A Literature Review on Software Testing Techniques
- First Online: 27 September 2022
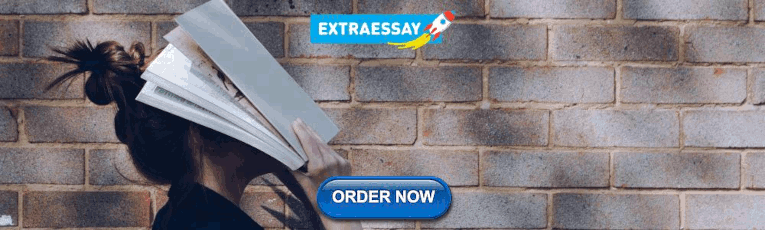
Cite this chapter
- Kainat Khan 6 &
- Sachin Yadav 7
Part of the book series: EAI/Springer Innovations in Communication and Computing ((EAISICC))
415 Accesses
1 Citations
With expanding convolution of recent software, rapidly growing pressure made the quality standards better and took them to new and reform heights. Testing of software is an inescapable portion of software testing life cycle, and also thinking of its criticality during the pre-post process of development makes it a problem that must be dealt with improved and well-organized methodologies/techniques. A lot of studies have been conducted in order to enhance the performance of software. The crucial information of every software needs to be checked rigorously. Various testing techniques are present to carry out activities while testing. Testing is performed to make sure that the outcome will be successful. This work targets to review and analyze the already available and enhanced testing techniques for the improved and good quality product. Some recent research studies have been summed up in this work as software testing is acquiring more significance these days.
This is a preview of subscription content, log in via an institution to check access.
Access this chapter
Subscribe and save.
- Get 10 units per month
- Download Article/Chapter or eBook
- 1 Unit = 1 Article or 1 Chapter
- Cancel anytime
- Available as PDF
- Read on any device
- Instant download
- Own it forever
- Available as EPUB and PDF
- Compact, lightweight edition
- Dispatched in 3 to 5 business days
- Free shipping worldwide - see info
- Durable hardcover edition
Tax calculation will be finalised at checkout
Purchases are for personal use only
Institutional subscriptions
Similar content being viewed by others
A Study on Identification of Issues and Challenges Encountered in Software Testing
A Comparative Study of Software Testing Techniques
Software Testability (Its Benefits, Limitations, and Facilitation)
Anitha, A. (2013). A brief overview of software testing techniques and metrics . [Online]. Available: www.ijarcce.com
Anwar, N., & Kar, S. (2019). Review paper on various software testing techniques & strategies .
Google Scholar
Atifi, M., Mamouni, A., & Marzak, A. (2017). A comparative study of software testing techniques. Lecture Notes in Computer Science (including subseries Lecture Notes in Artificial Intelligence and Lecture Notes in Bioinformatics), 10299 , 373–390., LNCS. https://doi.org/10.1007/978-3-319-59647-1_27
Article Google Scholar
Babbar, H. (2017). Software testing: Techniques and test cases . [Online]. Available: www.ijrcar.com
Baresi, L., & Pezzè, M. (2006). An introduction to software testing. Electronic Notes in Theoretical Computer Science, 148 (1 Special Issues), 89–111. https://doi.org/10.1016/j.entcs.2005.12.014
Chaudhary, S. (2017). Latest software testing tools and techniques: A review. International Journal of Advanced Research in Computer Science and Software Engineering, 7 (5), 538–540. https://doi.org/10.23956/ijarcsse/sv7i5/0138
Divyani, M., Taley, S., & Pathak, B. (n.d.). Comprehensive study of software testing techniques and strategies: A review . [Online]. Available: www.ijert.org
Garousi, V., & Mäntylä, M. V. (2016). A systematic literature review of literature reviews in software testing. Information and Software Technology. Elsevier, 80 , 195–216. https://doi.org/10.1016/j.infsof.2016.09.002
Iqbal Malik, K., & Hassan, S. (2013). Software testing methodologies for finding errors software architecture refactoring tools and techniques: a comparative study view project . [Online]. Available: www.theinternationaljournal.org
Jamil, M. A., Arif, M., Abubakar, N. S. A., & Ahmad, A. (2017). Software testing techniques: A literature review. In Proceedings – 6th international conference on information and communication technology for the Muslim world, ICT4M 2016 (pp. 177–182). https://doi.org/10.1109/ICT4M.2016.40
Chapter Google Scholar
Jammalamadaka, K., & Parveen, N. (2019). Holistic research of software testing and challenges. International Journal of Innovative Technology and Exploring Engineering, 8 (6 Special Issue 4), 1506–1521. https://doi.org/10.35940/ijitee.F1307.0486S419
Jat, S., & Sharma, P. (2017). Analysis of different software testing techniques. International Journal of Scientific Research in Research Paper. Computer Science and Engineering, 5 (2), 77–80. [Online]. Available: www.isroset.org
Jovanović, I. (n.d.) Software testing methods and techniques .
Kaur Chauhan Ȧ, R., & Singh Ḃ Ȧ, I. (2004). Latest research and development on software testing techniques and tools . [Online]. Available: http://inpressco.com/category/ijcet
Kaur, M., & Singh, R. (2014). A review of software testing techniques . [Online]. Available: http://www.irphouse.com .
Kumar, R. (2016). Software testing techniques and strategies . [Online]. Available: http://www.ijeast.com
Malviya, A. (n.d.) Software testing: Concepts and issues . [Online]. Available: https://ssrn.com/abstract=3351067
Naik, K., & Tripathy, P. (2011). Software testing and quality assurance: Theory and practice .
Nidhra, S. (2012). Black Box and White Box testing techniques – A literature review. International Journal of Embedded Systems and Applications, 2 (2), 29–50. https://doi.org/10.5121/ijesa.2012.2204
Okezie, F., Odun-Ayo, I., & Bogle, S. (2019). A critical analysis of software testing tools. Journal of Physics: Conference Series, 1378 (4). https://doi.org/10.1088/17426596/1378/4/042030
Orso, A., & Rothermel, G. (2014). Software testing: A research travelogue (2000–2014). In Future of software engineering, FOSE 2014 – Proceedings (pp. 117–132). https://doi.org/10.1145/2593882.2593885
Pardeshi, S. N. (2013). Study of testing strategies and availabletools. International Journal of Scientific and Research Publications, 3 (3) [Online]. Available: www.ijsrp.org
Pezzè, M., & Young, M. (2008). Software testing and analysis: Process, principles, and techniques . Wiley.
MATH Google Scholar
Rosero, R. H., Gómez, O. S., & Rodríguez, G. (2016). 15 years of software regression testing techniques – A survey. International Journal of Software Engineering and Knowledge Engineering. World Scientific Publishing, 26 (5), 675–689. https://doi.org/10.1142/S0218194016300013
Roshan, R., & Sharma, C. M. (2012). Review of search based techniques in software testing . Rabins Porwal ITS.
Book Google Scholar
Sawant, A. A., Bari, P. H., & Chawan, P. M. (2015). Software testing techniques and strategies (Vol. 2, pp. 980–986) [Online]. Available: www.ijera.com
M. A. Sethi (n.d.), “A review paper on levels, types & techniques in software testing,” International Journal of Advanced Research in Computer Science 8, 7, https://doi.org/10.26483/ijarcs.v8i7.4236 .
Sharma, C., Sabharwal, S., Sibal, R., Hind, A., & Marg, F. (2013). A survey on software testing techniques using genetic algorithm . [Online]. Available: www.IJCSI.org
Singh Ghuman, S. (2014). International Journal of Computer Science and Mobile Computing Software Testing Techniques . [Online]. Available: www.ijcsmc.com
Singhal, A., Bansal, A., & Kumar, A. (2013). A critical review of various testing techniques in aspectoriented software systems. ACM SIGSOFT Software Engineering Notes, 38 (4), 1–9. https://doi.org/10.1145/2492248.2492275
II. TESTING TYPES. (2013). [Online]. Available: http://www.toolsjournal.com/testinglists/item/404-10-
Vos, T. E. J., Marínt, B., Escalona, M. J., & Marchetto, A. (2012). A methodological framework for evaluating software testing techniques and tools. In Proceedings – International conference on quality software (pp. 230–239). https://doi.org/10.1109/QSIC.2012.16
Download references
Author information
Authors and affiliations.
Department of Computer Science, Delhi Technological University, Delhi, India
Kainat Khan
School of Engineering Technology, Noida International University, Noida, Uttar Pradesh, India
Sachin Yadav
You can also search for this author in PubMed Google Scholar
Editor information
Editors and affiliations.
School of Computer & Systems Sciences, Jawaharlal Nehru University, New Delhi, Delhi, India
Manju Khari
Department of MCA, GITA Autonomous College, Bhubaneswar, India
Deepti Bala Mishra
Department of Computer Engineering-AI, Marwadi University, Rajkot, Gujarat, India
Biswaranjan Acharya
Computer Science and Technology, Universidad Internacional de La Rioja, La Rioja, Spain
Ruben Gonzalez Crespo
Rights and permissions
Reprints and permissions
Copyright information
© 2022 Springer Nature Switzerland AG
About this chapter
Khan, K., Yadav, S. (2022). A Literature Review on Software Testing Techniques. In: Khari, M., Mishra, D.B., Acharya, B., Gonzalez Crespo, R. (eds) Optimization of Automated Software Testing Using Meta-Heuristic Techniques. EAI/Springer Innovations in Communication and Computing. Springer, Cham. https://doi.org/10.1007/978-3-031-07297-0_5
Download citation
DOI : https://doi.org/10.1007/978-3-031-07297-0_5
Published : 27 September 2022
Publisher Name : Springer, Cham
Print ISBN : 978-3-031-07296-3
Online ISBN : 978-3-031-07297-0
eBook Packages : Intelligent Technologies and Robotics Intelligent Technologies and Robotics (R0)
Share this chapter
Anyone you share the following link with will be able to read this content:
Sorry, a shareable link is not currently available for this article.
Provided by the Springer Nature SharedIt content-sharing initiative
- Publish with us
Policies and ethics
- Find a journal
- Track your research
Software Testing: Issues and Challenges of Artificial Intelligence & Machine Learning
12 Pages Posted: 22 Nov 2021
Kishore Sugali
Purdue university.
Date Written: October 24, 2021
The history of Artificial Intelligence and Machine Learning dates back to 1950’s. In recent years, there has been an increase in popularity for applications that implement AI and ML technology. As with traditional development, software testing is a critical component of an efficient AI/ML application. However, the approach to development methodology used in AI/ML varies significantly from traditional development. Owing to these variations, numerous software testing challenges occur. This paper aims to recognize and to explain some of the biggest challenges that software testers face in dealing with AI/ML applications. For future research, this study has key implications. Each of the challenges outlined in this paper is ideal for further investigation and has great potential to shed light on the way to more productive software testing strategies and methodologies that can be applied to AI/ML applications.
Keywords: Artificial Intelligence (AI), Machine Learning (ML), Software Testing.
Suggested Citation: Suggested Citation
Kishore Sugali (Contact Author)
610 Purdue Mall West Lafayette, IN 47907 United States
Do you have a job opening that you would like to promote on SSRN?
Paper statistics, related ejournals, artificial intelligence ejournal.
Subscribe to this fee journal for more curated articles on this topic
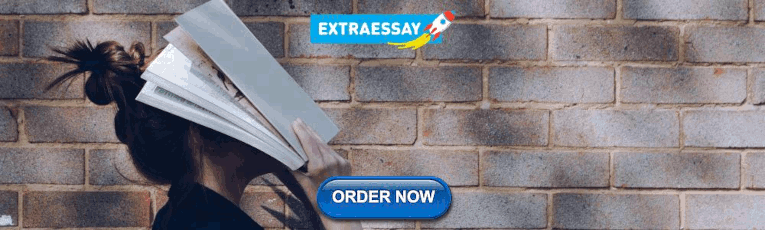
Electrical Engineering eJournal
Export Citations
- Please download or close your previous search result export first before starting a new bulk export. Preview is not available. By clicking download, a status dialog will open to start the export process. The process may take a few minutes but once it finishes a file will be downloadable from your browser. You may continue to browse the DL while the export process is in progress.
- Download citation
- Copy citation
We are preparing your search results for download ...
We will inform you here when the file is ready.
Your file of search results citations is now ready.
Your search export query has expired. Please try again.
Information
- Author Services
Initiatives
You are accessing a machine-readable page. In order to be human-readable, please install an RSS reader.
All articles published by MDPI are made immediately available worldwide under an open access license. No special permission is required to reuse all or part of the article published by MDPI, including figures and tables. For articles published under an open access Creative Common CC BY license, any part of the article may be reused without permission provided that the original article is clearly cited. For more information, please refer to https://www.mdpi.com/openaccess .
Feature papers represent the most advanced research with significant potential for high impact in the field. A Feature Paper should be a substantial original Article that involves several techniques or approaches, provides an outlook for future research directions and describes possible research applications.
Feature papers are submitted upon individual invitation or recommendation by the scientific editors and must receive positive feedback from the reviewers.
Editor’s Choice articles are based on recommendations by the scientific editors of MDPI journals from around the world. Editors select a small number of articles recently published in the journal that they believe will be particularly interesting to readers, or important in the respective research area. The aim is to provide a snapshot of some of the most exciting work published in the various research areas of the journal.
Original Submission Date Received: .
- Active Journals
- Find a Journal
- Proceedings Series
- For Authors
- For Reviewers
- For Editors
- For Librarians
- For Publishers
- For Societies
- For Conference Organizers
- Open Access Policy
- Institutional Open Access Program
- Special Issues Guidelines
- Editorial Process
- Research and Publication Ethics
- Article Processing Charges
- Testimonials
- Preprints.org
- SciProfiles
- Encyclopedia
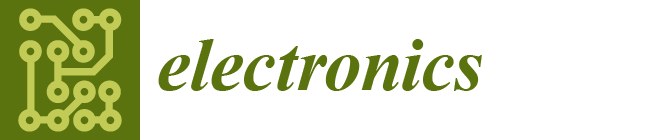
Article Menu
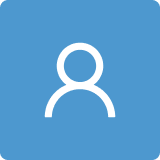
- Subscribe SciFeed
- Recommended Articles
- Google Scholar
- on Google Scholar
- Table of Contents
Find support for a specific problem in the support section of our website.
Please let us know what you think of our products and services.
Visit our dedicated information section to learn more about MDPI.
JSmol Viewer
A decade of intelligent software testing research: a bibliometric analysis.

1. Introduction
- Using AI to automatically generate test cases based on the code and requirements of the software [ 3 ].
- Using ML to predict which test cases are most likely to find defects, and prioritizing those test cases for execution [ 4 ].
- Using AI to automatically identify patterns in test results and suggest areas of the code that may be prone to defects [ 5 ].
- Automation: Intelligent testing uses AI and ML to automate the generation and execution of test cases, reducing the need for manual effort [ 10 ];
- Coverage: Intelligent testing techniques can help to ensure that a greater proportion of the code is covered by test cases, increasing the likelihood of finding defects [ 11 ];
- Prioritization: Using ML, intelligent testing can prioritize the execution of test cases based on their likelihood of finding defects, allowing testers to focus on the most important tests first [ 12 ];
- Defect prediction: AI can be used to analyze test results and identify patterns that may indicate the presence of defects in the code [ 13 ].
2. Related Work
3. data collection, 4. research method.
- Main information: A table showing the fundamental traits of our dataset;
- Scientific production: A graph of the annual scientific production of the studied field;
- Citations per year: A graph showing the growth in citations by year;
- Relationship between countries, authors, and titles: Which is a Three-Field Plot showing the relationship between countries, authors, and title of articles.
- The Most Successful and Highly Cited Journals: A graph showing the best ten most active journals based on the quantity of articles they published and the top cited journals based on the quantity of citations they got;
- The Most Important Organizations: Based on the quantity of documents created, a graph showing the top 10 most prolific institutions;
- The Most Relevant Authors: A graph showing the top 10 authors based on the volume of articles created;
- The Top Developing Nations According to Corresponding Authors: A graph showing the top 10 most relevant countries based on the amount of articles produced, either in single country publication or multiple countries publication;
- The Most Globally cited authors: A graph of the top 10 most cited authors using all citations in the Web of Science database;
- The Most Globally cited documents: A graph of the top 10 most cited documents using all citations in the Web of Science database;
- Scientific Productivity Frequency Distribution (Lotka’s Law): A graph and table displaying the frequency analysis of research publications according to Lotka’s law;
- The Most Frequent Words: A graph and a word cloud of the most used words in the documents’ abstract, title and keywords;
- Co-occurrence network of keywords: A graph that depicts the relationship between key words and divides them into smaller clusters;
- Collaboration World Map and Network: A graph and a world map showing the relationship between countries in terms of collaboration;
- Organisations Co-authorship: A graph showing a network of collaborations between organizations;
- Authors co-citation network: A graph of the network of connection between authors based on the co-citations;
- Journals co-citations network: A graph of the network of correlation between journals based on the co-citations.
- Thematic Map: A network graph, referred to as a thematic map, is created by the keywords and how they are connected. Each thematic map’s labels are identified using the name of the keyword that appears most frequently in the connected topic [ 73 ];
- Multiple Correspondence Analysis (MCA): For visually and mathematically analyzing such data, multivariate categorical analysis (MCA) is aa method for multidimensional analysis method [ 74 ];
- Correspondence Analysis (CA): Correspondence analysis is a visual method of comprehending the connection between items in a frequency table. It is intended to assess connections between qualitative variables and is a development of principal component analysis (or categorical data) [ 75 ];
- Multidimensional scaling: Just like MCA and CA, a dimensionality reduction approach called multidimentional scaling is used to create a map of the network under study using normalized data [ 69 ].
5.1. Overview
5.1.1. main information, 5.1.2. annual scientific production, 5.1.3. average citation per year, 5.1.4. relationship between countries, authors, and titles, 5.2. science mapping, 5.2.1. the most productive and top-cited sources, 5.2.2. the most relevant institutions, 5.2.3. the most relevant authors, 5.2.4. the most relevant countries by corresponding authors, 5.2.5. the most globally cited authors, 5.2.6. the most globally cited documents, 5.2.7. the most locally cited documents, 5.2.8. frequency distribution of scientific productivity (lotka’s law), 5.2.9. the most frequent words, 5.2.10. keywords co-occurrence network, 5.2.11. collaboration world map and network, 5.2.12. organisations co-authorship.
- Cluster 1: − The Certus Centre for Software Validation and Verification − Korea Advanced Institute of Science & Technology − Kyungpook National University − Nanjing University of Aeronautics and Astronautics − Simula Research Laboratory − University of Luxembourg − University of Milano-Bicocca − University of Ottawa − University of Sheffield
- Cluster 2: − Blekinge Institute of Technology − Ericsson AB − Mälardalen University − Queen’s University Belfast − Technical University of Clausthal − University of Innsbruck
- Cluster 3: − McGill University − Microsoft Research − Stanford University − University of Cambridge − University of Edinburgh − University of Oxford
5.2.13. Authors Co-Citation Network
5.2.14. journals co-citations network, 5.3. network analysis, 5.3.1. thematic map, 5.3.2. multiple correspondence analysis, 5.3.3. correspondence analysis, 5.3.4. multidimensional scaling, 6. discussion, 7. conclusions, author contributions, data availability statement, conflicts of interest.
- Tahvili, S.; Hatvani, L. Uncertainty, Computational Techniques, and Decision Intelligence. In Artificial Intelligence Methods for Optimization of the Software Testing Process with Practical Examples and Exercises ; Elsevier Science: Amsterdam, The Netherlands, 2022. [ Google Scholar ]
- Khari, M.; Mishra, D.; Acharya, B.; Crespo, R. Optimization of Automated Software Testing Using Meta-Heuristic Techniques ; Springer International Publishing: Berlin/Heidelberg, Germany, 2022. [ Google Scholar ]
- Meziane, F.; Vadera, S. Artificial Intelligence Applications for Improved Software Engineering Development: New Prospects ; IGI Global: Harrisburg, PA, USA, 2009. [ Google Scholar ]
- Qiao, L.; Li, X.; Umer, Q.; Guo, P. Deep learning based software defect prediction. Neurocomputing 2020 , 385 , 100–110. [ Google Scholar ] [ CrossRef ]
- Ren, J.; Qin, K.; Ma, Y.; Luo, G. On software defect prediction using machine learning. J. Appl. Math. 2014 , 2014 , 785435. [ Google Scholar ] [ CrossRef ]
- Battina, D.S. Artificial intelligence in software test automation: A systematic literature review. Int. J. Emerg. Technol. Innov. Res. 2019 , 6 , 2349–5162. [ Google Scholar ]
- Karhu, K.; Repo, T.; Taipale, O.; Smolander, K. Empirical observations on software testing automation. In Proceedings of the International Conference on Software Testing Verification and Validation, Denver, CO, USA, 1–4 April 2009; pp. 201–209. [ Google Scholar ]
- Sivakumar, N.; Vivekanandan, K. Comparing the Testing Approaches of Traditional, Object-Oriented and Agent-Oriented Software System. Int. J. Comput. Sci. Eng. Technol. (IJCSET) 2012 , 3 , 498–504. [ Google Scholar ]
- Briand, L.C.; Labiche, Y.; Bawar, Z. Using machine learning to refine black-box test specifications and test suites. In Proceedings of the The 8th International Conference on Quality Software, Oxford, UK, 12–13 August 2008; pp. 135–144. [ Google Scholar ]
- Rafi, D.M.; Moses, K.R.K.; Petersen, K.; Mäntylä, M.V. Benefits and limitations of automated software testing: Systematic literature review and practitioner survey. In Proceedings of the 7th International Workshop on Automation of Software Test (AST), Zurich, Switzerland, 2–3 June 2012; pp. 36–42. [ Google Scholar ]
- Okutan, A.; Yıldız, O.T. Software defect prediction using Bayesian networks. Empir. Softw. Eng. 2014 , 19 , 154–181. [ Google Scholar ] [ CrossRef ]
- Perini, A.; Susi, A.; Avesani, P. A machine learning approach to software requirements prioritization. IEEE Trans. Softw. Eng. 2012 , 39 , 445–461. [ Google Scholar ] [ CrossRef ]
- Wahono, R.S. A systematic literature review of software defect prediction. J. Softw. Eng. 2015 , 1 , 1–16. [ Google Scholar ]
- Donthu, N.; Kumar, S.; Mukherjee, D.; Pandey, N.; Lim, W.M. How to conduct a bibliometric analysis: An overview and guidelines. J. Bus. Res. 2021 , 133 , 285–296. [ Google Scholar ] [ CrossRef ]
- Trudova, A.; Dolezel, M.; Buchalcevova, A. Artificial Intelligence in Software Test Automation: A Systematic. Available online: https://pdfs.semanticscholar.org/9fca/3577e28c06ff27f16bfde7855f29b8d8236c.pdf (accessed on 27 March 2023).
- Lima, R.; da Cruz, A.M.R.; Ribeiro, J. Artificial intelligence applied to software testing: A literature review. In Proceedings of the 15th Iberian Conference on Information Systems and Technologies (CISTI), Seville, Spain, 24–27 June 2020; pp. 1–6. [ Google Scholar ]
- Serna, M.E.; Acevedo, M.E.; Serna, A.A. Integration of properties of virtual reality, artificial neural networks, and artificial intelligence in the automation of software tests: A review. J. Software Evol. Process. 2019 , 31 , e2159. [ Google Scholar ] [ CrossRef ]
- Garousi, V.; Bauer, S.; Felderer, M. NLP-assisted software testing: A systematic mapping of the literature. Inf. Softw. Technol. 2020 , 126 , 106321. [ Google Scholar ] [ CrossRef ]
- Garousi, V.; Felderer, M.; Kılıçaslan, F.N. A survey on software testability. Inf. Softw. Technol. 2019 , 108 , 35–64. [ Google Scholar ] [ CrossRef ]
- Zardari, S.; Alam, S.; Al Salem, H.A.; Al Reshan, M.S.; Shaikh, A.; Malik, A.F.K.; Masood ur Rehman, M.; Mouratidis, H. A Comprehensive Bibliometric Assessment on Software Testing (2016–2021). Electronics 2022 , 11 , 1984. [ Google Scholar ] [ CrossRef ]
- Ghiduk, A.S.; Girgis, M.R.; Shehata, M.H. Higher order mutation testing: A systematic literature review. Comput. Sci. Rev. 2017 , 25 , 29–48. [ Google Scholar ] [ CrossRef ]
- Jamil, M.A.; Arif, M.; Abubakar, N.S.A.; Ahmad, A. Software testing techniques: A literature review. In Proceedings of the 6th International Conference on Information and Communication Technology for the Muslim World (ICT4M), Jakarta, Indonesia, 22–24 November 2016; pp. 177–182. [ Google Scholar ]
- Garousi, V.; Mäntylä, M.V. A systematic literature review of literature reviews in software testing. Inf. Softw. Technol. 2016 , 80 , 195–216. [ Google Scholar ] [ CrossRef ]
- Garousi, V.; Mäntylä, M.V. When and what to automate in software testing? A multi-vocal literature review. Inf. Softw. Technol. 2016 , 76 , 92–117. [ Google Scholar ] [ CrossRef ]
- Wiklund, K.; Eldh, S.; Sundmark, D.; Lundqvist, K. Impediments for software test automation: A systematic literature review. Softw. Testing, Verif. Reliab. 2017 , 27 , e1639. [ Google Scholar ] [ CrossRef ]
- Zhang, D. Machine learning in value-based software test data generation. In Proceedings of the 18th International Conference on Tools with Artificial Intelligence (ICTAI’06), Arlington, VA, USA, 13–15 November 2006; pp. 732–736. [ Google Scholar ]
- Esnaashari, M.; Damia, A.H. Automation of software test data generation using genetic algorithm and reinforcement learning. Expert Syst. Appl. 2021 , 183 , 115446. [ Google Scholar ] [ CrossRef ]
- Zhu, X.-M.; Yang, X.-F. Software test data generation automatically based on improved adaptive particle swarm optimizer. In Proceedings of the International Conference on Computational and Information Sciences, Chengdu, China, 17–19 December 2010; pp. 1300–1303. [ Google Scholar ]
- Koleejan, C.; Xue, B.; Zhang, M. Code coverage optimisation in genetic algorithms and particle swarm optimisation for automatic software test data generation. In Proceedings of the Congress on Evolutionary Computation (CEC), Sendai, Japan, 25–28 May 2015; pp. 1204–1211. [ Google Scholar ]
- Huang, M.; Zhang, C.; Liang, X. Software test cases generation based on improved particle swarm optimization. In Proceedings of the 2nd International Conference on Information Technology and Electronic Commerce, Dalian, China, 20–21 December 2014; pp. 52–55. [ Google Scholar ]
- Liu, C. Research on Software Test Data Generation based on Particle Swarm Optimization Algorithm. In Proceedings of the 5th International Conference on Trends in Electronics and Informatics (ICOEI), Tirunelveli, India, 3–5 June 2021; pp. 1375–1378. [ Google Scholar ]
- Khari, M.; Kumar, P. A novel approach for software test data generation using cuckoo algorithm. In Proceedings of the 2nd International Conference on Information and Communication Technology for Competitive Strategies, Udaipur, India, 4–5 March 2016; pp. 1–6. [ Google Scholar ]
- Alshraideh, M.; Bottaci, L. Search-based software test data generation for string data using program-specific search operators. Softw. Testing, Verif. Reliab. 2006 , 16 , 175–203. [ Google Scholar ] [ CrossRef ]
- Mairhofer, S.; Feldt, R.; Torkar, R. Search-based software testing and test data generation for a dynamic programming language. In Proceedings of the 13th Annual Conference on Genetic and Evolutionary Computation, Dublin, Ireland, 12–16 June 2011; pp. 1859–1866. [ Google Scholar ]
- McMinn, P. Search-based software test data generation: A survey. Softw. Testing Verif. Reliab. 2004 , 14 , 105–156. [ Google Scholar ] [ CrossRef ]
- De Lima, L.F.; Peres, L.M.; Grégio, A.R.A.; Silva, F. A Systematic Literature Mapping of Artificial Intelligence Planning in Software Testing. In Proceedings of the ICSOFT, Paris, France, 7–9 July 2020; pp. 152–159. [ Google Scholar ]
- Dahiya, S.S.; Chhabra, J.K.; Kumar, S. Application of artificial bee colony algorithm to software testing. In Proceedings of the 21st Australian Software Engineering Conference, Auckland, New Zealand, 6–9 April 2010; pp. 149–154. [ Google Scholar ]
- Mala, D.J.; Mohan, V.; Kamalapriya, M. Automated software test optimisation framework—An artificial bee colony optimisation-based approach. IET Softw. 2010 , 4 , 334–348. [ Google Scholar ] [ CrossRef ]
- Karnavel, K.; Santhoshkumar, J. Automated software testing for application maintenance by using bee colony optimization algorithms (BCO). In Proceedings of the International Conference on Information Communication and Embedded Systems (ICICES), Chennai, India, 21–22 February 2013; pp. 327–330. [ Google Scholar ]
- Lakshminarayana, P.; SureshKumar, T. Automatic generation and optimization of test case using hybrid cuckoo search and bee colony algorithm. J. Intell. Syst. 2021 , 30 , 59–72. [ Google Scholar ] [ CrossRef ]
- Lijuan, W.; Yue, Z.; Hongfeng, H. Genetic algorithms and its application in software test data generation. In Proceedings of the International Conference on Computer Science and Electronics Engineering, Hangzhou, China, 23–25 March 2012; Volume 2, pp. 617–620. [ Google Scholar ]
- Berndt, D.; Fisher, J.; Johnson, L.; Pinglikar, J.; Watkins, A. Breeding software test cases with genetic algorithms. In Proceedings of the 36th Annual Hawaii International Conference on System Sciences, Big Island, HI, USA, 6–9 January 2003; p. 10. [ Google Scholar ]
- Yang, S.; Man, T.; Xu, J.; Zeng, F.; Li, K. RGA: A lightweight and effective regeneration genetic algorithm for coverage-oriented software test data generation. Inf. Softw. Technol. 2016 , 76 , 19–30. [ Google Scholar ] [ CrossRef ]
- Khan, R.; Amjad, M. Automatic test case generation for unit software testing using genetic algorithm and mutation analysis. In Proceedings of the UP Section Conference on Electrical Computer and Electronics (UPCON), Allahabad, India, 4–6 December 2015; pp. 1–5. [ Google Scholar ]
- Dong, Y.; Peng, J. Automatic generation of software test cases based on improved genetic algorithm. In Proceedings of the International Conference on Multimedia Technology, Hangzhou, China, 26–28 July 2011; pp. 227–230. [ Google Scholar ]
- Bouchachia, A. An immune genetic algorithm for software test data generation. In Proceedings of the 7th International Conference on Hybrid Intelligent Systems (HIS 2007), Kaiserslautern, Germany, 17–19 September 2007; pp. 84–89. [ Google Scholar ]
- Peng, Y.P.; Zeng, B. Software Test Data Generation for Multiple Paths Based on Genetic Algorithms. Appl. Mech. Mater. 2013 , 263 , 1969–1973. [ Google Scholar ] [ CrossRef ]
- Cohen, K.B.; Hunter, L.E.; Palmer, M. Assessment of software testing and quality assurance in natural language processing applications and a linguistically inspired approach to improving it. In Trustworthy Eternal Systems via Evolving Software, Data and Knowledge, Proceedings of the 2nd International Workshop (EternalS 2012), Montpellier, France, 28 August 2012 ; Springer: Berlin/Heidelberg, Germany, 2012; pp. 77–90. [ Google Scholar ]
- Ahsan, I.; Butt, W.H.; Ahmed, M.A.; Anwar, M.W. A comprehensive investigation of natural language processing techniques and tools to generate automated test cases. In Proceedings of the 2nd International Conference on Internet of things, Data and Cloud Computing, Cambridge, UK, 22–23 March 2017; pp. 1–10. [ Google Scholar ]
- Ansari, A.; Shagufta, M.B.; Fatima, A.S.; Tehreem, S. Constructing test cases using natural language processing. In Proceedings of the 3rd International Conference on Advances in Electrical, Electronics, Information, Communication and Bio-Informatics (AEEICB), Chennai, India, 27–28 February 2017; pp. 95–99. [ Google Scholar ]
- Wang, H.; Bai, L.; Jiezhang, M.; Zhang, J.; Li, Q. Software testing data analysis based on data mining. In Proceedings of the 4th International Conference on Information Science and Control Engineering (ICISCE), Changsha, China, 21–23 July 2017; pp. 682–687. [ Google Scholar ]
- Last, M.; Friedman, M.; Kandel, A. Using data mining for automated software testing. Int. J. Softw. Eng. Knowl. Eng. 2004 , 14 , 369–393. [ Google Scholar ] [ CrossRef ]
- Yu, S.; Ai, J.; Zhang, Y. Software test data generation based on multi-agent. In Advances in Software Engineering, Proceedings of the International Conference on Advanced Software Engineering and Its Applications (ASEA 2009), Jeju Island, Republic of Korea, 10–12 December 2009 ; pp. 188–195.
- Dhavachelvan, P.; Uma, G. Complexity measures for software systems: Towards multi-agent based software testing. In Proceedings of the International Conference on Intelligent Sensing and Information Processing, Chennai, India, 4–7 January 2005; pp. 359–364. [ Google Scholar ]
- Tang, J. Towards automation in software test life cycle based on multi-agent. In Proceedings of the International Conference on Computational Intelligence and Software Engineering, Wuhan, China, 10–12 December 2010; pp. 1–4. [ Google Scholar ]
- Alyahya, S. Collaborative Crowdsourced Software Testing. Electronics 2022 , 11 , 3340. [ Google Scholar ] [ CrossRef ]
- Górski, T. The k + 1 Symmetric Test Pattern for Smart Contracts. Symmetry 2022 , 14 , 1686. [ Google Scholar ] [ CrossRef ]
- Bijlsma, A.; Passier, H.J.; Pootjes, H.; Stuurman, S. Template Method test pattern. Inf. Process. Lett. 2018 , 139 , 8–12. [ Google Scholar ] [ CrossRef ]
- López, J.; Kushik, N.; Yevtushenko, N. Source code optimization using equivalent mutants. Inf. Softw. Technol. 2018 , 103 , 138–141. [ Google Scholar ] [ CrossRef ]
- Segura, S.; Troya, J.; Durán, A.; Ruiz-Cortés, A. Performance metamorphic testing: A proof of concept. Inf. Softw. Technol. 2018 , 98 , 1–4. [ Google Scholar ] [ CrossRef ]
- Harzing, A.W.; Alakangas, S. Google Scholar, Scopus and the Web of Science: A longitudinal and cross-disciplinary comparison. Scientometrics 2016 , 106 , 787–804. [ Google Scholar ] [ CrossRef ]
- Norris, M.; Oppenheim, C. Comparing alternatives to the Web of Science for coverage of the social sciences’ literature. J. Inf. 2007 , 1 , 161–169. [ Google Scholar ] [ CrossRef ]
- Chadegani, A.A.; Salehi, H.; Yunus, M.M.; Farhadi, H.; Fooladi, M.; Farhadi, M.; Ebrahim, N.A. A comparison between two main academic literature collections: Web of Science and Scopus databases. arXiv 2013 , arXiv:1305.0377. [ Google Scholar ] [ CrossRef ]
- Feng, X.W.; Hadizadeh, M.; Cheong, J.P.G. Global Trends in Physical-Activity Research of Autism: Bibliometric Analysis Based on the Web of Science Database (1980–2021). Int. J. Environ. Res. Public Health 2022 , 19 , 7278. [ Google Scholar ] [ CrossRef ] [ PubMed ]
- Skute, I. Opening the black box of academic entrepreneurship: A bibliometric analysis. Scientometrics 2019 , 120 , 237–265. [ Google Scholar ] [ CrossRef ]
- Zhao, D.; Strotmann, A. Analysis and visualization of citation networks. Synth. Lect. Inf. Concepts Retr. Serv. 2015 , 7 , 1–207. [ Google Scholar ]
- Ma, X.; Zhang, L.; Wang, J.; Luo, Y. Knowledge domain and emerging trends on echinococcosis research: A scientometric analysis. Int. J. Environ. Res. Public Health 2019 , 16 , 842. [ Google Scholar ] [ CrossRef ] [ PubMed ]
- Lee, Y.C.; Chen, C.; Tsai, X.T. Visualizing the knowledge domain of nanoparticle drug delivery technologies: A scientometric review. Appl. Sci. 2016 , 6 , 11. [ Google Scholar ] [ CrossRef ]
- Aria, M.; Cuccurullo, C. bibliometrix: An R-tool for comprehensive science mapping analysis. J. Inf. 2017 , 11 , 959–975. [ Google Scholar ] [ CrossRef ]
- Van Eck, N.; Waltman, L. Software survey: VOSviewer, a computer program for bibliometric mapping. Scientometrics 2010 , 84 , 523–538. [ Google Scholar ] [ CrossRef ]
- Chen, C. Science mapping: A systematic review of the literature. J. Data Inf. Sci. 2017 , 2 , 1–40. [ Google Scholar ] [ CrossRef ]
- Negahban, M.B.; Zarifsanaiey, N. Network analysis and scientific mapping of the e-learning literature from 1995 to 2018. Knowl. Manag. E-Learn. Int. J. 2020 , 12 , 268–279. [ Google Scholar ]
- Cobo, M.J.; López-Herrera, A.G.; Herrera-Viedma, E.; Herrera, F. An approach for detecting, quantifying, and visualizing the evolution of a research field: A practical application to the Fuzzy Sets Theory field. J. Inf. 2011 , 5 , 146–166. [ Google Scholar ] [ CrossRef ]
- Matute, J.; Linsen, L. Evaluating Data-type Heterogeneity in Interactive Visual Analyses with Parallel Axes. In Proceedings of the Computer Graphics Forum, Reims, France, 25–29 April 2022. [ Google Scholar ]
- Ejaz, H.; Zeeshan, H.M.; Ahmad, F.; Bukhari, S.N.A.; Anwar, N.; Alanazi, A.; Sadiq, A.; Junaid, K.; Atif, M.; Abosalif, K.O.A.; et al. Bibliometric Analysis of Publications on the Omicron Variant from 2020 to 2022 in the Scopus Database Using R and VOSviewer. Int. J. Environ. Res. Public Health 2022 , 19 , 12407. [ Google Scholar ] [ CrossRef ]
- Jaffe, K.; Ter Horst, E.; Gunn, L.H.; Zambrano, J.D.; Molina, G. A network analysis of research productivity by country, discipline, and wealth. PLoS ONE 2020 , 15 , e0232458. [ Google Scholar ] [ CrossRef ] [ PubMed ]
Click here to enlarge figure
Description | Results |
---|---|
Timespan | 2012–2022 |
Sources | 162 |
Documents | 336 |
Average number of citations per document | 12.88 |
References | 11,280 |
Annual growth rate | 14.87% |
Keywords Used by Authors (DE) | 1075 |
Keywords Plus (ID) | 280 |
Authors of Single-Authored Documents | 20 |
Authors of Multi-Authored Documents | 1033 |
Single-authored documents | 20 |
Co-authors per document | 3.64 |
International Co-authorships | 26.79% |
Country | Articles | Frequency | Single-Country Publication | Multiple-Country Publication | Multiple-Country Publication Ratio |
---|---|---|---|---|---|
China | 67 | 0.199 | 55 | 12 | 0.179 |
India | 48 | 0.143 | 47 | 1 | 0.021 |
USA | 27 | 0.080 | 19 | 8 | 0.296 |
Brazil | 13 | 0.039 | 11 | 2 | 0.154 |
Malaysia | 13 | 0.039 | 7 | 6 | 0.462 |
Germany | 10 | 0.030 | 7 | 3 | 0.300 |
Korea | 9 | 0.027 | 7 | 2 | 0.222 |
Spain | 9 | 0.027 | 5 | 4 | 0.444 |
United Kingdom | 9 | 0.027 | 5 | 4 | 0.444 |
Turkey | 8 | 0.024 | 4 | 4 | 0.500 |
Author, Year, Journal | Article Title | Total Citations | Total Citations per Year |
---|---|---|---|
CHEN TY, 2013, JOURNAL OF SYSTEMS AND SOFTWARE | AN ORCHESTRATED SURVEY OF METHODOLOGIES FOR AUTOMATED SOFTWARE TEST CASE GENERATION | 326 | 29.636 |
ARCURI A, 2014, ACM TRANSACTIONS ON SOFTWARE ENGINEERING AND METHODOLOGY | A LARGE-SCALE EVALUATION OF AUTOMATED UNIT TEST GENERATION USING EVOSUITE | 113 | 11.3 |
ARCURI A, 2013, IEEE TRANSACTIONS ON SOFTWARE ENGINEERING | GENERATING TEST DATA FROM OCL CONSTRAINTS WITH SEARCH TECHNIQUES | 88 | 8 |
ALI S, 2013, IEEE TRANSACTIONS ON SOFTWARE ENGINEERING | GENERATING TEST DATA FROM OCL CONSTRAINTS WITH SEARCH TECHNIQUES | 88 | 8 |
GAROUSI V, 2016, INFORMATION AND SOFTWARE TECHNOLOGY | WHEN AND WHAT TO AUTOMATE IN SOFTWARE TESTING? A MULTI-VOCAL LITERATURE REVIEW | 77 | 9.625 |
ARCURI A, 2015, EMPIRICAL SOFTWARE ENGINEERING | ACHIEVING SCALABLE MUTATION-BASED GENERATION OF WHOLE TEST SUITES | 64 | 7.111 |
KHARI M, 2019, SOFT COMPUTING | AN EXTENSIVE EVALUATION OF SEARCH-BASED SOFTWARE TESTING: A REVIEW | 24 | 4.8 |
KHARI M, 2013, INTERNATIONAL JOURNAL OF BIO-INSPIRED COMPUTATION | HEURISTIC SEARCH-BASED APPROACH FOR AUTOMATED TEST DATA GENERATION: A SURVEY | 23 | 2.091 |
KHARI M, 2018, SOFT COMPUTING | OPTIMIZED TEST SUITES FOR AUTOMATED TESTING USING DIFFERENT OPTIMIZATION TECHNIQUES | 22 | 3.667 |
GONG DW, 2016, AUTOMATED SOFTWARE ENGINEERING | TEST DATA GENERATION FOR PATH COVERAGE OF MESSAGE-PASSING PARALLEL PROGRAMS BASED ON CO-EVOLUTIONARY GENETIC ALGORITHMS | 18 | 2.25 |
Documents Written | Number of Authors | Proportion of Authors |
---|---|---|
1 | 927 | 0.880 |
2 | 96 | 0.091 |
3 | 23 | 0.022 |
4 | 3 | 0.003 |
5 | 2 | 0.002 |
6 | 2 | 0.002 |
The statements, opinions and data contained in all publications are solely those of the individual author(s) and contributor(s) and not of MDPI and/or the editor(s). MDPI and/or the editor(s) disclaim responsibility for any injury to people or property resulting from any ideas, methods, instructions or products referred to in the content. |
Share and Cite
Boukhlif, M.; Hanine, M.; Kharmoum, N. A Decade of Intelligent Software Testing Research: A Bibliometric Analysis. Electronics 2023 , 12 , 2109. https://doi.org/10.3390/electronics12092109
Boukhlif M, Hanine M, Kharmoum N. A Decade of Intelligent Software Testing Research: A Bibliometric Analysis. Electronics . 2023; 12(9):2109. https://doi.org/10.3390/electronics12092109
Boukhlif, Mohamed, Mohamed Hanine, and Nassim Kharmoum. 2023. "A Decade of Intelligent Software Testing Research: A Bibliometric Analysis" Electronics 12, no. 9: 2109. https://doi.org/10.3390/electronics12092109
Article Metrics
Article access statistics, further information, mdpi initiatives, follow mdpi.
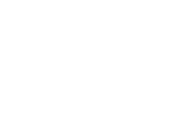
Subscribe to receive issue release notifications and newsletters from MDPI journals
Academia.edu no longer supports Internet Explorer.
To browse Academia.edu and the wider internet faster and more securely, please take a few seconds to upgrade your browser .
- We're Hiring!
- Help Center
Software Testing
- Most Cited Papers
- Most Downloaded Papers
- Newest Papers
- Last »
- Software Engineering Follow Following
- Empirical Software Engineering Follow Following
- Software Architecture Follow Following
- Computer Science Follow Following
- Software Maintenance Follow Following
- Software Development Follow Following
- Software Engineering education Follow Following
- Software Testing (Computer Science) Follow Following
- Search Based Software Engineering Follow Following
- Requirements Engineering Follow Following
Enter the email address you signed up with and we'll email you a reset link.
- Academia.edu Journals
- We're Hiring!
- Help Center
- Find new research papers in:
- Health Sciences
- Earth Sciences
- Cognitive Science
- Mathematics
- Computer Science
- Academia ©2024
IEEE Account
- Change Username/Password
- Update Address
Purchase Details
- Payment Options
- Order History
- View Purchased Documents
Profile Information
- Communications Preferences
- Profession and Education
- Technical Interests
- US & Canada: +1 800 678 4333
- Worldwide: +1 732 981 0060
- Contact & Support
- About IEEE Xplore
- Accessibility
- Terms of Use
- Nondiscrimination Policy
- Privacy & Opting Out of Cookies
A not-for-profit organization, IEEE is the world's largest technical professional organization dedicated to advancing technology for the benefit of humanity. © Copyright 2024 IEEE - All rights reserved. Use of this web site signifies your agreement to the terms and conditions.
- Venue: Sheraton Grand, Sacramento, California
- Accommodations
- Diversity and Inclusion Plan
- Code of Conduct
- Travel Support
- Visa Letters
- Social Events
- ASE 2024 Keynotes
- Registration
- Sponsorship
Week Overview
- Research Papers
- Industry Showcase
- Journal-first Papers
- Artifact Evaluation Track
- Tool Demonstrations
- Student Research Competition
- Most Influential Papers (MIP)
- Doctoral Symposium
- [Workshop] RENE
- [Workshop] ASYDE
- [Workshop] HCSE&CS
- [Workshop] A-Mobile
- [Workshop] VARSE
- ASE 2024 Committees
- Organizing Committee
- Track Committees
- Student Research Competition Chair
- Program Committee
- Contributors
- People Index
- Past Editions
Please note that the main ASE conference will be from Tuesday to Thursday (October 29-31)
This is an overview of the ASE week:
Monday Oct 28 | Tuesday Oct 29 | Wednesday Oct 30 | Thursday Oct 31 | Friday Nov 1 |
---|---|---|---|---|
Workshops: RENE ASYDE HCSE&CS Tutorials Doctoral Symposium Reception | Main Conference Keynotes Tool Demos Posters Student Research Competition | Workshops: A-Mobile VARSE Tutorials | ||
Banquet |
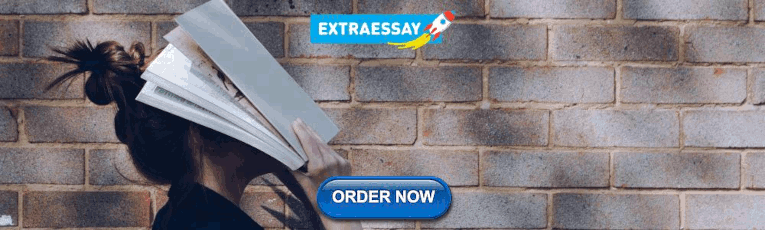
IMAGES
COMMENTS
Software testing is the process of evaluating software applications to determine whether there are any defects, ensuring the quality of the software product. However, software testing is generally ...
Artificial Intelligence is gradually changing the landscape of software engineering in general [5] and software testing in particular [6] both in research and industry as well. In the last two decades, AI has been found to have made a considerable impact on the way we are approach-ing software testing.
Software testing is a crucial component of software development. With the increasing complexity of software systems, traditional manual testing methods are becoming less feasible. Artificial Intelligence (AI) has emerged as a promising approach to software testing in recent years. This review paper aims to provide an in-depth understanding of the current state of software testing using AI. The ...
This paper aims to discuss the existing as well as improved testing techniques for the better quality assurance purposes. Published in: Article #: Date of Conference: 22-24 November 2016. Date Added to IEEE Xplore: 16 January 2017. ISBN Information: Electronic ISBN: 978-1-5090-4521-1. Print on Demand (PoD) ISBN: 978-1-5090-4522-8.
This paper provides a comprehensive review of various software testing models and optimization techniques available in the literature, emphasizing their performance analysis and related research ...
Abstract: Software Testing is a process, which involves, executing of a software program/application and finding all errors or bugs in that program/application so that the result will be a defect-free software. Quality of any software can only be known through means of testing (software testing). Through the advancement of technology around the world, there increased the number of verification ...
Software Testing, Verification and Reliability (STVR) is an international journal, publishing 8 issues per year. It publishes papers on theoretical and practical issues of software testing, verification and reliability. The goal of the journal is to publish high-quality papers that help researchers, educators and practitioners understand ...
The three basic steps in the software testing are Unit testing, Integration testing and System testing. Each of these steps is. either tested by the software d eveloper or the quality. assurance ...
All the research work is maintained in a sequential manner, and ambiguous and unrelated studies were neglected. As this work is related to software testing techniques, there were an acceptance and excluding criteria to shortlist papers based on software testing techniques. Acceptance criteria are as follows: 1.
Owing to these variations, numerous software testing challenges occur. This paper aims to recognize and to explain some of the biggest challenges that software testers face in dealing with AI/ML applications. For future research, this study has key implications. Each of the challenges outlined in this paper is ideal for further investigation ...
Despite the efforts of the software testing research community in the last several decades, various studies show that still many practitioners in the industry report challenges in their software testing tasks. ... We conclude that the focal points of industrial work and academic research in software testing differ. Furthermore, the paper at ...
Context: Artificial intelligence (AI) methods and models have extensively been applied to support different phases of the software development lifecycle, including software testing (ST). Several secondary studies investigated the interplay between AI and ...
Internal Validity: We based our analysis on publications retrieved using the term "software testing". This pool of papers included publications unrelated to software testing, e.g., the use of software to test hardware or as part of student examination. ... Research in software testing is growing and rapidly-evolving. Based on the keywords ...
In this study, we used the Web of Science database to acquire bibliometric data about intelligent software testing papers which were conducted between 2012 and 2022, and we used Biblioshiny from the R bibliomerix package, alongside with VOSViewer in order to analyze the data and extract insights and answer research questions about the authors ...
Software Testing with Large Language Models: Survey, Landscape, and Vision Junjie Wang, Yuchao Huang, Chunyang Chen, Zhe Liu, Song Wang, Qing Wang ... from both the software testing and LLMs perspectives. The paper presents a detailed discussion of the software testing tasks for ... research in this area, highlighting potential avenues for ex- ...
There have been rapid recent developments in automated software test design, repair and program improvement. Advances in artificial intelligence also have great potential impact to tackle software testing research problems. In this paper we highlight open research problems and challenges from an industrial perspective. This perspective draws on our experience at Meta Platforms, which has been ...
To more clearly understand, characterize and distinguish software-testing education in universities from training in industry, we model the concepts as a context diagram, as shown in Fig. 1.To clarify the focus of this SLM paper, we have highlighted our focus with a grey background in Fig. 1.On the left-hand side of Fig. 1 are the higher-education institutions that train students and produce ...
Binary Test-Suites Using Covering Arrays. Software testing is an essential activity to ensure the quality of software systems. Combinatorial testing is a method that facilitates the software testing process; it is based on an empirical evidence where almost all faults in a... more. Download. by Himer Avila-George. 2.
Online ISSN: 0975-4172 & Print ISSN: 0975-4350. Review Paper on Various Software Testing Techniques &. Strategies. By Nahid Anwar & Susmita Kar. Banglades h University of Business and Technology ...
Software Testing: A Comparative Study. Model Based Testing VS Test Case Based Testing. Rakesh Reddy Polamreddy Syed Ali Irtaza. ii. This thesis is submitted to the School of Engineering at Blekinge Institute of Technology in partial fulfillment of the requirements for the degree of Master of Science in Software Engineering.
Also, ML has been used to evaluate test oracle construction and to predict the cost of testing-related activities. The results of this paper outline the ML algorithms that are most commonly used to automate software-testing activities, helping researchers to understand the current state of research concerning ML applied to software testing.
1 Paper 141-30 Software Testing Fundamentals—Concepts, Roles, and Terminology John E. Bentley, Wachovia Bank, Charlotte NC ABSTRACT SAS® software provides a complete set of application development tools for building stand-alone, client-server, and Internet-enabled applications, and SAS Institute provides excellent training in using their software.
Explore the latest full-text research PDFs, articles, conference papers, preprints and more on SOFTWARE QUALITY ASSURANCE. Find methods information, sources, references or conduct a literature ...
Welcome to the website of the 39th IEEE/ACM International Conference on Automated Software Engineering (ASE 2024). The ASE conference is the premier research forum for Automated Software Engineering. Each year, it brings together researchers and practitioners from academia and industry to discuss foundations, techniques, and tools for automating the analysis, design, implementation, testing ...