any weight \(\lambda \) .
We verify this assumption using the well-known \(\varepsilon \) -Pareto approximation approach for the MOO [ 2 , 59 ]
Definition 1
( \(\varepsilon \) -Pareto Domination). For a MOO problem and \(\varepsilon >0\) , if \({f}_{i}\left({\pi }_{a}\right)\le \left(1+\varepsilon \right){f}_{i}\left({\pi }_{b}\right), \forall i\in \{1,\dots , m\}\) , then \({\pi }_{a}\) \(\varepsilon \) -dominate \({\pi }_{b}\) ( \({\pi }_{a}\) \({\prec }_{\varepsilon }\) \({\pi }_{b}\) ) is true.
The (1 + \(\varepsilon \) ) approximation for single-objective optimization is a straightforward generalization of the formulation provided here. This idea allows us to define \(\varepsilon \) -approximate Pareto sets [ 60 ] as follows:
Definition 2
( \(\varepsilon \) -Approximate Pareto Set). For an \(\varepsilon >0\) , a set \({\mathcal{P}}_{\varepsilon }\) is an \(\varepsilon \) -approximate Pareto set, if for any feasible solution \(\pi \) , there exists a solution \({\pi }{\prime}\in {\mathcal{P}}_{\varepsilon }\) such that \({\pi }{\prime}{\prec }_{\varepsilon }\pi \) .
Some solutions in \({\mathcal{P}}_{\varepsilon }\) can potentially dominate nearly all feasible solutions to the MOO [ 3 , 59 ]. The \(\varepsilon \) -approximate Pareto set would be a logical choice for practice when finding Pareto sets is challenging and intractable. Although several \(\varepsilon \) -approximate Pareto sets may exist, each MOO has a distinct Pareto set. The effectiveness of our policy on each single-objective subproblem substantially influences its capacity to identify \(\varepsilon \) -approximate Pareto sets.
Let \({\pi }^{*}\) represents the optimal solution to the problem (4) with weight \(\lambda \) . We can produce a “ \(\varepsilon \) -approximate Pareto set \({\mathcal{P}}_{\varepsilon }\) ” for the MOO, if the proposed method can produce an approximate solution \(\pi {{\prec }_{\varepsilon }\pi }^{*}\) for any weight \(\lambda \) .
Let \(\mathcal{P}\) be the Pareto set of the MOO problem. Lemma 1 states that for each \({\pi }_{Pareto}\in \mathcal{P}\) , there exists a weight vector \(\lambda >0\) such that \(\pi ={\pi }^{*}\) is an optimal solution to subproblem (4) with a weight \(\lambda \) . As a result, our strategy can produce an approximated solution \(\pi {{\prec }_{\varepsilon }\pi }^{*}={\pi }_{Pareto}\) . Our approach can produce a \(\varepsilon \) -approximate Pareto set \({\mathcal{P}}_{\varepsilon }\) for the MOO by producing approximate solutions for all \({\pi }_{Pareto}\in \mathcal{P}\) .
Experiment settings
Datasets for MOTSP : DPML was evaluated on the Euclidean dual objective TSP [ 61 ], a standard MOTSP. This issue has two objectives that must be optimized concurrently, each with its Euclidean distance matrix. A node within the problem has two coordinate features, each uniformly sampled from \([0, 1]\times [0, 1]\) from a standard benchmark instance or normalized to \([0, 1]\times [0, 1]\) . The Euclidean distance of each objective is calculated using each coordinate feature. We set the number of weight vectors \(N\) in the Pareto front construction to 100. They are uniformly distributed between (0,1) and (1, 0), i.e., \({\lambda }^{1}=\left(0, 1\right)\) , \({\lambda }^{2}=(\frac{1}{99}, \frac{98}{99})\) ,…, \({\lambda }^{100}=(1, 0)\) .
Datasets for MOVRPTW : The instances for MOVRPTW are created in the same way as the Solomon dataset’s “R” group (random group) [ 39 ]. The capacity for problem sizes 20 and 50 is set to \({Q}^{20}=500\) and \({Q}^{50}=750\) , respectively. \([{a}_{0}=0, {b}_{0}=1000]\) is the whole time range, whereas the service duration \({h}_{i}\) is uniformly set to 10. The additional material has extra information. We employ the same vehicle capacities \({Q}^{20}\) =30 and \({Q}^{50}=40\) , and the same validation and test sets as indicated in [ 30 ]. For the MOVRPTW solution, its total travel time ( \({f}_{1}\) ) is larger than its makespan ( \({f}_{2}\) ). As a result, we aim to balance the weights to achieve these two objectives. The following changes are made to the aggregation function of the \(j-th\) subproblem to be optimized:
Here \({f}_{1}^{*}\) and \({f}_{2}^{*}\) are the global minimum value of the corresponding objectives. Euclidean instances with 4-D input and mixed instances with 3-D input are generated as the training set for our method. They are all generated from [0, 1] uniform distribution.
Baseline and evaluation metrics : Whereas most state-of-the-art learning-based approaches and solution solvers have been designed to cope with single-objective optimization, the proposed methodology focuses on MOO in real-world applications. Therefore, we choose representative state-of-the-art learning-based methods such as AM [ 30 ] (deal with different subproblems separately), MODRL/D-AM [ 15 ], MODRL/D-EL [ 52 ] and DRL-MOA [ 14 ] as baselines. We also choose the traditional evolutionary algorithms NSGA-II [ 8 ] and MOEA/D [ 9 ], two of the most popular multi-objective evolutionary algorithms widely used in real-world applications.
We apply Hypervolume (HV) and the number of non-dominated solutions (|NDS|) to evaluate various execution methods. HV is an important metric to evaluate the combined convergence and diversity of the Pareto front. At the same time, NDS reflects the diversity of the Pareto front when the HV values are close. In general, methods with larger HVs or NDSs perform better.
Hyper-parameters : The policy network’s node encoder comprises three SA blocks with a dim of 128. \({d}_{node}=128\) is the embedding dimension of all issues. We employ a hidden dimension of 256 for the decoder. As meta-node and edge knowledge learners, we employ two FCNs (two layers with the same number of hidden cells) and do a grid search for the number of hidden cells on {4,8,16,32,64}. We employ the same number of hidden cells for the task-actor encoder and Meta-GAT and run a grid search for the number across {16,32,64,128}. The model is trained for 50 epochs, each with 1,024,000 training instances, with issue sizes ranging from \({BS}^{20}=512\) to \({BS}^{50}=128\) . We apply the Adam optimizer with a smooth learning rate decay schedule depending on \({\eta }_{{t}_{e}}=(\frac{1}{1+\gamma {t}_{e}}){\eta }_{{t}_{e}-1}\) at epoch \({t}_{e}\) with an initial learning rate of \({10}^{-4}\) and decay factor \(\upgamma =0.001\) . Our model mainly converges after 50 epochs, although the results are comparable to other research that only converges after 100. We use Hypervolume (HV) and the number of non-dominated solutions (|NDS|) as metrics to evaluate different methods, and the method with higher values performs better. Our implementation is available at https://github.com/Anonymousauthorx/DPML .
Experimental results
We trained and evaluated various learning-based algorithms on 128 random instances of MOTSP-20 and MOTSP-30 with 20 and 50 nodes, respectively, to assess their performance. We trained on random cases MOTSP-50, MOTSP-80, and MOTSP-100 and then tested each instance independently to see if the presented meta-model could generalize to diverse learning tasks. Unlike previous meta-learning techniques, ours does not require specific parameter fine-tuning and merely requires training a meta-critic network to correspond to policies with various data distributions. On the other hand, MODRL/D-AM [ 30 ], DRL-MOA [ 12 ] and MODRL/D-EL [ 52 ] are exposed to one-on-one correspondence training and testing using MOTSP-30, MOTSP-80, and MOTSP-100.
“T-step” denotes updating the stochastic model and meta-model for each sub-problem. When using DRL-MOA with T-step, we first use AM to update the initial model in 5000 steps for the first subproblem with weight vector \({\uplambda }^{1}=(0 ,1)\) . Then, using \(T\) update steps, the next model is derived from the previous sub-model for each of the remaining 99 sub-problems. In these instances, we set the reference point for calculating HV to (60, 60). The average findings for HV and |NDS| across 128 random occurrences are shown in Table 1 .
Regarding accuracy (i.e., MOTSP20 and MOTSP30), our technique beats AM and DRL-MOA by requiring extremely few updates (time) steps to attain good results. The findings also suggest that the proposed meta-models have significant generalization skills when adapting to new challenges at various dimensions. DPML is superior to AM and DRL-MOA since they are trained directly on relevant problems without scaling issues. The proposed meta-critic-based meta-model captures some standard (data distribution) MOTSP properties. A small amount of training on diverse cases is enough to provide promising results for various sizes of instances.
For MOVRPTW, we train our meta-models using Solomon instances, namely R101, R102, and R103, and test them separately. These instances have 100 clients, and their coordinates are normalized to \([0, 1]\times [0, 1]\) . Table 2 shows HV and |NDS| for learning-based approaches. We see that DPML is better than the other methods in performance and generalization. Other methods can only follow the traditional training and testing paradigm of training a model with one dataset and then testing it on another. In contrast, our method can train a meta-critic using different datasets and then generate policies based on different data distributions in different test sets.
The Pareto fronts obtained by the three learning algorithms on the VRPTW instance of Solomon (R101) can be observed in Fig. 3 a. The non-dominated solutions discovered by DPML are located in the lower-left corner of the figure, exhibiting a uniform dispersion. MOVRPTW, on the other hand, reveals a smaller number of non-dominated solutions due to the positive correlation between the evaluated objectives (total tour time and completion time), which implies that optimizing one objective can improve the other. Figure 3 a illustrates that DPML provides decision-makers with valuable trade-off options. In Fig. 3 b, the fluctuation of |NDS|, derived from the scatterplot test of the first four epochs of DPML (trained on R101 and tested on R102), can be observed as the number of time steps increases. It is noteworthy that DPML exhibits consistent fluctuations within a relatively stable range during the test, even when dealing with unknown data distributions. This demonstrates its strong adaptability in learning diverse policies through the meta-model.
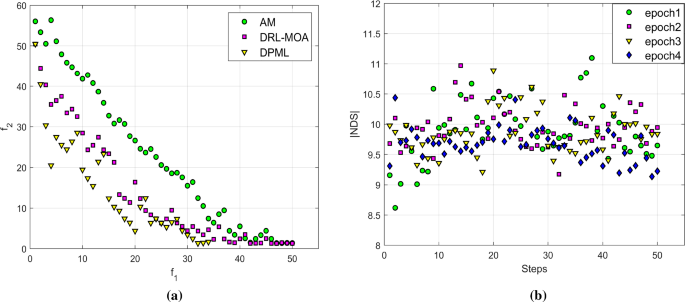
The left figure a shows the Pareto fronts obtained by different learning-based algorithms on the Solomon VRPTW instance, and the right figure b shows the |NDS| scatter plot obtained during testing
Effect of DPML on other datasets : We also performed comparison experiments on three commonly used benchmark MOTSP instances: kroAB100, kroAB150, and kroAB200 [ 14 ]. These instances are constructed from kroA and kroB in the TSP library. We set the reference point used to calculate HV to (90, 90) for these instances. The results are shown in Table 3 .
We compared our proposed DPML method with the state-of-the-art reinforcement learning techniques tailored explicitly for MOO, namely MODRL/D-AM and MODRL/D-EL, on the R201, R202, and R203 datasets. While MODRL/D-AM employs decomposition and AM for MOO, MODRL/D-EL takes a step further by integrating evolutionary algorithms to fine-tune its parameters based on MODRL/D-AM.
The results presented in Table 4 demonstrate the competitive performance of DPML in terms of both the HV and the Cardinality of the |NDS|. This noteworthy achievement can be attributed to the distinctive features of DPML, particularly the incorporation of meta-learning and dynamic programming algorithms. These enhancements contribute to the heightened flexibility and precision of DPML compared to its counterparts, MODRL/D-AM and MODRL/D-EL.
We also compared our method with state-of-the-art evolutionary algorithms; the results are shown in Table 5 .
The results show that DPML outperforms other learning-based methods on many MOTSP datasets and outperforms evolutionary algorithms with only a few update steps required.
Table 6 compares the results between DPML and traditional methods on the Solomon dataset. Again, it can be seen that our method requires very few update steps to outperform traditional evolutionary algorithms.
Unseen weight vectors : Table 7 compares our technique to DRL-MOA for the new subproblem of unseen weight vectors. For the test, twenty weight vectors were created. \({\uplambda }_{1}{f}_{1}+{\lambda }_{2}{f}_{2}\) , which gives the weighted-sum cost of the relevant sub-problem and yields the \(f\) column. The neighboring submodels utilized for fine-tuning in DRL-MOA are shown in the “submodel” column. DPML beats DRL-MOA for all new subproblems for the reasons listed below, as shown in the figure. DRL-MOA trains submodels that are particular to the subproblem. A competent submodel for the new subproblem may not be obtained by fine-tuning the nearby submodels in a few steps.
On the other hand, the meta-model in DPML learns from diverse subproblems and captures their common characteristics. As a reason, it can better handle new sub-problems using weight vectors that aren’t observable. In this example, DPML only needs to keep one meta-model in memory, but DRL-MOA requires ten sub-models. DPML is a good learning paradigm for MOP, according to all evidence.
Ablation study : Due to the composition of various components in the proposed method, it is necessary to conduct ablation studies to validate the effectiveness of each component. We individually removed dynamic programming, meta-learning, and task-actor encoders to observe the performance of DPML. The experimental results of the ablation studies are presented in Table 8 , with results obtained from R201, R202, and R203. When dynamic programming was removed, DPML’s HV decreased by 5.06%, 1.88%, and 1.41%, respectively. Simultaneously, |NDS| decreased by 33.33%, 30.77%, and 41.67%. Removing meta-learning decreased DPML’s HV by 9.47%, 8.78%, and 19.93%, while |NDS| decreased by 40%, 46.15%, and 50%. Similarly, when the task-actor encoders were removed, DPML’s HV decreased by 2.01%, 1.53%, and 1.38%, and |NDS| decreased by 20%, 15.38%, and 25%. These results indicate the crucial role played by the three components in the performance of DPML, with meta-learning and dynamic programming being particularly important.
A range of experiments has substantiated the efficiency and effectiveness of the proposed method, and the underlying reasons can be summarized as follows: Firstly, by leveraging meta-learning, we circumvent the need for excessive individual models, thereby streamlining the computational complexity associated with identifying the complete Pareto set. Secondly, incorporating dynamic programming principles within our meta-models empowers us to decompose and address the intricacies of MOO effectively. Integrating divide-and-conquer strategies with meta-learning enhances solution quality and enables us to navigate the MOO more efficiently.
The capacity of deep learning tends to enhance as the model’s complexity increases. However, this increased complexity also creates a computational burden, necessitating additional computing resources. Furthermore, when utilizing deep meta-reinforcement learning to simulate dynamic programming, the meticulous design of neural networks is essential to preserve their differentiability, resulting in a relatively higher overall workload. To address these concerns, we have taken measures to balance the capacity and complexity of our deep learning model. We have optimized the computational efficiency by carefully designing our neural networks to maintain differentiability, thereby reducing the overall workload. Additionally, we have carefully managed the allocation of computing resources to ensure efficient utilization while maximizing the model’s capacity for enhanced performance.
We propose a novel meta-reinforcement learning approach based on dynamic programming to tackle real-world multi-objective routing optimization problems. Our method introduces a policy network with a transformer architecture designed for complex routing problems. This transformer allows the policy network to capture and utilize rich contextual information from nodes and graphs. To incorporate meta-learning into the RL framework, we introduce a meta-critic network that leverages multiple tasks. This network comprises a task-actor encoder and a meta-value network, which work together to guide the policy network in handling diverse sub-problems. The meta-value network is constructed using a meta-attention network, which combines meta-knowledge to approximate the value function. Additionally, we employ a GRU-based task-actor encoder to provide historical trajectories for the meta-critics, enhancing the effectiveness of RL training in practical scenarios.
Data availability
All data supporting the findings of this study are available from the corresponding author upon reasonable request. Where applicable, data have been deposited in publicly available repositories.
Ehrgott M, Gandibleux X (2000) A survey and annotated bibliography of multiobjective combinatorial optimization. OR Spectrum 22:425–460. https://doi.org/10.1007/s002910000046
Article MathSciNet Google Scholar
Herzel A, Ruzika S, Thielen C (2021) Approximation methods for multiobjective optimization problems: a survey. INFORMS J Comput. https://doi.org/10.1287/ijoc.2020.1028
Song F, Xing H, Wang X, Luo S, Dai P, Xiao Z, Zhao B (2022) Evolutionary multi-objective reinforcement learning based trajectory control and task offloading in UAV-assisted mobile edge computing. IEEE Trans Mob Comput. https://doi.org/10.1109/TMC.2022.3208457
Article Google Scholar
Wang H, Fu T, Du Y, Gao W, Huang K, Liu Z, Chandak P, Liu S, Van Katwyk P, Deac A, Anandkumar A, Bergen K, Gomes CP, Ho S, Kohli P, Lasenby J, Leskovec J, Liu T-Y, Manrai A, Marks D, Ramsundar B, Song L, Sun J, Tang J, Veličković P, Welling M, Zhang L, Coley CW, Bengio Y, Zitnik M (2023) Scientific discovery in the age of artificial intelligence. Nature 620:47–60. https://doi.org/10.1038/s41586-023-06221-2
Ehrgott M, Engau A, Wiecek MM (2022) Theory, computation, and practice of multiobjective optimisation. Ann Oper Res 319:1477–1478. https://doi.org/10.1007/s10479-022-05051-1
Jozefowiez N, Semet F, Talbi E-G (2008) Multi-objective vehicle routing problems. Eur J Oper Res 189:293–309. https://doi.org/10.1016/j.ejor.2007.05.055
Lust T, Teghem J (2010) The multiobjective traveling salesman problem: a survey and a new approach. Presented at the. https://doi.org/10.1007/978-3-642-11218-8_6
Deb K, Pratap A, Agarwal S, Meyarivan T (2002) A fast and elitist multiobjective genetic algorithm: NSGA-II. IEEE Trans Evol Comput 6:182–197. https://doi.org/10.1109/4235.996017
Zhang Q, Li H (2007) MOEA/D: a multiobjective evolutionary algorithm based on decomposition. IEEE Trans Evol Comput 11:712–731. https://doi.org/10.1109/TEVC.2007.892759
Wang Q, Tang C (2021) Deep reinforcement learning for transportation network combinatorial optimization: a survey. Knowl Based Syst 233:107526. https://doi.org/10.1016/j.knosys.2021.107526
Wang Q, Lai KH, Tang C (2023) Solving combinatorial optimization problems over graphs with BERT-based deep reinforcement learning. Inf Sci (N Y) 619:930–946. https://doi.org/10.1016/j.ins.2022.11.073
Wang Q, Hao Y, Chen F (2021) Deepening the IDA* algorithm for knowledge graph reasoning through neural network architecture. Neurocomputing 429:101–109. https://doi.org/10.1016/j.neucom.2020.12.040
Bengio Y, Lodi A, Prouvost A (2021) Machine learning for combinatorial optimization: a methodological tour d’horizon. Eur J Oper Res 290:405–421. https://doi.org/10.1016/j.ejor.2020.07.063
Li K, Zhang T, Wang R (2021) Deep reinforcement learning for multiobjective optimization. IEEE Trans Cybern 51:3103–3114. https://doi.org/10.1109/TCYB.2020.2977661
Wu H, Wang J, Zhang Z (2020) MODRL/D-AM: multiobjective deep reinforcement learning algorithm using decomposition and attention model for multiobjective optimization. Presented at the https://doi.org/10.1007/978-981-15-5577-0_45
Wang Q (2021) VARL: a variational autoencoder-based reinforcement learning framework for vehicle routing problems. Appl Intell 52:8910–8923. https://doi.org/10.1007/s10489-021-02920-3
Wang Q, Hao Y, Cao J (2021) Learning to traverse over graphs with a Monte Carlo tree search-based self-play framework. Eng Appl Artif Intell 105:104422. https://doi.org/10.1016/j.engappai.2021.104422
Trivedi A, Srinivasan D, Sanyal K, Ghosh A (2016) A survey of multiobjective evolutionary algorithms based on decomposition. IEEE Trans Evol Comput. https://doi.org/10.1109/TEVC.2016.2608507
Xing H, Xiao Z, Qu R, Zhu Z, Zhao B (2022) An efficient federated distillation learning system for multitask time series classification. IEEE Trans Instrum Meas 71:1–12. https://doi.org/10.1109/TIM.2022.3201203
- International
- Schools directory
- Resources Jobs Schools directory News Search
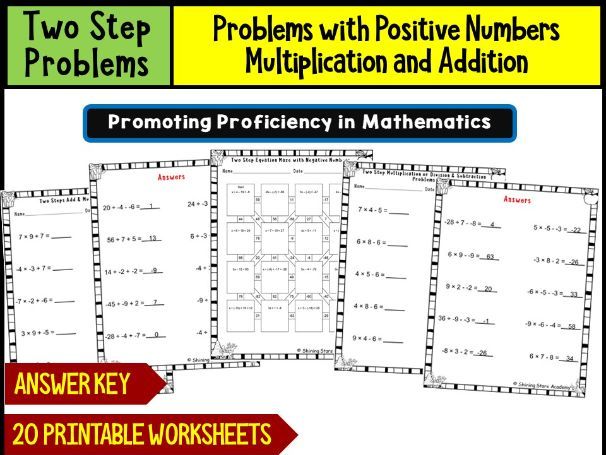
Two Step Problems with Positive Numbers Multiplication and Addition Worksheets Math Problems
Subject: Mathematics
Age range: 5-7
Resource type: Worksheet/Activity

Last updated
- Share through email
- Share through twitter
- Share through linkedin
- Share through facebook
- Share through pinterest
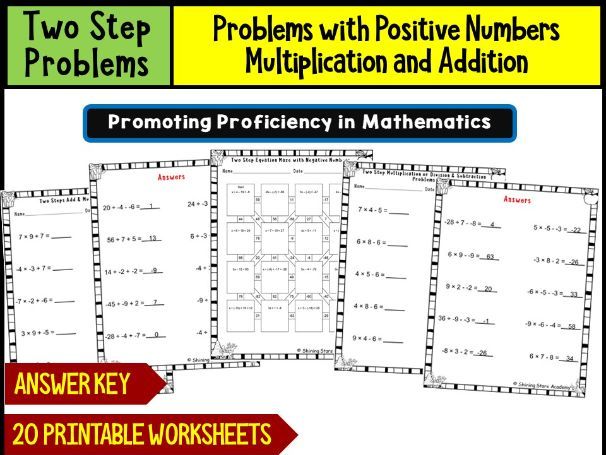
Two Step Problems with Positive Numbers Multiplication and Addition Worksheets Math Problems “Two-step Addition, Subtraction, Division & Multiplication Worksheets Problems” is a comprehensive resource designed to strengthen students’ proficiency in fundamental arithmetic operations. These worksheets offer a structured approach to mastering two-step problem-solving, integrating addition, subtraction, division, and multiplication. Varied Problem Types: Each worksheet presents a range of two-step problems, ensuring students encounter diverse scenarios that require different combinations of operations. Progressive Difficulty: Problems are organized by complexity, allowing students to gradually advance from basic to more challenging tasks. Answer Keys: Detailed answer keys accompany each worksheet, facilitating easy assessment and providing students with immediate feedback. Skill Reinforcement: Through repeated practice, students reinforce their understanding of addition, subtraction, division, and multiplication, building fluency and confidence. Whether used in the classroom, for homework assignments, or as supplementary practice, “Two-step Addition, Subtraction, Division & Multiplication Worksheets Problems” equips students with the skills they need to tackle multi-step mathematical problems with confidence and precision. Worksheets are made in 8.5” x 11” Standard Letter Size. This resource is helpful in students’ assessment, Independent Studies, group activities, practice and homework. This product is available in PDF format and ready to print as well.
Tes paid licence How can I reuse this?
Your rating is required to reflect your happiness.
It's good to leave some feedback.
Something went wrong, please try again later.
This resource hasn't been reviewed yet
To ensure quality for our reviews, only customers who have purchased this resource can review it
Report this resource to let us know if it violates our terms and conditions. Our customer service team will review your report and will be in touch.
Not quite what you were looking for? Search by keyword to find the right resource:
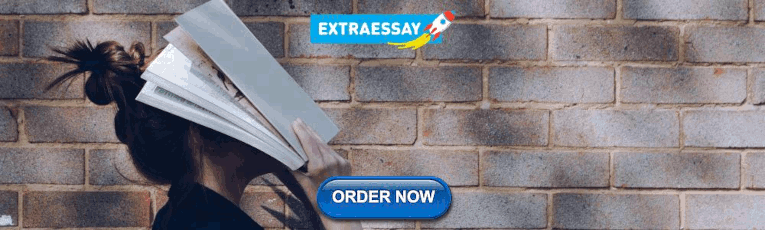
IMAGES
VIDEO
COMMENTS
The multi-step word problems worksheets are the best way to help students practice their skills in solving complex math problems. Our worksheets are the best on the internet and they are completely free to use. These worksheets provide a variety of problems that require students to use their critical thinking and problem-solving skills in order to find the solution.
Find the answers to the four word problems. Students will need to use reasoning skills to determine whether they should add, subtract, divide, or multiply. 4th and 5th Grades. View PDF. Multiple Step, Advanced #3. Here are four multiple-step word problems that will require a combination of addition, subtraction, multiplication, or division.
This lesson uses multisteps (more than one step) to solve multiplication problems, using the "Draw a Diagram" strategy.
Solve multi-step problems in contexts, deciding which operations and methods to use and why. ... One way to address the problem is through the use of interactive activities and this web site provides many of those. The Go ... Level 2 - Multiplication and division multi-step problems. Level 3 - Mixed multi-step problems.
Learn for free about math, art, computer programming, economics, physics, chemistry, biology, medicine, finance, history, and more. ... Multiplication word problem: pizza. Report a problem. ... Represent multi-step word problems using equations. Multi-step word problems with whole numbers. Math > 4th grade >
Learn how to Solve Multi-step Problems Involving Multiplication and Addition or Subtraction(Grade 4 Math)Made easy Lessons from cherrysclassroom.com: Solving...
GRADE 4 MATHEMATICS WEEK 5 LESSON 11: Solving Multistep Word Problems Involving Multiplication and Addition or SubtractionModule Page: 24-25This is an intera...
The printable PDF worksheets presented here involve single-digit multiplication word problems. Each worksheet carries five word problems based on day-to-day scenarios. Multiplication Word Problems: Two-digit times Single-digit. The word problems featured here require a grade 3 learner to find the product by multiplying a two-digit number by a ...
4567+. Kids often develop misconceptions about concepts in mathematics, including multiplication. It is important to help them get over those misconceptions. This multi-step word problems worksheet help students get a good grasp of the concepts. Here they work with multi-operation scenarios to find the unknown quantity.
Your one stop solution for all grade learning needs. Boost your child's understanding of multiplication with this worksheet. Students will work with a set of multi-step word problems. They will make sense of multi-operation scenarios and find the unknown quantity. Your young learner will have an exciting time playing this worksheet.
4th Grade Multiplication Problems. Here you will find a range of problem solving worksheets involving multiplication. Each sheet involves solving a range of written multiplication problems. There are 3 levels of difficulty for each worksheet below: A,B and C. Worksheet A is the easiest level, suitable for children at the beginning of their grade.
Multiplication word problems are multiplication problems laid out in a sentence format that relates to a real-life scenario. Children are asked to read the problem, work out what the multiplication problem is and how to solve it using the clues provided. Examples of multiplication word problems that are included in this resource pack are:
Here's how that looks in a bar chart: 9 x 3 = 27, so there are 27 green apples. So in total we have 27 + 9 apples, which comes to 36 apples altogether. If you are looking for some multiplication word problems you'll find them in our free math worksheet center. Here are the multiplication word problem worksheets for grade 3, grade 4 and grade 5.
Our wonderful pack of KS2 multiplication word problem flashcards is great for testing your KS2 children's knowledge of multistep multiplication and helping them to gain confidence in working on maths problems in verbal format rather than as raw mathematical sums. The flashcards will help your KS2 class understand the concept of long worded ...
Multi-Step Word Problem #1. Solution. Step 1: Break down each sentence of the problem and identify the information needed to solve the problem. The first sentence states that "Steven is reading a book that has 260 pages.". Hence, the total number of pages of that particular book is 260.
Multi-step word problems. IXL's SmartScore is a dynamic measure of progress towards mastery, rather than a percentage grade. It tracks your skill level as you tackle progressively more difficult questions. Consistently answer questions correctly to reach excellence (90), or conquer the Challenge Zone to achieve mastery (100)!
Multi-Step Word Problems worksheets for Grade 4 are an essential tool for teachers to help their students develop strong problem-solving skills in math. These worksheets provide a variety of challenging math word problems that require students to use multiple steps and operations to find the solution. By incorporating these worksheets into ...
236. $2.50. PDF. Simply print, cut, and use! These 40 cards are perfect for practicing multistep word problem solving skills. The cards can be used as an around the room scavenger hunt, desk scoot game, or simply as a stack of task cards in a math center for partner review. Could also be used as an assessment if s.
Challenge Year 6 children to choose the correct operations and solve these real-life puzzles.Complete with eight tricky multi-step word problems, this teacher-made worksheet is a quick and easy way of reinforcing your teaching and gauging the progress of your students. The worksheet is jam packed with topics that are essential to the completion of the Australian Curriculum. For example ...
In this lesson you will learn how to solve multi-step problems involving multiplication and addition or subtraction of decimals, mixed decimals and whole nu...
Multi-Step Word Problems worksheets are an essential tool for teachers looking to challenge their students in the realm of Math. These worksheets provide a variety of Math Word Problems that require students to use critical thinking and problem-solving skills to find the solution. By incorporating multiple steps, these worksheets help students ...
Welcome to the math word problems worksheets page at Math-Drills.com! On this page, you will find Math word and story problems worksheets with single- and multi-step solutions on a variety of math topics including addition, multiplication, subtraction, division and other math topics. It is usually a good idea to ensure students already have a strategy or two in place to complete the math ...
These worksheets contain simple multiplication word problems. Students derive a multiplication equation from the word problem, solve the equation by mental multiplication and express the answer in appropriate units. Students should understand the meaning of multiplication before attempting these worksheets. Worksheet #1 Worksheet #2 Worksheet ...
These worksheets offer a structured approach to mastering two-step problem-solving, integrating addition, subtraction, division, and multiplication. Varied Problem Types: Each worksheet presents a range of two-step problems, ensuring students encounter diverse scenarios that require different combinations of operations.
The superintendent and county math coaches were thrilled with the high levels of engagement they saw in the classrooms, as well as the significant changes in state test scores. There are several problems with the systems of mathematics education in many states, and proposed solutions often spark disagreement. But perhaps we should all agree on ...
Teachers, Use for Free. 4413+. 4567+. Help your child become an expert in multiplication with this worksheet. Young learners will make connections between math and the real world as they solve a set of multi-step word problems involving a variety of scenarios. In these problems, they comprehend the scenarios and find the final answer.
When using it to solve a combinatorial optimization problem, we usually consider it a multi-step decision problem. Making an optimal decision at each step should lead to the optimal solution for the overall problem. ... is the element-wise multiplication. ... Zhang L, Chen Z, Tang J (2021) Learning to iteratively solve routing problems with ...
Worksheets Math Problems "Two-step Addition, Subtraction, Division & Multiplication Worksheets Problems" is a comprehensive resource designed to strengthen students' proficiency in fundamental arithmetic operations. These worksheets offer a structured approach to mastering two-step problem-solving, integrating addition, subtraction ...