Top 10 Manufacturing Analytics Use Cases in 2024

Cem is the principal analyst at AIMultiple since 2017. AIMultiple informs hundreds of thousands of businesses (as per Similarweb) including 60% of Fortune 500 every month.
Cem's work focuses on how enterprises can leverage new technologies in AI, automation, cybersecurity(including network security, application security), data collection including web data collection and process intelligence.
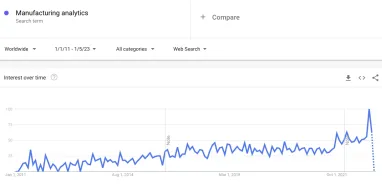
IDC estimates that the global big data analytics market revenue would’ve reached ~$274B by 2022. This would have made manufacturing as one of the top 3 industries with largest analytics growth.
Manufacturing analytics is one of the critical steps for manufacturing digital transformation required for Industry 4.0 , which aims to:
- Automate traditional manufacturing processes
- Reduce costs
- Improve efficiency
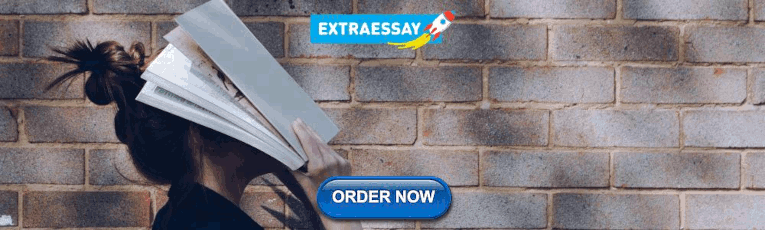
What is manufacturing analytics?
Manufacturing analytics is the practice of capturing, cleansing, and analyzing machine data in order to predict their future use, prevent failures, forecast maintenance requirements, and identify areas for improvement. Manufacturing data includes all structured and unstructured information collected manually or by using software from machines and humans during every stage of production until a product is launched to the market.
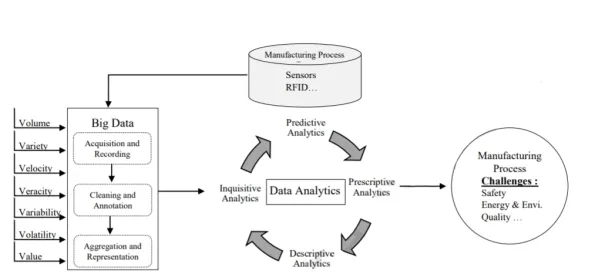
What are the use cases of manufacturing analytics?
Manufacturing processes produce a large volume of data from:
- Machines: robotics, sensors, actuators, IoT devices, etc.
- Operators: ERP, sales, logistics, etc.
This data can be collected and analytics can be applied to it for:
Supply chain
1. demand forecasting.
Demand forecasting relies heavily on historical data about supply levels, material costs, purchase trends, and customer behavior. Manufacturers can leverage analytics to:
- define the types of products to be manufactured in a certain period
- define out of stock products
- calculate the number of products to be manufactured
- forecast sales opportunities
2. Inventory management
Forecasting demands enables manufacturers to manage their inventory, purchase materials, and optimize storage capacities in a data-driven manner. Analytics also provides insights about:
- sales to inventory ratio which represents the average inventory over the net sales
- days in inventory which is the number of days a manufacturer holds their product before selling it)
- gross margin return on inventory (GMROI) which indicates how much gross margin a manufacturer gets back for each dollar invested in inventory.
Explore inventory management in more details.
3. Order management
Manufacturers can leverage predictive analytics to optimize the order management workflow by identifying products in demand, calculating the time required to build and ship every product, and defining the inventory needed to meet the demand for the finished product.
Explore order management in more details.
4. Maintenance optimization
Data collected from different manufacturing machines, tools, and devices, as well as data about operations and which machines they require, can be analyzed in order to:
- Predict when a machine will require maintenance based on time it’s been used and operations used in.
- Detect anomalies in operations which are caused by or will lead to machine failure.
- Prevent down time by planning machine breaks, fixes, or replacements.
Explore predictive maintenance in more details.
5. Risk management
Implementing analytics enables manufacturers to manage risks in a data-driven manner, such that they can:
- Determine recurring errors and prevent repetitive losses
- Predict insurance needs
- Monitor real-time machinery and operator work
- Identify real-time fails and system anomalies
- Plan risk management strategies
6. Price optimization
Leveraging analytics can help manufacturers understand the real price of a product based on the prices of materials, cost of operations, machines, and tools used or purchased for manufacturing. Additionally, manufacturers can leverage data about competitors, market trends, consumer behavior, and purchase history to optimize prices accordingly. Analytics can also help set dynamic prices which are based on demand, supply, competition price, and subsidiary product prices.
7. Automation and robotics
Analytics can provide an overall view of a manufacturing process, operation costs, as well as the number of operators and hours spent on a product. Large manufacturing firms can leverage these analyses to uncover automation or robotization opportunities which can reduce the time and cost of launching certain products
8. Transportation allocation
Manufacturers can leverage analytics on:
- Historical data : For predicting transportation time and vehicle requirements to deliver products to businesses or consumers.
- Real time data : For analyzing the impact of unplanned transportation events such as labor strikes or road works.
Product development
9. product progress measurement.
Based on historical data about the same or similar products, materials, machines, and tools used, as well as allocated employees for production, analytics can provide an estimation about the production process, when the product will be launched, which errors or pitfalls may be faced, and create a roadmap for the following procedures.
10. End user experience estimation
Product development teams can leverage analytics on product features, consumer behavior, and comments on online platforms, as well as competitor products, to estimate why end users buy certain products, when to launch similar products, and which features require optimization.
What other technologies are used in manufacturing?
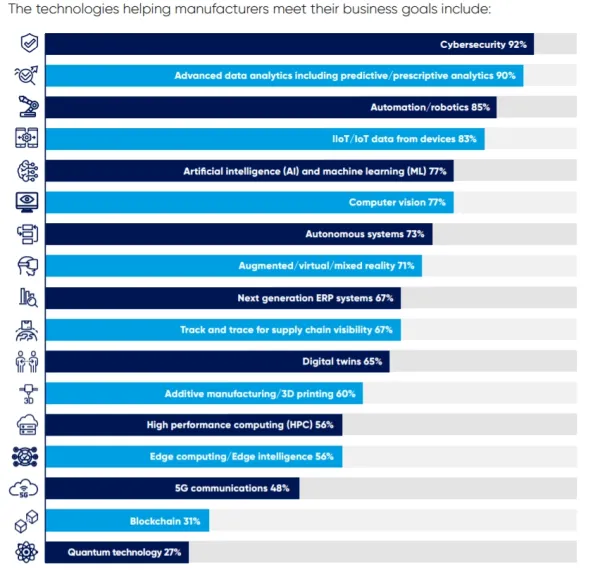
Some of the technologies leveraged today by manufacturers include:
Robotic process automation (RPA)
RPA is a type of software capable of replicating human interactions with computers in order to automate repetitive processes. Manufacturers can leverage RPA for supply chain management and stock optimization.
To explore use cases, feel free to read our article about the benefits and top 8 use cases of RPA in manufacturing .
AI has numerous applications in manufacturing including:
- Digital twins and digital twin of an organization
- Augmented reality
- Demand forecasting
- Generative design
- Quality assurance
- Process optimization
To explore AI use cases in manufacturing, read our in-depth article top 12 use cases and applications of AI in manufacturing .
If you believe your business will benefit from manufacturing technologies, feel free to check our data-driven lists of vendors for:
- Manufacturing Analytics Software
- Manufacturing API
- IoT Analytics Platform
- RPA Software
- Web Crawler
And you can contact us to guide you through the process

Cem's work has been cited by leading global publications including Business Insider, Forbes, Washington Post, global firms like Deloitte, HPE, NGOs like World Economic Forum and supranational organizations like European Commission. You can see more reputable companies and media that referenced AIMultiple.
Cem's hands-on enterprise software experience contributes to the insights that he generates. He oversees AIMultiple benchmarks in dynamic application security testing (DAST), data loss prevention (DLP), email marketing and web data collection. Other AIMultiple industry analysts and tech team support Cem in designing, running and evaluating benchmarks.
Throughout his career, Cem served as a tech consultant, tech buyer and tech entrepreneur. He advised enterprises on their technology decisions at McKinsey & Company and Altman Solon for more than a decade. He also published a McKinsey report on digitalization.
He led technology strategy and procurement of a telco while reporting to the CEO. He has also led commercial growth of deep tech company Hypatos that reached a 7 digit annual recurring revenue and a 9 digit valuation from 0 within 2 years. Cem's work in Hypatos was covered by leading technology publications like TechCrunch and Business Insider.
Cem regularly speaks at international technology conferences. He graduated from Bogazici University as a computer engineer and holds an MBA from Columbia Business School.
AIMultiple.com Traffic Analytics, Ranking & Audience , Similarweb. Why Microsoft, IBM, and Google Are Ramping up Efforts on AI Ethics , Business Insider. Microsoft invests $1 billion in OpenAI to pursue artificial intelligence that’s smarter than we are , Washington Post. Data management barriers to AI success , Deloitte. Empowering AI Leadership: AI C-Suite Toolkit , World Economic Forum. Science, Research and Innovation Performance of the EU , European Commission. Public-sector digitization: The trillion-dollar challenge , McKinsey & Company. Hypatos gets $11.8M for a deep learning approach to document processing , TechCrunch. We got an exclusive look at the pitch deck AI startup Hypatos used to raise $11 million , Business Insider.
To stay up-to-date on B2B tech & accelerate your enterprise:
Next to Read
Top 6 digital twins use cases for manufacturing in 2024, 14 case studies of manufacturing analytics in 2024, self-service reporting in 2024: the ultimate guide.
Your email address will not be published. All fields are required.
Related research
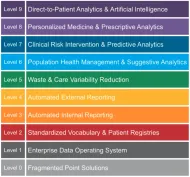
Healthcare Analytics Adoption Model: In-Depth Guide in 2024
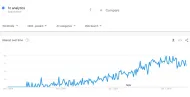
Top 6 Benefits of HR Analytics in 2024
Driving Efficiency: Data Analytics Use Cases in Manufacturing Excellence
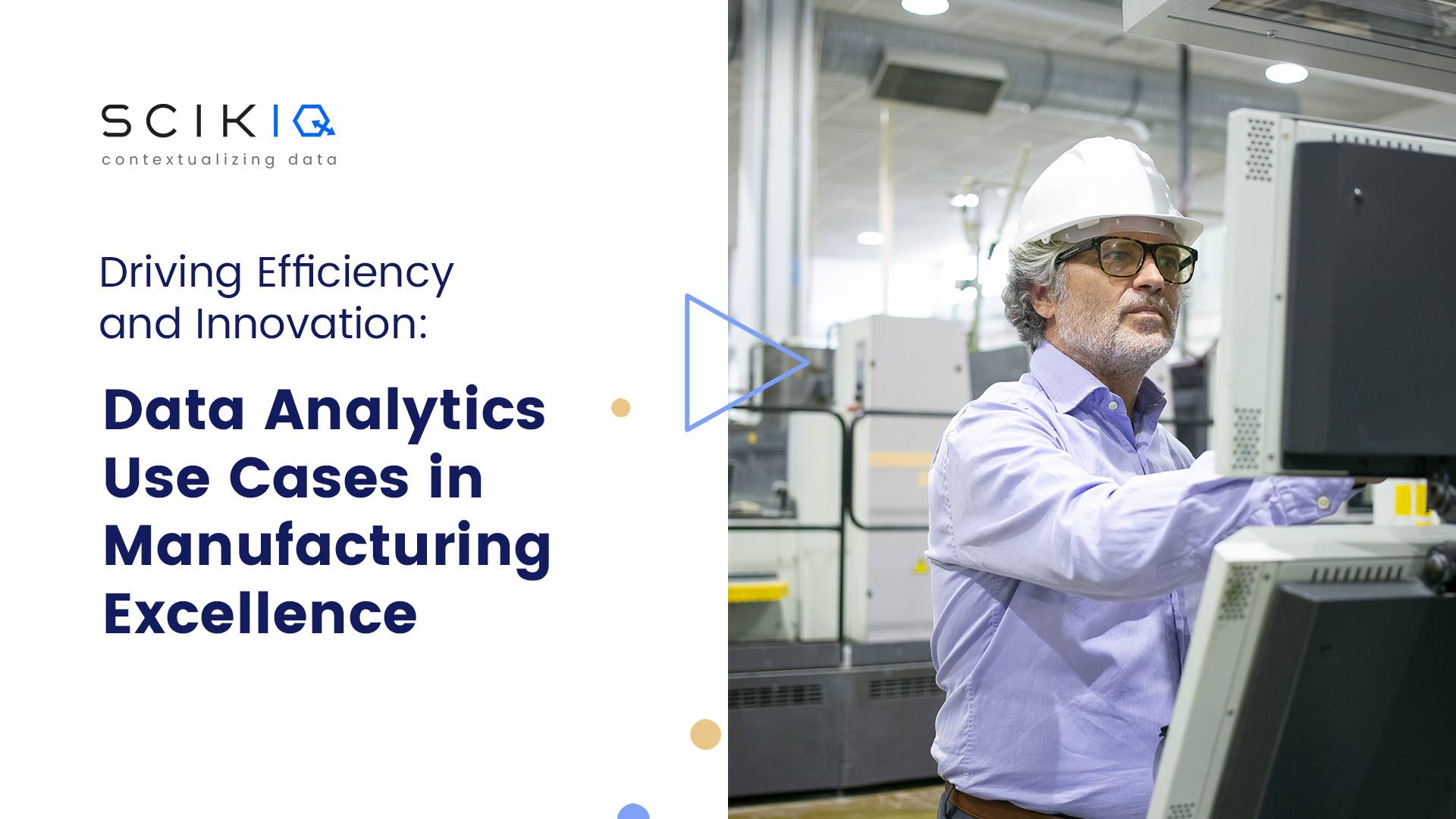
- June 16, 2023 July 21, 2023
This article delves into the world of data analytics use cases in manufacturing excellence, exploring how organizations leverage data-driven strategies to streamline operations, improve product quality, enhance efficiency, and stay ahead in a competitive market.
Data analytics in manufacturing excellence goes beyond traditional methods of production optimization. It leverages advanced analytics techniques, machine learning algorithms, and real-time data to extract meaningful insights from a wealth of manufacturing data.
- 90% of manufacturing executives say that data analytics is important to their business.
- 70% of manufacturing companies that use data analytics have seen a return on investment (ROI) of at least 20%.
- Data analytics can help manufacturers improve production efficiency, reduce costs, and improve product quality.
- The global market for data analytics in manufacturing is expected to reach $12.9 billion by 2023.
Examples of how data analytics is being used in manufacturing
Siemens: Siemens is using data analytics to improve the efficiency of its production lines. The company has installed sensors on its equipment to collect data on production processes. This data is then analyzed to identify areas where efficiency can be improved. As a result of these efforts, Siemens has been able to reduce the time it takes to produce a product by 20%.
General Electric: General Electric is using data analytics to improve the quality of its products. The company has developed a system that uses data analytics to identify potential defects in products before they are shipped to customers. This system has helped General Electric to reduce the number of defects in its products by 50%.
Nike: Nike is using data analytics to improve the performance of its athletes. The company has developed a system that uses data analytics to track the performance of athletes during training. This data is then used to provide athletes with personalized training plans that help them to improve their performance.
Data analytics enables manufacturers to improve overall equipment effectiveness (OEE), optimize energy usage, perform root cause analysis, and achieve continuous improvement through lean manufacturing principles. By leveraging data-driven insights, manufacturers can enhance productivity, reduce costs, improve product quality, and drive sustainable growth.
The top 20 use cases of data analytics in manufacturing:
- Predictive maintenance: Leveraging data analytics to predict equipment failures and optimize maintenance schedules, reducing downtime and improving operational efficiency.
- Quality control and defect detection: Analyzing data from sensors, inspection systems, and production processes to identify patterns and anomalies, enabling early detection of quality issues and reducing waste.
- Supply chain optimization: Integrating data from various sources to gain end-to-end visibility into the supply chain, optimizing inventory levels, improving demand forecasting, and enhancing overall supply chain efficiency.
- Overall equipment effectiveness (OEE) improvement: Analyzing production data to identify opportunities for process optimization, reduce downtime, minimize waste, and improve production efficiency.
- Root cause analysis: Utilizing data analytics to perform in-depth analysis of production data, identifying underlying causes of issues or defects and implementing corrective actions.
- Energy management and sustainability: Analyzing energy consumption data to identify opportunities for energy savings, optimize resource utilization, and reduce environmental impact.
- Demand forecasting and planning: Leveraging historical and market data to predict demand trends, optimize production planning, and improve inventory management.
- Product lifecycle management: Utilizing data analytics to gain insights throughout the product lifecycle, from design and development to production and maintenance, improving product quality and time-to-market.
- Supplier performance analysis: Analyzing supplier data to assess performance, identify bottlenecks, and optimize supplier relationships, ensuring a reliable and efficient supply chain.
- Process optimization: Using data analytics to analyze process data and identify areas for improvement, optimizing production processes, and reducing variability.
- Real-time production monitoring: Implementing real-time data analytics to monitor production metrics, identify deviations, and enable timely interventions to maintain production efficiency.
- Warranty and service analytics: Analyzing warranty and service data to identify recurring issues, improve product reliability, and optimize service and maintenance strategies.
- Continuous improvement and lean manufacturing: Utilizing data analytics to identify opportunities for continuous improvement, reduce waste, and implement lean manufacturing principles.
- Asset utilization and optimization: Analyzing data from production equipment to optimize asset utilization, improve equipment reliability, and minimize downtime.
- Productivity and labor optimization: Leveraging data analytics to analyze labor data, identify productivity bottlenecks, and optimize workforce allocation and scheduling.
- Supply chain risk management: Applying data analytics to identify and mitigate supply chain risks, enabling proactive risk management strategies and ensuring business continuity.
- Regulatory compliance and reporting: Utilizing data analytics to ensure compliance with regulations, automate reporting processes, and improve accuracy and efficiency in compliance management.
- Customer sentiment analysis: Analyzing customer feedback and sentiment data to gain insights into product satisfaction, identify improvement areas, and enhance customer experience.
- Smart factory optimization: Leveraging IoT data and real-time analytics to optimize operations, monitor equipment performance, and enable data-driven decision-making in a smart factory environment.
- Continuous data-driven improvement: Implementing a culture of continuous improvement through data analytics, using insights to drive ongoing enhancements across all aspects of manufacturing operations.
The use cases of data analytics in manufacturing excellence are vast and diverse, ranging from predictive maintenance and quality control to supply chain optimization and process efficiency.
By harnessing advanced analytics techniques, machine learning algorithms, and real-time data, manufacturers can make informed decisions, proactively address issues, and drive innovation. Learn how a CEO Leads the Company to Success with Data-Driven Insights. The journey towards manufacturing excellence continues, and data analytics serves as a guiding light, empowering organizations to unlock their full potential, drive operational efficiency, and achieve remarkable outcomes.
Explore what Data Analytics use cases can be applied to Manufacturing, Finance, Marketing, Telecom, and Banking.
Explore more about what we do best
SCIKIQ Data Lineage Solutions : Data Lineage steps beyond the limitations of traditional tools. SCIKIQ Data Visualization : Transforming BI with Innovative Reporting and Visualization SCIKIQ Data curation: AI in Action with Data Prep Studio Automating Data Governance : A game changer for efficient data management & great Data Governance.
chandan Mishra
Unlocking financial success: data analytics use cases for finance transformation, data analytics case studies that will inspire you., related product.
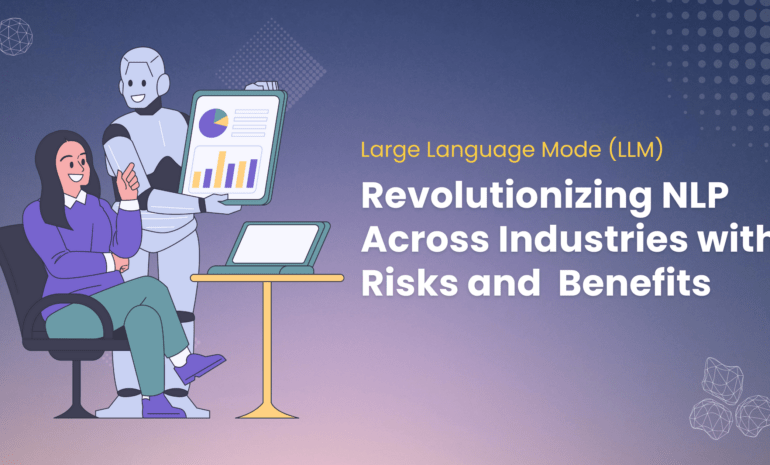
- Banking Blogs Cyber Data mangement Development Healthcare Logistics Retail SCIKIQ
What is LLM or Large Language model ?
- April 12, 2024 May 24, 2024
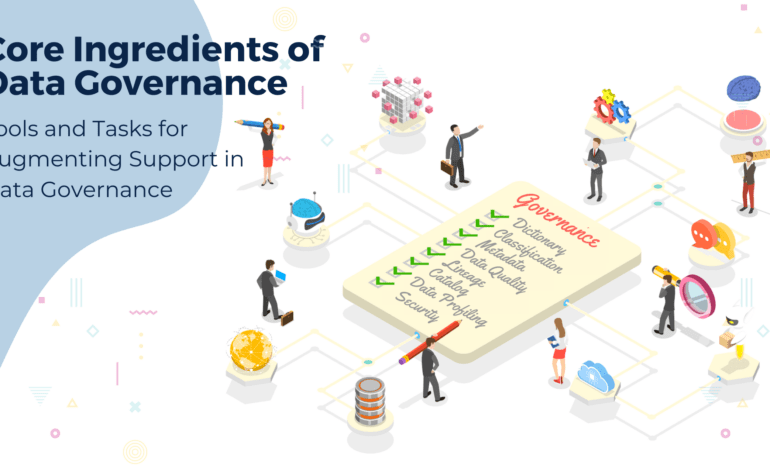
- Agency Banking Blogs Data mangement Development Healthcare Logistics Retail Software Supply chain Telecom
The Critical Ingredients for Successful Data Governance & automation
- April 5, 2024 May 24, 2024
- Pingback: Data Analytics Case Studies
- Pingback: Top 20 Use Cases of Data Analytics for Finance Transformation
- Pingback: Pivoting through Telecom Analytics and Network Innovation
- Pingback: SCIKIQ: Data Analytics and Data Management Articles and thoughts
- Pingback: The top 20 most popular statistical models for Data Analytics
Leave a Reply Cancel reply
Your email address will not be published. Required fields are marked *
Save my name, email, and website in this browser for the next time I comment.
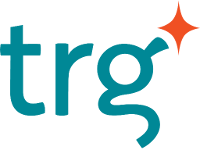
Data Analytics for Manufacturing: the Tesla’s Case Study (Part 1)
Posted by Thai Pham on Sun, Apr 30, 2017
If Tesla Motors is to achieve the aggressive goal of making 1 million cars by 2020 set out by its CEO Elon Musk, it will partly be because of the application of data analytics in its manufacturing operations.
Data analytics for manufacturing
- Blindness to data
Tableau’s advantages
Looking at data from different angles, process monitoring.
This April marked a historic moment for the automotive industry when Tesla briefly became the US’ most valuable carmaker. Its market capitalisation reached US$51 billion on April 10, surpassing US$50 billion of General Motors (GM) and US$45 billion of Ford.
Why do investors value Elon Musk’s electric car manufacturer more than GM and Ford even though Tesla’s 2016 sales volume was only 76,000 vehicles, a fraction of Ford’s (6.6 million) and GM’s (10 million), and it is still in the red, posting a net loss of US$675 million last year?
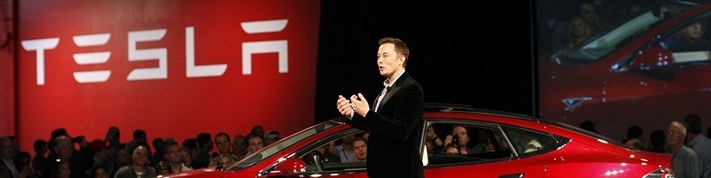
Musk’s bold vision for the future of transportation, in which all vehicles will eventually be all-electric and fully autonomous, certainly plays an important part. To make that vision a reality, CEO Elon Musk has set ambitious goals for Tesla for production capacity.

In 2013, Tesla delivered just around 20,000 Model S full-size sedans, the company’s first series production vehicle. 3 years later, the annual production rate reached 100,000. And Elon Musk is aiming to deliver 500,000 vehicles by 2018 and 1,000,000 by 2020.
Last December, the city of Fremont, California, approved Tesla’s plans to double the size of its factory there. There will be an additional 420,000 square meters of manufacturing space. And the number of workers is expected to increase from 6,000 to 9,000.
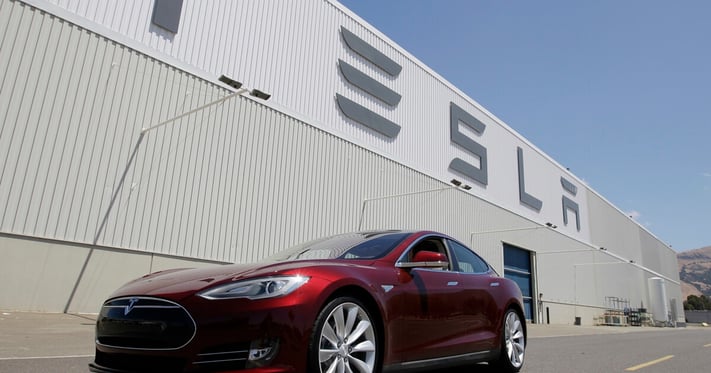
This massive expansion is crucial as Tesla’s product line continues to grow rapidly. Prior to 2015, the Fremont factory only built the Model S, which was later joined by the Model X SUV. Production of the more affordable Model 3 is scheduled to commence later this year. At present, Tesla has received over 370,000 pre-orders for Model 3.
Such rapid production growth and aggressive goals can undoubtedly create a lot of chaos. And Tesla is no stranger to missed deadlines. According to the Wall Street Journal, the company has failed to meet its projections more than 20 times in the past 5 years. As part of the ongoing effort to tame chaos and improve manufacturing efficiency, Tesla has turned to advanced data analytics software .
Back to the top
Tesla production data is fed into several different key systems. The first major one is in the MES (Manufacturing Execution System). This software essentially is the air traffic controller of the entire production process. It can tell a certain item in the assembly line where it should be heading, keep track of the production orders and quality issues, and collect basic measurements. The MES uses Oracle/SQL Server databases.
More in-depth and customised test data is stored in a separate MySQL Test database which was developed by Tesla’s in-house dev team. Additionally, the web-enabled QuickBase is also used to track process changes and ongoing quality issues due to its flexibility and ease of use. And finally, Excel spreadsheets are used for one-off manual reports.
Not only does data go into many different places, but it also has to serve highly diverse audiences, who have very different interests. Whereas people working in Production care about yield and rework calculation, people in Equipment Sustaining may care about preventive maintenance, and people in Process Sustaining are interested in root cause investigation. Sometimes, people do not know where to get the data they need.
Read more: Business Intelligence in the Manufacturing Industry
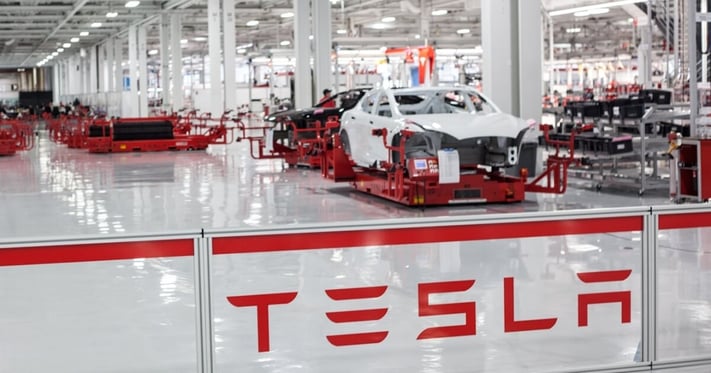
‘Blindness to data’
Apart from a wide variety of databases and data reporting needs, there were plenty of different tools employed by Tesla staff. Most relied on Excel or an in-house app built on LabView, and a select few knew how to use MySQL Workbench or R.
This situation caused a lot of frustration when people had a hard time looking for the right data. Bottlenecks were created since only a handful of people had direct access to most data as well as the expertise to use advanced data analytics tools. And because the majority of staff had to use Excel for data handling, the process was slow and inefficient. Consequently, people were often unaware of what was going on in other parts of the production line.
The top data reporting needs are:
- Production counts and yield
- Quality defect tracking/analysis
- Statistical Process Control (SPC) and Process monitoring
- Root-cause investigation
- Open-ended data exploration
- Statistical analysis - DOE, GRR, etc.
It is worth noting that most of these reporting needs are heavy on data visualisation and data exploration rather than on statistics and scripting. This played an important part in Tesla’s decision to adopt Tableau data analytics software .
Read more: 3 Best Business Intelligence & Analytics Vendors 2017
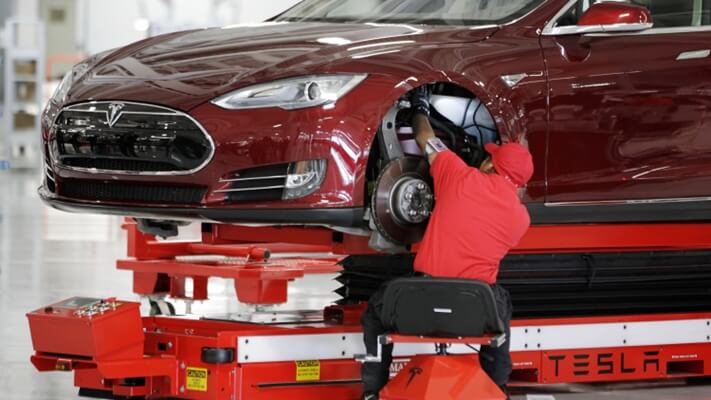
One distinct advantage of Tableau is “data source agnostic”, i.e. users no longer have to care where the data comes from. They can pull any data from any source at any time. They can view data located in MES, Test DB, QuickBase or Excel files side-by-side on the same dashboard.
Tableau also allows ordinary users, even those who are not programmers, to easily create powerful and tailored visualisations. Before that, they would have had to spend at least 6 months learning R to build such data visualisations.
Another huge improvement brought about by Tableau is publishing and sharing. In the past, those capable of using analytics tools often had to export their works and send them to others via email. This approach was time-consuming and lacked interactions. Conversely, Tableau users can easily publish their works and let others interact with them on their web browsers.
In the next sections, we will explore some applications of Tableau in building production reporting tools at the Tesla factory.
To have a complete picture, people need to be able to look at and drill down data from multiple angles. Then trends and patterns are much easier to detect.
The following visualisation workbook shows 3 different views of a single set of data: the number of quality issues.
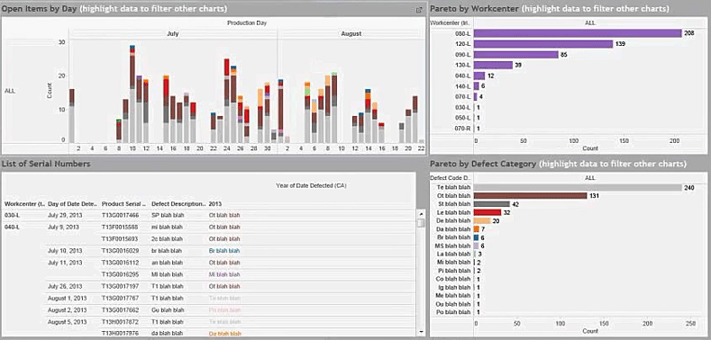
The top left visualisation shows the defect counts over time. The top right visualisation counts the number of defects by work centres. And at the bottom right corner is the visualisation of defects by categories.
Users from Process Sustaining use this workbook in their day-to-day jobs. They can drill down the data in any way they want. For instance, they can easily see the number of defects in each work centre by dates and by categories just by clicking on that particular work centre.
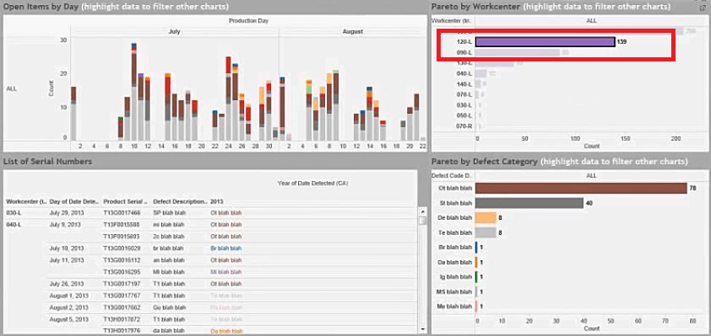
Then they can filter down even further by clicking on a defect category and see the number of that specific defect type over time.
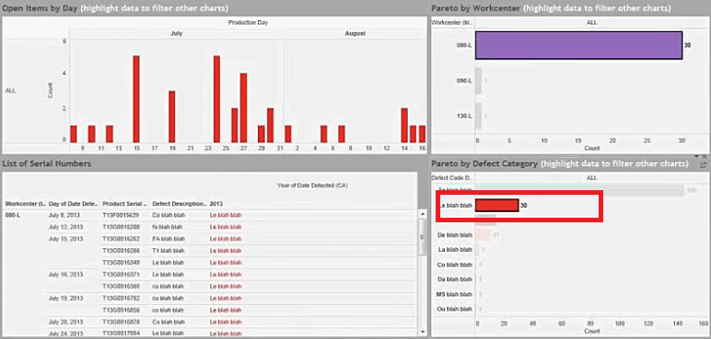
The bottom left corner is where users can see the detailed information on each quality defect, including notes from individuals who conducted the inspection. So users can perform a context drill-down from high-level data to individual serial numbers on the same screen.
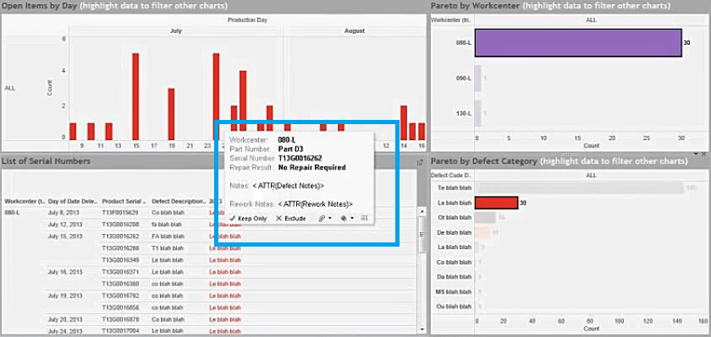
The following dashboard monitors the number of false part rejects, which happen when parts are scanned by barcode scanners and falsely rejected. This could be due to several reasons. The parts may not be held at the right angle towards the scanners or the scanners are misaligned.
The following dashboard shows data from 19 work centres (MAC01 to MAC19); each has 10 nests (A to J) where the barcodes are scanned. The false reject rates are represented by colours. A green nest means the false reject rate is within the limit.
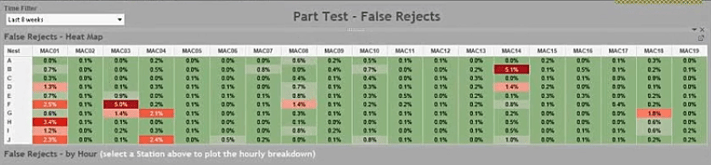
Users can click on one particular work centre and see the trends of false rejects of individual nests over time. More important, they can pull equipment logs of the same period of time from a separate database and see if someone has already logged the scanners down and alerted the maintenance crew and if the false reject rate of a scanner has dropped after it was repaired.
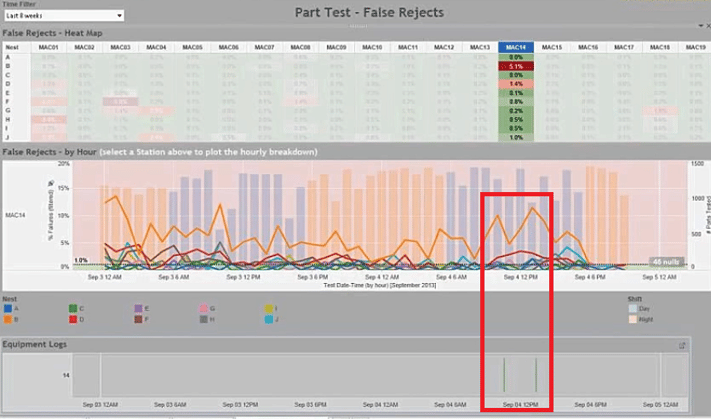
In this particular case, there were 2 maintenance alerts put in for the scanner of nest B / work centre MAC14. And the rate has gone down after the 2 nd repair.
The point of such process monitoring is to be able to see not only what is going on but also the deeper context of the actions taken.
This is the 1 st part of our article. Please read the second part or subscribe to our blog for the latest content about Business Intelligence and Analytics.
You can also request a Tableau demo to see how this solution can help your business.
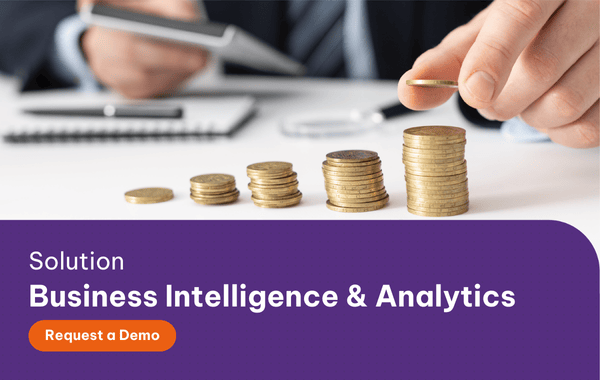
Topics: Business Intelligence , Analytics
- What You Need to Know about Data Fabric and Do You Need One?
- 8 Ways Technology Can Help Hotels Succeed In the Next Decade (Pt.2)
- The Use of Spreadsheets and Modern Cloud Adoption in Businesses
- Infor SaaS: The Trusted Cloud Infrastructure for Your Business Solutions
- Proper Data Preparation: Key Ingredient Ensuring a Smooth Cloud Migration
- The Popularisation of AI-Powered Applications: Should You Be Afraid of ChatGPT?
Subscribe to TRG Blog
Posts by topic.
- Amazon Web Services (1)
- Analytics (69)
- Business Intelligence (72)
- Cloud Computing (101)
- Cloud OS (1)
- CSR activities (1)
- cybersecurity (8)
- Digital Transformation (60)
- digital workplace (2)
- Enterprise Performance Management (EPM) (111)
- Enterprise Resource Planning ERP (148)
- Financial Accounting Management Software (290)
- Financial consolidation, planning and reporting (111)
- Hospitality solutions (103)
- Inbound marketing (4)
- Infor SunSystems (92)
- Planning and Budgeting (68)
- project management (1)
- Retail Management System (130)
- Rick Yvanovich (1)
- Talent Management (322)
- Technology trends (116)
Upcoming TRG Events
Latest Posts
Most viewed posts, our editorial mission.

With TRG International Blogs, it is our mission to be your preferred partner providing solutions that work and we will make sure to guide your business to greatness every day.
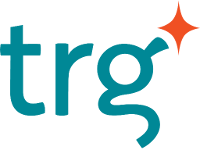
IT, Talent and F&B - we think it's a great combination.
We've thrived since 1994 resulting in lots of experience to share, we are beyond a companion, to more than 1,000 clients in 80+ countries.
Follow us on Social Media
Enterprise solutions.
- Financial Management
- Hospitality
- Resource Planning (ERP)
- BI & Analytics
- Retail Management
- Performance Management
Talent Solutions
- Recruitment
- Training & Development
- Sales & Customer Services
- Retention & Engagement
- Customised Solution
- Join our team
- Urgent Vacancies
- Internships
- Career Days
- Be our partner

© 2023 TRG International. Privacy Policy / Тerms & Conditions / Site map / Contact Us
TRG encourages websites and blogs to link to its web pages. Articles may be republished without alteration with the attribution statement "This article was first published by TRG International (www.trginternational.com)" and a clickable link back to the website. We are changing support for TLS 1.0 and older browsers. Please check our list of supported browsers.
The future of manufacturing is powered by data and analytics. Here's why
Just 39% of manufacturing executives report that they have successfully scaled data-driven use cases beyond the production process of a single product. Image: REUTERS/Noah Berger/File Photo
.chakra .wef-1c7l3mo{-webkit-transition:all 0.15s ease-out;transition:all 0.15s ease-out;cursor:pointer;-webkit-text-decoration:none;text-decoration:none;outline:none;color:inherit;}.chakra .wef-1c7l3mo:hover,.chakra .wef-1c7l3mo[data-hover]{-webkit-text-decoration:underline;text-decoration:underline;}.chakra .wef-1c7l3mo:focus,.chakra .wef-1c7l3mo[data-focus]{box-shadow:0 0 0 3px rgba(168,203,251,0.5);} Memia Fendri
Felipe bezamat, ruben behaeghe.
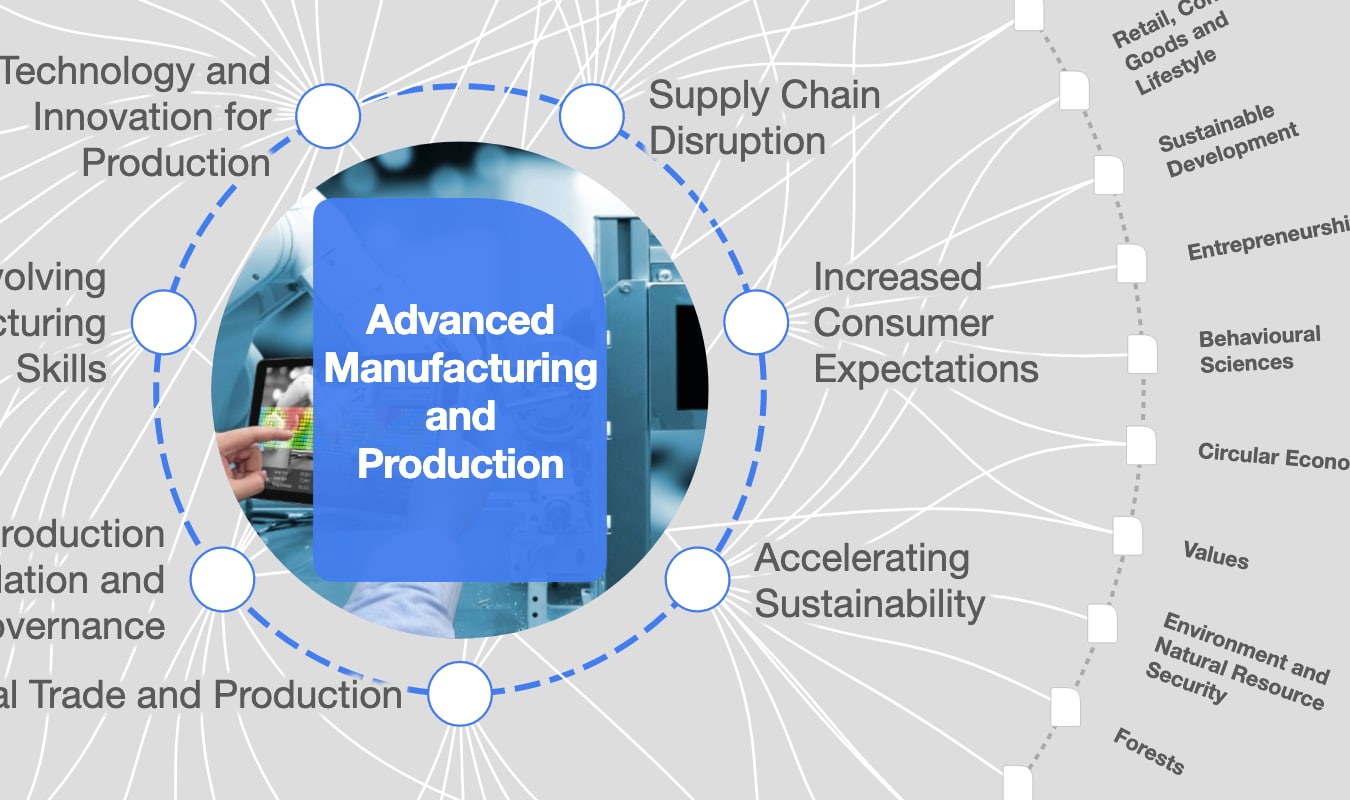
.chakra .wef-9dduvl{margin-top:16px;margin-bottom:16px;line-height:1.388;font-size:1.25rem;}@media screen and (min-width:56.5rem){.chakra .wef-9dduvl{font-size:1.125rem;}} Explore and monitor how .chakra .wef-15eoq1r{margin-top:16px;margin-bottom:16px;line-height:1.388;font-size:1.25rem;color:#F7DB5E;}@media screen and (min-width:56.5rem){.chakra .wef-15eoq1r{font-size:1.125rem;}} Advanced Manufacturing is affecting economies, industries and global issues
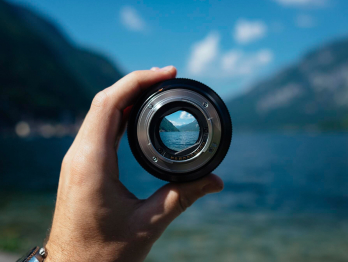
.chakra .wef-1nk5u5d{margin-top:16px;margin-bottom:16px;line-height:1.388;color:#2846F8;font-size:1.25rem;}@media screen and (min-width:56.5rem){.chakra .wef-1nk5u5d{font-size:1.125rem;}} Get involved with our crowdsourced digital platform to deliver impact at scale
Stay up to date:, advanced manufacturing and supply chains.
- Effective use of data in manufacturing can make businesses more sustainable and more profitable.
- But just 39% of manufacturing executives report that they have successfully scaled data-driven use cases beyond the production process of a single product.
- The Forum’s Manufacturing Data Excellence Framework provides a guide to developing new analytics capabilities and partnerships, giving manufacturers a playbook for extracting greater value from their data.
The manufacturing industry is on the verge of a data‑driven revolution. Companies are collaborating in hyperconnected value networks, using data‑and‑analytics applications to drive productivity, develop new customer experiences and improve the societal and environmental impact of companies.
This technological advance has come at a time of uncertainty. Climate change, supply chain disruption and conflict plague the global system — but the innovative use of data in manufacturing could actually be a stabilizing force for the global manufacturing industry.
Have you read?
Reducing the carbon footprint of the manufacturing industry through data sharing, advanced manufacturing: 3 priorities for industry and government leaders, how to master manufacturing's data and analytics revolution, data, analytics and manufacturing.
To realize this vision of hyperconnected value networks, manufacturers must employ a large variety of data‑and‑analytics applications, such as predictive maintenance, advanced robotics and tracking and tracing in supply networks. The underlying data assets are the lifeblood of these applications. This data can:
- Provide actionable insights by discerning patterns from data through human analysis of reports and dashboards.
- Predict future outcomes for business stakeholders to act upon, using advanced analytics on historical data.
- Enable self-optimizing systems that take autonomous action through self-learning self-steering algorithms, with input from historical and real-time data.
While most manufacturers have now begun their journey towards manufacturing data excellence, many are still struggling to capture significant value from it. A 2021 study , surveying over 1300 manufacturing executives, revealed that just 39% had successfully scaled data-driven use cases beyond the production process of a single product and thus achieved a clearly positive business case.
Unlocking value by sharing data
To accelerate the development of globally connected manufacturing data ecosystems, the Forum and its community have developed a Manufacturing Data Excellence Framework. The framework helps companies develop new capabilities, build new partnerships and evaluate the maturity of their data-driven applications. They can also use it to assess their progress in establishing the organizational and technological enablers required to extract value from data at a company, supply chain and ecosystem level.
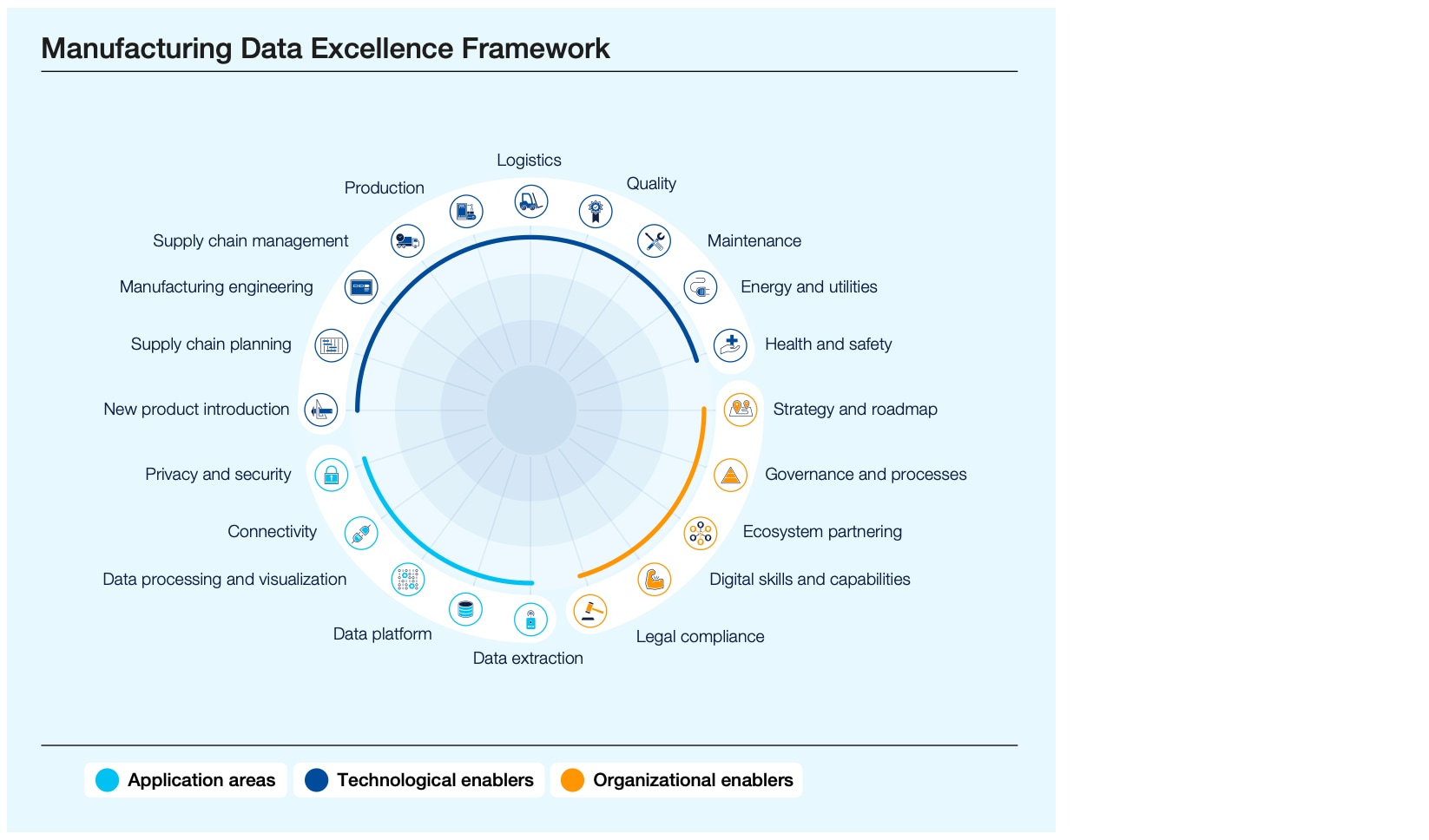
We asked members of the Forum’s “ Unlocking Value in Manufacturing Through Data Sharing ” initiative to share how they addressed these challenges and how engaging in the community and applying the Manufacturing Data Excellence Framework has helped their businesses.
Here’s what they had to say:
Michelangelo Canzoneri, Global Head of Group Smart Manufacturing, Merck Group
Cutting-edge plug-and-produce technologies, advanced robotics and AI-enabled systems have placed smart manufacturing at the heart of the Fourth Industrial Revolution. At the forefront of this wave is the encapsulation of product and manufacturing knowledge through Digital Twins, powered by data. By converging science and technology, Digital Twins offer various manufacturing advantages, including the simulation of production processes and supply chains for improved prediction as well as a more efficient approach to quality-by-design.
It has been invaluable to be part of this pioneering community, learning together and sharing best practices. These collaborations have enabled us to evaluate how to further incubate and scale data ecosystems for manufacturing across the value chain. The next step for manufacturing stakeholders using Digital Twins will be to develop a collaborative and safe approach to share data and models to overcome the interoperability challenge, exchange data on a common framework and adapt with speed and agility to the ever-changing environment.
Gabriel Gonzalez, Senior Vice President, Corporate Production, ZF Group
Data ecosystems are a huge advantage to operations excellence, whether inside our plants, across global supply chains or at the level of our final customers. At ZF, we have been developing our Digital Manufacturing Platform that we are currently rolling out through all our plants to bring them to a level of digital usage and thus leverage our complete performance: condition monitoring or OEE analyzers as well as shopfloor management applications.
Participating in the World Economic Forum’s Unlocking Value in Manufacturing through Data Sharing initiative helped us increase knowledge about our plant's digital maturity. We learned to work with it with an intensive program of six self-assessments in plants from Europe and North America. Clear advantages are the quick assessment time needed, an easy management system without any need of external assistance for the plants and the possibility of comparing results and best practices with many other companies.
The Framework could be improved to increase the granularity of the assessment, and to derive a roadmap to full digitalization that considers cybersecurity and connectivity.
Dr. Gunter Beitinger, Senior Vice-President Manufacturing and Head of Factory Digitalization, Siemens
Winning the race against global warming and limiting it to below 2.0 °C is one of the biggest challenges humanity faces, and decarbonizing industrial value chains is a necessary action. But we will only win this race if we start to share data along the supply chain to aid decarbonization and take responsible and sustainable actions in all aspects based on actual emission numbers, not on estimates.
Data ecosystems are a prerequisite to addressing this challenge. In the World Economic Forum’s working group Unlocking Value in Manufacturing through Data Sharing, we defined how such ecosystems must be designed to overcome the fundamental conflict between transparency and confidentiality. We developed an approach for trustworthy exchange in supply chains and funded an association on these principles, which allows us to use a decentral, open and trustworthy ecosystem that also guaranties data sovereignty.
Haldun Dingec, Executive Director, Digital Production Techniques, Arçelik
In manufacturing, data helps create end-to-end connected value chains. You need this to be more sustainable and agile, to improve productivity and drive an innovative customer journey. In this digital era, Fourth Industrial Revolution technologies are making data-powered ecosystems a reality in order to build applications such as demand forecasting, quality assurance intelligence, smart energy management, smart workforce management, field surveillance, supplier connectivity and fully customized products.
Engaging in the World Economic Forum’s Unlocking Value in Manufacturing through Data Sharing initiative opened my eyes to the possibilities surrounding data exchanges. It also allowed me to overcome the barriers of intellectual property protection and sharing technical knowledge because I had the opportunity to consider otherwise. Completing one project with a team of experts coming from different companies was an unbelievable experience, and happily, the project was about sustainability.
We look forward to continuing this journey with the community to realize a vision of hyperconnected value networks driven by data and explore how intelligent manufacturing technologies such as artificial intelligence can further help capitalize on the power of data.
Don't miss any update on this topic
Create a free account and access your personalized content collection with our latest publications and analyses.
License and Republishing
World Economic Forum articles may be republished in accordance with the Creative Commons Attribution-NonCommercial-NoDerivatives 4.0 International Public License, and in accordance with our Terms of Use.
The views expressed in this article are those of the author alone and not the World Economic Forum.
Related topics:
The agenda .chakra .wef-n7bacu{margin-top:16px;margin-bottom:16px;line-height:1.388;font-weight:400;} weekly.
A weekly update of the most important issues driving the global agenda
.chakra .wef-1dtnjt5{display:-webkit-box;display:-webkit-flex;display:-ms-flexbox;display:flex;-webkit-align-items:center;-webkit-box-align:center;-ms-flex-align:center;align-items:center;-webkit-flex-wrap:wrap;-ms-flex-wrap:wrap;flex-wrap:wrap;} More on Manufacturing and Value Chains .chakra .wef-17xejub{-webkit-flex:1;-ms-flex:1;flex:1;justify-self:stretch;-webkit-align-self:stretch;-ms-flex-item-align:stretch;align-self:stretch;} .chakra .wef-nr1rr4{display:-webkit-inline-box;display:-webkit-inline-flex;display:-ms-inline-flexbox;display:inline-flex;white-space:normal;vertical-align:middle;text-transform:uppercase;font-size:0.75rem;border-radius:0.25rem;font-weight:700;-webkit-align-items:center;-webkit-box-align:center;-ms-flex-align:center;align-items:center;line-height:1.2;-webkit-letter-spacing:1.25px;-moz-letter-spacing:1.25px;-ms-letter-spacing:1.25px;letter-spacing:1.25px;background:none;padding:0px;color:#B3B3B3;-webkit-box-decoration-break:clone;box-decoration-break:clone;-webkit-box-decoration-break:clone;}@media screen and (min-width:37.5rem){.chakra .wef-nr1rr4{font-size:0.875rem;}}@media screen and (min-width:56.5rem){.chakra .wef-nr1rr4{font-size:1rem;}} See all
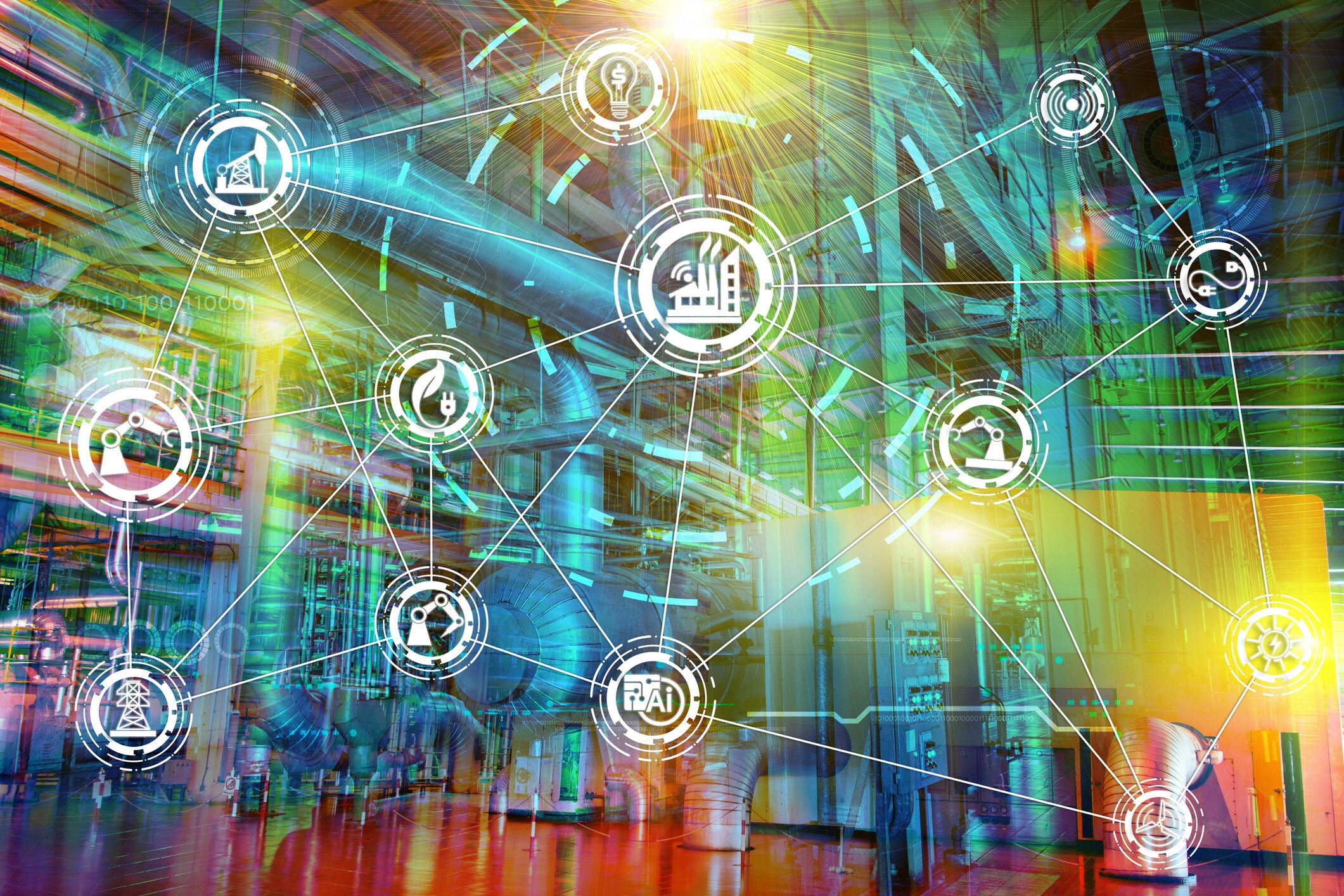
3 ways manufacturers can build a culture of cyber resilience
Blake Moret and Kiva Allgood
June 7, 2024
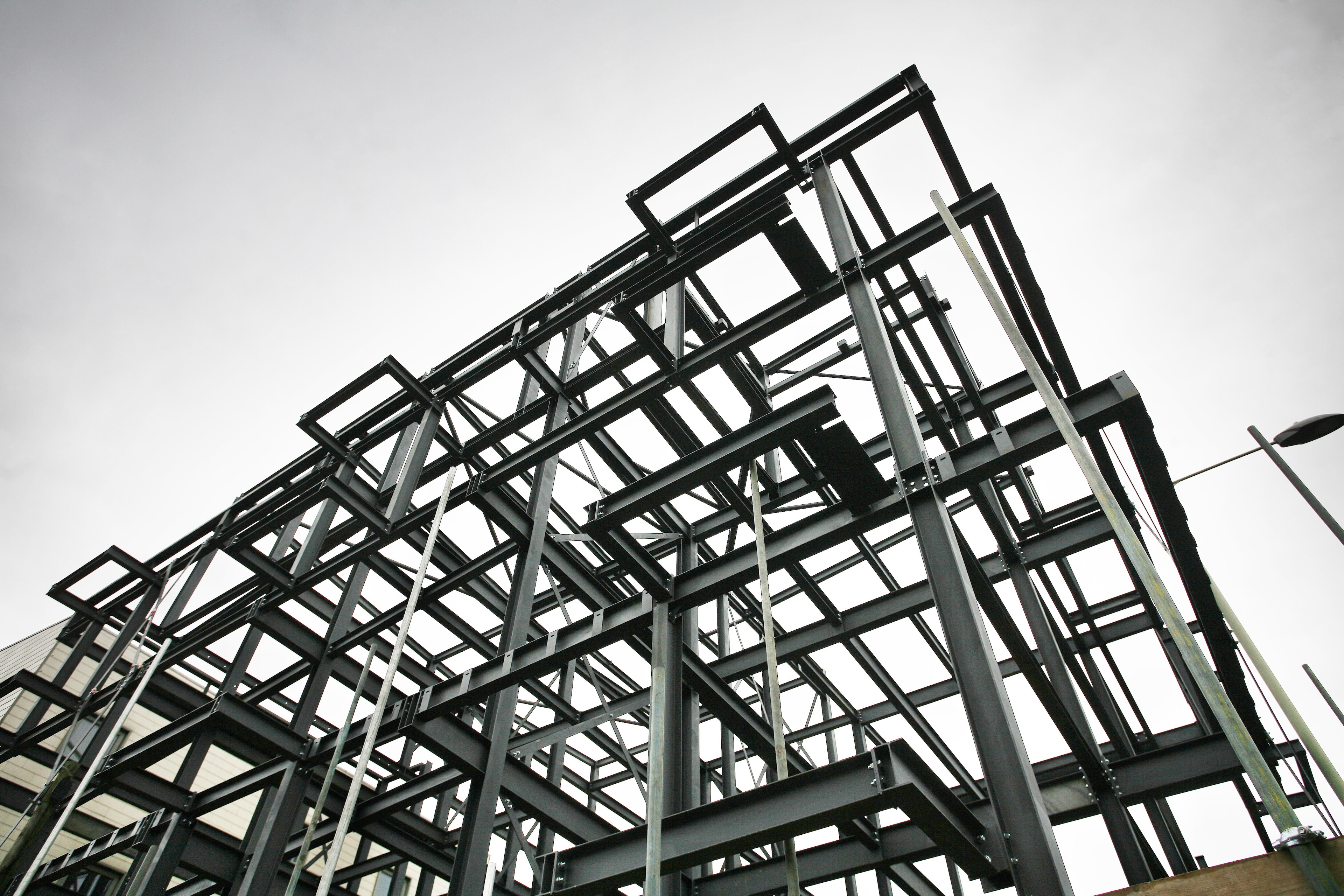
How the First Movers Coalition is working to decarbonize the aluminium, steel and cement and concrete sectors
Jelena Aleksić and Daniel Boero Vargas
June 4, 2024
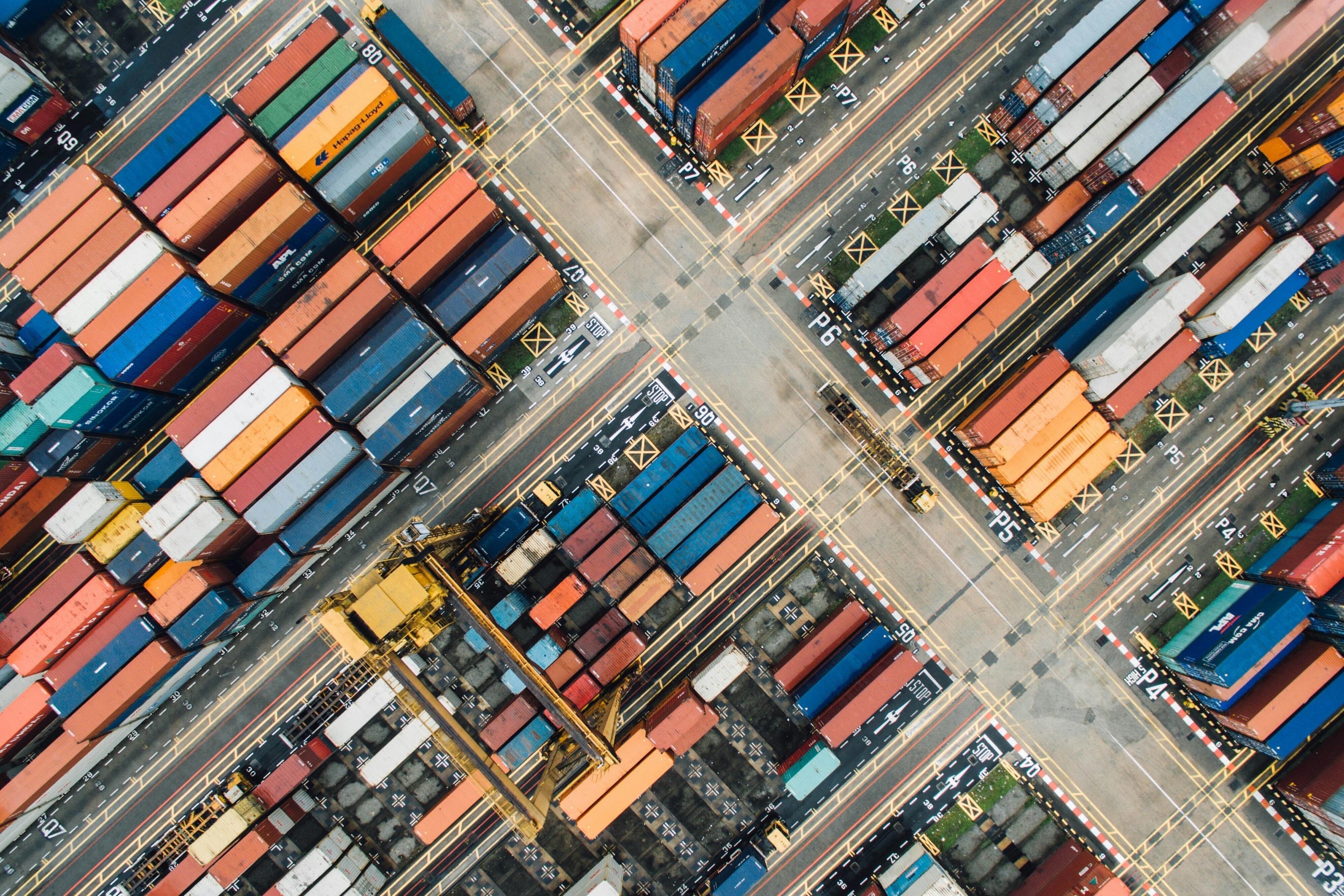
Are these five trends disrupting or driving logistics growth?
Ali Alwaleed Al-Thani and Sheikh Mohammed bin Hamad bin Faisal Al-Thani
May 24, 2024
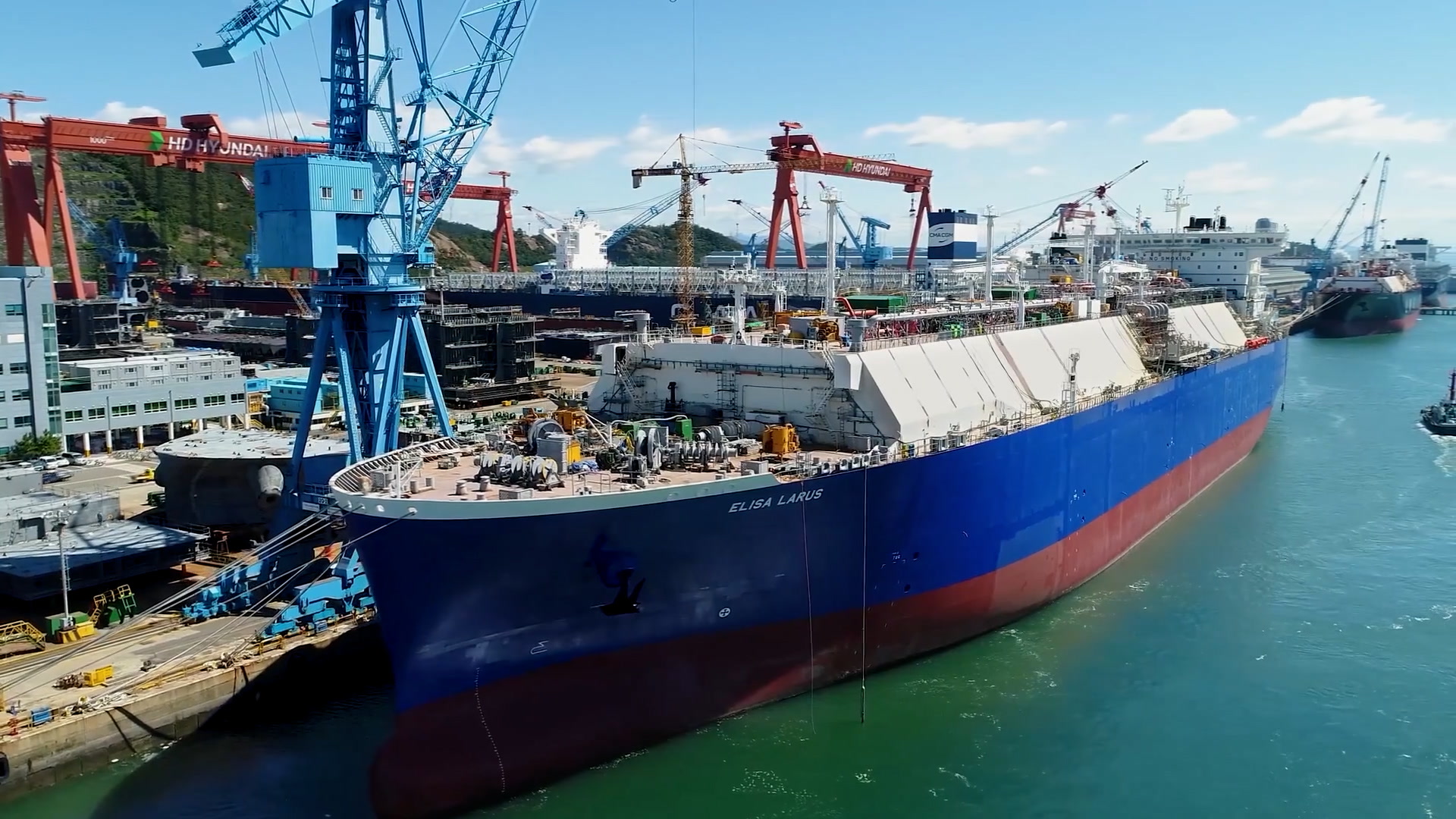
Here's how the metaverse is transforming ship design
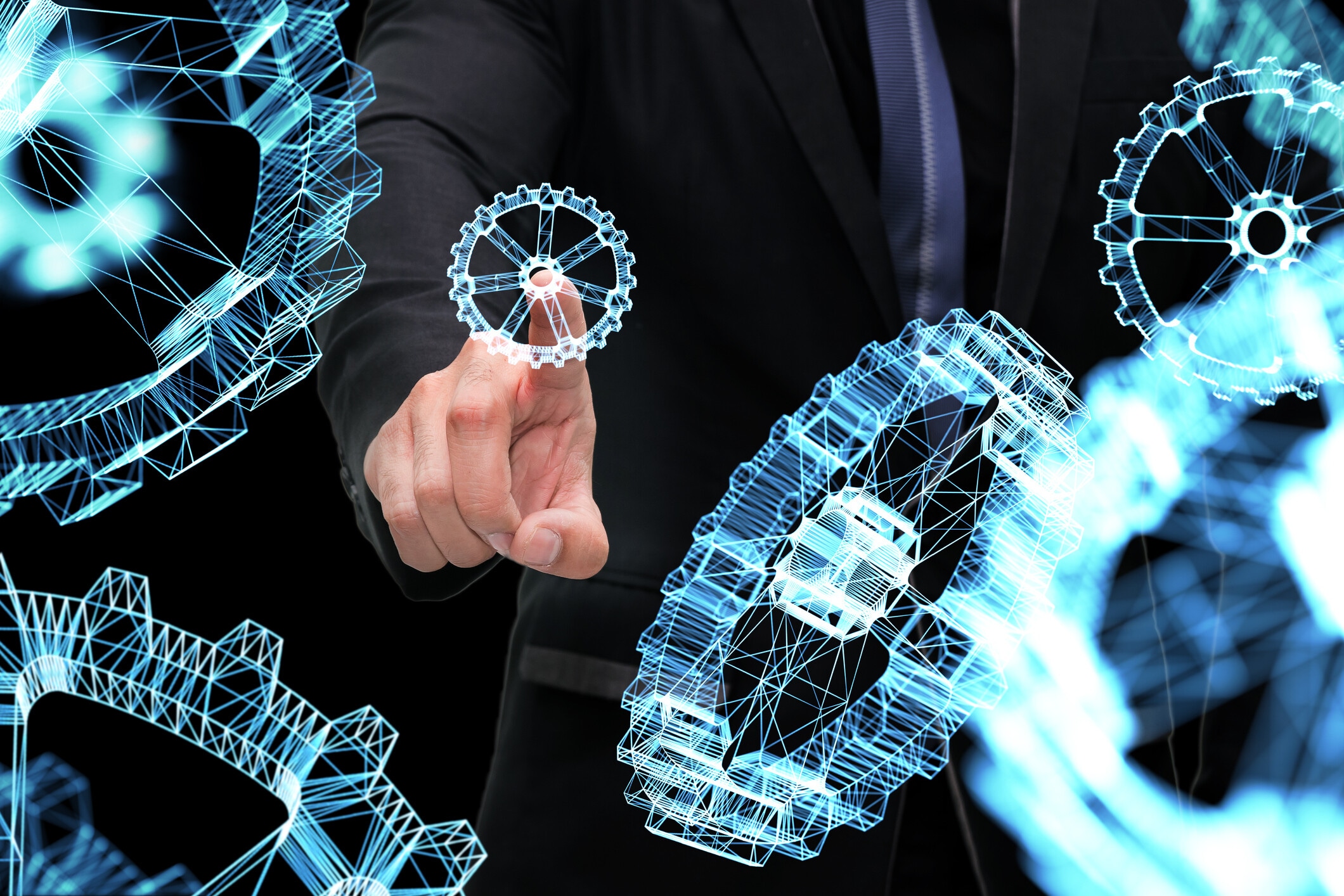
Digital spare parts: Manufacturing's solution to supply chain woes
Aleksander Ciszek and Devin Culham
April 25, 2024
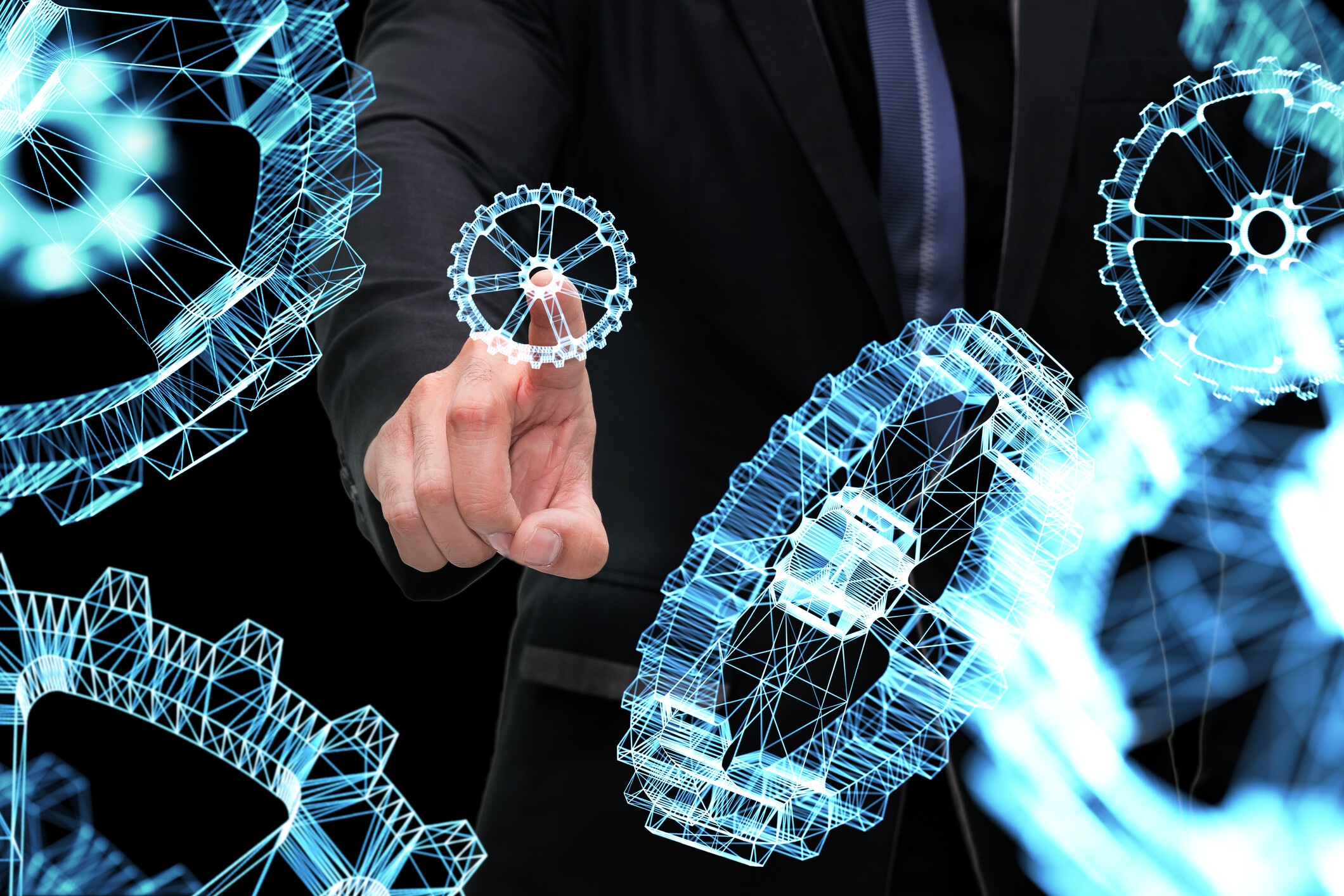
Why Large Language Models are the future of manufacturing
Andre Yoon and Kyoung Yeon Kim
April 23, 2024
Data and Analytics Case Study
Made possible by ey, exclusive global insights case study sponsor.
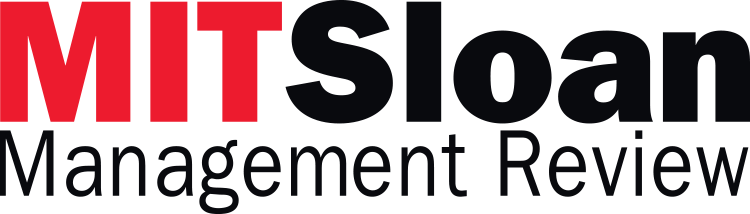
GE’s Big Bet on Data and Analytics
Seeking opportunities in the internet of things, ge expands into industrial analytics., february 18, 2016, by: laura winig.
If software experts truly knew what Jeff Immelt and GE Digital were doing, there’s no other software company on the planet where they would rather be. –Bill Ruh, CEO of GE Digital and CDO for GE
In September 2015, multinational conglomerate General Electric (GE) launched an ad campaign featuring a recent college graduate, Owen, excitedly breaking the news to his parents and friends that he has just landed a computer programming job — with GE. Owen tries to tell them that he will be writing code to help machines communicate, but they’re puzzled; after all, GE isn’t exactly known for its software. In one ad, his friends feign excitement, while in another, his father implies Owen may not be macho enough to work at the storied industrial manufacturing company.
Owen's Hammer
Ge's ad campaign aimed at millennials emphasizes its new digital direction..
The campaign was designed to recruit Millennials to join GE as Industrial Internet developers and remind them — using GE’s new watchwords, “The digital company. That’s also an industrial company.” — of GE’s massive digital transformation effort. GE has bet big on the Industrial Internet — the convergence of industrial machines, data, and the Internet (also referred to as the Internet of Things) — committing $1 billion to put sensors on gas turbines, jet engines, and other machines; connect them to the cloud; and analyze the resulting flow of data to identify ways to improve machine productivity and reliability. “GE has made significant investment in the Industrial Internet,” says Matthias Heilmann, Chief Digital Officer of GE Oil & Gas Digital Solutions. “It signals this is real, this is our future.”
While many software companies like SAP, Oracle, and Microsoft have traditionally been focused on providing technology for the back office, GE is leading the development of a new breed of operational technology (OT) that literally sits on top of industrial machinery.
About the Author
Laura Winig is a contributing editor to MIT Sloan Management Review .
1. Predix is a trademark of General Electric Company.
2. M. LaWell, “Building the Industrial Internet With GE,” IndustryWeek, October 5, 2015.
3. D. Floyer, “Defining and Sizing the Industrial Internet,” June 27, 2013, http://wikibon.org.
i. S. Higginbotham, “BP Teams Up With GE to Make Its Oil Wells Smart,” Fortune, July 8, 2015.
More Like This
Add a comment cancel reply.
You must sign in to post a comment. First time here? Sign up for a free account : Comment on articles and get access to many more articles.
Comment (1)
Home > Resources > Articles > From Concept to Use Cases: Big Data Analytics in Manufacturing
From Concept to Use Cases: Big Data Analytics in Manufacturing
#manufacturingtechnology.
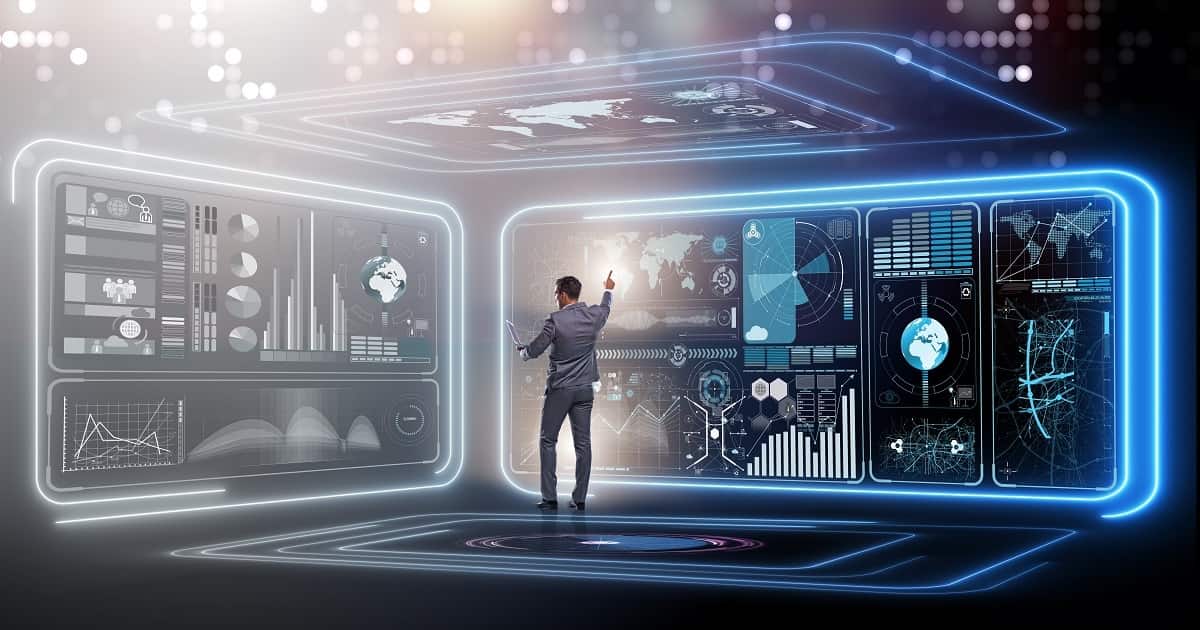
“Data is the new science. Big data holds the answers.” – Pat Gelsinger, CEO at VMware
Why Do Manufacturers Need Big Data?
- According to a KRC research report, 67% of manufacturing executives considered investing in manufacturing data analytics, especially in the face of pressure to minimize costs in this volatile market.
- According to MicroStrategy, 59% of organizations worldwide are using big data analytics.
Big Data: From the Past to the Future
Companies using big data analytics , big data in manufacturing: use cases, supply chain management, machine maintenance, daily production, quality assessment, is data science the same as big data, what purpose does data serve in manufacturing, how are manufacturing data defined.
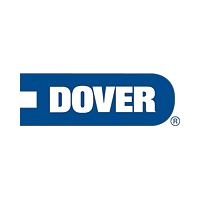
Dover Corporation
Dover is a diversified global manufacturer with annual revenue of approximately $7 billion. We deliver innovative equipment and components, specialty systems, consumable supplies, software and digital solutions, and support services through three operating segments: Engineered Systems, Fluids and Refrigeration & Food Equipment.
OTHER ARTICLES
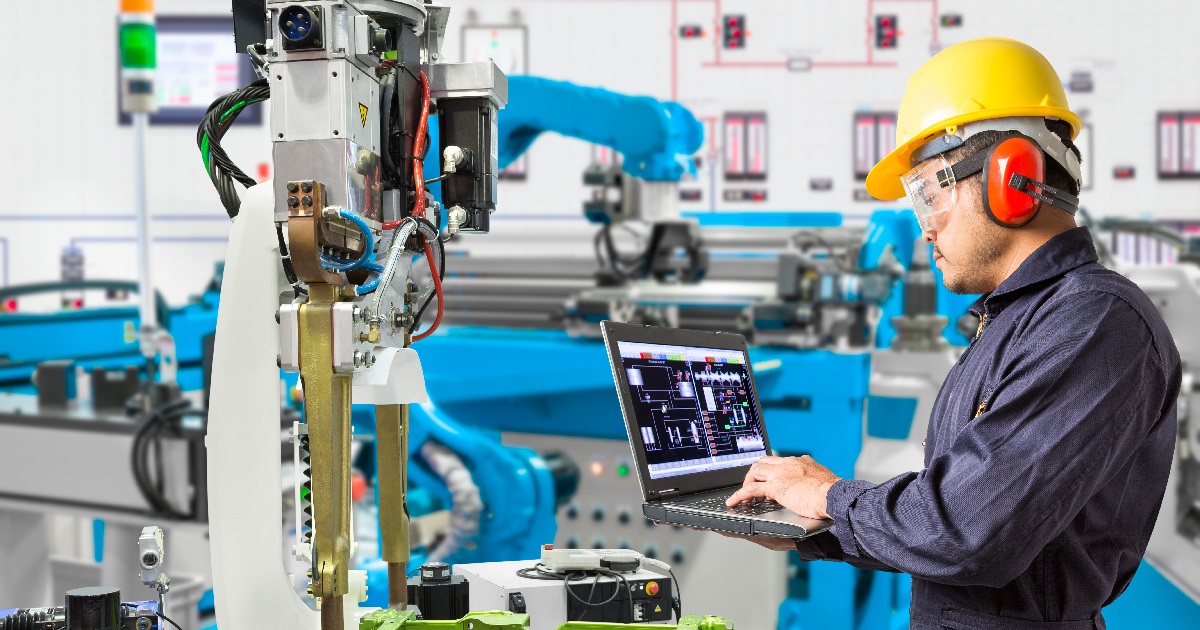
Digital Transformation
Leading bim software for construction management.
Article | March 28, 2024
Leverage advanced tools from renowned BIM software providers for construction management and engineering excellence. Gain newer levels of precision and efficiency with leading BIM software. Contents 1. Impact of BIM Technology on Architects and Engineers 2. Top BIM Tools for Construction Management 2.1. midas Civil 2.2. BEXEL Manager 2.3. GoRPM 2.4. Kairnial 2.5. Terabase 2.6. WIZZCAD 2.7. PROKON Structural Analysis and Design 2.8. Edificius 2.9. Allplan BIMplus 2.10. GeoHECRAS Solutions Inc 3. BIM Software Trends Shaping Construction Industry 1. Impact of BIM Technology on Architects and Engineers Building Information Modeling (BIM) represents a leap forward in designing complex building systems for engineers. BIM software for civil engineers facilitates a more detailed and accurate representation of structural components, HVAC systems, and other critical engineering aspects. This precision not only aids in the construction phase but also contributes to the building's long-term sustainability and efficiency. Through BIM, architects, structural engineers, and MEP (mechanical, electrical, and plumbing) engineers work concurrently on a single shared model. This synergy not only reduces the risk of errors and inconsistencies but also streamlines revisions and updates, ensuring all team members are always on the same page. Through detailed modeling, architects and engineers can predict and quantify materials, labor, and other costs more accurately, enabling more precise budgeting and cost management. Additionally, the BIM tool for construction management includes features like clash detection, which identifies and resolves conflicts in the design phase, thereby mitigating risks associated with construction errors and omissions. Through simulation tools integrated with the best BIM softwaresolutions, engineers can assess the impact of their designs on energy consumption, environmental sustainability, and occupant comfort, making informed decisions that align with green building principles. The impact of BIM technology on architects and engineers is profound and far-reaching. As BIM for construction continues to evolve, it will undoubtedly lead to more innovative, sustainable, and efficient building designs. 2. Top BIM Tools for Construction Management For harnessing the capabilities of BIM technology, selecting a powerful 3D modeling software is of paramount importance. Our curated list of top BIM software has been chosen to elevate construction management processes and bring innovation and efficiency to engineering projects. From BIM software solutions that streamline project delivery to BIM tools for construction management, the featured software products have been chosen for their proven benefits of BIM in enhancing project visualization, coordination, and execution. 2.1midas Civil MIDAS IT is a prominent player in the realm of civil, structural, and mechanical engineering software development. One of their flagship offerings is midas Civil, a powerful Finite Element Analysis software tailored for bridge analysis and design. Key Features Integrates sophisticated pre- and post-processing functionalities that streamline the modeling and analysis phases, enhancing the workflow efficiency for structural engineers. Quick solver accelerates the process of modeling and analyzing bridges. Parameter modification tools support parametric analysis. Conventional module provides intuitive wizards for quick modeling of both 2D and 3D conventional bridges. 2.2BEXEL Manager BEXEL Manager stands as a pioneering BIM solution, integrating advanced visualization, model management, and collaboration tools to optimize construction project delivery. One of its standout characteristics is its openBIM compatibility, supporting the IFC file format certified by buildingSMART International. This ensures seamless collaboration and data exchange across different software platforms. Key Features Advanced 3D visualization and model data management. Automated clash detection and quantity takeoff. Detailed cost estimation and intelligent scheduling. Comprehensive data analysis and reporting tools for informed decision-making. 2.3GoRPM The GoRPM (Geospatial Real Property Management) Enterprise Software Solution offers a robust platform for real property and facilities management, integrating asset data to generate actionable insights. A significant milestone was reached in November 2018 when R&K Solutions' Enterprise GoRPM Software as a Service achieved the ‘In Process’ status within the US Federal Risk and Authorization Management Program (FedRAMP). Key Features Empowers organizations by facilitating the collection, integration, and analysis of real property. Powerful data integration and business intelligence generation. Featured on the FedRAMP Marketplace for recognized compliance. Streamlines real property management and decision-making processes. 2.4Kairnial Kairnial facilitates project data management and collaboration, offering an integrated platform for managing BIM models, documents, and project communications. This innovative solution provides seamless access to an array of essential resources, all accessible through a suite of fully integrated modules that are compatible with web and mobile devices. Key Features Comprehensive suite for document, BIM management, and progress tracking. Modules for QSHE inspections, defects, and equipment management. Enhances project communication and collaboration across teams. Streamlines operations and decision-making throughout the asset lifecycle. 2.5Terabase Terabase is designed for development project management, fostering collaboration and optimizing project workflows. It stands out for its 3D project optimization and analytics, providing insights for data-driven decision-making. It is dedicated to simplifying the complexities of project development, reducing risks, and helping achieve success by providing a unified platform that fosters collaboration and informed decision-making. Key Features Centralized project data repository with real-time access. Enhanced collaboration tools for team communication. 3D visualization for project optimization and analysis. Technical support and guidance for overcoming project challenges. 2.6WIZZCAD WIZZCAD supports the digital transformation of construction projects through its BIM-native SaaS solution. It focuses on improving project outcomes across the building lifecycle, from design to operation. Its unified collaborative platform serves as the nucleus for managing the intricacies of design, construction, and operation, whether in 2D or 3D. Key Features Digitalization of business processes in design, construction, and operation. Unified collaborative platform for all project stakeholders. Offers tools for managing safety, quality, and environmental standards. Streamlines workflows and enhances project outcomes with digital tools. 2.7PROKON Structural Analysis and Design PROKON offers a suite of over forty specialized programs for structural analysis, design, and detailing. Established in 1989, it has become a trusted name globally for its modular and integrated approach. PROKON has garnered global recognition, serving users across over eighty countries. Key Features Comprehensive suite for structural engineering projects. Modular design with seamless integration among analysis, design, and detailing programs. Promotes accuracy and efficiency in workflow management. Trusted by professionals worldwide for tackling complex structural challenges. 2.8Edificius Edificius provides a versatile solution for architectural design, blending BIM technology with user-friendly tools for rendering and project presentation. It offers a wide range of options for custom Working Drawing layouts and effortless import/export capabilities in standard formats. Key Features Advanced BIM application with real-time rendering capabilities. Tools for landscaping and exterior design, alongside a vast library of resources. Regular updates to align with regulations and technological advancements. Real-time HD rendering and scene photomontage for high-quality presentations. 2.9Allplan BIMplus Allplan BIMplus is a leading open BIM platform, facilitating collaborative work across disciplines in the construction industry. It acts as a centralized hub for managing BIM model data, enhancing project transparency and efficiency. Key Features Centralized management of BIM model data, documents, and tasks. Facilitates cross-disciplinary collaboration and optimizes building lifecycle management. Streamlines communication and project execution among stakeholders. Empowers professionals to manage projects from inception to maintenance. 2.10GeoHECRAS GeoHECRAS accelerates river modeling projects by enhancing the HEC-RAS process with an intuitive 2D/3D interface. It integrates with major CAD and GIS platforms, offering flexibility and efficiency in hydraulic analyses. Key Features User-friendly interface for HEC-RAS model creation and visualization. Interoperability with AutoCAD, MicroStation, and ESRI ArcGIS. Streamlines the analysis of hydraulic structures and floodplain encroachments. Provides comprehensive tools for efficient and accurate water surface profile computations. 3. BIM Software Trends Shaping Construction Industry The adoption rates of BIM are soaring, driven by its proven value in project optimization. A notable uptick in BIM utilization across Europe, as reported by USP’s Architectural Barometer, underscores the growing recognition of BIM's potential to improve project quality, reduce timeframes, and facilitate better clash detection, among other benefits. Here, we delve into the key emerging trends that are setting the stage for the future of BIM, offering insights for decision makers to stay ahead in the AEC industry. Cloud-Based BIM: Cloud technology has revolutionized BIM by enabling real-time collaboration, access, and updates. This advancement facilitates seamless integration of products into BIM models and enhances project management efficiency by ensuring all stakeholders are working with the most current data. The trend toward cloud-based BIM is likely to accelerate, breaking down barriers to collaboration and information sharing within the AEC industry. Prefabrication and BIM: The synergy between prefabrication and BIM is reshaping construction processes, making them faster, more efficient, and less prone to errors. By facilitating the creation of digital models that can be directly integrated into the overall project, BIM is instrumental in streamlining the prefabrication process, from design through to assembly. The Rise of Automation: Automation, powered by AI and robotics, is transforming BIM processes, making them more efficient and less labor-intensive. This trend is about enhancing productivity and improving safety on construction sites, besides enabling more flexible and adaptive production methods. AI and VR: Artificial intelligence (AI) and virtual reality (VR) technologies are revolutionizing the way BIM is utilized for design and project interaction. AI's data processing capabilities, combined with VR's immersive experience, are enhancing visualization, improving design accuracy, and facilitating more effective stakeholder communication. Digital Twins: Digital twins represent a leap forward in how the AEC industry approaches design and simulation. By creating virtual replicas of physical assets, digital twins offer unparalleled insights into the performance and operational dynamics of projects, allowing for preemptive maintenance, optimized performance, and informed decision-making. This trend is poised to redefine the parameters of design excellence and operational efficiency. Blockchain for Enhanced Security: As BIM involves handling vast amounts of sensitive data, security is paramount. Blockchain technology offers a robust solution, ensuring the integrity, traceability, and security of BIM data, thereby mitigating the risk of data breaches and unauthorized access. IoT Integration: The Internet of Things (IoT) is elevating BIM's potential to deliver smarter, more efficient buildings. By embedding IoT security sensors in construction projects, stakeholders can monitor performance in real-time, leading to optimized maintenance schedules, improved energy efficiency, and ultimately, smarter building management. Sustainability: With a growing emphasis on sustainability, BIM is increasingly being used to model energy consumption and evaluate green technology integrations. This trend is critical for designing buildings that are not only efficient but also environmentally responsible, aligning with global sustainability goals. Mandatory BIM Compliance: Governments and regulatory bodies worldwide are increasingly mandating BIM for public projects, recognizing its significant benefits in project management efficiency and overall quality. This shift toward compulsory BIM integration is not solely driven by the aim to harness technological potential but also by a broader objective to enhance national competitiveness and address socio-economic and environmental challenges. For manufacturers and AEC professionals, staying abreast of these mandates is crucial for remaining competitive and meeting market demands effectively. The AEC industry stands at the cusp of a technological renaissance, with BIM at its core. Incorporating the best BIM software can significantly elevate project outcomes. For decision makers, understanding and leveraging these emerging trends is essential for driving innovation, enhancing competitiveness, and delivering projects that meet the shifting demands of the modern construction world.
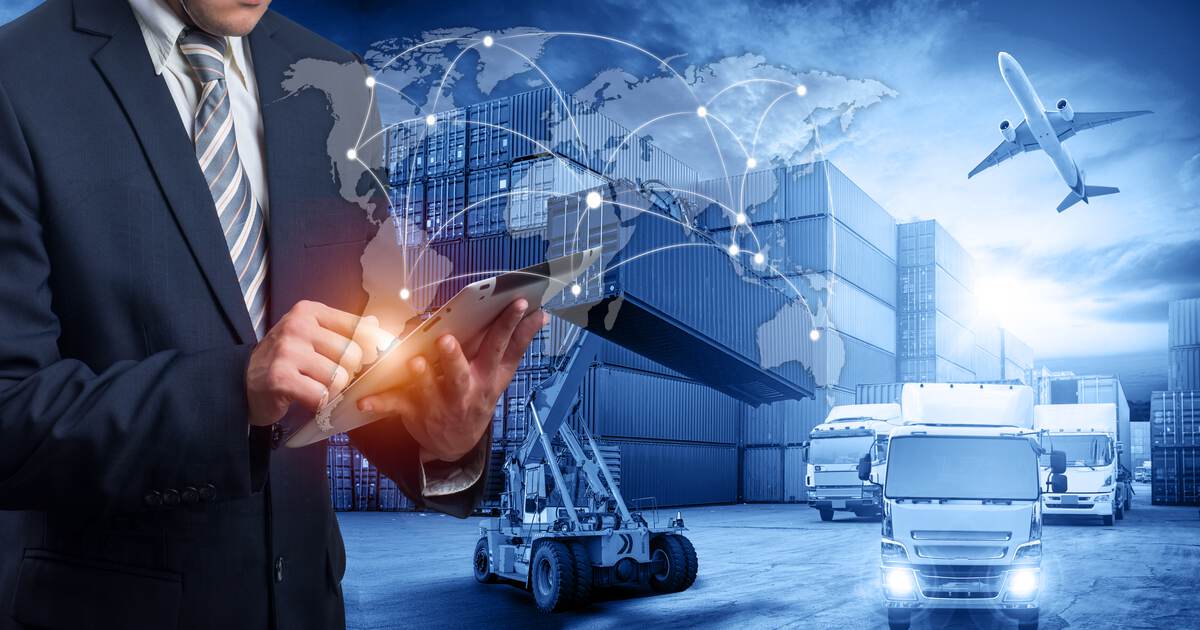
Best BIM Software For Design Excellence and Engineering
Article | March 27, 2024
Explore the transformative benefits of the best BIM software. Know how BIM software helps achieve architectural excellence with a list of top BIM companies worldwide. Contents 1. BIM Technology in Designing & Architecture 2. Advantages of BIM Implementation 3. Leading Companies Providing BIM Solutions 3.1. GraphiSoft 3.2. Hexagon AB 3.3. Vectorworks 3.4. Newforma 3.5. CADMATIC 3.6. Reconstruct Inc 3.7. ZWSOFT 3.8. TestFit 3.9. cove.tool 3.10. Transoft Solutions Inc 4. Tomorrow’s Architecture: Growing Impact of BIM on AEC Industry 1. BIM Technology in Designing & Architecture Building Information Modeling is redefining project management, design efficiency, and collaboration within the Architecture, Engineering, and Construction industry. For an accurate evaluation of building performance during the initial design and preconstruction stages, it's crucial to have a detailed collection of data concerning the structure's design, materials, environment, and systems. BIM facilitates the integration of diverse information within a single model, enabling the seamless inclusion of sustainability practices throughout the design phase. As defined by both the National Building Information Modeling Standards Committee and the Associated General Contractors of America, BIM encompasses more than three-dimensional modeling. It integrates intelligent, data-rich elements into a cohesive model that supports a myriad of uses from initial planning to demolition. The versatility of BIM enables stakeholders to engage in a more integrated project delivery (IPD), optimizing efficiency and reducing waste across all project phases. This shift toward a collaborative construction process is instrumental in overcoming traditional adversarial relationships within the industry. Moreover, BIM's role in supporting sustainable design and construction is paramount, offering a platform for analyzing building performance early in the design phase and throughout the building's lifecycle. However, the adoption of BIM is not without challenges. The technology and process-related risks, including interoperability issues, lack of standardized protocols, and the learning curve associated with its implementation pose significant barriers. Legal and contractual considerations, particularly regarding data ownership and the accuracy of BIM data, also present hurdles that need to be addressed for broader implementation. Despite these challenges, the benefits of BIM, including improved project visualization, enhanced collaboration, and the potential for cost and time savings, are driving its adoption across the AEC industry. The advancement of BIM technologies and methodologies, together with the establishment of industry standards and the incorporation of BIM into educational programs, indicates a bright future for its application beyond traditional sectors. Through an in-depth examination of the best BIM software, the benefits of its implementation, and the transformative impact it has on construction, architecture, and engineering, readers can gain insights into the strategic advantages of BIM. 2. Advantages of BIM Implementation Since its inception in the mid-1980s, BIM has steadily evolved, gaining global recognition and adoption. Its ability to streamline the design and construction process has not only improved project outcomes, but also significantly increased the profitability of investments in the construction industry. A comprehensive analysis reveals compelling statistics that underscore the value BIM brings to construction projects: According to a McGraw Hill report, an overwhelming majority of Architectural BIM Modeling users have experienced positive ROI, with 83% of expert users reporting significant returns. The contractors most highly engaged with BIM cited improved process outcomes (77%) and improved productivity of personnel (43%) more frequently than those on the lowest engagement (47% and 34%, respectively) tier. This is a natural outcome of their extension of BIM benefits beyond improved visualization into substantial business benefits related to workflow efficiency, improved utilization of resources and better project outcomes. [Source: SmartMarket Report] 2.1 BIM's Strategic Advantages for Construction Projects The strategic advantages of BIM extend beyond individual project savings to encompass broader industry benefits offered by the best BIM software, such as: Cost Reduction: BIM's precision and efficiency in planning and execution significantly reduce unnecessary expenditures and resource wastage. Time Savings: Enhanced coordination and clash detection capabilities streamline the construction process, saving valuable time. Quality Improvement: BIM facilitates a higher standard of construction quality, reducing the likelihood of errors and rework. 2.2 How BIM Software Helps: Case Studies Demonstrating BIM's Value Excelize reported some stunning statistics and case studies demonstrating the real-world impact of BIM through specific project examples, showcasing how BIM technology delivers ROI, drives cost savings, enhances efficiency, and improves project timelines: University of Colorado, Denver Health Sciences Centre: Achieved a 74% reduction in construction RFIs during the foundation phase and a 47% reduction during the steel erection phase, resulting in a project that was completed two months ahead of schedule. Sutter Health Medical Centre, Castro Valley: Saved over USD 1.2 million on a USD 320 million project, highlighting BIM's potential for substantial financial savings. Department of Energy, US: Detected approximately 500 serious problems early on, saving USD 10 million on a USD 100 million project through effective clash detection. Aquarium Hilton Garden Inn, Atlanta: Identified 55 clashes in the early design stage, resulting in an estimated cost saving of USD 800,000. 2.3 Economic Advantage with Advanced Planning The best BIM software embodies a model-based stratagem, instrumental in the meticulous orchestration of buildings and infrastructures' planning, design, and management by the construction industry's maestros. BIM integration for construction gives an edge with advanced planning in multiple ways: Empowered Collaboration: BIM stands as a pillar of real-time information exchange, fostering a culture of transparency and minimizing the shadows of misunderstandings or errors. Project Visualization: With the power to peer into the project's heart in 3D, stakeholders are equipped to make enlightened decisions, sculpting the future with precision. Elevated Efficiency: BIM's clairvoyance identifies potential snags early, conserving both the clock's ticks and treasure troves that might otherwise vanish in the correction of construction missteps. Streamlined Project Management: BIM's comprehensive nature orchestrates a symphony of effective project management, harmonizing the chords of scheduling, resource allocation, and risk management. 2.4 Optimizing Resource Management through BIM The implementation of BIM technology brings a radical improvement in resource utilization across construction projects. By leveraging BIM, stakeholders gain invaluable insights, resulting in: Enhanced Resource Allocation: BIM's detailed models ensure optimal use of materials, labor, and time, preventing waste and ensuring projects stay within budget. Precision in Materials Management: Through accurate 3D models, BIM facilitates exact material specifications and quantities, reducing surplus and shortages. Risk Reduction in Project Execution: By identifying potential conflicts and issues in the virtual environment, BIM minimizes on-site errors and delays. Harmonized Workflow Management: BIM enables a synchronized approach to project tasks, aligning timelines and resources for smoother execution. 2.5 Facilitating Sustainable and Green Building through BIM BIM's capability extends to enhancing the sustainability quotient of construction projects, offering: Sustainability Analysis: Early-stage BIM analysis helps integrate sustainable design principles, promote energy efficiency, and reduce carbon footprint. Lifecycle Environmental Impact Assessment: BIM allows for the assessment of environmental impact over a building's lifecycle, encouraging sustainable material and design choices. Efficient Energy Modeling: With BIM, stakeholders can simulate and analyze energy consumption patterns, facilitating the design of energy-efficient buildings. Green Certification Facilitation: BIM simplifies the process of obtaining green building certifications by providing detailed documentation and compliance analysis. 2.6 Transforming Facility Management with BIM Post-construction, BIM continues to deliver value by transforming how buildings are managed, through: Streamlined Asset Management: BIM provides detailed information about every component of a building, making asset management more efficient. Proactive Maintenance Scheduling: Access to detailed building data allows for predictive maintenance, ensuring the longevity and performance of building systems. Enhanced Space Utilization: BIM aids in optimizing space usage, improving facility planning and management. Integration with IoT for Smart Buildings: BIM models can integrate with IoT devices, enabling real-time monitoring and control for smarter facility management. 2.7 Advancing Project Delivery with IPD and BIM The synergy between BIM and IPD methodologies significantly advances project delivery by: Fostering Collaborative Project Environments: BIM and IPD together promote a culture of collaboration among all project participants, leading to more successful project outcomes. Reducing Legal and Financial Risks: By clarifying roles and expectations, BIM and IPD minimize disputes and financial uncertainties. Enhancing Project Transparency: The combined approach ensures all stakeholders have access to up-to-date project information, fostering trust and transparency. Accelerating Project Timelines: Integrated workflows and real-time data sharing lead to faster decision-making and project completion. 2.8 Boosting Innovation in Construction Adopting BIM propels firms into new heights of competitiveness and innovation, through: Driving Adoption of Tech: BIM encourages the use of advanced technologies, such as AR/VR, for immersive design and planning experiences. Catalyzing Process Innovation: The BIM process stimulates the development of new construction methodologies, improving overall industry standards. Enhancing Market Position: Companies proficient in BIM are viewed as industry leaders, attracting more clients and projects. Empowering Knowledge Transfer: BIM facilitates the sharing of knowledge and best practices, elevating the capabilities of the construction sector as a whole. At its core, this digital artisan collects data, rendering a tangible prelude of buildings and infrastructures across various lifecycle stages. The realm of BIM software unfurls a panorama of benefits as discussed above, enriching the entire building project lifecycle within the construction process and enhancing building performance post-handover. 3. Leading Companies Providing BIM Solutions In the galaxy of BIM innovation, several BIM solution companies shine with unparalleled brilliance, guiding the AEC sector toward a future where efficiency, collaboration, and sustainability are not merely aspirations, but tangible realities. These vanguards, with their commitment to advancing BIM technology, are participants in the industry's transformation; they are its architects, crafting the edifice of tomorrow's construction. 3.1GraphiSoft GraphiSoft has established itself as an ingenious force in BIM software development, particularly with its flagship product, Archicad. Archicad has been recognized as the BIM software of choice among architects, offering a comprehensive suite for design and documentation workflows. The company also provides BIMx, a popular app for mobile and web platforms, facilitating an immersive BIM experience for all project stakeholders. Graphisoft's BIMcloud represents a significant advancement in cloud-based collaboration, enabling real-time teamwork across the globe. As a part of the Nemetschek Group, Graphisoft continues to be at the forefront of technological innovation in the AEC industry, demonstrating the transformative benefits of BIM technology in designing and architecture. 3.2Hexagon AB Hexagon AB is playing a notable role in the digital transformation of the AEC industry. The company's commitment to empowering a sustainable and autonomous future is evident through its digital reality solutions, which enhance productivity, quality, and safety. Hexagon's technologies promote interconnected and autonomous ecosystems, ensuring scalable and sustainable development. The company's impact on BIM in modern construction is profound, as it leverages data to optimize processes and improve decision-making, embodying the advantages of BIM implementation in construction management and the broader industry. 3.3 Vectorworks Vectorworks, as a global leader in design and BIM software, serves over 650,000 professionals in architecture, landscape, and entertainment. Since its inception in 1985, Vectorworks has been dedicated to supporting designers' creative processes while fostering innovation. The software's capability to construct data-rich, visual models without compromising the design integrity exemplifies the top benefits of BIM technology. Moreover, Vectorworks promotes collaboration among teams, enhancing project outcomes throughout the lifecycle, and demonstrating the importance of BIM in construction and design. 3.4 Newforma Newforma addresses the critical need for information management and collaboration in the AECO sector by providing platforms that streamline communication, administration, and real-time collaboration. With over 500,000 users worldwide, Newforma's solutions have significantly improved project outcomes, highlighting the advantages of BIM in construction management. The company's platforms support the entire project lifecycle, underscoring the critical role of effective information management and collaboration in leveraging BIM benefits in construction. 3.5 CADMATIC CADMATIC is passionate about leveraging digital and intelligent design solutions to enhance the AEC and marine industries. The company's mission to make design, engineering, construction, and operation processes better, faster, and easier is aligned with the core benefits of BIM. CADMATIC's support for digitalization across project life cycles embodies the transformative power of BIM, showcasing its role in reducing costs, shortening project timelines, and improving design quality. Through technology that facilitates remote work and supports construction sites, CADMATIC exemplifies the significant advantages of BIM implementation in the modern construction landscape. 3.6 Reconstruct Inc Reconstruct’s ‘Visual Command Center,’ is a platform offering Remote Quality Control & Measurable Progress Monitoring for the real estate sector. It enables stakeholders to track their portfolio globally, aligning reality with design to sub-inch accuracy. By overlaying actual construction progress over planned designs, Reconstruct ensures that stakeholders are always informed about the current state of their projects compared to expected outcomes. This level of precision and transparency is crucial for maintaining project timelines and budgets, showcasing Reconstruct’s innovative approach to construction management. 3.7 ZWSOFT ZWSOFT is committed to offering all-in-one CAx solutions that meet the design and engineering needs of professionals worldwide. With a focus on affordability and reliability, ZWSOFT has attracted over 1.4 million customers from more than 90 countries since its inception in 1998. Their products cater to a wide range of industries, demonstrating their versatility and commitment to quality. ZWSOFT's growth, even through challenging times, is a testament to their dedication to meeting the diverse needs of designers and engineers with innovative solutions. 3.8 TestFit TestFit is revolutionizing real estate feasibility with its platform, enabling rapid site planning for developers, architects, and contractors. By automating tedious tasks like parking counts, design drafting, and yield calculations, TestFit saves valuable time and reduces risk in deal acquisition. Their AI configurators optimize design solutions, providing instant insights into design, constructability, and cost. This level of innovation streamlines the site planning process, making TestFit a crucial tool for maximizing site potential and accelerating project timelines. 3.9 cove.tool cove.tool is the first cloud-based network of tools to create interconnectivity between all teams working in the design and preconstruction cycle. It reduces risk, boosts transparency, and maximizes productivity, saving time and money for those in the AEC sector, while building a better, sustainable future. Building product manufacturers can maximize revenue with revgen.tool by seamlessly integrating building products into cove.tool's ecosystem of building performance analysis tools. This allows them to easily showcase real-time, verified product performance data to design teams earlier in the process, building trust among these teams. But it doesn't stop there. cove.tool's suite of products (analysis.tool, loadmodeling.tool, and drawing.tool) integrates with workflow to meet the needs of each role in the AEC space while improving the overall project and process. 3.10 Transoft Solutions Inc Since 1991, Transoft Solutions has developed advanced and highly specialized software solutions for aviation, civil infrastructure, transportation and operational professionals. Its portfolio of planning, simulation, modeling, design, and road safety analysis solutions is used every day by thousands of organizations in over 150 countries worldwide. Clients include government agencies at the federal, state, county and municipal levels, as well as major international airports and top engineering and architectural design firms. 4. Tomorrow’s Architecture: Growing Impact of BIM on AEC Industry The evolution of BIM signals a future where digital precision meets architectural ambition, fundamentally altering the lifecycle of buildings from their inception to demolition. By enabling detailed simulations and analyses, BIM supports the creation of buildings that are not only aesthetically pleasing, but also energy-efficient and resource-conscious. This shift toward sustainable design is critical for addressing the pressing challenges of climate change and resource depletion, positioning BIM as a crucial tool for future-proofing our built environment. By facilitating a more integrated approach to design and construction, BIM allows for the exploration of novel materials and construction methods that can lead to more durable, resilient, and adaptable structures. This capability is essential for meeting the diverse needs of tomorrow's cities and populations, ensuring that buildings can evolve in response to changing uses and environmental conditions. Moreover, BIM's role in the AEC industry is set to expand further with the integration of smart manufacturing technologies such as artificial intelligence, the internet of things, and augmented reality. These BIM software technologies have the potential to revolutionize the design and construction process, offering unprecedented levels of precision, efficiency, and interactivity. This convergence of digital technologies paves the way for smarter, more connected buildings that can adapt to their occupants' needs, enhancing comfort, safety, and productivity. The question isn’t why BIM, it is why not BIM for creating a legacy of intelligent, sustainable, and human-centric architecture that will define the built environment for generations to come.
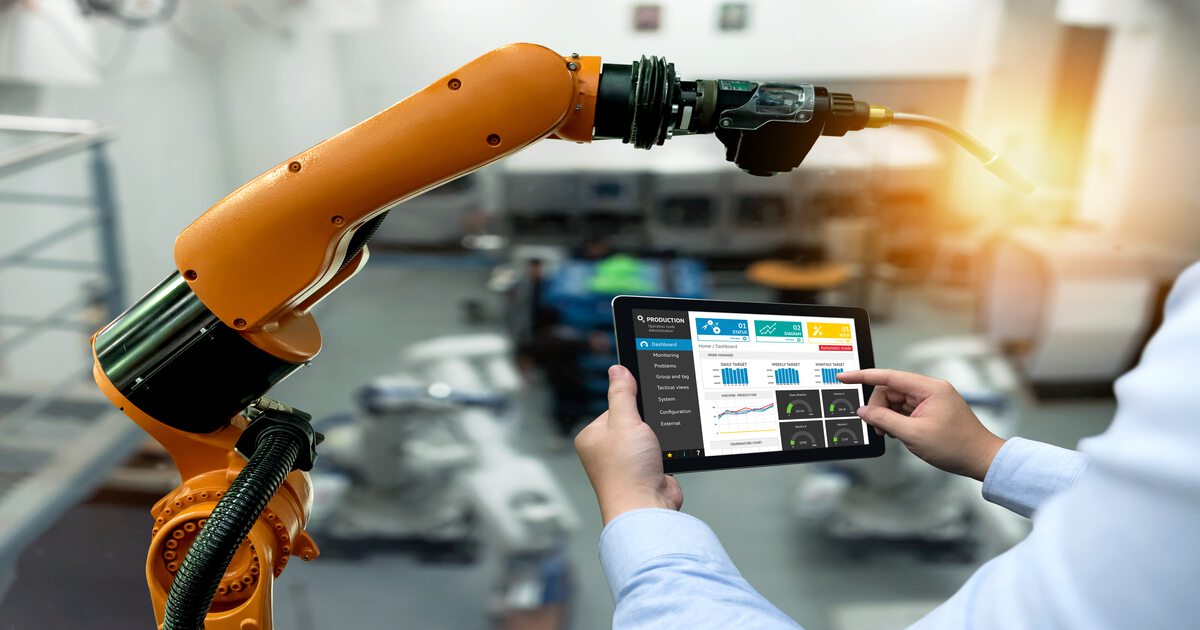
3D Printing
The future of production: best 3d printing software unveiled.
Article | March 15, 2024
Discover precision & innovation with the best 3D printing software—where designs come to life effortlessly. Know how 3D printing software applications are revolutionizing production lines. Contents 1. The 3D Printing Revolution 2. Expanding Horizons with Additive Manufacturing 3. How Advanced Software is Transforming Production 4. Featured Companies: Leading Solutions in 3D Printing 5. Future Prospects 1. The 3D Printing Revolution From the intricate algorithms of slicer software to the sophisticated functionalities of CAD integrations, the 3D printing revolution continues to set new benchmarks in manufacturing and design. Additive manufacturing, also known as 3D printing, is shifting paradigms across a multitude of industries. Additive manufacturing in the aerospaceindustry,for instance, is being utilized for making rocket components and parts of astronaut suits. In defense, AM is streamlining production of various equipment like bullets, tanks, and bunkers, enhancing efficiency and quality while reducing costs. Metal 3D printing is leading to producing large car parts quickly and affordably. There are companies that have already introduced laser techniques to revolutionize manufacturing by establishing low-cost print depots for on-demand part production, enhancing supply chain resilience. We all can collectively agree that the innovative AM approach, characterized by its layer-by-layer fabrication method, transcended its initial applications of prototyping and hobbyist projects decades ago, evolving into a core manufacturing process capable of producing durable, safe, and complex products in varying quantities. The essence of additive manufacturing lies in its unmatched flexibility, enabling not only the customization of goods at an unprecedented scale, but also the optimization of production processes to eliminate traditional constraints such as assembly requirements and inventory management. 3D printing market is forecast by Hubs, a marketplace for manufacturing services, to almost triple in size by 2026, with a value of $44.5bn. Construction is one of the growth areas. [Source: Guardian] The development of new materials and advancements in printer technologies are broadening the applications of 3D printing, enabling its adoption in sectors ranging from construction to electronics and even healthcare. The potential for creating more complex, functional, and integrated products is expanding, paving the way for innovations for manufacturers. The integration of advanced 3D printing solutions, from sophisticated 3D printing software applications to state-of-the-art 3D printer design software, is redefining what's possible in engineering and manufacturing. 2. Expanding Horizons with Additive Manufacturing The concept of 3D printing acting as a global savior is not new, yet current developments suggest the enthusiasm can finally be justified. Though not all advancements will have immediate effects on our lives, the remarkable progress in 3D printing renders the technology truly captivating. Here are some notable innovations that reflect the kind of groundbreaking work being done through 3D printing technology: 3D Printed Mosque: Constructed through collaboration between Fursan Real Estate Company and China’s Guanli, specializing in 3D printing for construction, a 5,600 square-meter 3D printed mosque aligns with Saudi Vision 2030 of reducing construction waste. 3D Printed Living Tissue for Ear Reconstruction: In a significant advancement in regenerative medicine, scientists have successfully used 3D printing in a ear transplant case. A woman's ear was 3D printed using living tissue. This approach could revolutionize treatments for a variety of tissue and organ repairs. 3D Modeling in Orthopaedic Surgery: Within the realm of orthopaedic surgery, the amalgamation of advanced Tissue Engineering and Regenerative Medicine sciences and the burgeoning interest in 3D modeling and printing applications is prompting a growing cohort of surgeons to integrate these technologies into current clinical applications and research is also going on in the area of treatment of long bone defects. This convergence represents a significant stride in the field, allowing for enhanced surgical planning and precise execution. Innovative Space Construction: Icon, a 3D printing firm based in Austin, Texas, was granted $57.2 million by NASA in December 2022 to further its research and development endeavors aimed at facilitating the construction of structures on the moon's desolate terrain. That builds upon their Project Olympus, aiming for humanity's first off-Earth construction, vital for sustainable space exploration via NASA's Artemis program and opens newer horizons for 3D printed construction on the moon. Customization in Automotive Manufacturing: Several examples of leveraging 3D printing in the automotive industry have emerged in the last two decades. Porsche has been employing sports car seating concept by employing 3D printing and lattice design, featuring customizable polyurethane cushion components in hard, medium, and soft firmness levels. Inspired by motorsport, Porsche aims for driver-specific seat fitting, with plans to further personalize seats based on individual body shapes using 3D printing technology, offering unparalleled customization. Disaster-resilient 3D Printed House: The Indian Army inaugurated a 3D printed dwelling unit in December 2022, designed to withstand disasters and meet earthquake and green building standards. Located in Ahmedabad Cantt, the innovative structure marked the Army's first 3D printed house for soldiers. 3. How Advanced Software is Transforming Production Organizations are now faced with the imperative to reevaluate their operational strategies in light of the opportunities presented by additive manufacturing. This involves a comprehensive analysis of how products are designed, where and how they are produced, and the manner in which these processes can be optimized to leverage the benefits of 3D printing software. A new metal 3D printing technology could revolutionize the way large industrial products like planes and cars are made, reducing the cost and carbon footprint of mass manufacturing. [Source: Axios] There are several such use cases and citations on how strategic implications of leveraging advanced 3D printing applications extend into the commercial ecosystem, necessitating a rethinking of supply chain logistics, distribution networks, and even customer engagement models to fully capitalize on this technology's potential. 3.1 Advancing Prototyping Efficiency with 3D Printing 3D printing revolutionizes prototyping compared to traditional manufacturing methods like precision grinding, CNC milling, laser cutting, and turning, as it swiftly transforms designs into physical objects at a fraction of the time and cost. With its low investment and rapid production, top end 3D printing software are particularly prized for prototyping, enabling manufacturers to showcase complex concepts and receive immediate feedback on design and functionality. 3.2 Elevating Aerospace Manufacturing Amidst a transformative era in production, GE Aerospace, a division of General Electric (GE), recently earmarked over $650 million to enhance its global manufacturing and supply chain capabilities, emphasizing the pivotal role of 3D printing in aerospace advancement. Investing substantially in scaling its 3D printing-enabled LEAP engines, used in Airbus, Boeing, and COMAC aircraft, GE Aerospace aims to meet surging demand from commercial and defense sectors. 3.3 Revolutionizing Reef Conservation Innovative efforts in marine habitat restoration are underway with the deployment of 3D printed artificial reefs off the coast of Destin-Fort Walton Beach, Florida. Collaborating with reefmaker Walter Marine and 3D printing company 1Print, the project pioneers the use of 3D printing for artificial reef construction. This venture showcases the potential of 3D printing in marine conservation, offering scalable solutions for habitat restoration while addressing challenges related to durability and ecological integration. 3.4 Custom Tooling with 3D Printing 3D printing custom tooling represents one of the earliest and most widely adopted applications of this technology. Volkswagen has embraced 3D printed custom tooling since 2014, utilizing it to manufacture components for their assembly line. This approach eliminates the need for ordering specialized tools, drastically reducing tool development costs by 95%. Similarly, General Motors and Ford have reported significant cost reductions, with Ford claiming up to a 1000% cost reduction since 2015. Also, beer manufacturer Heineken has utilized 3D printing to bring down tool costs by 70% in Spain, demonstrating the versatility and cost-saving potential of 3D printing techniques for various industries. 3.5 3D Printing Reshaping Air Force The US Air Force has leveraged metal additive manufacturing to produce critical parts for the GE F110 engine, driving the F-16 jet fighter. With many aircraft models dating back decades, sourcing spare parts has been a daunting task. However, 3D printing enables the creation of new and customized spare parts, facilitating the maintenance and reuse of aging equipment. 3.6 Optimizing Weapon Systems Rapid manufacturing holds significant promise within the defense industry. It offers versatile applications, including the creation and customization of weapons and their components, as well as maintenance and production of spare parts. Through topology optimization, military weapons produced via 3D printing can achieve unparalleled performance. This process maximizes part efficiency by using minimal material, resulting in lighter yet stronger components. Reduced weight enhances mobility, handling, and speed, ensuring superior performance on the battlefield. In conclusion, the integration of advanced software with 3D printing techniques is reshaping various sectors. To explore further advancements in additive manufacturing and leverage its full potential, companies are increasingly turning to top-tier 3D printing software applications. Let’s delve further into the 3D printing revolution and potential of 3D printing engineering with a deep dive into advanced 3D printing solutions and the best 3D printing service providers. 4. Featured Companies: Leading Solutions in 3D Printing Companies leading in 3D printing are not just providing advanced manufacturing solutions; they are reshaping the very fabric of industry, driving toward a more agile, sustainable, and customized manufacturing era. Here is a list of some of the best 3D printing software with their features: 4.1 UltiMaker UltiMaker is a global leader in 3D printing, focused on shaping the future of manufacturing and product development. With a wide range of cutting-edge 3D printing solutions, including the popular S and Method series, and an extensive portfolio of 3D printing materials, UltiMaker is paving the way for innovation across industries. As part of its dedication to advancing education, UltiMaker also offers the MakerBot sub-brand and Sketch series, providing one of the most comprehensive 3D printing ecosystems for classroom learning. With a focus on reliability, precision, and innovation, UltiMaker is empowering customers to push the boundaries of what’s possible. 4.2 Formlabs Formlabs is expanding access to digital fabrication, so anyone can make anything. It is a professional choice of 3D printer for engineers, designers, manufacturers, and decision makers around the globe. Formlabs products include the Form 3, Form 3B, Form 3L, and Form 3BL powered by an advanced form of stereolithography (SLA) called Low Force Stereolithography 3D printing, Form Wash and Form Cure post-processing solutions, besides Fuse 1 SLS 3D printer, and Form Cell manufacturing solution. Through its Factory Solutions offering for industrial users, Formlabs provides the factories of tomorrow with the flexibility and versatility needed for demanding industrial applications. Formlabs also develops its own suite of high-performance materials that continue to push the boundaries for 3D printing, as well as best-in-class 3D printing software. 4.3 Markforged Markforged is on a mission to unlock the next 10x innovation in design and manufacturing. It builds an industrial 3D printing platform to liberate designers and engineers from decades-old, slow part creation processes. NASA, Google, Ford, Amazon, Siemens and thousands of companies in 50 countries use Markforged to print same-day prototypes and produce stronger end-use parts than they did before. With Markforged, customers are able to ship 50X faster, spend 20X less, and build products that are 23X stronger. The Markforged platform includes a full ecosystem of 3D printers for metal, composite, and plastic parts; purpose-built metal & carbon-reinforced materials for strength and beautiful finishes; and cloud software for turning drawings into high-strength printing. 4.4 Oqton Oqton accelerates intelligent manufacturing by providing comprehensive software solutions for additive production and 3D scanning, helping industrial and healthcare organizations drive innovation and efficiency. Its additive production software enables complete traceability and visibility across an organization, delivering AI-powered capabilities for additive design, build prep, MES, additive inspection, simulation, and medical image segmentation. Dental labs worldwide use Oqton's Manufacturing OS to automate 3D printing and milling production. Oqton is the developer of the industry-leading Geomagic 3D scanning solutions for reverse engineering and inspection applications. Oqton is supported by partnerships with machine and scanner vendors, software partners, and ERP/CAD/PLM integrations. 4.5 CoreTechnologie CoreTechnologie is a leader in global 3D CAD data management and the most robust technical ally on the market. It develops four software expert in 3D CAD data optimization for CAD users and 3D printers in the automotive, aerospace, robotics, machine tool, luxury and medical industries, namely, 3D Evolution for exact and tessellated conversion and simplification, 3D Analyzer, a pro viewer for advanced analysis, 3D Kernel_IO, a CAD interface library and 4D Additive, a software dedicated to data preparation for 3D printing (repair in exact geometry, textures or lattices). 4D_Additive serves as an advanced preparatory tool for AM, designed to enhance the quality of 3D printing processes. It boasts rapid and efficient automatic nesting capabilities for both 2D and 3D models and features a state-of-the-art texture application module. 4.6 Creatz3D Creatz3D is an authorized reseller of professional industrial-grade 3D printing solutions, which include brands like Stratasys, MakerBot, Desktop Metal, SISMA, XJET, Digital Wax Systems (DWS), and 3DCeram for plastics, metals, and ceramics 3D printing in Singapore. It also distributes DLyte machines from GPA Innova, the world's first dry electropolishing system for precious metals. It has partnered with Materialise for their Rapid Prototyping Software Solutions: 3-matic, Magics RP, and Mimics Innovation Suite, which can aid design and production workflow. Companies using Creatz3D solutions can be found in multiple sectors such as medical, education, animation, aerospace, automotive, consumer goods & electronics, toys, etc. With over a variety of 60 materials, prototypes can accurately represent the look, feel, and function of actual design with Creatz3D. 4.7 Desktop Metal Desktop Metal is driving Additive Manufacturing 2.0, a new era of on-demand, digital mass production of industrial, medical, and consumer products. Its innovative 3D printers, materials, and software deliver the speed, cost, and part quality required for this transformation. Its inventors aim to empower this shift, besides binder jetting and digital light processing. Today, its systems print metal, polymer, sand, and other ceramics, as well as foam and recycled wood. Manufacturers use its technology worldwide to save time and money, reduce waste, increase flexibility, and produce designs that solve the world’s toughest problems and enable once-impossible innovations. 4.8 Divergent Divergent has created the world's first end-to-end software-hardware production system for industrial digital manufacturing – the Divergent Adaptive Production System – allowing customers to design, additively manufacture, and automatically assemble complex structures for automotive, aerospace, and defense applications. DAPS transforms the economics and environmental impact of manufacturing complex structures for vehicles of all types by optimizing designs, dematerializing structures, and eliminating upfront capex. Divergent's innovative approach transforms the traditional automotive structure, tooling, and associated factory assets with an all-encompassing proprietary system that encompasses vehicle design, large-scale manufacturing, and assembly. 4.9 Essentium, Inc. Essentium is fundamentally changing how things are made. As innovators in both materials and production platforms, it is disrupting traditional manufacturing processes by bringing speed and strength together, at scale, with a no compromise material set. It is committed to creating industrial solutions for the world’s top manufacturers and bridging the gap between 3D printing and machining. Essentium has pioneered a new echelon in Additive Manufacturing with its High Speed Extrusion technology, transforming the traditionally slow extrusion 3D printing landscape, typified by FFF and FDM techniques, into a rapid production process. Previously, such printing methods would laboriously take hours for simple shapes and even days for more intricate models. 4.10 ETEC ETEC is a leading global provider of professional-grade 3D printing solutions. As the original inventor of digital light processing 3D printing technology, ETEC has one of the most advanced portfolios of precision polymer printers and materials in the market today. Supported by more than 140 issued and pending patents, ETEC also has more than 190 qualified materials for its platforms. In all thousands of customers across a broad range of industries, including automotive, aerospace, medical devices, and jewelry have relied on ETEC solutions for more than two decades. The company’s solutions are used for prototypes, tooling and low-volume to mass production. 5. Future Prospects For businesses aiming to remain competitive, adopting 3D printing technologies is no longer optional but a strategic imperative. The best 3D printing service providers and solution companies are ready to guide these businesses through their transformation, ensuring that they harness the full potential of this revolutionary technology. The question for decision-makers is not if they should adopt the technology despite 3D printing software challenges, but how quickly they can integrate these advanced solutions to revolutionize their operations and drive innovation across sectors. In embracing the momentum of the present, the march toward the future of additive manufacturing is characterized by exciting trends and innovations. The best 3D printing software is ushering in a new age of manufacturing—one where complexity bows to creativity, and the lead times and costs of traditional fabrication become relics of the past. As industries converge upon this technological nexus, the only constant will be change—change towards more sustainable, efficient, and personalized production. Beyond the present horizon, the landscape of manufacturing is poised for a radical transformation. The adoption of the best 3D printing software will not just be a matter of competitive advantage but of survival. Companies at the vanguard of this revolution are positioned to redefine norms, challenge the status quo, and ultimately, chart the course for Industry 4.0.
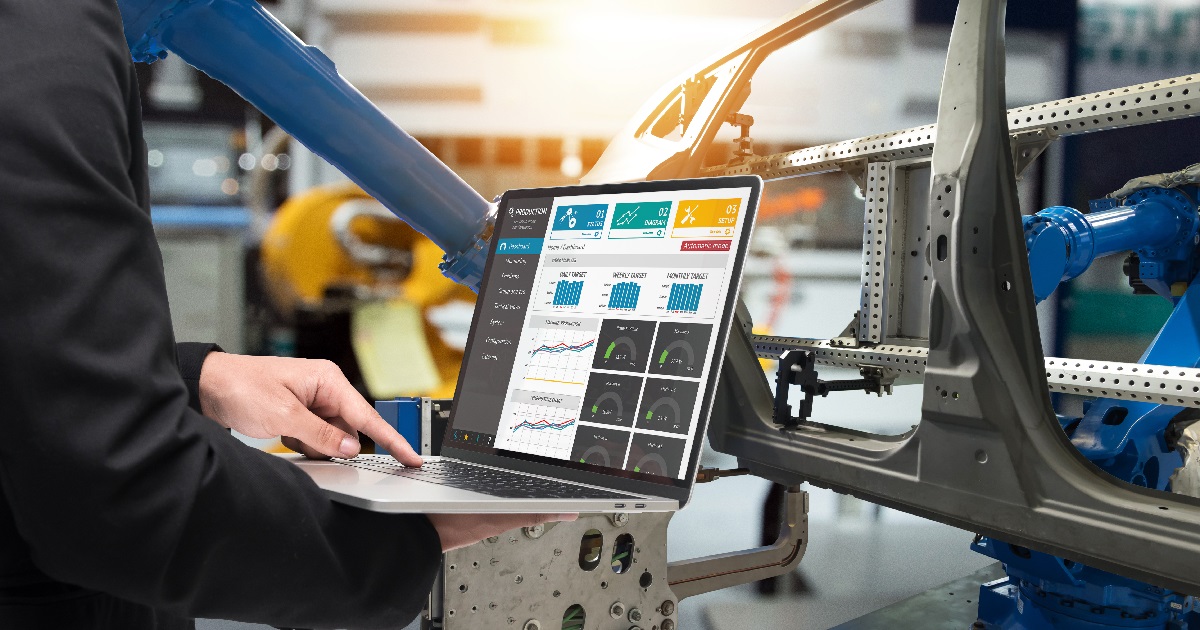
Surpassing 3D Printing Software Challenges With Precise Solutions
Article | March 14, 2024
Unravel techniques to conquer 3D printing challenges. This piece reveals actionable solutions for 3D printing challenges, envisioning the progressive path forward for 3D printing innovation. Contents 1. Deciphering 3D Printing’s Complex Landscape 2. The Challenges of Material Limitations 3. Cost Issues and Economic Viability 4. Inadequate Additive Manufacturing Infrastructure 5. Technical Hurdles 6. Workforce Skillgaps 7. Strategic Solutions for 3D Printing Challenges 8. 3D Printing’s Impact on Future of Manufacturing 1. Deciphering 3D Printing’s Complex Landscape In the dynamic world of manufacturing, 3D printing shines as a beacon of innovation, offering boundless possibilities. However, akin to any revolutionary technology, it grapples with intricate challenges. This article delves into the multifaceted landscape of 3D printing, unraveling various 3D printing software challenges and presents strategic solutions for maneuvering through the additive manufacturing sector seamlessly. At the forefront of advanced manufacturing, 3D printing commands attention with its unique approach to crafting intricate designs layer by layer. But the question is what is the future direction of 3D printing? And can manufacturers fully answer that without understanding the technical issues in additive manufacturing or common 3D printing problems? To truly comprehend and harness its potential, let’s answer a common yet important question, ‘what are the most common challenges of 3d printing’ and uncover solutions for 3D printing challenges. The journey begins with an in-depth understanding of 3D printing software, a crucial facilitator translating 3D models into printer-understandable data. Commonly known as slicer software, it dissects models into sections, enabling printers to create objects slice by slice. Used in makerspaces and creative studios, this software plays a pivotal role in the 3D printing process, often collaborating with other design and CAD software. Additive Manufacturing (AM) processes, contrary to subtractive methods, involve adding material until the product is complete. As researchers explore the possibilities of manufacturing with minimal material waste, 3D printing emerges as a viable solution, capable of producing complex and custom objects. The past year witnessed companies leveraging 3D printing to decentralize production, fostering a flexible, resilient, and sustainable supply chain. As of 2023, the 3D printing market size was reported to be $23.2 billion, with a projected compound annual growth rate exceeding 22% from 2022 to 2026. Future trends in 3D printing suggest that the software segment within the industry is anticipated to demonstrate a compounded annual growth of 20% during the period spanning 2022 to 2026. This category encompasses diverse applications and 3D printing software solutions integral to the additive manufacturing processes. From revolutionizing practices in healthcare, aerospace, automotive and mobility to saving lives with precise 3D-printed models in the medical field, the impact of 3D printing resonates across diverse industries. Despite its advantages, 3D printing presents challenges such as material selection, printing speeds, and accuracy. This article explores 3D printing challenges with a thorough analysis of 3D printing and provides strategic approaches to 3D printing issues to fully experience advancements in additive manufacturing technology. 2. The Challenges of Material Limitations 3D printing is seeing noble innovations across variegated industries, yet challenges persist, particularly concerning material limitations. These challenges encompass a range of issues that impact the effectiveness and versatility of 3D printing technologies. Here, we delve into common 3D printing material limitations: 2.1 Limited Variety of Available Materials The range of materials suitable for 3D printing is still relatively narrow compared to traditional manufacturing methods. Predominantly, thermoplastics like ABS, PLA, and PETG are used due to their affordability and availability. However, these materials may not meet the requirements for specific applications due to limitations in their mechanical properties and durability. This restriction impacts industries requiring specialized materials, like aerospace and healthcare, where the material's performance is critical. Also, not all plastics or metals can be sufficiently heated or cooled to support 3D printing. In addition, many of the materials cannot be recycled and very few are food safe. 2.2 Inconsistencies in Material Properties There's a significant gap in the material properties of 3D printed objects compared to those produced by conventional manufacturing. For example, 3D printed ABS plastic exhibits different impact resistance characteristics compared to ABS plastic produced through micro machining or injection molding. This variability leads to challenges in ensuring consistency and reliability, especially in industries like automotive and aerospace, where material performance is non-negotiable. Companies like BASF, Arkema, and DuPont are developing materials such as carbon-reinforced polymers, offering strengths comparable to metals. Markforged's introduction of Onyx FR, a flame-retardant composite, exemplifies such advancements. Yet, these innovations are hindered by material inconsistencies, which challenge the repeatability and reliability of 3D printing. 2.3 Limited Material Development and Database The challenge of limited material development and database in the 3D printing industry is multi-faceted. The absence of a comprehensive database that details the printing parameters and specifications of various materials hinders the development of a consistent and repeatable 3D printing process. The industry is in need of a robust database that includes information on mechanical and thermal properties, along with specifications for successful printing, to standardize materials for specific applications and increase the reliability of 3D printing as a manufacturing technique. The slow pace of material development and the lack of a comprehensive database have delayed a wider adoption of 3D printing technology. 2.4 High Material Costs and Limited Selection As of 2024, the economic environment of the 3D printing sector has been significantly affected by disruptions in the supply chain and escalating inflation, resulting in heightened costs and production delays for key materials such as metals and resins. The material costs in 3D printing vary widely depending on the type of material and its applications. For instance, standard PETG costs between $20 to $60 per 1 KG spool, while TPU, known for its flexibility and durability, ranges from $40 to $100 per 1 KG spool. ABS, a popular choice for strength and temperature resistance in FDM 3D printing, ranges from $15 to $20 per kilogram. Comparatively, Nylon, with its flexibility and chemical resistance, is priced higher, ranging from $50 to $73 per kilogram. Polycarbonate, known for its strength and good electrical insulation properties, varies significantly in price, ranging from $30 to $93 per kilogram. 2.5 Material Suitability for Complex Applications 3D printing faces challenges in developing materials that are suitable for complex and high-performance applications. This includes sectors like biomedicine, where materials need to meet specific standards for safety and efficacy. The technology's potential in creating parts with high complexity and functionality across mechanical, biological, and electrical domains is hindered by the limited choice of materials that meet the stringent requirements of these fields. The challenge of seamlessly combining various materials with differing properties into a unified, high-definition 3D printing process continues to be a significant hurdle. 3. Cost Issues and Economic Viability For organizations contemplating the integration of additive manufacturing, understanding and managing 3D printing cost issues is crucial. A notable challenge in the adoption of AM at an operational level is the lack of established business and cost-calculation models based on AM technology. This absence of precedent can make it difficult for buyers to accurately assess and plan for the full range of costs associated with implementing and maintaining 3D printing technology in their operations. 3.1 Cost Challenges in Different Sectors Identifying prospects for additive manufacturing reveals that different industries encounter distinct challenges with 3D printing. Different industries face varying challenges when it comes to 3D printing. For instance, the consumer electronics, heavy equipment, and industrial machines industries primarily struggle with the cost of pre- and post-processing. In contrast, healthcare, automotive, and packaging industries grapple with limited material selection. These sector-specific challenges underline the complexity of cost issues in 3D printing. Also, for significant returns on investment in 3D printing software, buyers need to maintain a consistent and ample flow of 3D printing projects. 3.2 Adoption and Utilization Rates There's evidence suggesting a slower adoption rate of new 3D printing technologies than anticipated. This trend affects the industry’s profit-making ability, as even major players like Shapeways, Velo3D, Stratasys, and Markforged have faced financial instability, indicating difficulties in achieving profitability. The 3D printing sector recently experienced a dip in investor trust, a reflection of the performance of its publicly traded companies. This decline in investor confidence underscores the need for the sector to prove its ability to sustain demand, beating diverse 3D printing’s financial challenges in order to achieve long-term profitability. 3.3 Maintenance and Supply Chain Concerns As 3D printing technology continues to advance, equipment breakdowns can still occur, necessitating regular maintenance. These maintenance needs and the associated downtime can represent significant expenses, often a concern for investors. Additionally, lingering supply chain issues may require keeping essential parts in stock, leading to increased inventory carrying costs. Another cost factor is post-processing, which almost all 3D printed parts require. This stage of production often involves additional labor, adding to the overhead costs for each unit produced. 4. Inadequate Additive Manufacturing Infrastructure Inadequate infrastructure stands as a significant barrier, impeding the efficient management of 3D printing operations in numerous companies. The substantial financial outlay for software, materials, training, and facility setup poses a significant barrier, despite the potential benefits of AM. Inefficiencies and errors during the production process emerge due to the lack of integration. Collaborative efforts with AM hardware and software providers can lead to integrated solutions, streamlining workflows and enhancing user experience. Let’s understand infrastructure challenges in 3D printing in greater detail: 4.1 Limited Digital Infrastructure Insufficient digital infrastructure hampers the efficient management of 3D printing operations in many companies. This deficiency spans across various software aspects, including design, simulation, process optimization, and production management. As a result, workflows become disjointed, leading to inefficiencies and increased production costs. To tackle this challenge, businesses need to invest in specialized workflow management software tailored for 3D printing operations. Such software enables centralized production planning, better traceability, and data synchronization between systems, simplifying the integration of 3D printing into digital manufacturing environments. 4.2 High Initial Investment The adoption of additive manufacturing (AM) requires substantial financial resources beyond equipment acquisition. This encompasses investment in software, materials, employee training, post-processing equipment, certification, and facility setup. The significant upfront investment often discourages organizations from embracing AM technology, despite its potential benefits. However, efforts are underway to make AM more accessible, with the development of entry-level 3D printers at a fraction of the cost of high-end systems. These initiatives aim to democratize AM technology and make it more feasible for businesses to invest in. 4.3 Workflow Integration Issues The fragmented nature of the AM ecosystem poses challenges in workflow integration. Companies struggle to navigate disparate solutions and synchronize them effectively. This lack of integration leads to inefficiencies, operational complexity, and higher chances of errors during the production process. To address this, companies are collaborating with AM hardware and software providers to develop integrated solutions that streamline workflows and enhance user experience to deal with common challenges in additive manufacturing. Additionally, efforts to consolidate the AM value chain are underway, aiming to simplify the adoption of AM technology. 5. Technical Hurdles As enthusiasts and professionals alike delve deeper into this captivating technology, encountering common technical issues is inevitable. Let’s understand the most prevalent problems encountered in both FDM (Fused Deposition Modeling) and SLA (Stereolithography) 3D printing processes, and get equipped with the knowledge and solutions needed to transcend obstacles and achieve printing perfection. 5.1 FDM Printing Inconsistencies Inconsistent layer adhesion manifests as visible seams or weak bonds between layers, compromising the structural integrity of prints. Warping and curling occur when corners or edges of prints lift from the print bed, resulting in misshapen or failed prints. Also, extrusion problems, such as under-extrusion or over-extrusion, lead to incomplete or excessive deposition of filament, resulting in flawed prints. 5.2 SLA Printing Concerns Incomplete curing of resin results in soft, tacky prints with poor surface finish and reduced mechanical strength. Delamination can also happen when layers of a 3D print fail to adhere properly, resulting in visible cracks or separation between the printed layers. Various causes like inadequate bonding between layers due to improper print settings, insufficient material compatibility, inadequate surface preparation, or variations in temperature during printing resulting in differential cooling rates between layers can exacerbate adhesion issues and promote delamination. 5.3 Production Speed One of the primary reasons behind the slower production speed in 3D printing is the layer-by-layer additive manufacturing process. Unlike subtractive manufacturing techniques that can rapidly carve out a final product from a block of material, 3D printing builds objects layer by layer, which inherently takes more time. Additionally, factors such as intricate geometries, print resolution, and material properties can further slow down the printing process. 5.4 Streamlining Post-Production Post-processing activities, including cleaning, finishing, and support removal, present intricate obstacles that impede efficiency and elevate costs. One critical aspect of post-production in 3D printing is the removal of support structures. While necessary for printing complex geometries, these structures often leave behind residues that require meticulous manual labor to eliminate. Such manual intervention not only prolongs lead times but also escalates production costs, diminishing the cost-effectiveness of additive manufacturing. Moreover, achieving the desired surface finish on 3D printed parts poses another challenge in post-processing. Depending on the application, parts may require smoothing, polishing, or coating to meet quality standards. Manual finishing techniques are time-consuming and labor-intensive, demanding skilled personnel and specialized equipment. 5.5 Quality Concerns Quality discrepancies in 3D printed parts can have far-reaching consequences, manifesting as functional defects, structural weaknesses, and compromised performance. These issues not only erode product integrity but also escalate the risk of product failure, liability, and associated costs. Additionally, inconsistent quality presents hurdles in regulatory compliance and certification processes, impeding market acceptance and scalability. Notably, issues stemming from bad filament quality, be it due to variations in diameter or length, result in dismal material extrusion rates, yielding substandard 3D prints with potential business ramifications. Such discrepancies can wreak havoc on hardware, especially the extruder, where thin filament leads to inadequate pressure and wide filament strains the extruder motor or hot end opening, thereby jeopardizing 3D printer functionality and print quality. 6. Workforce Skillgaps One of the primary facets of 3D printing workforce skills gap revolves around technical proficiency. Mastery of CAD software, for instance, is paramount for effective 3D modeling and design. Additionally, expertise in slicing software, which translates 3D models into printable instructions, is essential. Understanding the intricacies of various printing materials is crucial for successful 3D printing operations. Workforce skill gaps must be addressed to realize the full potential of 3D printing. 6.1 Complexity of AM Processes 3D printing involves complex processes such as design optimization to fuel innovation easily with additive manufacturing techniques, material selection, and machine operation. Business leaders must recognize the technical nuances of AM and invest in comprehensive training programs to equip their resources with the necessary skills. Skills in digital design, CAD modeling, materials science, and additive manufacturing principles are essential for effective utilization of 3D printing. 6.2 Integration with Existing Workflows Integrating 3D printing into existing manufacturing workflows requires a deep understanding of production processes and supply chain dynamics. Cross-functional collaboration and interdisciplinary training can ensure alignment between 3D printing initiatives and organizational objectives. Also, skill gaps may emerge as new materials, processes, and applications are introduced, requiring proactive measures to stay abreast of industry developments. Engaging with industry experts, research institutions, and educational organizations can provide valuable insights and resources for skill development 7. Strategic Solutions for 3D Printing Challenges Despite 3D printing software challenges, the industry is evolving rapidly, with advancements in technology, materials, and automation. Efforts to bridge knowledge gaps and foster partnerships indicate a promising future for the industry. Companies are actively investing in research and development to overcome existing limitations and unlock the full potential of additive manufacturing. Following are some precise solutions for diverse 3D printing challenges: 7.1 Technical Hurdles Technical challenges in 3D printing are akin to solving a multidimensional puzzle where each piece represents a variable in printing precision, software compatibility, and hardware reliability. Overcoming these challenges is critical for advancing the capabilities of additive manufacturing. These difficulties serve as both a barrier and a catalyst for growth. Addressing them requires a deep dive into the complex interplay between software settings, material behavior, and printer mechanics. 7.2 Material Challenges Material constraints in 3D printing span beyond just availability, touching on aspects of cost, performance, and environmental impact. These can restrict 3D printing's application across various industries. Bridging this gap involves exploring new material compositions and enhancing existing ones to meet specific requirements. The quest for the perfect print material parallels the search for the holy grail in 3D printing. 7.3 Financial Challenges Securing the financial foundations of 3D printing projects is a daunting challenge that requires a delicate balance between innovation, cost-efficiency, and market demand. Overcoming these obstacles is key to the technology's broader adoption. These hurdles underscore the importance of efficient cost management and investment in innovation. Strategic financial management leads to sustainable business growth with 3D printing processes. 7.4 Infrastructural Issues Infrastructural challenges in 3D printing, including inadequate digital frameworks as well as integration issues, highlight the need for cohesive systems that streamline the entire manufacturing process from design to production. Building robust infrastructures is crucial for operational excellence. This includes the development of advanced digital ecosystems and the integration of seamless workflows. 7.5 Quality Problems Quality-related challenges in 3D printing, such as inconsistencies in part strength and surface finish, underscore the necessity for stringent quality control measures and continuous technological refinement. Achieving high-quality outputs in 3D printing is a multifaceted challenge that demands attention to detail in every aspect of the printing process, from material selection to post-processing techniques. 7.6 Skill Gaps Bridging the skills gap in 3D printing involves addressing the shortage of technical proficiency and fostering an environment of continuous learning and innovation within the workforce. The rapid evolution of 3D printing technology presents this formidable challenge of keeping the workforce adept and well-versed in the latest techniques, necessitating ongoing education and skill development through training programs. 8. 3D Printing's Impact on Future of Manufacturing 3D printing, a transformative force in manufacturing, is reshaping the industry by offering unparalleled efficiency, sustainability, and the potential for customization. This technology is making significant strides, especially in sectors like housing and construction, with innovations from startups such as Tvasta, and extending its influence to the electronics sector through the creation of complex, customized components. The adaptability and cost-effectiveness of 3D printing promises to revolutionize manufacturing processes, moving toward more personalized and customized production, thereby enhancing product quality and fostering innovation in design. However, the journey of 3D printing toward becoming a cornerstone of future manufacturing is not without 3D printing software challenges. These hurdles encompass a range of technical challenges in 3D printing, from 3D printing material limitations to skill gap challenges in additive manufacturing. Addressing these common challenges in additive manufacturing require a methodical plan to find strategic solutions for 3D printing challenges. Looking into the future of 3D printing technology, it's clear that despite 3D printing problems and solutions, the industry is poised for growth, driven by advance 3D printing technology and an innovative approach to overcoming 3D printing challenges. The opportunities and challenges of 3D printing highlight the need for continuous learning, cross-sector collaboration, and investment in technology development to surmount cost challenges in additive manufacturing and material limitations. As we anticipate 3D printing in the coming year, it's crucial to address the biggest challenges within 3D printing processes, which point towards the necessity for industry-wide efforts to address 3D printing issues and ensure a sustainable, efficient future for manufacturing. The potential intellectual property challenges underscore the complexity of 3D printing's impact on future manufacturing. The ease of reproducing protected intellectual property through 3D printing calls for safeguarding companies' interests and ensuring the integrity of the supply chain and product quality. Licensing opportunities present a way forward, aligning production with demand and potentially reducing costs. In summary, while 3D printing's impact on future manufacturing is profound, successfully navigating its path requires addressing both the primary and secondary challenges it presents. Embracing strategic solutions to these challenges will enable the industry to unlock the full potential of 3D printing, marking a new era of manufacturing characterized by efficiency, customization, and innovation.
MACHINEMETRICS BLOG
Machine vs. production monitoring: the road to production..., 2024 product releases: an inside look, introducing batch connect machines, introducing condition monitoring workflows.
Ready to empower your shop floor?
- MachineMetrics
- Product Updates
- Data Science
- Lean Manufacturing
Machine Monitoring
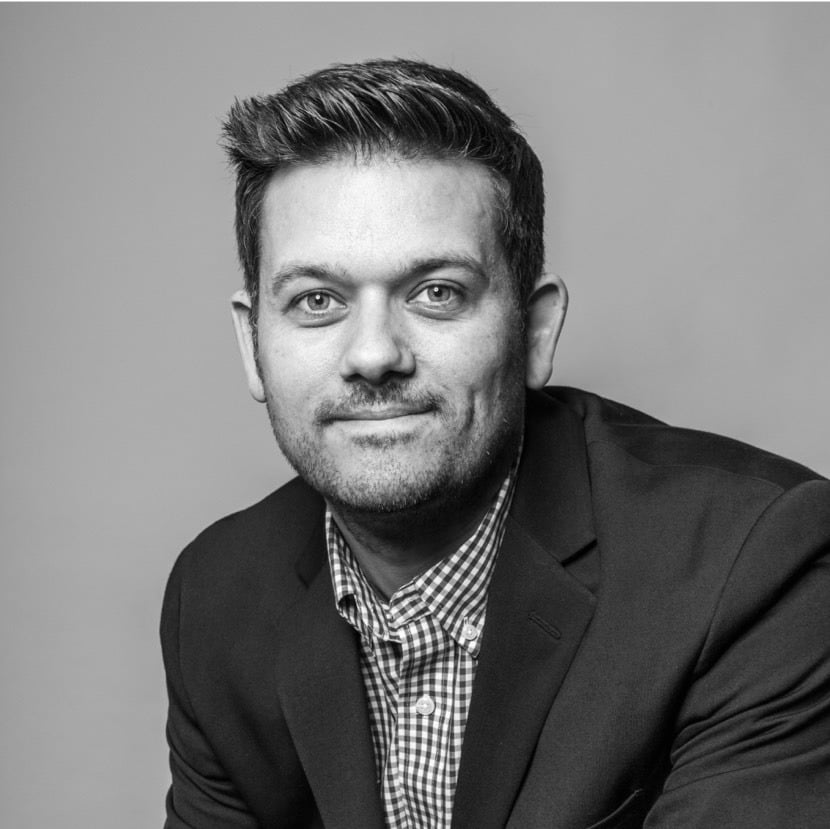
Big Data in Manufacturing: Unlocking Valuable Use Cases
The adoption of big data analytics in manufacturing.
The term “big data” refers to increasingly complex, massive data stores that can’t be effectively processed using traditional methods. In manufacturing, big data can refer to information collected from a variety of sources including machine sensor data, quality assurance information, data from suppliers, production output, maintenance, financial information, and basically any other measurable process that goes into modern manufacturing.
There’s a reason manufacturers are collecting such vast swathes of data about anything and everything. Big data can be processed and refined into business insights that can fuel massive financial growth, customer retention, savings on maintenance, warehousing, and unexpected downtime, and more.
Using the power of big data and manufacturing analytics , manufacturers are more easily able to add efficiency and productivity to their businesses while knowing that the moves they make are calculated and based on accurate data. This adds not only a boost to the probability of success, but also confidence in the ideas that are implemented.
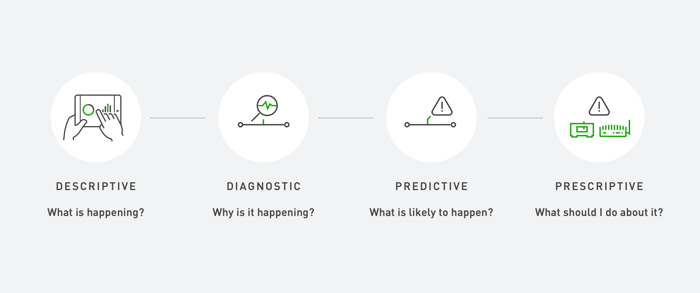
Why is the Use of Data Growing in the Manufacturing Industry?
The answer to this question is two-fold. To make increasingly complex decisions and gain deeper insights, manufacturers are relying more and more on data from a variety of sources. As more data is collected from the shop floor and transformed into usable reports, data-driven decisions can be made that were simply not possible before.
Another reason the use of data is growing in the manufacturing industry is because it’s easier to access. The barrier to entry for implementing IIoT devices and smart factory equipment is at a historical low. Manufacturers can easily and affordably measure many aspects of their business, both in terms of data capture and data warehousing and storage. For example, with MachineMetrics, manufacturers can deploy plug-and-play solutions to provide immediate visibility into shop floor performance .
Additionally, modern markets push manufacturers toward the use of big data in order to stay flexible, efficient, and relevant to their target consumers, while remaining competitive in the marketplace. Data is unlocking the next step in manufacturers’ continuous improvement journey.
Big Data Use Cases in Manufacturing
Big data has a place in nearly every aspect of running a manufacturing business. Some of the most prominent use cases for big data in manufacturing include:
Machine Utilization
When machines are underutilized, manufacturers lose time, money, and opportunity. By analyzing data about when factory machines are utilized, manufacturers can clearly see which machines serve as bottlenecks, which are being underutilized, and which are being pushed to the brink of their capacities.
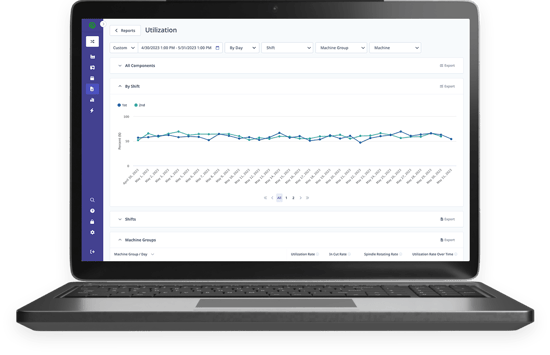
Machine Utilization Report from MachineMetrics.
Product Design
Big data can be used to gather information and inspiration about potential new products, augment understanding of how a product is actually used by customers to develop changes and improvements that better align with use expectations, as well as to determine product viability with greater ease and effectiveness.
Product Quality
Big data has been used with great success alongside machine learning to understand sentiment in customer reviews and support tickets to determine which points of failure are most frequent and frustrating for consumers. Big data can also be used for on-the-line quality control and quality assurance using technology to capture and report machine condition data .
Demand Forecasting
Big data offers manufacturers a peek into the future of what customers will want and when. By forecasting demand, manufacturers realize savings on warehouse costs, wasted supplies, and production time that could be otherwise spent elsewhere.
Customer Experience
Customers will feel more heard when their concerns are addressed. Big data offers the insight to not only address the concerns that arise but to spot and prevent future ones. Also, big data usually leads to higher quality products at lower costs and with speedier times for delivery.
Supply Chain Optimization
By analyzing supply chain data, manufacturers can cut costs both by finding cheaper suppliers and by bundling related products from single suppliers, boost quality, see and find solutions to logistics issues—such as snow storms and natural disasters—to continue business without missing a beat.
Benefits of Big Data In Manufacturing
Manufacturers who make effective use of big data see notable benefits to business from multiple angles. Since data can be applied in a broad manner, both use cases and benefits can be limitless, increasing in complexity and incremental value based on the "data-maturity" of the manufacturer. Some of the top benefits of big data in manufacturing that are usually the first to spur data collection and analysis include:
Competitive Advantage
Access to accurate, real-time production data allows for unprecedented business flexibility and agility that can even mesh with customer expectations. With insight into the shop floor, better decisions can be made with ease, giving manufacturers a strong advantage over less data-savvy competitors.
Predicting trends and being able to more quickly iterate on product design leads data-driven manufacturers to innovate better. In the same vein, time and money saved on supplies and production thanks to the use cases above means that manufacturers have more resources and flexibility to commit to innovation while still seeing success.
Lower Costs
Not buying excess supplies, optimizing warehouse space, finding the most cost-effective quality suppliers available, and circumventing logistical struggles all lead to cost savings. Further, machine maintenance that keeps equipment running smoothly reduces downtime and catastrophic ( and costly ) equipment failure .
Improved Customer Service
The ability to analyze customer data at every stage of their journey from marketing to sales to reviews on social media means that customers are able to receive top-notch, data-driven service that addresses their real wants, needs, and concerns.
Examples of Big Data in Manufacturing
One of our customers, BC Machining, utilizes data to understand when their machining tools are going to break. Via our High Frequency Data Adapter , BC Machining is able to extract high frequency data straight from the control of the machine. After building an algorithm, we are able to predict and prevent machine tool failures by monitoring certain thresholds.
This advanced big data use case has been able to eliminate nearly 100% of scrap parts. Furthermore, it has saved operator time, as BC Machining no longer needs to sort the scrap part, allowing both them and the machines to be focused on producing good parts and generating revenue for the company. The result has been $72,000 in annual savings per machine.
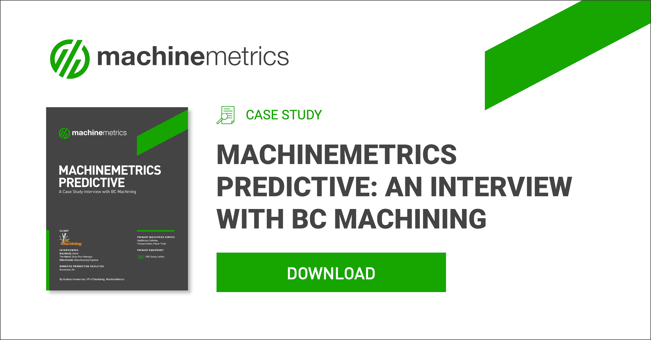
Other manufacturers use big data to keep factory floor workers on track through the use of visible stats that update in real-time. With this, workers are able to understand where they stand in relation to production goals, as well as react quickly to any problems on the shop floor, such as a downtime event.
Subscribe to our mailing list
Related posts, read the latest.
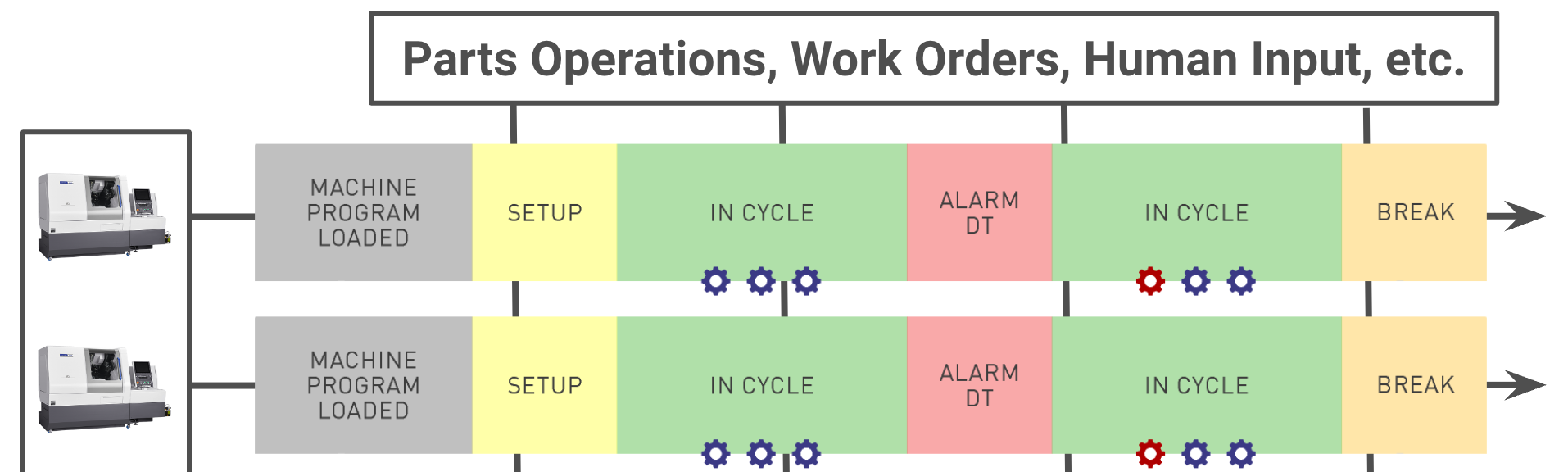
- MachineMetrics (382)
- Lean Manufacturing (72)
- Industry 4.0 (50)
- Manufacturing data (23)
- Manufacturing News (20)
- Data Science (15)
- data collection (14)
- Product Updates (12)
- Machine Monitoring (10)
- manufacturing analytics (10)
- Connected Factory (9)
- Smart Manufacturing (9)
- industrial iot (9)
- CNC Machines (8)
- Productivity (8)
- Downtime (7)
- Industrial Automation (7)
- Tool Monitoring (7)
- Big Data (6)
- Data Visualisation (5)
- Edge Computing (5)
- Maintenance (5)
- Process Optimization (5)
- Shop Floor (5)
- digital manufacturing (5)
- Automotive (4)
- Machine Learning (4)
- Quality Assurance (4)
- Aerospace and Defense (3)
- Contract Manufacturing (3)
- Data Cleaning (3)
- Death of MES (3)
- Heavy Machinery Manufacturing (3)
- MES software (3)
- Medical Device Manufacturing (3)
- OEE Software (3)
- Oil and Gas Manufacturing (3)
- capacity (3)
- continuous improvement (3)
- inustrial IOT (3)
- preventative maintenance (3)
- security (3)
- 8 wastes (2)
- Condition Monitoring (2)
- Dashboards (2)
- Internet of things (2)
- Production Monitoring (2)
- Strategic Partnerships (2)
- management (2)
- press release (2)
- real-time analytics (2)
- Digital Transformation (1)
- Downtime Categorization (1)
- FANUC FOCAS (1)
- Line Balancing (1)
- Machine Tool Distributors (1)
- Machinery (1)
- Manufacturing Innovation (1)
- Production Scheduling (1)
- Quality Control (1)
- Supply Chain (1)
- Turnkey contracts (1)
- coaching (1)
- elon musk (1)
- employee engagement (1)
- link roundup (1)
- manufacturing (1)
- manufacturing software (1)
- networks (1)
- release notes (1)
844-822-0664
Easthampton Office
116 Pleasant St, Suite 332, Easthampton, MA 01027
Edge Platform
Cloud Platform
APIs & Applications
Production Monitoring
Condition Monitoring
Predictive Maintenance
Process Optimization
For Machine Builders and Distributors
For Developers
Aerospace & Defense
Contract Manufacturers
Heavy Machinery
Medical devices
Oil & Gas
Precision Metalworking
ERP Integration
Metal Stamping & Fabrication
Tool, Die & Mold CNC
ROI Calculator
Waste Calculator
Connectivity Hub
Partner Program
Privacy Policy
Data Processing Addendum
Service Level Agreement
Website Terms
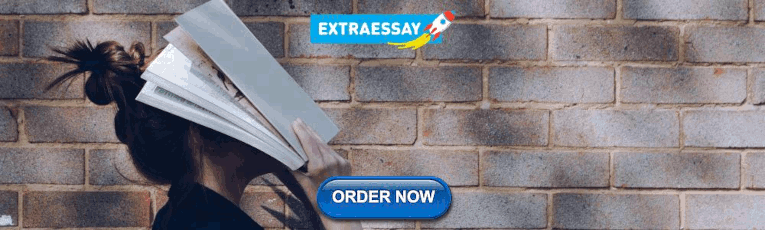
Big Data Analytics for Predictive Manufacturing Control - A Case Study from Process Industry
Ieee account.
- Change Username/Password
- Update Address
Purchase Details
- Payment Options
- Order History
- View Purchased Documents
Profile Information
- Communications Preferences
- Profession and Education
- Technical Interests
- US & Canada: +1 800 678 4333
- Worldwide: +1 732 981 0060
- Contact & Support
- About IEEE Xplore
- Accessibility
- Terms of Use
- Nondiscrimination Policy
- Privacy & Opting Out of Cookies
A not-for-profit organization, IEEE is the world's largest technical professional organization dedicated to advancing technology for the benefit of humanity. © Copyright 2024 IEEE - All rights reserved. Use of this web site signifies your agreement to the terms and conditions.
From Big Data to Actionable Business Insights: JAKALA's Success with ArcGIS GeoAnalytics Engine
Challenge: JAKALA faced challenges in analyzing consumer movement due to the large volume of data, complex analytics, and the need for timely delivery of insights, which required scalable workflows and faster processing speeds.
Solution: The solution involved implementing Esri's ArcGIS GeoAnalytics Engine within the Azure Databricks environment, providing JAKALA with a comprehensive and scalable analytic solution that combines the spatial analytic capabilities of Esri technology with the computing power of Databricks. The solution allows for seamless integration and eliminates the need for JAKALA to manage its own infrastructure.
Result: Implementing GeoAnalytics Engine in the Azure Databricks environment has improved JAKALA's analytics processing time, enabling faster data analysis; facilitating timelier data delivery; and allowing for analysis on larger datasets, leading to expanded service offerings.
Products: The Esri product featured in this story is ArcGIS GeoAnalytics Engine. The managed Spark service featured is Azure Databricks.
Partner: JAKALA is a MarTech company driving digital transformation by combining a personalized touch with data analytics and technology. JAKALA helps companies build and accelerate their digital business.
JAKALA, a marketing technology (MarTech) company headquartered in Milan with offices worldwide, specializes in marketing and sales, with a focus on artificial intelligence. Founded in 2000 by Matteo de Brabant, the company's 3,000 employees strive to deliver unique and customized solutions to more than 900 clients in more than 30 countries by integrating strategy, technology, and creativity.
By utilizing the power of location intelligence, JAKALA aims to help companies unlock their potential by uncovering new opportunities, empowering decision-making, and enabling sustainable growth.
Gaining a comprehensive understanding of consumer movement demands a significant volume of data, and processing this data can be time-consuming. JAKALA needed a way to efficiently analyze large volumes of data so that the company could provide valuable and timely insights for its clients.
To overcome this challenge, JAKALA leveraged ArcGIS GeoAnalytics Engine in the Azure Databricks environment to provide insights more efficiently for the company's clients.
Analyzing consumer movement is a critical component to understanding how various geographic areas attract visitors to points of interest (POIs) across different market sectors. Examples of POIs include hotels, restaurants, theaters, museums, shopping malls, and tourist attractions. To make sense of the data, it is important to understand not only the types of visitors, but also the POIs visited, the frequency of visits, and overall movement patterns. And, most importantly, it is critical that this data is analyzed and reported on in a timely fashion to facilitate decision-making. JAKALA faced several challenges around scaling up its analytic workflows to deliver quality data and analytics on the timeline needed by the company's clients.
Data volume and analytic complexity present a challenge when performing big data analysis. JAKALA works with large-volume people movement data that the company sources from a vendor that amalgamates opt-in location tracking. The sourced data relies on more than 10 million devices, generating around 1 billion data points per day. For monthly analytics, this typically means 30–40 billion data points that need to be incorporated in the analyses. The size of the data made it so that many geospatial analysis tools could not handle the entire dataset at once; the JAKALA team had to subset the data into weekly segments to complete the workflow. While segmented data is useful in some instances, longer time periods of data need to be used for forecasting as well as achieving richer pattern analyses.
For big data projects, it is important to have systems that scale easily. JAKALA's large volume of data in the workflow could not all be analyzed in one place or with one tool. This led to the shuffling of data between different analytics tools and systems to combine the data and generate final products. For this workflow, manual data compilation and analysis were time-consuming and inefficient, hindering JAKALA's ability to deliver timely insights to its clients.
Shortening the time to delivering insights is critical to decision-making, and processing large datasets needs to be fast. Once one month's data was received, it would take almost another month to complete data cleaning and analysis, resulting in a significant lag between data collection and analysis delivery.

JAKALA needed a single environment that would facilitate working with large volumes of data and spatial and tabular inputs as well as allow easy interoperability across all the company's data warehouses and analytics environments.
To find the right solution, JAKALA explored several of Esri's big data products, including ArcGIS GeoAnalytics Engine. GeoAnalytics Engine enables spatial analysis of big data by extending Apache Spark with ready-to-use SQL functions and analysis tools. During the evaluation of a large analysis case with several billion data points, GeoAnalytics Engine demonstrated an optimal combination of analytical capabilities, swift performance, and seamless integration with JAKALA's data stored in a Microsoft Azure data store.
Fabio D'Ovidio, head of the location intelligence platform at JAKALA, says, "In testing one of our largest datasets in GeoAnalytics Engine, we saw great performance. This made us realize that if this analysis case worked, then all of the other analyses for our use cases would also work quickly in GeoAnalytics Engine."
JAKALA is a Databricks partner and utilizes a comprehensive integration of Databricks’ platform to leverage the scalability and performance provided by that platform. Databricks provides an Apache Spark-based cloud platform with a unified set of tools for building, managing, and working with data at scale. GeoAnalytics Engine can work with Databricks using Azure, Amazon Web Services (AWS), or the Google Cloud platform as part of an integrated spatial analytics environment connected with the Databricks lakehouse architecture.
Stefano Angarano, manager of web development and mobile data analysis at JAKALA, says, "Since our infrastructure is all on Azure, we can take our data stored there and easily combine it on Databricks with Esri's GeoAnalytics Engine. It's easier and more efficient to combine these environments instead of having to move the data somewhere else for analytics."
Esri's ArcGIS GeoAnalytics Engine provided the perfect solution with a Spark-native library of over 160 spatial functions and tools that work seamlessly within a Databricks workflow. As a bonus, JAKALA is now able to save time and energy on maintenance because working with GeoAnalytics Engine in Databricks allows staff to focus on the analytics and not on managing the company's own cloud or server infrastructure.

The integration of GeoAnalytics Engine with Azure Databricks has resulted in a substantial improvement in analytics processing time for JAKALA. Additionally, GeoAnalytics Engine has proved to be a practical and efficient solution, streamlining the overall analysis process and improving automation, scalability, and maintenance efficiency.
JAKALA staff saw immense improvement in analytic processing time when handling the company's monthly data—a result of the computing power of Databricks and GeoAnalytics Engine.
"With GeoAnalytics Engine, we are able to receive all the data and perform the necessary analysis within a couple of days, whereas without it, it would take us weeks. This makes it much easier to provide our clients the data that they need, at the speed needed to make timely decisions," says Angarano.
The shortened analysis time not only facilitates timelier data delivery, but also the performance gained makes it possible to complete analysis on much larger datasets. For instance, staff can now examine annual patterns instead of weekly or monthly patterns to facilitate longer-range analysis and forecasting. This helps staff conduct more comprehensive and accurate analyses and provide valuable insights and forecasts to the company's clients based on a more detailed understanding of annual patterns and trends.
JAKALA also found that GeoAnalytics Engine helped streamline the overall process. Using GeoAnalytics Engine in the Databricks environment improved staff's ability to automate and scale the analysis. They were able to analyze large volumes of data in a more efficient manner and could adjust the scale of resources based on the complexity of the analysis. Maintenance of the system was also simplified as GeoAnalytics Engine is cloud based and requires minimal manual updates.
"Performing monthly data analysis is a recurring task for us, and each month we have a consistent set of operations to perform. Having an automated solution is crucial for us to streamline the analysis process and eliminate the need for manual analysis, ensuring efficiency and accuracy in our operations," says Angarano.
Using GeoAnalytics Engine to drive the analysis workflow has allowed JAKALA to offer new services to its customers, such as additional movement analysis and the ability to bring in larger amounts and longer timespans of data for improved context and forecasting. In the future, JAKALA is expanding to new data sources such as vehicle-generated data to complement the existing data staff work with from personal devices. This will open new doors for richer analyses about human movement and patterns.
"We are excited about the network analysis capabilities offered by GeoAnalytics Engine. The ability to leverage network-based service areas and closest facilities opens up new possibilities for us to incorporate additional metrics and enhance our analysis," says Angarano.
To explore a real-world use case about JAKALA utilizing GeoAnalytics Engine to analyze consumer movement data and evaluate the retail landscape, please read this blog to learn how retail mobility analytics can drive success. The use case delves into how JAKALA analyzes billions of data points to better understand geographic patterns, target advertising campaigns, and improve marketing strategies for its clients.
GeoAnalytics Engine has provided valuable insights to our clients, enabling them to make informed decisions about marketing campaigns, site evaluations, and service development.
Transform your big data into insights
Workplace performance measurement: digitalization of work observation and analysis
- Open access
- Published: 29 May 2024
Cite this article
You have full access to this open access article
- Janusz Nesterak ORCID: orcid.org/0000-0001-9114-4947 1 ,
- Marek Szelągowski ORCID: orcid.org/0000-0002-5114-6793 2 &
- Przemysław Radziszewski ORCID: orcid.org/0009-0008-7447-0343 1
174 Accesses
Explore all metrics
Process improvement initiatives require access to frequently updated and good quality data. This is an extremely difficult task in the area of production processes, where the lack of a process digital footprint is a very big challenge. To solve this problem, the authors of this article designed, implemented, and verified the results of a new work measurement method. The Workplace Performance Measurement (WPM) method is focused not only on the measurement of task duration and frequency, but also on searching for potential anomalies and their reasons. The WPM method collects a wide range of workspace parameters, including workers' activities, workers' physiological parameters, and tool usage. An application of Process Mining and Machine Learning solutions has allowed us to not only significantly increase the quality of analysis (compared to analog work sampling methods), but also to implement an automated controlling solution. The genuine value of the WPM is attested to by the achieved results, like increased efficiency of production processes, better visibility of process flow, or delivery of input data to MES solutions. MES systems require good quality, frequently updated information, and this is the role played by the WPM, which can provide this type of data for Master Data as well as for Production Orders. The presented authorial WPM method reduces the gap in available scholarship and practical solutions, enabling the collection of reliable data on the actual flow of business processes without their disruption, relevant for i.a. advanced systems using AI.
Similar content being viewed by others
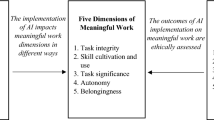
The Ethical Implications of Artificial Intelligence (AI) For Meaningful Work
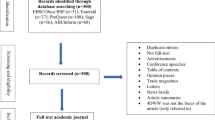
The four-day work week: a chronological, systematic review of the academic literature
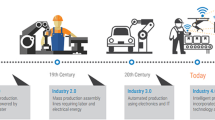
Literature review of Industry 4.0 and related technologies
Avoid common mistakes on your manuscript.
Introduction
In Industry 4.0 (Schwab, 2016 ), also known as the Digital Transformation (Bounfour, 2016 ), organizations are forced to continuously improve their business processes. This improvement is powered by the increasingly new possibilities offered by the extremely fast development and the practical absorption of information technology (IT) (Novak et al., 2023 ). This is a prerequisite for maintaining a competitive position and sustainable development (Van Looy, 2021 ). Business process management (BPM), which provides a holistic view of the organization, focused on strategic alignment and, at the same time, ongoing efficiency, is one of the most frequently used methods enabling management in accordance with the requirements of Industry 4.0. The implementation of BPM enables organizations not only to ensure ongoing effective operations, but also to continuously redesign and reengineer them (Bispo et al., 2019 ; Hammer, 2015; Davenport, 2015). However, this requires the organization to constantly monitor ongoing processes in order to simultaneously, sustainably use its opportunities to increase their current efficiency and ensure constant readiness to implement radical innovation (Helbin & Van Looy, 2019 ). For this to be possible, ongoing access to data on ongoing processes, the context of their implementation, and the ability to use data on previously implemented processes are necessary. It results in business process management (BPM) in Industry 4.0 practically becoming strictly tied to the use of diverse information systems (IS) (Pena et al., 2022 ). The possibility of modeling and managing process flow is available not only in workflow software or business process management systems (BPMS), but also in enterprise resource planning (ERP) systems or customer relationship management (CRM) systems, whose logs contain up-to-date key data for the operations of the organization (Szelągowski et al., 2022 ). The ability to use a wide range of detailed data is increasingly important because of the use of intelligent IS incorporating elements of artificial intelligence (AI) (Zirar et al., 2023 ). The efficiency of using these systems, and thus the efficiency of the business processes they support, depends on the data on which they were trained and on which they work (Bartlett et al., 2023 ; Farrow, 2019; Thesmar et al., 2019). Therefore, methodologies and tools for obtaining reliable, diverse, detailed data are of great importance for the ongoing efficiency and improvement of operations management, including production processes. Unfortunately, the methodologies of work observation and measurement used so far, such as work sampling, snapshot analysis, or timing, as well as process mining techniques using data from IS event logs, have numerous limitations (IEEE Task Force on Process Mining, 2012 ). On the one hand, their labor consumption and costs, and on the other, the subjectivity and incompleteness of the collected data make their use ineffective or even impossible in many areas of the organization's operation. The availability of accurate, current, high-quality data is of particular importance for Manufacturing Execution Systems (MES), which operate in real time. Also the effectiveness of AI solutions, including machine learning (ML), which are becoming more frequently planned and implemented to improve the efficiency of business processes, are burdened by a crucial limitation in the scope, detail, and quality of data on the implemented business processes. The lack of required data or the low quality of accessible input data significantly affect the value of the output generated by process mining techniques or AI algorithms. This issue can be perceived as the main barrier for the common usage of AI technologies in business process improvement initiatives. And while the literature contains numerous positions discussing the above-mentioned limitations (van der Aalst, 2019a , 2019b ), there is a lack of scholarship which would propose both a methodology and practical solutions. In answer to the mentioned problem, the authors of this article have designed, created, and implemented in conducted research a proprietary (copyrighted) method called the Workspace Performance Measurement (WPM). The WPM as a solution consists of a methodology of operation and intelligent IS enabling the collection of digitalized data based on the visual observation of work performance. The analysis of the image of the work performed by the WPM enables the collection of digital data describing the work, their automatic initial analysis, and entering the processed information into various types of IS and AI. Due to a wide range of registered parameters, the WPM not only reflects a flow of activities in processes, but goes deeper into a cognitive mechanism focused on finding answers to a simple question: Why? Why a given activity takes longer than planned or expected? Why the performance of a stakeholder involved in a process dynamically changes and affects the process? This is why the WPM as an method perfectly fits all kinds of process improvement frameworks, solutions, and tools: from Lean Management and Process Mining to systems based on ML and AI algorithms. At the current stage of development, the WPM is dedicated in a special way to the continuous monitoring of operations management, but the method of its work allows for data collection and analysis of both traditional and dynamically managed business processes.
The article has the following structure: The next section presents a historical overview of the hitherto used methods of work observation and measurement and their limitations. The requirements and opportunities created by observing work in Industry 4.0 are also presented. The second section presents previous attempts to digitalize work observations. The third section introduces the working methods and architecture of the WPM. This section also discusses how the WPM eliminates or significantly mitigates the limitations of existing methods of work observation and measurement. The fourth section presents a case study on the use of the WPM. Finally, the summary section outlines the key implications of using the WPM. Future directions of research and current limitations of the WPM are also discussed.
State of the art
Considered to be the forerunner of BPM, Scientific Management (Taylor, 1911 ) referred only to structured, repetitive mass production processes. Since then, in over 100 years of its development, BPM has covered all organizational processes, regardless of their nature or place in the value chain, and even crossed the boundaries of the organization (Mendling et al., 2020 ; Szelągowski, 2019 ). The development of BPM was accompanied by the development of methods for observing and measuring the implementation of business processes.
Historical outline of the methods of observing the implementation of business processes
The history of business process measurement methods begins during the industrial revolution. Those methods are characterized by the application of a leading way of information acquisition: observation. Observation as a method of building knowledge about a flow of activities in a production process has been used for centuries. One of the most important books, which played a crucial role in the stimulation of theoretical discussion about the division of labor, published by Adam Smith ( 1776 ), presents a list of activities "seen in a small manufactory".
Observation methods performed before and during the eighteenth century were immature, spontaneous, and missing a quantitative perspective. Starting from the beginning of the twentieth century, the observation method focused on the discovery of specific rules which decide on the production workspace being more effective. As such, the method has become more structured and scientific. In 1911, Taylor published his work The Principles of Scientific Management , which has begun a new era of production management. He added an important factor to the analysis of work, which is time. The method of observation shifted from not only how production is organized in sets of different activities, but also how long each activity takes. He also noted that the measurement of work and the effort of collecting and analyzing data on process flows must be minimal, and access to information for controllers must be in real or near real time.
Further development of the work observation method in management science has appeared again when a young construction worker, Frank Gilbreth, was observing the bricklaying process. Thanks to the results of this observation, he modified the existing process and more than tripled its effectiveness. That experience became a trigger to design and develop a new method of workspace observation called a “motion study.” Gilbreth went much deeper in his analysis then Taylor (Gilbreth & Kent, 1911 ). In a center of motion study, analysis is not only an action or a step taken in a particular process, but a move that observed workers perform. He classified all moves he started to call 'therbligs' and created a list of 17 unique ones. In a more detailed version of this analysis, it is suggested to analyze each hand separately.
Indisputably, the motion study method described above is the most detailed way to measure activities conducted by workers in a workplace. However, the effort and size of resources which are required to execute the method regularly or on a big scale is so huge that it will always be applied to a limited degree. To break this barrier and make it possible for work measurement to be executed more frequently and on a wider scale, Tippett ( 1935 ) has designed and announced a simpler version of the motion and the time study analysis known as a “work sampling.” The main assumption behind the new method was that instead of measuring the duration of each activity that a particular production process consists of, an observer responsible for the research should only register information about its appearance. That method significantly popularized the application of statistical techniques into scientific management and business process management as well. It made it possible to reduce labor consumption, but did not remove other limitations of work observation and measurement methods, such as:
The impact of the very fact of observation on the implementation of processes,
The subjectivity of interpretation by the observer in the case of non-numerical data,
The need to allocate additional resources for observation,
The need to train observers in the methodology of observation and in the scope of the observed processes in order to avoid misinterpretation of the activities carried out,
The usually low resolution of recorded events, resulting from limiting the labor intensity and costs of observation,
Not taking into account actions between individual samples,
The fragmentation of observations, collecting data from one place of observation of a process that is usually carried out in many places and even different organizational units.
The vast majority of work efficiency measurement methods developed in the first half of the twentieth century are a development of or improvement on earlier ideas and methods. Method Time Measurement (MTM), published in 1948 by Maynard et al., has been created as a development of the Glibreths method. Still, it maintains the same weaknesses as mentioned above (e.g. lack of sequential order). In 2012, Balaila and Gilad ( 2012 ) published Process of Determining Manpower Standards (PDMS), a method that incorporates values from work measurement and metrics from other production outputs.
New technology: process mining
In Industry 4.0, ISs are used to analyze the course and support the implementation and improvement of business processes. Using process mining techniques based on the data collected in their event logs, it is possible to identify the course of the processes being carried out, and on this basis, compare them with the applicable standard and specify proposals for improvements allowing for the preparation of an improved standard (Badakhshan et al., 2022 ; Choi et al., 2022 ; IEEE Task Force on Process Mining, 2012 ; Lamghari et al., 2021 ; Lorenz et al., 2021 ; Mamudu et al., 2023 ). This is the scope of identification and analysis that fully covers earlier observation methods such as work sampling, snapshot observations, or work timing. However process mining techniques, similarly to the previously used observation techniques, have significant limitations are significantly limited on the one hand to data foreseen by the creators of the system supporting business process execution, and on the other – to data consciously input by the user. According to van der Aalst ( 2019a , 2019b ), Vogelgesang et al., ( 2016 ), or Jans ( 2012 ), these are:
Analysis limited to selected data sources
The process mining analysis covers only the data collected in the logs of selected ISs, e.g. ERP, MRPII, BPMS, or workflow. Data from other sources are completely omitted, e.g. data from e-mail, social media, SMS, or telephone calls, and often also service systems, "logistics applications," etc.
Incomplete data
The analysis does not take into account activities carried out between successive entries in the IS event logs, for which no data has been saved, e.g. dynamic interpersonal interactions, downtime at work etc. Especially in the case of data entered by process contractors, the analysis includes only data entered at previously identified points in the process. Typically, these are data on starting or finishing tasks, known already in the design phase and included in the design of the business process and the IS that supports it. Even in the case of processes in which the project provides for many variants of execution, we can talk about tracking the implementation of the process rather than discovering the actual paths of the process implementation.
Data unreliability
The input of data in systems supporting business process execution is decided upon in a subjective manner by the user executing the process and operating the system. Striving to limit the labour-intensity of data input, the user will limit such data to the bare minimum, which will further strengthen the limitation set in point 2 “Data incompleteness.” In most cases, the subjective selection of the input data, including the conscious or unconscious desire to present one's actions in a positive light, may lead to the unreliability of such data and disrupt the complete picture of the executed process, the reasons behind troubles in its execution, or the failure to achieve the set goals.
Data discontinuity ("quantization"/"density" in time).
For process contractors, manually entering data into the IS is an additional activity, often perceived as redundant and distracting from the performance of work. Therefore, the data entered by process performers is usually related to completed tasks, and sometimes refers to when tasks start and end. Even if many activities are performed as part of the task (e.g. measurements of important process parameters), only result data or, at best, quantized data including values at defined points in time are entered (e.g. if patient blood pressure measurements are carried out every hour, then there is usually one entry per day in the documentation). Only in the case of data collected from automatic sensors is it possible to collect and analyze basically any "dense" time data on how the process is carried out.
Unpredictability and uniqueness
Processes may require individual adaptation to a specific execution context, customer requirements, or quick changes in the way of execution (Löhr et al., 2022 ). This may make it difficult to analyze the collected data (e.g. a large and rapidly growing number of process implementation variants) or require significant work, time, and costs to collect detailed retail data.
Technological limitations
For obvious reasons, the results of process mining depend on the quality of the data, including its completeness, consistency, and reliability. The result is also significantly affected by the limitations of process mining techniques, such as:
Lack of flexibility in process analysis – process analysis methods that work for one process may not work for another,
Methodological problems with the analysis and modeling of complex processes or their fragments carried out concurrently,
Limited possibilities of identification and analysis of different process variants,
Focus of process mining analyses on discovering the course of processes, with much less diagnostic or predictive possibilities.
Organizational restrictions
The implementation of business processes is often divided between various organizations, and within an organization between many organizational units, often with different ISs (e.g. production processes are usually dispersed in various departments, production centers, or teams; diagnostic and therapeutic processes between various healthcare units, and within individual units between different departments and support units (laboratories, pathology departments, genetics departments etc.) – this can lead to difficulties in identifying and analyzing the overall business process throughout the implementation period.
Difficulties related to the protection of sensitive data
This applies, for example, to personal data or confidential information that needs to be identified and protected so that it does not fall into the wrong hands and is not used for unintended purposes.
Process mining, therefore, provides limited insight into process execution. The incompleteness and discontinuity of data in many cases do not allow for the reliable reconstruction of the actual process flow (Löhr et al., 2022 ). At the same time, it does not explain why certain events occurred, what caused them, or what happened between registered events. It is obvious that if the data is incomplete and of low quality, then the results of the analysis and the decisions made on its basis will also be of low quality and subject to the risk of error. Incomplete or discontinuous data will not allow us to answer the question: Why does a given action take longer than anticipated? And the subjective selection of data, in many cases bordering on being unreliable, will not allow for the reliable analysis of why the actions of the process executor deviated from the anticipated workflow.
Industry 4.0 requirements and opportunities for business process observation
In the age of Industry 4.0 (Schwab, 2016 ; Hermann et al. 2015), organizations are forced to constantly raise their operational efficiency and flexibility on the basis of the use of technologies such as cyber-physical systems, cloud computing, process mining, robotics, and artificial intelligence (AI). This paradigm shift had led to the an unprecedented degree of automation, connectivity, and exchange of data in industrial environments, providing support to what is often called the concept of the “smart factory” (Lasi et al., 2014 ; Lu, 2017 ; Oztemel & Gursev, 2020 ; Zonnenshain & Kenett, 2020 )). By going outside of Industry 3.0, the goal of which was to automate work, Industry 4.0 sets its goal as autonomous operation, in which decisions and actions are undertaken autonomously in real time on the basis of data available directly in the production hall itself, thanks to different Internet of Things (IoT) or Industrial Internet of Things (IIoT) sensors, without the need of human input. This vision is possible particularly thanks to artificial intelligence, which thanks to predictive algorithms not only allows for the optimization of business processes, but also for avoiding potential defects and problems prior to their appearance (Hervas-Oliver et al., 2019 ).
BPM in Industry 4.0 is in practice closely related to the use of various ISs (Bazan & Estevez, 2022 ; Beerepoot et al., 2023 ; Mahendrawathi et al., 2017 ). They accelerate the implementation, reduce the labor intensity and the risk of error in the implementation of business processes, and provide data for their analysis and learning by the organization during and after the implementation of business processes (van der Aalst et al., 2016 ). The effectiveness of IS support for the implementation and improvement of business processes depends on the scope, detail, and reliability of data available to them (Zirar et al., 2023 ). In particular, this applies to the increasingly used and important intelligent IS containing AI elements. The lack of data, their fragmentation, or inconsistency with the actual course of business processes immediately increases the risk of incorrect learning for the AI, and thus of errors in the support of managers by IS (Pery et al., 2022 ). The consequence is that managers make and implement wrong decisions that have negative economic consequences (Mariani et al., 2023 ). Therefore, a prerequisite for the effectiveness of IS support for BPM is the possession of reliable data on the course and context of the implementation of processes, such as the execution time of individual tasks, the time interval between the execution of individual tasks, the involvement of working and waiting resources, waiting times for resources to be made available etc. It should be emphasized that this condition has become particularly important with the start of the use of intelligent IS.
ISs supporting the implementation of business processes normally use data collected in event logs in the Implementation and Execution BPM Lifecycle phases, based on information entered by employees servicing them and information automatically provided by sensors, e.g. Internet of Things (IoT) / Industrial Internet of Things (IIoT) (Dumas et al., 2018 ; Ghosh et al., 2022 ; Sestino et al., 2020 ; Szelągowski, 2019 ). In the case of intelligent IS, the effectiveness of support for the implementation of business processes is additionally influenced by the availability and reliability of data contained in the BigData sets used, describing the current execution context, the course and results of previous executions of the process, or other data having at least an indirect impact on the implementation of the process. ISs are not able to create this data on their own, or even refine the data they already have (Zirar et al., 2023 ).
Earlier attempts to digitalize the observation of work
In past years, researchers and practitioners have taken several initiatives to digitalize work sampling analysis. Snapshot analysis is a method in which data samples are collected at fixed time intervals (snapshots). This technique has wide applications in many fields of science, such as: finance, industrial production, healthcare, and sociology. In Industry 4.0, thanks to different sensors including those of the Internet of Things (IoT) or the Industrial Internet of Things (IIoT), data may be collected automatically and autonomously in real time in virtually any small-time intervals. For example, data from sensors in CNC machining centers or electroencephalographic (EEG) or electrocardiographic (ECG) signals can be collected automatically (and autonomously) in real time, without virtually any noticeable delays.
One of the earliest solutions applied to researches focused on the digitalization of the work sampling method used a Personal Digital Assistant (PDA), a piece of equipment that allows one to input information in digital form. The PDA was adopted to work sampling to replace the paper form. That solution no doubt has two main benefits. It collects data in fully digital form and significantly reduces the time required to prepare the gathered information for the following phase, namely analysis. A second benefit is the possibility to automatically register a timestamp for each entered activity. The description of a case discussed by Robinson ( 2009 ) presents an implementation of a PDA in work sampling. The practical aspects of this implementation shoved clearly some key weaknesses of this new method. The most important one, and the one which can be a subject for a separate discussion, is a problem of who is responsible for entering information into a PDA form. If this role is assigned to a worker whose performance is subject to measurement, then two additional questions arise. Are those data trustful when an observer performs an observation? How to measure the steps in a given process when the measurement method itself is causing the extension of the list of activities conducted by the observed persons? A more general overview of the PDA work sampling method is presented by Groover ( 2014 ), where the division of roles in the observation method is more traditional. The PDA and the electronic form installed on it replaces paper and still remain in the researcher's or analyst's hands. Another separate type of solution was described in Knoch et al. ( 2018 ). The proposed approach to work sampling digitalization covers much more advanced technologies then mentioned above in the case of a PDA. The new method uses hardware like microsensors and micro cameras. This approach can certainly deliver very detailed information about each and every activity conducted in a measured process however it has its own list of limitations. Sensors proposed in this method are designed as wearable equipment, which makes this measurement process fully automated, but also strongly interferes with a given workplace. Another important barrier pertains to the cost and effort involved with the implementation of this particular method.
Luoa et al. ( 2018 ) proposed a video analysis solution to digitalize a work sampling analysis implemented in a skyscraper’s construction project. The recommended solution is based on video registration hardware which is placed above the construction area. The captured video file is divided into separate frames. And then a predefined image analysis algorithm is executed to detect the location of workers taking part in a given process. The proposed method of digitalization of work sampling is designed not only to locate workers but assign them to the execution of particular activities. This part of the analysis is possible to conduct thanks to small changes in a workplace. Employees are assigned to different roles and activities in a given construction process. This assignment is communicated by a separate color. This small change, which minorly interferes which the natural flow of the process, makes this method a very promising direction for further development.
It has to be stated in short that out of the three methods of work sampling digitalization presented above, the last one is the one which interferes the least in an observed process and is the most efficient.
Workplace performance measurement
Workplace Performance Measurement (WPM) is a proprietary managerial tool, the purpose of which is to enable the collection of data on work performance and their automatic analysis without interfering with the work itself. It was developed in response to the challenges faced primarily by managers in production companies. The WPM consists of the methodology of operating in the tested environment and intelligent IS offering the possibility of workplace performance analysis. This enables researchers and managers using the WPM to flexibly respond to emerging limitations and barriers. The WPM can be applied in organizations of all sizes, regardless of industry, to collect data which can help to solve problems appearing in”scheduling in discrete manufacturing systems” (Grabot & Letouzey, 2000 ). These systems require access to good quality data defining standard times of activities in production processes, and the WPM can be implemented to provide that data.
The WPM method for improving business processes
The WPM allows you to collect and analyze digital traces of actually implemented processes. The precision and level of detail of the data it collects is much greater than those of data recorded in IS event logs, because the WPM allows for the collection of data and subsequent analysis of activities that are not usually recorded in IS event logs. These may be, by their nature, unpredictable activities related to the work being performed or activities not related to the ongoing process at all. By way of an example:
Conversations during ad hoc arranged informal breaks at work,
Smaller or larger breaks caused by failures or technical breaks at work,
Additional actions caused by defects or material shortages,
Additional ad hoc activities related to the configuration or calibration of tools,
Short breaks related to waiting for interactions with other employees or processes, e.g. waiting for semi-finished products delivered by other teams or processes.
The WPM is not based on predicting the course of processes, but based on the analysis of recorded images and data from IS event logs, it allows you to build a precise model of the implemented process. The results of the analysis of such a model are fully consistent with the actual situation and definitely more reliable than the results of analyzes or predictions based on IS event logs.
The WPM method for improving business process follows the following sequence of tasks (Fig. 1 ):
Selection of a process to be analyzed,
Setup of parameters which should be registered in a workspace and setup of cameras, sensors, and registrators,
Video registration of activities conducted during production process,
Digitalization and analysis of acquired video content by the WPM tool and matching acquired data with process parameters,
Discussion and assessment of proposed changes and development of a recommendation,
Implementation of recommended changes into analyzed process,
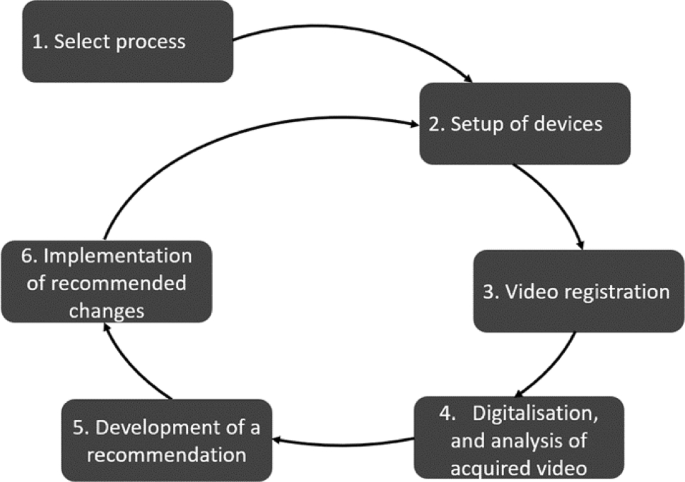
The WPM method (WPM) for improving business process
The above-mentioned improvement process can be repeated many times for a selected process or for a group of processes.
The first element of the method results in the selection of a process and a definition of a list of business process parameters which will be analyzed. In the second step, sensors are selected such as cameras, thermometers, light intensity sensors, ECG, or eye trackers (Nesterak & Nesterak, 2021 ). The selection of proper sensors is determined by a scope of the analysis and type of process. For manufacturing processes, the first-choice sensor is a camera. Other sensors could be added to the list when researchers are looking for more data to help answer questions related to potential factors or variables affecting some of the anomalies appearing in an analyzed process in a particular workspace.
A second element of the WPM method is the preparation of a definition of the process parameters in the WPM tool. Of most importance is a list of tasks or activities defining a process flow. Other parameters which should be taken under consideration are types and categories of tasks or conditions of the workspace, such as temperature and tools. The last element of the WPM method is a process conducted in the WPM software, where data collected via sensors and registration are merged with definitions of process parameters. As shown in Fig. 2 , sensor data (e.g., parameters of product components at the workplace) are dynamically detected and captured during the survey. The presented screenshot from the dashboard generated by the WPM tool shows a set of charts presenting changes over time in parameter values recorded by sensors selected for a specific study. The idea behind the WPM tool is to provide researchers with the flexibility to determine which of the sensors supported by the WPM are best suited to their planned research project. Therefore, some of the sensors not selected for the presented sample study do not collect data and do not display any values on the dashboard in Fig. 2 .
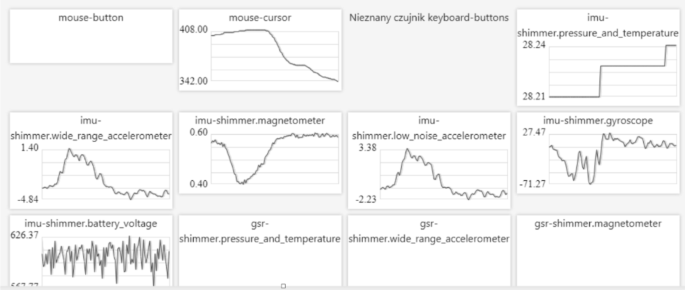
Dynamic detection and recording of product element parameters
As an outcome of this element, the WPM delivers analyses and reports presenting a detailed picture of workspace effectiveness. The novelty of this method is that it was applied to processes without a digital footprint to collect digital data that can be used to conduct multidimensional Process Mining analysis. The other not less important new value is that thanks to this method it is possible to extend the period of measurement without the necessity to increasing the costs of the project.
The WPM method allows us to measure the time of usage of detected resources and then calculate the acquired values into other metrics like cost or energy efficiency, but to also add other measurements to the same timeline. The WPM is an open method that can use other sensors such as: ECG, temperature, or eye trackers, which provide more detailed information, allowing researchers and managers to more precisely assess the effectiveness of a process conducted in particular workspace. The WPM method consists of procedures dedicated to:
Configuring of video registrations in particular in a manufacturing workplace,
Configuring of work sampling analysis form,
Conducting work sampling analysis,
Configuring of a machine learning component.
Due to the fact that each manufacturing workspace can differ from others even in the same company, the mentioned procedure is more like a list of best practices then a precise manual containing a list of steps to follow.
Phases of the WPM implementation project
The primary purpose of using the WPM is to analyze the implementation and streamline the business process. The WPM method consists of two phases of process improvement initiatives: Phase I Analysis and Phase II Automatic controlling (Fig. 3 ).
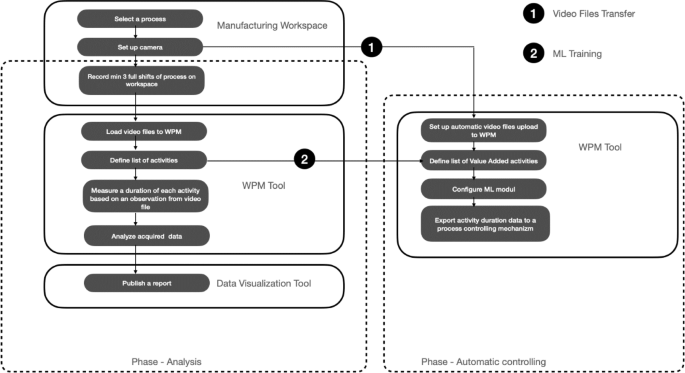
Source: results of own research
Schema of an implementation of the WPM method.
Phase I Analytics presented on Fig. 3 is focused on research, discovery, and analysis of workspace activities of the analyzed process. The result of this phase is a report. This phase consists of three elements:
Research setup and information collection – provided mostly in manufacturing workspace,
Information processing and data extraction – processed by the WPM tool,
Report with insight and recommendation delivery with support of the Data Visualization tool.
The purpose of this phase is to analyze current flow and performance of an as-is process to discover inefficiencies, better understand their reasons, and finally create a list of recommended changes in a process and workspace organization, as well as tools and resource configuration.
Phase II Automatic controlling of WPM implementation is dedicated to supporting modern controlling mechanisms, which in a fully automated manner monitor if process performance meets planned values or KPIs. Human engagement in this phase is significantly reduced to the setup of data registration tools (including video) and to the configuration of a ML module. ML setup is a step where the person responsible for controlling mechanism implementation has to select one of the algorithms, which best detects Value Added Activities. Figure 4 presents a visual example of one of those defined algorithms. Each video frame captured by the camera is automatically analyzed to detect specific parameters of an image. The idea behind this particular ML solution is to apply for each workspace algorithm and image analysis option which the best fits to particular process. The image with huge number of green lines it is just a graphical representation of number of elements detected by an algorithm on analyzed frame from captured video file. What an algorithm is focused on is a dynamic of changed values. The algorithm calculates quantity of appearances of key parameters on each frame and compares values between captured frames.
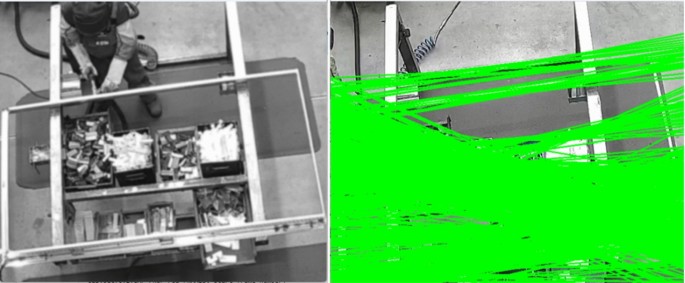
ML video parameters detection executed controlled by algorithm
Functionality of the WPM tool
Functionality of the WPM tool is offered as set of modules. Each of the mentioned modules is responsible for serving a different element of the WPM method (Fig. 5 ).
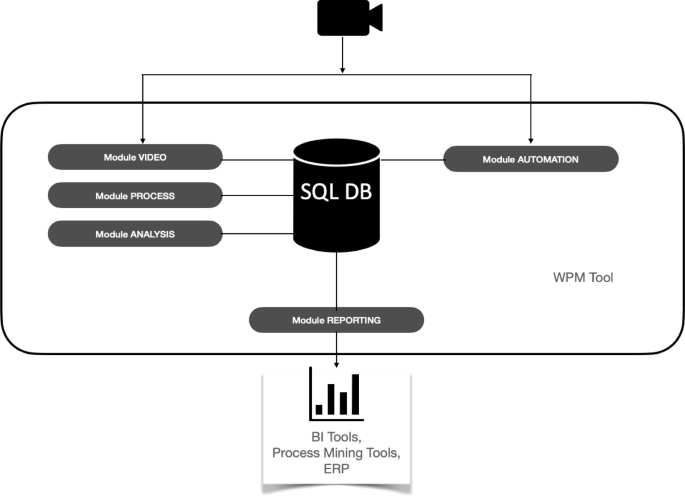
WPM modules.
The WPM tool consists of the following modules:
Module VIDEO allows us to define a new workspace and to upload registered video files. This module offers a mechanism to configure a list of required sensors or registrations, which the analysis should be equipped with. This is a form with a list of hardware and software that can be added to an observation.
Module PROCESS is dedicated to the configuration of process parameters. It allows us to create a list of activities of which the process consists of. In the same module it is possible to add a set of categories of activities. The most important feature of this module is its flexibility. Each initially created list of activities can be modified at any given moment when the analyst finds during an observation that additional activity has to be added to cover the real flow of the process.
Module ANALYSIS performs the role of transforming work observations into digital data structured in an event log form. By using a form containing the list of process steps, an analyst can assign to each repeated activity a start and end timestamp. The pace of observation can be controlled by the analyst by setting up a video player speed parameter. An analytical module allows us to merge data from WPM observations with data extracted from other systems.
Module AUTOMATION is a machine learning functionality that has been designed to let the WPM solution detect a begging and an end of each repeated value-added activity in the process. Detected values are automatically stored in a database. The outcome of this module can be displayed either in the third module or in any tool dedicated to data processing.
Module REPORTING is a set analytical mechanism to deliver datasets for reports enriched by the value of insights.
Data from all modules are saved and available for further analysis in the SQL database. The WPM limits the size of the required database by only collecting changes in sensor states. All analyses are designed and performed on views, not on separate and dedicated analytical and reporting tables. The mentioned mechanisms let us explore the effectiveness of workspace resources applying several metrics linked to the same unified timeline. Standard video registration can be combined with thermometers, ECG, or eye trackers to deepen the impact of other factors on work efficiency.
One of the most important assumptions which has been made by a team of researchers is the data analysis component. It has been decided to not develop any kind of new, sophisticated data analysis and visualization components. The data related output of the module is a high value dataset, which can be integrated with:
Most of Business Intelligence (BI) tools currently available on the market,
Process Mining solutions,
Machine Learning solutions.
The dataset is equipped with not only acquired metrics describing observed processes, but with a full range of dimensions provided by the WPM in the configuration phase.
The digitalization of work monitoring by the WPM allows for the elimination of most of the limitations of the hitherto used methods of work monitoring and measurement. The observation of work is not carried out by dedicated observers, so it does not affect the way the work is carried out, and at the same time it does not require additional training of the individuals conducting the observation. Conducting the observation does not burden the people carrying out the work with additional activities, such as filling out paper questionnaires or entering data into the IS. Image registration and analysis means that the resolution of the analyzed events depends on the configuration of the analytical module. The method of analysis used in the WPM allows fort the collection of data with a precision of even 0,25 of a second, and raising the density of the collected data in no way hinders the work itself, as it does not require breaks in work caused by the need to enter data. Data on all elements of work performance observed by the WPM are obviously analyzed, including those that were not previously recorded in observation questionnaires or IS logs, e.g. dynamic interpersonal interactions, overcoming additional obstacles, performing activities not provided for in the standard work plan, work breaks etc. Full data on process execution are registered. They do not undergo subjective selection by process executors or other stakeholders. The registered data are automatically provided with timestamps and workplace identifiers, which allows the analysis to automatically track mutual relations between processes carried out in different locations in the company or between the company and its contractors. Of course, the analysis of the data provided by the WPM may also include data from the event logs of selected ISs that affect the course of the processes being carried out. The WPM provides data whose scope and detail allows for the elimination or at least minimization of most of the limitations of process mining. In this way, it allows for the establishment of the actual process workflow, including reliable analysis of why a given action lasts longer than planned or expected. Thanks to the possibility of analysing data outside of SI logs themselves, it also enables the analysis of not only traditional, predictable business processes, but also unpredictable and often unique business processes that require dynamic management. By offering access to a broad spectrum of reliable data, which is not the result of subjective selection, the WPM may be a source of data for any AI methodologies using ML to improve business processes (Pery et al., 2022 ).
Workplace performance measurement implementation case study
The aim of the WPM implementation project presented below was to provide managers of a production company with a tool to track and digitalize data on the implementation of production processes that require manual work, as well as to develop and implement their improvements. The described researched project has been conducted in close cooperation with a private manufacturing company located in the south part of Poland, specializing in processing steel and delivering products to the construction industry. One of the key production processes that require manual labor in this company is the process of welding elements. Despite noticing its low efficiency, the management staff was unable to plan its improvement without reliable, accurate, and up-to-date data describing its actual course. The issue also had a significant negative impact on several other production and management processes, including ongoing production planning and price calculations. The lack of visibility of the actual course of activities in the process and abnormal times did not allow for the implementation of effective planning activities and caused turbulence in the calculation of costs and margins.
The situation faced
The management of the company was definitely aware of unsatisfactory efficiency and fluctuations in execution time, and the resulting need to streamline and stabilize the process. To achieve this, it had to overcome problems such as:
Lack of a business process flows related data which could be used to support improvement initiatives,
Lack of information permanently flowing to controlling mechanisms, which supports the management of production planning processes,
Lack of access to on-line data presenting production process performance.
To provide a full picture of the challenge, it is necessary to better describe production workplaces that the company managed during the time when the team of researchers conducted the project. Majority of tasks conducted in those workplaces required manual effort. Workers have been equipped with specialized tools such as welders, but there were no options to install dedicated equipment to register timestamps for the beginning and end of relevant tasks.
In the mentioned production environments, some ways of controlling activities have been implemented and occasionally executed. During interviews conducted with process owners, it was identified that the most common measurement method to collect data from workplaces was work sampling. The organization was aware of the weaknesses and limitations of the mentioned method. The main weaknesses highlighted by managers are quality of data, form of data, costs and frequency of the method. The company clearly stated that will not invest in any specialized, expensive, dedicated measurement solutions. The expected output of the project was to define as two objectives:
The design and implementation of a digital version of work sampling, which could eliminate the weaknesses of the standard method and deliver valuable insight for process improvement teams.
The design and implementation of a solution which could constantly and fully automatically deliver digital information to a controlling mechanism supporting production planning management.
Action taken
The implementation of the task described above involved the use of the Process Redesign method processed by Davenport and Short ( 1990 ) (Dumas et al., 2018 ; Hanafizadeh et al., 2009 ). This method is dedicated to the ongoing improvement of business processes on the basis of experiences derived from their execution (Fehrer et al., 2022 ; Wastell et al., 1994 ). This method was described as set of steps conducted by companies which successfully implemented Business Process Redesign with support of Information Technologies (Kubrak et al., 2023 ; Palma-Mendoza et al., 2014 ). This method consists of five observed steps:
Develop Business Vision and Process Objectives,
Identify Processes to be Redesigned,
Understand and Measure Existing Processes,
Identify IT Levers, and
Design and Prototype Process.
The mentioned method was applied to solve both identified problems related to the lack of valuable, trustful information required by the controlling processes. Following the Business Process Redesign method steps, the team created a simple table containing relevant answers (Table 1 ).
Following the steps presented above, the team has decided to build a prototype of a digital version of a work sampling form and conduct research on video files registered in the regular manufacturing environment. The first set of materials analyzed with a use of prototype confirmed the high value of the designed solutions and delivered information which helped to create a list of valuable improvements.
Further analysis became a source of data that fully answered the requirements defined by the management team of the manufacturing company. Standard times calculation and detection of inefficiencies in process flows became the first two gains which the researched solution delivered to the company. The usage of video files in the semi-automatic solution showed that traditional process of work sampling measurement transformed with the support of Information technology applied in a manufacturing environment to processes without digital footprint can significantly improve controlling mechanisms.
The second challenge in the project required us to find a solution which could work in the fully automatic mode. In order to design and implement an automated controlling solution using the WPM tool, the 5-step Process Redesign method was used in the same way as before. It led the team to design and implement a solution which requires incorporation of an effective ML algorithm to conduct image analysis. As a result of the conducted analyses, it was agreed that in the constantly executed mechanism of acquiring data for controlling purpose solution should be focused on a limited number of process steps or phases or, from another perspective, should focus on the statuses of a product flowing in a particular process. As a consequence of the taken assumptions, a prototype has been developed and tested on video files containing recordings from over 20 h of manufacturing process conduction. To validate and assess the accuracy of the applied algorithms, the team could reuse data from the previously conducted work sampling method. The purpose of this assessment process was to verify if all targeted statuses were detected by ML algorithms. In general, one hour of video content covering up to 20 repetitions of the observed process was enough to train the system.
In contrast to phase one of the project, where the WPM has been used to measure and analyze all types of activities in production process, phase two followed a different approach. To achieve effective results by a design solution focused on supporting the controlling mechanism in the manufacturing process, the authors decided, according to the LEAN methodology, to measure only these activities or steps which are classified as adding value (meaning: relating to operations related to the creation or logistics of the product). It was a conscious decision to not measure all other types of activities. Calculated distance between two consecutive value adding steps can be treated as a time dedicated to non-value adding activities.
Results achieved
There are at least two perspectives that should be applied to summarize and assess the results of the completed project. Those perspectives are:
The business value of the designed and implemented solution,
The scientific value of the proposed solution.
It is stated that in the first area of assessment the results achieved can be declares as positive. The digital version of the work sampling measurement method has proven its business value by delivering the required information to support managerial processes in the manufacturing environment. Collected data from the observed workplace confirmed its significant value not only because of their high quality (resolution, limited number of errors) but due to the structure and shape of the dataset as well. The reported results of the analysis helped to detect weaknesses of the process and define a set of new values of standard times for key activities. The short-term benefits of the first phase of WPM implementation focused on:
Finding inefficiencies in the process, mainly in detecting and measuring activities that do not provide added value,
Recommendations for changes in the course of the process, such as the division of tasks between skilled and unskilled employees,
Data collection to determine new standard times, which improved the quality of the planning process.
A measurable benefit of the first phase of the project is the significant improvement in the KPI values. Process Effectiveness KPI, calculated as a duration of time spent by welders on value added activities vs total contracted time, has been selected as a most basic one. Another KPI was Number of Frames Assembled per Hour. After implementing the recommended improvements, the organization achieved an increase in the value of KPIs of Process Effectiveness, which rose from 60% before the implementation of WPM to over 90%.
Thanks to the WPM, it was possible to identify the reasons behind workers leaving the workspace. It was caused by the design of the process, where welders were responsible for the transport of finished products to the warehouse. Calculation of the total duration of this type of activity highlighted how inefficient the mentioned design was. 30% of productive time lost for non-added value activity.
The long-term benefit of the project is the implementation of a solution based on ML, which continuously, in a fully automated way, provides data allowing for constant monitoring of key parameters of the production process without interfering with its course. The solution provides data to the controlling tool as well as to the production planning tool. The quality, accuracy, and structure of the acquired data is often used to support process improvement initiatives and to assess the effects of changes. The accuracy of automated detection of product state (begin, end) on analyzed workspace reached 0.25 of a second thanks to designed and implemented algorithm.
Lessons learned
Given the second perspective om assessing the results of the project, it has to be stated that the designed solution can be used in a future by other teams to conduct research in organizations characterized by a lack of a digital footprint in manufacturing processes. In comparison with the traditional method of work sampling analysis, its improved digital alternative will allow other researchers to benefit from the high quality of data that can used in the inference process. Similarly, from scientific perspective the solution which automatically measures duration of added value steps by analyzing video file content will bring an indisputable benefit for researchers. The flexibility of the proposed algorithms and quality of data delivered from huge samples can be treated as a convincing argument.
The first phase of the project showed how important is close cooperation with a management team of a production site to properly understand the analyzed process. Process owner is a right source of knowledge which can let correctly identify and interpret the observed activities and steps. This is the most important support for the implementation of Machine Learning solution in phase two.
The team has learned that to apply automatic detection of motions in phase one, the production workspace should be equipped with more cameras, registering video from different perspectives.
Conclusions
The WPM of production processes, without interfering with the processes themselves and without increasing their labor consumption or exceeding the costs acceptable to users, enables:
The collection and analysis of data on the course of processes, to an extent that goes beyond the scope available so far (e.g. IS event log data).
The identification of errors and irregularities in the implementation of work based on the analysis of data from cameras and sensors or IS. The second accuracy of the WPM allows for detailed monitoring of production processes to detect any anomalies, whether repetitive (e.g. incorrect placement of components) or unique (e.g. mistakes in assembly).
Improvement of efficiency by identifying areas where manufacturing processes can be optimized, which can lead to better resource utilization, reduced machine downtime, and improved overall production performance.
The WPM method is devoid of most limitations and provides a more holistic picture of the analyzed process carried out in the selected work area than the existing work measurement methods, such as: work sampling, snapshot analysis, and timing and process mining. In the WPM, the entire context of the process is observed. The data collected with the accuracy of a second, covering the activities of employees and objects of the manufacturing process, also includes data usually not available in observation questionnaires or IS event logs. After all, WPM cameras also record what is happening in the environment, as well as between individual tasks. The scope of the analyzed data may include not only the image from the surveillance cameras, but also data from various sensors or IS supporting the work. Thanks to this, the results of the analysis conducted by the WPM include full data about the context and course of the process. This allows us not only to identify the activities performed, but also to identify their causes, the relationships between them, the involvement of individual participants, the impact of the availability or unavailability of resources etc. The WPM enables a detailed analysis of the effectiveness of the activities carried out. It also provides the opportunity to optimize them by showing the observed anomalies, inefficiencies, or wasted time, and the reasons for their occurrence. Thanks to continuous observation and analysis, the WPM allows for a quick response to identified errors and anomalies, and thus reduces the costs associated with their re-execution or repairs. The digital form of data and analyses provided by the WPM enables their further use as the basis for AI operation for analysis and optimization of work, including in real time.
The WPM method reduces the gap identified in available scholarship, particularly with respect to practical solutions enabling the collection of data on the actual flow of business processes, without having to disrupt them or raise their labor-intensity. The breakthrough nature of the WPM lies in the fact that it eliminates the limitations of the existing work measurement methods resulting from the limited scope of data. What is more, while earlier methods focus on detecting processes and anomalies, the WPM allows you to understand the factors and causes of their formation at the same time. These can be various factors that are not visible in traditional work sampling or IS event logs, and therefore are not visible in snapshot analysis, timing, or process mining reports. The quality and ergonomics of tools, environmental parameters, the condition of employees and their level of stress, seniority and the level of skills of the employee, method of communication, or rules for coordinating the tasks performed are factors that play an important role in the course of the process, and therefore researchers should take them into account. The key benefit of the WPM is the wide range of measured factors represented in the workplace and the ability to integrate them as part of a holistic view of job performance.
Further development of the WPM method will, on the one hand, be aimed at expanding the set of process efficiency factors, defining the method of their identification and defining their potential impact on the efficiency of business processes. These are factors that have an indirect, often hidden impact on the outcome of the work. It is usually omitted in the analyses, although it does not have to be negligible. The WPM provides researchers with great opportunities to discover how various combinations, including those usually beyond the scope of factor analysis, can determine the effectiveness of the process carried out in the workspace.
The second direction of future work will be to expand the use of AI. Work is planned to support the WPM's decision-making during work execution, optimization, and simulation of selected variants of process implementation based on the analysis of collected data, predictive analyses enabling prediction of future trends and proactive preparation for upcoming challenges. So the WPM could also be the answer or part of an answer to new challenges posed by emerging ideas generated by requirements like “an irregular demand using annualized hours.”
The limitation of the presented solution is the lack of verification of a larger number of various business processes. This causes a limitation in the available set of standard WPM tool configurations, including elements using AI. The article also does not refer to the necessary solution to ethical issues related to image capture and continuous assessment of human resources performance.
Data availability
The datasets generated during and analyzed during the current study are not publicly available due because of their confidentiality, but are available from the corresponding author on reasonable request.
Badakhshan, P., Wurm, B., Grisold, T., Jerome Geyer-Klingeberg, J., Mendling, J., & vom Brocke, J. (2022). Creating business value with process mining. The Journal of Strategic Information Systems, 31 (4), 101745. https://doi.org/10.1016/j.jsis.2022.101745
Article Google Scholar
Balaila, I., & Gilad, I. (2012). A manpower allocation model for service jobs. International Journal of Service Science Management Engineering and Technology, 3 (2), 13–34. https://doi.org/10.4018/jssmet.2012040102
Bartlett, L., Kabir, M., & Han, J. (2023). A review on business process management system design: the role of virtualization and work design. IEEE Access, 11 , 116786–116819. https://doi.org/10.1109/ACCESS.2023.3323445
Bazan, P., & Estevez, E. (2022). Industry 4.0 and business process management: state of the art and new challenges. Business Process Management Journal, 28 (1), 62–80. https://doi.org/10.1108/BPMJ-04-2020-0163
Beerepoot, I., Di Ciccio, C., Reijers, H. A., Rinderle-Ma, S., Bandara, W., Burattin, A., & Zerbato, F. (2023). The biggest business process management problems to solve before we die. Computers in Industry, 146 , 103837. https://doi.org/10.1016/j.compind.2022.103837
Bispo, G., Relim, T.E., Reis, A.C., Mariano, A.M., Ladeira, M., & Serrano, A.L. (2019). Measurement of maturity in process management in the Brazilian public sector: a multicriteria approach. In K. Soliman (Ed.), Vision 2025: Education Excellence and Management of Innovations through Sustainable Economic Competitive Advantage , Vol. 1, presented at the 34th International Business Information Management Association Conference (IBIMA 2019), Madrid.
Bounfour, A. (2016). Digital futures, digital transformation from lean production to acceluction . Springer-Verlag. https://doi.org/10.1007/978-3-319-23279-9
Book Google Scholar
Choi, D., R’bigui, R., & Cho, C. (2022). Enabling the gab between RPA and process mining: user interface interactions recorder. IEEE Access, 10 , 39604–39612. https://doi.org/10.1109/ACCESS.2022.3165797
Davenport, T., & Short, J. (1990). The new industrial engineering: Information technology and business process redesign. Sloan Management Review, 31 (4), 11–27.
Google Scholar
Dumas, M., La Rosa, M., Mendling, J., & Reijers, H. (2018). Fundamentals of business process management (2nd ed.). Springer. https://doi.org/10.1007/978-3-662-56509-4
European Commission, Directorate-General for Research and Innovation. (2021). Industry 5.0: towards a sustainable, human-centric and resilient European industry . https://doi.org/10.2777/308407
Fehrer, T., Fischer, D., Leemans, S., Röglinger, M., & Wynn, M. (2022). An assisted approach to business process redesign. Decision Support Systems . https://doi.org/10.1016/j.dss.2022.113749
Ghosh, S., Hughes, M., Hodgkinson, I., & Hughes, P. (2022). Digital transformation of industrial businesses: A dynamic capability approach. Technovation, 113 , 102414. https://doi.org/10.1016/j.technovation.2021.102414
Gilbreth, F., & Kent, R. (1911). Motion study . Constable.
Grabot, B., & Letouzey, A. (2000). Short-term manpower management in manufacturing systems: New requirements and DSS prototyping. Computers in Industry, 43 (1), 11–29. https://doi.org/10.1016/S0166-3615(00)00058-0
Groover, M. (2014). Work Systems: The Methods, Measurement and Management of Work . Pearson Education Limited.
Hanafizadeh, P., Moosakhani, M., & Bakhshi, J. (2009). Selecting the best strategic practices for business process redesign. Business Process Management Journal, 15 (4), 609–627. https://doi.org/10.1108/14637150910975561
Helbin, T., & Van Looy, A. (2019). Business Process Ambidexterity and its impact on Business-IT alignment: A Systematic Literature Review. In13th International Conference on Research Challenges in Information Science (RCIS), pp. 1–12. https://doi.org/10.1109/RCIS.2019.8877073
Hermann, M., Pentek, T., & Boris, O. (2016). Design Principles for Industrie 4.0 Scenarios: A Literature Review. Working Paper No. 01/2015, Dortmund: Technische Universität Dortmund Fakultät Maschinenbau.
Hervas-Oliver, J., Estelles-Miguel, S., Mallol-Gasch, G., & Boix-Palomero, J. (2019). A place-based policy for promoting Industry 40: The case of the Castellon ceramic tile district. European Planning Studies, 27 (9), 1838–1856. https://doi.org/10.1080/09654313.2019.1642855
IEEE Task Force on Process Mining. (2012). Process Mining Manifesto . Retrieved October 22, 2023, from https://www.tf-pm.org/resources/manifesto
Jans, M. (2012). Process mining in auditing: From current limitations to future challenges. In F. Daniel, K. Barkaoui, & S. Dustdar (Eds.), Business Process Management Workshops. BPM 2011 . Lecture Notes in Business Information Processing, Vol. 100. Springer, Berlin, Heidelberg. https://doi.org/10.1007/978-3-642-28115-0_37
Knoch, S., Herbig, N., Ponpathirkoottam, S., Kosmalla, F., Staudt, P., Fettke, P., & Loos, P. (2018). Business process management workshops, BPM 2018 International Workshops Sydney, NSW, Australia, September 9–14, Revised Papers.
Kubrak, K., Milani, F., & Nava, J. (2023). Digital technology-driven business process redesign: A classification framework. In S. Nurcan, A. Opdahl, H. Mouratidis, & A. Tsohou (Eds.), Research challenges in information science: Information science and the connected world: RCIS 2023—Lecture notes in business information processing. (Vol. 476). Cham: Springer. https://doi.org/10.1007/978-3-031-33080-3_13
Chapter Google Scholar
Kumar, S., & Strehlow, R. (2004). Business process redesign as a tool for organizational development. Technovation, 24 (11), 853–861. https://doi.org/10.1016/S0166-4972(02)00182-7
Lamghari, Z., Radgui, M., Saidi, R., & Rahmani, M. D. (2021). An operational support approach for mining unstructured business processes. Revista De Informática Teórica E Aplicada, 28 (1), 22–38. https://doi.org/10.22456/2175-2745.106277
Lasi, H., Fettke, P., Kemper, H. G., Feld, T., & Hoffmann, M. (2014). Industry 4.0. Business & Information Systems Engineering, 6 , 239–242. https://doi.org/10.1007/s12599-014-0334-4
Löhr, B., Brennig, K., Bartelheimer, C., Beverungen, D., & Müller, O. (2022). Process mining of knowledge-intensive processes: An action design research study in manufacturing. In C. Di Ciccio, R. Dijkman, A. del Río-Ortega, & S. Rinderle-Ma (Eds.), Business process management: BPM 2022—Lecture notes in computer science. (Vol. 13420). Cham: Springer. https://doi.org/10.1007/978-3-031-16103-2_18
Lorenz, R., Senoner, J., Sihn, W., & Netland, T. (2021). Using process mining to improve productivity in make-to-stock manufacturing. International Journal of Production Research, 59 (16), 4869–4880. https://doi.org/10.1080/00207543.2021.1906460
Lu, Y. (2017). Cyber physical system (CPS)-based industry 4.0: A survey. Journal of Industrial Integration and Management, 2 (3), 1750014. https://doi.org/10.1142/S2424862217500142
Luoa, X., Lia, H., Caob, D., Yua, Y., Yanga, X., & Huanga, T. (2018). Towards efficient and objective work sampling: Recognizing workers’ T activities in site surveillance videos with two-stream convolutional networks. Automation in Construction, 94 , 360–370. https://doi.org/10.1016/j.autcon.2018.07.011
Mahendrawathi, E., Zayin, S., & Pamungkas, F. (2017). ERP post implementation review with process mining: A case of procurement process. Procedia Computer Science, 124 , 216–223. https://doi.org/10.1016/j.procs.2017.12.149
Mamudu, A., Bandara, W., Leemans, S., & Wynn, M. (2023). A process mining impacts framework. Business Process Management Journal, 29 (3), 690–709. https://doi.org/10.1108/BPMJ-09-2022-0453
Mans, R. S., van der Aalst, W., Vanwersch, R. J., & Moleman, A. J. (2013). Process mining in healthcare: Data challenges when answering frequently posed questions. In R. Lenz, S. Miksch, M. Peleg, M. Reichert, D. Riaño, & A. Teije (Eds.), Process support and knowledge representation in health care: ProHealth KR4HC 2012—Lecture notes in computer science. (Vol. 7738). Berlin, Heidelberg: Springer. https://doi.org/10.1007/978-3-642-36438-9_10
Marchewka, M., Nesterak, J., Sołtysik, M., Szymla, W., & Wojnarowska, M. (2020). Multitasking effects on individual performance: An experimental eye-tracking study. European Research Studies Journal, 23 (1), 107–116. https://doi.org/10.35808/ersj/1539
Mariani, M., Machado, I., Magrelli, V., & Dwivedi, Y. (2023). Artificial intelligence in innovation research: A systematic review, conceptual framework, and future research directions. Technovation, 122 , 102623. https://doi.org/10.1016/j.technovation.2022.102623
Maynard, H. B., Stegemerten, G. J., & Schwab, J. (1948). Methods-time measurement . McGrow-Hill Book Company Inc.
Mendling, J., Pentland, B., & Recker, J. (2020). Building a complementary agenda for business process management and digital innovation. European Journal of Information Systems . https://doi.org/10.1080/0960085X.2020.1755207
Munoz-Gama, J., Martin, N., Fernandez-Llatas, C., Johnson, O., Sepúlveda, M., & Zerbato, F. (2022). Process mining for healthcare: Characteristics and challenges. Journal of Biomedical Informatics . https://doi.org/10.1016/j.jbi.2022.103994
Nesterak, J., & Nesterak, N. (2021). Eye-tracking w zastosowaniach naukowych i komercyjnych (Eye-tracking in scientific and commercial applications). In A. Jaki & B. Ziębicki (Eds.), Wiedza – Gospodarka -Społeczeństwo: Wyzwania współczesnych organizacji i gospodarek (Knowledge–Economy–Society: Challenges of contemporary organizations and economies) (pp. 35–47). Kraków: INE PAN.
Nesterak, J., & Siudy, J. (2020). Automatyzacja analizy migawkowej w przedsiębiorstwie produkcyjnym. In J. Nesterak & B. Ziębicki (Eds.), Wiedza – Gospodarka – Społeczeństwo: Społeczno-gospodarcze uwarunkowania rozwoju współczesnych organizacji (pp. 163–174). Toruń: Towarzystwo Naukowe Organizacji i Kierownictwa – Dom Organizatora.
Nguyen, H., Dumas, M., La Rosa, M., Maggi, F., & Suriadi, S. (2016). Business process deviance mining: Review and evaluation. Arxiv . https://doi.org/10.48550/arXiv.1608.08252
Novak, C., Pfahlsberger, L., Bala, S., Revoredo, K., & Mendling, J. (2023). Enhancing decision-making of IT demand management with process mining. Business Process Management Journal, 29 (8), 230–259. https://doi.org/10.1108/BPMJ-12-2022-0631
Oztemel, E., & Gursev, S. (2020). Literature review of Industry 4.0 and related technologies. Journal of Intelligent Manufacturing, 31 , 127–182. https://doi.org/10.1007/s10845-018-1433-8
Palma-Mendoza, J., Neailey, K., & Roy, R. (2014). Business process re-design methodology to support supply chain integration. International Journal of Information Management, 34 (2), 167–176. https://doi.org/10.1016/j.ijinfomgt.2013.12.008
Park, G., Benzin, J., & van der Aalst, W. M. (2022). Detecting context-aware deviations in process executions. In C. Di Ciccio, R. Dijkman, A. del Río-Ortega, & S. Rinderle-Ma (Eds.), Business process management forum: BPM 2022—Lecture notes in business information processing. (Vol. 458). Cham: Springer. https://doi.org/10.1007/978-3-031-16171-1_12
Pena, J., Bravo, A., & Resinas, M. (2022). BPM in Digital Transformation: New Tools and Productivity Challenges. In Business Process Management: 20. Conference BPM 2022 , Münster, Sep. 11–16, Proceedings, pp. 21–26. Cham: Springer International Publishing.
Pery, A., Rafiei, M., Simon, M., & van der Aalst, W.M. (2022). Trustworthy Artificial Intelligence and Process Mining: Challenges and Opportunities. In J. Munoz-Gama, & X. Lu (Eds.), Process Mining Workshops. ICPM 2021 . Lecture Notes in Business Information Processing, vol 433. Springer, Cham. https://doi.org/10.1007/978-3-030-98581-3_29
Robinson, A. (2009). Work sampling: Methodological advances and new applications. Human Factors and Ergonomics in Manufacturing & Service Industries, 20 , 42–60. https://doi.org/10.1002/hfm.20186
Schwab, K. (2016). The fourth industrial revolution . World Economic Forum.
Sestino, A., Prete, M., Piper, L., & Guido, G. (2020). Internet of things and big data as enablers for business digitalization strategies. Technovation, 98 , 102173. https://doi.org/10.1016/j.technovation.2020.102173
Smith, A. (1776). An Inquiry into the Nature and Causes of the Wealth of Nations , for Heilbroner, R.L. "The Wealth of Nations". Encyclopedia Britannica. Retrieved July 17, 2023 from https://www.britannica.com/topic/the-Wealth-of-Nations
Stein Dani, V., Leopold, H., van der Werf, J. M., & Reijers, H. (2024). Progressing from process mining insights to process improvement: challenges and recommendations. In H. Proper, L. Pufahl, D. Karastoyanova, M. van Sinderen, & J. Moreira (Eds.), Enterprise design, operations, and computing: EDOC 2023—Lecture notes in computer science (Vol. 14367, pp. 152–168). Cham: Springer. https://doi.org/10.1007/978-3-031-46587-1_9
Szelągowski, M. (2019). Dynamic BPM in the knowledge economy: Creating value from intellectual capital. Series: Lecture Notes in Networks and Systems (LNNS) 71 . Berlin/Heidelberg, Germany: Springer. https://doi.org/10.1007/978-3-030-17141-4
Szelągowski, M., Lupeikiene, A., & Berniak-Woźny, J. (2022). Drivers and evolution paths of BPMS: State-of-the-Art and future research directions. Informatica, 33 (2), 399–420. https://doi.org/10.15388/22-INFOR487
Taylor, F. W. (1911). The principles of scientific management . Harper & Brothers.
Tippett, L. (1935). Statistical methods in textile research. Part 3: A snap-reading method of making time-studies of machines and operatives in factory surveys. Journal of the Textile Institute Transactions, 26 (2), T51–T70. https://doi.org/10.1080/19447023508661637
van der Aalst, W. M. (2019a). A practitioner’s guide to process mining: Limitations of the directly-follows graph. Procedia Computer Science, 164 , 321–328. https://doi.org/10.1016/j.procs.2019.12.189
van der Aalst, W.M. (2019b). Object-centric process mining: Dealing with divergence and convergence in event data. In Proceedings of the 17th International Conference on Software Engineering and Formal Methods (SEFM 2019), LNCS, Springer-Verlag, Berlin.
van der Aalst, W., La Rosa, M., & Santoro, F. (2016). Don’t forget to improve the process! Business & Information Systems Engineering, 58 , 1–6. https://doi.org/10.1007/s12599-015-0409-x
Van Looy, A. (2021). A quantitative and qualitative study of the link between business process management and digital innovation. Information & Management . https://doi.org/10.1016/j.im.2020.103413
Vogelgesang, T., Kaes, G., Rinderle-Ma, S., & Appelrath, H. (2016). Multidimensional Process Mining: Questions, Requirements, and Limitations. In S. Espana, M. Ivanovic, & M. Savi ́c (Eds.), Proceedings of the CAiSE’16 Forum at the 28th International Conference on Advanced Information Systems Engineering , Ljubljana, Slovenia, 13–17.6.2016.
Wastell, D., White, P., & Kawalek, P. (1994). A methodology for business process redesign: Experiences and issues. Journal of Strategic Information Systems, 3 (1), 23–40. https://doi.org/10.1016/0963-8687(94)90004-3
Zirar, A., Ali, S. I., & Islam, N. (2023). Worker and workplace Artificial Intelligence (AI) coexistence: Emerging themes and research agenda. Technovation, 124 , 102747. https://doi.org/10.1016/j.technovation.2023.102747
Zonnenshain, A., & Kenett, R. (2020). Quality 4.0 -the challenging future of quality engineering. Quality Engineering, 32 (4), 614–626. https://doi.org/10.1080/08982112.2019.1706744
Download references
The authors have no relevant financial or non-financial interests to disclose. The authors did not receive support from any organization for the submitted work.
Author information
Authors and affiliations.
Department of Economics and Organization of Enterprises, Institute of Computer Science, Accounting and Controlling, College of Management and Quality Sciences, Krakow University of Economics, 27, Rakowicka St., 31-510, Kraków, Poland
Janusz Nesterak & Przemysław Radziszewski
Systems Research Institute of the Polish Academy of Sciences, Newelska 6, 01-447, Warsaw, Poland
Marek Szelągowski
You can also search for this author in PubMed Google Scholar
Contributions
All authors contributed to the study conception and design. Data collection and analysis were performed by J. Nesterak and P. Radziszewski. The first draft of the manuscript was written by J. Nesterak and M. Szelągowski and all authors commented on previous versions of the manuscript. All authors read and approved the final manuscript.
Corresponding author
Correspondence to Marek Szelągowski .
Ethics declarations
Conflict of interest.
The authors have no competing interests to declare that are relevant to the content of this article.
Research involving human and/or animals
No human or animal studies were conducted in the studies described in the article.
Informed consent
No human studies were conducted in the studies described in the article.
Additional information
Publisher's note.
Springer Nature remains neutral with regard to jurisdictional claims in published maps and institutional affiliations.
Rights and permissions
Open Access This article is licensed under a Creative Commons Attribution 4.0 International License, which permits use, sharing, adaptation, distribution and reproduction in any medium or format, as long as you give appropriate credit to the original author(s) and the source, provide a link to the Creative Commons licence, and indicate if changes were made. The images or other third party material in this article are included in the article's Creative Commons licence, unless indicated otherwise in a credit line to the material. If material is not included in the article's Creative Commons licence and your intended use is not permitted by statutory regulation or exceeds the permitted use, you will need to obtain permission directly from the copyright holder. To view a copy of this licence, visit http://creativecommons.org/licenses/by/4.0/ .
Reprints and permissions
About this article
Nesterak, J., Szelągowski, M. & Radziszewski, P. Workplace performance measurement: digitalization of work observation and analysis. J Intell Manuf (2024). https://doi.org/10.1007/s10845-024-02419-x
Download citation
Received : 02 September 2023
Accepted : 03 May 2024
Published : 29 May 2024
DOI : https://doi.org/10.1007/s10845-024-02419-x
Share this article
Anyone you share the following link with will be able to read this content:
Sorry, a shareable link is not currently available for this article.
Provided by the Springer Nature SharedIt content-sharing initiative
- Workplace Performance Measurement (WPM)
- Business process management (BPM)
- Process mining
- Machine learning (ML)
- Artificial intelligence (AI)
- Industry 4.0
- Find a journal
- Publish with us
- Track your research
The state of AI in early 2024: Gen AI adoption spikes and starts to generate value
If 2023 was the year the world discovered generative AI (gen AI) , 2024 is the year organizations truly began using—and deriving business value from—this new technology. In the latest McKinsey Global Survey on AI, 65 percent of respondents report that their organizations are regularly using gen AI, nearly double the percentage from our previous survey just ten months ago. Respondents’ expectations for gen AI’s impact remain as high as they were last year , with three-quarters predicting that gen AI will lead to significant or disruptive change in their industries in the years ahead.
About the authors
This article is a collaborative effort by Alex Singla , Alexander Sukharevsky , Lareina Yee , and Michael Chui , with Bryce Hall , representing views from QuantumBlack, AI by McKinsey, and McKinsey Digital.
Organizations are already seeing material benefits from gen AI use, reporting both cost decreases and revenue jumps in the business units deploying the technology. The survey also provides insights into the kinds of risks presented by gen AI—most notably, inaccuracy—as well as the emerging practices of top performers to mitigate those challenges and capture value.
AI adoption surges
Interest in generative AI has also brightened the spotlight on a broader set of AI capabilities. For the past six years, AI adoption by respondents’ organizations has hovered at about 50 percent. This year, the survey finds that adoption has jumped to 72 percent (Exhibit 1). And the interest is truly global in scope. Our 2023 survey found that AI adoption did not reach 66 percent in any region; however, this year more than two-thirds of respondents in nearly every region say their organizations are using AI. 1 Organizations based in Central and South America are the exception, with 58 percent of respondents working for organizations based in Central and South America reporting AI adoption. Looking by industry, the biggest increase in adoption can be found in professional services. 2 Includes respondents working for organizations focused on human resources, legal services, management consulting, market research, R&D, tax preparation, and training.
Also, responses suggest that companies are now using AI in more parts of the business. Half of respondents say their organizations have adopted AI in two or more business functions, up from less than a third of respondents in 2023 (Exhibit 2).
Gen AI adoption is most common in the functions where it can create the most value
Most respondents now report that their organizations—and they as individuals—are using gen AI. Sixty-five percent of respondents say their organizations are regularly using gen AI in at least one business function, up from one-third last year. The average organization using gen AI is doing so in two functions, most often in marketing and sales and in product and service development—two functions in which previous research determined that gen AI adoption could generate the most value 3 “ The economic potential of generative AI: The next productivity frontier ,” McKinsey, June 14, 2023. —as well as in IT (Exhibit 3). The biggest increase from 2023 is found in marketing and sales, where reported adoption has more than doubled. Yet across functions, only two use cases, both within marketing and sales, are reported by 15 percent or more of respondents.
Gen AI also is weaving its way into respondents’ personal lives. Compared with 2023, respondents are much more likely to be using gen AI at work and even more likely to be using gen AI both at work and in their personal lives (Exhibit 4). The survey finds upticks in gen AI use across all regions, with the largest increases in Asia–Pacific and Greater China. Respondents at the highest seniority levels, meanwhile, show larger jumps in the use of gen Al tools for work and outside of work compared with their midlevel-management peers. Looking at specific industries, respondents working in energy and materials and in professional services report the largest increase in gen AI use.
Investments in gen AI and analytical AI are beginning to create value
The latest survey also shows how different industries are budgeting for gen AI. Responses suggest that, in many industries, organizations are about equally as likely to be investing more than 5 percent of their digital budgets in gen AI as they are in nongenerative, analytical-AI solutions (Exhibit 5). Yet in most industries, larger shares of respondents report that their organizations spend more than 20 percent on analytical AI than on gen AI. Looking ahead, most respondents—67 percent—expect their organizations to invest more in AI over the next three years.
Where are those investments paying off? For the first time, our latest survey explored the value created by gen AI use by business function. The function in which the largest share of respondents report seeing cost decreases is human resources. Respondents most commonly report meaningful revenue increases (of more than 5 percent) in supply chain and inventory management (Exhibit 6). For analytical AI, respondents most often report seeing cost benefits in service operations—in line with what we found last year —as well as meaningful revenue increases from AI use in marketing and sales.
Inaccuracy: The most recognized and experienced risk of gen AI use
As businesses begin to see the benefits of gen AI, they’re also recognizing the diverse risks associated with the technology. These can range from data management risks such as data privacy, bias, or intellectual property (IP) infringement to model management risks, which tend to focus on inaccurate output or lack of explainability. A third big risk category is security and incorrect use.
Respondents to the latest survey are more likely than they were last year to say their organizations consider inaccuracy and IP infringement to be relevant to their use of gen AI, and about half continue to view cybersecurity as a risk (Exhibit 7).
Conversely, respondents are less likely than they were last year to say their organizations consider workforce and labor displacement to be relevant risks and are not increasing efforts to mitigate them.
In fact, inaccuracy— which can affect use cases across the gen AI value chain , ranging from customer journeys and summarization to coding and creative content—is the only risk that respondents are significantly more likely than last year to say their organizations are actively working to mitigate.
Some organizations have already experienced negative consequences from the use of gen AI, with 44 percent of respondents saying their organizations have experienced at least one consequence (Exhibit 8). Respondents most often report inaccuracy as a risk that has affected their organizations, followed by cybersecurity and explainability.
Our previous research has found that there are several elements of governance that can help in scaling gen AI use responsibly, yet few respondents report having these risk-related practices in place. 4 “ Implementing generative AI with speed and safety ,” McKinsey Quarterly , March 13, 2024. For example, just 18 percent say their organizations have an enterprise-wide council or board with the authority to make decisions involving responsible AI governance, and only one-third say gen AI risk awareness and risk mitigation controls are required skill sets for technical talent.
Bringing gen AI capabilities to bear
The latest survey also sought to understand how, and how quickly, organizations are deploying these new gen AI tools. We have found three archetypes for implementing gen AI solutions : takers use off-the-shelf, publicly available solutions; shapers customize those tools with proprietary data and systems; and makers develop their own foundation models from scratch. 5 “ Technology’s generational moment with generative AI: A CIO and CTO guide ,” McKinsey, July 11, 2023. Across most industries, the survey results suggest that organizations are finding off-the-shelf offerings applicable to their business needs—though many are pursuing opportunities to customize models or even develop their own (Exhibit 9). About half of reported gen AI uses within respondents’ business functions are utilizing off-the-shelf, publicly available models or tools, with little or no customization. Respondents in energy and materials, technology, and media and telecommunications are more likely to report significant customization or tuning of publicly available models or developing their own proprietary models to address specific business needs.
Respondents most often report that their organizations required one to four months from the start of a project to put gen AI into production, though the time it takes varies by business function (Exhibit 10). It also depends upon the approach for acquiring those capabilities. Not surprisingly, reported uses of highly customized or proprietary models are 1.5 times more likely than off-the-shelf, publicly available models to take five months or more to implement.
Gen AI high performers are excelling despite facing challenges
Gen AI is a new technology, and organizations are still early in the journey of pursuing its opportunities and scaling it across functions. So it’s little surprise that only a small subset of respondents (46 out of 876) report that a meaningful share of their organizations’ EBIT can be attributed to their deployment of gen AI. Still, these gen AI leaders are worth examining closely. These, after all, are the early movers, who already attribute more than 10 percent of their organizations’ EBIT to their use of gen AI. Forty-two percent of these high performers say more than 20 percent of their EBIT is attributable to their use of nongenerative, analytical AI, and they span industries and regions—though most are at organizations with less than $1 billion in annual revenue. The AI-related practices at these organizations can offer guidance to those looking to create value from gen AI adoption at their own organizations.
To start, gen AI high performers are using gen AI in more business functions—an average of three functions, while others average two. They, like other organizations, are most likely to use gen AI in marketing and sales and product or service development, but they’re much more likely than others to use gen AI solutions in risk, legal, and compliance; in strategy and corporate finance; and in supply chain and inventory management. They’re more than three times as likely as others to be using gen AI in activities ranging from processing of accounting documents and risk assessment to R&D testing and pricing and promotions. While, overall, about half of reported gen AI applications within business functions are utilizing publicly available models or tools, gen AI high performers are less likely to use those off-the-shelf options than to either implement significantly customized versions of those tools or to develop their own proprietary foundation models.
What else are these high performers doing differently? For one thing, they are paying more attention to gen-AI-related risks. Perhaps because they are further along on their journeys, they are more likely than others to say their organizations have experienced every negative consequence from gen AI we asked about, from cybersecurity and personal privacy to explainability and IP infringement. Given that, they are more likely than others to report that their organizations consider those risks, as well as regulatory compliance, environmental impacts, and political stability, to be relevant to their gen AI use, and they say they take steps to mitigate more risks than others do.
Gen AI high performers are also much more likely to say their organizations follow a set of risk-related best practices (Exhibit 11). For example, they are nearly twice as likely as others to involve the legal function and embed risk reviews early on in the development of gen AI solutions—that is, to “ shift left .” They’re also much more likely than others to employ a wide range of other best practices, from strategy-related practices to those related to scaling.
In addition to experiencing the risks of gen AI adoption, high performers have encountered other challenges that can serve as warnings to others (Exhibit 12). Seventy percent say they have experienced difficulties with data, including defining processes for data governance, developing the ability to quickly integrate data into AI models, and an insufficient amount of training data, highlighting the essential role that data play in capturing value. High performers are also more likely than others to report experiencing challenges with their operating models, such as implementing agile ways of working and effective sprint performance management.
About the research
The online survey was in the field from February 22 to March 5, 2024, and garnered responses from 1,363 participants representing the full range of regions, industries, company sizes, functional specialties, and tenures. Of those respondents, 981 said their organizations had adopted AI in at least one business function, and 878 said their organizations were regularly using gen AI in at least one function. To adjust for differences in response rates, the data are weighted by the contribution of each respondent’s nation to global GDP.
Alex Singla and Alexander Sukharevsky are global coleaders of QuantumBlack, AI by McKinsey, and senior partners in McKinsey’s Chicago and London offices, respectively; Lareina Yee is a senior partner in the Bay Area office, where Michael Chui , a McKinsey Global Institute partner, is a partner; and Bryce Hall is an associate partner in the Washington, DC, office.
They wish to thank Kaitlin Noe, Larry Kanter, Mallika Jhamb, and Shinjini Srivastava for their contributions to this work.
This article was edited by Heather Hanselman, a senior editor in McKinsey’s Atlanta office.
Explore a career with us
Related articles.

Moving past gen AI’s honeymoon phase: Seven hard truths for CIOs to get from pilot to scale
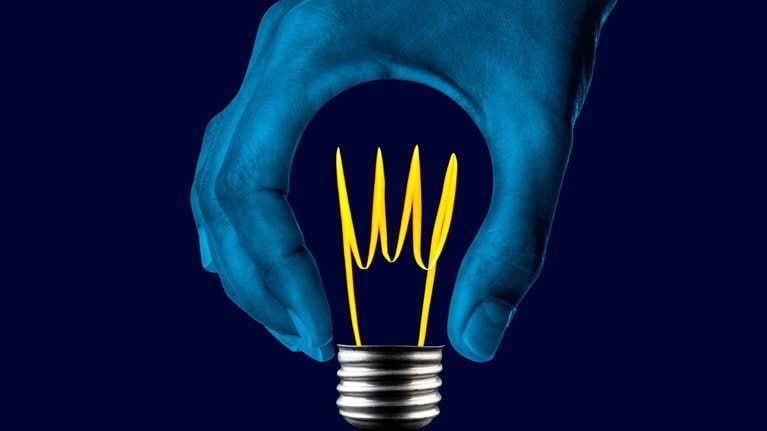
A generative AI reset: Rewiring to turn potential into value in 2024

Implementing generative AI with speed and safety
TechRepublic
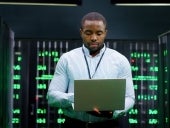
8 Best Data Science Tools and Software
Apache Spark and Hadoop, Microsoft Power BI, Jupyter Notebook and Alteryx are among the top data science tools for finding business insights. Compare their features, pros and cons.
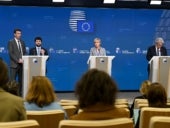
EU’s AI Act: Europe’s New Rules for Artificial Intelligence
Europe's AI legislation, adopted March 13, attempts to strike a tricky balance between promoting innovation and protecting citizens' rights.
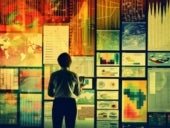
10 Best Predictive Analytics Tools and Software for 2024
Tableau, TIBCO Data Science, IBM and Sisense are among the best software for predictive analytics. Explore their features, pricing, pros and cons to find the best option for your organization.
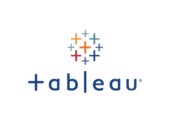
Tableau Review: Features, Pricing, Pros and Cons
Tableau has three pricing tiers that cater to all kinds of data teams, with capabilities like accelerators and real-time analytics. And if Tableau doesn’t meet your needs, it has a few alternatives worth noting.
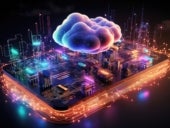
Top 6 Enterprise Data Storage Solutions for 2024
Amazon, IDrive, IBM, Google, NetApp and Wasabi offer some of the top enterprise data storage solutions. Explore their features and benefits, and find the right solution for your organization's needs.
Latest Articles
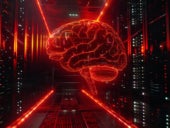
Some Generative AI Company Employees Pen Letter Wanting ‘Right to Warn’ About Risks
Both the promise and the risk of "human-level" AI has always been part of OpenAI’s makeup. What should business leaders take away from this letter?
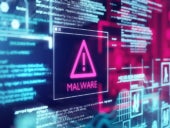
Cisco Talos: LilacSquid Threat Actor Targets Multiple Sectors Worldwide With PurpleInk Malware
Find out how the cyberespionage threat actor LilacSquid operates, and then learn how to protect your business from this security risk.
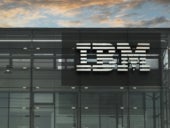
IBM’s Think 2024 News That Should Help Skills & Productivity Issues in Australia
TechRepublic interviewed IBM’s managing director for Australia about how announcements from the recent Think event could impact the tech industry in particular.
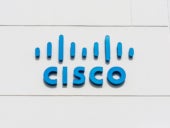
Cisco Live 2024: New Unified Observability Experience Packages Cisco & Splunk Insight Tools
The observability suite is the first major overhaul for Splunk products since the Cisco acquisition. Plus, Mistral AI makes a deal with Cisco’s incubator.
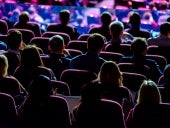
Top Tech Conferences & Events to Add to Your Calendar in 2024
A great way to stay current with the latest technology trends and innovations is by attending conferences. Read and bookmark our 2024 tech events guide.
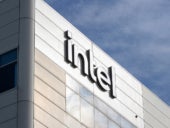
Intel Lunar Lake NPU Brings 48 TOPS of AI Acceleration
Competition for AI speed heats up. Plus, the first of the two new Xeon 6 processors is now available, and Gaudi 3 deals have been cinched with manufacturers.
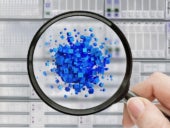
Cisco Live 2024: Cisco Unveils AI Deployment Solution With NVIDIA
A $1 billion commitment will send Cisco money to Cohere, Mistral AI and Scale AI.
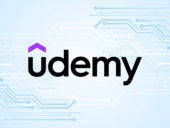
The 5 Best Udemy Courses That Are Worth Taking in 2024
Udemy is an online platform for learning at your own pace. Boost your career with our picks for the best Udemy courses for learning tech skills online in 2024.
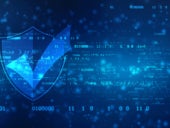
What Is Data Quality? Definition and Best Practices
Data quality refers to the degree to which data is accurate, complete, reliable and relevant for its intended use.
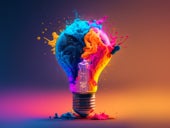
TechRepublic Premium Editorial Calendar: Policies, Checklists, Hiring Kits and Glossaries for Download
TechRepublic Premium content helps you solve your toughest IT issues and jump-start your career or next project.
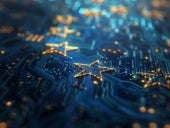
What is the EU’s AI Office? New Body Formed to Oversee the Rollout of General Purpose Models and AI Act
The AI Office will be responsible for enforcing the rules of the AI Act, ensuring its implementation across Member States, funding AI and robotics innovation and more.
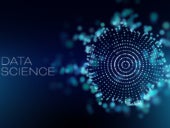
What is Data Science? Benefits, Techniques and Use Cases
Data science involves extracting valuable insights from complex datasets. While this process can be technically challenging and time-consuming, it can lead to better business decision-making.
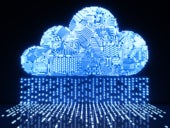
Gartner’s 7 Predictions for the Future of Australian & Global Cloud Computing
An explosion in AI computing, a big shift in workloads to the cloud, and difficulties in gaining value from hybrid cloud strategies are among the trends Australian cloud professionals will see to 2028.
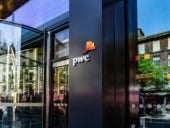
OpenAI Adds PwC as Its First Resale Partner for the ChatGPT Enterprise Tier
PwC employees have 100,000 ChatGPT Enterprise seats. Plus, OpenAI forms a new safety and security committee in their quest for more powerful AI, and seals media deals.
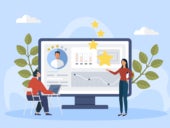
What Is Contact Management? Importance, Benefits and Tools
Contact management ensures accurate, organized and accessible information for effective communication and relationship building.
Create a TechRepublic Account
Get the web's best business technology news, tutorials, reviews, trends, and analysis—in your inbox. Let's start with the basics.
* - indicates required fields
Sign in to TechRepublic
Lost your password? Request a new password
Reset Password
Please enter your email adress. You will receive an email message with instructions on how to reset your password.
Check your email for a password reset link. If you didn't receive an email don't forgot to check your spam folder, otherwise contact support .
Welcome. Tell us a little bit about you.
This will help us provide you with customized content.
Want to receive more TechRepublic news?
You're all set.
Thanks for signing up! Keep an eye out for a confirmation email from our team. To ensure any newsletters you subscribed to hit your inbox, make sure to add [email protected] to your contacts list.
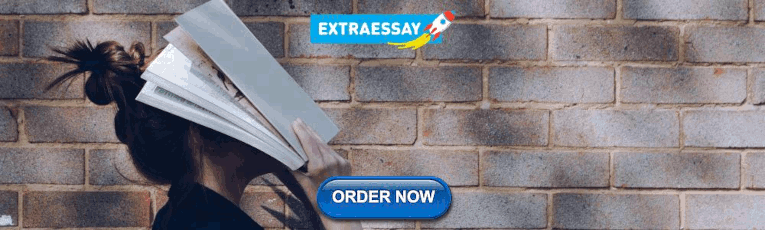
IMAGES
VIDEO
COMMENTS
Smart manufacturing (SM) is a term generally applied to the improvement in manufacturing operations through integration of systems, linking of physical and cyber capabilities, and taking advantage of information including leveraging the big data evolution. SM adoption has been occurring unevenly across industries, thus there is an opportunity to look to other industries to determine solution ...
14 Case Studies of Manufacturing Analytics in 2024. Manufacturing is one of the top 3 industries which account for the biggest share of the big data and analytics revenues worldwide. Manufacturing analytics have numerous use cases which enable businesses to predict machinery's future use, prevent failures, forecast maintenance requirements ...
IDC estimates that the global big data analytics market revenue would've reached ~$274B by 2022. This would have made manufacturing as one of the top 3 industries with largest analytics growth. Manufacturing analytics is one of the critical steps for manufacturing digital transformation required for Industry 4.0, which aims to: . Automate traditional manufacturing processes
A big data analytics platform for smart factories in small and medium-sized manufacturing enterprises: An empirical case study of a die casting factory International Journal of Precision Engineering and Manufacturing , 18 ( 10 ) ( 2017 ) , pp. 1353 - 1361
analytics based on a real world case study. These. concepts include data understanding, data prepara- ... there is an increasing need of handling big manufacturing data characterized by high ...
The big data era has only just emerged, but the practice of advanced analytics is grounded in years of mathematical research and scientific application. It can be a critical tool for realizing improvements in yield, particularly in any manufacturing environment in which process complexity, process variability, and capacity restraints are present.
In 2017, Kusiak advocated for manufacturing industries to embrace and employ the concepts of Big Data Analytics in order to increase efficiency and profits. Tao et al. ... Case studies analyzing manufacturing-derived data sets that illustrate the application of most of the tools described in this section are presented in [5, 16, 52].
Big-data analytics capability (BDAC) is crucial for the value creation process and collaboration innovation of manufacturing enterprises. Based on the knowledge-based view, this study aims to explore the relationship between BDAC, the value creation process (collaboration breadth and collaboration depth), and collaboration innovation quality while considering the contingency of technology risk.
SM leverages a corresponding trend in manufacturing of the advances in volume, velocity, and. variety of data, often referred to as "big data," and applies "big data analytics" to improve ...
The adopted Model-based Big Data Analytics-as-a-Service framework and the configured cloud-based Big Data platform (to run the entire BDA pipeline in the aeronautical manufacturing case study) overcame the major challenges that have, so far, prevented many companies from reaping the full benefits of Big Data analytics.
The global market for data analytics in manufacturing is expected to reach $12.9 billion by 2023. Examples of how data analytics is being used in manufacturing. Siemens: Siemens is using data analytics to improve the efficiency of its production lines. The company has installed sensors on its equipment to collect data on production processes.
The study develops a theoretical structure to investigate if big data coupled with different production solutions can provide for a dispersed production system and discovers several uses of distributed manufacturing principles to evaluate the current production processes worked for larger customer product solutions by using analytics and ...
And Elon Musk is aiming to deliver 500,000 vehicles by 2018 and 1,000,000 by 2020. Last December, the city of Fremont, California, approved Tesla's plans to double the size of its factory there. There will be an additional 420,000 square meters of manufacturing space. And the number of workers is expected to increase from 6,000 to 9,000.
Manufacturing and Value Chains. The future of manufacturing is powered by data and analytics. Here's why. Sep 9, 2022. Just 39% of manufacturing executives report that they have successfully scaled data-driven use cases beyond the production process of a single product. Image: REUTERS/Noah Berger/File Photo.
GE has bet big on the Industrial Internet — the convergence of industrial machines, data, and the Internet. The company is putting sensors on gas turbines, jet engines, and other machines; connecting them to the cloud; and analyzing the resulting flow of data. The goal: identify ways to improve machine productivity and reliability.
Big data analytics in manufacturing is all you need to improve your business's efficiency, quality, and productivity in today's data-driven industry. ... Case Studies Demonstrating BIM's Value Excelize reported some stunning statistics and case studies demonstrating the real-world impact of BIM through specific project examples, showcasing how ...
In a case of big-data analytics, unlike more conventional data analytics, the choice of technology and storage and management techniques often strongly depends on the subsequently used data-analysis methods, and on the properties of the data under consideration. ... "Big Data in Manufacturing: A Systematic Mapping Study." Journal of Big ...
This advanced big data use case has been able to eliminate nearly 100% of scrap parts. Furthermore, it has saved operator time, as BC Machining no longer needs to sort the scrap part, allowing both them and the machines to be focused on producing good parts and generating revenue for the company. The result has been $72,000 in annual savings ...
Data Analytics for Smart Manufacturing: A Case Study. Nadeem Iftikhar1, Thorkil Baattrup-Andersen2, Finn Ebertsen Nordbjerg1, Eugen Bobolea1. and Paul-Bogdan Radu1. 1University College of Northern Denmark, Aalborg 9200, Denmark. 2Dolle A/S, Frøstrup 7741, Denmark Keywords: Industry 4.0, Data Analytics, Smart Manufacturing, Logistic Regression ...
Big Data Analytics for Predictive Manufacturing Control - A Case Study from Process Industry ... However, without dedicated approaches of big data analytics, the sample company cannot utilize the potential of already available data for a proactive process control. Hence, the article forms a working and discussion basis for further research in ...
A bibliometric analysis was conducted with published data from 2011 to 2020 regarding intelligent manufacturing driven by big data gathered from the Web of Science database (as shown in Fig. 1), which shows an almost steady increase in papers on this topic. Fig. 1 (a) shows the number of published articles about intelligent manufacturing from 2011 to 2020.
This paper presents a systematic approach to developing big data analytics for manufacturing process-relevant decision-making activities from the perspective of smart manufacturing. The proposed analytics consist of five integrated system components: (1) Data Preparation System, (2) Data Exploration System, (3) Data Visualization System, (4) Data Analysis System, and (5) Knowledge Extraction ...
Data volume and analytic complexity present a challenge when performing big data analysis. JAKALA works with large-volume people movement data that the company sources from a vendor that amalgamates opt-in location tracking. The sourced data relies on more than 10 million devices, generating around 1 billion data points per day.
The respondents in this study were workers of Indonesia's upstream oil and gas sector. The results of this study indicate that Big Data, IoT, and Physical Cyber Systems (PCS) have a positive and significant effect on the human factor. In addition, there is a significant relationship between human factors and sustainable manufacturing.
Process improvement initiatives require access to frequently updated and good quality data. This is an extremely difficult task in the area of production processes, where the lack of a process digital footprint is a very big challenge. To solve this problem, the authors of this article designed, implemented, and verified the results of a new work measurement method. The Workplace Performance ...
If 2023 was the year the world discovered generative AI (gen AI), 2024 is the year organizations truly began using—and deriving business value from—this new technology. In the latest McKinsey Global Survey on AI, 65 percent of respondents report that their organizations are regularly using gen AI, nearly double the percentage from our ...
Big Data Big Data Tableau Review: Features, Pricing, Pros and Cons . Tableau has three pricing tiers that cater to all kinds of data teams, with capabilities like accelerators and real-time analytics.
Today, in a smart manufacturing environment based on the Industry 4.0 paradigm, people, technological infrastructure and machinery equipped with sensors can constantly generate and communicate a huge volume of data, also known as Big Data.The manufacturing industry takes advantage of Big Data and analytics evolution by improving its capability to bring out valuable information and knowledge ...
Industrial production and operation have been completely transformed by lean methods made possible by lean management (LM) technologies in the industry. This paper investigates industry practices regarding the application of lean tools in the manufacturing sector. For the purpose of gathering data, a questionnaire survey was distributed among the manufacturing professionals in the organization ...
ICBAR '23: Proceedings of the 2023 3rd International Conference on Big Data, Artificial Intelligence and Risk Management COVID-19 Risk Analysis Based on Population Migration Big Data: A Case Study of Wuhan