Thank you for visiting nature.com. You are using a browser version with limited support for CSS. To obtain the best experience, we recommend you use a more up to date browser (or turn off compatibility mode in Internet Explorer). In the meantime, to ensure continued support, we are displaying the site without styles and JavaScript.
- View all journals
- Explore content
- About the journal
- Publish with us
- Sign up for alerts
- Open access
- Published: 26 April 2021
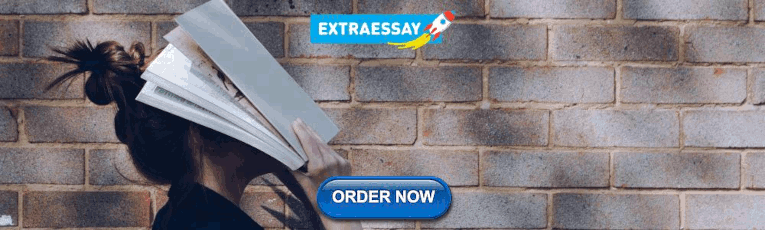
A study on Electrical Discharge Machining of Titanium Grade2 with experimental and theoretical analysis
- Emmanouil L. Papazoglou 1 ,
- Panagiotis Karmiris-Obratański 1 , 2 ,
- Beata Leszczyńska-Madej 3 &
- Angelos P. Markopoulos 1
Scientific Reports volume 11 , Article number: 8971 ( 2021 ) Cite this article
5648 Accesses
41 Citations
Metrics details
- Engineering
- Materials science
Titanium alloys, due to their unique properties, are utilized in numerous modern high-end applications. Electrical Discharge Machining (EDM) is a non-conventional machining process, commonly used in machining of hard-to-cut materials. The current paper, presents an experimental study regarding the machining of Titanium Grade2 with EDM, coupled with the development of a simulation model. The machining performance indexes of Material Removal Rate, Tool Wear Ratio, and Average White Layer Thickness were measured and calculated for different pulse-on currents and pulse-on times. Moreover, the developed model that integrates a heat transfer analysis with deformed geometry, allows to estimate the power distribution between the electrode and the workpiece, as well as the Plasma Flushing Efficiency, giving an insight view of the process. Finally, by employing the Response Surface Methodology, educed regression models that correlate the machining parameters with the corresponding results, while for all the aforementioned indexes, ANOVA was performed.
Similar content being viewed by others
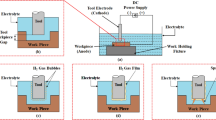
Experimental investigation on machining characteristics of titanium processed using electrolyte sonicated µ-ECDM system
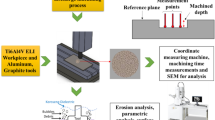
Application of non-ionic liquids-based modified dielectrics during electric discharge machining (EDM) of Ti6Al4V alloy to enhance machining efficiency and process optimization
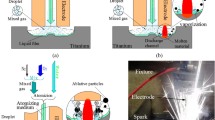
Experimental investigations into the performance of die-sinking mixed-gas atomization discharge ablation process on titanium alloy
Introduction.
Electrical Discharge Machining (EDM) is classified as one of the earliest non-conventional machining processes, but it still finds extensive use and application in modern industry as a leading edge machining process in treating hard-to-cut materials 1 . The fundamental principle of EDM is that material removal is resulted by means of rapid repetitive spark discharges, which occur between a working electrode and the workpiece. During EDM, a pulse voltage difference is applied between the workpiece and the electrode, both of which have to be immersed into proper dielectric fluid. Under specific conditions, i.e., voltage and gap between electrode and workpiece, an intense column of electromagnetic flux is formed, best known as plasma channel, having energy densities in the range of 10 11 –10 14 W/m 2 . Due to the high electromagnetic energy density, extremely high temperatures are topically developed, i.e. 6000–12,000 °C, resulting in the melting and/or ablation of material from both the electrode and the workpiece 2 , 3 . EDM has been successfully used in the machining of hard-to-cut material, facilitating and achieving high dimensional accuracy, in complex shapes and geometries. Moreover, EDM is a non-contact machining process, since no contact exists between the working electrode and the workpiece, thus, no cutting forces are developed, leading to absence of mechanically induced residual stresses in the workpiece material. Due to the inherent advantages of EDM in comparison with conventional cutting processes and its capability to handle any electrical conductive material, regardless of its mechanical properties, EDM finds extensive use in the production of dies and molds, in automotive and aerospace industry, as well as in the field of surgical components production 4 .
EDM is a complicated, multi-parameter process; machining parameters include the pulse-on current (I P ), the pulse-on time (T on ), the duty factor (η), the machining voltage (V), the polarity, the discharge gap and the dielectric flushing pressure and method. At the same time, the machining results depend on the thermo-physical properties of the working electrode and the workpiece. Different materials like copper, graphite and tungsten have been proposed and tested as working electrodes, as well as composite and powder metallurgy-made materials 2 , 5 . The performance of the machining process is assessed mainly in terms of the Material Removal Rate (MRR), the Tool Wear Ratio (TWR), and the resulted machined Surface Quality (SQ). The improvement of MRR and the decrease of TWR are vital, in order for EDM to become economically competitive in comparison to conventional machining processes. Moreover, the decrease of TWR and the reduction of tool wear, affects beneficially the machining precision, as the electrode’s profile and geometry change at slower pace, keeping the machining precision at high level. Since EDM is a multi-parameter process, extended experiments have to be carried out so that the machining of a range of materials under different parameters can be studied. Thus, the development of models and simulations procure the advance of research, limiting the need of experiments, while, and at the same time, a more adequate and in-depth analysis of the process can be attained.
Modeling and simulation are not a straightforward and unambiguous process, since complicated multi-physical phenomena that occur, have to be modeled in an accurate and realistic way, considering the given limitations in computer processing power, as well as the lack of robust theoretical background. Definitely, there are some basic guidelines that are followed, nevertheless, there are certain topics concerning the modeling and simulation of EDM that are still scientifically open. More specifically, in the vast majority of EDM models, a single spark is simulated 6 , with conduction considered as the dominant heat transfer mechanism 7 . The MRR is estimated based on the developed temperature profile, as well as the resulted crater's geometrical characteristics, i.e. depth and width 8 , 9 . On the contrary, in many aspects, the proposed models differentiate as a result of a vague theoretical background. The plasma channel is simulated as a boundary heat flux, nevertheless, there are different approaches regarding its spatial and temporal distribution. In sake of simplicity, in some studies, the plasma is considered as a point heat source 10 , 11 , while in most cases, it is modeled either as a disk heat source or having a Gaussian spatial distribution 9 , 12 . Escobar et al. 13 and Weingärtner et al. 14 have presented interesting studies concerning the different simulation results that emerged by employing different types of heat sources in models. Adopting the more realistic approach that plasma channel has a spatial distribution, means that subsequently its radius has to be defined. Again, there is not a comprehensively accepted method, rather semi-empirical relations that correlate the plasma radius with the machining parameters I P and T on 2 , 15 . In some simulations the plasma radius is considered as time dependent 16 , 17 , 18 , although there is no absolutely realistic way to confirm such models 19 . One more open research topic is the distribution of energy between the workpiece and electrode. In the most classical and simplified approach, according to DiBitonto et al. 10 , 18.3% of the plasma energy is absorbed by the workpiece, an estimation that has been adopted in several models 6 , 8 , 20 . On the other hand, experimental 21 , 22 and simulation studies 7 , 15 , 16 , 23 have proved that the absorbed by the workpiece amount of energy is not constant, but depends on the machining parameters, namely the pulse-on current and the pulse-on time. Klocke et al. 24 have conducted an interesting study regarding the variability of existing models in simulation of EDM, concluding that different modeling parameters have a considerable discrepancy in obtained results. One more modeling aspect that necessitates careful definition is the Plasma Flushing Efficiency (PFE), which indicates the fraction of the molten material that is efficiently removed by the workpiece with every spark. Aiming on a simplified approach, in some simulations the PFE is considered 100% 25 or silently neglected, implying that molten material is totally removed by the formatted crater. However, such a hypothesis is clearly inaccurate, since it cannot interpret the White Layer (WL) formation and be in agreement with experimental results. In more realistic approaches, the PFE is calculated in respect to machining parameters, according to experimental data, often using reverse engineering 9 , 23 . For all the aforementioned reasons, the importance of developing a model that is accurate, detailed and in agreement with experimental results, is deduced. It pertains to a model that not only explains and describes the occurring physical phenomena, but is also capable of predicting the results of the machining process.
Titanium-based alloys find extensive applicability in numerous modern industrial fields, such as aerospace, automobile, gas-turbine engines, nuclear and chemical industry, sports and medical applications. Their extended utilization is owned to their unique properties, namely the high strength at low to moderate temperature, the superior strength to weigh ratio, the excellent corrosion and wear resistance, the fatigue durability and their high biocompatibility 5 , 26 . Nevertheless, titanium alloys are considered hard-to-cut materials, suffering from poor machinability, due to their inherent mechanical and thermo-physical properties. Low thermal conductivity, high chemical reactivity, as well as the low modulus of elasticity are the main drawbacks in their machining with conventional processes 27 , 28 . Thus, non-conventional machining techniques, like EDM, are utilized as feasible alternatives.
Already from the late 90' s the machining of titanium alloys with EDM gathered the research interest, with experimental studies being conducted mainly. The MRR, TWR and SQ were the most common research topics, and the way that these machining performance indexes are effected by the machining conditions, i.e. the pulse-on current, pulse-on time, the dielectric fluid, and the electrode material 29 , 30 , 31 . The significantly low MRR and high TWR lead to the use of different electrode materials, namely graphite, copper, aluminum or composite, in order to render the process more efficient and thus, economically feasible 32 , 33 , 34 . As Fonda et al. 1 concluded, the productivity in machining Ti–6A–4V (the most commonly used titanium alloy) with EDM, depends directly to the temperature of the workpiece during machining, entailing that the properly chosen machining parameters are of great importance. Moreover, the energy distribution between electrode and workpiece is a fundamental cause of the low MRR and the high TWR that is observed in machining titanium alloys with EDM. Shen et al. 35 investigated this distribution, inferring that the energy distribution ratio is highly affected by the machining parameters, namely, the energy density, the pulse duration and the polarity. Some peculiarities in machining of titanium based alloys with EDM have been investigated by Sen et al. 36 and Holsten et al. 37 . The former studied the significant change in machinability of Ti–6Al–4V with the addition of Boron, and as the Boron content and the grain size change. The latter investigators, in a conceptually similar study, ascertained the differences in machining titanium alloys according to their Al content, emphasizing on the major role of polarity and dielectric fluid type. Although many researches have been carried out concerning the machining of Ti–6Al–4V and Ti–6Al–4V ELI with EDM 38 , 39 , 40 , 41 , research regarding other titanium grades is limited and probably inadequate 42 , 43 , 44 . Ahuja et al. 45 presented a study regarding the bioactivity of commercially pure titanium processed by micro-electric discharge drilling, establishing that EDM is a feasible method for titanium machining. Finally, and under the scope of utilizing titanium alloys in bio-medical applications, the Ti-6Al-4Nb (an alloy where the cytotoxic vanadium has been replaced by niobium) was studied, concerning its surface integrity, its bio-activity and the performance characteristics during and after its machining with EDM 46 .
The objective of the current study is to present a thorough research regarding the machining of pure titanium with EDM. The main performance indexes will be measured and evaluated, while, at the same time, and by employing simulation models, parameters and coefficients that are extremely difficult, or even impossible to be experimentally measured, will be estimated. At first, a series of experiments was conducted, in machining Titanium Grade2 with EDM, by using graphite electrode, and for a wide range of per pulse energy, i.e., from 6.75 up to 150 mJ. The MRR and the TWR were calculated, and by metallographic analysis the Average White Layer Thickness (AWLT) was measured. Subsequently, to simulate the process, a detailed Finite Element Method (FEM) model was developed, integrating a heat transfer analysis with deformed geometry, aiming to more realistic and accurate results. Employing the experimental data and through reverse engineering, important parameters of the process, such as the energy distribution ratio between the workpiece and the electrode, the PFE, as well as secondary data like the craters' geometrical characteristics, were determined. Finally, based on Response Surface Methodology (RSM), an Analysis of Variance (ANOVA) was performed, while equations that correlate the machining parameters with the most important process' performance indexes were proposed. In summation, the aim of the current paper is to present a comprehensive investigation of machining Titanium Grade2 with EDM, providing experimental, and simulation data, while via the semi-empirical correlations, a predictability, regarding the process, is gained. The aim of the current study is to provide scientifically interesting results, which will advance and promote further research in the relevant field, along with useful and more applicable data. In Fig. 1 the flow chart presents a graphical overview of the current study.
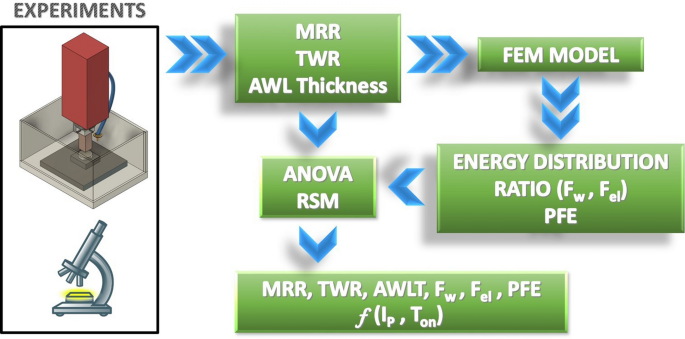
Graphical overview.
Experimental procedure
The experiments were conducted in an Agie-Charmilles Roboform 350 Sp Die-Sinking EDM employing square type of pulses. A plate of Titanium Grade2 (see Table 1 for Titanium Grade2 chemical composition and the maximum accepted limits of elements) was used as a workpiece, and a graphite working electrode, with nominal dimensions of 19 × 14 mm. Synthetic hydrocarbon oil (kerosene) was utilized as dielectric fluid, which was properly channeled into the working tank through a flushing nozzle. A full-scale experiment was carried out, with 4-levels control parameters the pulse-on current and the pulse-on time, taking values from 9 up to 25A and 25 up to 200 μs, respectively. That way, a wide range of per pulse energies was studied, i.e. 6.75 mJ up to 150 mJ, giving a justified reliability on the subsequently developed models and the conclusions that were drawn. All other machining parameters were kept constant, namely, the duty factor was set at 50%, the flushing pressure at 0.7MP and the nominal cutting depth at 0.5 mm. In Table 2 the machining parameters are listed in detail.
The MRR and the TWR were calculated according to Eqs. ( 1 ) and ( 2 ) respectively 47 .
where MRR in [mm 3 /min], W st , W fin the workpiece weight before and after the machining respectively in [gr], t mach the machining time in [min], ρ the workpiece material density in [gr/mm 3 ], and El st , El fin the working electrode weight before and after machining respectively in [gr]. It has to be pointed out that in-between experiments the electrode was properly dried, to remove any absorbed dielectric, since graphite is, by nature, a porous medium.
Following the machining, a metallographic analysis of the cross-section of the workpiece took place. The specimens were cut perpendicular to the machined surfaces and after the necessary chemical etching, they were observed in optical microscope in order for the AWLT to be estimated. The AWLT was calculated as the quotient of the WL area divided by the corresponding length. In Fig. 2 an enlightening snapshot of the measuring method is depicted.
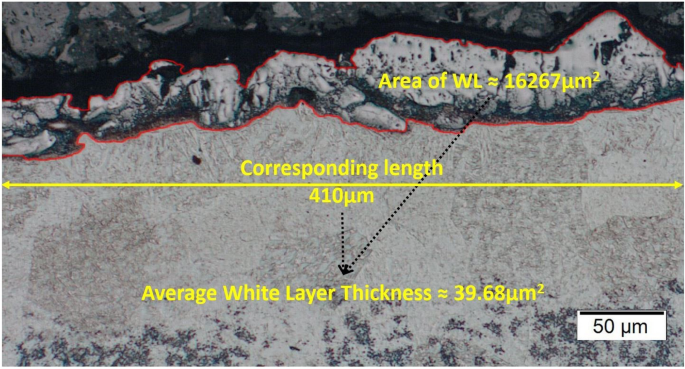
Measurement of the AWLT for I P 25A and T on 200 μs.
Modeling and simulation
In the current study, a FEM model is developed and proposed in order to simulate the material erosion during EDM. Since EDM is by nature a stochastic and chaotic process, some basic assumptions and simplifications have to be made, in order for its modeling and simulation to become attainable.
In the current model, the following assumption/simplifications have been considered:
At first, the concept of a "typical spark" is introduced, implying that all the sparks are identical, and a single plasma channel is formed on each discharge. In the work of Klocke et al. 24 is stated that the single discharge experiments do not represent the material removal of a continuous discharge process. Hence, the introduction of a "typical spark" that can describe the continuous process is preferable than conducting and modeling single discharge experiments.
Conduction is regarded as the main and only heat transfer mechanism into the material 48 .
The energy distribution between workpiece and electrode depends on the machining parameters.
The plasma channel is modeled employing a Gaussian heat flux distribution 17 , 23 .
The erosion of the molten material is taken into consideration, while the PFE also depends on the machining parameters.
The material is considered homogenous and isotropic, with temperature dependent properties. Moreover, latent heats of the materials are also considered.
As only a relevantly small material volume is removed per spark, compared to workpiece and tool electrodes’ volumes, both the workpiece and the electrode can be considered as semi-infinite bodies.
Governing equations and boundary conditions
The conduction heat transfer, the main and only mechanism of heat transfer into the material, is mathematically expressed by Eq. ( 3 ) 12 , 49 :
with T the temperature in [K], ρ the density in [kg/m 3 ], Cp the heat capacity in [J/kgK], k the thermal conductivity in [W/mK], and Q a heat source or a heat sink in [W/m 3 ].
Since the plasma channel is modeled as a heat flux with Gaussian distribution, it can be described as 12 , 49 :
with q pl being the heat flux due to the plasma channel in [W/m 2 ], Q o the maximum power intensity at the center of the plasma channel in [W/m 2 ], r the distance from the center of the plasma channel in [m], and σ the standard deviation of the Gaussian distribution. Considering that the plasma channel radius R pl [m] is equal to three standard deviations (R pl = 3σ), and that the integral of the Gaussian distribution has to be coequal with the total absorbed power, the Q o can be calculated according to Eq. ( 5 ) 12 , 49 :
with F i being the fraction of energy that is absorbed either by the workpiece (F w ) or by the electrode (F el ), V the voltage in [V], and I P the pulse-on current in [A]. Since square pulses were employed, the pulse-on current and the voltage are considered steady throughout the discharge time. Hence, the power distribution can be expressed as 12 , 49 :
It was previously mentioned that there is a divergence of views regarding the method of estimation of the plasma radius, since no adequately robust theoretical model exists, but only semi-empirical relations. In a previous study 49 it has been determined that when a thermal model is coupled with deformed geometry, the most suited correlation between machining parameters and plasma radius, leading in more accurate and realistic results is 2 , 49 :
with R pl the plasma radius in [m].
As both electrode and workpiece are submerged into the dielectric fluid, heat flux due to convection takes place, which is calculated as 50 :
with q conv being the heat flux from the workpiece to dielectric fluid due to convection in [W/m 2 ], h diel the heat transfer coefficient between the workpiece and the dielectric oil with value h diel = 10 5 [W/(m 2 K)] 51 and T diel the dielectric oil temperature and equal to 293.15 K.
The heat losses due to radiation can be estimated as 50 :
with q rad being the heat flux due to radiation in [W/m 2 ], ε i the emissivity coefficient of the electrode (ε el ) or the workpiece (ε w ), T amb the ambient temperature in [K].
As it was previously stated, the current model couples heat transfer analysis with deformed geometry that simulates the material erosion. The material erosion denotes the simultaneous energy removal from the system; hence, the erosion rate can be calculated in respect to the removed energy rate. Assuming that the total amount of molten (in case of the graphite electrode, ablated) material is removed, the surface temperature remains constant just below the phase change temperature, the removed energy rate can be calculated by employing Eq. ( 10 ) 49 :
with q ph.ch. being the heat flux due to material melting in [W/m 2 ], T ph.ch. the material’s phase change temperature in [K] and h ph.ch. the heat transfer coefficient in [W/(m 2 K)], which is zero for T ≤ T ph.ch. and increases linearly for T > T ph.ch. .
In basis of Eq. ( 10 ), the erosion rate, i.e. the normal velocity of the eroding surface, can be defined as 49 :
with u ph.ch. being the material’s eroding velocity in [m/s], ρ i the density of the electrode or the workpiece in [kg/m 3 ], and LH i the material’s latent heat in [J/kg]. In case of the Titanium workpiece the latent heat of phase change refers to the latent heat of melting, while for the graphite electrode to the latent heat of ablation.
At this point, the model's boundary conditions have been fully defined, except of the absorption coefficients F w and F el , which are calculated through reverse engineering. Conceptually, reverse engineering is a method to estimate an unknown parameter, which cannot be defined experimentally or analytically, by tuning it in such a way that simulation is in line with experimental results. In the current model the inputs parameters, based on which the absorption coefficients were tuned/calculated are the "average crater volume” (ACV) (WACV and EACV for workpiece and electrode, respectively) and the AWLT. The WACV and EACV are directly resulted by the MRR and TWR, while the AWLT was measured through metallographic analysis, see Experimental Procedure section.
In workpiece simulation, at some point during the spark-on time, when the formatted crater's volume becomes equal to WACV, the material removal is adjusted to zero (by zeroing the q melt ), thus, overheated volume of material (T > T melt ) is formed. This melted, but not abstracted material, forms the WL, which, for a certain value of F w , has thickness equal to AWLT. On the other hand, graphite does not form any type of WL since for relatively low pressures it does not melt, but sublimes, for temperature near 4000 K. Consequently, the electrode absorption coefficient is calculated based on the EACV, namely, for the F el that the formatted crater's volume equals to EACV. The WACV and EACV can be calculated by Eqs. ( 12 ) and ( 13 ) respectively.
with WACV and EACV being the workpiece and electrode average crater volumes, respectively in [m 3 ], T on the pulse-on time in [μs], ρ gr , ρ ti the densities of graphite and Titanium Grade2, respectively in [kg/m 3 ], and η the duty factor.
Finally, the PFE can be estimated. To calculate the PFE, maximum theoretical crater volume has to be defined, namely, the crater volume in case of a zero WL. Having already defined the workpiece absorption coefficient, the maximum theoretical average crater volume (WACV theor ) is computed by allowing the material removal through the whole pulse-on time, hence, no volume of not abstracted melt, i.e., WL, is formed. Knowing the WACV theor , PFE can be estimated according to Eq. ( 14 ) 52 :
In Fig. 3 , by a flow chart, the reverse engineering method that was analyzed afore is graphically presented.
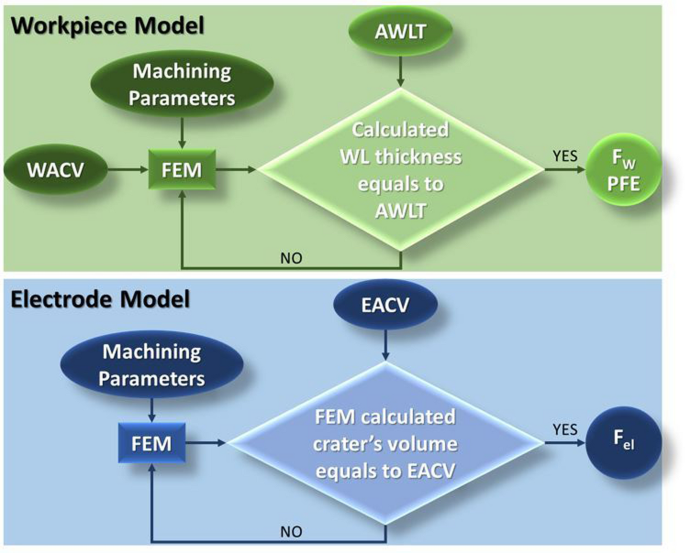
Flow chart of the reverse engineering method.
Material properties and material models
Weingärtner et al. 14 in their study indicated the significance of a comprehensive and accurate material's thermophysical properties definition. In the current study, the major materials’ properties and constants were determined as temperature dependent according to literature, while the phase change latent heats were also taken into consideration, since they are in the core of the model.
For Titanium Grade2, the thermo-physical properties values, as Mills 53 recommends them, were adopted. Density, specific heat, thermal conductivity and emissivity were defined and introduced into the model, as temperature dependent. Moreover, the specific heat of phase change between Titanium phase α and Titanium phase β were also encompassed. The transition from phase α to phase β is temperature defined at 1155 [K] with latent heat of transition LH α→β = 87 [kJ/kg], while the melting point of Titanium Grade2 set on 1941 [K] with melting latent heat LH melt = 295[kJ/kg].
The modeling of electrode material is more complicated, since the graphite electrode is a porous medium, and thus, the thermo-physical properties have to be defined properly, considering its porosity. The effective volumetric heat capacity is calculated according to Eq. ( 15 ) 54 :
with φ gra being the volume fraction of graphite, ρ gr and ρ ker [kg/m 3 ] the densities of graphite and kerosene respectively and Cp gr and Cp ker the specific heats in [J/kgK]. According to the supplier, the electrode has a nominal density of 1.8 [g/cm 3 ], while graphite's density is 2.26 [g/cm 3 ] 55 , thus, the graphite volume fraction in the electrode can be estimated as φ gra ≈1.8/2.26≈0.796. On the contrary with the calculation of the effective volumetric heat capacity, where the volume average method was employed, the effective thermal conductivity has to be defined based on the power law 54 :
with k eff being the effective thermal conductivity in [W/mK], and k gr and k ker the thermal conductivity of graphite and kerosene, respectively, in [W/mK]. Since graphite is an anisotropic material, its relative thermal conductivity is used, based on values that are reported by Pierson 55 . For the graphite thermo-physical properties, the values that are referred by Pierson 55 were used, while for kerosene the ones cited by Zhang et al. 56 are employed. As in titanium, the phase change latent heats were also were considered and integrated into the model. The latent heat of sublimation for graphite defined as LH sub = 39,716 [kJ/kg] at 4000 [K], while for the kerosene the ablation latent heat LH abl = 251 [kJ/kg] at 502 [K].
Modeling parameters
Aiming at economy in computational time and power, the model control volume was set up as axonometric, a justified selection since the spark is considered as uniform. The control volume dimension was defined in respect to the plasma radius; specifically, the width was set equal to 1.2 of the R pl and the height to 0.5 of the R pl . Moreover, a scaled mesh was adopted, finer in the volume where high gradients of temperature and material erosion occur and coarser in the remaining volume, see Fig. 4 , while mesh independence was ensured. Finally, the maximum time step was set at 0.1 μs. In Fig. 4 the boundary conditions, as well as the mesh of the control volume are presented.
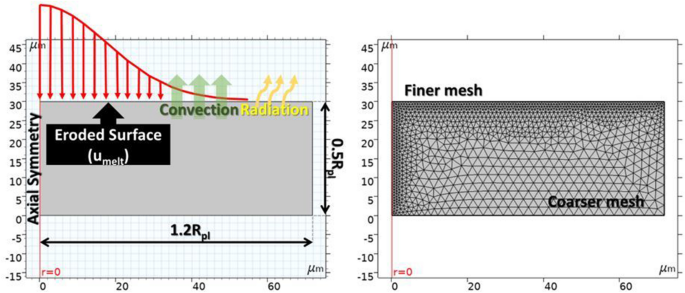
Model’s boundary conditions and meshing.
Response surface methodology
Response Surface Methodology (RSM) is a collection of mathematical and statistical techniques, which are generally used in modeling and analysis of a problem, namely, to determine the correlation between several explanatory variables and response variables. The next step after the Design of Experiment (DOE), is to define the form of relationship between response and independent variables. Optimum model may consist of linear, squared and cross-product terms of the independent variables. Model's coefficients are estimated based on the least squares method, while, subsequently, ANOVA is commonly performed to define the adequacy of the model and the statistical importance of its terms. There is no general rule regarding the order of model that is best suited on each problem, rather than a trial-and-error procedure, driven by the obtained results. The RSM can be mathematically expressed as 57 , 58 :
with f(x 1 ,x 2 ,…,x k ) being the corresponding response variable yield. In studying and modeling the EDM process, RSM is frequently employed 43 , 59 , 60 .
Results and discussion
Material removal rate, tool wear ratio and average white layer thickness.
In Table 3 the experimental results regarding the MRR, TWR, and AWLT are presented.
MRR and TWR are major productivity and efficiency indexes, straight related with machining parameters, and affected by the machining power and the per pulse discharge energy. EDM is a multi-parameter complicated process, with a nonlinear response, indicating that increase in machining power, or in per pulse discharge energy, does not compulsorily leads in increased MRR. On the contrary, there is a limit to the maximum attained MRR, and by employing more intense machining conditions, e.g. increased per pulse energy, MRR not only may not increase, but it could be negatively affected. This MRR limit can be attributed to three main reasons: the plasma growth, the carbon decomposition and its subsequent deposition, and the debris concentration. Increased pulse duration leads to an expanding plasma channel that consumes a significant amount of energy. At the same time, high localized temperatures, due to increased pulse-on time, result to the decomposition of dielectric fluid's carbon, which subsequently is deposited and bonded on the electrode and the workpiece. This carbon and carbide layer, acts like a "barrier" and "shield", decreasing the machining efficiency and the MRR, while on the other hand, affects beneficially the electrode by shielding it and limiting its wear. Likewise, the debris that concentrate between the electrode and the workpiece act as a "barrier" as well. More intense machining conditions and/or extended pulse duration, increase their concentration, resulting to an amount of energy to be consumed by their re-melting. The in-brief aforementioned mechanisms that take place during machining indicate the complexity of the process, as well as the necessity of its comprehensive and thorough study. In Fig. 5 , the Main Effects Plot and the Interaction Plot of MRR are presented.
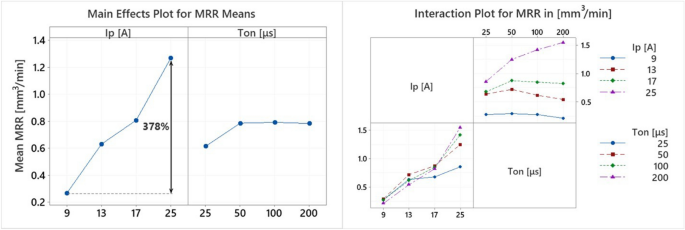
Main Effects Plot and Interaction Plot of MRR.
Obviously, the MRR behavior confirms the above discussed underlying mechanisms. The pulse-on current has a clear effect on MRR, as increase of I p results to an increase in MRR; as the interaction plot indicates, this tendency applies to all pulse-on times. Specifically, the mean MRR rises by 378% as the I p increases from 9 to 25A. On the other hand, T on affects in an ambiguous way the MRR, since, up to 50 μs pulse duration, an increase in MRR is recorded, while, for higher pulse-on times MRR is mainly stable or slightly decreased. Only for I p 25A the MRR keeps increasing for higher T on . These conclusions are in line with the relevant literature; Chen et al. 29 and Kumar et al. 39 have reported similar results concerning the EDM of Ti–6Al–4V, namely, the increase of T on beyond a certain limit is indifferently or even negatively affecting MRR. Taking in mind the obtained results, it would be justified to say that, as a rule of thumb, a higher MRR could easier be obtained by increasing the machining power, i.e., the pulse-on current, rather than by utilizing higher pulse-on times. On the other hand, these observations concern only MRR and no other machining results, namely TWR, AWLT or SQ, thus, a higher I p is not always an appropriate solution.
An exceedingly useful tool in production planning is the capability of accurately predicting the machining results in respect to the machining parameters. Thus, employing the RSM, a correlation model between pulse-on current, pulse-on time and MRR is proposed:
with MRR in [mm 3 /min], I p in [A], and T on in [μs].
The proposed model has a high level of fit, with R-sq greater than 95% and S value lower than 0.1. Moreover, the adequate fit is also confirmed by the p-value of the model, see Fig. 6 , which is less than 0.05. At this point, it is interesting to highlight that I p and the interaction of I p with T on have the highest contribution in MRR, implying that pulse-on current, and not pulse-on time, mainly affects the MRR, a conclusion that is in absolute agreement with experimental results. The model's appropriate fit that was theoretically analyzed above, is depicted in Fig. 6 where the MRR and the MRR Predicted values are plotted, having only a slight deviation.
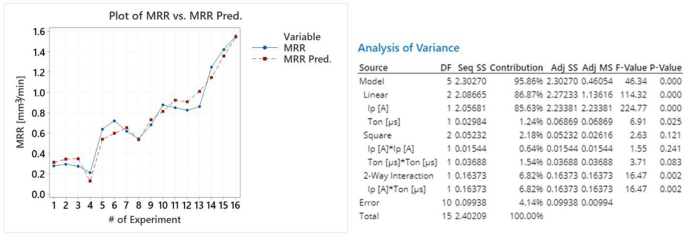
Plot of MRR vs MRR predicted and the model’s ANOVA.
TWR is the other major index for evaluating the efficiency and the feasibility of the process. Based on the theory and the previously discussed, TWR is expected to respond differently than MRR in respect to machining parameters variation. In Fig. 7 , the Main Effects Plot and the Interaction Plot for TWR are presented. The pulse-on current seems to have a fuzzy effect on TWR, since for 13A, a maximum is observed, while for further increase in I p , the mean TWR decreases. By a more in-depth analysis, and considering the interaction plot, it is concluded that indeed for 13A I p the highest TWR is recorded, while the lowest one, depending on the pulse-on time, is defined at 9 or 25A. On the other hand, T on affects the TWR in a clear manner. Namely, the increase of pulse-on time results to a consecutive decrease in TWR. Finally, the interaction plot indicates that only for I p of 9A, TWR has a vague response to the increase of T on . Kumar et al. 42 have presented analogous results and a similar interpretation regarding the shielding effect of decomposed carbon as well.
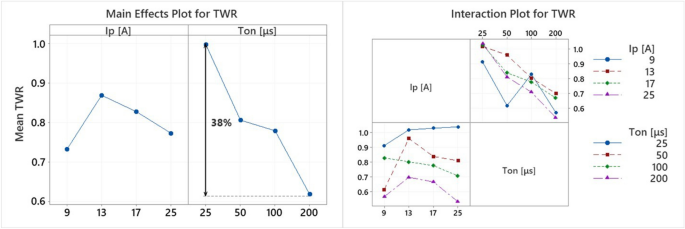
Main Effects Plot and Interaction Plot of TWR.
As in MRR, the prediction competence of TWR is extremely useful and advantageous in planning a feasible production. Hence, employing the RSM, a relation between machining parameters and TWR can be obtained:
with I p in [A], and T on in [μs].
The fit of the regression is considered sufficient, with R-sq 78.71%, S value less than 0.1, and model's P value 0.004, entailing that model can explain variations in the response. Comparatively with MRR, for TWR the parameter of major importance is the pulse-on time, having a 63.88% contribution, see Fig. 8 , to the model's total Sequential Sums of Squares (Seq SS). Finally, by plotting the TWR values along with the model predicted ones, see Fig. 8 , its suitability is confirmed, as it can follow the change of TWR in respect to machining parameters.
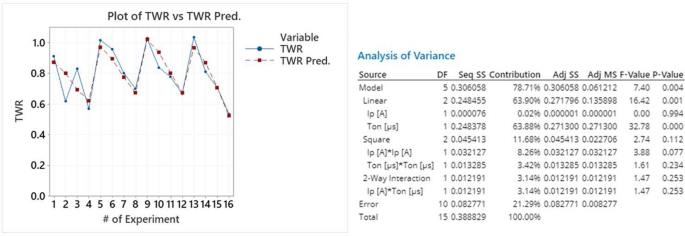
Plot of TWR vs TWR predicted and the model’s ANOVA.
Closing the current section, the formation of the WL will be discussed. During EDM, only an amount of molten material is efficiently removed by the workpiece, with the rest of it being re-solidified. Additionally, debris and/or ablated material that have remained close to the surface, may be re-condensed and adhered on the surface. The re-solidified and re-condensed material forms a layer known as White Layer. WL consist of amorphous material since it was solidified under extremely high cooling rates, thus it is easily distinct after the proper chemical etching. AWLT is an important parameter in EDM since it is related with the SQ, cracks formation and part's mechanical properties. Izman et al. 61 have pointed out that lower WL thickness reduces the risk of part premature failure during operation, while Mower 62 proved a reduction of fatigue strength, attributed to the presence of stress concentrating defects within the EDM recast layers. Thus, the AWLT is an important parameter in the production planning, as it is associated with manufactured component’s functionality. Figure 9 presents the change in AWLT depending on the machining parameters.
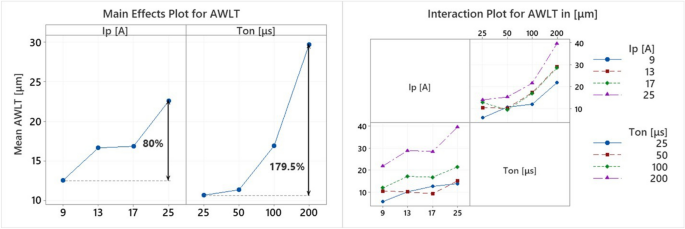
Main Effects Plot and Interaction Plot of AWLT.
As the Main Effects Plot and Interaction Plot of Fig. 9 indicate, the AWLT is clearly affected by pulse-on current and time. Increase of I p and T on results to higher AWLT. More specifically, as the machining current increased from 9 to 25A the AWLT was increased 80%, while an increase in T on from 25 to 200 μs resulted to a 179% thicker WL. The Interaction Plot totally confirms this interaction, since, in most cases, for more intense machining parameters, the AWLT increases. The rapid increase of the AWLT for higher pulse-on times consists a reasonable explanation regarding the reason behind the fact that for higher T on s the mean MRR does not keep increasing, but on the contrary, it is stabilized. Although a greater material volume melts, it is not be removed, but it is re-solidified forming a thicker WL. Hence, with an increase to pulse-on time, the mean MRR does not have a significant increase for T on s greater than 50 μs, while the mean AWLT is constantly increased.
The main causes of WL increased thickness for more intense pulse-on currents and times are the increase in machining power and per-pulse energy, as well the increasing inefficiency of the dielectric fluid to flush away the molten material. As the machining power increases for higher I p , the absorbed power increases, thus, a higher volume of molten material is formed. At the same time, prolonged pulses allow the electro-discharge energy to penetrate deeper into the material, making difficult the efficient flushing 63 , 64 . In Fig. 10 the successive forming of a thicker and more uniform WL, as the pulse-on time increases, is presented.
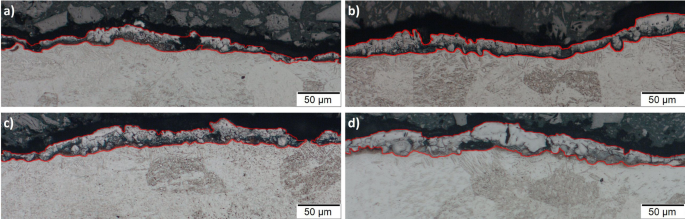
The WL for pulse-on current 17A and pulse-on times ( a ) 25 μs, ( b ) 50 μs, ( c ) 100 μs and ( d ) 200 μs.
Considering the importance to control the AWLT, a correlation between machining conditions and machining results is of extreme interest and practical importance. Again, based on RSM, a semi-empirical model is proposed:
with AWLT in [μm], I p in [A], and T on in [μs].
The regression fit is regarded as excellent, since the R-sq is over 96.5%, the S value 2.05 and model's P value approximately zero. Moreover, by plotting the AWLT along with model's predictions, see Fig. 11 , it is clear that the values have only a limited deviation. Finally, it is worth to point out, that based on the ANOVA table, see Fig. 11 , pulse-on current and pulse-on time have both significant contributions, although the contribution of T on is about 450% greater.
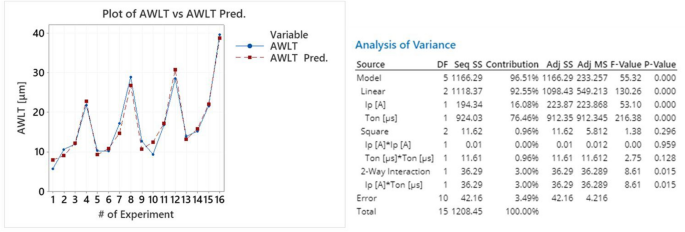
Plot of AWLT vs AWLT predicted and the model’s ANOVA.
Modeling and simulation-power distribution and plasma flushing efficiency
The numerical simulation provides a useful insight of the process, providing with data and specifics that are extremely difficult or even impossible to be obtained through experiments. Nevertheless, before proceeding in a further analysis, the accuracy and consistency of the model have to verified, in order for the robustness of the deduced conclusions to be ensured. The choice to introduce and base the analysis on "typical spark" was justified previously. Yet, and considering the chaotic nature of EDM 65 , a straight comparison between experimental and simulation results is complicated, involving high risk of error, thus, an indirect verification of the model is more appropriate.
Since consecutive sparks form a random-like surface, it would be inaccurate to compare the topography of the machined surface with the single crater's simulated geometry. Hence, bibliographic data were used to confirm that the simulated geometry is realistic and that "typical sparks" result to craters that in overlap can potentially form an EDM machined surface. In the studies of Klocke et al. 24 and Zhang et al. 56 it can be seen that EDM craters are shallow with high ratio of diameter to depth. In fact, sometimes the WL has almost fully filled the crater's cavity, indicating that very limited material removal occurred 66 . In Fig. 12 , the simulated craters for the lowest and highest per pulse discharge energies are depicted. The craters are shallow indeed, while they have a high ratio of diameter to depth. In Fig. 12 , the formatted WL is also presented. Since the craters may overlap, the most proper point to estimate the WL thickness is in the crater's center, thus, based on this measurement and assumption, all the subsequent calculations have been made. Obviously, the volume of WL is increased for higher pulse-on current and time. Finally, one last observation that endorses the trusting to the current model is the correlation between the radius of the plasma channel, the heat affected area and the molten area. Kojima et al. 67 through their experiments concluded that plasma channel diameter is significantly larger than that of the heat affected area, which in turn, is larger than molten area diameter. Practically, the latter means that due to the plasma channel spatial power distribution, there are areas where power density is inefficient to melt the material or even to affect it thermally. The simulation results of the current model, as shown in Fig. 12 , are absolutely in line with these findings.
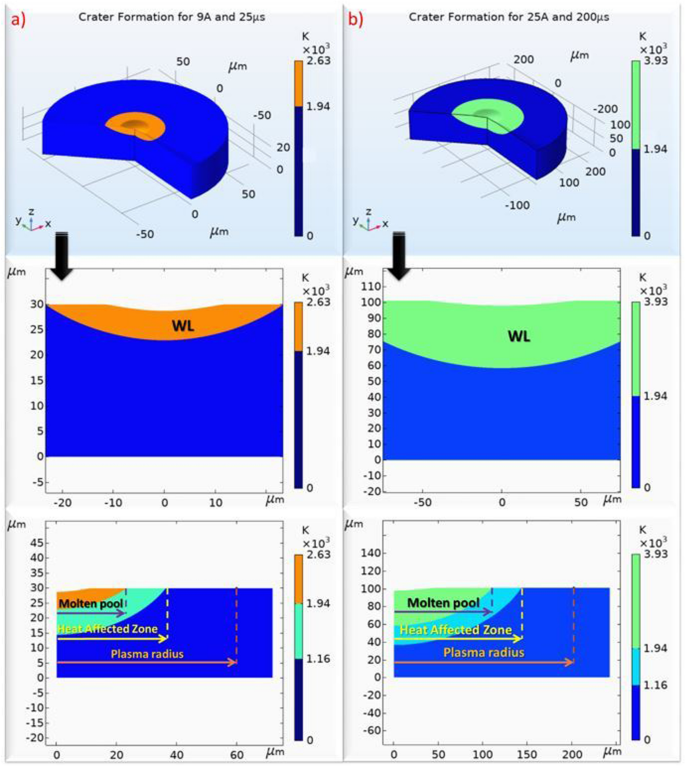
Simulated results for ( a ) 9A and 25 μs, ( b ) 25A and 200 μs where the formatted craters, as well as the WL and Heat Affected areas are depicted.
In Table 4 . The calculated absorption coefficients for the electrode (F el ) and the workpiece (F w ) are listed, as well as the Plasma Flushing Efficiency (PFE), as it is determined in simulations. These coefficients provide an insight of the process, granting a more complete and detailed understanding of the underlying physical mechanisms.
The energy absorption by the workpiece is definitely related to the machining efficiency and the MRR. Nevertheless, its correlation is quite different from the expected, explainable but thorny. In Fig. 13 , the Main Effects Plot and the Interaction Plot for F w are presented.
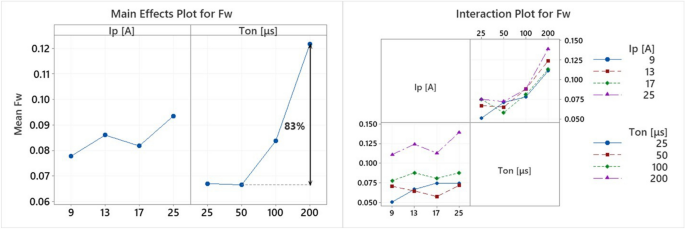
Main effects plot and interaction plot of F w .
Seemingly, the pulse-on time has a clear effect on the F w , while I p has a more vague one. More specifically, an increase in pulse-on time results to an increase in energy absorption by the workpiece of approximately 83%, while an increase in pulse-on current has an insignificant effect, as F w slightly increases or decreases. At first glance, this seems paradoxical and opposite with the above conclusions regarding MRR, since in MRR the I p has the major effect. However, the interpretation lies on the understanding of the undergoing heat transfer mechanism. Indeed, as the T on increases, a more expanded plasma channel is formed, hence, the heat transfer is facilitated, thus, increased amount of energy is absorbed by the workpiece. On the other hand, higher pulse-on current, i.e., higher machining power and power density, seems not to have any particular favorable or negative effect on energy absorption. The reasons why the higher energy absorption does not lead to higher MRR are the WL and the flushing capabilities. As there are limited flushing capabilities, greater volumes of molten material form thicker WL, thus, the increased absorbed energy is consumed by the melting and re-melting of the thick formed WL, and does not increase MRR. The aforementioned explanation is evidenced by the following analysis of PFE, where the PFE is significantly decreased for more intense machining parameters. The estimation of F w is important and useful, not only to accurate develop models and simulations, but as an index of machining efficiency in respect to the machining parameters. Thus, based in RSM, a correlation of F w with pulse-on current and time is proposed:
with I P in [A] and T on in [μs].
In the current model, only the linear terms were necessary to be included, with the T on having over 58% contribution on the Seq SS. The fit considered sufficient, as R-sq is over 90%, S value 0.0081, and model's P value almost zero. This semi-empirical relation is considered that can be employed in modeling single EDM sparks for titanium and titanium alloys with similar thermophysical properties.
As was previously mentioned, PFE is a decisive parameter for process efficiency, and attainment of high MRR. The PFE depends on machining conditions, namely pulse current, pulse duration, flushing method, and is related to the AWLT. Insufficient flushing, i.e., lower PFE, creates thicker WL, affecting the process in overall. In Fig. 14 , the Mean Effects Plot and the Interaction Plot of PFE are depicted. On the contrary with the former examined machining indexes, PFE correlation to the machining parameters is not clear. Nevertheless, some useful conclusions can be deduced; specifically, for the most intense machining parameters the lowest mean PFE was estimated, while for most pulse-on currents, the higher PFE was observed for pulses at 50 μs. These observations denoted that intense machining conditions lack of efficiency in power consumption, while, at the same time, there is an optimal set of parameters to maximize PFE.
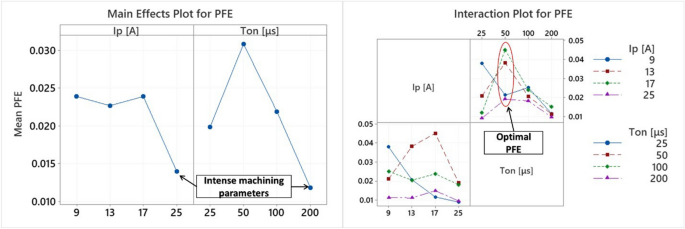
Main effects plot and interaction plot of PFE.
Since multiple factors affect the overall process feasibility and efficiency, e.g., TWR, and/or SQ requirements, the optimal machining conditions have to be carefully picked, as the case may be. Taking in mind all the above mentioned, regarding the utility to estimate accurately the PFE, based on RSM, a semi empirical correlation is proposed:
with I P in [A] , T on in [μs] and AWLT in [μs].
The model is adequately fitted to the data, having R-sq over 94%, S value less than 0.004, and P value 0.004. An important highlight at this point is that for PFE, for the regression model, apart from the pulse-on current and time that until now were employed, the AWLT was also integrated into the equation. The terms that include the AWLT contribute in Seq SS over 50%, implying and statistically prove the close relation between the PFE and the WL formation. In Fig. 15 , it is juxtaposed the material removal with a low PFE, Fig. 15 a with PFE = 0.9%, and with a theoretical PFE 100%, Fig. 15 b, without the formation of WL. A PFE of 100% is certainly unrealistic, but this comparison gives a notion regarding the potential of improvement in MRR, utilizing a more effective flushing of the molten material, and thus, increasing the PFE.
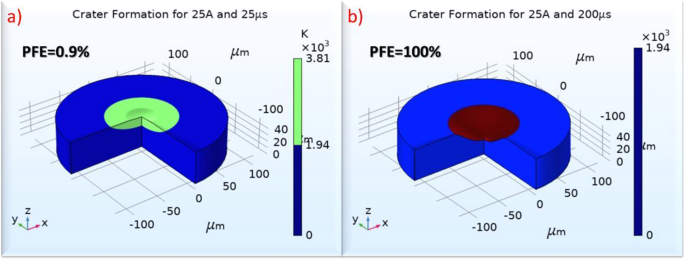
Craters formation for 25A and 25 μs with ( a ) PFE equals to experimental measured (0.9%), and ( b ) PFE = 100% (theoretical maximum with no WL formation).
The final process parameter that will be analyzed is the power absorption coefficient of the electrode (F el ). It is an interesting and important index, which can provide a clue about electrode’s erosion mechanism, and hence, the TWR. In Fig. 16 , the Main Effects Plot and the Interaction Plot of F el are presented.
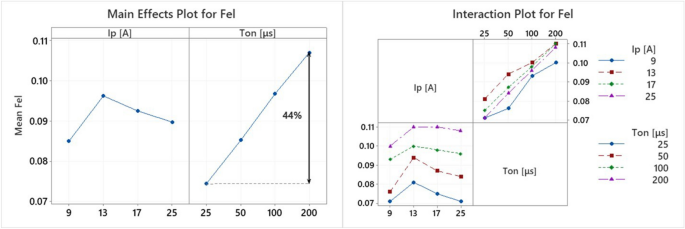
Main effects plot and interaction plot of F el .
F el follows similar pattern with F w , namely, changes in pulse-on current seems to have a fuzzy and trifling impact, while the change in pulse-on time has a clear effect. The increase of F el in respect to pulse duration is almost linear, for all the pulse-on currents; the mean F el increased approximately by 44% between 25 and 200 μs T on . The improvement of TWR for higher T on can be partially attributed to this change. Although more energy is absorbed by the electrode, causing additional wear, the F w is increased in almost double rate compared with F el , see Fig. 13 , thus, it may subsist increased wear, but, at the same time, increased material removal occurs, limiting in such a way the TWR. The second causation of lower TWR for high T on s is the power distribution, a conclusion that can be justified by the simulation results. Although with an increase in pulse-on time, higher proportion of energy is absorbed by the electrode, the power is distributed in a wider area, since the plasma channel radius increases, thus, the power density is significantly affected. This reduction is depicted in Fig. 17 , where the plasma channel's heat flux density is gradually decreased as T on increases.
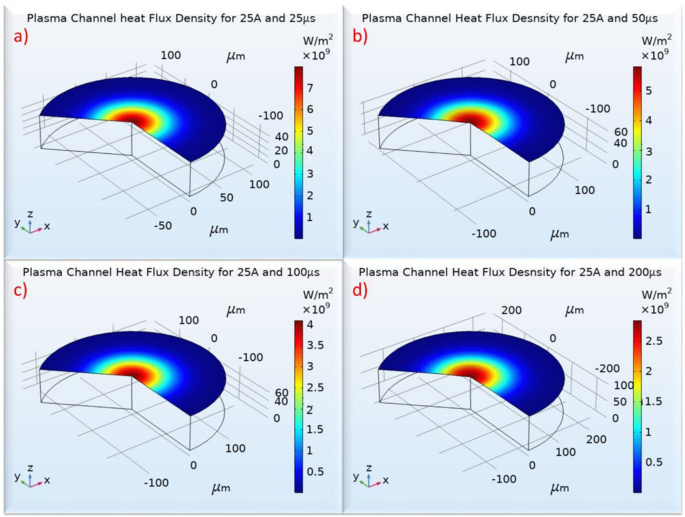
Electrode’s Plasma Channel’s Heat Flux for 25A and ( a ) 25 μs, ( b ) 50 μs, ( c ) 100 μs and ( d ) 200 μs.
The above-mentioned mechanisms result to a decrease in electrode's erosion rate and a delay in erosion initiation as T on increases. This can characteristically be observed in the plots of Fig. 18 , where for the same pulse-on current, the simulated erosion for higher pulse-on times is more "smooth" and delayed favoring a lower TWR.
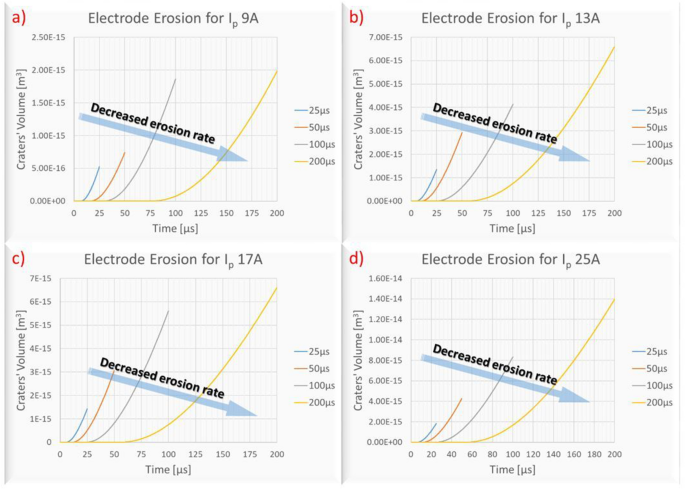
Erosion rates for different machining parameters for ( a ) 9A, ( b ) 13A, ( c ) 17A and ( d ) 25A.
Finally, by employing the RSM, a correlation between the F el and the machining parameters is proposed:
with I P in [A] , and T on in [μs]. The regression model is adequately fitted on the data, having R-sq over 94%, S value less than 0.004, and P value almost zero.
In closing, the concurrent erosion of electrode and workpiece is presented in Fig. 19 , through some characteristic snapshots of the simulation. More specifically:
At 2.2 μs the erosion of the workpiece starts, while the electrode's high sublimation temperature delays the electrode's erosion initiation.
At 3.4 μs the crater on the workpiece has been fully formed, and the material is overheated, forming the WL.
The temperature on the electrode reaches the sublimation limit at 5.5 μs, when the electrode erosion starts, with the delay that previously was mentioned.
At 25 μs, in the end of the pulse, the two craters have been formed, observing that due to the differences in materials' thermo-physical properties and the material removal mechanism (ablation vs melting), the electrode's crater is wider and shallower, while higher temperature gradients are developed in electrode due to its lower thermal conductivity.
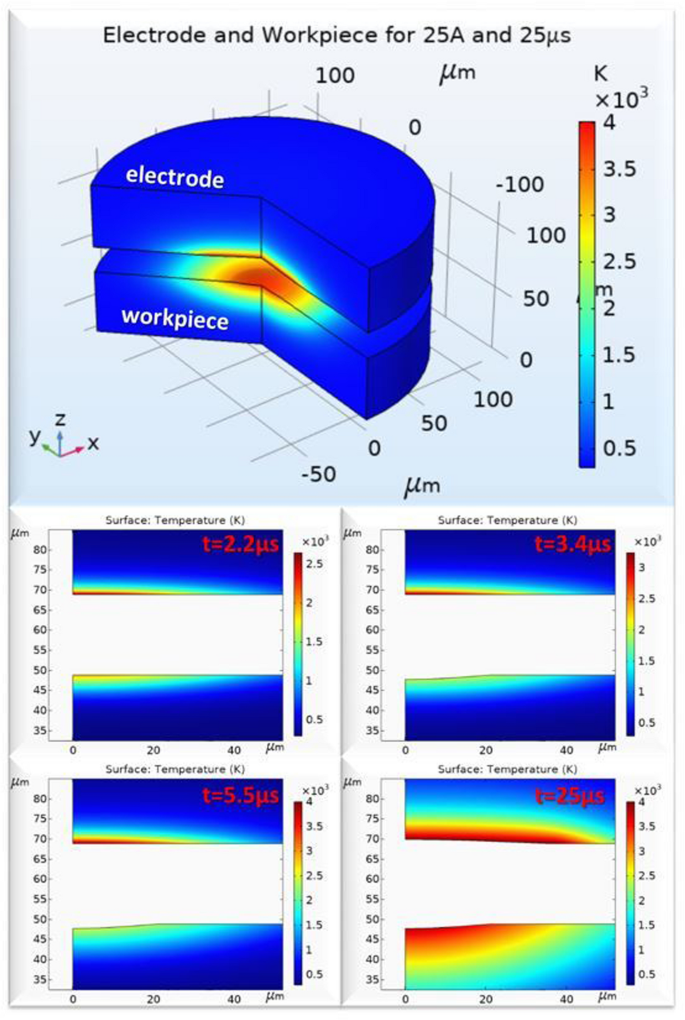
Electrode and workpiece erosion simulation.
Conclusions
In the current study, the machining of Titanium Grade2 with EDM was investigated, by combining a series of experiments with modeling, aiming to an insight view of the process. A full-scale experimental procedure was conducted, with control parameters the pulse-on current and pulse-on time, using graphite electrode. The MRR, TWR and AWLT were calculated as the major machining performance indexes. Moreover, a heat transfer numerical model with coupled deformed geometry was developed, in order to accurately and realistically simulate the spark erosion mechanism and to estimate parameters that cannot be obtained through experiments. Namely, the power distribution between electrode and workpiece was estimated, as well as the plasma flushing efficiency. For all the above-mentioned process indexes ANOVA was performed, while the Response Surface Methodology was employed in order to define the correlation between machining parameters and results, and to propose respective semi-empirical relations. Finally, emphasis was placed on the interpretation of the results, based on the undergoing physical mechanisms. In brief, the deduced conclusions of the current study are:
The MRR is mainly affected by the pulse-on current, with an increase of I p resulting to a higher MRR. The pulse-on time affects MRR in an ambiguous way, since there is an upper limit of MRR for a certain T on , and further increase has insignificant or negative result on MRR.
The TWR has a major dependence on pulse-on time, with an increase in pulse duration resulting to lower TWR. On the other hand, variations in I p have a fuzzy influence on TWR. The TWR is a percentage comparative index that indicates the ratio of material removal between the electrode and the workpiece, thus, it should not be straightly compared with MRR. TWR is an index of process’ efficiency in term of electrode consumption rate, and based on the experimental results, it is deduced that it increases for higher pulse-on times.
The AWLT is increased for more intense machining parameters, i.e., for higher pulse-on currents and times. The mean value of AWLT increased by 80% and 197.5% as the I p and T on increased from 9A to 25A and from 25 to 200 μs, respectively. The melted material volume increases as more intense machining parameters are employed, and hence, the re-solidified material volume is increased, too, forming a thicker WL.
The absorption coefficients F el and F w increase for higher pulse-on times, while pulse-on current seems to only slightly affect them.
As EDM is a multi-parameter and complex process, factors like the power density distribution, materials’ thermo-physical properties, flushing efficiency and combination of machining parameters have always to be considered in production planning, to optimize and render it as a feasible alternative.
Employing the proposed correlations about MRR, TWR and AWLT, an optimal machining planning can be achieved, as the case may be, saving time and resources. At the same time, modeling consists a robust analysis method, with realistic and accurate results, rendering simulation as a powerful tool in research for improving and optimizing the EDM process. Starting from the machining conditions and by following the presented methodology, a comprehensive and detailed view of the process and its results can be obtained.
Data availability
The datasets generated and analyzed during the current study are available from the corresponding author on reasonable request.
Fonda, P., Wang, Z., Yamazaki, K. & Akutsu, Y. A fundamental study on Ti-6Al-4V’s thermal and electrical properties and their relation to EDM productivity. J. Mater. Process. Technol. 202 , 583–589 (2008).
Article CAS Google Scholar
Jahan, M. P. Electrical Discharge Machining (EDM): Types, Technologies and Applications . (Nova Science Publishers, 2015).
Jameson, E. C. Electrical discharge machining . (Society of Manufacturing Engineers, 2001).
Mohd Abbas, N., Solomon, D. G. & Fuad Bahari, M. A review on current research trends in electrical discharge machining (EDM). Int. J. Mach. Tools Manuf. 47 , 1214–1228 (2007).
Qudeiri, J. E. A., Mourad, A. I., Ziout, A., Abidi, M. H. & Elkaseer, A. Electric discharge machining of titanium and its alloys : review. (2018).
Shabgard, M. R., Seyedzavvar, M., Oliaei, S. B. & Ivanov, A. A numerical method for predicting depth of heat affected zone in EDM process for AISI H13 tool steel. J. Sci. Ind. Res. (India) 70 , 493–499 (2011).
CAS Google Scholar
Shao, B. & Rajurkar, K. P. Modelling of the crater formation in micro-EDM. Procedia CIRP 33 , 376–381 (2015).
Article Google Scholar
Guo, Y. B., Klink, A. & Klocke, F. Multiscale modeling of sinking-EDM with Gaussian heat flux via user subroutine. Procedia CIRP 6 , 438–443 (2013).
Jithin, S., Raut, A., Bhandarkar, U. V. & Joshi, S. S. FE modeling for single spark in EDM considering plasma flushing efficiency. Procedia Manuf. 26 , 617–628 (2018).
DiBitonto, D. D., Eubank, P. T., Patel, M. R. & Barrufet, M. A. Theoretical models of the electrical discharge machining process. I. A simple cathode erosion model. J. Appl. Phys. 66 , 4095–4103 (1989).
Assarzadeh, S. & Ghoreishi, M. Electro-thermal-based finite element simulation and experimental validation of material removal in static gap singlespark die-sinking electro-discharge machining process. Proc. Inst. Mech. Eng. Part B J. Eng. Manuf. 231 , 28–47 (2017).
Tang, J. & Yang, X. A thermo-hydraulic modeling for the formation process of the discharge crater in EDM. Procedia CIRP 42 , 685–690 (2016).
Escobar, A. M., De Lange, D. F., Medellín Castillo, H. I. & Pérez Gutiérrez, F. G. Influence of modeling assumptions on the simulated EDM performance. ASME Int. Mech. Eng. Congr. Expo. Proc. 8 C , V08CT09A079 (2013).
Weingärtner, E., Kuster, F. & Wegener, K. Modeling and simulation of electrical discharge machining. Procedia CIRP 2 , 74–78 (2012).
Singh, H. Experimental study of distribution of energy during EDM process for utilization in thermal models. Int. J. Heat Mass Transf. 55 , 5053–5064 (2012).
Kumar, A., Bagal, D. K. & Maity, K. P. Numerical modeling of Wire Electrical Discharge machining of super alloy inconel 718. Procedia Eng. 97 , 1512–1523 (2014).
Tlili, A., Ghanem, F. & Salah, N. Ben. A contribution in EDM simulation field. Int. J. Adv. Manuf. Technol. 79 , 921–935 (2015).
Liu, J. F. & Guo, Y. B. Thermal modeling of EDM with progression of massive random electrical discharges. Procedia Manuf. 5 , 495–507 (2016).
Vishwakarma, U. K., Dvivedi, A. & Kumar, P. FEA modeling of material removal rate in electrical discharge machining of Al6063/SiC composites. Int. Conf. Mech. Ind. Manuf. Eng. (ICMIME, 2012), Zurich, Switzerland, Jan. 15–17. 6 , 586–591 (2012).
Salah, N. Ben, Ghanem, F. & Atig, K. Ben. Numerical study of thermal aspects of electric discharge machining process. Int. J. Mach. Tools Manuf. 46 , 908–911 (2006).
Khatter, C. P. & Pandey, O. P. Analysis of energy distribution during electric discharge machining of tungsten carbide. Tribol. - Mater. Surfaces Interfaces 3 , 2–15 (2009).
Oßwald, K., Schneider, S., Hensgen, L., Klink, A. & Klocke, F. Experimental investigation of energy distribution in continuous sinking EDM. CIRP J. Manuf. Sci. Technol. 19 , 36–43 (2017).
Shabgard, M., Ahmadi, R., Seyedzavvar, M. & Oliaei, S. N. B. Mathematical and numerical modeling of the effect of input-parameters on the flushing efficiency of plasma channel in EDM process. Int. J. Mach. Tools Manuf. 65 , 79–87 (2013).
Klocke, F. et al. A comparative study of polarity-related effects in single discharge EDM of titanium and iron alloys. Procedia CIRP 68 , 52–57 (2018).
Joshi, S. N. & Pande, S. S. Thermo-physical modeling of die-sinking EDM process. J. Manuf. Process. 12 , 45–56 (2010).
Manjaiah, M., Narendranath, S. & Basavarajappa, S. A review on machining of titanium based alloys using EDM and WEDM. Rev. Adv. Mater. Sci. 36 , 89–111 (2014).
Davim, P. J. Machining of Titanium Alloys . Machining of Titanium Alloys (Springer International Publishing, 2014). doi: https://doi.org/10.1007/978-3-662-43902-9 .
Klocke, F., Zeis, M., Klink, A. & Veselovac, D. Technological and economical comparison of roughing strategies via milling, EDM and ECM for titanium-and nickel-based blisks. Procedia CIRP 2 , 98–101 (2012).
Chen, S. L., Yan, B. H. & Huang, F. Y. Influence of kerosene and distilled water as dielectrics on the electric discharge machining characteristics of Ti–6A1–4V. J. Mater. Process. Technol. 87 , 107–111 (1999).
Lin, Y. C., Yan, B. H. & Chang, Y. S. Machining characteristics of titanium alloy (Ti–6Al–4V) using a combination process of EDM with USM. J. Mater. Process. Technol. 104 , 171–177 (2000).
Chow, H. M., Yan, B. H., Huang, F. Y. & Hung, J. C. Study of added powder in kerosene for the micro-slit machining of titanium alloy using electro-discharge machining. J. Mater. Process. Technol. 101 , 95–103 (2000).
Hasçalik, A. & Çaydaş, U. Electrical discharge machining of titanium alloy (Ti–6Al–4V). Appl. Surf. Sci. 253 , 9007–9016 (2007).
Article ADS CAS Google Scholar
Sivakumar, K. M. & Gandhinathan, R. Establishing optimum process parameters for machining titanium alloys ( Ti6–Al–4V ) in spark electric discharge machining. Int. J. Eng. Adv. Technol. 2 , 201–204 (2013).
Google Scholar
Mhatre, M. S., Sapkal, S. U. & Pawade, R. S. Electro discharge machining characteristics of Ti–6Al–4V alloy: a grey relational optimization. Procedia Mater. Sci. 5 , 2014–2022 (2014).
Shen, Y. et al. Determining the energy distribution during electric discharge machining of Ti–6Al–4V. Int. J. Adv. Manuf. Technol. 70 , 11–17 (2014).
Sen, I., Karthikeyan, G., Ramkumar, J. & Balasubramaniam, R. A study on machinability of B-modified Ti–6Al–4V alloys by EDM. Mater. Manuf. Process. 27 , 348–354 (2012).
Holsten, M., Koshy, P., Klink, A. & Schwedt, A. Anomalous influence of polarity in sink EDM of titanium alloys. CIRP Ann. 67 , 221–224 (2018).
Verma, V. & Sahu, R. Process parameter optimization of die-sinking EDM on Titanium grade -V alloy (Ti–6Al–4V) using full factorial design approach. Mater. Today Proc. 4 , 1893–1899 (2017).
Kumar, R. et al. Analysis of MRR and surface roughness in machining Ti–6Al–4V ELI titanium alloy using EDM process. Procedia Manuf. 20 , 358–364 (2018).
Nair, S., Dutta, A., Narayanan, R. & Giridharan, A. Investigation on EDM machining of Ti6Al4V with negative polarity brass electrode. Mater. Manuf. Process. 00 , 1–8 (2019).
Ahmed, N. et al. The potentiality of sinking EDM for micro-impressions on Ti-6Al-4V: keeping the geometrical errors (axial and radial) and other machining measures (tool erosion and work roughness) at minimum. Sci. Rep. https://doi.org/10.1038/s41598-019-52855-6 (2019).
Article PubMed PubMed Central Google Scholar
Kumar Baroi, B., Kar, S. & Kumar Patowari, P. Electric discharge machining of titanium grade 2 alloy and its parametric study. Mater. Today Proc. 5 , 5004–5011 (2018).
Bhaumik, M. & Maity, K. Effect of different tool materials during EDM performance of titanium grade 6 alloy. Eng. Sci. Technol. Int. J. 21 , 507–516 (2018).
Baroi, B. K., Debnath, T., Jagadish & Patowari, P. K. Machinability assessment of titanium grade 2 alloy using deionized water in EDM. Mater. Today Proc. 26 , 2221–2225 (2019).
Ahuja, N., Sharma, N., Hegab, H., Khanna, R. & Khan, A. M. Bioactivity measurement of commercially pure titanium processed by micro-electric discharge drilling. Int. J. Adv. Manuf. Technol. 107 , 2797–2805 (2020).
Sharma, N., Singh, G., Gupta, M., Hegab, H. & Mia, M. Investigations of surface integrity, bio-activity and performance characteristics during wire-electrical discharge machining of Ti-6Al-7Nb biomedical alloy. Mater. Res. Express 6 , (2019).
Shabgard, M., Seyedzavvar, M. & Oliaei, S. N. B. Influence of input parameters on the characteristics of the EDM process. Stroj. Vestnik/J. Mech. Eng. 57 , 689–696 (2011).
Roy, T., Datta, D. & Balasubramaniam, R. Numerical modelling, simulation and fabrication of 3-D hemi-spherical convex micro features using Reverse Micro EDM. J. Manuf. Process. 32 , 344–356 (2018).
Papazoglou, E. L., Markopoulos, A. P., Papaefthymiou, S. & Manolakos, D. E. Electrical discharge machining modeling by coupling thermal analysis with deformed geometry feature. Int. J. Adv. Manuf. Technol. https://doi.org/10.1007/s00170-019-03850-8 (2019).
Holman, J. P. Heat Transfer 10th Ed. High Education - Department of Mechanical Engineering Southern Methodist University (2008).
Moarrefzadeh, A. Study of workpiece thermal profile in Electrical Discharge Machining (EDM) process. WSEAS Trans. Appl. Theor. Mech. 7 , 83–92 (2012).
Shabgard, M. R., Shotorbani, R. M. & Alenabi, S. H. Mathematical Analysis of Electrical Discharge Machining on FW4 Weld Metal Keywords : (2012).
Mills, K. C. Recommended Values of Thermophysical Properties for Selected Commercial Alloys. Recommended Values of Thermophysical Properties for Selected Commercial Alloys (2002). doi: https://doi.org/10.1533/9781845690144 .
Nield, D. A. & Bejan, A. Convection in porous media. Convection Porous Media https://doi.org/10.1007/978-3-319-49562-0 (2017).
Article MATH Google Scholar
Pierson, H. Handbook of Carbon, Graphite, Diamond and Fullerenes. Noyes Publications (1994).
Zhang, Y. et al. Investigation on the influence of the dielectrics on the material removal characteristics of EDM. J. Mater. Process. Technol. 214 , 1052–1061 (2014).
Montgomery, D. C. Design and Analysis of Experiments . (Wiley, 2013).
Karkalos, N. E. & Markopoulos, A. P. Computational Methods for Application in Industry 4.0 . (Springer, 2019).
Pradhan, M. K. & Biswas, C. K. Modeling and analysis of process parameters on Surface Roughness in EDM of AISI D2 tool steel by RSM approach. World Acad. Sci. Eng. Technol. 33 , 814–819 (2009).
Rahman, M. M., Khan, M. A. R., Kadirgama, K., Noor, M. M. & Bakar, R. A. Modeling of material removal on machining of Ti–6Al–4V through EDM using copper tungsten electrode and positive polarity. World Acad. Sci. Eng. Technol. 71 , 576–581 (2010).
Izman, S., Hodsiyeh, D. G., Hamed, T., Rosliza, R. & Rezazadeh, M. Effects of adding multiwalled carbon nanotube into dielectric when EDMing titanium alloy. Adv. Mater. Res. 463–464 , 1445–1449 (2012).
Mower, T. M. Degradation of titanium 6Al–4V fatigue strength due to electrical discharge machining. Int. J. Fatigue 64 , 84–96 (2014).
Santos, I., Polli, M. L. & Daniel, H. Influence of input parameters on the electrical discharge machining of titanium alloy (TI-6AL-4V). Int. J. Manuf. Res. 10 , 286 (2015).
Lee, H. T., Hsu, F. C. & Tai, T. Y. Study of surface integrity using the small area EDM process with a copper-tungsten electrode. Mater. Sci. Eng. A https://doi.org/10.1016/j.msea.2003.08.046 (2004).
Aich, U. Investigation for the presence of chaos in surface topography generated by EDM. Tribol. Int. 120 , 411–433 (2018).
Zhang, Y. et al. A novel method of determining energy distribution and plasma diameter of EDM. Int. J. Heat Mass Transf. 75 , 425–432 (2014).
Kojima, A., Natsu, W. & Kunieda, M. Spectroscopic measurement of arc plasma diameter in EDM. CIRP Ann. - Manuf. Technol. 57 , 203–207 (2008).
Download references
Author information
Authors and affiliations.
School of Mechanical Engineering, Laboratory of Manufacturing Technology, National Technical University of Athens, Athens, Greece
Emmanouil L. Papazoglou, Panagiotis Karmiris-Obratański & Angelos P. Markopoulos
Department of Manufacturing Systems, Faculty of Mechanical Engineering and Robotics, AGH University of Science and Technology, Cracow, Poland
- Panagiotis Karmiris-Obratański
Department of Materials Science and Non-Ferrous Metals Engineering, Faculty of Non-Ferrous Metals, AGH University of Science and Technology, Cracow, Poland
Beata Leszczyńska-Madej
You can also search for this author in PubMed Google Scholar
Contributions
M.L.P. and P.K.-O. carried out the experiments, statistical analysis and simulations, while A.P.M and B.L.M. as supervisors, discussed the methods and the conclusions. Finally, all authors reviewed the paper.
Corresponding authors
Correspondence to Panagiotis Karmiris-Obratański or Angelos P. Markopoulos .
Ethics declarations
Competing interests.
The authors declare no competing interests.
Additional information
Publisher's note.
Springer Nature remains neutral with regard to jurisdictional claims in published maps and institutional affiliations.
Rights and permissions
Open Access This article is licensed under a Creative Commons Attribution 4.0 International License, which permits use, sharing, adaptation, distribution and reproduction in any medium or format, as long as you give appropriate credit to the original author(s) and the source, provide a link to the Creative Commons licence, and indicate if changes were made. The images or other third party material in this article are included in the article's Creative Commons licence, unless indicated otherwise in a credit line to the material. If material is not included in the article's Creative Commons licence and your intended use is not permitted by statutory regulation or exceeds the permitted use, you will need to obtain permission directly from the copyright holder. To view a copy of this licence, visit http://creativecommons.org/licenses/by/4.0/ .
Reprints and permissions
About this article
Cite this article.
Papazoglou, E.L., Karmiris-Obratański, P., Leszczyńska-Madej, B. et al. A study on Electrical Discharge Machining of Titanium Grade2 with experimental and theoretical analysis. Sci Rep 11 , 8971 (2021). https://doi.org/10.1038/s41598-021-88534-8
Download citation
Received : 18 September 2020
Accepted : 31 March 2021
Published : 26 April 2021
DOI : https://doi.org/10.1038/s41598-021-88534-8
Share this article
Anyone you share the following link with will be able to read this content:
Sorry, a shareable link is not currently available for this article.
Provided by the Springer Nature SharedIt content-sharing initiative
This article is cited by
Understanding the machined material’s behaviour in electro-discharge machining (edm) using a multi-phase smoothed particle hydrodynamics (sph) modelling.
- Ahmad W. Alshaer
- Ramy Abdallah
- Omonigho B. Otanocha
The International Journal of Advanced Manufacturing Technology (2024)
Machine learning for multi-dimensional performance optimization and predictive modelling of nanopowder-mixed electric discharge machining (EDM)
- Muhammad Sana
- Muhammad Asad
- Muhammad Talha
Experimental investigation and optimization with vegetable oil-based biodiesels as dielectric in EDM: green initiative towards sustainable manufacturing
- Rajnitu Rakshaskar
Journal of the Brazilian Society of Mechanical Sciences and Engineering (2024)
A comparative study on the effect of deep and shallow cryogenic electrodes on tool wear rate and overcut with waste bio-oil in electric discharge machining
- Kashif Ishfaq
- Muhammad Arif Mahmood
Theoretical and experimental analysis of plasma radius expansion model in EDM: a comprehensive study
- Emmanouil L. Papazoglou
- Angelos P. Markopoulos
The International Journal of Advanced Manufacturing Technology (2023)
By submitting a comment you agree to abide by our Terms and Community Guidelines . If you find something abusive or that does not comply with our terms or guidelines please flag it as inappropriate.
Quick links
- Explore articles by subject
- Guide to authors
- Editorial policies
Sign up for the Nature Briefing newsletter — what matters in science, free to your inbox daily.

Information
- Author Services
Initiatives
You are accessing a machine-readable page. In order to be human-readable, please install an RSS reader.
All articles published by MDPI are made immediately available worldwide under an open access license. No special permission is required to reuse all or part of the article published by MDPI, including figures and tables. For articles published under an open access Creative Common CC BY license, any part of the article may be reused without permission provided that the original article is clearly cited. For more information, please refer to https://www.mdpi.com/openaccess .
Feature papers represent the most advanced research with significant potential for high impact in the field. A Feature Paper should be a substantial original Article that involves several techniques or approaches, provides an outlook for future research directions and describes possible research applications.
Feature papers are submitted upon individual invitation or recommendation by the scientific editors and must receive positive feedback from the reviewers.
Editor’s Choice articles are based on recommendations by the scientific editors of MDPI journals from around the world. Editors select a small number of articles recently published in the journal that they believe will be particularly interesting to readers, or important in the respective research area. The aim is to provide a snapshot of some of the most exciting work published in the various research areas of the journal.
Original Submission Date Received: .
- Active Journals
- Find a Journal
- Proceedings Series
- For Authors
- For Reviewers
- For Editors
- For Librarians
- For Publishers
- For Societies
- For Conference Organizers
- Open Access Policy
- Institutional Open Access Program
- Special Issues Guidelines
- Editorial Process
- Research and Publication Ethics
- Article Processing Charges
- Testimonials
- Preprints.org
- SciProfiles
- Encyclopedia
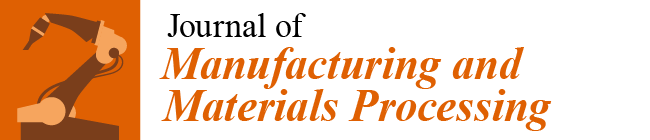
Article Menu

- Subscribe SciFeed
- Recommended Articles
- Google Scholar
- on Google Scholar
- Table of Contents
Find support for a specific problem in the support section of our website.
Please let us know what you think of our products and services.
Visit our dedicated information section to learn more about MDPI.
JSmol Viewer
Recent trends and developments in the electrical discharge machining industry: a review.
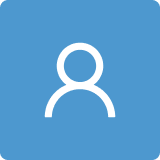
1. Introduction
2. edm processing, edm process physics, 3. empirical research, 3.1. factors affecting the process, 3.2. experimental data, 4. edm for surface engineering of biomaterials.
Click here to enlarge figure
Additives | Density (g/cm ) | Fusing Point (°C) | Electrical Resistivity (μ-ohm-cm) | Thermal Conductivity (W/mK) | Molecular Weight (gm/mol) |
---|---|---|---|---|---|
Hydroxyapatite (HA) | 3.08 | 1250 | Higher | 0.01 | 502.31 |
Silicon | 2.33 | 1410 | 6.00 × 10 | 150 | 28.08 |
SiC | 3.21 | 2987 | 1.00 × 10 | 500 | 40.11 |
Aluminum | 2.7 | 660 | 2.45 | 238 | 26.982 |
Titanium | 4.72 | 1668 | 55 | 22 | 47.88 |
TiO | 4.23 | 1855 | 1.00 × 10 | 7.4 | 79.866 |
CNT | 2 | 2800 | 50 | 4000 | N/M |
Graphite | 1.95 | 4550 | 500–3000 | 470 | 12.011 |
Silver | 10.49 | 962 | 1.6 | 430 | 107.86 |
Zinc | 7.13 | 420 | 5.5 | 120 | 65.38 |
Copper | 8.96 | 1083 | 1.59 | 416 | 63.54 |
Chromium | 7.16 | 1875 | 2.6 | 67 | 51.996 |
Molybdenum | 10.2 | 2610 | 5.27 | 139 | 95.94 |
Silicon | 2.33 | 1410 | 6.00 × 10 | 150 | 28.08 |
5. Optimization of Process Parameters
6. mathematical description of the edm process and numerical simulation, numerical simulation of the edm process, 7. process modification and development directions, 7.1. green manufacturing, 7.2. smart manufacturing, 7.3. new technological solutions, hybrid technologies, 8. discussion, the significant results identified during the review.
- The dependence of the black layer size on the pulse duty factor is observed when processing steel with a copper-tungsten electrode; the composition of the black layer is multi-element (the main chemical elements are carbon, silicon, iron, and oxygen) [ 85 ].
- Classical TE can no longer meet the ever-increasing demands in EDM. Modification of the creation methods and geometric features of the TE is required [ 140 , 146 ]. A hollow porous electrode allows for increasing the efficiency of using the working fluid by ~5 times; coating the electrode with a heat- and corrosion-resistant layer with increased strength allows for improving processing parameters by 10–25%, etc.
- Modification of EDM machines using various hybrid technologies is necessary to improve the final processing result [ 75 , 236 ]. The use of magnetism solves the short circuit problem, electrode vibration reduces the crater and effectively dissipates discharge energy, etc. But the use of magnetism or vibration further complicates the technology’s description.
- Taking into account the effect of corona discharging is required in models describing EDM with a curved geometry of the electrode working surface. This can be conducted using an electric model [ 200 ].
- Present EDM modeling approaches cannot describe the actual roughness and surface morphology after machining due to the limitations of the models in terms of describing pores formation and built-up zones near the crater [ 41 ].
9. Conclusions and Development Directions
- The need to generalize the data on the process, accumulated by the scientific group for more than 10 years of work, in a scientific monograph.
- Analysis of the EDM process using a workpiece and tool made from ordinary steel with a well-known and verified set of chemical, physical-mechanical, and thermomechanical properties.
- These areas of research are a priority for further development of the technological process, as well as mathematical and numerical methods for its description.
Author Contributions
Data availability statement, conflicts of interest.
- Priestley, J. The History and Present State of Electricity, with Original Experiments ; Cambridge University Press: London, UK, 1767. [ Google Scholar ]
- Chang, H. Joseph Priestly (1733–1804). Philos. Chem. 2012 , 6 , 55–62. [ Google Scholar ] [ CrossRef ]
- Ho, K.H.; Newman, S.T. State of the art electrical discharge machining (EDM). Int. J. Mach. Tools Manuf. 2003 , 43 , 1287–1300. [ Google Scholar ] [ CrossRef ]
- Jibin, T.P.; Jose, M.; Basil, K. Transition from EDM to PMEDM—Impact of suspended particulates in the dielectric on Ti6Al4V and other distinct material surfaces: A review. J. Manuf. Process. 2021 , 64 , 1105–1142. [ Google Scholar ] [ CrossRef ]
- Kartsev, V.P.V.V. Petrov’s Hypothetical Experiment and Electrical Experiments of the 18th Century. In Nature Mathematized ; Shea, W.R., Ed.; The University of Western Ontario Series in Philosophy of Science; Springer: Dordrecht, The Netherlands, 1983; Volume 20. [ Google Scholar ] [ CrossRef ]
- Bekaev, A.A.; Sokovikov, V.K.; Strokov, P.I. Electrohydraulic devices based on the Yutkin effect. Russ. Eng. Res. 2014 , 34 , 620–623. [ Google Scholar ] [ CrossRef ]
- Livshits, A.L. Electro-Erosion Machining of Metals ; Department of Scientific & Industrial Research, Butterworth & Co.: London, UK, 1960. [ Google Scholar ]
- Bibliography Boris Lazarenko. Available online: http://bsclupan.asm.md/src/userfiles/src/bibliografii/boris_lazarenko.pdf (accessed on 25 July 2023).
- Zia, M.K.; Pervaiz, S.; Anwar, S.; Samad, W.A. Reviewing Sustainability Interpretation of Electrical Discharge Machining Process using Triple Bottom Line Approach. Int. J. Precis. Eng. Manuf.-Green Technol. 2019 , 6 , 931–945. [ Google Scholar ] [ CrossRef ]
- Tricarico, C.; Delpretti, R.; Dauw, D.F. Geometrical Simulation of the EDM Die-Sinking Process. CIRP Ann. 1988 , 37 , 191–196. [ Google Scholar ] [ CrossRef ]
- DiBitonto, D.D.; Eubank, P.T.; Patel, M.R.; Barrufet, M.A. Theoretical models of the electrical discharge machining process. I. A simple cathode erosion model. J. Appl. Phys. 1989 , 66 , 4095–4103. [ Google Scholar ] [ CrossRef ]
- Patel, M.R.; Barrufet, M.A.; Eubank, P.T.; DiBitonto, D.D. Theoretical models of the electrical discharge machining process. II. The anode erosion model. J. Appl. Phys. 1989 , 66 , 4104–4111. [ Google Scholar ] [ CrossRef ]
- Eubank, P.T.; Patel, M.R.; Barrufet, M.A.; Bozkurt, B. Theoretical models of the electrical discharge machining process. III. The variable mass, cylindrical plasma model. J. Appl. Phys. 1993 , 73 , 7900–7909. [ Google Scholar ] [ CrossRef ]
- Yeo, S.H.; Kurnia, W.; Tan, P.C. Critical assessment and numerical comparison of electro-thermal models in EDM. J. Mater. Process. Technol. 2008 , 203 , 241–251. [ Google Scholar ] [ CrossRef ]
- Devgan, S.; Mahajan, A.; Singh, G.; Singh, G.; Sidhu, S.S. Surface Integrity of Powder Mixed Electrical Discharge Treated Substrate at High Discharge Energies. In Sustainable Machining Strategies for Better Performance. Lecture Notes in Mechanical Engineering ; Srinivasa Pai, P., Krishnaraj, V., Eds.; Springer: Singapore, 2022. [ Google Scholar ] [ CrossRef ]
- Sasmit, Y.; Rajesh, V. Multi Criteria Optimization Approaches in Electric Discharge Machining. Mater. Today Proc. 2019 , 18 , 4243–4252. [ Google Scholar ] [ CrossRef ]
- Sidhu, A.S. Surface texturing of non-toxic, biocompatible titanium alloys via electro-discharge. Rep. Mech. Eng. 2021 , 2 , 51–56. [ Google Scholar ] [ CrossRef ]
- Ming, W.; Zhang, S.; Zhang, G.; Du, J.; Ma, J.; He, W.; Cao, C.; Liu, K. Progress in modeling of electrical discharge machining process. Int. J. Heat Mass Transf. 2022 , 187 , 122563. [ Google Scholar ] [ CrossRef ]
- Tyagi, R.; Mandal, A.; Das, A.K.; Tripathi, A.; Prakash, C.; Campilho, R.; Saxena, K.K. Electrical Discharge Coating a Potential Surface Engineering Technique: A State of the Art. Processes 2022 , 10 , 1971. [ Google Scholar ] [ CrossRef ]
- Hasan, M.M.; Saleh, T.; Sophian, A.; Rahman, M.A.; Huang, T.; Ali, M.S.M. Experimental modeling techniques in electrical discharge machining (EDM): A review. Int. J. Adv. Manuf. Technol. 2023 , 127 , 2125–2150. [ Google Scholar ] [ CrossRef ]
- Devgan, S.; Mahajan, A.; Sidhu, S.S. Multi-walled carbon nanotubes in powder mixed electrical discharge machining: An experimental study, state of the art and feasibility prospect. Appl. Phys. A Mater. Sci. Process. 2021 , 127 , 806. [ Google Scholar ] [ CrossRef ]
- Jahan, M.P.; Rahman, M.; Wong, Y.S. A review on the conventional and micro-electrodischarge machining of tungsten carbide. Int. J. Mach. Tools Manuf. 2011 , 51 , 837–858. [ Google Scholar ] [ CrossRef ]
- Nahak, B.; Gupta, A. A review on optimization of machining performances and recent developments in electro discharge machining. Manuf. Rev. 2019 , 6 , 2. [ Google Scholar ] [ CrossRef ]
- Chen, Y.; Hu, S.; Li, A.; Cao, Y.; Zhao, Y.; Ming, W. Parameters Optimization of Electrical Discharge Machining Process Using Swarm Intelligence: A Review. Metals 2023 , 13 , 839. [ Google Scholar ] [ CrossRef ]
- Singh, T.; Sharma, S.; Sidhu, S.S.; Shlykov, E.S.; Ablyaz, T.R. Determination of Nanoindentation Behavior of HAZ on Glass Material Machined via ECSM Process through Simulation Approach. Materials 2022 , 15 , 5870. [ Google Scholar ] [ CrossRef ]
- Mahajan, A.; Devgan, S.; Kalyanasundaram, D. Surface alteration of Cobalt-Chromium and duplex stainless steel alloys for biomedical applications: A concise review. Mater. Manuf. Process. 2023 , 38 , 260–270. [ Google Scholar ] [ CrossRef ]
- Baroi, B.K.; Jagadish; Patowari, P.K. A review on sustainability, health, and safety issues of electrical discharge machining. J. Braz. Soc. Mech. Sci. Eng. 2022 , 44 , 59. [ Google Scholar ] [ CrossRef ]
- Zhu, X.; Li, G.; Mo, J.; Ding, S. Electrical discharge machining of semiconductor materials: A review. J. Mater. Res. Technol. 2023 , 25 , 4354–4379. [ Google Scholar ] [ CrossRef ]
- Grigoriev, S.N.; Hamdy, K.; Volosova, M.A.; Okunkova, A.A.; Fedorov, S.V. Electrical discharge machining of oxide and nitride ceramics: A review. Mater. Des. 2021 , 209 , 109965. [ Google Scholar ] [ CrossRef ]
- Shabgard, M.; Gholipoor, A.; Baseri, H. A review on recent developments in machining methods based on electrical discharge phenomena. Int. J. Adv. Manuf. Technol. 2016 , 87 , 2081–2097. [ Google Scholar ] [ CrossRef ]
- Choudhary, S.K.; Jadoun, R.S. Current Advanced Research Development of Electric Discharge Machining (EDM): A Review. Int. J. Res. Advent Technol. 2014 , 2 , 273–297. [ Google Scholar ]
- Nafi, M.A.; Jahan, M.P. Functional Surface Generation by EDM—A Review. Micromachines 2023 , 14 , 115. [ Google Scholar ] [ CrossRef ]
- Liu, Y.; Qu, J.; Zhao, K.; Zhang, X.; Zhang, S. Study of the High-Efficiency Ejecting-Explosion EDM of SiCp/Al Composite. Micromachines 2023 , 14 , 1315. [ Google Scholar ] [ CrossRef ] [ PubMed ]
- Laperrière, L.; Reinhart, G. CIRP Encyclopedia of Production Engineering ; Springer: Berlin/Heidelberg, Germany, 2014. [ Google Scholar ] [ CrossRef ]
- Schumacher, B.M. After 60 years of EDM the discharge process remains still disputed. J. Mater. Process. Technol. 2004 , 149 , 376–381. [ Google Scholar ] [ CrossRef ]
- Sidhu, S.S.; Singh, H.; Gepreel, M.A.H. A review on alloy design, biological response, and strengthening of β-titanium alloys as biomaterials. Mater. Sci. Eng. C 2021 , 121 , 111661. [ Google Scholar ] [ CrossRef ]
- Oniszczuk-Świercz, D.; Świercz, R.; Kopytowski, A.; Nowicki, R. Experimental Investigation and Optimization of Rough EDM of High-Thermal-Conductivity Tool Steel with a Thin-Walled Electrode. Materials 2023 , 16 , 302. [ Google Scholar ] [ CrossRef ] [ PubMed ]
- Burek, J.; Babiarz, R.; Płodzień, M.; Buk, J. The influence of electrode infeed in finishing wire electrical discharge machining process on disks fir tree slot accuracy. Mechanik 2018 , 10 , 915–917. [ Google Scholar ] [ CrossRef ]
- Le, V.T. The machined performance and recast layer properties of AISI H13 steel processed by powder mixed EDM process: An investigation and comparison in fine-finishing and semi-finishing processes. J. Braz. Soc. Mech. Sci. Eng. 2021 , 43 , 514. [ Google Scholar ] [ CrossRef ]
- Mahajan, A.; Devgan, S.; Kalyanasundaram, D. Electrical discharge machining—A futuristic technique for surface engineering of biomedical alloys: A concise review. Surf. Topogr. Metrol. Prop. 2022 , 10 , 043001. [ Google Scholar ] [ CrossRef ]
- Borges, P.; Hensen, M.; Marra, L.; Kuffa, M.; Wegener, K. Wire-EDM geometrical simulation based on superposition of single craters. Procedia CIRP 2023 , 117 , 243–248. [ Google Scholar ] [ CrossRef ]
- Grigoriev, S.N.; Volosova, M.A.; Okunkova, A.A.; Fedorov, S.V.; Hamdy, K.; Podrabinnik, P.A.; Pivkin, P.M.; Kozochkin, M.P.; Porvatov, A.N. Electrical Discharge Machining of Oxide Nanocomposite: Nanomodification of Surface and Subsurface Layers. J. Manuf. Mater. Process. 2020 , 4 , 96. [ Google Scholar ] [ CrossRef ]
- Rajeswari, R.; Shunmugam, M.S. Investigations into process mechanics of rough and finish die sinking EDM using pulse train analysis. Int. J. Adv. Manuf. Technol. 2019 , 100 , 1945–1964. [ Google Scholar ] [ CrossRef ]
- Kunieda, M.; Lauwers, B.; Rajurkar, K.P.; Schumacher, B.M. Advancing EDM through Fundamental Insight into the Process. CIRP Ann. 2005 , 54 , 64–87. [ Google Scholar ] [ CrossRef ]
- Singh, G.; Mahajan, A.; Devgan, S.; Sidhu, S.S. Comparison of Copper and Tungsten Electrodes for the Electric Discharge Machined SUS-316L. In Sustainable Machining Strategies for Better Performance. Lecture Notes in Mechanical Engineering ; Srinivasa Pai, P., Krishnaraj, V., Eds.; Springer: Singapore, 2022. [ Google Scholar ] [ CrossRef ]
- Sidhu, S.S.; Batish, A.; Kumar, S. Fabrication and electrical discharge machining of metal–matrix composites: A review. J. Reinf. Plast. Compos. 2013 , 32 , 1310–1320. [ Google Scholar ] [ CrossRef ]
- Richard, J.; Giandomenico, N. Electrode Profile Prediction and Wear Compensation in EDM-milling and Micro-EDM-Milling. Procedia CIRP 2018 , 68 , 819–824. [ Google Scholar ] [ CrossRef ]
- Hou, S.; Bai, J. Electrode wear prediction and offline compensation for micro-EDM drilling through-hole array using geometry simulation. Int. J. Adv. Manuf. Technol. 2022 , 120 , 6877–6889. [ Google Scholar ] [ CrossRef ]
- Jamkamon, K.; Janmanee, P. Improving Machining Performance for Deep Hole Drilling in the Electrical Discharge Machining Process Using a Step Cylindrical Electrode. Appl. Sci. 2021 , 11 , 2084. [ Google Scholar ] [ CrossRef ]
- Uhlmann, E.; Polte, J.; Streckenbach, J.; Dinh, N.C.; Yabroudi, S.; Polte, M.; Börnstein, J. High-Performance Electro-Discharge Drilling with a Novel Type of Oxidized Tool Electrode. J. Manuf. Mater. Process. 2022 , 6 , 113. [ Google Scholar ] [ CrossRef ]
- Ghanbarzadeh-Dagheyan, A.; Jalili, N.; Ahmadian, M.T. A holistic survey on mechatronic Systems in Micro/Nano scale with challenges and applications. J. Micro-Bio Robot. 2021 , 17 , 1–22. [ Google Scholar ] [ CrossRef ]
- Ji, Y.; Yang, W.; Wang, Y.; Li, Z. Overview of Foreign Inertial Technology Development. Lect. Notes Electr. Eng. 2022 , 861 , 975–983. [ Google Scholar ] [ CrossRef ]
- Fassi, I.; Modica, F. Editorial for the Special Issue on Micro-Electro Discharge Machining: Principles, Recent Advancements and Applications. Micromachines 2021 , 12 , 554. [ Google Scholar ] [ CrossRef ]
- Singh, T.; Dvivedi, A.; Sidhu, S.S.; Shlykov, E.S.; Muratov, K.R.; Ablyaz, T.R. Energy Channelization Analysis of Rough Tools Developed by RM-MT-EDM Process during ECSM of Glass Substrates. Materials 2022 , 15 , 5598. [ Google Scholar ] [ CrossRef ]
- Yukui, W.; Chen, X.; Gan, W.; Wang, Z.; Guo, C. Complex Rotary Structures Machined by Micro-WEDM. Procedia CIRP 2016 , 42 , 743–747. [ Google Scholar ] [ CrossRef ]
- Chen, Z.; Zhou, H.; Yan, Z.; Han, F.; Yan, H. Machining characteristics of 65 vol.% SiCp/Al composite in micro-WEDM. Ceram. Int. 2021 , 47 , 13533–13543. [ Google Scholar ] [ CrossRef ]
- Jahan, M.P.; Wong, Y.S.; Rahman, M. A study on the fine-finish die-sinking micro-EDM of tungsten carbide using different electrode materials. J. Mater. Process. Technol. 2009 , 209 , 3956–3967. [ Google Scholar ] [ CrossRef ]
- Zhang, L.; Du, J.; Zhuang, X.; Wang, Z.; Pei, J. Geometric prediction of conic tool in micro-EDM milling with fix-length compensation using simulation. Int. J. Mach. Tools Manuf. 2015 , 89 , 86–94. [ Google Scholar ] [ CrossRef ]
- Maddu, J.; Karrolla, B.; Shaik, R.U. Experimental optimization of electrical discharge coatings using conventional electrode. Mater. Sci. Eng. B 2022 , 286 , 116069. [ Google Scholar ] [ CrossRef ]
- Maddu, J.; Karrolla, B.; Shaik, R.U.; Elahi, H.; Arkanti, K. Evaluation of Bronze Electrode in Electrical Discharge Coating Process for Copper Coating. Micromachines 2023 , 14 , 136. [ Google Scholar ] [ CrossRef ] [ PubMed ]
- Yussefian, N.Z.; Koshy, P.; Buchholz, S.; Klocke, F. Electro-erosion edge honing of cutting tools. CIRP Ann. 2010 , 59 , 215–218. [ Google Scholar ] [ CrossRef ]
- Yussefian, N.Z.; Koshy, P. Geometric simulation of electro-erosion edge honing: Insights into process mechanisms. Precis. Eng. 2017 , 48 , 1–8. [ Google Scholar ] [ CrossRef ]
- Jibin, T.P.; Deepak, K.; Jose, M.; Basil, K. Experimental Investigations on the Tribological Performance of Electric Discharge Alloyed Ti-6Al-4V at 200–600 °C. J. Tribol. 2020 , 142 , 1–24. [ Google Scholar ] [ CrossRef ]
- Zhao, W.; Kang, X.; GU, L.; Xi, X.; Zhang, Y.; Hu, J.; Zhao, F. Application of electrical discharge machining technology in aerospace manufacturing. Acta Aeronaut. Astronaut. Sin. 2022 , 43 , 525936. Available online: https://hkxb.buaa.edu.cn/CN/10.7527/S1000-6893.2021.25936 (accessed on 10 October 2023).
- Petersen, T.; Zeis, M.; Bergs, T. Tolerance-Based Optimization of Sinking EDM for Industrial Seal Slot Manufacture. In Proceedings of the ASME Turbo Expo 2021: Turbomachinery Technical Conference and Exposition, New York, NY, USA, 7–11 June 2021. [ Google Scholar ] [ CrossRef ]
- Wang, J.; Gao, Q.; Xi, X.-C.; Zhao, W.-S. Experimental study of EDM milling of 3D-shaped diffuser for film cooling holes on turbine blades. Procedia CIRP 2022 , 113 , 160–165. [ Google Scholar ] [ CrossRef ]
- Natarajan, U.; Suganthi, X.H.; Periyanan, P.R. Modeling and Multiresponse Optimization of Quality Characteristics for the Micro-EDM Drilling Process. Trans. Indian Inst. Met. 2016 , 69 , 1675–1686. [ Google Scholar ] [ CrossRef ]
- Chaubey, S.K.; Gupta, K. Sustainable Manufacturing of Asymmetric Miniature-Sized Ratchet Wheels by Wire Electrical Discharge Machining. Machines 2022 , 10 , 506. [ Google Scholar ] [ CrossRef ]
- Wu, B.; Zhu, L.; Zhou, Z.; Guo, C.; Cheng, T.; Wu, X. An Efficient Method to Fabricate the Mold Cavity for a Helical Cylindrical Pinion. Processes 2023 , 11 , 2033. [ Google Scholar ] [ CrossRef ]
- Farooq, M.U.; Anwar, S.; Bhatti, H.A.; Kumar, M.S.; Ali, M.A.; Ammarullah, M.I. Electric Discharge Machining of Ti6Al4V ELI in Biomedical Industry: Parametric Analysis of Surface Functionalization and Tribological Characterization. Materials 2023 , 16 , 4458. [ Google Scholar ] [ CrossRef ] [ PubMed ]
- Mahajan, A.; Devgan, S.; Sidhu, S.S. Surface alteration of biomedical alloys by electrical discharge treatment for enhancing the electrochemical corrosion, tribological and biological performances. Surf. Coat. Technol. 2020 , 405 , 126583. [ Google Scholar ] [ CrossRef ]
- Geetha, M.; Singh, A.K.; Asokamani, R.; Gogia, A.K. Ti based biomaterials, the ultimate choice for orthopaedic implants—A review. Prog. Mater. Sci. 2009 , 54 , 397–425. [ Google Scholar ] [ CrossRef ]
- Aliofkhazraei, M. Modern Surface Engineering Treatments ; IntechOpen Limited: London, UK, 2013. [ Google Scholar ] [ CrossRef ]
- Bhattacharya, S.; Mishra, A.; Singh, T.; Bandyopadhyay, S.; Kumar, S.; Dey, G.K.; Kain, V. Simulation of residual stress using thermomechanical analysis on wire EDM cut surface. Mater. Today Proc. 2019 , 19 , 462–467. [ Google Scholar ] [ CrossRef ]
- Hou, S.; Bai, J.; Liu, H.; Zhou, Z.; Lu, Z. Study on material erosion mechanism of ultrasonic vibration-assisted micro-EDM based on heat-flow coupling analysis. Int. J. Adv. Manuf. Technol. 2023 , 125 , 465–478. [ Google Scholar ] [ CrossRef ]
- Yue, X.; Yang, X. Molecular dynamics simulation of material removal process and mechanism of EDM using a two-temperature model. Appl. Surf. Sci. 2020 , 528 , 147009. [ Google Scholar ] [ CrossRef ]
- Singh, H.; Singh, J.; Sharma, S.; Singh Chohan, J. Parametric Optimization of MRR & TWR of the Al6061/SiC MMCs Processed During Die-Sinking EDM Using Different Electrodes. Mater. Today Proc. 2021 , 48 , 1001–1008. [ Google Scholar ] [ CrossRef ]
- Ablyaz, T.R.; Shlykov, E.S.; Muratov, K.R. Modeling of EDM Process Flushing Mechanism. Materials 2023 , 16 , 4158. [ Google Scholar ] [ CrossRef ]
- Hess, R.; Heidemanns, L.; Herrig, T.; Klink, A.; Bergs, T. Model Based Prediction of the Heat Affected Zone in a Steel Workpiece Induced by an EDM Single Discharge. Procedia CIRP 2023 , 117 , 263–268. [ Google Scholar ] [ CrossRef ]
- Salvati, E.; Korsunsky, A.M. Micro-scale measurement & FEM modelling of residual stresses in AA6082-T6 Al alloy generated by wire EDM cutting. J. Mater. Process. Technol. 2020 , 275 , 116373. [ Google Scholar ] [ CrossRef ]
- Wu, H.; Ma, J.; Meng, Q.; Jahan, M.P.; Alavi, F. Numerical modeling of electrical discharge machining of Ti–6Al–4V. Procedia Manuf. 2018 , 26 , 359–371. [ Google Scholar ] [ CrossRef ]
- Fazli Shahri, H.R.; Mahdavinejad, R.; Ashjaee, M.; Abdullah, A. A comparative investigation on temperature distribution in electric discharge machining process through analytical, numerical and experimental methods. Int. J. Mach. Tools Manuf. 2017 , 114 , 35–53. [ Google Scholar ] [ CrossRef ]
- Yue, X.; Fan, J.; Li, Q.; Yang, X.; Xu, Z.; Chen, Z. Influence of Discharge Gap On Material Removal and Melt Pool Movement in EDM Discharge Process. Int. J. Adv. Manuf. Technol. 2021 , 119 , 7827–7842. [ Google Scholar ] [ CrossRef ]
- Li, Q.; Yang, X. Thermo-hydraulic analysis of melt pool dynamics and material removal on anode in electrical discharge machining. Int. J. Heat Mass Transf. 2023 , 203 , 123816. [ Google Scholar ] [ CrossRef ]
- Rizvi, S.; Agarwal, S.; Singh, A.; Bhardwaj, U.; Shukla, R. Modeling of Black Layer and Tool Wear in EDM of AISI 4340 using Cu-W electrode. Procedia CIRP 2020 , 95 , 389–392. [ Google Scholar ] [ CrossRef ]
- Sidhu, S.S.; Batish, A.; Kumar, S. Analysis of residual stresses in particulate reinforced aluminium matrix composite after EDM. Mater. Sci. Technol. 2015 , 31 , 1850–1859. [ Google Scholar ] [ CrossRef ]
- Philip, J.T.; Mathew, J.; Kuriachen, B. Numerical simulation of the effect of crater morphology for the prediction of surface roughness on electrical discharge textured Ti6Al4V. J. Braz. Soc. Mech. Sci. Eng. 2020 , 42 , 248. [ Google Scholar ] [ CrossRef ]
- Sidhu, S.S.; Batish, A.; Kumar, S. Neural network–based modeling to predict residual stresses during electric discharge machining of Al/SiC metal matrix composites. Proc. Inst. Mech. Eng. Part B J. Eng. Manuf. 2013 , 227 , 1679–1692. [ Google Scholar ] [ CrossRef ]
- Rajhi, W.; Alatawi, I.; Subhani, T.; Ayadi, B.; Al-Ghamdi, A.; Khaliq, A. A contribution to numerical prediction of surface damage and residual stresses on die-sinking EDM of Ti6Al4V. J. Manuf. Process. 2021 , 68 , 1458–1484. [ Google Scholar ] [ CrossRef ]
- Rebelo, J.C.; Morao Dias, A.; Kremer, D.; Lebrun, J.L. Influence of EDM pulse energy on the surface integrity of martensitic steels. J. Mater. Process. Technol. 1998 , 84 , 90–96. [ Google Scholar ] [ CrossRef ]
- Shabgard, M.; Ahmadi, R.; Seyedzavvar, M.; Oliaei, S.N.B. Mathematical and numerical modeling of the effect of inputparameters on the flushing efficiency of plasma channel in EDM process. Int. J. Mach. Tools Manuf. 2013 , 65 , 79–87. [ Google Scholar ] [ CrossRef ]
- Singh, H. Experimental study of distribution of energy during EDM process for utilization in thermal models. Int. J. Heat Mass Transf. 2012 , 55 , 5053–5064. [ Google Scholar ] [ CrossRef ]
- Sahoo, R.; Singh, N.K.; Bajpai, V. A novel approach for modeling MRR in EDM process using utilized discharge energy. Mech. Syst. Signal Process. 2023 , 185 , 109811. [ Google Scholar ] [ CrossRef ]
- Gostimirovic, M.; Kovac, P.; Sekulic, M. An inverse optimal control problem in the electrical discharge machining. Sādhanā 2018 , 43 , 70. [ Google Scholar ] [ CrossRef ]
- Yushchishina, A.N.; Kuskova, N.I.; Malyushevskaya, A.P. Study of chemical reactions during electric discharge in water and aqueous solutions of inorganic substances. Surf. Eng. Appl. Electrochem. 2007 , 43 , 347–349. [ Google Scholar ] [ CrossRef ]
- Gopal, R.; Thangadurai, K.R.; Thirunavukkarasu, K. Behavior of ECAP processed copper electrodes in electrical discharge machining of AISI H13 steel. Mater. Today Proc. 2020 , 21 , 295–298. [ Google Scholar ] [ CrossRef ]
- Shastri, R.K.; Mohanty, C.P.; Dash, S.; Gopal, K.M.P.; Annamalai, A.R.; Jen, C.-P. Reviewing Performance Measures of the Die-Sinking Electrical Discharge Machining Process: Challenges and Future Scopes. Nanomaterials 2022 , 12 , 384. [ Google Scholar ] [ CrossRef ]
- Ishfaq, K.; Sana, M.; Mahmood, M.A.; Anwar, S.; Waseem, M.U. Evaluating Surface Quality of Inconel 617 by Employing Deep Cryogenically Treated Electrodes in Surfactant-Added Dielectrics of Transformer Oil. Metals 2023 , 13 , 1092. [ Google Scholar ] [ CrossRef ]
- Patel, K.M.; Pulak, M.; Pandey, P.; Venkateswara, R. Surface integrity and material removal mechanisms associated with the EDM of Al 2 O 3 ceramic composite. Int. J. Refract. Met. Hard Mater. 2009 , 27 , 892–899. [ Google Scholar ] [ CrossRef ]
- Lauwers, B.; Kruth, J.P.; Liu, W.; Eeraerts, W.; Schacht, B.; Bleys, P. Investigation of material removal mechanisms in EDM of composite ceramic materials. J. Mater. Process. Technol. 2004 , 149 , 347–352. [ Google Scholar ] [ CrossRef ]
- Oßwald, K.; Brandl, L.; Lochmahr, I. Experimental investigation into material removal mechanisms in High Speed Wire EDM. Int. J. Adv. Manuf. Technol. 2020 , 111 , 2163–2170. [ Google Scholar ] [ CrossRef ]
- Yue, X.; Li, Q.; Yang, X. Influence of thermal stress on material removal of Cf_SiC composite in EDM. Ceram. Int. 2020 , 46 , 7998–8009. [ Google Scholar ] [ CrossRef ]
- Anand, B.; Giri, A.; Mohanty, C.P.; Sharma, D. Tool wear and energy consumption optimization in EDM of chromium tool steel. Mater. Today Proc. 2021 , 43 , 268–272. [ Google Scholar ] [ CrossRef ]
- Straka, L.; Šmidová, S. Optimization of material removal rate and tool wear rate of Cu electrode in die-sinking EDM of tool steel. Int. J. Adv. Manuf. Technol. 2018 , 97 , 389–392. [ Google Scholar ] [ CrossRef ]
- Jaharah, A.G.; Liang, C.G.; Wahid, S.Z.; Ab Rahman, M.N.; Che Hassan, C.H. Performance of copper electrode in electical discharge machining (EDM) of AISI H13 harden steel. Int. J. Mech. Mater. Eng. 2008 , 3 , 25–29. [ Google Scholar ]
- Ablyaz, T.R.; Shlykov, E.S.; Muratov, K.R. Improving the Efficiency of Electrical Discharge Machining of Special-Purpose Products with Composite Electrode Tools. Materials 2021 , 14 , 6105. [ Google Scholar ] [ CrossRef ]
- Torres, A.; Luis, C.J.; Puertas, I. EDM machinability and surface roughness analysis of TiB2, using copper electrodes. J. Alloys Compd. 2017 , 690 , 337–347. [ Google Scholar ] [ CrossRef ]
- Kiyak, M.; Aldemir, B.; Altan, E. Effects of discharge energy density on wear rate and surface roughness in EDM. Int. J. Adv. Manuf. Technol. 2015 , 79 , 513–518. [ Google Scholar ] [ CrossRef ]
- Gov, K. The effects of the dielectric liquid temperature on the hole geometries drilled by electro erosion. Int. J. Adv. Manuf. Technol. 2017 , 92 , 1255–1262. [ Google Scholar ] [ CrossRef ]
- Hosseini Kalajahi, M.; Rash Ahmadi, S.; Nadimi Bavil Oliaei, S. Experimental and finite element analysis of EDM process and investigation of material removal rate by response surface methodology. Int. J. Adv. Manuf. Technol. 2013 , 69 , 687–704. [ Google Scholar ] [ CrossRef ]
- Sidhu, S.S.; Ablyaz, T.R.; Bains, P.S.; Muratov, K.R.; Shlykov, E.S.; Shiryaev, V.V. Parametric Optimization of Electric Discharge Machining of Metal Matrix Composites Using Analytic Hierarchy Process. Micromachines 2021 , 12 , 1289. [ Google Scholar ] [ CrossRef ] [ PubMed ]
- Ablyaz, T.R.; Bains, P.S.; Sidhu, S.S.; Muratov, K.R.; Shlykov, E.S. Impact of Magnetic Field Environment on the EDM Performance of Al-SiC Metal Matrix Composite. Micromachines 2021 , 12 , 469. [ Google Scholar ] [ CrossRef ]
- Ablyaz, T.R.; Shlykov, E.S.; Muratov, K.R.; Sidhu, S.S. Analysis of Wire-Cut Electro Discharge Machining of Polymer Composite Materials. Micromachines 2021 , 12 , 571. [ Google Scholar ] [ CrossRef ] [ PubMed ]
- Ablyaz, T.R.; Shlykov, E.S.; Muratov, K.R.; Zhurin, A.V. Study of the EDM Process of Bimetallic Materials Using a Composite Electrode Tool. Materials 2022 , 15 , 750. [ Google Scholar ] [ CrossRef ]
- Gostimirovic, M.; Radovanovic, M.; Madic, M.; Rodic, D.; Kulundzic, N. Inverse electro-thermal analysis of the material removal mechanism in electrical discharge machining. Int. J. Adv. Manuf. Technol. 2018 , 97 , 1861–1871. [ Google Scholar ] [ CrossRef ]
- Qin, Z.; Wu, Y.-T.; Eizad, A.; Lyu, S.-K.; Lee, C.-M. Advancement of Mechanical Engineering in Extreme Environments. Int. J. Precis. Eng. Manuf.-Green Technol. 2021 , 8 , 1767–1782. [ Google Scholar ] [ CrossRef ]
- Hriţuc, A.; Coteaţă, M.; Dodun, O.; Nagîţ, G.; Slătineanu, L.; Rîpanu, M.I.; Beşliu, I.; Mikhailov, A. Wear of the tool electrode at simultaneous electrical discharge machining of different materials. Procedia CIRP 2020 , 95 , 419–424. [ Google Scholar ] [ CrossRef ]
- Bachchhav, B.D.; Gadakh, V.S. Relative Tool Wear and Metal Removal Studies in Electric Discharge Machining of AISI D2 Die Steel Using Cu-Cr-Zr, Cu-Cd, and Cu-Be Electrode Materials. J. Mater. Eng. Perform. 2023 . [ Google Scholar ] [ CrossRef ]
- Bańkowski, D.; Młynarczyk, P. Influence of EDM Process Parameters on the Surface Finish of Alnico Alloys. Materials 2022 , 15 , 7277. [ Google Scholar ] [ CrossRef ]
- Devgan, S.; Sidhu, S.S.; Mahajan, A. Capabilities of Powder-Mixed EDM using Carbon Nanotubes for Biomedical Application. In Non-Conventional Hybrid Machining Processes ; CRC Press: Boca Raton, FL, USA, 2020; pp. 95–104. [ Google Scholar ]
- Singh, M.; Maharana, S.; Yadav, A.; Singh, R.; Maharana, P.; Nguyen, T.V.T.; Yadav, S.; Loganathan, M.K. An Experimental Investigation on the Material Removal Rate and Surface Roughness of a Hybrid Aluminum Metal Matrix Composite (Al6061/SiC/Gr). Metals 2021 , 11 , 1449. [ Google Scholar ] [ CrossRef ]
- Daud, N.D.; Hasan, M.N.; Saleh, T.; Leow, P.L.; Ali, M.S.M. Non-traditional machining techniques for silicon wafers. Int. J. Adv. Manuf. Technol. 2022 , 121 , 29–57. [ Google Scholar ] [ CrossRef ]
- Singh, S.; Maheshwari, S.; Pandey, P.C. Some investigations into the electric discharge machining of hardened tool steel using different electrode materials. J. Mater. Process. Technol. 2004 , 149 , 272–277. [ Google Scholar ] [ CrossRef ]
- Niamat, M.; Sarfraz, S.; Shehab, E.; Ismail, S.O.; Khalid, Q.S. Experimental Characterization of Electrical Discharge Machining of Aluminum 6061 T6 Alloy using Different Dielectrics. Arab. J. Sci. Eng. 2019 , 44 , 8043–8052. [ Google Scholar ] [ CrossRef ]
- Yu, H.-L.; Luan, J.-J.; Li, J.-Z.; Zhang, Y.-S.; Yu, Z.; Guo, D.-M. A new electrode wear compensation method for improving performance in 3D micro EDM milling. J. Micromech. Microeng. 2010 , 20 , 055011. [ Google Scholar ] [ CrossRef ]
- Shabgard, M.; Khosrozadeh, B. Investigation of carbon nanotube added dielectric on the surface characteristics and machining performance of Ti–6Al–4V alloy in EDM process. J. Manuf. Process. 2017 , 25 , 212–219. [ Google Scholar ] [ CrossRef ]
- Bhaumik, M.; Maity, K. Effect of Different Tool Materials during EDM Performance of Titanium Grade 6 Alloy. Eng. Sci. Technol. Int. J. 2018 , 21 , 507–516. [ Google Scholar ] [ CrossRef ]
- Rao, K.M.; Kumar, D.V.; Shekar, K.C.; Singaravel, B. Optimization of EDM process parameters using TOPSIS for machining AISI D2 steel material. Mater. Today Proc. 2021 , 46 , 701–706. [ Google Scholar ] [ CrossRef ]
- Nas, E.; Özbek, O.; Bayraktar, F.; Kara, F. Experimental and Statistical Investigation of Machinability of AISI D2 Steel Using Electroerosion Machining Method in Different Machining Parameters. Adv. Mater. Sci. Eng. 2021 , 2021 , 1241797. [ Google Scholar ] [ CrossRef ]
- Amorim, F.; Weingaertner, W. The Behavior of Graphite and Copper Electrodes on the Finish Die-Sinking Electrical Discharge Machining (EDM) of AISI P20 Tool Steel. J. Braz. Soc. Mech. Sci. Eng. 2007 , 29 , 366–371. [ Google Scholar ] [ CrossRef ]
- Kumar, S.; Batra, U. Surface modification of die steel materials by EDM method using tungsten powder-mixed dielectric. J. Manuf. Process. 2012 , 14 , 35–40. [ Google Scholar ] [ CrossRef ]
- Rizvi, S.A.H.; Agarwal, S. An Investigation on surface integrity in EDM Process with a Copper Tungsten Electrode. Procedia CIRP 2016 , 42 , 612–617. [ Google Scholar ] [ CrossRef ]
- Dilip, D.G.; Ananthan, S.P.; Panda, S.; Mathew, J. Numerical simulation of the influence of fluid motion in mushy zone during micro-EDM on the crater surface profile of Inconel 718 alloy. J. Braz. Soc. Mech. Sci. Eng. 2019 , 41 , 107. [ Google Scholar ] [ CrossRef ]
- Ishfaq, K.; Sana, M.; Rehman, M.; Anwar, S.; Alfaify, A.Y.; Zia, A.W. Role of biodegradable dielectrics toward tool wear and dimensional accuracy in Cu-mixed die sinking EDM of Inconel 600 for sustainable machining. J. Braz. Soc. Mech. Sci. Eng. 2023 , 45 , 235. [ Google Scholar ] [ CrossRef ]
- Jing, Q.; Zhang, Y.; Kong, L.; Xu, M.; Ji, F. An Investigation into Accumulative Difference Mechanism in Time and Space for Material Removal in Micro-EDM Milling. Micromachines 2021 , 12 , 711. [ Google Scholar ] [ CrossRef ]
- Heo, S.; Jeong, Y.H.; Min, B.-K.; Lee, S.J. Virtual EDM simulator: Three-dimensional geometric simulation of micro-EDM milling processes. Int. J. Mach. Tools Manuf. 2009 , 49 , 1029–1034. [ Google Scholar ] [ CrossRef ]
- Rafaqat, M.; Mufti, N.A.; Ahmed, N.; Rehman, A.U.; AlFaify, A.Y.; Farooq, M.U.; Saleh, M. Hole-Making in D2-Grade Steel Tool by Electric-Discharge Machining through Non-Conventional Electrodes. Processes 2022 , 10 , 1553. [ Google Scholar ] [ CrossRef ]
- Uhlmann, E.; Roehner, M. Investigations on reduction of tool electrode wear in micro-EDM using novel electrode materials. CIRP J. Manuf. Sci. Technol. 2008 , 1 , 92–96. [ Google Scholar ] [ CrossRef ]
- Wang, Y.; Zhao, F.; Wang, J. Wear-resist Electrodes for Micro-EDM. Chin. J. Aeronaut. 2009 , 22 , 339–342. [ Google Scholar ] [ CrossRef ]
- Shirguppikar, S.; Patil, M.S.; Nguyen Huu, P.; Muthuramalingam, T.; Dong, P.; Tam, N.C.; Tien Tai, B.; Minh, N.D.; Duc, N.V. Assessing the Effects of Uncoated and Coated Electrode on Response Variables in Electrical Discharge Machining for Ti-6Al-4V Titanium Alloy. Tribol. Ind. 2021 , 43 , 524–534. [ Google Scholar ] [ CrossRef ]
- Chiou, A.H.; Tsao, C.C.; Hsu, C.Y. A study of the machining characteristics of micro EDM milling and its improvement by electrode coating. Int. J. Adv. Manuf. Technol. 2015 , 78 , 1857–1864. [ Google Scholar ] [ CrossRef ]
- Inaba, Y.; Li, S.; Yamaguchi, A.; Okada, A. Fundamental Study on Internal Space Forming by EDM. Procedia CIRP 2020 , 95 , 215–219. [ Google Scholar ] [ CrossRef ]
- Sato, T.; Imai, Y.; Goto, A.; Magara, T.; Takuchi, A.; Watanabe, K. A new grooving method based on steady wear model in EDM. Int. J. Electr. Mach. 2000 , 5 , 41–49. [ Google Scholar ] [ CrossRef ]
- Shervani-Tabar, M.T.; Rambarzin, F.; Shabgard, M.R.; Payamani, R. Numerical study on the dynamics of an electrical discharge generated vapor bubble in EDM with different shapes of the tool and the workpiece. Int. J. Adv. Manuf. Technol. 2011 , 56 , 151–159. [ Google Scholar ] [ CrossRef ]
- Narasimham, G.S.V.L.; Babu, A.V.; Reddy, S.S.; Dhanasekaran, R. Recent Trends in Mechanical Engineering. Lecture Notes in Mechanical Engineering ; Springer: Singapore, 2020. [ Google Scholar ] [ CrossRef ]
- Jiang, Y.; Kong, L.; Yu, J.; Hua, C.; Zhao, W. Experimental research on preparation and machining performance of porous electrode in electrical discharge machining. J. Mech. Sci. Technol. 2022 , 36 , 6201–6215. [ Google Scholar ] [ CrossRef ]
- Mahajan, A.; Singh, G.; Devgan, S. Additive manufacturing of metallic biomaterials: A concise review. Arch. Civ. Mech. Eng. 2023 , 23 , 187. [ Google Scholar ] [ CrossRef ]
- Yi, J.; Qi, L.; Kong, L.; Ping, X.; Zhao, W. Electrical Discharge Machining for Complex Cavity with a Porous Electrode. Procedia CIRP 2016 , 42 , 618–622. [ Google Scholar ] [ CrossRef ]
- Shen, X.Y.; Xu, H.Q.; Gerdroodbary, M.B.; Valiallah Mousavi, S.; Musa Abazari, A.; Imani, S.M. Numerical simulation of blood flow effects on rupture of aneurysm in middle cerebral artery. Int. J. Mod. Phys. C 2021 , 33 , 1–18. [ Google Scholar ] [ CrossRef ]
- Munir, K.; Biesiekierski, A.; Wen, C.; Li, Y. Surface modifications of metallic biomaterials. Met. Biomater. Process. Med. Device Manuf. 2020 , 2020 , 387–424. [ Google Scholar ] [ CrossRef ]
- Devgan, S.; Sidhu, S.S. Evolution of surface modification trends in bone related biomaterials: A review. Mater. Chem. Phys. 2019 , 233 , 68–78. [ Google Scholar ] [ CrossRef ]
- Al-Amin, M.; Abdul-Rani, A.M.; Danish, M.; Thompson, H.M.; Abdu Aliyu, A.A.; Hastuty, S.; Tuj Zohura1, F.; Bryant, M.G.; Rubaiee, S.; Rao, T.V.V.L.N. Assessment of PM-EDM cycle factors influence on machining responses and surface properties of biomaterials: A comprehensive review. Precis. Eng. 2020 , 66 , 531–549. [ Google Scholar ] [ CrossRef ]
- Prakash, C.; Singh, S.; Pabla, B.S.; Uddin, M.S. Synthesis, characterization, corrosion and bioactivity investigation of nano-HA coating deposited on biodegradable Mg-Zn-Mn alloy. Surf. Coat. Technol. 2018 , 346 , 9–18. [ Google Scholar ] [ CrossRef ]
- Santosh, S.; Ibrahim, S.J.S.; Saravanamuthukumar, P.; Rajkumar, K.; Harikrishna, K.L. Nano Graphite Powder Assisted Electric Discharge Machining Characteristics of ZM21 Magnesium Alloy. Appl. Mech. Mater. 2015 , 787 , 406–410. [ Google Scholar ] [ CrossRef ]
- Lamichhane, Y.; Singh, G.; Bhui, A.S.; Mukhiya, P.; Kumar, P.; Thapa, B. Surface modification of 316L SS with HAp nano-particles using PMEDM for enhanced Biocompatibility. Mater. Today Proc. 2019 , 15 , 336–343. [ Google Scholar ] [ CrossRef ]
- Singh, G.; Lamichhane, Y.; Bhui, A.S.; Sidhu, S.S.; Bains, P.S.; Mukhiya, P. Surface morphology and microhardness behavior of 316L in hap-pmedm. Facta Univ. Ser. Mech. Eng. 2019 , 17 , 445–454. [ Google Scholar ] [ CrossRef ]
- Singh, G.; Singh Sidhu, S.; Singh Bains, P.; Singh Bhui, A. Improving microhardness and wear resistance of 316L by TiO 2 powder mixed electro-discharge treatment. Mater. Res. Express 2019 , 6 , 086501. [ Google Scholar ] [ CrossRef ]
- Bains, P.S.; Bahraminasab, M.; Sidhu, S.S.; Singh, G. On the machinability and properties of Ti–6Al–4V biomaterial with n-HAp powder–mixed ED machining. Proc. Inst. Mech. Eng. Part H J. Eng. Med. 2020 , 234 , 232–242. [ Google Scholar ] [ CrossRef ]
- Gosai, N.D.; Joshi, A.Y. Experimental Investigation and Optimization of Process Parameters Used in the Silicon Powder Mixed Electro Discharge Machining of Ti-6Al-4V Alloy Using Response Surface Methodology. J. Manuf. Sci. Prod. 2016 , 16 , 21–32. [ Google Scholar ] [ CrossRef ]
- Bhui, A.S.; Bains, P.S.; Sidhu, S.S.; Singh, G. Parametric optimization of ED machining of Ti-6Al-4V in CNTs mixed dielectric medium. Mater. Today Proc. 2019 , 18 , 1532–1539. [ Google Scholar ] [ CrossRef ]
- Devgan, S.; Sidhu, S.S. Surface modification of β-type titanium with multi-walled CNTs/ μ-HAp powder mixed Electro Discharge Treatment process. Mater. Chem. Phys. 2020 , 239 , 122005. [ Google Scholar ] [ CrossRef ]
- Abdul-Rani, A.M.; Nanimina, A.M.; Ginta, T.L.; Razak, M.A. Machined Surface Quality in Nano Aluminum Mixed Electrical Discharge Machining. Procedia Manuf. 2017 , 7 , 510–517. [ Google Scholar ] [ CrossRef ]
- Prakash, C.; Kansal, H.K.; Pabla, B.S.; Puri, S. Potential of Silicon Powder-Mixed Electro Spark Alloying for Surface Modification of β-Phase Titanium Alloy for Orthopedic Applications. Mater. Today Proc. 2017 , 4 , 10080–10083. [ Google Scholar ] [ CrossRef ]
- Farooq, M.U.; Mughal, M.P.; Ahmed, N.; Mufti, N.A.; Al-Ahmari, A.M.; He, Y. On the Investigation of Surface Integrity of Ti6Al4V ELI Using Si-Mixed Electric Discharge Machining. Materials 2020 , 13 , 1549. [ Google Scholar ] [ CrossRef ] [ PubMed ]
- Prakash, C.; Uddin, M.S. Surface modification of β-phase Ti implant by hydroaxyapatite mixed electric discharge machining to enhance the corrosion resistance and in-vitro bioactivity. Surf. Coat. Technol. 2017 , 326 , 134–145. [ Google Scholar ] [ CrossRef ]
- Ashok kumar, U.; Laxminarayana, P. Optimization of Electrode Tool Wear in micro holes machining by Die Sinker EDM using Taguchi Approach*. Mater. Today Proc. 2018 , 5 , 1824–1831. [ Google Scholar ] [ CrossRef ]
- Bose, G.K.; Mahapatra, K.K. Parametric study of die sinking EDM process on AISI H13 tool steel using statistical techniques. Adv. Prod. Eng. Manag. 2014 , 9 , 168–180. [ Google Scholar ] [ CrossRef ]
- Sharma, A.; Kumar, V.; Babbar, A.; Dhawan, V.; Kotecha, K.; Prakash, C. Experimental Investigation and Optimization of Electric Discharge Machining Process Parameters Using Grey-Fuzzy-Based Hybrid Techniques. Materials 2021 , 14 , 5820. [ Google Scholar ] [ CrossRef ]
- Joshi, S.N.; Pande, S.S. Development of an intelligent process model for EDM. Int. J. Adv. Manuf. Technol. 2009 , 45 , 300–317. [ Google Scholar ] [ CrossRef ]
- Vidyapati, K.; Sunny, D.; Shankar, C. Teaching-learning-based parametric optimization of an electrical discharge machining process. Facta Univ. Ser. Mech. Eng. 2020 , 18 , 281–300. [ Google Scholar ] [ CrossRef ]
- Karmiris-Obratański, P.; Papazoglou, E.L.; Leszczyńska-Madej, B.; Karkalos, N.E.; Markopoulos, A.P. An Optimalization Study on the Surface Texture and Machining Parameters of 60CrMoV18-5 Steel by EDM. Materials 2022 , 15 , 3559. [ Google Scholar ] [ CrossRef ]
- Sidhu, S.S.; Bains, P.S. Study of the recast layer of particulate reinforced metal matrix composites machined by EDM. Mater. Today: Proc. 2017 , 4 , 3243–3251. [ Google Scholar ] [ CrossRef ]
- Hsu, C.Y.; Lin, Y.Y.; Lee, W.S.; Lo, S.P. Machining characteristics of Inconel 718 using ultrasonic and high temperature-aided cutting. J. Mater. Process. Technol. 2008 , 198 , 359–365. [ Google Scholar ] [ CrossRef ]
- Chen, W.-H.; Uribe, M.C.; Kwon, E.E.; Lin, K.-Y.A.; Park, Y.-K.; Ding, L.; Saw, L.H. A comprehensive review of thermoelectric generation optimization by statistical approach: Taguchi method, analysis of variance (ANOVA), and response surface methodology (RSM). Renew. Sustain. Energy Rev. 2022 , 169 , 112917. [ Google Scholar ] [ CrossRef ]
- Karna, S.K.; Sahai, R. An overview on Taguchi method. Int. J. Eng. Math. Sci. 2012 , 1 , 11–18. [ Google Scholar ]
- Taguchi, G. Quality Engineering in Japan. Bull. Jpn. Soc. Precis. Eng. 1985 , 19 , 237–242. [ Google Scholar ] [ CrossRef ]
- Jung, J.H.; Kwon, W.T. Optimization of EDM process for multiple performance characteristics using Taguchi method and Grey relational analysis. J. Mech. Sci. Technol. 2010 , 24 , 1083–1090. [ Google Scholar ] [ CrossRef ]
- Lovric, M. International Encyclopedia of Statistical Science ; Springer: Berlin/Heidelberg, Germany, 2010. [ Google Scholar ] [ CrossRef ]
- The New Palgrave Dictionary of Economics ; Palgrave Macmillan: London, UK, 2018. [ CrossRef ]
- Rajesh, A.; Venkatesh, J. Taguchi method and Pareto ANOVA: An approach for process parameters optimization in micro-EDM drilling. Int. J. Sci. Eng. Res. 2014 , 5 , 38–42. [ Google Scholar ]
- Snoyes, R.; Van Dijck, F. Investigations of EDM operations by means of thermo mathematical models. Ann. CIRP 1971 , 20 , 35. [ Google Scholar ]
- Van Dijck, F.S.; Dutre, W.L. Heat conduction model for the calculation of the volume of molten metal in electric discharges. J. Phys. D Appl. Phys. 1974 , 7 , 899. [ Google Scholar ] [ CrossRef ]
- Zarepour, H.; Tehrani, A.F.; Karimi, D.; Amini, S. Statistical analysis on electrode wear in EDM of tool steel DIN 1.2714 used in forging dies. J. Mater. Process. Technol. 2007 , 187–188 , 711–714. [ Google Scholar ] [ CrossRef ]
- Ishfaq, K.; Mahmood, M.A.; Khan, A.R.; Rehman, M. Mathematical modelling with experimental correlation for multiple craters dimension, material removal rate and surface roughness in electrical discharge machining. Int. J. Adv. Manuf. Technol. 2022 , 120 , 227–236. [ Google Scholar ] [ CrossRef ]
- Singh, A.; Ghosh, A. A thermo-electric model of material removal during electric discharge machining. Int. J. Mach. Tools Manuf. 1999 , 39 , 669–682. [ Google Scholar ] [ CrossRef ]
- Dehghani, D.; Yahya, A.; Khamis, N.H.; Alzaidi, A.I. Dynamic Mathematical Model for Low Power Electrical Discharge Machining Applications. J. Low Power Electron. 2019 , 15 , 11–18. [ Google Scholar ] [ CrossRef ]
- Chen, J.; Sun, Z.-M.; Lu, G.-D. Simulation for Electrode Wear Predication in Die-Sinking EDM Based on Geometry Model. In Proceedings of the Fifth International Conference on Measuring Technology and Mechatronics Automation, Hong Kong, China, 16–17 December 2013. [ Google Scholar ] [ CrossRef ]
- Papazoglou, E.L.; Markopoulos, A.P.; Papaefthymiou, S.; Manolakos, D.E. Electrical discharge machining modeling by coupling thermal analysis with deformed geometry feature. Int. J. Adv. Manuf. Technol. 2019 , 103 , 4481–4493. [ Google Scholar ] [ CrossRef ]
- Hinduja, S.; Kunieda, M. Modelling of ECM and EDM processes. CIRP Ann. 2013 , 62 , 775–797. [ Google Scholar ] [ CrossRef ]
- Tlili, A.; Ghanem, F.; Salah, N.B. A contribution in EDM simulation field. Int. J. Adv. Manuf. Technol. 2015 , 79 , 921–935. [ Google Scholar ] [ CrossRef ]
- Rajhi, W.; Kolsi, L.; Abbassi, R.; Jerbi, H.; Kchaou, M. Estimation of MRR and thermal stresses in EDM process: A comparative numerical study. Int. J. Adv. Manuf. Technol. 2022 , 121 , 7037–7055. [ Google Scholar ] [ CrossRef ]
- Kaisare, N.S. Computational Techniques for Process Simulation and Analysis Using MATLAB ; CRC Press: Boca Raton, FL, USA, 2018. [ Google Scholar ] [ CrossRef ]
- Orlande, H.R.B.; Colaço, M.J.; Cotta, R.M. Finite Difference Methods in Heat Transfer ; CRC Press: Boca Raton, FL, USA, 2017. [ Google Scholar ] [ CrossRef ]
- Pepper, D.W.; Heinrich, J.C. The Finite Element Method: Basic Concepts and Applications with MATLAB, MAPLE, and COMSOL ; CRC Press: Boca Raton, FL, USA, 2017. [ Google Scholar ] [ CrossRef ]
- Yadav, V.; Jain, V.K.; Dixit, P.M. Thermal stresses due to electrical discharge machining. Int. J. Mach. Tools Manuf. 2002 , 42 , 877–888. [ Google Scholar ] [ CrossRef ]
- Mehta, H.N. Modeling of Electrical Discharge Machining Process. Int. J. Eng. Res. Technol. 2015 , 4 , 153–156. [ Google Scholar ] [ CrossRef ]
- Singh, M.; Sharma, S.; Ramkumar, J. Numerical Simulation of Melt-Pool Hydrodynamics in μ-EDM Process. Procedia CIRP 2020 , 95 , 226–231. [ Google Scholar ] [ CrossRef ]
- Pei, J.; Zhang, L.; Du, J.; Zhuang, X.; Zhou, Z.; Wu, S.; Zhu, Y. A model of tool wear in electrical discharge machining process based on electromagnetic theory. Int. J. Mach. Tools Manuf. 2017 , 117 , 31–41. [ Google Scholar ] [ CrossRef ]
- Shervani-Tabar, M.T.; Abdullah, A.; Shabgard, M.R. Numerical study on the dynamics of an electrical discharge generated bubble in EDM. Eng. Anal. Bound. Elem. 2006 , 30 , 503–514. [ Google Scholar ] [ CrossRef ]
- Mullya, S.A.; Karthikeyan, G.; Ganachari, V.S. Electric discharge milling: A state-of-the-art review. J. Braz. Soc. Mech. Sci. Eng. 2021 , 43 , 424. [ Google Scholar ] [ CrossRef ]
- Quarto, M.; D’Urso, G.; Giardini, C.; Maccarini, G. FEM model development for the simulation of a micro-drilling EDM process. Int. J. Adv. Manuf. Technol. 2020 , 106 , 3095–3104. [ Google Scholar ] [ CrossRef ]
- Li, Z.; Liu, Y.; Cao, B.; Li, W. Modeling of material removal morphology and prediction of surface roughness based on WEDM successive discharges. Int. J. Adv. Manuf. Technol. 2022 , 120 , 2015–2029. [ Google Scholar ] [ CrossRef ]
- Ni, Q.; Zhang, Y.; Zhou, K.; Wang, G.; Ji, L.; Yang, W. Prediction and analysis of EDM performances considering random multiple-pulse discharges based on geometric optimization modeling. Int. J. Adv. Manuf. Technol. 2022 , 120 , 4123–4138. [ Google Scholar ] [ CrossRef ]
- Kunieda, M.; Kaneko, Y.; Natsu, W. Reverse simulation of sinking EDM applicable to large curvatures. Precis. Eng. 2012 , 36 , 238–243. [ Google Scholar ] [ CrossRef ]
- Papazoglou, E.L.; Karmiris-Obratański, P.; Leszczyńska-Madej, B.; Markopoulos, A.P. A study on Electrical Discharge Machining of Titanium Grade2 with experimental and theoretical analysis. Sci. Rep. 2021 , 11 , 8971. [ Google Scholar ] [ CrossRef ]
- Wu, L.; Sha, K.; Tao, Y.; Ju, B.; Chen, Y. A Hybrid Deep Learning Model as the Digital Twin of Ultra-Precision Diamond Cutting for In-Process Prediction of Cutting-Tool Wear. Appl. Sci. 2023 , 13 , 6675. [ Google Scholar ] [ CrossRef ]
- Liu, H.; Bai, J. The Tool Electrode Wear and Gap Fluid Field Simulation Analysis in Micro-EDM Drilling of Micro-hole Array. Procedia CIRP 2020 , 95 , 220–225. [ Google Scholar ] [ CrossRef ]
- Grigoriev, S.N.; Kozochkin, M.P.; Porvatov, A.N.; Fedorov, S.V.; Malakhinsky, A.P.; Melnik, Y.A. Investigation of the Information Possibilities of the Parameters of Vibroacoustic Signals Accompanying the Processing of Materials by Concentrated Energy Flows. Sensors 2023 , 23 , 750. [ Google Scholar ] [ CrossRef ] [ PubMed ]
- Rafaqat, M.; Mufti, N.A.; Saleem, M.Q.; Ahmed, N.; Rehman, A.U.; Ali, M.A. Machining of Triangular Holes in D2 Steel by the Use of Non-Conventional Electrodes in Die-Sinking Electric Discharge Machining. Materials 2023 , 16 , 3865. [ Google Scholar ] [ CrossRef ]
- Papazoglou, E.L.; Karmiris-Obratański, P.; Karkalos, N.E.; Thangaraj, M.; Markopoulos, A.P. Theoretical and experimental analysis of plasma radius expansion model in EDM: A comprehensive study. Int. J. Adv. Manuf. Technol. 2023 , 126 , 2429–2444. [ Google Scholar ] [ CrossRef ]
- Kuriachen, B.; Varghese, A.; Somashekhar, K.P.; Panda, S.; Mathew, J. Three-dimensional numerical simulation of microelectric discharge machining of Ti-6Al-4V. Int. J. Adv. Manuf. Technol. 2015 , 79 , 147–160. [ Google Scholar ] [ CrossRef ]
- Huang, H.; Zhang, Z.; Ming, W.; Xu, Z.; Zhang, Y. A novel numerical predicting method of electric discharge machining process based on specific discharge energy. Int. J. Adv. Manuf. Technol. 2017 , 88 , 409–424. [ Google Scholar ] [ CrossRef ]
- Izquierdo, B.; Sánchez, J.A.; Plaza, S.; Pombo, I.; Ortega, N. A numerical model of the EDM process considering the effect of multiple discharges. Int. J. Mach. Tools Manuf. 2009 , 49 , 220–229. [ Google Scholar ] [ CrossRef ]
- Niamat, M.; Sarfraz, S.; Ahmad, W.; Shehab, E.; Salonitis, K. Parametric Modelling and Multi-Objective Optimization of Electro Discharge Machining Process Parameters for Sustainable Production. Energies 2020 , 13 , 38. [ Google Scholar ] [ CrossRef ]
- Gouda, D.; Panda, A.; Nanda, B.K.; Kumar, R.; Sahoo, A.K.; Routara, B.C. Recently evaluated Electrical Discharge Machining (EDM) process performances: A research perspective. Mater. Today Proc. 2021 , 44 , 2087–2092. [ Google Scholar ] [ CrossRef ]
- Shirguppikar, S.S.; Dabade, U.A. Experimental Investigation of Dry Electric Discharge Machining (Dry EDM) Process on Bright Mild Steel. Mater. Today Proc. 2018 , 5 , 7595–7603. [ Google Scholar ] [ CrossRef ]
- Liqing, L.; Yingjie, S. Study of Dry EDM with Oxygen-Mixed and Cryogenic Cooling Approaches. Procedia CIRP 2013 , 6 , 344–350. [ Google Scholar ] [ CrossRef ]
- Ganachari, V.S.; Chate, U.N.; Waghmode, L.Y.; Mullya, S.A.; Shirguppikar, S.S.; Salgar, M.M.; Kumbhar, V.T. A Comparative Performance Study of Dry and Near Dry EDM Processes in Machining of Spring Steel Material. Mater. Today Proc. 2019 , 18 , 5247–5257. [ Google Scholar ] [ CrossRef ]
- Dhakar, K.; Dvivedi, A. Experimental Investigation on Near-dry EDM using Glycerin-Air Mixture as Dielectric Medium. Mater. Today Proc. 2017 , 4 , 5344–5350. [ Google Scholar ] [ CrossRef ]
- Wiessner, M.; Macedo, F.T.B.; Martendal, C.P.; Kuster, F.; Wegener, K. Fundamental Investigation of EDM Plasmas, Part I: A Comparison between Electric Discharges in Gaseous and Liquid Dielectric Media. Procedia CIRP 2018 , 68 , 330–335. [ Google Scholar ] [ CrossRef ]
- Macedo, F.T.B.; Wiessner, M.; Bernardelli, G.C.; Kuster, F.; Wegener, K. Fundamental Investigation of EDM Plasmas, Part II: Parametric Analysis of Electric Discharges in Gaseous Dielectric Medium. Procedia CIRP 2018 , 68 , 336–341. [ Google Scholar ] [ CrossRef ]
- Bayki, S.; Mujumdar, S. A 1D model for prediction of dry electrical discharge machining (dry-EDM) plasma characteristics. J. Manuf. Process. 2023 , 102 , 417–428. [ Google Scholar ] [ CrossRef ]
- Banu, A.; Ali, M.Y.; Rahman, M.A.; Konneh, M. Investigation of process parameters for stable micro dry wire electrical discharge machining. Int. J. Adv. Manuf. Technol. 2019 , 103 , 723–741. [ Google Scholar ] [ CrossRef ]
- Banu, A.; Ali, M.Y.; Rahman, M.A.; Konneh, M. Stability of micro dry wire EDM: OFAT and DOE method. Int. J. Adv. Manuf. Technol. 2020 , 106 , 4247–4261. [ Google Scholar ] [ CrossRef ]
- Bhandare, A.S.; Dabade, U.A. Modeling of Dry EDM process parameters during machining of Inconel 718 using artificial neural network. Mater. Today Proc. 2023 . [ Google Scholar ] [ CrossRef ]
- Leão, F.N.; Pashby, I.R. A review on the use of environmentally-friendly dielectric fluids in electrical discharge machining. J. Mater. Process. Technol. 2004 , 149 , 341–346. [ Google Scholar ] [ CrossRef ]
- Kao, J.Y.; Tarng, Y.S. A neutral-network approach for the on-line monitoring of the electrical discharge machining process. J. Mater. Process. Technol. 1997 , 69 , 112–119. [ Google Scholar ] [ CrossRef ]
- Kao, C.C.; Shih, A.J. Sub-nanosecond monitoring of micro-hole electrical discharge machining pulses and modeling of discharge ringing. Int. J. Mach. Tools Manuf. 2006 , 46 , 1996–2008. [ Google Scholar ] [ CrossRef ]
- Yu, S.; Lee, B.; Lin, W. Waveform Monitoring of Electric Discharge Machining by Wavelet Transform. Int. J. Adv. Manuf. Technol. 2001 , 17 , 339–343. [ Google Scholar ] [ CrossRef ]
- Portillo, E.; Marcos, M.; Cabanes, I.; Orive, D. Real-time monitoring and diagnosing in wire-electro discharge machining. Int. J. Adv. Manuf. Technol. 2009 , 44 , 273–282. [ Google Scholar ] [ CrossRef ]
- Kozochkin, M.P.; Grigor’ev, S.N.; Okun’kova, A.A.; Porvatov, A.N. Monitoring of electric discharge machining by means of acoustic emission. Russ. Eng. Res. 2016 , 36 , 244–248. [ Google Scholar ] [ CrossRef ]
- Grigoriev, S.N.; Kozochkin, M.P.; Volosova, M.A.; Okunkova, A.A.; Fedorov, S.V. Vibroacoustic Monitoring Features of Radiation-Beam Technologies by the Case Study of Laser, Electrical Discharge, and Electron-Beam Machining. Metals 2021 , 11 , 1117. [ Google Scholar ] [ CrossRef ]
- Evertz, S.; Dott, W.; Eisentraeger, A. Electrical discharge machining: Occupational hygienic characterization using emission-based monitoring. Int. J. Hyg. Environ. Health 2006 , 209 , 423–434. [ Google Scholar ] [ CrossRef ]
- Mu, X.; Zhou, M. An innovation approach of multiple discharging channels in EDM precision machining by a novel adaptive gap voltage control system. J. Phys. Conf. Ser. 2019 , 1176 , 052047. [ Google Scholar ] [ CrossRef ]
- Ismail, M.R.M.; Karmiris-Obratański, T.P.; Papazoglou, E.; Karkalos, N. Design of Real-Time Extremum-Seeking Controller-Based Modelling for Optimizing MRR in Low Power EDM. Materials 2023 , 16 , 434. [ Google Scholar ] [ CrossRef ]
- Sisodiya, M.S.; Shukla, S.; Bajpai, V. Feasibility analysis of novel Maglev EDM by comparing with conventional micro EDM. Sci. Rep. 2022 , 12 , 2613. [ Google Scholar ] [ CrossRef ]
- GF Machining Solutions. Available online: https://www.gfms.com/com/en.html (accessed on 25 September 2023).
- Electronica India Limited. Available online: https://electronicagroup.com/ (accessed on 25 September 2023).
- TECHMA EDM Technology. Available online: https://www.techma.de/ (accessed on 25 September 2023).
- Patowari, P.K.; Saha, P.; Mishra, P.K. Artificial neural network model in surface modification by EDM using tungsten–copper powder metallurgy sintered electrodes. Int. J. Adv. Manuf. Technol. 2010 , 51 , 627–638. [ Google Scholar ] [ CrossRef ]
Publication Number | Title | Inventors | Assignee | Publication Year | Ref. |
---|---|---|---|---|---|
RU2802609 | Device for electro discharge firmware opening with electrode tool | Ablyaz T., Shlykov E., Osinnikov I., Makarova L., Muratov K., Shiryaev V. | Perm National Research Polytechnic University | 2023 | (accessed on 15 November 2023) |
USOO9452483B2 | Electric discharge machining die sinking device and related method of operation | Yuefeng Luo William Edward Adis Michael Lewis Jones | General Electric Company, Schenectady, NY, USA | 2016 | (accessed on 15 November 2023) |
RU2772410 | Method for electric discharge wire cutting machining | Ablyaz T., Shlykov E., Gashev E., Muratov K., Shiryaev V., Sarabdjeet S. | Perm National Research Polytechnic University | 2022 | (accessed on 15 November 2023) |
RU2730321 | Method of electro-discharge hole sewing | Ablyaz T., Muratov K., Makarova L., Shlykov E., Shipunov G., Shakirzyanov T. | Perm National Research Polytechnic University | 2020 | (accessed on 15 November 2023) |
RU2721245 | Method for texturing a metal surface | Ablyaz T., Muratov K., Makarova L., Shlykov E., Kochergin E. | Perm National Research Polytechnic University | 2020 | (accessed on 15 November 2023) |
ES2750567T3 | Small hole EDM machining apparatus | Suzuki E., Shimoda Y. | Astec Co., Ltd. (Tokyo Japan) | 2020 | (accessed on 15 November 2023) |
US8629368B2 | High-speed ultra precision manufacturing station that combines direct metal deposition and EDM | Jyoti Mazumder Jun Ni Albert Shih | DM3D Technology, LLC, Troy, MI, USA | 2014 | (accessed on 15 November 2023) |
The statements, opinions and data contained in all publications are solely those of the individual author(s) and contributor(s) and not of MDPI and/or the editor(s). MDPI and/or the editor(s) disclaim responsibility for any injury to people or property resulting from any ideas, methods, instructions or products referred to in the content. |
Share and Cite
Kamenskikh, A.A.; Muratov, K.R.; Shlykov, E.S.; Sidhu, S.S.; Mahajan, A.; Kuznetsova, Y.S.; Ablyaz, T.R. Recent Trends and Developments in the Electrical Discharge Machining Industry: A Review. J. Manuf. Mater. Process. 2023 , 7 , 204. https://doi.org/10.3390/jmmp7060204
Kamenskikh AA, Muratov KR, Shlykov ES, Sidhu SS, Mahajan A, Kuznetsova YS, Ablyaz TR. Recent Trends and Developments in the Electrical Discharge Machining Industry: A Review. Journal of Manufacturing and Materials Processing . 2023; 7(6):204. https://doi.org/10.3390/jmmp7060204
Kamenskikh, Anna A., Karim R. Muratov, Evgeny S. Shlykov, Sarabjeet Singh Sidhu, Amit Mahajan, Yulia S. Kuznetsova, and Timur R. Ablyaz. 2023. "Recent Trends and Developments in the Electrical Discharge Machining Industry: A Review" Journal of Manufacturing and Materials Processing 7, no. 6: 204. https://doi.org/10.3390/jmmp7060204
Article Metrics
Article access statistics, further information, mdpi initiatives, follow mdpi.
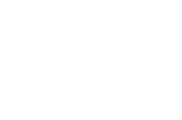
Subscribe to receive issue release notifications and newsletters from MDPI journals
- Open access
- Published: 23 July 2022
Performance evaluation of electric discharge machining of titanium alloy-a review
- Rajusing Rathod 1 ,
- Dinesh Kamble 1 &
- Nitin Ambhore ORCID: orcid.org/0000-0001-8468-8057 1
Journal of Engineering and Applied Science volume 69 , Article number: 64 ( 2022 ) Cite this article
4918 Accesses
10 Citations
Metrics details
Titanium alloy has a high specific resistance, excellent machining performance is non-corrosive, and the capability to withstand greater temperatures while maintaining outstanding mechanical properties. This alloy is, therefore, the right choice for aerospace, maritime, biomedical, and industrial applications. But machinability of titanium alloy is challenging as a result of its poor thermal conductivity, highly chemically reactive, and low elastic modulus hence it is treated as a difficult-to-cut material. Fast tool wear is observed during the machining of titanium alloy in conventional machining methods. Therefore, unconventional processing methods are used for the treatment of titanium alloy. Electric discharge machining (EDM) is one of these unconventional machining processes which are used for cutting with high precision, having a high degree of machinability, and getting a better surface finish. It is considered the best choice for machining titanium alloy. In the EDM process, different techniques are used to understand the effects of process parameters such as polarity, peak current, electrode type, pulse on time, and gap voltage on material removal rate, tool wear rate, surface roughness, and wear ratio. This paper critically investigates different types of EDM processes, experimental setups used for machining of titanium alloy, the effect of different tool electrodes and dielectric media on machining parameters, machined surface characteristics, and metal removal rate and tool wear rate.
Introduction
New challenges in the manufacturing sector, have lead manufacturers to development of reliable and high-quality products as per requirements. Nowadays, to enhance productivity and maintain the surface quality of complex parts, researchers have focused on high-speed machining technologies that involve electron beam machining (EBM), computer numerical control (CNC) machining, chemical machining, laser beam machining (LBM), and electric discharge machining (EDM) [ 1 ]. Chemical machining creates a hazardous environment while EBM and LBM need significant investments relative to EDM [ 2 ]. Therefore, EDM is the highly preferred machining process to cut very hard materials and worked on the electro-thermal principle [ 3 ]. This process is highly popular in manufacturing industries because of its ability to produce desired and complex shapes of rigid materials and alloys and good quality surface finish [ 4 ]. Another benefit of the EDM process is the absence of residual stresses due to the method’s non-contact character, as there is no physical contact between the working electrode and the workpiece, and thus no cutting forces are generated [ 5 ]. In the EDM process, workpiece and electrode are submerged in dielectric media, the desired voltage is generated in the small gap between the workpiece and tool, it formed plasma channels and generates high-frequency electric discharges between the workpiece and electrode [ 6 ]. Electrical discharges generate very high temperatures in the range 6000–20000 o C, it melts or vaporizes materials of a tool as well as a workpiece and removed materials from a workpiece [ 7 ]. At the end of the process, plasma temperature drops quickly and the workpiece cooled, waste residues in the form of spark erosion are flushed away as debris by dielectric media [ 8 ]. EDM is widely used for manufacturing cutting tools, molds, dies [ 9 ], bio-implants [ 10 ], and sectors such as automobile and aerospace [ 11 , 12 ]. The principle of spark erosion during the process is shown in Fig. 1 .
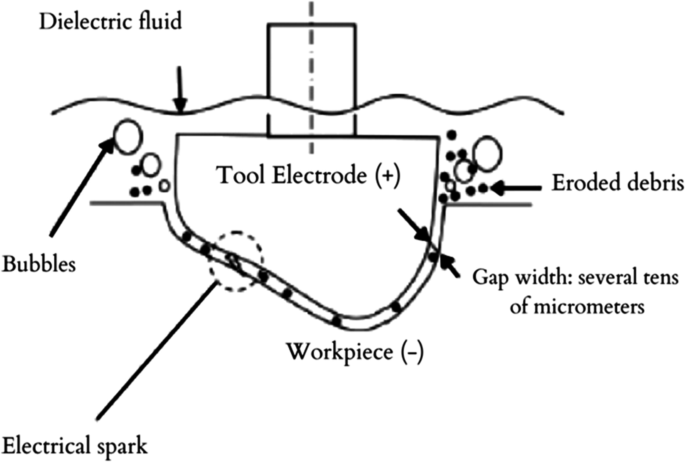
Spark erosion process [ 9 ]
The performance of the EDM depends upon several parameters and these mainly include pulse-on time and current, voltage, polarity, duty factor, the flushing pressure, and the gap in electrode and workpiece [ 13 ]. Over the last few years, several researchers have evaluated the performance of the EDM process for various parameters such as material removal rate (MRR) and tool wear ratio (TWR), and this resulted in machined surface quality. The workpiece and electrode material are the most important factors in determining the performance of EDM parameters. As a result, alternative EDM parameters and electrode materials must be investigated for the microstructure and surface roughness of the machined surface. This paper focuses on different types of EDM processes and various parameters influencing the surface characteristics of titanium aluminium during the EDM process.
- Electric discharge machining
This section provides a brief overview of titanium alloy and tool materials, as well as EDM processes and methods used for titanium alloy machining.
Titanium alloy and cutting tool material
The titanium-based alloys are employed in a wide range of engineering applications such as automobile, aerospace, marine, nuclear, chemical vessels, sports, and medical applications. However, due to their intrinsic mechanical and thermo-physical properties, titanium alloys are considered difficult-to-cut materials with poor machinability. The primary limitations of machining with standard techniques are low thermal conductivity, strong chemical reactivity, and low modulus of elasticity. Thus, non-conventional machining techniques, like EDM, are utilized as feasible alternatives. EDM is a machining process that uses electrical discharge to remove material and produce a desired surface and shape on a specific material or alloy. Many researchers agreed that titanium and its alloys are difficult to machine, are extremely hard, and have a high melting point, making them good material for testing the electrical discharge machining process capability.
Modern industry demands to find the best tool materials or alternate tool materials for machining titanium alloys. To explore the EDM performance of monel 400 alloys, Kumar et al. [ 14 ] created copper-titanium diboride electrode material. The finding of their study revealed that 16% titanium diboride gives optimized machining results for material removal rate and tool wear.
Papazoglou et al. [ 15 ] conducted several experiments for titanium grade 2 with EDM using graphite electrodes. It is revealed that MRR is mainly affected by the pulse-on current and with an increase of pulse-on current which results in a higher MRR. Fonda et al. [ 16 ] presented a machining evaluation of Ti–6A–4V with EDM. They concluded that machining performance depends on the temperature of the workpiece.
Sivaprakasam et al. [ 17 ] studied micro-wire electro discharge machining (Micro-WEDM) on titanium alloy (Ti–6Al–4V) with the zinc-coated copper electrode material. The author discovered that as discharge voltage rises surface roughness (Ra) values rise sharply and then fall, and Ra values rise as capacitance rises. They also discovered a fine surface polish at 120 V, 0.1 nF capacitance, and a fixed feed rate of 10 μm/s.
Prakash et al. [ 18 ] carried out the electrical discharge machining experiments on titanium grade 2 with brass as the electrode and examined the findings. The result showed that peak current plays a vital impact in the machining of titanium grade 2 material. Kumar et al. [ 19 ] conducted a comprehensive overview of different methods used to improve the performance of materials using the EDM process. In their research, it was concluded mixing the powder into the dielectric gives a better surface finish.
Karmiris-Obrataski et al. [ 20 ] used a graphite electrode and a high-power EDM during the processing of material Ti–6Al–4V ELI. ANOVA model revealed that both the pulse-on time and current have a strong influence on the MRR with contributions of 61.65% and 21.94%, and provided some combinations of machining parameters that result in a lower TWR and thus lower machining costs, making the machining economically feasible.
Phan et al. [ 21 ] examined the surface roughness of machined titanium alloy (Ti–6Al–4V) surfaces using Al and coated AlCrNi electrodes in EDM. The melting point of AlCrNi coated tool electrodes produce a better surface finish with fewer micro-cracks, surface roughness, and voids than uncoated tool electrodes. Jing et al. [ 22 ] developed a MIMO adaptive control system for steady and quick electrical discharge machining on titanium alloy. The designed system regulates temperature in the gap between electrode and work-piece rising so fast that the deionization of dielectric liquid and thus produced quality alloy surface.
Abdulkareem et al. [ 23 ] investigated the effect of cooling on the copper electrode and process parameters on titanium alloy (Ti–6Al–4V). Liquid nitrogen was introduced as a coolant into the electrode at a temperature of 195 °C and electrode wear was the machinability aspect that was investigated. It is found 27% reduction in EW as compared to EDM of the same material without electrode cooling as pulse off-time and voltage increase to their highest values of 6 μ and 24 V respectively.
Shirguppikar et al. [ 24 ] studied the effect of thin-film titanium nitride (TiN) coating on tungsten carbide (WC) micro-electrode on TWR, overcut, and depth of machining in micro-drilling of Ti-6Al-4 V. The experimental findings showed that as compared to an uncoated micro-tool electrode, TWR fell by 16. 32 percent, depth of machining increased by 20. 83% and overcut decreased by 12%.
Mohanty et al. [ 25 ] investigated the effect of various micro-tools (brass and tungsten), powder infusion in EDM oil (dielectric liquid), and coating process parameters on the surface modification of Ti-alloy. Parameters like voltage, duty factor, and powder concentration were taken into account while evaluating the micro-hardness, and the surface roughness parameters. It was discovered that sparking occurs more frequently at greater duty factors, resulting in higher surface roughness values. In a comparative analysis, it was found that a detailed study is required to evaluate the tribological properties of the tiny component coated samples and the identification of various intermetallic phases generated on the recast surface is required.
Concerning the above literature, it is observed that research on the machining of titanium alloy has been widely done because it is utilized in numerous applications. With the properties of Ti-6Al-4V alloy, EDM is the best option for its machinability. During machining, the selection of suitable electrodes is very important because it directly influences machining efficiency. According to literature reviews, copper, brass, graphite, copper-tungsten, and tungsten carbide are the popular electrode materials utilized in EDM. Table 1 shows the chemical composition of Ti 6Al–4V (grade 5) and Table 2 shows the thermo-physical characteristics of work and tool materials.
Various EDM processes
With the advancement of the EDM processes, various EDM techniques were developed. The EDM techniques, various processes, and methods used for machining Ti–6Al–4V for evaluating material removal, and surface characteristics of titanium alloy, are presented in this section.
Wire-electrical discharge machining (WEDM)
Wire electrical discharge machining (WEDM) is a type of electro-thermal machining in which material is removed from a workpiece by causing electrical discharges between the wire tool electrode and the workpiece. Because of the circulation of dielectric fluid, the minute chips created by this process are washed away from the working area. The wire tool electrode unwinds on one wheel, which is guided in the correct cutting route, and wraps around another wheel throughout this operation. The minute chips produced by this process are washed away from the working area because of the circulation of dielectric fluid [ 25 ]. Figure 2 shows the basic WEDM Principle.
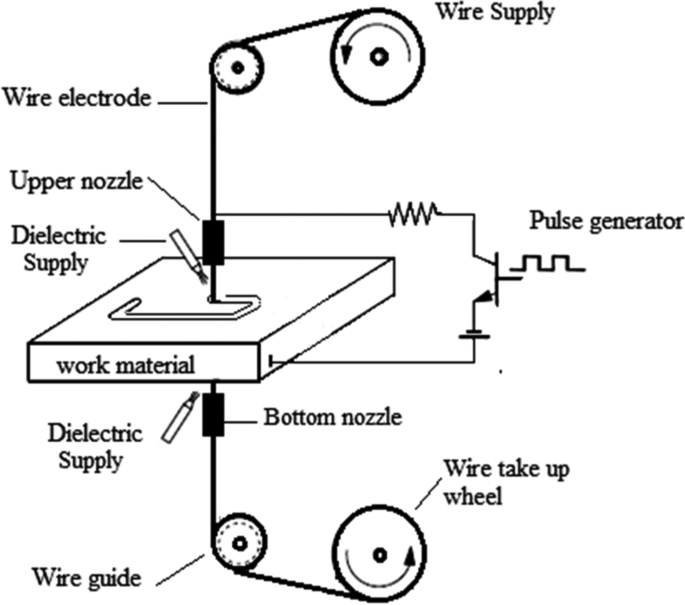
Basic WEDM Principle [ 26 ]
The complexity of the WEDM process has been investigated by several researchers. It is vital to investigate and analyze various aspects and their effects on WEDM processing to obtain the optimum potential processing settings.
Nourbakhsh et al. [ 26 ], studied wire electro-discharge machining (WEDM) on Ti6Al4V alloy with the application of different electrodes as high-speed brass wire and zinc-coated brass wire, and the dielectric medium is de-ionized water. In experiment result, in it shows that pulse width and peak current are proportional to cutting speed and inversely proportional to surface roughness. When the duration between two pulses is increased during machining, cutting speed falls, and surface roughness increases. This demonstrates that pulse width and peak current are proportional to cutting speed and inversely proportional to surface roughness. When the duration between two pulses is increased during machining, cutting speed falls and surface roughness increases with an increase in wire tension.
Pramanik et al. [ 27 ] used WEDM on Ti6Al4V alloy to investigate parameters such as material removal rate, surface generation, discharge gap, kerf width, and wire degradation. Figure 3 shows a cross-section of a wire EDM-machined Ti6Al4V alloy surface. The outside layers are flaky like an island and discontinuous, while the interior layer is solid with many cracks and holes. The population of flaks fluctuates depending on the machining condition. A shorter pulse on times results in lower surface roughness (4 and 6 ms). There is no substantial variation and manner of surface roughness when the wire tension and flushing pressure are changed. When the pulse rate increases, the kerf widths at the top and bottom of the gap widen. It seems higher at the top than at the bottom when the flushing pressure is lower. When wire tension is increased, the top and bottom kerf widths narrow. It demonstrates that the influence of input parameters on wire form and composition is negligible.
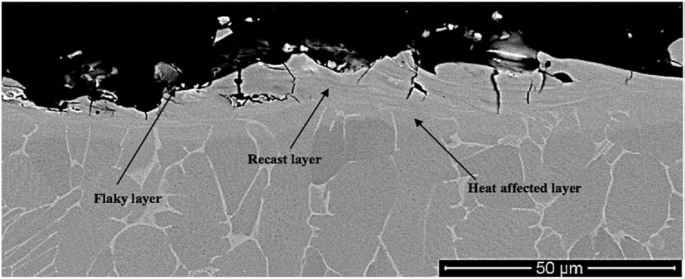
Typical cross-sectional view of Ti6Al4V surface machined by wire EDM [ 21 ]
Sivaprakasam et al. [ 17 ] experimentally studied the possibility of obtaining super surface polish at the nano level, smaller kerf width, and high material removal rate in machining titanium alloy (Ti–6Al–4V) with the Micro-WEDM process. They concluded that when the discharge voltage rises, the value of Ra rises, and then it falls. It has been observed that capacitance is the most critical parameter influencing the performance of the micro WEDM process. With a capacitance of 10 nF, the entire machining performance of titanium alloy was achieved, with minimal machining time, decreased kerf width, and higher surface quality of less than one micrometer.
It has been observed that several studies have been published in the last year, and many topics have been addressed about the WEDM process. According to the literature, saw-like material cutting with WEDM is quite common in industries and produces very precise and delicate cuts or impressions during production. The researchers' main goals are to improve the performance and broaden the application possibilities of the WEDM method. The future goals are to identify new ways to apply new versions of the WEDM process and to use these processes on materials that are discovered in the future. Further efforts are required to optimize WEDM processing to meet the requirements of the Industry 4.0 stages.
- Die-sinking EDM
In contrast to WEDM, die-sinking EDM employs an anticipated die to replace the wire in the former case. Die-sinking EDM finds a better option to machine difficult profiles in titanium alloy. Similar to WEDM, the mechanism of die-sink EDM is also controlled by the properties of the workpiece material. In this process, the workpiece is mounted on the table of the machine tool and the electrode is attached to the ram of the machine. A DC servo unit moves the ram and electrode vertically while maintaining the electrode’s proper position about the workpiece. The servo mechanism and power supply control the positioning automatically and with exceptional precision. The electrode never touches the workpiece in normal operation but is separated by a small spark gap. The ram drives the electrode toward the workpiece until there is enough space between them for the voltage in the gap to ionize the dielectric fluid and allow an electrical discharge or spark to travel from the electrode to the workpiece. Figure 4 shows the EDM die-sink process [ 29 ].
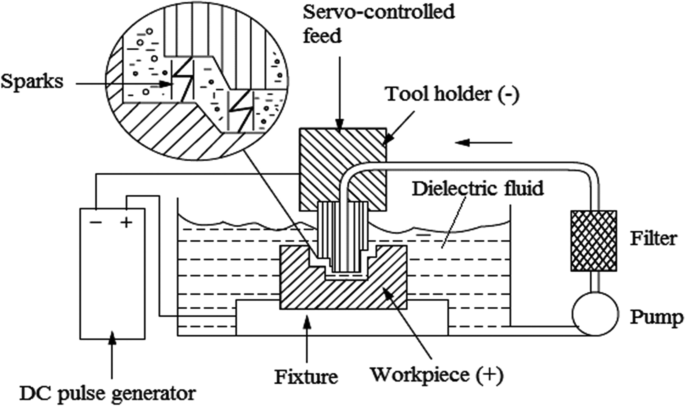
Schematic of an EDM die-sink process [ 28 ]
Rahman [ 30 ] has created an ANN model for estimating output as TWR, MRR, and SR utilizing an EDM die-sinking machine for cutting Ti–6Al–4V. They used the radial basis function neural network (RBFN) model to create the ANN model and discussed the relationship between sensitivity and input parameters. It observed peak current has the greatest impact on performance indicators. The best surface roughness is achieved with a peak current of 15 A, a servo voltage of 95 V, a pulse on time of 85 μs, and a pulse off time of 232 μs. Zainal et al. [ 31 ] examined the dimensional accuracy (DA) of Ti–6AL–4V during die-sinking electrical discharge machining (EDM) with positive polarity copper-tungsten electrode. They created a mathematical model to investigate the impact of cutting parameters on workpiece dimensional correctness. The importance of cutting parameters was determined using the Analysis of Variance (ANOVA) technique. They concluded that the most critical criteria that determine DA are pulse on time, pulse off time, peak current, and servo voltage. Hascalık and Caydas [ 32 ] tested graphite, copper, and aluminum electrode materials for die-sinking EDM machining of Ti6Al4V, and found that graphite electrode has the highest MRR, followed by copper and aluminum electrodes.
From the above literature, it was discovered that more emphasis should be placed on electrical properties in this area, with various parameters such as peak current, pulse width, and off-times requiring further investigation during machining. Other significant effects of the Ti6Al4V die-sinking process must also be investigated.
EDM ultrasonic and laser-EDM
To improve machinability, the hybrid machining method combines conventional EDM with other technologies. The basic goal is to reduce the drawbacks of a given process by incorporating another process. With the use of ultrasonic, laser, and magnetic fields, the EDM method has been successfully employed to improve machinability on titanium alloy [ 26 ]. Mugilan et al. [ 33 ] used hybrid micro-electrical discharge machining to experiment with various vibration frequencies and dielectric combinations on Ti–6Al–4V to optimize the machining process and eliminate the negative impacts of residues accumulated in drilled holes. The material removal rate improved when the pulse off time was increased in a hybrid micro-electrical discharge machining kit that was devised and implemented. Figure 5 shows schematic diagram of die-sinking electrical discharge machining with vibration kit.
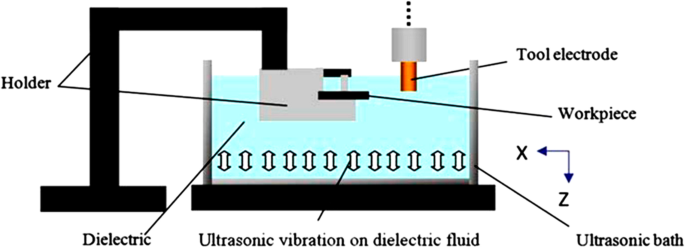
Ultrasonic vibrating set-up [ 34 ]
Researchers have conducted extensive research to increase slurry circulation, injection or suction through an electrode or workpiece holes. Despite that, these are not performing easily and generate defects on the workpiece. Several strategies have been developed to minimize unwanted impacts when modifying structures. The use of ultrasonic vibration electrical discharge machining improves these results. The use of ultrasonic vibrating movement on the electrode improves slurry circulation in this procedure. The debris was forced away and a new dielectric was pulled in by the high-frequency pumping action. It improves machining efficiency and yields a higher removal rate.
Kremer et al. [ 34 ] investigated ultrasonic vibrations and their impact on EDM performance. After comparing operations with and without ultrasonic vibrations, it was discovered that with ultrasonic vibrations in the electrode, the process improved. The high-frequency vibrating electrode accelerates slurry circulation, resulting in machining times for finishing processes that are typically 5 times shorter. The significant pressure changes in the gap are found to provide efficient discharges that remove more debris from each crater and increase the surface layer, thermal residual stresses, few micro-cracks seen, and fatigue resistance is improved by up to 6 times. It demonstrates that the process is stable when there is a small gap. As a result, combining EDM with USM can improve the manufacturing of complex shapes. Chen et al. [ 35 ] discovered that using ultrasonic vibration in EDM machining on Ti–6Al–4V alloy improves material removal rate and results in better-machined surfaces. Because of the regulated discharges at specific locations and the creation of minute debris, the alloy’s overall machinability has improved.
Zhang et al. [ 36 ] worked on SiC abrasive particles in ultrasonic-assisted EDM milling to improve the surface quality and reduce surface fractures. Wansheng et al. [ 37 ], employed an ultrasonically vibrating tool electrode in the EDM process to help drill deep and small holes in titanium alloys, which was previously thought to be a difficult approach due to low heat conductivity and strong tenacity. Singh et al. [ 38 ] performed an in-depth parametric analysis on Ti alloy μ-USEDM by using a tungsten carbide electrode. It was discovered that as the ultrasonic power is increased, the tool wear and taper angle of the drilled hole decreases progressively for a given EDM parameter.
Shabgard and Alenabi [ 39 ] studied EDM of Ti–6Al–4V alloy with copper tool vibration at ultrasonic frequency and its impact on output parameters. Experiments were performed on die-sinking EDM and planned as per the full factorial design of experiments (DOE) method. During experiments, it was observed that the rate of material removal in US-EDM was significantly higher than in conventional, induced high dielectric flow which helps to clean gap distance. Tool wear ratio is lower in US-EDM and it was less in short pulse duration than in EDM, but it happened inversely as the duration of pulses rises. This study found that ultrasonic vibration of an EDM tool in finishing modes increased MRR and decreased TWR considerably.
Because of its flexibility and accuracy, as well as its ability to cut practically all materials, laser machining is a significant tool for the production of micro features in materials. We can combine laser-cutting technology with high-speed electrical discharge machining when high accuracy and metallurgical quality are required (EDM).
Al-Ahmari et al. [ 40 ] evaluated the feasibility and uniqueness of a hybrid method by machining micro-holes with a diameter of 200 mm in Ni–Ti-based SMA using a combined laser and micro-EDM drilling process. Using laser and micro-EDM, a new hybrid machining technique was devised. Micro-holes are first laser-machined for maximal material removal, then ringed by micro EDM drilling to save time while maintaining dimensional precision and surface quality. A revolutionary hybrid machining method reduces machining time by 50–60% and increases MRR by 46–65% without compromising micro-hole quality. Prihandana et al. [ 41 ] investigated the effect of micro-powder suspension and ultrasonic vibration of dielectric fluid in micro-EDM processes. The results showed that the introduction of MoS 2 micro-powder in dielectric fluid and using ultrasonic vibration significantly increase the material removal rate and improve surface quality by providing a flat surface free of black carbon spots.
The summary of contributions of the previous researchers has been presented in Table 3 .
Performance measures of EDM
Material removal rate and tool wear rate.
MRR is one of the key parameters considered in EDM. It has a direct impact on product quality, productivity, and cost-effectiveness. The MRR is the rate at which material is removed from a machined component in a given amount of time during the machining process. MRR is determined using Eq. 1 [ 15 ].
Where W i is the initial weight of the workpiece in grams, W f is the weight of the workpiece after machining, p w represents the density of the workpiece and t is the machining time in minutes. TWR is another key parameter widely used to evaluate the performance of EDM. It is the ratio of the volume of material removed from the electrode to the volume of material removed from the workpiece and is calculated by Eq. 2 [ 15 ].
Where E i is the electrode weight before machining in grams, E f is the electrode weight after machining in grams, t is the machining time in minutes and p e is the density of electrode material. Extensive literature is available relating to the effect of process parameters on the performance of EDM of the titanium alloy. The performance of EDM is evaluated by MRR and TWR [ 6 ]. Accordingly, Ishfaq et al. [ 42 ] studied the influence of electrode materials, tool polarity, servo voltage, flushing time, pulse–time ratio, and current on the output response in terms of MRR and TWR for Ti–6Al–4V. The finding revealed that the maximum MRR (7.602 mm 3 /min) was achieved with graphene mixed dielectric (64.5%) which was greater as compared to that obtained with kerosene (4.621 mm 3 /min). But, the minimum TWR obtained for graphene-based dielectric (0.17 mg/min) is approximately 1.5 times less than that achieved with kerosene. The minimal magnitude of TWR is observed with the positive polarity using a copper electrode in a graphene-based slurry. Alam et al. [ 1 ] investigated the performance of power mixed EDM in the machining of Ti–6Al–4V in terms of MRR and TWR. The study adopted surface response methodology to investigate the influence of process parameters including peak current, powder type, and powder concentration on MRR, TWR. The result showed that TiO 2 powder has a much higher (3.42 times) effect on MRR compared to graphite powder, and for MMR, it was observed 1.73 times lower compared to that of graphite powder.
Furthermore, Singh et al. [ 47 ] conducted an extensive literature survey related to dielectric fluids and their effect on MRR. The authors concluded that metal partials in dielectric fluids reduce the insulating strength of the dielectric fluid and increase the spark gap between the tool and workpiece. As a result, the process becomes more stable, and the metal removal rate and surface finish increase. Abdudeen et al. [ 48 ] studied a different aspect of mixing various powders such as silicon, aluminum, graphite, chromium, nickel, and silicon carbide micro powder with the dielectric media. The authors concluded that the powder-mixed EDM process improves the performance in terms of MRR and surface. Suresh et al. [ 29 ] studied several types of dielectric fluids and their use in the EDM process. Dielectric medium is considered as de-ionized water or tap water, hydrocarbon oils, kerosene, mineral and transformer oil, gas, and a mixture of liquid and gas. The machined surface shows fewer defects. The use of powder-mixed EDM and composite electrodes helps to create a defect-free surface.
Devarajaiah and Muthumari [ 43 ] investigated the effect of machining parameters including pulse-on-time, pulse-off-time, current, and wire-speed on the material removal rate. The authors reported wire speed and current were the significant variables to MRR of Ti–6Al–4V however the pulse-off time was found insignificant. Gu et al. [ 44 ], studied the Ti–6Al–4V electrical discharge machining process with a bundled die-sinking electrode. A 3-factor, 3-level experimental design was adopted to study the influence of MRR and TWR on fluid flow rate, peak current, and pulse duration. The tool wear is pointedly influenced by the rate of dielectric flow and peak current in die-sinking EDM. The effect of pulse on-time, flushing pressure, and tension in wire on MRR while WEDM of titanium alloy showed in Fig. 6 .
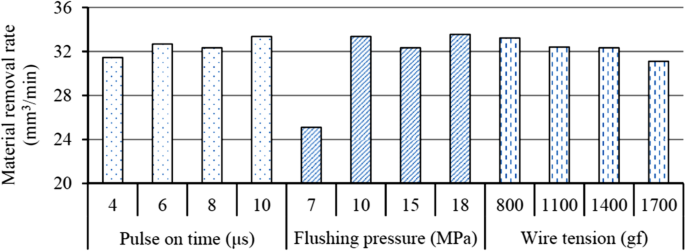
Influence of pulse on-time, flushing pressure and tension in wire on MRR while WEDM of titanium alloy [ 49 ]
Other research investigations looked into the machining characteristics of titanium alloy. For example, Kolli et al. [ 45 ] studied the machining characteristics of titanium alloy in the wire EDM process, and, in another investigation [ 50 ], the effects of machining parameters on the performance parameters in EDM of titanium alloy were studied.
- Surface integrity
Surface integrity refers to the overall appearance of the surface as well as the numerous machined surface characteristics including surface roughness, residual stress geometric, crack density micro-hardness profile, etc. These characteristics are very important components performance, durability, and reliability. Many research studies were published to investigate the use of the EDM technique in surface treatment, and found that the surface was improved [ 1 , 27 , 47 ].
Suresh et al. [ 29 ] investigated the effects of surface hardness and roughness, as well as the rate of surface wear on the electrode and the metal. Three electrodes, namely copper, graphite, and brass, were used to create the comparison results. Three elements, namely electrode material, spark gap, and depth of cut, are used to develop an experimental design at three levels (3 3 ) surface roughness, surface hardness, and dimensional differential. Input factors and responses were recorded for each run of the experimental design. Statistical software was used to examine the recorded data. The impact of each input parameter on each response, as well as their correlations, is illustrated below.
Figure 7 indicates that surface roughness is lowest when machined with fewer spark gaps and shallower depths (experiment runs 1, 4, and 7) for the above three-electrode materials. Figure 8 shows the deviation of points from the starting hardness reference line (blue dash line), indicating a non-uniform pattern of deviation, and the Fig. 9 shows that the graphite electrode is the most efficient of all electrodes, with plots that are very close to 0 when compared to brass and copper plots. The research demonstrates that graphite performs better in EDM die-sinking machining on Ti6Al4V with three electrodes, and this performance is attributable to graphite’s superior electrical characteristics.
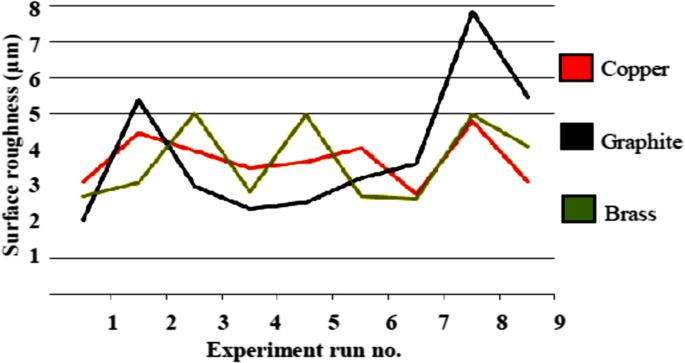
Surface roughness comparison [ 29 ]
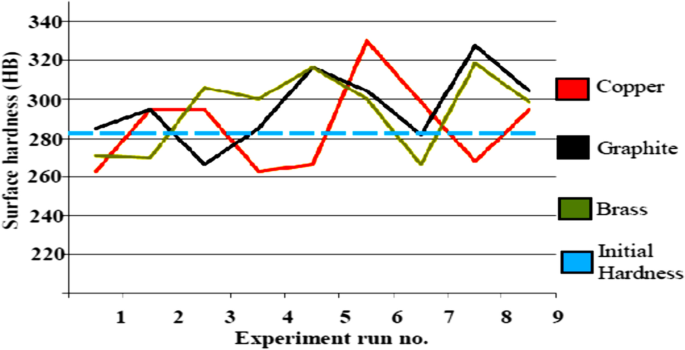
Surface hardness comparison [ 29 ]
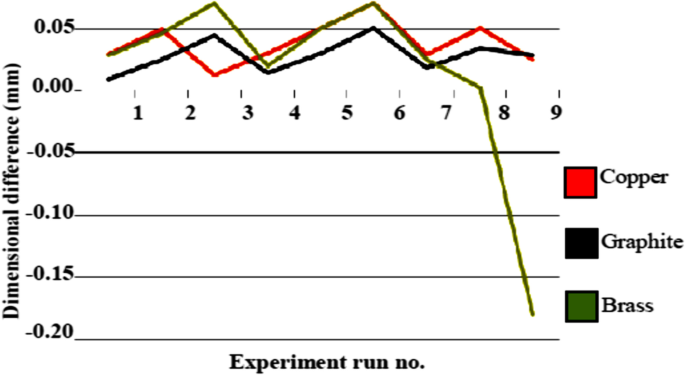
Dimensional differential plot [ 29 ]
Kumar and Davim [ 46 ] investigated the powder mixed EDM process which concluded that surface quality drastically increases at a high machining rate. Rupesh and Jatinder [ 51 ] studied the surface integrity of wire and work specimen in WEDM of titanium using brass wire. The process variables considered were pulse on time, pulse off time, applied current and wire current, wire offset, and wire feed rate. The finding raveled that lower values of pulse on-time and wire offset were found to yield the best surface quality with zinc-coated wire electrodes.
In another study, Alias et al. [ 52 ] reported that the variation in surface topography is minimal concerning wire feed rate. When MRR is lower, a comparably smoother surface is obtained. Surface quality degrades as cutting speed increases almost linear with a surface roughness of 2.44 μm at 2.65 mm/min machining speed. Surface roughness deteriorates dramatically as machining speed is increased above this point. Pulse-off-time does not affect the surface finish or dimensional inaccuracies.
Surface qualities play a key and extremely important function in new bone production and future tissue growth, according to Schwartz et al. [ 53 ]. During in vivo and in vitro studies, surface features such as surface roughness, surface topography, surface porosity, surface chemistry, and surface energy were discovered to have a substantial impact on new tissue creation and collagen or protein absorption.
Opoz et al. [ 54 ] worked on Ti–6Al–4V (grade 5) for surface improvement with hydroxyapatite powder mixed electrical discharge machining using titanium electrode and deionized water dielectric fluid. Machining condition was selected as 22 A pulse current and 12 μs pulse on duration. The experiment showed that PMEDM-made surface features have specific features and morphologies as compared with EDM-made surfaces without powder in deionized water. EDM-made surfaces show the development of smooth molten materials and relatively shallow craters, indicating a lower average roughness and when the concentration is raised, the surface roughness increases. It observed that EDM-made surfaces consist of a few micro-cracks when the machining process used only demonized water.
Sabitha [ 55 ] studied the impact of process parameters on surface roughness on Ti–6Al–4V during EDM. The effect of various input parameters such as pulse on time, current, and level of a gap on the surface roughness Ti–6Al–4V (Figs. 10 a and 11 c). Authors observed that surface roughness increased with an increase in the peak current to ascertain the level of current and afterward the surface roughness decreased even though the increase in peak current. Also found surface roughness decreases as an increase in pulse on time up to a certain level and afterward surface roughness increases as an increase in pulse on time. Regarding the level of a gap, the authors found that the surface roughness increases as an increase in the level of the gap.
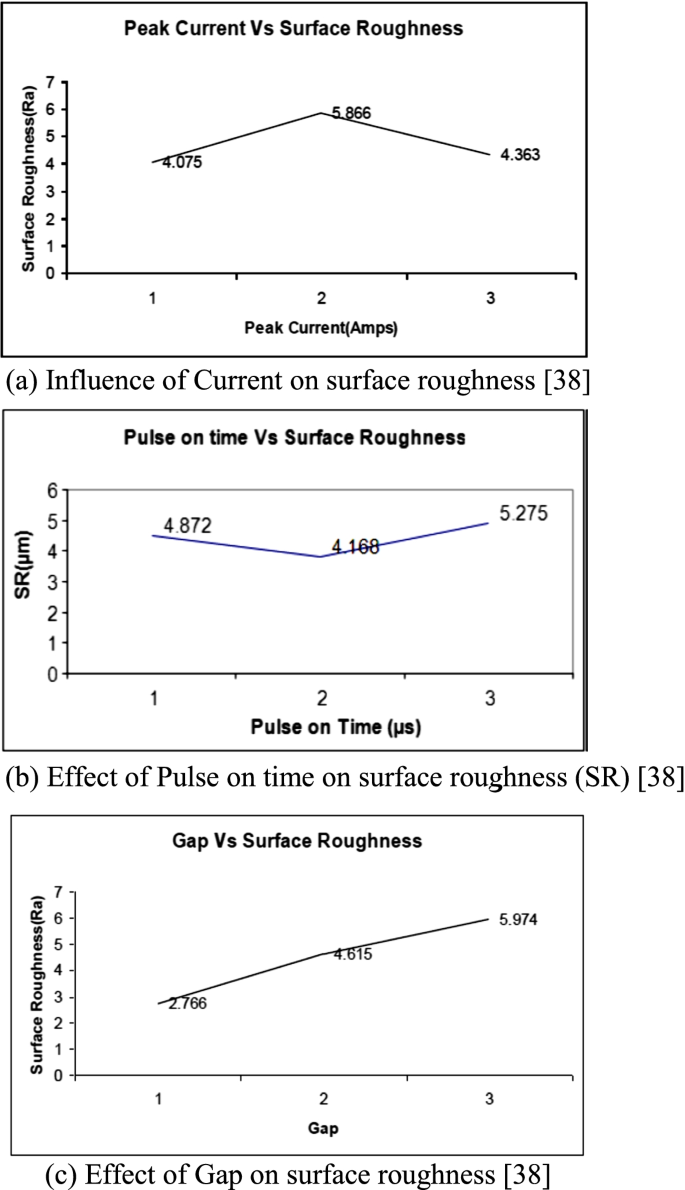
a Influence of current on surface roughness [ 37 ]. b Effect of pulse on time on surface roughness (SR) [ 37 ]. c Effect of gap on surface roughness [ 37 ]
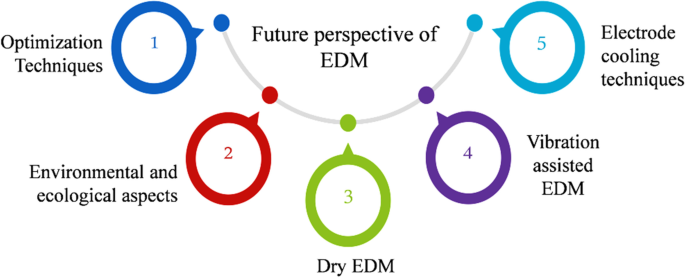
Future research directions for the EDM
Chakraborty et al. [ 56 ] presented the performance of Ti–6Al–4V by mixing the powder in different dielectrics during WEDM. In the first stage, the experiment was conducted using a brass electrode of diameter 0.25 mm. with two dielectric fluids such as deionized water and kerosene. In the second stage, experiments were performed by mixing surfactant Span 20 (chemical composition = C18H34O6) in these dielectrics. The study focused on the effects of four input parameters on MRR, SR, and dielectric consumption. The result showed that increasing the pulse on-time results in a greater MRR, whereas the pulse-off time value of 6 μs yields the best results. During the process, surfactant helps to increase the plasma channel and it leads to the best output.
Sidhu [ 57 ] worked on the surface alteration of designed nontoxic β-Ti alloys and presents the optimum process parameter to obtain a biocompatible surface. The experiment was performed on EDM with different current values and pulse on and pulse off with negative and positive polarities at a preset gap voltage of 140 V between electrodes. By using deionized water as a dielectric medium and a fine-grained graphite tool, the finished surface has been examined for the % hemolysis test. It was found that surface-modified after electro-discharge process reduces % hemolysis due to carbide/oxide surface formation. The formation of a rough porous surface at a high current (10A) improves cell anchoring with implants and stimulates the patient’s post-surgery healing process.
Pramanik et al. [ 27 ] examined the impacts of varied machining parameters such as pulse on time, flushing pressure, and wire tension on machined surface morphology by using WEDM on Ti6Al4V alloy. Swiercz and Holubek [ 58 ] investigated the effects of discharge current and pulse time on the surface roughness and the average white layer thickness. The finding revealed that machined surface layer properties play a critical role in manufacturing component performance.
Future aspects
Titanium alloy is an expensive metal that is utilized mostly in aerospace and biomedical applications due to its unique characteristics. For those applications, titanium alloy parts must be defect-free, and these parts are generally made using standard machining techniques. Manufacturing sectors have become more concerned in recent years about environmental and ecological aspects of production, in addition to productivity and component quality. Dry EDM processing also gaining popularity in the research community. Other than quality and productivity, the reduction in power consumption leads to a reduction in energy waste and lower machining expenses has and which are environmentally friendly. Furthermore, advances in hybrid methods by combining numerous methods may overcome the drawbacks of different methods and the benefits of different methods are maximized to improve machining performance. High-frequency vibration has a notable effect on the MRR therefore vibration-assisted EDM develop in the future. The different optimization techniques and electrode cooling techniques may be implemented to lower the tool wear rate. The future study is to be focused on the development of a new powder that can be used in EDM. Figure 11 presents a future research direction for the EDM.
Conclusions
This research paper reviewed the types of EDM machines, machining process, process parameters, and their effect on the performance of titanium alloy. It is an attempt to study of work of various researchers in the area of EDM and titanium alloy. EDM is highly recommended for difficult-to-cut materials. EDM is a highly recommended industry for high material rates using different types and materials of tool electrodes, dielectric fluid, and machining parameters. The review also revealed that ultrasonic vibration has a significant influence on the performance of the EDM process. The surface integrity is better in an ultrasonic-assisted process than in conventional EDM. Titanium alloy is widely used for implant-bone replacement therefore surface qualities play a key and extremely important function in new bone production and future tissue growth. For better bio-adaptability, there is scope for research on electrical discharge machining in surface modification of titanium alloy. The performance enhancement EDM on titanium alloy, a comprehensive analysis of process parameters, cutting characteristics, and surface integrity need to investigate.
Availability of data and materials
Data sharing is not applicable to this article as no datasets were generated or analyzed during the current study.
Abbreviations
Electron beam machining
Computer numerical control
Laser beam machining
Material removal rate
Tool wear ratio
Multiple-input multiple-output
Wire-electrical discharge machining
Artificial neural network
Surface roughness
Analysis of variance
Ultrasonic machining
Micro-ultrasonic-assisted electric discharge machining
Design of experiments
Alam ST, Amin AN, Hossain MI, Huq M, Hossain S (2021) Performance evaluation of graphite and titanium oxide powder mixed dielectric for electric discharge machining of Ti–6Al–4V. SN Appl. Sci. 3:435. https://doi.org/10.1007/s42452-021-04450-6
Article Google Scholar
Ndaliman MB, Khan AA, Ali MY (2011) Surface modification of titanium alloy through electrical discharge machining (EDM). Int J Mech Mater Eng 2(6):380–384. https://doi.org/10.1177/0954405412470443
Prihandana GS, Mahardika M, Sriani T (2020) Micromachining in Powder-Mixed Micro Electrical Discharge Machining. Appl. Sci. 10:3795. https://doi.org/10.3390/app10113795
Ahmed N, Anwar S, Ishfaq K et al (2019) The potentiality of sinking EDM for micro-impressions on Ti-6Al-4V: keeping the geometrical errors (axial and radial) and other machining measures (tool erosion and work roughness) at minimum. Sci Rep 9:17218. https://doi.org/10.1038/s41598-019-52855-6
Singh A, Ghadai KGR, Kanak K, Chatterjee P (2020) EDM process parameter optimization for efficient machining of inconel-718 Facta Universitatis. Series Mechanical Engineering. 18(3):473–490. https://doi.org/10.22190/FUME200406035S
Debnath B, Banik DR, Huang H, Routara BC (2019) Study of controlling parameters during electro-discharge machining of titanium alloy using grey relation analysis coupled with Taguchi philosophy, IOP Conf. Series. Mater Sci Eng 653:012049. https://doi.org/10.1088/1757-899X/653/1/012049
Furutani K, Sato H, Suzuki M (2009) Influence of electrical conditions on performance of electrical discharge machining with powder suspended in working oil for titanium carbide deposition process. Int J Adv Manuf Tech 40:1093–1101
Kuriachen B, Mathew J (2016) Effect of powder mixed dielectric on material removal and surface modification in microelectric discharge machining of Ti-6Al-4V. Mater Manuf Process 31(4):439–446
Torres A, Luis CJ, Puertas I (2015) Analysis of the influence of EDM parameters on surface finish, material removal rate, and electrode wear of an INCONEL 600 alloy. Int. J. Adv. Manuf. Technol. 80:123–140
Prakash C, Kansal HK, Pabla BS, Puri S, Aggarwal A (2016) Electric discharge machining – A potential choice for surface modification of metallic implants for orthopedic applications: a review. Proc Institution Mechanical Eng Part B J Eng Manuf. https://doi.org/10.1177/0954405415579113
Liua X, Chu PK, Ding C (2004) Surface modification of titanium, titanium alloys, and related materials for biomedical applications. Mater. Sci. Eng. R Rep. 47:49–121
Li L, Zhao L, Li ZY, Feng L, Bai X (2017) Surface characteristics of Ti-6Al-4V by SiC abrasive-mixed EDM with magnetic stirring. Mater. Manuf. Process. 32:83–86
Ou SF, Wang CY (2017) Effects of bioceramic particles in dielectric of powder-mixed electrical discharge machining on machining and surface characteristics of titanium alloys. J. Mater. Process. Technol. 245:70–79
Kumar PM, Sivakumar K, Jayakumar N (2018) Multiobjective optimization and analysis of copper–titanium diboride electrode in EDM of monel 400TM alloy. Mater. Manuf. Process. 33:1429–1437
Papazoglou EL, Karmiris-Obratański P, Leszczyńska-Madej B et al (2021) A study on electrical discharge machining of titanium grade2 with experimental and theoretical analysis. Sci Rep 11:8971. https://doi.org/10.1038/s41598-021-88534-8
Fonda P, Wang Z, Yamazaki K, Akutsu Y (2008) A fundamental study on Ti-6Al-4V’s thermal and electrical properties and their relation to EDM productivity. J. Mater. Process. Technol. 202:583–589
Sivaprakasam P, Hariharan P, Gowri S (2014) Analysis of machining parameters of μ-WEDMon titanium alloy. Int J of Appl Eng Res 9(26):8893–8896
Google Scholar
Prakash DJ, Kumar PV (2017) optimization of electrical discharge machining parameters for machining of titanium grade 2 using design of experiments approach. Int J Mech Eng Technol 8(4):413–423
Kumar SS, Varol T, Canakci A, Kumaran ST, Uthayakumar M (2021) A review on the performance of the materials by surface modification through EDM. Int J Light weight Mater Manuf 4:127–144. https://doi.org/10.1016/j.ijlmm.2020.08.002
Karmiris-Obrataski P, Papazoglou EL, Leszczynska-Madej B, Zagórskiand K, Markopoulos AP (2021) A comprehensive study on processing Ti–6Al–4V ELI with high power EDM. Materials 14:303. https://doi.org/10.3390/ma14020303
Phan N, Dong P, Muthuramalingam T, Thien N, Dung H, Hung T, Duc N, Ly N (2021) Experimental investigation of uncoated electrode and PVD AlCrNi coating on surface roughness in electrical discharge machining of Ti-6Al-4V. Int J Eng 34(4):928–934. https://doi.org/10.5829/ije.2021.34.04a.19
Jing H, Zhou M, Yang J, Yao S (2018) Stable and fast electrical discharge machining titanium alloy with mimo adaptive control system. Procedia CIRP 68:666–671
Shirguppikar SS, Patil MS (2020) Experimental investigation on micro-electro discharge machining process using tungsten carbide and titanium nitride-coated micro-tool electrode for machining of Ti-6Al-4V. Adv Mater Processing Technol. https://doi.org/10.1080/2374068X.2020.1833399
Mohanty S, Das AK, Dixit AR (2021) Influence of tool materials on surface modification using μEDC process. Surface Engineering 37:1084–1097. https://doi.org/10.1080/02670844.2021.1900518
Slătineanu L, Dodun O, Coteaţa M, Nagîţ G, Băncescu IB, Hriţuc A (2020) Wire electrical discharge machining—a review. Machines. 8:1–28. https://doi.org/10.3390/machines8040069
Nourbakhsh F, Rajurkar KP, Malshe AP, Cao J (2013) Wire Electro-Discharge Machining of Titanium Alloy. Procedia CIRP 5:13–18. https://doi.org/10.1016/j.procir.2013.01.003
Pramanik A, Basak AK, Prakash C (2019) Understanding the wire electrical discharge machining of Ti6Al4V alloy. Heliyon 5(4):1–17. https://doi.org/10.1016/j.heliyon.2019.e01473
Abdulkareem S, Khan AA, Konneh M (2010) Cooling effect on electrode and process parameters in EDM. Mater Manuf Proc 25:462–466. https://doi.org/10.1080/15394450902996619
Suresh S, Jamil MA, Sulaiman S, Shokor MRM (2016) Optimization of electrode material for EDM die-sinking of titanium alloy grade 5 - Ti6Al4V. Int J Adv Sci Eng Information Technol 6(4):534–540
Rahman MM, Modeling of machining parameters of Ti-6Al-4V for electric discharge machining: a neural network approach Scientific Research and Essays. 7(8):881-890, https://doi.org/10.5897/SRE10.1116 .
Zainal N, Zain AM, Sharif S, Hamed HNA (2017) A study of dimensional accuracy on die sinking electrical discharge machining of Ti-6AL-4V. Indian Journal of Science and Technology. 10(12):1–5. https://doi.org/10.17485/ijst/2017/v10i12/112971
Hasçalık A, Çaydas U (2007) Electrical discharge machining of titanium alloy (Ti–6Al–4V). Appl. Surf. Sci. 253(22):9007–9016
Mugilan T, Vallavi MSA, Santhosh S, Sugumar D (2020) Machining of micro holes in Ti-6Al-4V by hybrid micro electrical discharge machining to improve process parameters and flushing properties. Bulletin of the polish academy of sciences technical sciences 68(3):565–574. https://doi.org/10.24425/bpasts.2020.133366
Kremer D, Lebrun J, Hosari B, Moisan A (1989) Effects of ultrasonic vibrations on the performances in EDM. CIRP Annals 38(1):199–202
Chen S, Huang F, Suzuki Y, Yan B (1997) Improvement of material removal rate of Ti-6Al-4V alloy by electrical discharge machining with multiple ultrasonic vibrations. Keikinzoku 4(4):220–225
Zhang YP, Sun GB, Zhang AZ (2012) Study on the surface quality of titanium alloy in ultrasonic-assisted EDM milling. Applied Mechanics and Materials 184-185:1267–1271. https://doi.org/10.4028/www.scientific.net/AMM.184-185.1267
Wansheng Z, Zhenlong W, Shichun D, Guanxin C, Hongyu W (2002) Ultrasonic and electric discharge machining to deep and small hole on titanium alloy. J. Mater. Process. Technol. 120(1-3):101–106
Singh P, Yadava V, Narayan A (2018) Parametric study of ultrasonic-assisted hole sinking micro-EDM of titanium alloy. Int. J. Adv. Manuf. Technol. 94(5-8):2551–2562
Shabgarda MR, Alenabia H (2015) Ultrasonic assisted electrical discharge machining of Ti-6Al-4V alloy. Materials and Manufacturing Processes 30:991–1000. https://doi.org/10.1080/10426914.2015.1004686
Al-Ahmari A, Rasheed MS, Mohammed MK, Saleh T (2016) A hybrid machining process combining Micro-EDM and laser beam machining of Nickel–Titanium-Based shape memory alloy Mater. Manuf. Process. 31(4):447–455
Prihandana GS, Mahardika M, Hamdi M, Wong Y, Mitsui K (2009) Effect of micro-powder suspension and ultrasonic vibration of dielectric fluid in micro-EDM processes-Taguchi approach. Int. J. Mach. Tools Manuf. 49:1035–1041
Ishfaq K, Asad M, Anwar S, Pruncu CI, Saleh M, Ahmad S (2021) A comprehensive analysis of the effect of graphene-based dielectric for sustainable electric discharge machining of Ti-6Al-4V. Materials (Basel) 14(1):23
Devarajaiah D, Muthumari C (2018) Evaluation of power consumption and MRR in WEDM of Ti–6Al–4V alloy and its simultaneous optimization for sustainable production. J. Braz. Soc. Mech. Sci. Eng. 40(8):400. https://doi.org/10.1007/s40430-018-1318-y
Gu L, Li L, Zhao W, Rajurkar K (2012) Electrical discharge machining of Ti6Al4V with a bundled electrode. Int. J. Mach. Tool Manufact. 53(1):100–106
Kolli M (1957–1965) Kumar A (2014) Effect of boron carbide powder mixed into dielectric fluid on electrical discharge machining of titanium alloy. Procedia Mater Sci
Kumar H, Davim JP (2011) Role of powder in the machining of Al-10%Sicp metal matrix composites by powder mixed electric discharge machining. J Compos Mater 45(2):133–151. https :// doi.org/10.1177/00219 98310 37154 3
Singh S, Bhardwaj A (2011) Review to EDM by using water and powder-mixed dielectric fluid. Journal of Minerals & Materials Characterization & Engineering 10:199–230
Abdudeen A, Abu Qudeiri JE, Kareem A, Ahammed T, Ziout A (2020) Recent Advances and Perceptive Insights into Powder-Mixed Dielectric Fluid of EDM. Micromachines . 11(8):754. https://doi.org/10.3390/mi11080754
Pramanik A, Basak A (2018) Sustainability in wire electrical discharge machining of titanium alloy: understanding wire rupture. J Clean Prod 198:472–479
Qudeiri JEA, Mourad AHI, Ziout A, Abidi MH, Elkaseer A (2018) Electric discharge machining of titanium and its alloys. Int. J. Adv. Manuf Technol:1319–1339
Rupesh C, Jatinder K (2016) Investigation of the machining parameters and integrity of the work and wire surfaces after finish cut WEDM of commercially pure titanium. J Braz Soc Mech Sci Eng 38:883–911
Alias A, Abdullah B, Abbas NM (2012) Influence of machine feed rate in WEDM of titanium Ti-6Al-4 V with constant current (6A) using brass wire. Procedia Eng 41:1806–1811
Schwartz Z, Raz P, Zhao G, Barak Y, Tauber M, Yao H, Boyan BD (2008) Effect of micrometer-scale roughness of the surface of Ti6Al4V pedicle screws in vitro and in vivo. J Bone Joint Surg Am Vol 90(11):2485
Opoz T, Yasar H, Murphy MF, Ekmekci N, Ekmekci B Ti6Al4V Surface Modification by Hydroxyapatite Powder Mixed Electrical Discharge Machining for Medical Applications. Int J Adv Eng Pure Sci 1:1–10. https://doi.org/10.7240/jeps.450383
Sabitha KC (2021) Influence of process parameters on surface roughness of titanium alloy Ti-6Al-4V through electro discharge machining. Int Res J Eng Technol 8:14–18
Chakraborty S, Mitra S, Bose D (2020) Performance analysis on eco-friendly machining of Ti6Al4V using powder mixed with different dielectrics in WEDM. Int J Automotive Mech Eng 17:8128–8139. https://doi.org/10.15282/ijame.17.3.2020.06.0610
Sidhu AS (2020) Surface texturing of non-toxic, biocompatible titanium alloys via electro-discharge. Rep Mech Eng 2:51–56. https://doi.org/10.31181/rme200102051s
Świercz R (2020) Holubek R (2020) Experimental investigation of influence electrical discharge energy on the surface layer properties after EDM. Welding Technol Rev 92(5)
Download references
Acknowledgements
Not applicable.
No funding was received for conducting this study.
Author information
Authors and affiliations.
Department of Mechanical Engineering, Vishwakarma Institute of Information Technology, SPPU, Pune, India
Rajusing Rathod, Dinesh Kamble & Nitin Ambhore
You can also search for this author in PubMed Google Scholar
Contributions
The first draft of the manuscript was written by RR. DK and NA performed the conceptualization of the research idea, participated in the interpretation of the results, and reviewed the edited manuscript. All authors have made a substantial contribution to the manuscript. All authors read and approved the final manuscript.
Corresponding author
Correspondence to Nitin Ambhore .
Ethics declarations
Competing interests.
The authors declare that they have no competing interests.
Additional information
Publisher’s note.
Springer Nature remains neutral with regard to jurisdictional claims in published maps and institutional affiliations.
Rights and permissions
Open Access This article is licensed under a Creative Commons Attribution 4.0 International License, which permits use, sharing, adaptation, distribution and reproduction in any medium or format, as long as you give appropriate credit to the original author(s) and the source, provide a link to the Creative Commons licence, and indicate if changes were made. The images or other third party material in this article are included in the article's Creative Commons licence, unless indicated otherwise in a credit line to the material. If material is not included in the article's Creative Commons licence and your intended use is not permitted by statutory regulation or exceeds the permitted use, you will need to obtain permission directly from the copyright holder. To view a copy of this licence, visit http://creativecommons.org/licenses/by/4.0/ . The Creative Commons Public Domain Dedication waiver ( http://creativecommons.org/publicdomain/zero/1.0/ ) applies to the data made available in this article, unless otherwise stated in a credit line to the data.
Reprints and permissions
About this article
Cite this article.
Rathod, R., Kamble, D. & Ambhore, N. Performance evaluation of electric discharge machining of titanium alloy-a review. J. Eng. Appl. Sci. 69 , 64 (2022). https://doi.org/10.1186/s44147-022-00118-z
Download citation
Received : 20 February 2022
Accepted : 06 July 2022
Published : 23 July 2022
DOI : https://doi.org/10.1186/s44147-022-00118-z
Share this article
Anyone you share the following link with will be able to read this content:
Sorry, a shareable link is not currently available for this article.
Provided by the Springer Nature SharedIt content-sharing initiative
- Titanium-alloy
- Hybrid processes
- Dielectric fluid
IEEE Account
- Change Username/Password
- Update Address
Purchase Details
- Payment Options
- Order History
- View Purchased Documents
Profile Information
- Communications Preferences
- Profession and Education
- Technical Interests
- US & Canada: +1 800 678 4333
- Worldwide: +1 732 981 0060
- Contact & Support
- About IEEE Xplore
- Accessibility
- Terms of Use
- Nondiscrimination Policy
- Privacy & Opting Out of Cookies
A not-for-profit organization, IEEE is the world's largest technical professional organization dedicated to advancing technology for the benefit of humanity. © Copyright 2024 IEEE - All rights reserved. Use of this web site signifies your agreement to the terms and conditions.
A Review of Current Researches on Powder Mixed Electrical Discharge Machining (PMEDM) Technology
- Conference paper
- First Online: 26 November 2020
- Cite this conference paper
- Pradipta Kumar Rout 11 &
- Pankaj Charan Jena 12
Part of the book series: Lecture Notes in Mechanical Engineering ((LNME))
870 Accesses
9 Citations
Nowadays, electrical discharge machining (EDM) is becoming a more popular option for manufacturing hard material and complex geometry parts that are extremely difficult to cut by conventional machining processes. Continuous demand for the high-tech industry to use high and superalloys compelled the manufacturing industry to adhere EDM process. In present times, many researchers have been working in this area to find process suitability and optimum machining parameters. Powder mixed electric discharge machining (PMEDM) is a recent outcome in which different powders are mixed with the dielectric fluids to increase the material removal rate (MRR), surface roughness (SR), and property improvement of the machining surface. In this paper, an attempt has been made to review and discuss on PMEDM work of researchers. The paper discusses the EDM variables, optimum parameters, tool wear, surface texture, and mechanical properties of the machined surface. The outline of the discussion is stated in subsequent parts of the paper.
This is a preview of subscription content, log in via an institution to check access.
Access this chapter
Subscribe and save.
- Get 10 units per month
- Download Article/Chapter or eBook
- 1 Unit = 1 Article or 1 Chapter
- Cancel anytime
- Available as PDF
- Read on any device
- Instant download
- Own it forever
- Available as EPUB and PDF
- Compact, lightweight edition
- Dispatched in 3 to 5 business days
- Free shipping worldwide - see info
Tax calculation will be finalised at checkout
Purchases are for personal use only
Institutional subscriptions
Similar content being viewed by others
The Impact of SiC Powder Additive on Parameters of EDM: A Review
Powder-Mixed Microelectric Discharge Machining
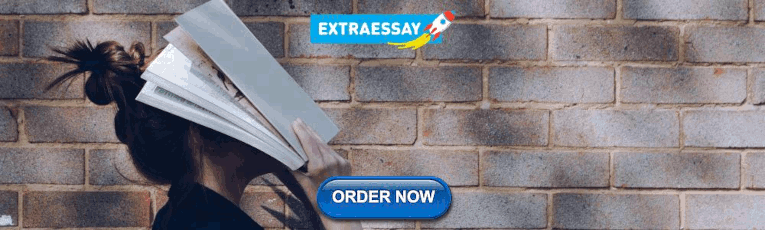
Powder Mixed Near Dry Electric Discharge Machining Parameter Optimization for Tool Wear Rate
Kansal HK, Singh S, Kumar P (2007) Technology and research developments in powder mixed electric discharge machining (PMEDM). J Mater Process Technol 184:32–41
Google Scholar
Rozenek M, Kozak J, Dabrowski L (2003) Electrical discharge machining in dielectric-powder media. Proc Inst Mech Eng Part B J Eng Manufact 217:1597–1602
Rana D, Pal AK, Tiwari P (2015) Study of powder mixed dielectric in EDM—a review. Intl J Eng Sci Adv Res 1(2):69–74
Singh S, Bhardwaj A (2011) Review to EDM by using water and powder-mixed dielectric fluid. J Min Mater Character Eng 10(2):199–230
Zhao WS, Meng QG, Wang ZL (2002) The application of research on powder mixed EDM in rough machining. J Mater Process Technol 129:30–33
Article Google Scholar
Abbas NM, Yusoff N, Mahmod@Wahab R (2012) Electrical discharge machining (EDM): practices in Malaysian industries and possible change towards green manufacturing. In: International symposium on robotics and intelligent sensors 2012 (IRIS 2012)
Ho KH, Newman ST (2003) State of the art electrical discharge machining (EDM). Advanced Manufacturing Systems and Technology Centre, Wolfson School of Mechanical and Manufacturing Engineering, Loughborough University, Loughborough, Leicestershire LE11 3TU, UK
Singh NK, Pandey PM, Singh KK, Sharma MK (2016) Steps towards green manufacturing through EDM process: a review. Cogent Eng 3:1272662
Singh H, Chatha SS, Singh H (2013) Role of dielectric and tool material on EDM performance: a review. Int J Eng Res Dev 7:67–72
Abdussabur MY, Ali M, Maleque A, Khan AA (2013) Investigation of material removal characteristics in EDM of nonconductive ZrO2 ceramic. Proc Eng 56:696–701
Tai TY, Lu SJ (2009) Improving the fatigue life of electro-discharge-machined SDK11 tool steel via the suppression of surface cracks. Int J Fatigue 31:433–438
Jamadar MM, Kavade MV (2014) Effect of aluminium powder mixed EDM on machining characteristics of die steel (AISI D3). Int J Mech Prod Eng 2:2320–2092
Gudur S, Potdar VV, Gudur S (2007) A review on effect of aluminum & silicon powder mixed EDM on response variables of various materials. Int J Innov Res Sci Eng Technol 12:17937–17945
Singh P, Kumar A, Beri N, Kumar V (2010) Some experimental investigation on aluminum powder mixed EDM on machining performance of hastelloy steel. Int J Adv Eng Technol 1:28–45
Patel S, Thesiya D, Rajurkar A (2018) Aluminium powder mixed rotary electric discharge machining (PMEDM) on Inconel 718. Aust J Mech Eng 16:21–30
Shinde R, Patil N, Raut D, Pawade R, Brahmankar P (2017) Experimental investigations into powder-mixed electrical discharge machining (PMEDM) of HCHCR D2 die steel. In: ICCASP/ICMMD-2016. Advances in intelligent systems research, vol 137, pp 298–303
Syed KH, Palaniyandi K (2012) Performance of electrical discharge machining using aluminium powder suspended distilled water. Turkish J Eng Env Sci 36:195–207
Kansal HK, Singh S, Kumar P (2007) Effect of silicon powder mixed EDM on machining rate of AISI D2 die steel. J Manufact Process 9:13–22
Razak MA, Abdul-Rani AM, Nanimina AM (2015) Improving EDM efficiency with silicon carbide powder-mixed dielectric fluid. Int J Mater Mech Manufact 3(1):40–43
Jeswani ML Effect of the addition of graphite powder to kerosene used as the dielectric fluid in electrical discharge machining, vol 70. Elsevier Sequoia SA, Lausanne, pp 133–139
Prihandana GS, Sriani T, Mahardika M (2012) Improvement of machining time in micro-EDM with workpiece vibration and graphite powder mixed in dielectric fluid. Indian J Eng Mater Sci 19:375–378
Singh B, Kumar J, Kumar S (2015) Influences of process parameters on MRR improvement in simple and powder-mixed EDM of AA6061/10%SiC composite. Mater Manufact Process 30:303–312
Sachinmohal HK (2017) Study on the multiwalled carbon nano tube mixed EDM of Al-SiC p metal marix composite. Mater Today Proc 4:3987–3993
Sagar P, Thesiya D, Rajurkar A (2018) Aluminium powder mixed rotary electric discharge machining (PMEDM) on Inconel 718. Aust J Mech Eng 16(1):21–30
Marashi H, Sarhan AAD, Hamdi M (2015) Employing Tinano-powder dielectric to enhance surface characteristics in electrical discharge machining of AISI D2 steel. Appl Surf Sci 357:892–907
Tripathy S, Tripathy DK (2017) An approach for increasing the micro-hardness in electrical discharge machining by adding conductive powder to the dielectric. Mater Today Proc 4:1215–1224
Gangadharudutalla T, Gangopadhyay S, Biswas CK (2017) Influence of graphite powder mixed EDM on the surface integrity characteristics of Inconel 625. Part Sci Technol Int J 35:219–226
Kumar S (2012) Batra U (2012) Surface modification of die steel materials by EDM method using tungsten powder-mixed dielectric. J Manufact Process 14:35–40
Abdul-Rani AM, Nanimina AM, Ginta TL, Razak MA (2017) Machined surface quality in nano aluminum mixed electrical discharge machining. Proc Manufact 7:510–517
Diwakar NV, Bhagyanathan C, Rathnaraj JD (2014) Analysis of mechanical properties of En19 steel and En41b steel used in diesel engine camshaft. Int J Curr Eng Technol, 162–167
Shashikant V, Roy AK, Kumar K (2014) Effect and optimization of machine process parameters on MRR for EN19 & EN41 materials using Taguchi. Proc Technol 14:204–210
Muraharikolli AK (2015) Effect of dielectric fluid with surfactant and graphite powder on electrical discharge machining of titanium alloy using Taguchi method. Eng Sci Technol Int J 18:524–535
Tripathy S, Tripathy DK (2017) Surface characterization and multi-response optimization of EDM process parameters using powder mixed dielectric. Mater Today Proc 4:2058–2067
Kansal HK, Singh S, Kumar P (2005) Parametric optimization of powder mixed electrical discharge machining by response surface methodology. J Mater Process Technol 169, 427–436 (2005)
Srivastava V, Pandey PM (2013) Study of ultrasonic assisted cryogenically cooled EDM process using sintered (Cu–TiC) tooltip. J Manufact Process 15:158–166
Download references
Author information
Authors and affiliations.
Kalinga Institute of Industrial Technology (KIIT), Deemed to be University, Campus-8, Patia, Bhubaneswar, Odisha, 751024, India
Pradipta Kumar Rout
Veer Surendra Sai University of Technology, Burla, Odisha, 768018, India
Pankaj Charan Jena
You can also search for this author in PubMed Google Scholar
Corresponding author
Correspondence to Pradipta Kumar Rout .
Editor information
Editors and affiliations.
Department of Metallurgical Engineering and Materials Science, Indian Institute of Technology Bombay, Mumbai, Maharashtra, India
Department of Mechanical Engineering, Indian Institute of Technology Bombay, Mumbai, Maharashtra, India
Sushil K. Mishra
School of Mechanical Engineering, KIIT Deemed to be University, Bhubaneswar, Odisha, India
Purna Chandra Mishra
Rights and permissions
Reprints and permissions
Copyright information
© 2021 Springer Nature Singapore Pte Ltd.
About this paper
Cite this paper.
Rout, P.K., Jena, P.C. (2021). A Review of Current Researches on Powder Mixed Electrical Discharge Machining (PMEDM) Technology. In: Pant, P., Mishra, S.K., Mishra, P.C. (eds) Advances in Mechanical Processing and Design. Lecture Notes in Mechanical Engineering. Springer, Singapore. https://doi.org/10.1007/978-981-15-7779-6_43
Download citation
DOI : https://doi.org/10.1007/978-981-15-7779-6_43
Published : 26 November 2020
Publisher Name : Springer, Singapore
Print ISBN : 978-981-15-7778-9
Online ISBN : 978-981-15-7779-6
eBook Packages : Engineering Engineering (R0)
Share this paper
Anyone you share the following link with will be able to read this content:
Sorry, a shareable link is not currently available for this article.
Provided by the Springer Nature SharedIt content-sharing initiative
- Publish with us
Policies and ethics
- Find a journal
- Track your research

- Conferences
- Last Updated: September 13, 2024
- In AI Mysteries
Top Machine Learning Research Papers
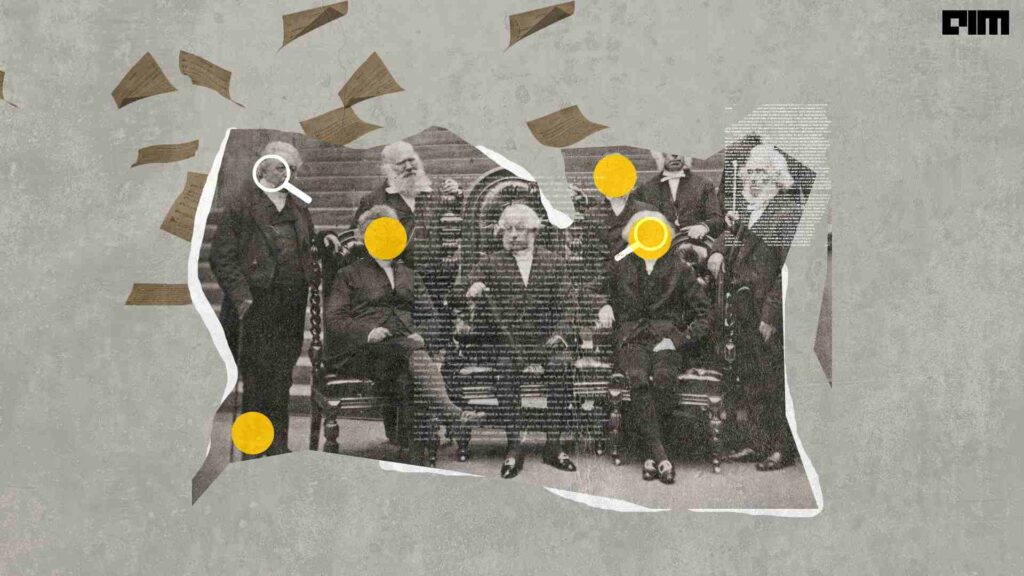
- by Dr. Nivash Jeevanandam
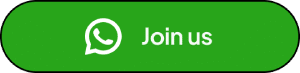
Advances in machine learning and deep learning research are reshaping our technology. Machine learning and deep learning have accomplished various astounding feats, and key research articles have resulted in technical advances used by billions of people. The research in this sector is advancing at a breakneck pace and assisting you to keep up. Here is a collection of the most important scientific study papers in machine learning.
Rebooting ACGAN: Auxiliary Classifier GANs with Stable Training
The authors of this work examined why ACGAN training becomes unstable as the number of classes in the dataset grows. The researchers revealed that the unstable training occurs due to a gradient explosion problem caused by the unboundedness of the input feature vectors and the classifier’s poor classification capabilities during the early training stage. The researchers presented the Data-to-Data Cross-Entropy loss (D2D-CE) and the Rebooted Auxiliary Classifier Generative Adversarial Network to alleviate the instability and reinforce ACGAN (ReACGAN). Additionally, extensive tests of ReACGAN demonstrate that it is resistant to hyperparameter selection and is compatible with a variety of architectures and differentiable augmentations.
This article is ranked #1 on CIFAR-10 for Conditional Image Generation.
For the research paper, read here .
For code, see here .
Dense Unsupervised Learning for Video Segmentation
The authors presented a straightforward and computationally fast unsupervised strategy for learning dense spacetime representations from unlabeled films in this study. The approach demonstrates rapid convergence of training and a high degree of data efficiency. Furthermore, the researchers obtain VOS accuracy superior to previous results despite employing a fraction of the previously necessary training data. The researchers acknowledge that the research findings may be utilised maliciously, such as for unlawful surveillance, and that they are excited to investigate how this skill might be used to better learn a broader spectrum of invariances by exploiting larger temporal windows in movies with complex (ego-)motion, which is more prone to disocclusions.
This study is ranked #1 on DAVIS 2017 for Unsupervised Video Object Segmentation (val).
Temporally-Consistent Surface Reconstruction using Metrically-Consistent Atlases
The authors offer an atlas-based technique for producing unsupervised temporally consistent surface reconstructions by requiring a point on the canonical shape representation to translate to metrically consistent 3D locations on the reconstructed surfaces. Finally, the researchers envisage a plethora of potential applications for the method. For example, by substituting an image-based loss for the Chamfer distance, one may apply the method to RGB video sequences, which the researchers feel will spur development in video-based 3D reconstruction.
This article is ranked #1 on ANIM in the category of Surface Reconstruction.
EdgeFlow: Achieving Practical Interactive Segmentation with Edge-Guided Flow
The researchers propose a revolutionary interactive architecture called EdgeFlow that uses user interaction data without resorting to post-processing or iterative optimisation. The suggested technique achieves state-of-the-art performance on common benchmarks due to its coarse-to-fine network design. Additionally, the researchers create an effective interactive segmentation tool that enables the user to improve the segmentation result through flexible options incrementally.
This paper is ranked #1 on Interactive Segmentation on PASCAL VOC
Learning Transferable Visual Models From Natural Language Supervision
The authors of this work examined whether it is possible to transfer the success of task-agnostic web-scale pre-training in natural language processing to another domain. The findings indicate that adopting this formula resulted in the emergence of similar behaviours in the field of computer vision, and the authors examine the social ramifications of this line of research. CLIP models learn to accomplish a range of tasks during pre-training to optimise their training objective. Using natural language prompting, CLIP can then use this task learning to enable zero-shot transfer to many existing datasets. When applied at a large scale, this technique can compete with task-specific supervised models, while there is still much space for improvement.
This research is ranked #1 on Zero-Shot Transfer Image Classification on SUN
CoAtNet: Marrying Convolution and Attention for All Data Sizes
The researchers in this article conduct a thorough examination of the features of convolutions and transformers, resulting in a principled approach for combining them into a new family of models dubbed CoAtNet. Extensive experiments demonstrate that CoAtNet combines the advantages of ConvNets and Transformers, achieving state-of-the-art performance across a range of data sizes and compute budgets. Take note that this article is currently concentrating on ImageNet classification for model construction. However, the researchers believe their approach is relevant to a broader range of applications, such as object detection and semantic segmentation.
This paper is ranked #1 on Image Classification on ImageNet (using extra training data).
SwinIR: Image Restoration Using Swin Transformer
The authors of this article suggest the SwinIR image restoration model, which is based on the Swin Transformer . The model comprises three modules: shallow feature extraction, deep feature extraction, and human-recognition reconstruction. For deep feature extraction, the researchers employ a stack of residual Swin Transformer blocks (RSTB), each formed of Swin Transformer layers, a convolution layer, and a residual connection.
This research article is ranked #1 on Image Super-Resolution on Manga109 – 4x upscaling.
Artificial Replay: A Meta-Algorithm for Harnessing Historical Data in Bandits
Ways to incorporate historical data are still unclear: initialising reward estimates with historical samples can suffer from bogus and imbalanced data coverage, leading to computational and storage issues—particularly in continuous action spaces. The paper addresses the obstacles by proposing ‘Artificial Replay’, an algorithm to incorporate historical data into any arbitrary base bandit algorithm.
Read the full paper here .
Bootstrapped Meta-Learning
Author(s) – Sean R. Sinclair et al.
The paper proposes an algorithm in which the meta-learner teaches itself to overcome the meta-optimisation challenge. The algorithm focuses on meta-learning with gradients, which guarantees performance improvements. Furthermore, the paper also looks at how bootstrapping opens up possibilities.
Read the full paper here .
LaMDA: Language Models for Dialog Applications
Author(s) – Sebastian Flennerhag et al.
The research describes the LaMDA system which caused chaos in AI this summer when a former Google engineer claimed that it had shown signs of sentience. LaMDA is a family of large language models for dialogue applications based on Transformer architecture. The interesting feature of the model is its fine-tuning with human-annotated data and the possibility of consulting external sources. This is a very interesting model family, which we might encounter in many applications we use daily.
Competition-Level Code Generation with AlphaCode
Author(s) – Yujia Li et al.
Systems can help programmers become more productive. The following research addresses the problems with incorporating innovations in AI into these systems. AlphaCode is a system that creates solutions for problems that require deeper reasoning.
Privacy for Free: How does Dataset Condensation Help Privacy?
Author(s) – Tian Dong et al.
The paper focuses on Privacy Preserving Machine Learning, specifically deducting the leakage of sensitive data in machine learning. It puts forth one of the first propositions of using dataset condensation techniques to preserve the data efficiency during model training and furnish membership privacy.
Why do tree-based models still outperform deep learning on tabular data?
Author(s) – Léo Grinsztajn, Edouard Oyallon and Gaël Varoquaux
The research answers why deep learning models still find it hard to compete on tabular data compared to tree-based models. It is shown that MLP-like architectures are more sensitive to uninformative features in data compared to their tree-based counterparts.
Multi-Objective Bayesian Optimisation over High-Dimensional Search Spaces
Author(s) – Samuel Daulton et al.
The paper proposes ‘MORBO’, a scalable method for multiple-objective BO as it performs better than that of high-dimensional search spaces. MORBO significantly improves the sample efficiency and, where existing BO algorithms fail, MORBO provides improved sample efficiencies over the current approach.
A Path Towards Autonomous Machine Intelligence Version 0.9.2
Author(s) – Yann LeCun
The research offers a vision about how to progress towards general AI. The study combines several concepts: a configurable predictive world model, behaviour driven through intrinsic motivation, and hierarchical joint embedding architectures trained with self-supervised
learning.
TranAD: Deep Transformer Networks for Anomaly Detection in Multivariate Time Series Data
Author(s) – Shreshth Tuli, Giuliano Casale and Nicholas R. Jennings
This is a specialised paper applying transformer architecture to the problem of unsupervised anomaly detection in multivariate time series. Many architectures which were successful in other fields are, at some point, also being applied to time series. The research shows improved performance on some known data sets.
Differentially Private Bias-Term only Fine-tuning of Foundation Models
Author(s) – Zhiqi Bu et al.
In the paper, researchers study the problem of differentially private (DP) fine-tuning of large pre-trained models—a recent privacy-preserving approach suitable for solving downstream tasks with sensitive data. Existing work has demonstrated that high accuracy is possible under strong privacy constraints yet requires significant computational overhead or modifications to the network architecture.
ALBERT: A Lite BERT
Usually, increasing model size when pretraining natural language representations often result in improved performance on downstream tasks, but the training times become longer. To address these problems, the authors in their work presented two parameter-reduction techniques to lower memory consumption and increase the training speed of BERT. The authors also used a self-supervised loss that focuses on modelling inter-sentence coherence and consistently helped downstream tasks with multi-sentence inputs. According to results, this model established new state-of-the-art results on the GLUE, RACE, and squad benchmarks while having fewer parameters compared to BERT-large.
Check the paper here .
Beyond Accuracy: Behavioral Testing of NLP Models with CheckList
Microsoft Research, along with the University of Washington and the University of California, in this paper, introduced a model-agnostic and task agnostic methodology for testing NLP models known as CheckList. This is also the winner of the best paper award at the ACL conference this year. It included a matrix of general linguistic capabilities and test types that facilitate comprehensive test ideation, as well as a software tool to generate a large and diverse number of test cases quickly.
Linformer is a Transformer architecture for tackling the self-attention bottleneck in Transformers. It reduces self-attention to an O(n) operation in both space- and time complexity. It is a new self-attention mechanism which allows the researchers to compute the contextual mapping in linear time and memory complexity with respect to the sequence length.
Read more about the paper here .
Plug and Play Language Models
Plug and Play Language Models ( PPLM ) are a combination of pre-trained language models with one or more simple attribute classifiers. This, in turn, assists in text generation without any further training. According to the authors, model samples demonstrated control over sentiment styles, and extensive automated and human-annotated evaluations showed attribute alignment and fluency.
Reformer
The researchers at Google, in this paper , introduced Reformer. This work showcased that the architecture of a Transformer can be executed efficiently on long sequences and with small memory. The authors believe that the ability to handle long sequences opens the way for the use of the Reformer on many generative tasks. In addition to generating very long coherent text, the Reformer can bring the power of Transformer models to other domains like time-series forecasting, music, image and video generation.
An Image is Worth 16X16 Words
The irony here is that one of the popular language models, Transformers have been made to do computer vision tasks. In this paper , the authors claimed that the vision transformer could go toe-to-toe with the state-of-the-art models on image recognition benchmarks, reaching accuracies as high as 88.36% on ImageNet and 94.55% on CIFAR-100. For this, the vision transformer receives input as a one-dimensional sequence of token embeddings. The image is then reshaped into a sequence of flattened 2D patches. The transformers in this work use constant widths through all of its layers.
Unsupervised Learning of Probably Symmetric Deformable 3D Objects
Winner of the CVPR best paper award, in this work, the authors proposed a method to learn 3D deformable object categories from raw single-view images, without external supervision. This method uses an autoencoder that factored each input image into depth, albedo, viewpoint and illumination. The authors showcased that reasoning about illumination can be used to exploit the underlying object symmetry even if the appearance is not symmetric due to shading.
Generative Pretraining from Pixels
In this paper, OpenAI researchers examined whether similar models can learn useful representations for images. For this, the researchers trained a sequence Transformer to auto-regressively predict pixels, without incorporating knowledge of the 2D input structure. Despite training on low-resolution ImageNet without labels, the researchers found that a GPT-2 scale model learns strong image representations as measured by linear probing, fine-tuning, and low-data classification. On CIFAR-10, it achieved 96.3% accuracy with a linear probe, outperforming a supervised Wide ResNet, and 99.0% accuracy with full fine-tuning and matching the top supervised pre-trained models. An even larger model, trained on a mixture of ImageNet and web images, is competitive with self-supervised benchmarks on ImageNet, achieving 72.0% top-1 accuracy on a linear probe of their features.
Deep Reinforcement Learning and its Neuroscientific Implications
In this paper, the authors provided a high-level introduction to deep RL , discussed some of its initial applications to neuroscience, and surveyed its wider implications for research on brain and behaviour and concluded with a list of opportunities for next-stage research. Although DeepRL seems to be promising, the authors wrote that it is still a work in progress and its implications in neuroscience should be looked at as a great opportunity. For instance, deep RL provides an agent-based framework for studying the way that reward shapes representation, and how representation, in turn, shapes learning and decision making — two issues which together span a large swath of what is most central to neuroscience.
Dopamine-based Reinforcement Learning
Why humans doing certain things are often linked to dopamine , a hormone that acts as the reward system (think: the likes on your Instagram page). So, keeping this fact in hindsight, DeepMind with the help of Harvard labs, analysed dopamine cells in mice and recorded how the mice received rewards while they learned a task. They then checked these recordings for consistency in the activity of the dopamine neurons with standard temporal difference algorithms. This paper proposed an account of dopamine-based reinforcement learning inspired by recent artificial intelligence research on distributional reinforcement learning. The authors hypothesised that the brain represents possible future rewards not as a single mean but as a probability distribution, effectively representing multiple future outcomes simultaneously and in parallel.
Lottery Tickets In Reinforcement Learning & NLP
In this paper, the authors bridged natural language processing (NLP) and reinforcement learning (RL). They examined both recurrent LSTM models and large-scale Transformer models for NLP and discrete-action space tasks for RL. The results suggested that the lottery ticket hypothesis is not restricted to supervised learning of natural images, but rather represents a broader phenomenon in deep neural networks.
What Can Learned Intrinsic Rewards Capture
In this paper, the authors explored if the reward function itself can be a good locus of learned knowledge. They proposed a scalable framework for learning useful intrinsic reward functions across multiple lifetimes of experience and showed that it is feasible to learn and capture knowledge about long-term exploration and exploitation into a reward function.
AutoML- Zero
The progress of AutoML has largely focused on the architecture of neural networks, where it has relied on sophisticated expert-designed layers as building blocks, or similarly restrictive search spaces. In this paper , the authors showed that AutoML could go further with AutoML Zero, that automatically discovers complete machine learning algorithms just using basic mathematical operations as building blocks. The researchers demonstrated this by introducing a novel framework that significantly reduced human bias through a generic search space.
Rethinking Batch Normalization for Meta-Learning
Batch normalization is an essential component of meta-learning pipelines. However, there are several challenges. So, in this paper, the authors evaluated a range of approaches to batch normalization for meta-learning scenarios and developed a novel approach — TaskNorm. Experiments demonstrated that the choice of batch normalization has a dramatic effect on both classification accuracy and training time for both gradient-based and gradient-free meta-learning approaches. The TaskNorm has been found to be consistently improving the performance.
Meta-Learning without Memorisation
Meta-learning algorithms need meta-training tasks to be mutually exclusive, such that no single model can solve all of the tasks at once. In this paper, the authors designed a meta-regularisation objective using information theory that successfully uses data from non-mutually-exclusive tasks to efficiently adapt to novel tasks.
Understanding the Effectiveness of MAML
Model Agnostic Meta-Learning (MAML) consists of optimisation loops, from which the inner loop can efficiently learn new tasks. In this paper, the authors demonstrated that feature reuse is the dominant factor and led to ANIL (Almost No Inner Loop) algorithm — a simplification of MAML where the inner loop is removed for all but the (task-specific) head of the underlying neural network.
Your Classifier is Secretly an Energy-Based Model
This paper proposed attempts to reinterpret a standard discriminative classifier as an energy-based model. In this setting, wrote the authors, the standard class probabilities can be easily computed. They demonstrated that energy-based training of the joint distribution improves calibration, robustness, handout-of-distribution detection while also enabling the proposed model to generate samples rivalling the quality of recent GAN approaches. This work improves upon the recently proposed techniques for scaling up the training of energy-based models. It has also been the first to achieve performance rivalling the state-of-the-art in both generative and discriminative learning within one hybrid model.
Reverse-Engineering Deep ReLU Networks
This paper investigated the commonly assumed notion that neural networks cannot be recovered from its outputs, as they depend on its parameters in a highly nonlinear way. The authors claimed that by observing only its output, one could identify the architecture, weights, and biases of an unknown deep ReLU network. By dissecting the set of region boundaries into components associated with particular neurons, the researchers showed that it is possible to recover the weights of neurons and their arrangement within the network.
Cricket Analytics and Predictor
Authors: Suyash Mahajan, Salma Shaikh, Jash Vora, Gunjan Kandhari, Rutuja Pawar,
Abstract: The paper embark on predicting the outcomes of Indian Premier League (IPL) cricket match using a supervised learning approach from a team composition perspective. The study suggests that the relative team strength between the competing teams forms a distinctive feature for predicting the winner. Modeling the team strength boils down to modeling individual player‘s batting and bowling performances, forming the basis of our approach.
Research Methodology: In this paper, two methodologies have been used. MySQL database is used for storing data whereas Java for the GUI. The algorithm used is Clustering Algorithm for prediction. The steps followed are as
- Begin with a decision on the value of k being the number of clusters.
- Put any initial partition that classifies the data into k clusters.
- Take every sample in the sequence; compute its distance from centroid of each of the clusters. If sample is not in the cluster with the closest centroid currently, switch this sample to that cluster and update the centroid of the cluster accepting the new sample and the cluster losing the sample.
For the research paper, read here
2.Real Time Sleep / Drowsiness Detection – Project Report
Author : Roshan Tavhare
Institute : University of Mumbai
Abstract : The main idea behind this project is to develop a nonintrusive system which can detect fatigue of any human and can issue a timely warning. Drivers who do not take regular breaks when driving long distances run a high risk of becoming drowsy a state which they often fail to recognize early enough.
Research Methodology : A training set of labeled facial landmarks on an image. These images are manually labeled, specifying specific (x, y) -coordinates of regions surrounding each facial structure.
- Priors, more specifically, the probability on distance between pairs of input pixels. The pre-trained facial landmark detector inside the dlib library is used to estimate the location of 68 (x, y)-coordinates that map to facial structures on the face.
A Study of Various Text Augmentation Techniques for Relation Classification in Free Text
Authors: Chinmaya Mishra Praveen Kumar and Reddy Kumar Moda, Syed Saqib Bukhari and Andreas Dengel
Institute: German Research Center for Artificial Intelligence (DFKI), Kaiserslautern, Germany
Abstract: In this paper, the researchers explore various text data augmentation techniques in text space and word embedding space. They studied the effect of various augmented datasets on the efficiency of different deep learning models for relation classification in text.
Research Methodology: The researchers implemented five text data augmentation techniques (Similar word, synonyms, interpolation, extrapolation and random noise method) and explored the ways in which we could preserve the grammatical and the contextual structures of the sentences while generating new sentences automatically using data augmentation techniques.
Smart Health Monitoring and Management Using Internet of Things, Artificial Intelligence with Cloud Based Processing
Author : Prateek Kaushik
Institute : G D Goenka University, Gurugram
Abstract : This research paper described a personalised smart health monitoring device using wireless sensors and the latest technology.
Research Methodology: Machine learning and Deep Learning techniques are discussed which works as a catalyst to improve the performance of any health monitor system such supervised machine learning algorithms, unsupervised machine learning algorithms, auto-encoder, convolutional neural network and restricted boltzmann machine .
Internet of Things with BIG DATA Analytics -A Survey
Author : A.Pavithra, C.Anandhakumar and V.Nithin Meenashisundharam
Institute : Sree Saraswathi Thyagaraja College,
Abstract : This article we discuss about Big data on IoT and how it is interrelated to each other along with the necessity of implementing Big data with IoT and its benefits, job market
Research Methodology : Machine learning, Deep Learning, and Artificial Intelligence are key technologies that are used to provide value-added applications along with IoT and big data in addition to being used in a stand-alone mod.
Single Headed Attention RNN: Stop Thinking With Your Head
Author: Stephen Merity
In this work of art, the Harvard grad author, Stephen “Smerity” Merity, investigated the current state of NLP, the models being used and other alternate approaches. In this process, he tears down the conventional methods from top to bottom, including etymology.
The author also voices the need for a Moore’s Law for machine learning that encourages a minicomputer future while also announcing his plans on rebuilding the codebase from the ground up both as an educational tool for others and as a strong platform for future work in academia and industry.
EfficientNet: Rethinking Model Scaling for Convolutional Neural Networks
Authors: Mingxing Tan and Quoc V. Le
In this work, the authors propose a compound scaling method that tells when to increase or decrease depth, height and resolution of a certain network.
Convolutional Neural Networks(CNNs) are at the heart of many machine vision applications.
EfficientNets are believed to superpass state-of-the-art accuracy with up to 10x better efficiency (smaller and faster).
Deep Double Descent By OpenAI
Authors: Mikhail Belkin, Daniel Hsu, Siyuan Ma, Soumik Mandal
In this paper , an attempt has been made to reconcile classical understanding and modern practice within a unified performance curve.
The “double descent” curve overtakes the classic U-shaped bias-variance trade-off curve by showing how increasing model capacity beyond the point of interpolation results in improved performance.
The Lottery Ticket Hypothesis
Authors: Jonathan Frankle, Michael Carbin
Neural network pruning techniques can reduce the parameter counts of trained networks by over 90%, decreasing storage requirements and improving computational performance of inference without compromising accuracy.
The authors find that a standard pruning technique naturally uncovers subnetworks whose initializations made them capable of training effectively. Based on these results, they introduce the “lottery ticket hypothesis:”
On The Measure Of Intelligence
Authors: Francois Chollet
This work summarizes and critically assesses the definitions of intelligence and evaluation approaches, while making apparent the historical conceptions of intelligence that have implicitly guided them.
The author, also the creator of keras, introduces a formal definition of intelligence based on Algorithmic Information Theory and using this definition, he also proposes a set of guidelines for what a general AI benchmark should look like.
Zero-Shot Word Sense Disambiguation Using Sense Definition Embeddings via IISc Bangalore & CMU
Authors: Sawan Kumar, Sharmistha Jat, Karan Saxena and Partha Talukdar
Word Sense Disambiguation (WSD) is a longstanding but open problem in Natural Language Processing (NLP). Current supervised WSD methods treat senses as discrete labels and also resort to predicting the Most-Frequent-Sense (MFS) for words unseen during training.
The researchers from IISc Bangalore in collaboration with Carnegie Mellon University propose Extended WSD Incorporating Sense Embeddings (EWISE), a supervised model to perform WSD by predicting over a continuous sense embedding space as opposed to a discrete label space.
Deep Equilibrium Models
Authors: Shaojie Bai, J. Zico Kolter and Vladlen Koltun
Motivated by the observation that the hidden layers of many existing deep sequence models converge towards some fixed point, the researchers at Carnegie Mellon University present a new approach to modeling sequential data through deep equilibrium model (DEQ) models.
Using this approach, training and prediction in these networks require only constant memory, regardless of the effective “depth” of the network.
IMAGENET-Trained CNNs are Biased Towards Texture
Authors: Robert G, Patricia R, Claudio M, Matthias Bethge, Felix A. W and Wieland B
Convolutional Neural Networks (CNNs) are commonly thought to recognise objects by learning increasingly complex representations of object shapes. The authors in this paper , evaluate CNNs and human observers on images with a texture-shape cue conflict. They show that ImageNet-trained CNNs are strongly biased towards recognising textures rather than shapes, which is in stark contrast to human behavioural evidence.
A Geometric Perspective on Optimal Representations for Reinforcement Learning
Authors: Marc G. B , Will D , Robert D , Adrien A T , Pablo S C , Nicolas Le R , Dale S, Tor L, Clare L
The authors propose a new perspective on representation learning in reinforcement learning
based on geometric properties of the space of value functions. This work shows that adversarial value functions exhibit interesting structure, and are good auxiliary tasks when learning a representation of an environment. The authors believe this work to open up the possibility of automatically generating auxiliary tasks in deep reinforcement learning.
Weight Agnostic Neural Networks
Authors: Adam Gaier & David Ha
In this work , the authors explore whether neural network architectures alone, without learning any weight parameters, can encode solutions for a given task. In this paper, they propose a search method for neural network architectures that can already perform a task without any explicit weight training.
Stand-Alone Self-Attention in Vision Models
Authors: Prajit Ramachandran, Niki P, Ashish Vaswani,Irwan Bello Anselm Levskaya, Jonathon S
In this work, the Google researchers verified that content-based interactions can serve the vision models . The proposed stand-alone local self-attention layer achieves competitive predictive performance on ImageNet classification and COCO object detection tasks while requiring fewer parameters and floating-point operations than the corresponding convolution baselines. Results show that attention is especially effective in the later parts of the network.
High-Fidelity Image Generation With Fewer Labels
Authors: Mario Lucic, Michael Tschannen, Marvin Ritter, Xiaohua Z, Olivier B and Sylvain Gelly
Modern-day models can produce high quality, close to reality when fed with a vast quantity of labelled data. To solve this large data dependency, researchers from Google released this work , to demonstrate how one can benefit from recent work on self- and semi-supervised learning to outperform the state of the art on both unsupervised ImageNet synthesis, as well as in the conditional setting.
The proposed approach is able to match the sample quality of the current state-of-the-art conditional model BigGAN on ImageNet using only 10% of the labels and outperform it using 20% of the labels.
ALBERT: A Lite BERT for Self-Supervised Learning of Language Representations
Authors: Zhenzhong Lan, Mingda Chen, Sebastian Goodman, Kevin G, Piyush Sharma and Radu S
The authors present two parameter-reduction techniques to lower memory consumption and increase the training speed of BERT and to address the challenges posed by increasing model size and GPU/TPU memory limitations, longer training times, and unexpected model degradation
As a result, this proposed model establishes new state-of-the-art results on the GLUE, RACE, and SQuAD benchmarks while having fewer parameters compared to BERT-large.
GauGANs-Semantic Image Synthesis with Spatially-Adaptive Normalization
Author: Taesung Park, Ming-Yu Liu, Ting-Chun Wang and Jun-Yan Zhu
Nvidia in collaboration with UC Berkeley and MIT proposed a model which has a spatially-adaptive normalization layer for synthesizing photorealistic images given an input semantic layout.
This model retained visual fidelity and alignment with challenging input layouts while allowing the user to control both semantic and style.
📣 Want to advertise in AIM? Book here

Subscribe to The Belamy: Our Weekly Newsletter
Biggest ai stories, delivered to your inbox every week..
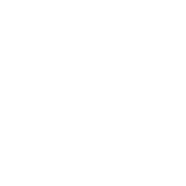
Discover how Cypher 2024 expands to the USA, bridging AI innovation gaps and tackling the challenges of enterprise AI adoption
© Analytics India Magazine Pvt Ltd & AIM Media House LLC 2024
- Terms of use
- Privacy Policy
Subscribe to Our Newsletter
The Belamy, our weekly Newsletter is a rage. Just enter your email below.
Subscribe to Our Youtube channel
A Review of recent work in wire electrical discharge machining (WEDM)
- This person is not on ResearchGate, or hasn't claimed this research yet.

- Ganpat University, U.V.Patel College of Engineering

Discover the world's research
- 25+ million members
- 160+ million publication pages
- 2.3+ billion citations

- MATH PROBL ENG
- Khondoker Safin Kaosar Saad

- Zayed Ur Rahman

- Shruti Sudhakar Dandge
- Shankar Chakraborty
- Claver Nsanzumuhire
- Bernard Munyazikwiye
- Vincent Niyigaba
- Ikua Bernard
- J NANOMATER
- K. Natarajan

- INT J ADV MANUF TECH
- Noor Dzulaikha Daud

- Saad K Shather
- Waqass S Khudhir

- CIRP ANN-MANUF TECHN
- K. Rajurkar

- R.F. Firestone
- W. Dekeyser
- C. Tricarico
- T. Tanimura
- C.J. Heuvelman

- J MANUF SYST

- Ivano Beltrami
- H.E. de Bruyn
- A.J. Pekelharing
- Recruit researchers
- Join for free
- Login Email Tip: Most researchers use their institutional email address as their ResearchGate login Password Forgot password? Keep me logged in Log in or Continue with Google Welcome back! Please log in. Email · Hint Tip: Most researchers use their institutional email address as their ResearchGate login Password Forgot password? Keep me logged in Log in or Continue with Google No account? Sign up
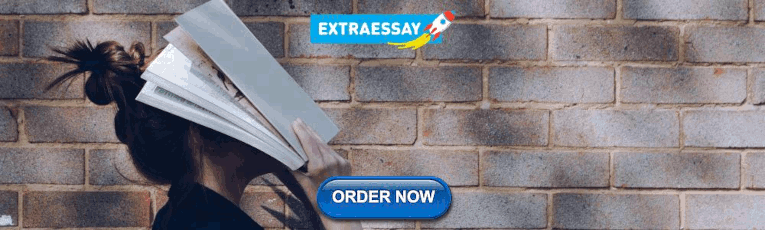
IMAGES
VIDEO
COMMENTS
Electrical Discharge Machining (EDM) is a non-conventional process that carries out machining depending on the materials' thermal conductivity, melting point and electric resistivity. ... Mahesh Dalvi, Rahul Chavan, Research paper on electrical discharge machining, Int. J. Eng. Appl. Sci. Technol. 6 (11) (2022) 72-75, ISSN No. 2455-2143 ...
Electro discharge mac hining (EDM) pro cess is a non -conventional and non-contact machining operation which is used. in industry for high precision products especially in manufacturing industries ...
Although many researches have been carried out concerning the machining of Ti-6Al-4V and Ti-6Al-4V ELI with EDM 38,39,40,41, research regarding other titanium grades is limited and ...
A paper entitled 'Discussion of electrical discharge machining in gas' written by Li et al. [33] recommend positive polarity to be employed in dry EDM because electrodes play main roles in collision and ionization and in order to ensure machining process stable at the spark discharge state, a certain gas pressure is necessary to strengthen ...
This paper reviews research studies on the EDM of different grades of tool steel materials. This article (i) pans out the reported literature in a modular manner with a focus on experimental and theoretical studies aimed at improving process performance, including material removal rate, surface quality, and tool wear rate, among others, (ii ...
Electrical discharge machining (EDM) is a widely used non-conventional machining technique in manufacturing industries, capable of accurately machining electrically conductive materials of any hardness and strength. ... Despite the fact that few research papers were published on this topic in the early 2000s, interest has steadily grown over ...
Electrical discharge machining (EDM) is a highly precise technology that not only facilitates the machining of components into desired shapes but also enables the alteration of the physical and chemical properties of workpieces. The complexity of the process is due to a number of regulating factors such as the material of the workpiece and tools, dielectric medium, and other process parameters.
Electric discharge machining (EDM) is widely used to fabricate complex 3D geometries with a high aspect ratio in electrically conductive hard materials. EDM-milling (EDMM) is an advancement of EDM which can cut complex features at the micro-level with high accuracy and precision. This study reports an overview of EDMM in micro- and macro-domain with recent advancement, challenges and future ...
This research paper reviewed the types of EDM machines, machining process, process parameters, and their effect on the performance of titanium alloy. It is an attempt to study of work of various researchers in the area of EDM and titanium alloy. EDM is highly recommended for difficult-to-cut materials.
This paper reviews the research trends in EDM on ultrasonic vibration, dry EDM machining, EDM with powder additives, EDM in water and modeling technique in predicting EDM performances. Introduction Electrical discharge machining (EDM) is a non-traditional concept of machining which has been widely used to produce dies and molds.
EDM oil or kerosene is a common type of dielectric liquid used, whereas gaseous dielectrics are also used (Fig. 1). Schematic of the EDM process [1] In precision manufacturing, electrical discharge machining (EDM) plays an important role [1, 2, 3]. EDM is widely used when precision is of great importance in the processing of conductive materials.
Abstract. Electrical discharge machining (EDM) is a highly regarded method for producing ultra-precise mechanical parts. In this study, the process parameters of die-sinking EDM using copper electrodes and American Iron and Steel Institute (AISI) P20 tool steel workpieces are optimized for various output responses.
In this paper, research works related to EDM (Electric Discharge Machining) of nimonic alloys, known for high specific strength and corrosion resistance at elevated temperatures, is discussed.
Electrical discharge machining (EDM) is a non-conventional machining technique that requires pulse generators to create sparks between the tool and the workpiece. ... pulse repetition frequency, etc. also can be controlled besides the voltage and current control. This paper has described various control schemes on top of the basic current and ...
Abstract. This paper focuses on the current machining process initiatives, carried out by various researchers relevant to the environmental aspects, thereafter predicting EDM performances. electrical discharge machining (EDM) is widely used unconventional machining method, because of its ability to process very hard material with precision.
This paper reviews the EDM machining superalloys like Nickel-based superalloys, Cobalt-based superalloys, iron based superalloy also the effect of parameters on MRR, EWR, and surface roughness.
In this paper, the research status of EDM-ECM working fluids is first reviewed. Secondly, the conversion of mechanisms in EDM-EDM machining is summarized. Then, the areas of application of EDM-ECM technology are introduced. Finally, the possible future efforts to expand the scope of the application of the technology are discussed and the ideas ...
Zhao WS, Meng QG, Wang ZL (2002) The application of research on powder mixed EDM in rough machining. J Mater Process Technol 129:30-33. Article Google Scholar Abbas NM, Yusoff N, Mahmod@Wahab R (2012) Electrical discharge machining (EDM): practices in Malaysian industries and possible change towards green manufacturing.
Sushil Kumar Chaudhary and Dr. R.S. Jadoun [2] has made a research review on the current advancement of EDM machine. They stated that Electrical discharge machining (EDM) is a process for shaping hard metals and forming deep complex shaped holes by arc erosion in all kinds of electro conductive materials.
I NTRODUCTION. Electrical discharge machining (EDM) is one of the most. extensively used non-conventional material removal p rocesses. It is an electro-thermal machining process where electrical ...
Sana M, Asad M, Farooq MU, et al. Machine learning for multi-dimensional performance optimization and predictive modelling of nanopowder-mixed electric discharge machining (EDM). Int J Adv Manuf Technol 2024; 130(11-12): 5641-5664.
Abstract. Electro-Discharge Machining (EDM) is one of the non-conventional. machining processes available, in which the material removal takes place. due to melting and vaporisation of electrode ...
This reviews paper describe the latest research trends in WEDM process in relation between different input process parameter and different output measure like material removal rate, surface roughness ,dimensional deviation , kerf width and wire wear ratio . ... Wire cut electrical discharge machining, Process parameters, Optimization methods 1 ...
Research Methodology: Machine learning, Deep Learning, and Artificial Intelligence are key technologies that are used to provide value-added applications along with IoT and big data in addition to being used in a stand-alone mod. For the research paper, read here. Single Headed Attention RNN: Stop Thinking With Your Head . Author: Stephen Merity
This paper reviews the vast array of. research work ca rried out from the EDM. process to the development of the WEDM. It. reports on the WEDM research involving the. optimization of the process ...