
An official website of the United States government
The .gov means it’s official. Federal government websites often end in .gov or .mil. Before sharing sensitive information, make sure you’re on a federal government site.
The site is secure. The https:// ensures that you are connecting to the official website and that any information you provide is encrypted and transmitted securely.
- Publications
- Account settings
- My Bibliography
- Collections
- Citation manager
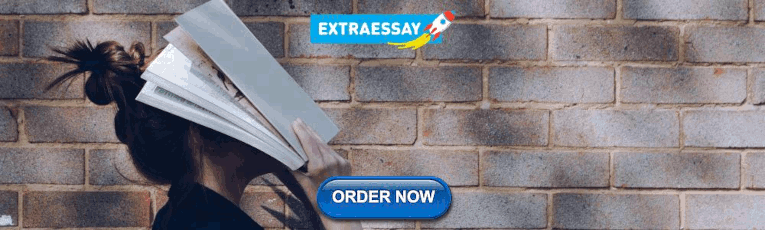
Save citation to file
Email citation, add to collections.
- Create a new collection
- Add to an existing collection
Add to My Bibliography
Your saved search, create a file for external citation management software, your rss feed.
- Search in PubMed
- Search in NLM Catalog
- Add to Search
X-ray diffraction: instrumentation and applications
Affiliation.
- 1 a SCIENT by CROMATEC_PLUS SRL, Research Center for Instrumental Analysis , Bucharest , Romania.
- PMID: 25831472
- DOI: 10.1080/10408347.2014.949616
X-ray diffraction (XRD) is a powerful nondestructive technique for characterizing crystalline materials. It provides information on structures, phases, preferred crystal orientations (texture), and other structural parameters, such as average grain size, crystallinity, strain, and crystal defects. X-ray diffraction peaks are produced by constructive interference of a monochromatic beam of X-rays scattered at specific angles from each set of lattice planes in a sample. The peak intensities are determined by the distribution of atoms within the lattice. Consequently, the X-ray diffraction pattern is the fingerprint of periodic atomic arrangements in a given material. This review summarizes the scientific trends associated with the rapid development of the technique of X-ray diffraction over the past five years pertaining to the fields of pharmaceuticals, forensic science, geological applications, microelectronics, and glass manufacturing, as well as in corrosion analysis.
Keywords: X-ray diffraction; applications; theory.
PubMed Disclaimer
Similar articles
- Quantitative analysis of crystalline pharmaceuticals in tablets by pattern-fitting procedure using X-ray diffraction pattern. Takehira R, Momose Y, Yamamura S. Takehira R, et al. Int J Pharm. 2010 Oct 15;398(1-2):33-8. doi: 10.1016/j.ijpharm.2010.07.043. Epub 2010 Jul 30. Int J Pharm. 2010. PMID: 20674727
- Strain measurement of pure titanium covered with soft tissue using X-ray diffraction. Fujisaki K, Tadano S. Fujisaki K, et al. J Biomech Eng. 2010 Mar;132(3):031004. doi: 10.1115/1.4000935. J Biomech Eng. 2010. PMID: 20459192
- Observation of divergent-beam X-ray diffraction from a crystal of diamond using synchrotron radiation. Glazer AM, Collins SP, Zekria D, Liu J, Golshan M. Glazer AM, et al. J Synchrotron Radiat. 2004 Mar 1;11(Pt 2):187-9. doi: 10.1107/S0909049504000949. Epub 2004 Feb 12. J Synchrotron Radiat. 2004. PMID: 14960784
- Phase recovery and lensless imaging by iterative methods in optical, X-ray and electron diffraction. Spence JC, Weierstall U, Howells M. Spence JC, et al. Philos Trans A Math Phys Eng Sci. 2002 May 15;360(1794):875-95. doi: 10.1098/rsta.2001.0972. Philos Trans A Math Phys Eng Sci. 2002. PMID: 12804284 Review.
- Applications of Powder X-Ray Diffraction in Small Molecule Pharmaceuticals: Achievements and Aspirations. Thakral NK, Zanon RL, Kelly RC, Thakral S. Thakral NK, et al. J Pharm Sci. 2018 Dec;107(12):2969-2982. doi: 10.1016/j.xphs.2018.08.010. Epub 2018 Aug 23. J Pharm Sci. 2018. PMID: 30145209 Review.
- Evaluation of cytotoxic potential of silver nanoparticles biosynthesized using essential oils of Jasminum sambac against breast cancer and bacterial cells. Bidan AK, Al-Ali ZSA. Bidan AK, et al. 3 Biotech. 2024 Oct;14(10):227. doi: 10.1007/s13205-024-04058-8. Epub 2024 Sep 10. 3 Biotech. 2024. PMID: 39268412
- Application of synthesized metal-trimesic acid frameworks for the remediation of a multi-metal polluted soil and investigation of quinoa responses. Zarrabi A, Ghasemi-Fasaei R, Ronaghi A, Zeinali S, Safarzadeh S. Zarrabi A, et al. PLoS One. 2024 Sep 6;19(9):e0310054. doi: 10.1371/journal.pone.0310054. eCollection 2024. PLoS One. 2024. PMID: 39240855 Free PMC article.
- Structure-based pharmacophore modeling for precision inhibition of mutant ESR2 in breast cancer: A systematic computational approach. Islam S, Amin MA, Rengasamy KRR, Mohiuddin AKM, Mahmud S. Islam S, et al. Cancer Med. 2024 Aug;13(15):e70074. doi: 10.1002/cam4.70074. Cancer Med. 2024. PMID: 39101505 Free PMC article.
- Keratin from Animal By-Products: Structure, Characterization, Extraction and Application-A Review. Banasaz S, Ferraro V. Banasaz S, et al. Polymers (Basel). 2024 Jul 12;16(14):1999. doi: 10.3390/polym16141999. Polymers (Basel). 2024. PMID: 39065316 Free PMC article. Review.
- NANO.PTML model for read-across prediction of nanosystems in neurosciences. computational model and experimental case of study. He S, Nader K, Abarrategi JS, Bediaga H, Nocedo-Mena D, Ascencio E, Casanola-Martin GM, Castellanos-Rubio I, Insausti M, Rasulev B, Arrasate S, González-Díaz H. He S, et al. J Nanobiotechnology. 2024 Jul 23;22(1):435. doi: 10.1186/s12951-024-02660-9. J Nanobiotechnology. 2024. PMID: 39044265 Free PMC article.
Publication types
- Search in MeSH
LinkOut - more resources
Full text sources.
- Taylor & Francis
Other Literature Sources
- The Lens - Patent Citations
Miscellaneous
- NCI CPTAC Assay Portal
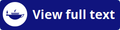
- Citation Manager
NCBI Literature Resources
MeSH PMC Bookshelf Disclaimer
The PubMed wordmark and PubMed logo are registered trademarks of the U.S. Department of Health and Human Services (HHS). Unauthorized use of these marks is strictly prohibited.
Thank you for visiting nature.com. You are using a browser version with limited support for CSS. To obtain the best experience, we recommend you use a more up to date browser (or turn off compatibility mode in Internet Explorer). In the meantime, to ensure continued support, we are displaying the site without styles and JavaScript.
- View all journals
- Explore content
- About the journal
- Publish with us
- Sign up for alerts
- Open access
- Published: 17 May 2019
Fast and interpretable classification of small X-ray diffraction datasets using data augmentation and deep neural networks
- Felipe Oviedo ORCID: orcid.org/0000-0001-9480-6539 1 na1 ,
- Zekun Ren 2 na1 ,
- Shijing Sun 1 ,
- Charles Settens 1 ,
- Zhe Liu 1 ,
- Noor Titan Putri Hartono ORCID: orcid.org/0000-0002-0748-0620 1 ,
- Savitha Ramasamy 3 ,
- Brian L. DeCost 4 ,
- Siyu I. P. Tian ORCID: orcid.org/0000-0002-8709-1798 1 ,
- Giuseppe Romano 1 ,
- Aaron Gilad Kusne 4 &
- Tonio Buonassisi 1 , 2
npj Computational Materials volume 5 , Article number: 60 ( 2019 ) Cite this article
24k Accesses
196 Citations
19 Altmetric
Metrics details
- Characterization and analytical techniques
- Computational methods
- Synthesis and processing
X-ray diffraction (XRD) data acquisition and analysis is among the most time-consuming steps in the development cycle of novel thin-film materials. We propose a machine learning-enabled approach to predict crystallographic dimensionality and space group from a limited number of thin-film XRD patterns. We overcome the scarce data problem intrinsic to novel materials development by coupling a supervised machine learning approach with a model-agnostic, physics-informed data augmentation strategy using simulated data from the Inorganic Crystal Structure Database (ICSD) and experimental data. As a test case, 115 thin-film metal-halides spanning three dimensionalities and seven space groups are synthesized and classified. After testing various algorithms, we develop and implement an all convolutional neural network, with cross-validated accuracies for dimensionality and space group classification of 93 and 89%, respectively. We propose average class activation maps, computed from a global average pooling layer, to allow high model interpretability by human experimentalists, elucidating the root causes of misclassification. Finally, we systematically evaluate the maximum XRD pattern step size (data acquisition rate) before loss of predictive accuracy occurs, and determine it to be 0.16° 2 θ , which enables an XRD pattern to be obtained and classified in 5.5 min or less.
Similar content being viewed by others
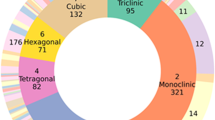
Automated classification of big X-ray diffraction data using deep learning models
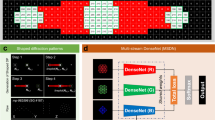
Identification of crystal symmetry from noisy diffraction patterns by a shape analysis and deep learning
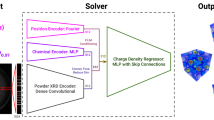
Towards end-to-end structure determination from x-ray diffraction data using deep learning
Introduction.
High-throughput material synthesis and rapid characterization are necessary ingredients for inverse design and accelerated material discovery. 1 , 2 X-ray diffraction (XRD) is a workhorse technique to determine crystallography and phase information, including lattice parameters, crystal symmetry, phase composition, density, space group, and dimensionality. 3 This is achieved by comparing XRD patterns of candidate materials with the measured or simulated XRD patterns of known materials. 4 Despite its indispensable utility, XRD is a common bottleneck in materials characterization and screening loops: 1 hour is typically required for XRD data acquisition with high angular resolution, and another 1–2 hours are typically required for Rietveld refinement by an expert crystallographer, assuming the possible crystalline phases are known. It is widely recognized that machine learning methods have potential to accelerate this process; however, practical implementations have thus far focused on well-established materials, 5 , 6 , 7 require combinatorial datasets spanning among various phases, 8 , 9 or require large datasets, 5 , 10 whereas material screening using the inverse design paradigm often involves less-studied materials, spanning multiple classes of different material/phase compositions, and smaller prototype datasets.
Typically, experimental XRD pattern data are analyzed by obtaining descriptors such as peak shape, height, and position. Matching descriptors of the test pattern to known XRD patterns in crystalline databases allows the identification of the compound of interest. 4 Refinement methods such as Rietveld refinement and Pawley refinement have been used for decades to analyze experimental XRD patterns. 4 For novel compounds in thin-film form, however, the use of Rietveld refinement is limited due to the lack of reference patterns in material databases, as well as unknown film textures. The direct-space method, statistical methods, and the growth of single crystals have been used to obtain crystal symmetry information for novel materials, 7 , 11 , 12 , 13 , 14 but the significant iteration time, feature engineering, human expertise, and knowledge of specific material required makes these methods impractical for high-throughput experimentation, where sample characterization rates are of the order of one material per minute or faster, 2 , 15 explored over various material families.
An alternative approach consists in using machine learning methods to obtain more robust spectral descriptors and quickly classify crystalline structure based on peak location and shape in the XRD pattern. Breakthrough methods have been developed for the similar problem of phase attribution in combinatorial alloys, 16 , 17 but only few studies have been developed for solution-processed material screening, such as perovskite screening, where phase attribution is usually not as important as correct classification of materials into groups according to crystal parameters. The most successful methods 10 , 18 for material screening use convolutional neural networks (CNNs) trained with hundreds of thousands of XRD powder patterns simulated with data from the Inorganic Crystalline Structure Database (ICSD). Further CNN and other deep learning algorithms have been employed to obtain crystalline information for other kinds of diffraction data. 19 , 20 , 21 In a couple studies, noise-based data augmentation, a common technique of image preprocessing for machine learning, has been used to avoid overfitting in a broader kind of X-ray characterization problems 20 , 22 , 23 and more broadly in other fields such as Tramission Electron Microscopy imaging; 21 however, the augmentation procedure has not been based in physical knowledge of actual experimental samples. Furthermore, the best-performing machine learning methods developed up-to-date for XRD analysis do not allow any kind of interpretation by the experimentalists, 10 hindering improvements of experimental design.
While similar approaches produce good results for crystal structure classification, we have found that applying them to high-throughput characterization of novel solution-processed compounds is generally not practical, given the limited access to large datasets of clean, preprocessed, relevant, XRD spectra. Furthermore, most materials of interest developed in high-throughput synthesis loops are thin-film materials. The preferred orientation of the crystalline planes in thin-films causes their experimental XRD patterns to differ from the thousands of simulated XRD powder patterns available in most databases. 24 , 25 Thin-film compounds usually will present spectrum shifting and periodic scaling of peaks in preferred orientations, reducing the accuracy of machine learning models trained with powder data, 8 , 9 , 26 even in the cases when noise-based data augmentation techniques are used. 18
Considering these challenges, we propose a supervised machine learning framework for rapid crystal structure identification of novel materials from thin-film XRD measurements. For this work, we created a library of 164 XRD patterns of thin-film halide materials extracted from the >100,000 compounds available in the ICSD 27 ; these 164 XRD patterns include lead halide perovskite 28 , 29 and lead-free perovskites-inspired materials. 30 These XRD patterns were manually classified among different crystal dimensionalities using ICSD information. Based on this small dataset of relevant XRD powder patterns extracted from the ICSD and an additional 115 experimental XRD patterns, we propose a model-agnostic, physics-informed data augmentation to generate a suitable and robust training dataset for thin-film materials, and subsequently test the space group and dimensionality classification accuracy of multiple machine learning algorithms. A one-dimensional (1D) implementation of an “ all convolutional neural network ” (a-CNN) 31 is proposed, implemented and identified as the most accurate and interpretable classifier for this problem. We propose a new way to use class activation maps (CAMs), 32 computed from the weight distribution of a global average pooling layer and adapted to the context of our problem, to provide interpretability of root causes of classification success or failure to the experimentalist. Subsequently, the effect of the augmented dataset size and the XRD pattern granularity is investigated. Our proposed methodology could be applied to other crystal descriptors of thin-film materials, such as lattice parameters or atomic coordinates, as long as labeled information is available.
Our contributions can be summarized as: (a) development of physics-informed data augmentation for thin-film XRD, which successfully addresses the sparse/scarce data problem, breaching the gap between the thousands of XRD patterns in crystalline databases and real thin-film materials, (b) development of highly interpretable, highly accurate, a-CNN for XRD material screening, (c) proposal of average class activation maps as a feasible interpretability tool in CNNs trained on spectral data.
Results and discussion
Framework for rapid xrd classification.
The framework developed for rapid classification of XRD thin-film patterns according to crystal descriptors is shown in Fig. 1a . The methodology makes use of both experimental and simulated XRD patterns to train a machine learning classification algorithm. A simulated dataset is defined by extracting crystal structure information from the ICSD. The experimental dataset consists of a set of synthesized samples, which are manually labeled for training and testing purposes. The datasets are subjected to data augmentation based on the three spectral transformations shown in Fig. 2 .
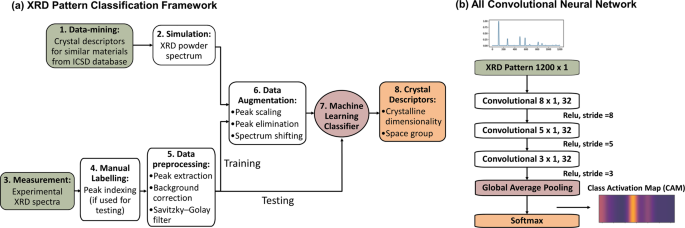
General framework and a-CNN architecture. a Schematic of our X-ray diffraction data classification framework, with physics-informed data augmentation. b Schematic of the best-performing algorithm, our all convolutional neural network
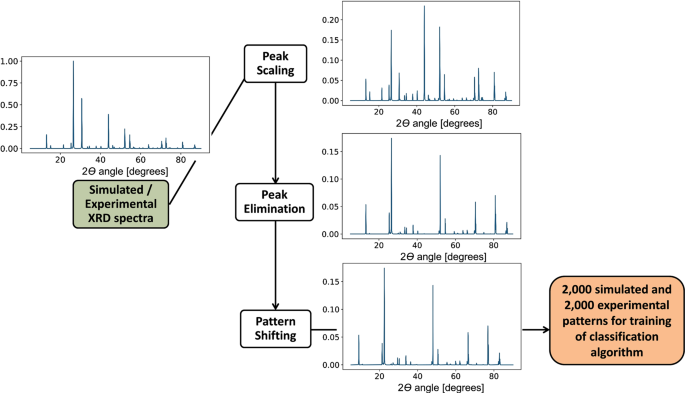
Physics-informed data augmentation: schematic of the physics-informed data augmentation algorithm, which accounts for the particularities of thin-film XRD spectra, as described by the subsequent transformations stated in Eqs. 1 – 3
The crystal descriptors of interest, space group and crystal dimensionality , are chosen because of their importance for material screening in accelerated material development. In many inorganic material systems, the crystalline dimensionality—i.e., a generalization of the crystalline symmetry into 0-dimensional (0D), 1D, 2D, or 3D symmetry— constitutes a figure of merit for experimental material screening as it correlates with observed charge-transport properties. 33 In perovskites and perovskites-inspired materials, for instance, 3D crystalline structures have been shown to have good carrier-transport properties for solar cells and light-emitting diode applications, 33 , 34 whereas 3D–2D mixtures have been found to have greater stability in lead halide perovskites than pure-phase 3D crystals. 35 With further detail, the space group number describes the standardized symmetry group of a configuration in space, classifying crystal symmetries into 230 groups. Identifying the space group number of a sample provides crystal information beyond dimensionality, including atomic bonding angles and relative distances, which are believed to be of importance for predicting material properties. 36 In this specific study, the framework relies on the relation between XRD patterns and the crystal descriptors of interest. For example, among perovskite-inspired materials for photovoltaic applications, 3D cubic lead halide perovskites of multiple compositions show distinct features in their XRD patterns compared with 2D layered bismuth perovskites. 37 , 38
Typically, the powder XRD pattern is used to identify space group through Rietveld refinement, but the compression of crystalline 3D crystallographic information into a 1D diffraction pattern causes the space group to be impossible to determine unambiguously in certain low-symmetry phases, independently of the measurement technique. 39 In this work, the space groups of interest are able to be determined from XRD information only.
To better account for noise measurement and the physical difference between randomly oriented powder patterns and experimental thin-film patterns, the patterns were subjected to a process of data augmentation based on domain knowledge. Subsequently, both augmented experimental and simulated XRD pattern datasets are used for testing, training and cross-validation of machine learning algorithms.
Figure 1b shows the architecture of the final a-CNN, which is proposed and identified as the best-performing machine learning algorithm in subsequent sections.
Experimental measurement and labeling of XRD patterns
The experimental dataset consists of 75 XRD patterns for dimensionality classification, summarized in Supplementary Table S1 , and 88 XRD patterns for space group classification, summarized in Supplementary Table S2 . A total of 115 unique labeled XRD patterns are considered among both datasets. For this work, perovskites-inspired 3D materials based on lead halide perovskites (space group \(Pm\bar 3m\) ), tin halide perovskite ( I 4/ mcm ), cesium silver bismuth bromide double perovskite ( \(Fm\bar 3m\) ), bismuth and antimony halide 2D ( \(P\bar 3m1\) , \({\it Pc}\) , \({P2}_{1}{\!/\!a}\) ), and 0D ( P 6 3 / mmc ) perovskite-inspired materials are synthesized and used as training and testing dataset. The details of the synthesis and characterization methodology are described in great detail in our experimental study. 40 Supplementary Fig. S1 shows the t-Distributed Stochastic Neighbor Embedding representation of the XRD patterns labeled with dimensionality and space group, providing evidence of the complexity of the classification problem.
In this case, Rietveld refinement is restricted due to the unknown preferred orientation and texture of the thin-film samples. In consequence, the XRD patterns are subjected to peak indexing and the dimensionality and space group are labeled based on ICSD data.
Data mining and simulation of XRD patterns
The simulated training dataset consists of 164 compounds extracted from ICSD with a similar composition, expected crystal symmetry, and space group as the synthesized materials of interest. All the possible single, double, ternary, and quaternary combinations of the elements of interest were extracted during database mining. The compositions of all the materials of interest along with labeled dimensionality and space group information is available in Supplementary Table S3 . The fundamental crystal descriptors extracted from the material database are used to simulate XRD powder patterns with random crystalline orientations, as explained in the Methods section.
Data augmentation based on domain knowledge
Experimental thin-film XRD patterns vary greatly compared to simulated, idealized, randomly oriented XRD patterns. Due to expansions and contractions in the crystalline lattice, XRD peaks shift along the 2 ϴ axis according to the specific size and location of the different elements present in a compound, while maintaining similar periodicity based on crystal space group. 3 , 8 , 26 , 41 In addition, for thin-film samples, the XRD pattern can be shifted due to strain in the film induced during the fabrication process. 42 Polycrystalline thin-films are also known to have preferred orientations along certain crystallographic planes. The preferred orientation is influenced by the crystal growth process and the growth substrate, 24 and is common for most solution-processing and vapor-deposition fabrication methods. Ideal random powders contain multiple grains without any preferred global orientations, thus all crystallographic orientations are represented evenly in the peak intensity and periodicity of the XRD pattern. As a consequence of their preferred orientations along crystallographic planes, thin-film XRD relative peak intensities are scaled up periodically in the preferred plane orientation, and scaled down periodically or even eliminated in the non-preferred orientations.
To increase the size and robustness of the limited training dataset and to account for these fundamental differences between real thin-films and simulated XRD powder patterns, we perform a three-step data augmentation procedure based on physical domain knowledge:
( 1 ) Peak scaling, ( 2 ) Peak elimination, and ( 3 ) Pattern shifting. These transformation are described in detail in Methods. Figure 2 summarizes the data augmentation steps and its effects on a representative pattern. Given the hyperparameters S and ε in Eqs. 1 – 3 in the Methods section, 2000 patterns are augmented from the simulated dataset, and 2000 patterns are augmented from experimentally measured spectra.
We choose to perform physics-informed data augmentation instead of explicit regularization for the following reasons: ( 1 ) data augmentation has been found to be more robust at avoiding overfitting than explicit regularization when using convolutional neural networks, 43 ( 2 ) data augmentation is model-agnostic, allowing our approach to successfully bridge the gap between experimental XRD patterns and thousands of XRD patterns available in databases without depending on a specific model that might not generalize well in all cases ( no-free-lunch theorem ), and ( 3 ) physics-informed data augmentation allows high interpretability, and is found to be more robust than traditional noise-based data augmentation approaches (Supplementary Table S6 ).
Classification results and a-CNN
Preprocessed, augmented experimental data, and augmented simulated data are fed into various supervised machine learning algorithms for training and testing purposes. The best-performing algorithm is evaluated. The XRD patterns are classified into three crystal dimensionalities (0D, 2D, and 3D) and seven space groups ( \(Pm\bar 3m\) , I 4/ mcm , \(Fm\bar 3m,P\bar 3m1\) , \({Pc}\) , P 2 1 / a , and P 6 3 / mmc ).
For this purpose, we represent the XRD pattern as either a vector or a time series. For each kind of data representation, different classification algorithms are considered. Using a vector representation of the XRD pattern, the following classification methods are tested: Naive Bayes, k -Nearest Neighbors, Logistic Regression, Random Forest, Decision Trees, Support Vector Machine, Gradient Boosting Decision Trees, a Fully Connected Deep Neural Network, and an a-CNN with a global pooling layer. 44 , 45 , 46 The XRD patterns are also analyzed as a time series with a normalized Dynamic Time Warping (DTW) distance metric 47 combined with a k -Nearest Neighbors classification algorithm, which was found in literature as the most adequate metric for measuring similarity among metal-alloy XRD spectra. 7 , 26
The problem of novel material development is inherently a multi-class classification problem, in which the classes for training and testing purposes can often be imbalanced as some material families are better characterized than others (e.g., lead-based perovskites are better represented in material databases than newer lead-free perovskites). 38 Common metrics for binary classification such as accuracy might not be the most adequate in this context. 45 The final choice for adequate metrics depends on the relative importance of false positive and false negatives in minority and majority classes, according to the goals of the experimentalist. For method development in this work, we consider the following metrics: subset accuracy , defined as the number of correctly classified patterns among all test patterns, and F 1 score. F 1 score in this problem can be interpreted as the weighted harmonic mean of precision and recall; the closer it is to 1.0, the higher the classifier’s precision and recall. 45 Intuitively, precision is the ability of the classifier not to produce a false positive, whereas recall is the ability of a classifier to find all the true positives. An F 1 metric is calculated for each class label, and it is combined into an overall score by taking either the micro or the macro average of the individual scores. The macro average calculates the mean of the metrics of all the individual classes, hence treating all classes equally. The micro average adds the individual contribution of all samples to compute the overall metric.
When there is class imbalance, accuracy and F 1 micro score characterize the classifier’s performance over all classes, whereas F 1 macro emphasizes the accuracy on infrequent classes. 45 Thus, a natural choice for high-throughput experiments across multiple material classes seems to be accuracy/F 1 micro score, except in those cases when we are especially interested in analyzing an infrequent material class, being F 1 macro a more representative metric in that case. In this work, we choose to report the classification accuracy and both F 1 micro and F 1 macro , whereas recall and precision results are included in the Supplementary Information (Supplementary Tables S4 and S5 ).
We measure the performance of the dimensionality and space group classification methods based on three different approaches of splitting the training and testing datasets:
Case 1: Exclusively simulated XRD patterns are used for testing and training. Fivefold cross-validation is performed.
Case 2: The simulated XRD patterns are used for training, and the experimental patterns for known materials are used for testing.
Case 3: All of the simulated data and 80% of the experimental data are used for training, and 20% of the experimental data are used for testing. Fivefold cross-validation is performed.
Each one of the training/testing cases mentioned earlier are tested for crystal dimensionality and space group prediction accuracy and micro / macro F 1 score. The results are reported in Table 1 . In each cell, the crystal dimensionality classification metric is reported first, followed by the metric for space group classification. Case 1, presenting fivefold cross-validation results of the simulated dataset, has the highest accuracy as it does not predict any experimental data and thus is free of experimental errors for both crystal descriptors. Case 2 performs the experimental prediction solely based on simulated patterns, thus having the lowest accuracy. Finally, Case 3 has a significant higher accuracy than Case 2 for both crystal dimensionality and space group prediction. F 1 scores follow these trends as well.
In general, the model’s accuracy and F 1 score is lower for space group classification than that for crystal dimensionality classification. This discrepancy is caused by the lower number of per-class labeled examples for space group classification compared to crystal dimensionality classes. Class imbalance can also systematically affect the training performance of the classifier, to avoid this issue, we performed an oversampling test with synthetic training data according to, 48 , 49 and observed little discrepancy of accuracy between the balanced and imbalanced datasets after fivefold cross-validation.
The use of experimental data as part of the training set increases the model’s accuracy and robustness. This fact can be explained by the high variability of experimental thin-film XRD patterns, even after data preprocessing. The relatively high accuracy with the relatively small number of experimental samples (on the order of 10–10 2 ) confirms the potential of our data augmentation strategy to yield high predictive accuracies even with small datasets. Supplementary Table S6 in the Supplementary Information compares our strategy with traditional noise-based augmentation approaches, and shows an average increase of classification accuracy of >12% absolute.
Naturally, the F 1 macro score is systematically lower than the F 1 micro score, reflecting the impact of misclassification of those dimensionality and space group classes with less training examples. However, the F 1 macro score is still fairly high for most classifiers. This fact reflects the importance of adequate experimental design to achieve good generalization among classes.
For all three test cases, the a-CNN classifier performs better than any other classification technique. The 1D a-CNN architecture implemented is composed of three 1D convolutional layers, with 32 filters each, and strides and kernel sizes of 8, 5, and 3 units, respectively. The activation function between layers is ReLu . A global average pooling layer 50 (acting as a weak regularizer) and a final dense layer with softmax activation is used. The loss function minimized is binary cross entropy. We use early stopping with a batch size of 128 during training, and use the Adam optimizer algorithm to minimize the loss function. The CNN is implemented in Keras 2.2.1 with the Tensorflow background. Figure 1b contains a schematic of the proposed a-CNN architecture.
Our a-CNN architecture, in contrast with other CNNs, does not have max pooling layers between convolutional layers, and also lacks a set of dense layers in the final softmax classification layer. These modifications, in contrast with the architectures used in, 10 , 18 significantly reduces the number of parameters in the neural network, allows faster and simpler training, and are less prone to overfitting. Another advantage of our implementation is the possibility to extract CAMs from the weight distribution of the global average pooling layer. This characteristic of the network, properly adapted to our problem, allows us to visualize how the classified XRD patterns are mapped to the weight distribution at the end of the a-CNN. The results are further discussed in the interpretability subsection.
The a-CNN trained after data augmentation has an accuracy of >93 and 89% for crystal dimensionality and space group classifications, respectively. As far as we know, the accuracy is among the highest described in literature for space group classification algorithms, comparable to those trained with thousands of ICSD patterns and manual labeling by human experts, 10 , 51 and is also comparable to similar approaches in other kinds of diffraction data. 8 , 19 The neural network seems to be the most adequate method for high-throughput synthesis and characterization loops, as it also performs relatively well in terms of algorithm speed and in conditions of class imbalance. In the future, our methodology can be extended to other materials systems, and may include other crystal descriptors as predicted outputs, such as lattice parameters and atomic coordinates.
Furthermore, the a-CNN performs better than the traditional k -nearest neighbors method using DTW. In our test case and dataset, the differences between thin-film and powder spectra seem not to be captured properly by DTW alone. Arguably, DTW could be more useful if a larger XRD thin-film pattern dataset is available for k -Nearest Neighbors classification, or if it exists greater similarity between XRD patterns of the same class, allowing the DTW warping path to be better captured within the DTW window under consideration. 26 CNNs have been found to perform better than DTW for classification of time series, which is consistent with our results. 52
Effect of augmented dataset size
The size of the dataset is critical for obtaining a high accuracy and F 1 score. To explore the effect of augmented dataset size, the a-CNN accuracy was computed for various combinations of augmented experimental patterns (i.e., number of augmented XRD spectra originated from the 88 measured spectra, varying S and ε in Eqs. 1 – 3 ) and augmented simulated dataset sizes (i.e., number of augmented XRD spectra originating from the 164 simulated ICSD spectra). Figure 3 a, b summarizes this sensitivity analysis for Case 3 training/testing conditions for dimensionality and space group classification. Twenty different fivefold cross-validation runs were performed to calculate the 1-standard deviation error bars for each data point.
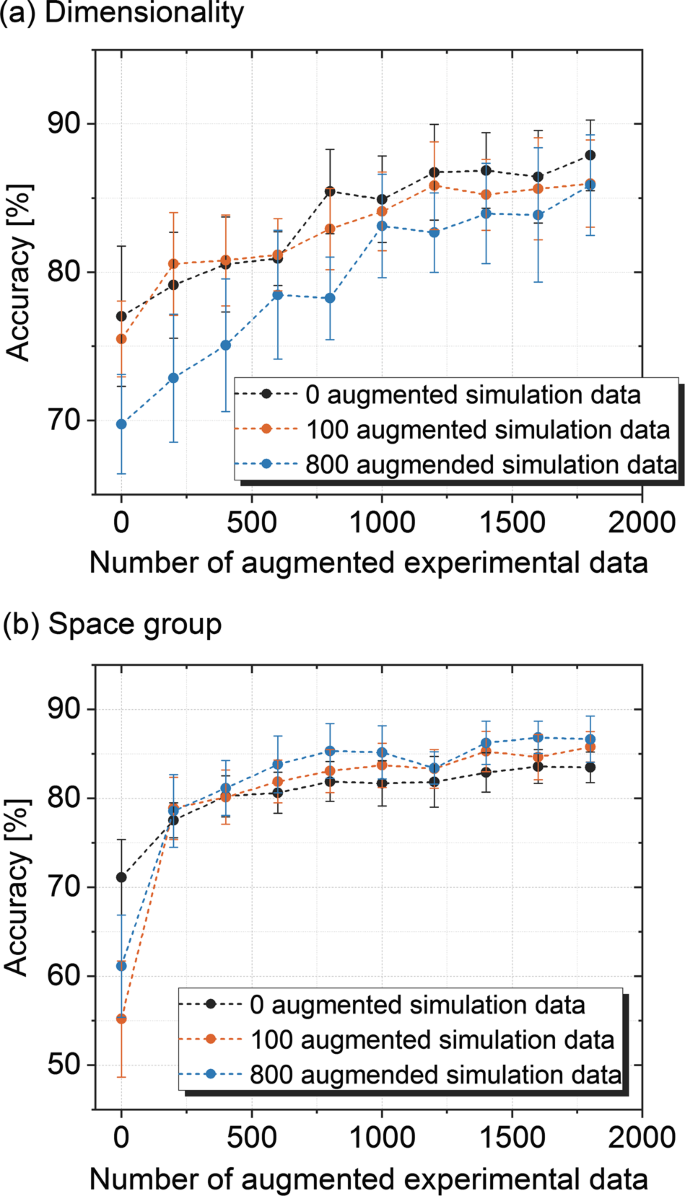
Accuracy of the a-CNN model with augmented data: line plot showing mean Case 3 a-CNN accuracy, as a function of the number of augmented patterns (Methods, Eqs. 1 – 3 ) included in the training set, for ( a ) dimensionality classification and ( b ) space group classification. The x axis shows augmented experimental data (based on the original experimental XRD patterns), and the legend shows simulated data (based on the 164 simulated powder diffraction patterns obtained from the ICSD). The error bars correspond to one standard deviation from the mean
In general, as the size of the experimental and augmented datasets increase, the mean accuracy quickly approaches the asymptotic accuracy reported in Table 1 . This trend reaffirms and quantifies the importance of data augmentation for the predictive accuracy of our model.
The critical augmented dataset size seems to be around 700 augmented spectra. The model’s accuracy is more sensitive to the augmented experimental dataset size, likely because most of the dataset variance comes from the experimental XRD patterns. The data augmentation of the simulated dataset causes the accuracy to grow monotonically in Fig. 3b ; however, this trend is not satisfied in the case of Fig. 3a , where no augmented simulated data seems on average to perform the best. We hypothesize that augmenting simulated data could actually introduce excessive noise to the model, hampering classification when the number of possible classes is small.
Figure 3 illustrates that if no data augmentation is used (i.e., the origin, 0, 0), the predictive accuracy could be below 50% for space group and below 70% for dimensionality. Our physics-informed data augmentation directly increase accuracy by up to 23% in the case of dimensionality and 19% in the case of space group classification. This result reinforces the need for data augmentation for sparse/scarce datasets, as is typical with early-stage material development.
Impact of data coarsening
To evaluate the trade-offs between accuracy and XRD acquisition speed, we investigate how data coarsening of the XRD pattern impacts the accuracy of ML algorithm prediction. In Fig. 4 , we report Case 3 accuracy with increasing 2 ϴ angle step size. The baseline step size of the 2 ϴ scan in our XRD patterns is 0.04°. Data coarsening is performed by selectively removing the data with increasing step sizes and rerunning the augmentation and classification algorithms. For crystal dimensionality and space group classification, the highest accuracies are achieved at 0.04–0.08°, whereas 85%+ accuracy is achieved when the 2 ϴ step size is 0.16° or less for both cases. Using the larger step size, the XRD pattern acquisition time can be reduced by 75%, allowing the full spectra to be measured and classified in <5.5 min with our tool setup.
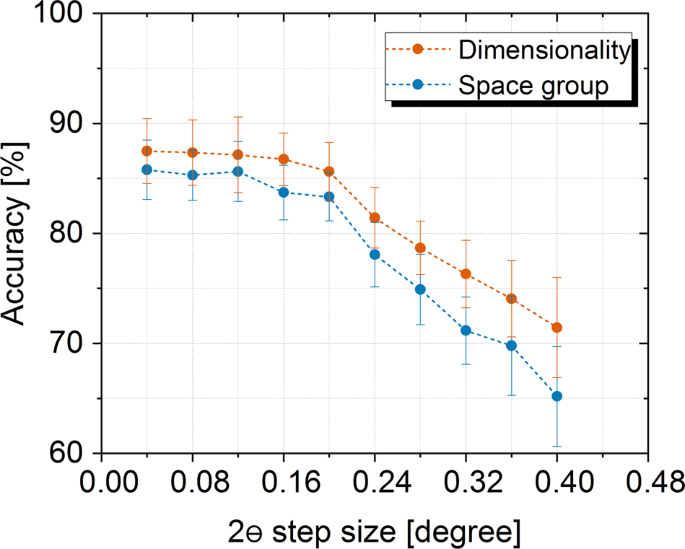
Reducing XRD pattern acquisition time: simulation of the trade-off between XRD pattern acquisition rate and predictive accuracy. Accuracies for crystal dimensionality and space group predictions are estimated by coarsening the XRD spectrum 2 ϴ step size for Case 3 conditions. The error bars correspond to one standard deviation from the mean
Interpretability using CAMs
Class activation maps (CAM) are representations of the weights in the last layer of a CNN, before performing classification. A CAM for a certain class and pattern indicates the main discriminative regions (in our case, peak and series of peaks in the XRD pattern) that the network uses to identify that class. 32 Details of the CAM computation are included in the Methods section.
A similar approach has been followed to interpret and improve object recognition in images and videos using 2D CNNs. 32 A single pattern or image produces a unique CAM revealing the main discriminative features. In addition, in the context of our problem, we propose to generalize CAMs to all training samples within a class by averaging over each of the computed CAM weights for all training samples within a class, as explained in the Methods section. This averaging procedure is justified as the location and periodicity of discriminative peaks within a class varies only slightly along all labeled samples in the training set, and can be extended to many spectral measurement techniques. This average CAM allows to visualize the main discriminative features of an XRD pattern that were used to classify all the training data belonging to a certain class.
By comparing the CAM for a single pattern with the average CAM for given class, we can identify the root causes of correct and incorrect classification by the a-CNN. Figure 5 illustrates this procedure for XRD patterns of space group classes Class 2 ( P 2 1 / a ) and Class 6 ( \(Pm\bar 3m\) ). Figure 5a, b shows the average CAM maps of Class 6 and Class 2 space groups. Figure 5c shows the CAM of an individual, correctly classified XRD pattern. If we compare the individual CAM with Class 6 Average CAM, we can see that the neural network is identifying the same reference pattern as most of Class 6 samples, which translates into classification as Class 6. In contrast, Fig. 5d shows an incorrectly classified XRD pattern, which was determined to belong to Class 6 by the CNN, when in reality it belongs to Class 2. A comparison of the individual CAM with the average CAMs of classes 6 and 2 reveals that the misclassified CAM is more similar to Fig. 5a than Fig. 5b . A closer look to the misclassified patterns, shows that the periodicity of peaks before 30° and the relative lack of peaks between 30° and 50° (likely caused by mixed phases), are causing the misclassification.
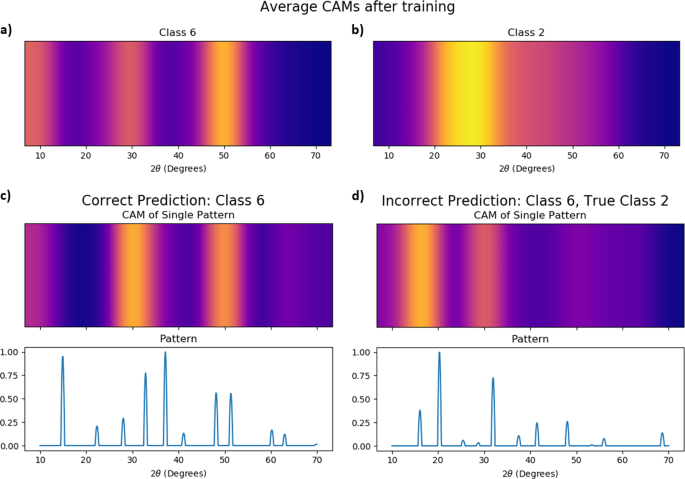
Class activation maps for misclassification interpretability: class activation maps (CAMs) generated by the a-CNN architecture, representing space group classification of Class 2 ( P 2 1 / a ) and Class 6 ( \(Pm\bar 3m\) ). ( a , b ) correspond to maps generated by averaging all training samples in a certain class, whereas ( c , d ) correspond to the CAMs of correctly classified and incorrectly classified individual patterns, respectively. The correctly predicted pattern of ( c ) is explained by the similarity of its CAM to the average CAM of Class 6; whereas, the incorrectly prediction ( d ) can be explained by comparing its CAM with the average CAMs of classes 2 and 6
Several representative misclassification cases are identified and included in the Section VII of Supplementary Information. In our work, by comparing the CAM of wrongly classified cases with the average CAM of all training data within certain class, we conclude there are three main causes for failed classification. The first cause is the mixture of phases in the sample, which increases the chance of the CAM of a particular pattern differing from the average class CAM. The second cause is lack of XRD patterns in the per-class training data. We only have <5 patterns in certain space group classes, thus the average CAM of minority classes has only a limited number of discriminative features compared to majority classes, increasing the testing error. The third cause of misclassification is missing peaks, or too few peaks, present in the XRD pattern; because of the lack of enough discriminative features, the CAM of the incorrectly labeled pattern could be ambiguously similar to two or more average class CAMs, causing the misclassification.
After the experimentalist identifies these root causes of error, they can be mitigated by increasing the number of experimental training points for certain classes or increasing the phase purity of the material coming from the synthesis and solution-processing procedure.
Summary of contributions
In this work, we develop a supervised machine learning framework to screen novel materials based on the analysis of their XRD spectra. The framework is designed specifically for cases when only sparse datasets are available, e.g., early-stage high-throughput material development and discovery loops. Specifically, we propose a physics-informed data augmentation method that extends small, targeted experimental and simulated datasets, and captures the possible differences between simulated XRD powder patterns and experimental thin-film XRD patterns. A few thousand augmented spectra are found to increase our classification accuracy from <60 to 93% for dimensionality and 89% for space group. The F 1 macro score is also over 0.85 for various algorithms, reflecting the model’s capacity to deal with significantly imbalanced classes.
When trained with both augmented simulated and experimental XRD spectra, a-CNNs are found to have the highest accuracy/ F 1 macro score among the many supervised machine learning methods studied. Our proposed a-CNN architecture allows high performance and interpretably through CAMs. The use of our proposed average CAMs, allow to identify the root cause of misclassification, and allow the design of a robust experiment. Furthermore, we find that the neural network model tolerates coarsening of the training data, providing future opportunities for online learning, i.e., the on-the-fly adaptive adjustment of XRD measurement parameters by taking feedback from machine learning algorithms. 15
Our approach can be extended to XRD-based high-throughput screening of any kind of thin-film materials, beyond perovskite and perovskite-inspired materials. Since most material databases lack information for various kinds of novel materials, the data augmentation approach to tackle data scarcity can be broadly applied. The underlying difference between XRD patterns of thin-films and powders is common to a broad range of materials and is commonly seen in most thin-film characterization experiments. The high interpretability of our approach could allow future work in semi-supervised or active learning, allowing the CAM maps to guide manual XRD refinement or actual XRD experiments. The framework may also be extended beyond XRD classification, to any spectrum containing information-rich features that require classification (XPS, XRF, PL, mass spectroscopy, etc.). The advantages of our approach include: ( 1 ) interpretable error analysis; ( 2 ) a data augmentation strategy that enables fast and accurate classification, even with small and imbalanced datasets.
Measurement and preprocessing of experimental XRD patterns
The XRD patterns for each sample are obtained by using a parallel beam, X-ray powder diffraction Rigaku SmartLab system 53 with 2ϴ angle from 5 to 60 ° with a step size of 0.04 ° . The tool is configured in a symmetric setup. We preprocess the raw XRD patterns to reduce the experimental noise and the background signal. For this purpose, the background signal is estimated and subtracted along the 2ϴ axis, and the spectrum is smoothed conserving the peak width and relative peak size applying the Savitzky-Golay filter. 54 A representative example of a preprocessed and raw XRD spectra is included in Supplementary Fig. S2 .
Simulation of powder XRD patterns
The powder XRD simulations are carried out with Panalytical Highscore v4.7 software based on the Rietveld algorithm implementation by Hill and Howard. 55 , 56 The XRD crystal is assumed to have fully random grain orientations. The unit cell lattice parameters, atomic coordinates, atomic displacement parameters, and space group information are considered for the structure factor calculation in the Rietveld model.
Physics-informed data augmentation
Suppose we describe the series of peaks in an XRD pattern by a discrete function \(\left( {2\theta } \right):I \to {\Bbb R}^ +\) , which maps a set of discrete angles I to positive real numbers \({\mathbb{R}^+}\) corresponding to peak intensities. We augment the data through the following sequential process of transformations \(\Phi _{S,c}^1,\Phi _S^2\) , and \(\Phi _\varepsilon ^3\) :
Random peak scaling is applied periodically along the 2 ϴ axis to account for different thin-film preferred orientations. A subset of random peaks at periodic angles S is scaled by factor c , such that
Random peak elimination (with a different randomly selected S than Eq. 1 ) is applied periodically along the 2 ϴ axis, to account for different thin-film preferred orientations, such that
Pattern shifting by small random value ε along the 2 ϴ direction to allow for different material compositions and film strain conditions, such that
We perform global average pooling in the last convolutional layer. The pooling results are defined as \(\mathop {\sum }\limits_x f_k\left( x \right)\) where f k ( x ) is the activation of unit k at convolution location x . The pooling results \(\mathop {\sum }\limits_x f_k\left( x \right)\) are subsequently fed into the softmax classifier. The class confidence score, thus can be computed as:
We define \(M_C\left( x \right) = \mathop {\sum}\nolimits_k {w_k^c} f_k\left( x \right)\) , as the CAM for class C , where \(w_k^C\) is the weight of unit k of the last convolution layer of class C. Therefore, Sc can be rewritten as:
M C ( x ) directly indicates the importance of the activation at the discrete location x for a given XRD pattern in class C .
In the context of XRD and other spectral measurements, the location of relevant intensity peaks is likely to be displaced only little from sample to sample within a certain class. Thus, the CAMs for all samples within a class are similar, and could be averaged to obtain the discriminative features during activation. Thus, we can define average CAMs for class C as \(\bar M_C\left( x \right)\) :
where n corresponds to the training patterns labeled as class C.
Data availability
The experimental dataset analyzed during the current study is available in the following GitHub repository: [ https://github.com/PV-Lab/AUTO-XRD/tree/master/Datasets/Experimental ]. The simulated and labeled data that support the findings of this study is available from the Inorganic Crystalline Structure Database (ICSD), but restrictions apply to the availability of these data, which were used under license for the current study, and so are not publicly available. Data are, however, available from the authors upon reasonable request and with permission of ICSD.
Code availability
The codes used for preprocessing, data augmentation, and classification are available in the GitHub repository [ https://github.com/PV-Lab/AUTO-XRD/ ]. The classification algorithms are implemented using scikit-learn 0.20 45 and the fastdtw python library. The a-CNN is implemented using Keras with the Tensorflow background.
Tabor, A., Roch, D. & Saikin, L. Lawrence Berkeley National Laboratory recent work title accelerating the discovery of materials for clean energy in the era of smart automation. Nat Rev Mater . https://doi.org/10.1038/s41578-018-0005-z (2018).
Article CAS Google Scholar
Correa-Baena, J.-P. et al. Accelerating materials development via automation, machine learning, and high-performance computing. Joule 2 , 1410–1420 (2018).
Dinnebier, R. E. Powder Diffraction: Theory and Practice . (RSC Publ, Cambridge, 2009).
Rietveld, H. M. A profile refinement method for nuclear and magnetic structures. J. Appl. Crystallogr. 2 , 65–71 (1969).
Carr, D. A., Lach-hab, M., Yang, S., Vaisman, I. I. & Blaisten-Barojas, E. Machine learning approach for structure-based zeolite classification. Microporous Mesoporous Mater. 117 , 339–349 (2009).
Baumes, L. A., Moliner, M., Nicoloyannis, N. & Corma, A. A reliable methodology for high throughput identification of a mixture of crystallographic phases from powder X-ray diffraction data. CrystEngComm 10 , 1321–1324 (2008).
Baumes, L. A., Moliner, M. & Corma, A. Design of a full-profile-matching solution for high-throughput analysis of multiphase samples through powder X-ray diffraction. Chem. - A Eur. J. 15 , 4258–4269 (2009).
Stanev, V. et al. Unsupervised phase mapping of X-ray diffraction data by nonnegative matrix factorization integrated with custom clustering. npj Comput. Mater . 4 , 43 (2018).
Kusne, A. G., Keller, D., Anderson, A., Zaban, A. & Takeuchi, I. High-throughput determination of structural phase diagram and constituent phases using GRENDEL. Nanotechnology 26 , 444002 (2015).
Park, W. B. et al. Classification of crystal structure using a convolutional neural network. IUCrJ . 4 , 486–494 (2017).
Park, W. B., Singh, S. P., Yoon, C. & Sohn, K. S. Combinatorial chemistry of oxynitride phosphors and discovery of a novel phosphor for use in light emitting diodes, Ca 1.5 Ba 0.5 Si 5 N 6 O 3 :Eu 2+ . J. Mater. Chem. C. 1 , 1832–1839 (2013).
Rybakov, V. B., Babaev, E. V., Pasichnichenko, K. Y. & Sonneveld, E. J. X-ray mapping in heterocyclic design: VI. X-ray diffraction study of 3-(isonicotinoyl)-2-oxooxazolo[3,2-a]pyridine and the product of its hydrolysis. Crystallogr. Rep. 47 , 473–477 (2002).
Google Scholar
Hirosaki, N., Takeda, T., Funahashi, S. & Xie, R. J. Discovery of new nitridosilicate phosphors for solid state lighting by the single-particle-diagnosis approach. Chem. Mater. 26 , 4280–4288 (2014).
Suram, S. K. et al. Automated phase mapping with AgileFD and its application to light absorber discovery in the V-Mn-Nb oxide system. ACS Comb. Sci. 19 , 37–46 (2017).
Ren, F. et al. Accelerated discovery of metallic glasses through iteration of machine learning and high-throughput experiments. Sci. Adv . 4 , eaaq1566 (2018).
Article Google Scholar
Bunn, J. K. et al. Generalized machine learning technique for automatic phase attribution in time variant high-throughput experimental studies. J. Mater. Res. 30 , 879–889 (2015).
Kusne, A. G. et al. On-the-fly machine-learning for high-throughput experiments: search for rare-earth-free permanent magnets. Sci. Rep. 4 , 1–7 (2014).
Vecsei, P. M. et al. Neural network-based classification of crystal symmetries from X-ray diffraction patterns. arXiv preprint arXiv:1812.05625 (2018).
Ziletti, A., Kumar, D., Scheffler, M. & Ghiringhelli, L. M. Insightful classification of crystal structures using deep learning. Nat. Commun. 9 , 1–10 (2018).
Ke, T. W. et al. A convolutional neural network-based screening tool for X-ray serial crystallography. J. Synchrotron Radiat. 25 , 655–670 (2018).
Ziatdinov, M. et al. Deep learning of atomically resolved scanning transmission electron microscopy images: chemical identification and tracking local transformations. ACS Nano. 11 , 12742–12752 (2017).
Le Bras, R. et al. A computational challenge problem in materials discovery: synthetic problem generator and real-world datasets. Proceedings of the Twenty-Eighth AAAI Conference of Artifical Intelligence System , pp. 438–443 (2014).
LeBras, R. et al. Constraint reasoning and kernel clustering for pattern decomposition with scaling. in Principles and Practice of Constraint Programming – CP 2011 (ed. Lee, J.) 508–522 (Springer, Berlin, Heidelberg, 2011).
Järvinen, M. Application of symmetrized harmonics expansion to correction of the preferred orientation effect. J. Appl. Crystallogr. 26 , 525–531 (1993).
Fewster, P. F., Langford, J. I. & Fewster, P. F. Reports on progress in physics related content X-ray analysis of thin films and multilayers. 59 , 11 (1996).
Iwasaki, Y., Kusne, A. G. & Takeuchi, I. Comparison of dissimilarity measures for cluster analysis of X-ray diffraction data from combinatorial libraries. npj Comput. Mater. 3 , 1–8 (2017).
Belkly, A., Helderman, M., Karen, V. L. & Ulkch, P. New developments in the Inorganic Crystal Structure Database (ICSD): accessibility in support of materials research and design. Acta Crystallogr. Sect. B Struct. Sci. 58 , 364–369 (2002).
Eperon, G. E. et al. Formamidinium lead trihalide: a broadly tunable perovskite for efficient planar heterojunction solar cells. Energy Environ. Sci. 7 , 982–988 (2014).
Lee, M. M., Teuscher, J., Miyasaka, T., Murakami, T. N. & Snaith, H. J. Efficient hybrid solar cells based on meso-superstructured organometal halide perovskites. Sci. (80-.) 338 , 643–647 (2012).
Hoye, R. L. Z. et al. Perovskite-inspired photovoltaic materials: toward best practices in materials characterization and calculations. Chem. Mater. 29 , 1964–1988 (2017).
Springenberg, J. T. et al. Striving for simplicity: the all convolutional net. arXiv preprint arXiv:1412.6806. (2014).
Zhou, B. et al. Learning deep features for discriminative localization. Proceedings of the IEEE conference on computer vision and pattern recognition . https://doi.org/10.1109/CVPR.2016.319 (2016).
Etgar, L. The merit of perovskite’s dimensionality; Can this replace the 3D halide perovskite? Energy Environ. Sci. 11 , 234–242 (2018).
Xiao, Z., Meng, W., Wang, J., Mitzi, D. B. & Yan, Y. Searching for promising new perovskite-based photovoltaic absorbers: the importance of electronic dimensionality. Mater. Horiz . https://doi.org/10.1039/C6MH00519E (2017).
Zhang, T., Long, M., Liu, P., Xie, W. & Xu, J.-B. Stable and efficient 3D-2D perovskite-perovskite planar heterojunction solar cell without organic hole transport layer. Joule https://doi.org/10.1016/j.joule.2018.09.022 (2018).
Kurchin, R. C., Gorai, P., Buonassisi, T. & Stevanović, V. Structural and chemical features giving rise to defect tolerance of binary semiconductors. Chem. Mater. 30 , 5583–5592 (2018).
Baikie, T. et al. Synthesis and crystal chemistry of the hybrid perovskite (CH3NH3)PbI3 for solid-state sensitised solar cell applications. J. Mater. Chem. A 1 , 5628–5641 (2013).
Sun, S. et al. Synthesis, crystal structure, and properties of a perovskite-related bismuth phase, (NH 4 ) 3 Bi 2 I 9 . APL Mater. 4 , 031101 (2016).
Coelho, A. A. TOPAS-Academic, Version 6: technical reference. (Coelho Software. Brisbane, Australia, 2016).
Shijing Sun, et al. Accelerating photovoltaic materials development via high-throughput experiments and machine-learning-assisted diagnosis. Joule 2 , 1410–1420 (2018).
Ermon, S. et al. Pattern decomposition with complex combinatorial constraints: application to materials discovery. AAAI'15 Proceedings of the Twenty-Ninth AAAI Conference on Artificial Intelligence . (2014).
Zhao, J. et al. Strained hybrid perovskite thin films and their impact on the intrinsic stability of perovskite solar cells. Sci. Adv. 3 , eaao5616 (2017).
Hernández-García, A. & König, P. Data augmentation instead of explicit regularization. arXiv preprint arXiv:1806.03852 (2018).
Goodfellow, I., Bengio, Y., Courville, A. & Bengio, Y. Deep Learning . 1 (MIT Press, Cambridge, 2016).
Pedregosa, F. et al. Scikit-learn: machine learning in {P}ython. J. Mach. Learn. Res. 12 , 2825–2830 (2011).
Friedman, J., Hastie, T. & Tibshirani, R. The Elements of Statistical Learning . 1 (Springer Series in Statistics, New York, NY, USA, 2001).
Salvador, S. & Chan, P. FastDTW: toward accurate dynamic time warping in linear time and space. Time 11 , 70–80 (2004).
Haixiang, G. et al. Learning from class-imbalanced data: review of methods and applications. Expert Syst. Appl. 73 , 220–239 (2017).
Chawla, N. V., Bowyer, K. W., Hall, L. O. & Kegelmeyer, W. P. SMOTE: synthetic minority over-sampling technique. J. Artif. Intell. Res. 16 , 321–357 (2002).
Lin, M., Chen, Q. & Yan, S. Network in network. arXiv preprint arXiv:1312.4400. (2013).
Yoon, C. H. et al. Unsupervised classification of single-particle X-ray diffraction snapshots by spectral clustering. Opt. Express 19 , 16542 (2011).
Wang, Z., Yan, W. & Oates, T. Time series classification from scratch with deep neural networks: a strong baseline. Proc. Int. Jt. Conf. Neural Netw. 2017 , 1578–1585 (2017).
Kobayashi, S. & Inaba, K. X-ray thin-film measurement techniques. Mass Spectrosc. Equipped a Ski. Interface 28 , 8 (2012).
CAS Google Scholar
Press, W. H. & Teukolsky, S. A. Savitzky-Golay smoothing filters. Comput. Phys. 4 , 669 (1990).
Hill, R. J. & Howard, C. J. Quantitative phase analysis from neutron powder diffraction data using the Rietveld method. J. Appl. Crystallogr. 20 , 467–474 (1987).
Degen, T., Sadki, M., Bron, E., König, U. & Nénert, G. The HighScore suite. Powder Diffr. 29 , S13–S18 (2014).
Download references
Acknowledgements
We thank FengXia Wei (A*STAR), Jason Hattrick-Simpers (NIST), Max Hutchinson (Citrine Informatics), and Zachary T. Trautt (NIST) for helpful suggestions on our analysis. Prof. Juan Pablo Correa Baena at Georgia Tech for sharing various experimental XRD patterns. This work was supported by a TOTAL SA research grant funded through MITei (supporting the experimental XRD), the National Research Foundation (NRF), Singapore through the Singapore Massachusetts Institute of Technology (MIT) Alliance for Research and Technology’s Low Energy Electronic Systems research program (supporting the machine learning algorithm development), the Accelerated Materials Development for Manufacturing Program at A*STAR via the AME Programmatic Fund by the Agency for Science, Technology and Research under Grant No. A1898b0043 (for ML algorithm error analysis), and by the U.S. Department of Energy under the Photovoltaic Research and Development program under Award DE-EE0007535 (for code framework development). This work made use of the CMSE at MIT, which is supported by NSF award DMR-0819762.
Author information
These authors contributed equally: Felipe Oviedo, Zekun Ren
Authors and Affiliations
Massachusetts Institute of Technology, Cambridge, MA, 02139, USA
Felipe Oviedo, Shijing Sun, Charles Settens, Zhe Liu, Noor Titan Putri Hartono, Siyu I. P. Tian, Giuseppe Romano & Tonio Buonassisi
Singapore-MIT Alliance for Research and Technology, Singapore, 138602, Singapore
Zekun Ren & Tonio Buonassisi
Institute for Infocomm Research (I2R), Agency for Science, Technology and Research (A*STAR), Singapore, 138632, Singapore
Savitha Ramasamy
National Institute of Standards and Technology, MS 8520, Gaithersburg, MD, 20899, USA
Brian L. DeCost & Aaron Gilad Kusne
You can also search for this author in PubMed Google Scholar
Contributions
F.O. and Z.R. contributed equally for this work. F.O., Z.R., S.S., and T.B. conceived of the research. C.S., F.O., and S.S. prepared the simulated XRD data. Z.R., F.O., C.S., B.L.D.C., and A.G.K. developed data augmentation. S.S., N.T.P.H., and C.S. collected the XRD data. F.O., Z.R., and G.R. developed and tested ML algorithms, with key intellectual contributions from R.S., Z.L., B.L.D.C., and A.G.K. F.O. and Z.R. implemented and tuned the final a-CNN architecture and adapted class activation maps to the context of the problem. F.O., Z.R., S.I.P.T., and T.B. wrote the manuscript, with input from all co-authors.
Corresponding authors
Correspondence to Felipe Oviedo , Zekun Ren or Tonio Buonassisi .
Ethics declarations
Competing interests.
The authors declare no competing interests.
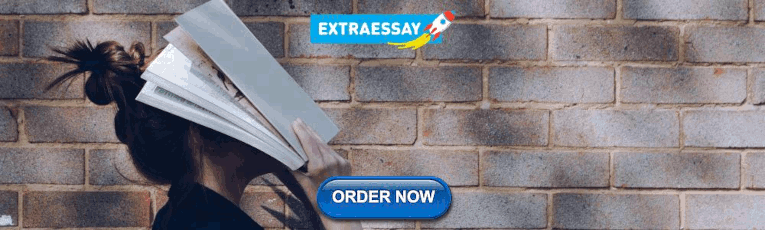
Additional information
Publisher’s note: Springer Nature remains neutral with regard to jurisdictional claims in published maps and institutional affiliations.
Supplementary information
Supplementary information, rights and permissions.
Open Access This article is licensed under a Creative Commons Attribution 4.0 International License, which permits use, sharing, adaptation, distribution and reproduction in any medium or format, as long as you give appropriate credit to the original author(s) and the source, provide a link to the Creative Commons license, and indicate if changes were made. The images or other third party material in this article are included in the article’s Creative Commons license, unless indicated otherwise in a credit line to the material. If material is not included in the article’s Creative Commons license and your intended use is not permitted by statutory regulation or exceeds the permitted use, you will need to obtain permission directly from the copyright holder. To view a copy of this license, visit http://creativecommons.org/licenses/by/4.0/ .
Reprints and permissions
About this article
Cite this article.
Oviedo, F., Ren, Z., Sun, S. et al. Fast and interpretable classification of small X-ray diffraction datasets using data augmentation and deep neural networks. npj Comput Mater 5 , 60 (2019). https://doi.org/10.1038/s41524-019-0196-x
Download citation
Received : 01 December 2018
Accepted : 25 April 2019
Published : 17 May 2019
DOI : https://doi.org/10.1038/s41524-019-0196-x
Share this article
Anyone you share the following link with will be able to read this content:
Sorry, a shareable link is not currently available for this article.
Provided by the Springer Nature SharedIt content-sharing initiative
This article is cited by
Enhanced accuracy through machine learning-based simultaneous evaluation: a case study of rbs analysis of multinary materials.
- Goele Magchiels
- Niels Claessens
- André Vantomme
Scientific Reports (2024)
Deep learning for symmetry classification using sparse 3D electron density data for inorganic compounds
- Seonghwan Kim
- Byung Do Lee
- Kee-Sun Sohn
npj Computational Materials (2024)
Integrated analysis of X-ray diffraction patterns and pair distribution functions for machine-learned phase identification
- Nathan J. Szymanski
- Gerbrand Ceder
- Judah Goldfeder
A deep learning approach for quantum dots sizing from wide-angle X-ray scattering data
- Lucia Allara
- Federica Bertolotti
- Antonietta Guagliardi
Quick links
- Explore articles by subject
- Guide to authors
- Editorial policies
Sign up for the Nature Briefing newsletter — what matters in science, free to your inbox daily.

Information
- Author Services
Initiatives
You are accessing a machine-readable page. In order to be human-readable, please install an RSS reader.
All articles published by MDPI are made immediately available worldwide under an open access license. No special permission is required to reuse all or part of the article published by MDPI, including figures and tables. For articles published under an open access Creative Common CC BY license, any part of the article may be reused without permission provided that the original article is clearly cited. For more information, please refer to https://www.mdpi.com/openaccess .
Feature papers represent the most advanced research with significant potential for high impact in the field. A Feature Paper should be a substantial original Article that involves several techniques or approaches, provides an outlook for future research directions and describes possible research applications.
Feature papers are submitted upon individual invitation or recommendation by the scientific editors and must receive positive feedback from the reviewers.
Editor’s Choice articles are based on recommendations by the scientific editors of MDPI journals from around the world. Editors select a small number of articles recently published in the journal that they believe will be particularly interesting to readers, or important in the respective research area. The aim is to provide a snapshot of some of the most exciting work published in the various research areas of the journal.
Original Submission Date Received: .
- Active Journals
- Find a Journal
- Journal Proposal
- Proceedings Series
- For Authors
- For Reviewers
- For Editors
- For Librarians
- For Publishers
- For Societies
- For Conference Organizers
- Open Access Policy
- Institutional Open Access Program
- Special Issues Guidelines
- Editorial Process
- Research and Publication Ethics
- Article Processing Charges
- Testimonials
- Preprints.org
- SciProfiles
- Encyclopedia
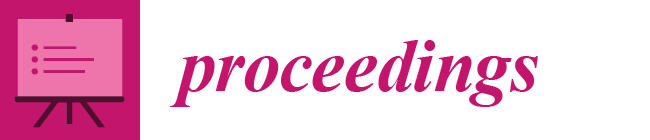
Article Menu
- Subscribe SciFeed
- Google Scholar
- on Google Scholar
- Table of Contents
Find support for a specific problem in the support section of our website.
Please let us know what you think of our products and services.
Visit our dedicated information section to learn more about MDPI.
JSmol Viewer
Xrd data visualization, processing and analysis with d1dplot and d2dplot software packages †.

1. Introduction
2. features overview, 2.1. d2dplot, 2.2. d1dplot.
- nP : Pattern number.
- nS : Series number. One pattern may contain multiple series (data, background, peaks, etc…)
- Name : Name of the series (by default the filename).
- Color : Color of the series.
- Scale : Y scale of the series.
- ZerOff : Offset of the series in the X axis.
- Wavel : Wavelength of the pattern.
- Xunits : Units of the X -axis. By default 2 θ.
- YOffset : Offset of the series in the Y axis.
- Marker : Marker size.
- Line : Line width.
- ErrBars : To show the error bars on Y.
- Show : To show or hide the current series on the plot area.
- Type : To differentiate and apply different plotting styles depending on the content of the data series (powder XRD data, peaks, background estimation or discreet 2 θ values to be used as reference of crystallographic phases).
2.3. Compound Database
2.4. comand-line mode, 3. applications, 4. technical description and availability, author contributions, acknowledgments, conflicts of interest.
- Vallcorba, O.; Rius, J. d2Dplot: 2D X-ray diffraction data processing and analysis for through-the-substrate microdiffraction. J. Appl. Crystallogr. 2019 , 52 , 478–484. [ Google Scholar ] [ CrossRef ]
- Rius, J.; Vallcorba, O.; Frontera, C.; Peral, I.; Crespi, A.; Miravitlles, C. Application of synchrotron through-the-substrate microdiffraction to crystals in polished thin sections. IUCrJ 2015 , 2 , 452–463. [ Google Scholar ] [ CrossRef ]
- Rius, J. Novel phasing method using the origin-free modulus sum function expressed in terms of the absolute electron density. Acta Crystallogr. Sect. A Found. Adv. 2020 , 76 , 489–493. [ Google Scholar ] [ CrossRef ]
- Vallcorba, O.; Rius, J.; Frontera, C.; Peral, I.; Miravitlles, C. DAJUST: A suite of computer programs for pattern matching, space-group determination and intensity extraction from powder diffraction data. J. Appl. Crystallogr. 2012 , 45 , 844–848. [ Google Scholar ] [ CrossRef ]
- Vallcorba, O.; Rius, J.; Frontera, C.; Miravitlles, C. TALP: A multisolution direct-space strategy for solving molecular crystals from powder diffraction data based on restrained least squares. J. Appl. Crystallogr. 2012 , 45 , 1270–1277. [ Google Scholar ] [ CrossRef ]
- Roisnel, T.; Rodríquez-Carvajal, J. WinPLOTR: A Windows Tool for Powder Diffraction Pattern Analysis. Mater. Sci. Forum 2001 , 378–381 , 118–123. [ Google Scholar ] [ CrossRef ]
- Hammersley, A.P. FIT2D: A multi-purpose data reduction, analysis and visualization program. J. Appl. Crystallogr. 2016 , 49 , 646–652. [ Google Scholar ] [ CrossRef ]
- Larson, A.C.; Von Dreele, R.B. General Structure Analysis System (GSAS). Los Alamos Natl. Lab. Rep. LAUR 2004 , 86–748. [ Google Scholar ]
- Altomare, A.; Cuocci, C.; Giacovazzo, C.; Moliterni, A.; Rizzi, R.; Corriero, N.; Falcicchio, A. EXPO2013: A kit of tools for phasing crystal structures from powder data. J. Appl. Crystallogr. 2013 , 46 , 1231–1235. [ Google Scholar ] [ CrossRef ]
- Markvardsen, A.J.; Puphaiboon, K.; Arjeneh, M.; Shankland, K.; Guest, H.L.; Griffin, T.A.N.; Badham, D.R.; Flannery, D.W. Jpowder: A Java-based program for the display and examination of powder diffraction data. J. Appl. Crystallogr. 2010 , 43 , 1532–1534. [ Google Scholar ] [ CrossRef ]
- Highscore Software. Available online: https://www.malvernpanalytical.com/en/products/category/software/x-ray-diffraction-software/highscore (accessed on 19 October 2020).
- Coelho, A.A. TOPAS and TOPAS-Academic: An optimization program integrating computer algebra and crystallographic objects written in C++. J. Appl. Crystallogr. 2018 , 51 , 210–218. [ Google Scholar ] [ CrossRef ]
- TTS_Software, ICMAB-CSIC. Available online: https://departments.icmab.es/crystallography/software (accessed on 19 October 2020).
- Rodríguez-Carvajal, J. Recent advances in magnetic structure determination by neutron powder diffraction. Phys. B 1993 , 192 , 55–69. [ Google Scholar ] [ CrossRef ]
- Degen, T. XrdML, a new way to store (and exchange) X-ray powder diffraction measurement data. arXiv 2002 , arXiv:physics/0210067. [ Google Scholar ]
- Juhás, P.; Davis, T.; Farrow, C.L.; Billinge, S.J.L. PDFgetX3 : A rapid and highly automatable program for processing powder diffraction data into total scattering pair distribution functions. J. Appl. Crystallogr. 2013 , 46 , 560–566. [ Google Scholar ] [ CrossRef ]
- Brückner, S. Estimation of the background in powder diffraction patterns through a robust smoothing procedure. J. Appl. Crystallogr. 2000 , 33 , 977–979. [ Google Scholar ] [ CrossRef ]
- Boultif, A.; Louër, D. Powder pattern indexing with the dichotomy method. J. Appl. Crystallogr. 2004 , 37 , 724–731. [ Google Scholar ] [ CrossRef ]
- de Pedro, I.D.; García-Saiz, A.; Dupont, J.; Migowski, P.; Vallcorba, O.; Junquera, J.; Rius, J.; Rodríguez Fernández, J. On the colossal and highly anisotropic thermal expansion exhibited by imidazolium salts. Cryst. Growth Des. 2015 , 15 , 5207–5212. [ Google Scholar ] [ CrossRef ]
- Hall, S.R.; Allen, F.H.; Brown, I.D. The crystallographic information file (CIF): A new standard archive file for crystallography. Acta Crystallogr. Sect. A Found. Adv. 1991 , 47 , 655–685. [ Google Scholar ] [ CrossRef ]
- Faber, J.; Fawcett, T. The Powder Diffraction File: Present and future. Acta Crystallogr. Sect. B Struct. Sci. Cryst. Eng. Mater. 2002 , 58 , 325–332. [ Google Scholar ] [ CrossRef ]
- Altomare, A.; Corriero, N.; Cuocci, C.; Falcicchio, A.; Moliterni, A.; Rizzi, R. QUALX2.0 : A qualitative phase analysis software using the freely available database POW_COD. J. Appl. Crystallogr. 2015 , 48 , 598–603. [ Google Scholar ] [ CrossRef ]
- Schmalen, P.; Plapper, P.; Peral, I.; Titov, I.; Vallcorba, O.; Rius, J. Composition and phases in laser welded Al-Cu joints by synchrotron X-ray microdiffraction. Procedia CIRP 2018 , 74 , 27–32. [ Google Scholar ] [ CrossRef ]
- Fauth, F.; Peral, I.; Popescu, C.; Knapp, M. The new Material Science Powder Diffraction beamline at ALBA Synchrotron. Powder Diffr. 2013 , 28 , S360–S370. [ Google Scholar ] [ CrossRef ]
- Emami, M.; Rozatian, A.S.H.; Vallcorba, O.; Anghelone, M.; Hadian Dehkordi, M.; Pritzel, C.; Trettin, R. Synchrotron micro-XRD study, the way toward a deeper characterizing the early prehistoric Iranian glass cylinders from Late Bronze Age (1280 BC). Eur. Phys. J. Plus 2020 , 135 , 1–14. [ Google Scholar ] [ CrossRef ]
- Beltrán, M.; Schibille, N.; Brock, F.; Gratuze, B.; Vallcorba, O.; Pradell, T. Modernist enamels: Composition, microstructure and stability. J. Eur. Ceram. Soc. 2020 , 40 , 1753–1766. [ Google Scholar ] [ CrossRef ]
- Vallcorba, O.; Canillas, M.; Audije-Gil, J.; Barroso-Barcenilla, F.; González-Martín, A.; Molera, J.; Rodríguez, M.A.; Cambra-Moo, O. Synchrotron X-ray microdiffraction to study life history events in Cretaceous crocodylomorphs through dental structures. Cretac. Res. 2020 . under review. [ Google Scholar ]
- Valido, I.H.; Fuentes-Cebrian, V.; Boada, R.; Vallcorba, O.; Resina-Gallego, M.; Valiente, M.; López-Mesas, M. Discriminating the formation origin of calcium oxalate monohydrate in kidney stones via synchrotron microdiffraction. Cryst. Growth Des. 2020 . under review. [ Google Scholar ] [ CrossRef ]
- Vallcorba, O.; Casas, L.; Colombo, F.; Frontera, C.; Rius, J. First terrestrial occurrence of the complex phosphate chladniite: Crystal-structure refinement by synchrotron through-the-substrate microdiffraction. Eur. J. Mineral. 2017 , 29 , 287–293. [ Google Scholar ] [ CrossRef ]
- Crespi, A.; Vallcorba, O.; Šics, I.; Rius, J. First identification and compositional study of brown aerinite directly on polished thin-sections by synchrotron through-the-substrate microdiffraction. Eur. J. Mineral. 2019 , 31 , 999–1005. [ Google Scholar ] [ CrossRef ]
- Portolés-Gil, N.; López-Periago, A.M.; Borrás, A.; Fraile, J.; Solano, E.; Vallcorba, O.; Planas, J.G.; Ayllón, J.A.; Domingo, C. Tuning the Structure and Flexibility of Coordination Polymers via Solvent Control of Tritopic Triazine Conformation during Crystallization. Cryst. Growth Des. 2020 , 20 , 3304–3315. [ Google Scholar ] [ CrossRef ]
- Schneider, C.A.; Rasband, W.S.; Eliceiri, K.W. NIH Image to ImageJ: 25 years of image analysis. Nat. Methods 2012 , 9 , 671–675. [ Google Scholar ] [ CrossRef ]
- ALBA Synchrotron (MSPD Beamline, Preparing Your Experiment and Software Section). Available online: https://www.cells.es/en/beamlines/bl04-mspd/preparing-your-experiment (accessed on 19 October 2020).
Click here to enlarge figure
MDPI stays neutral with regard to jurisdictional claims in published maps and institutional affiliations. |
Share and Cite
Vallcorba, O.; Rius, J. XRD Data Visualization, Processing and Analysis with d1Dplot and d2Dplot Software Packages. Proceedings 2020 , 62 , 9. https://doi.org/10.3390/proceedings2020062009
Vallcorba O, Rius J. XRD Data Visualization, Processing and Analysis with d1Dplot and d2Dplot Software Packages. Proceedings . 2020; 62(1):9. https://doi.org/10.3390/proceedings2020062009
Vallcorba, Oriol, and Jordi Rius. 2020. "XRD Data Visualization, Processing and Analysis with d1Dplot and d2Dplot Software Packages" Proceedings 62, no. 1: 9. https://doi.org/10.3390/proceedings2020062009
Article Metrics
Article access statistics, further information, mdpi initiatives, follow mdpi.
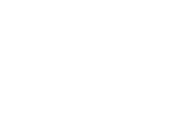
Subscribe to receive issue release notifications and newsletters from MDPI journals
Academia.edu no longer supports Internet Explorer.
To browse Academia.edu and the wider internet faster and more securely, please take a few seconds to upgrade your browser .
Enter the email address you signed up with and we'll email you a reset link.
- We're Hiring!
- Help Center
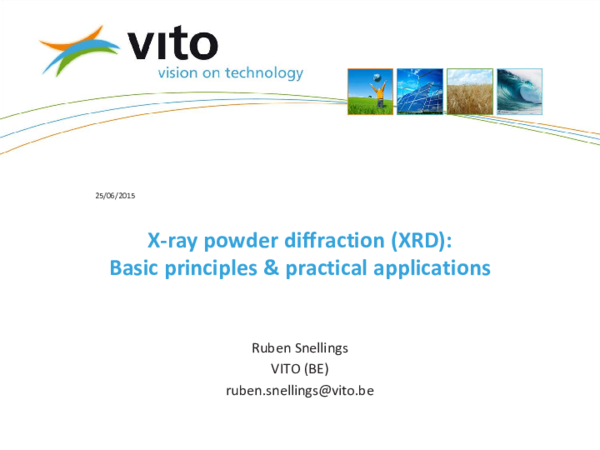
X-ray powder diffraction (XRD): Basic principles & practical applications

Related Papers
Cement and Concrete Research
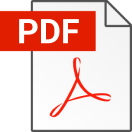
Laura León-reina
Geologica Belgica
abstRact The Rietveld method has become one of the most popular methods in quantitative mineralogical analysis based on X-ray powder diffraction. An estimate of the amorphous phase content can be made by introducing a known amount of an appropriate internal standard. This method was applied to a selected set of zeolitized tuffaceous rocks to develop guidelines for Rietveld quantitative phase analysis in complex mixtures. A local sensitivity analysis of selected refinable parameters was performed and phase abundance results for synchrotron and laboratory based X-ray diffraction data collection were compared. The calculated amorphous phase fraction showed a very high sensitivity towards the refined amount of internal standard, in particular when small amounts of amorphous phase were encountered in the samples. Optimal reproducibility of phase abundance results was obtained when a sufficient number of background polynomials was employed and the internal standard thermal parameters were...
Luis Ordoñez
Isabel Santacruz
This study presents the influence of the calcined kaolinite content of calcined clays on the hydration of Limestone Calcined Clay Cements containing 50% of clinker (LC 3-50). Above a calcined kaolinite content of 65% in calcined clay, further reaction of clinker is inhibited from 3 days onwards. Detail investigation indicates that this slowing down of clinker hydration is related to a significant refinement of pore connectivity. A limiting critical pore entry radius of 3-5 nm is reached, from which the porosity does not get further refined. The higher the calcined kaolinite content, the faster this refinement limit is reached. The formation of carboaluminate hydrates is also limited after reaching this refinement limit. As a consequence, the ongoing reaction of meta-kaolin impacts C-AS -H, mainly affecting gel porosity, which is not well characterized by MIP.
Cement hydration is a very complex set of processes. The evolution of the crystalline phases during hydration can be accurately followed by X-ray powder diffraction data evaluated by the Rietveld method. However, accurate measurements of some microstructural features, including porosity and amorphous content developments, are more challenging. Here, we combine laboratory X-ray powder diffraction and computed microtomography (μCT) to better understand the results of the μCT analyses. Two pastes with different water–cement ratios, 0.45 and 0.65, filled within capillaries of two sizes, ϕ = 0.5 and 1.0 mm, were analysed at 50 days of hydration. It was shown that within the spatial resolution of the measured μCTs, ~2 μm, the water capillary porosity was segmented within the hydrated component fraction. The unhydrated part could be accurately quantified within 2 vol% error. This work is a first step to accurately determining selected hydration features like the hydration degree of amorpho...
Loading Preview
Sorry, preview is currently unavailable. You can download the paper by clicking the button above.
RELATED PAPERS
Bulletin of the Geological Society of Greece
Vassilis Perdikatsis
Journal of Thermal Analysis and Calorimetry
Otávio Gomes
Harvey DeFord
Gilberto Artioli
Susana Conconi
International Conference on Raw Materials and Circular Economy
George Christidis
Journal of Applied Crystallography
Giuseppe Cruciani , Gilberto Artioli
Marijana Serdar
M. Angeles Gomez de la Torre
Sustainability
Dolores Quesada
Stéphane Poyet
Winfried Malorny
Powder Diffraction
Mark Pownceby , Justin Kimpton
Julia Stroh
Materials Letters
Robert C Pullar
Applied Sciences
Stijn Matthys
Boletín de la Sociedad Española de Cerámica y Vidrio
Alberto Viani
Chris Kooij
Leonardo Amaral
Scientific Reports
daniela novembre
Applied Clay Science
Waste and Biomass Valorization
Horacio Colina
Pamela Whitfield
Nordic Concrete Research
Harald Justnes
Harish Kumar Choudhary
Cement and Concrete Composites
Frank Winnefeld
American Mineralogist
Nicola Bursi Gandolfi , Giovanna Zanetti
Giuseppe De Natale
Milena Marroccoli
ISIJ International
Mark Pownceby
Jan Elsen , Fernando Morante
Journal of Urban Technology and Sustainability
Dimas Rambo
Anu M Kantola
Gabriele Vola
Rodrigo Lima
Yiannis Pontikes , Lucie Vandewalle
Journal of the American Ceramic Society
Maximina Romero
- We're Hiring!
- Help Center
- Find new research papers in:
- Health Sciences
- Earth Sciences
- Cognitive Science
- Mathematics
- Computer Science
- Academia ©2024
A Review on the Development of XRD in Ferrite Nanoparticles
- Published: 19 March 2022
- Volume 35 , pages 1033–1047, ( 2022 )
Cite this article
- Richa Jain 1
4062 Accesses
18 Citations
Explore all metrics
In the present study, an overview of the aspects of X-ray diffraction (XRD) in ferrite has been explored. Ferrite nanoparticles have a wide range of applications in various fields. XRD data could be used to measure the phases, crystal structure, and related parameters of ferrites. It can also determine the effect of doping and substitution on the crystal structure of the ferrite and the strain on the crystal lattice due to these variations. Cation distribution, bond length, interionic distances, bond angles, and hopping length can be calculated using XRD for the fruitful discussion of various properties of ferrite. From this study, it can be revealed that XRD is the ideal technique to elucidate not only the crystal structure but also the magnetic, electrical, optical, elastic behavior which could be explained by analyzing the XRD data systematically.
This is a preview of subscription content, log in via an institution to check access.
Access this article
Subscribe and save.
- Get 10 units per month
- Download Article/Chapter or eBook
- 1 Unit = 1 Article or 1 Chapter
- Cancel anytime
Price includes VAT (Russian Federation)
Instant access to the full article PDF.
Rent this article via DeepDyve
Institutional subscriptions
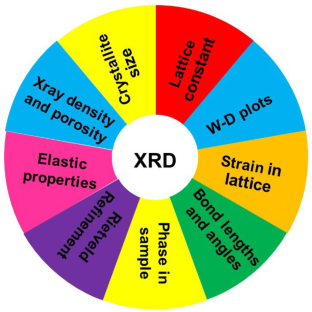
Similar content being viewed by others
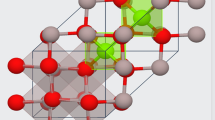
Synthesis of Nanostructured Ferrites and Cation Distribution Studies by X-ray Magnetic Circular Dichroism, Mössbauer Spectroscopy, and X-ray Absorption Spectroscopy
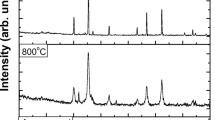
Manifestation of Magnetic Characteristics of Zinc Ferrite Nanoparticles Using the Langevin Function
Synthesis, characterization, optical properties, and electron paramagnetic resonance for nano zn 0 . 5 co 0 . 5 fe 2 − x pr x o 4.
Li, H., et al.: Hyaluronic acid-modified hydrothermally synthesized iron oxide nanoparticles for targeted tumor MR imaging. Biomaterials. 35 , 3666–3677 (2014)
Arantes, A.C.C., Silva, L.E., Wood, D.F., et al.: Bio-based thin films of cellulose nanofibrils and magnetite for potential application in green electronics. Carbohydr. Polym. 207 , 100–107 (2019)
Article Google Scholar
Freire, T.M., Dutra, L.M.U., Queiroz, D.C., et al.: Fast ultrasound assisted synthesis of chitosan-based magnetite nanocomposites as a modified electrode sensor. Carbohydr. Polym. 151 , 760–769 (2016)
Luong, D., Sau, S., et al.: Polyvalent folate-dendrimer-coated iron oxide theranostic nanoparticles for simultaneous magnetic resonance imaging and precise cancer cell targeting. Biomacromol 18 , 1197–1209 (2017)
Hornyak, G.L., Tibbals, H.F., Dutta, J., Moore, J.J.: Introduction to nanoscience and nanotechnology. CRC Press. 1st ed. (2009)
Kulkarni, S.K.: Nanotechnology: principles and practices. Capital Publ Company. 2nd ed. (2011)
Cullity, B.D., Stock, S.R.: Elements of X-ray diffraction. Pearson Publ. 3rd ed. (2014)
Waldron, R.D.: Infrared spectra of ferrites. Phys. Rev. 99 , 1727–1735 (1955)
Article ADS Google Scholar
Bird, S.M., Galloway, J.M., Rawlings, A.E., et al.: Taking a hard line with biotemplating: cobalt -doped magnetite magnetic nanoparticle arrays. Nanoscale 7 , 7340–7351 (2015)
Supriya, S., Kumar, S., Kar, M.: Correlation between AC and DC transport properties of Mn substituted cobalt ferrite. J. Appl. Phys. 120 , 215106 (2016)
Rahman, M.T., Ramana, V.C.: Impedance spectroscopic characterization of gadolinium substituted cobalt ferrite ceramics. J. Appl. Phys. 116 , 164108 (2014)
Jubb, A.M., Allen, H.C.: Vibrational spectroscopic characterization of hematite, maghemite, and magnetite thin films produced by vapor deposition. ACS Appl. Mater. Interfaces 2 , 2804–2812 (2010)
Panda, R.K., Muduli, R., Jayarao, G., et al.: Effect of Cr 3+ substitution on electric and magnetic properties of cobalt ferrite nanoparticles. J. Alloys Compd. 669 , 19–28 (2016)
Holder, C.F., Schaak, R.E.: Tutorial on powder X-ray diffraction for characterizing nanoscale materials. ACS Nano 13 , 7359–7365 (2019)
Coey, J.M.D.: Magnetism and magnetic materials. Cambridge University Press. 7th ed. (2010)
Walter, A., Billotey, C., Garofalo, A., et al.: Mastering shape and composition of dendronized iron oxide nanoparticles to tailor magnetic resonance imaging and hyperthermia. Chem. Mater. 26 , 5252–5264 (2014)
Mameli, V., Musinu, A., Ardu, A., et al.: Studying the effect of Zn-substitution on the magnetic and hyperthermic properties of cobalt ferrite nanoparticles. Nanoscale 8 , 10124–10137 (2016)
Hashim, M., Ahmed, A., Ali, S.A. et al.: Structural, optical, elastic and magnetic properties of Ce and Dy doped cobalt ferrites. J. Alloys Compd. 834 , 155089 (2020)
Jeng, H.T., Guo, G.Y., Huang, D.J.: Charge-orbital ordering and verwey transition in magnetite. Phys. Rev. Lett. 93 , 156403 (2004)
Gore, S.K., Jadhav, S.S., Jadhav, V.V., et al.: The structural and magnetic properties of dual phase cobalt ferrite. Sci Rep. 7 , 2524 (2017)
Dar, M.A., Majid, K., Najar, M.H., et al.: Surfactant-assisted synthesis of polythiophene/ Ni 0.5 Zn 0.5 Fe 2-x Ce x O 4 ferrite composites: study of structural, dielectric and magnetic properties for EMI-shielding applications. Phys. Chem. Chem. Phys. 19 , 10629–10643 (2017)
Sanusi, K., Stone, J.M., Nyokong, T.: Nonlinear optical behaviour of indium-phthalocyanine tethered to magnetite or silica nanoparticles. New J. Chem. 39 , 1665–1677 (2015)
Noh, J., Hong, S., Yoon, C.M., et al.: Dual external field-responsive polyaniline-coated magnetite/silica nanoparticles for smart fluid applications. Chem. Commun. 53 , 6645–6648 (2017)
Tirupanyam, B.V., Srinivas, C., Meena, S.S., et al.: Investigation of structural and magnetic properties of co-precipitated Mn–Ni ferrite nanoparticles in the presence of α-Fe 2 O 3 phase. J. Magn. Magn. Mater. 392 , 101–106 (2015)
Deepty, M., Srinivas, C., Kumar, E.R., et al.: XRD, EDX, FTIR and ESR spectroscopic studies of co-precipitated Mn–substituted Zn–ferrite nanoparticles. Ceram. Int. 45 , 8037–8044 (2019)
Andersen, H.L., Granados-Miralles, C., Saura-Múzquiz, M., et al.: Enhanced intrinsic saturation magnetization of Zn x Co 1-x Fe 2 O 4 nanocrystallites with metastable spinel inversion. Mater. Chem. Front. 3 , 668–679 (2019)
Gao, Y., Wang, Z., et al.: Structural, elastic, thermal and soft magnetic properties of Ni-Zn-Li ferrites. J. Alloys Compd. 774 , 1233–1242 (2019)
Sinha, A., Dutta, A.: Structural, optical, and electrical transport properties of some rare-earth-doped nickel ferrites: a study on effect of ionic radii of dopants. J. Phys. Chem. Solids. 145 , 109534 (2020)
Pawar, R.A., Patange, S.M., Shitre, A.R., et al.: Crystal chemistry and single-phase synthesis of Gd 3+ substituted Co–Zn ferrite nanoparticles for enhanced magnetic properties. RSC Adv. 8 , 25258–25267 (2018)
Hossain, M.D., Hossain, M.A., Khan, M.N.I., et al.: Frequency and temperature dependent magnetic properties with structural Rietveld refinement of Co 0.25 Zn 0.75 Y x Fe 2-x O 4 ferrites. J. Magn. Magn. Mater. 493 , 165696 (2020)
Patterson, A.L.: The scherrer formula for X-ray particle size determination. Phy. Rev. 56 , 978–982 (1939)
Jain, R., Luthra, V., Gokhale, S.: Dysprosium doping induced shape and magnetic anisotropy of Fe 3-x Dy x O 4 (x=0.01–0.1). J. Magn. Magn. Mater. 414 , 111–115 (2016)
Atif, M., Idrees, M., Nadeem, M., et al.: Investigation on the structural, dielectric and impedance analysis of manganese substituted cobalt ferrite i.e., Co 1-x Mn x Fe 2 O 4 (0.0 ≤ x ≤ 0.4). RSC Adv. 6 , 20876–20885 (2016)
Abu-Elsaad, N.I., Nawara, A.S., Mazen, S.A.: Synthesis, structural characterization, and magnetic properties of Ni-Zn nanoferrites substituted with different metal ions (Mn 2+ Co 2+ , and Cu 2+ ). J. Phys. Chem. Solids. 146 , 109620 (2020)
Shirsath, S.E., Mane, M.L., Yasukawa, Y., et al.: Self-ignited high temperature synthesis and enhanced super-exchange interactions of Ho 3+ –Mn 2+ –Fe 3+ –O 2- ferromagnetic nanoparticles. Phys. Chem. Chem. Phys. 16 , 2347–2357 (2014)
Issaoui, H., Benali, A., Bejar, M., et al.: Effect of Bi-substitution into the A-site of multiferroic La 0.8 Ca 0.2 FeO 3 on structural, electrical and dielectric properties. RSC Adv. 10 , 16132–16146 (2020)
Jain, R., Luthra, V., Arora, M., Gokhale, S.: Infrared spectroscopic study of magnetic behaviour of dysprosium doped magnetite nanoparticles. J. Supercond. Nov. Magn. 32 , 325–333 (2019)
Zhang, J.M., Zhang, Y., Xu, K.W., Ji, V.: General compliance transformation relation and applications for anisotropic hexagonal metals. Solid State Commun. 139 , 87–91 (2006)
Maksoud, M.I.A.A., El-Sayyad, G.S., Abokhadra, A., et al.: Influence of Mg 2+ substitution on structural, optical, magnetic and antimicrobial properties of Mn–Zn ferrite nanoparticles. J Mater Sci: Mater Electron 31 , 2598–2616 (2020)
Google Scholar
Mote, V.D., Purushotham, Y., Dole, B.N.: Williamson-Hall analysis in estimation of lattice strain in nanometer-sized ZnO particles. J. Theor. Appl. Phys. 6 , 1–8 (2012)
Kakade, S.G., Kambale, R.C., Ramanna, C.V., Kolekar, Y.D.: Crystal strain, chemical bonding, magnetic and magnetostrictive properties of erbium (Er 3+ ) ion substituted cobalt-rich ferrite (Co 1.1 Fe 1.9-x Er x O 4 ). RSC Adv. 6 , 33308- 33317 (2016)
Shafi, P.M., Bose, A.C.: Impact of crystalline defects and size on X-ray line broadening: a phenomenological approach for tetragonal SnO 2 nanocrystals. AIP Adv. 5 , 057137 (2015)
Alves, T.E., Pessoni, H.V., Junior, A.F.: The effect of Y 3+ substitution on the structural, optical band-gap, and magnetic properties of cobalt ferrite nanoparticles. Phys. Chem. Chem. Phys. 19 , 16395–16405 (2017)
Kadam, R.H., Borade, R.B., Mane, M.L., et al.: Structural, mechanical, dielectric properties and magnetic interactions in Dy 3+ substituted Co–Cu– Zn nanoferrites. RSC Adv. 10 , 27911–27922 (2020)
Amin, N., Hasan, M.S.U., Majeed, Z., et al.: Structural, electrical, optical and dielectric properties of yttrium substituted cadmium ferrites prepared by Co-Precipitation method. Ceram. Int. 46 , 20798–20809 (2020)
Aakash, G., Prasad, M., Mukherjee, S.: Dielectric and electrical characterizations of transition metal ions-doped nanocrystalline nickel ferrites. Appl. Phys. A. 125 , 853 (2019)
Batoo, K.M., Kumar, G., Yang, Y., et al.: Structural, morphological and electrical properties of Cd 2+ doped MgFe 2-x O 4 ferrite nanoparticles. J. Alloys Compd. 726 , 179–186 (2017)
Lakshmiprasanna, H.R., Angadi, V.J., Babu, B.R., et al.: Effect of Pr 3+ doping on the structural, elastic and magnetic properties of Mn–Zn ferrite nanoparticles prepared by solution combustion synthesis method. Chem. Data Coll. 24 , 100273 (2019)
Modi, K.B., Chhantbar, M.C., Sharma, P.U., Joshi. H.H.: Elastic constants determination for Fe 3+ substituted YIG through infra-red spectroscopy and heterogeneous metal mixture rule. J. Mater. Sci. 40 , 1247–1249 (2005)
Deepty, M., Srinivas, C., Kumar, E.R., et al.: XRD, EDX, FTIR and ESR spectroscopic studies of co-precipitated Mn–substituted Zn–ferrite nanoparticles. Ceram. Int. 45 , 8037–8044 (2019)
Anupama, A.V., Rathod, V., Jali, V.M., Sahoo, B.: Composition dependent elastic and thermal properties of Li-Zn ferrites. J. Alloys Compd. 728 , 1091–1100 (2017)
Mazen, S.A., Elsayed, H.M., Abu-Elsaad, N.I.: A comparative study of different concentrations of (Co/Ni/Cu) effects on elastic properties of Li–Mn ferrite employing IR spectroscopy and ultrasonic measurement. Ceram. Int . 47 , 26635–26642 (2021)
Desai, S.S., Pawar, R.A., Jadhav, S.S.: Role of coupling divalent and tetravalent metal ions on the elastic and electric properties of CoFe2O4 ferrites prepared by sol–gel method. J Supercond Nov Magn. 29 , 2635–2640 (2016)
Download references
Author information
Authors and affiliations.
Department of Physics, Motilal Nehru College, Benito Juarez Road, New Delhi, 110021, India
You can also search for this author in PubMed Google Scholar
Corresponding author
Correspondence to Richa Jain .
Ethics declarations
Conflict of interest.
The author declares no competing interests.
Additional information
Publisher's note.
Springer Nature remains neutral with regard to jurisdictional claims in published maps and institutional affiliations.
Rights and permissions
Reprints and permissions
About this article
Jain, R. A Review on the Development of XRD in Ferrite Nanoparticles. J Supercond Nov Magn 35 , 1033–1047 (2022). https://doi.org/10.1007/s10948-022-06213-9
Download citation
Received : 27 February 2022
Accepted : 10 March 2022
Published : 19 March 2022
Issue Date : May 2022
DOI : https://doi.org/10.1007/s10948-022-06213-9
Share this article
Anyone you share the following link with will be able to read this content:
Sorry, a shareable link is not currently available for this article.
Provided by the Springer Nature SharedIt content-sharing initiative
- Crystal structure
- Cation distribution
- Crystallite size
- Find a journal
- Publish with us
- Track your research
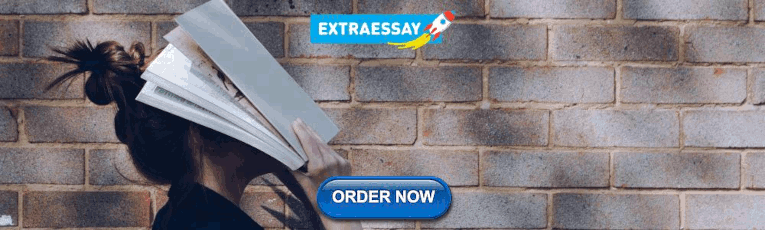
IMAGES
VIDEO
COMMENTS
X-ray diffraction is a high-tech, nondestructive technique. for analyzing a wide range of materials including fluids, met-. als, minerals, polymers, catalysts, plastics, pharmaceuticals, thin ...
Simulated powder X-ray diffraction patterns for wurtzite CdS spherical particles of different sizes that range from 1 μm to 1 nm. The inset shows the 1, 2, and 5 nm XRD patterns on an expanded y -axis scale for clarity. Not all nanoscale particles can be approximated by spheres, and powder XRD data can look different for particles of the same ...
Experimental Characterization Techniques. Jörgen Bergström, in Mechanics of Solid Polymers, 2015. 2.5.3 X-Ray Diffraction. X-ray diffraction is an important tool for studying both amorphous and semi-crystalline polymers [36, 37].It can be used to analyze many features of the microstructure of the material, including lattice parameters, presence of imperfections, crystallographic orientations ...
X-ray diffraction (XRD) is a powerful nondestructive technique for characterizing crystalline materials. It provides information on structures, phases, preferred crystal orientations (texture), and other structural parameters, such as average grain size, crystallinity, strain, and crystal defects.
X-ray diffraction analysis typically affords the static 3D structures of given compounds or materials, but to understand chemical processes, the visualization of fast structural changes is desirable.
X-Ray Diffraction Techniques for Mineral Characterization: A Review for Engineers of the Fundamentals, Applications, and Research Directions December 2021 DOI: 10.20944/preprints202112.0438.v1
The models trained on XRD patterns and virtual PDFs are evaluated using four datasets spanning two chemistries, Li-La-Zr-O and Li-Ti-P-O. To examine how the number of phases in a sample affects ...
X-ray diffraction (XRD) is an important and widely used material characterization technique. With the recent development in material science technology and understanding, various new materials are being developed, which requires upgrading the existing analytical techniques such that emerging intricate problems can be solved. Although XRD is a well-established non-destructive technique, it ...
Powder x-ray diffraction (XRD) is the golden standard for material characterization, where it produces a pattern that encodes information about the crystal symmetry, lattice parameters, types, and ...
X-ray diffraction (XRD) is a proven, powerful technique for determining the phase composition, structure, and microstructural features of crystalline materials. The use of machine learning (ML) techniques applied to crystalline materials research has increased significantly over the last decade. This review presents a survey of the scientific literature on applications of ML to XRD data ...
P owder X-ray diffraction (XRD) is a common character- ization technique for nanoscale materials. Analysis of a sample by powder XRD provides important information that is complementary to various microscopic and spectro-scopic methods, such as phase identi cation, sample purity, fi crystallite size, and, in some cases, morphology.
X-ray diffraction (XRD) is a powerful nondestructive technique for characterizing crystalline materials. It provides information on structures, phases, preferred crystal orientations (texture), and other structural parameters, such as average grain size, crystallinity, strain, and crystal defects. X-ray diffraction peaks are produced by ...
This book is a highly accessible introduction to XRD for materials research. It is written in concise and clear prose. The text creates a cohesive picture of XRD. After finishing this book, researchers will be able to understand the basics of many materials science and engineering research papers.
X-ray diffraction (XRD) is a standard technique that is widely applied in heterogeneous catalysis to determine phase composition, atomic structure, and size of crystallites. This review is focused on the application of in situ XRD for studying the catalysts during their "lifetime" (under synthesis, activation, operation, and deactivation conditions), limiting the objects of research to ...
Powder XRD Technique and its Applications in Science and Technology. Ashish Chauhan 1* and Priyanka Chauhan. Small and Medium Pharmaceutical Industry Centre, Mohali, Punjab, India. Shikhar S ...
X-ray diffraction (XRD) techniques are powerful, non-destructive characterization tool with minimal sample preparation. XRD provides the first information about the materials phases, crystalline structure, average crystallite size, micro and macro strain, orientation parameter, texture coefficient, degree of crystallinity, crystal defects etc. XRD analysis provides information about the bulk ...
Although various researched works have been carried out in x-ray crystallography and its applications, but there are still limited number of researches on crystallographic theories and industrial application of x-ray diffraction. The present study reviewed and provided detailed discussion on atomic arrangement of single crystals, mathematical concept of Bravais, reciprocal lattice, and ...
X-ray diffraction (XRD) data acquisition and analysis is among the most time-consuming steps in the development cycle of novel thin-film materials. We propose a machine learning-enabled approach ...
Powder X-ray diffraction (PXRD) is a cornerstone technique in materials characterization. However, complete structure determination from PXRD patterns alone remains time-consuming and is often intractable, especially for novel materials. Current machine learning (ML) approaches to PXRD analysis predict only a subset of the total information that comprises a crystal structure. We developed a ...
The d1Dplot and d2Dplot computer programs have been developed as user-friendly tools for the inspection and processing of 1D and 2D X-ray diffraction (XRD) data, respectively. d1Dplot provides general tools for data processing and includes the ability to generate comprehensive 2D plots of multiple patterns to easily follow transformation processes. d2Dplot is a full package for 2D XRD data ...
XRD can be applied to the materials analysis of. archaeological ceramics as well as to the st udy of. several other artifact classes mentioned above. F o rt e c h n i c a ld e t a i l so nt h et e ...
Academia.edu is a platform for academics to share research papers. X-ray powder diffraction (XRD): Basic principles & practical applications ... of selected refinable parameters was performed and phase abundance results for synchrotron and laboratory based X-ray diffraction data collection were compared. The calculated amorphous phase fraction ...
In the present study, an overview of the aspects of X-ray diffraction (XRD) in ferrite has been explored. Ferrite nanoparticles have a wide range of applications in various fields. XRD data could be used to measure the phases, crystal structure, and related parameters of ferrites. It can also determine the effect of doping and substitution on the crystal structure of the ferrite and the strain ...
X-Ray Powder Diffraction (XRD) is a technique used in forensic science to analyze various types of trace evidence, such as glass, paint and drugs. XRD has been shown to be a ... This paper will describe the method development for the use of this instrument to distinguish a counterfeit from an authentic product.