Advertisement
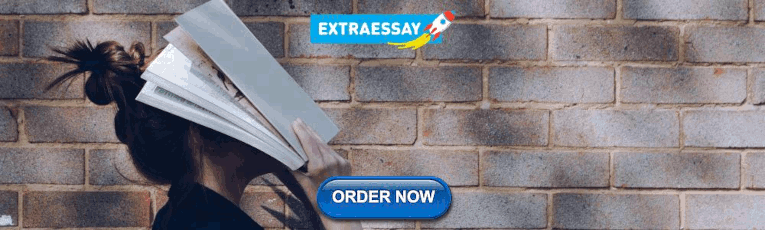
Trends in insomnia research for the next decade: a narrative review
- Review Article
- Published: 06 April 2020
- Volume 18 , pages 199–207, ( 2020 )
Cite this article
- Daniel Ruivo Marques 1 , 2 ,
- Ana Allen Gomes 2 , 3 ,
- Vanda Clemente 2 , 4 ,
- José Moutinho dos Santos 4 ,
- Joana Serra 4 &
- Maria Helena Pinto de Azevedo 5
706 Accesses
11 Citations
Explore all metrics
Insomnia disorder has known striking developments over the last few years. Partly due to advances in neuroimaging techniques and brain sciences, our understanding of insomnia disorder has become more fine-tuned. Besides, developments within psychological and psychiatric fields have contributed to improve conceptualization, assessment, and treatment of insomnia. In this paper, we present a list of promising 10 key “hot-topics” that we think in the next 10 years will continue to stimulate researchers in insomnia’s domain: increasing of systematic reviews and meta-analyses; improvement of existing self-report measures; increasing of genetic and epigenetic investigation; research on new pharmacological agents; advances in neuroimaging studies and methods; new psychological clinical approaches; effectiveness studies of e-treatments and greater dissemination of evidence-based therapies for insomnia; call for integrative models; network approach using in insomnia; and assessment of insomnia phenotypes. The breadth of all these topics demands the collaboration of researchers from different scientific fields within sleep medicine. In summarizing, in the next decade, it is predictable that insomnia’s research still benefit from different scientific disciplines.
This is a preview of subscription content, log in via an institution to check access.
Access this article
Subscribe and save.
- Get 10 units per month
- Download Article/Chapter or eBook
- 1 Unit = 1 Article or 1 Chapter
- Cancel anytime
Price includes VAT (Russian Federation)
Instant access to the full article PDF.
Rent this article via DeepDyve
Institutional subscriptions
Similar content being viewed by others
Pain-Insomnia-Depression Syndrome: Triangular Relationships, Pathobiological Correlations, Current Treatment Modalities, and Future Direction
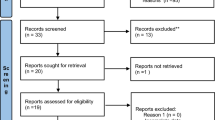
Insomnia and the Orexinergic Pathway in the Link with Psychopathology: Effects of DORAs in Insomnia Comorbid with Mental Disturbances
American Academy of Sleep Medicine. International classification of sleep disorders: diagnostic and coding manual. 3rd ed. Westchester: American Academy of Sleep Medicine; 2014.
Google Scholar
American Psychiatric Association. Diagnostic and statistical manual of mental disorders-5. 5th ed. Washington, DC: American Psychiatric Association; 2013.
Book Google Scholar
Baglioni C, Regen W, Teghen A, Spiegelhalder K, Feige B, Nissen C, Riemann D. Sleep changes in the disorder of insomnia: a meta-analysis of polysomnographic studies. Sleep Med Rev. 2014;18(3):195–21313. https://doi.org/10.1016/j.smrv.2013.04.001 .
Article PubMed Google Scholar
Bastien CH, Vallières A, Morin CM. Validation of the Insomnia Severity index as an outcome measure for insomnia research. Sleep Med. 2001;2(4):297–307. https://doi.org/10.1016/S1389-9457(00)00065-4 .
Berman M, Jonides J, Nee D. Studying mind and brain with fMRI. Soc Cogn Affect Neurosci. 2006;1(2):158–61. https://doi.org/10.1093/scan/nsl019 .
Article PubMed PubMed Central Google Scholar
Bheemsain T, Kar S. An overview of insomnia management. Delphi Psychiatry J. 2012;15(2):294–301.
Blanken T, Benjamins J, Borsboom D, Vermunt J, Paquola C, Ramautar J, Van Someren E. Insomnia disorder subtypes derived from life history and traits of affect and personality. Lancet Psychiatry. 2019;6(2):151–63. https://doi.org/10.1016/S2215-0366(18)30464-4 .
Borsboom D, Cramer A. Network analysis: an integrative approach to the structure of psychopathology. Annu Rev Clin Psychol. 2013;9(1):91–121. https://doi.org/10.1146/annurev-clinpsy-050212-185608 .
Borsboom D. A network theory of mental disorders. World Psychiatry. 2017;16(1):5–13. https://doi.org/10.1002/wps.20375 .
Bragantini D, Sivertsen B, Gehrman P, Lydersen S, Güzey IC. Genetic polymorphisms associated with sleep-related phenotypes; relationships with individual nocturnal symptoms of insomnia in the HUNT study. BMC Med Genet. 2019;20(1):179. https://doi.org/10.1186/s12881-019-0916 .
Broomfield N, Espie C. Towards a valid, reliable measure of sleep effort. J Sleep Res. 2005;14(4):401–7. https://doi.org/10.1111/j.1365-2869.2005.00481.x .
Busto U, Sykora K, Sellers E. A clinical scale to assess benzodiazepine withdrawal. J Clin Psychopharmacol. 1989;9(6):412–6. https://doi.org/10.1097/00004714-198912000-00005 .
Article CAS PubMed Google Scholar
Buysse D, Ancoli-Israel S, Edinger J, Lichstein K, Morin C. Recommendations for a standard research assessment of insomnia. Sleep. 2006;29(9):1155–73. https://doi.org/10.1093/sleep/29.9.1155 .
Buysse D, Germain A, Hall M, Monk T, Nofzinger E. A neurobiological model of insomnia. Drug Discov Today Dis Models. 2011;8(4):129–37. https://doi.org/10.1016/j.ddmod.2011.07.002 .
Buysse D, Harvey A. Insomnia: recent developments and future directions. In: Kryger M, Roth T, Dement W, editors. Principles and practices of sleep medicine. 6th ed. Philadelphia: Elsevier; 2017. p. 757–760.
Chapter Google Scholar
Buysse DJ, Reynolds CF, Monk TH, Berman SR, Kupfer DJ. The Pittsburgh sleep quality index: a new instrument for psychiatric practice and research. Psychiatry Res. 1989;28:193–21313. https://doi.org/10.1016/0165-1781(89)90047-4 .
Cassano GB, Petracca A, Cesana BM. A new scale for the evaluation of benzodiazepine withdrawal symptoms: sessb. Curr Therapeutic Res. 1994;55(3):275–89. https://doi.org/10.1016/s0011-393x(05)80171-7 .
Article Google Scholar
Dekker K, Blanken T, Van Someren E. Insomnia and personality: a network approach. Brain Sci. 2017;7(3):28. https://doi.org/10.3390/brainsci7030028 .
Article PubMed Central Google Scholar
Edinger J, Leggett M, Carney C, Manber R. Psychological and behavioral treatments for insomnia II: implementation and specific populations. In: Kryger M, Roth T, Dement W, editors. Principles and practices of sleep medicine. 6th ed. Philadelphia: Elsevier; 2017. p. 814–831.
Eisai Global. U.S. FDA approves Eisai’s Dayvigo TM (Lemborexant) for treatment of insomnia in adult patients. 2019. https://www.eisai.com/news/2019/news201993.html . Accessed 31 Jan 2020.
Espie C, Kyle S, Williams C, Ong J, Douglas N, Hames P, Brown J. A randomized, placebo-controlled trial of online cognitive behavioral therapy for chronic insomnia disorder delivered via an automated media-rich web application. Sleep. 2012;35(6):769–81. https://doi.org/10.5665/sleep.1872 .
Fernandez-Mendoza J. The insomnia with short sleep duration phenotype: an update on it’s importance for health and prevention. Curr Opin Psychiatry. 2017;30(1):56–63. https://doi.org/10.1097/YCO.0000000000000292 .
Field A, Gillett R. How to do a meta-analysis. Br J Math Stat Psychol. 2010;63:665–94. https://doi.org/10.1348/000711010X502733 .
Gehrman P, Pfeiffenberger C, Byrne E. The role of genes in the insomnia phenotype. Sleep Med Clin. 2013;8(3):323–31. https://doi.org/10.1016/j.jsmc.2013.04.005 .
Gilbert P. The origins and nature of compassion focused therapy. Br J Clin Psychol. 2014;53(1):6–41. https://doi.org/10.1111/bjc.12043 .
Gregory AM, Rijsdijk FV, Eley TC, Buysse DJ, Schneider MN, Parsons M, Barclay NL. A longitudinal twin and sibling study of associations between insomnia and depression symptoms in young adults. Sleep. 2016;39(11):1985–92. https://doi.org/10.5665/sleep.6228 .
Hadian S, Jabalameli S. The effectiveness of compassion-focused therapy (CFT) on rumination in students with sleep disorders: a quasi-experimental research, before and after. J Urmia Univ Med Sci. 2019;30(2):86–96.
Hein M, Lanquart J-P, Loas G, Hubain P, Linkowski P. Similar polysomnographic pattern in primary insomnia and major depression with objective insomnia: a sign of common pathophysiology?. BMC Psychiatry. 2017. https://doi.org/10.1186/s12888-017-1438-4
Hertenstein E, Feige B, Gmeiner T, Kienzler C, Spiegelhalder K, Johann A, Baglioni C. Insomnia as a predictor of mental disorders: a systematic review and meta-analysis. Sleep Med Rev. 2019;43:96–105. https://doi.org/10.1016/j.smrv.2018.10.006 .
Jansen P, Watanabe K, Stringer S, Skene N, Bryois J, Posthuma D. Genome-wide analysis of insomnia in 1,331,010 individuals identifies new risk loci and functional pathways. Nat Genet. 2019;51:394–403. https://doi.org/10.1038/s41588-018-0333-3 .
Jones S, van Hees V, Mazzotti D, Marques-Vidal P, Sabia S, van der Spek A, Wood A. Genetic studies of accelerometer-based sleep measures in 85,670 individuals yield new insights into human sleep behaviour. Nat Commun. 2018;10(1):1585. https://doi.org/10.1038/s41467-019-09576-1 .
Article CAS Google Scholar
Kobayashi M, Okajima I, Narisawa H, Kikuchi T, Matsui K, Inada K, Inoue Y. Development of a new benzodiazepine hypnotics withdrawal symptom scale. Sleep Biol Rhythm. 2018;16(3):263–71. https://doi.org/10.1007/s41105-018-0151-0 .
Lane J, Jones S, Dashti H, Wood A, Aragam K, Saxena R. Biological and clinical insights from genetics of insomnia symptoms. Nat Genet. 2019;51:387–93. https://doi.org/10.1038/s41588-019-0361-7 .
Article CAS PubMed PubMed Central Google Scholar
Lilenfeld S. What is “evidence” in psychotherapies? World Psychiatry. 2019;18(3):245–6. https://doi.org/10.1002/wps.20654 .
Lind MJ, Gehrman PR. Genetic pathways to insomnia. Brain Sci. 2016;6(4):64. https://doi.org/10.3390/brainsci6040064 .
Article CAS PubMed Central Google Scholar
Ma Z-R, Shi L-J, Deng M-H. Efficacy of cognitive behavioral therapy in children and adolescents with insomnia: a systematic review and meta-analysis. Braz J Med Biol Res. 2018;51(6):e7070. https://doi.org/10.1590/1414-431X20187070 .
Marques D. Do we need neuroimaging to treat insomnia effectively? Sleep Med. 2019;53:205. https://doi.org/10.1016/j.sleep.2017.08.005 .
Marques D. “Time to relax”: considerations on relaxation training for insomnia disorder. Sleep Biol Rhythm. 2019;17(2):263–4. https://doi.org/10.1007/s41105-018-00203-y .
Marques D. Self-report measures as complementary exams in the diagnosis of insomnia. Revista Portuguesa de Investigação Comportamental e Social. 2020. [Accepted for publication] .
Marques D, Azevedo MH. Potentialities of network analysis for sleep medicine. J Psychosom Res. 2018;111:89–90. https://doi.org/10.1016/j.jpsychores.2018.05.019 .
Marques D, Clemente V, Gomes A, Azevedo MH. Profiling insomnia using subjective measures: where are we and where are we going. Sleep Med. 2018;43:103–4. https://doi.org/10.1016/j.sleep.2017.12.006 .
Marques D, Gomes A, Azevedo MH. Utility of studies in community-based populations. 2019. [Manuscript submitted for publication] .
Marques D, Gomes A, Caetano G, Castelo-Branco M. Insomnia disorder and brain’s default-mode network. Curr Neurol Neurosci Rep. 2018;18(8):45. https://doi.org/10.1007/s11910-018-0861-3 .
Marques D, Gomes A, Clemente V, Santos J, Castelo-Branco M. Default-mode network activity and its role in comprehension and management of psychophysiological insomnia: a new perspective. New Ideas Psychol. 2015;36:30–7. https://doi.org/10.1016/j.newideapsych.2014.08.001 .
Marques D, Gomes A, Clemente V, Santos J, Duarte I, Caetano G, Castelo-Branco M. Unbalanced resting-state networks activity in psychophysiological insomnia. Sleep Biol Rhythm. 2017;15(2):167–77. https://doi.org/10.1007/s41105-017-0096-8 .
Marques D, Gomes AA, Clemente V, Moutinho J, Caetano G, Castelo-Branco M. An overview regarding insomnia disorder: conceptualization, assessment and treatment. In: Columbus AM, editor. Advances in psychology research. New York: Nova Science Publishers Inc; 2016. p. 81–116.
Marques D, Gomes A, Clemente V, Santos J, Caetano G, Castelo-Branco M. Neurobiological correlates of psychological treatments for insomnia: a review. Eur Psychol. 2016;21(3):195–205. https://doi.org/10.1027/1016-9040/a000264 .
McNally R. Can network analysis transform psychopathology? Behav Res Ther. 2016;86:95–104. https://doi.org/10.1016/j.brat.2016.06.006 .
Morales-Lara D, De-la-Peña C, Murillo-Rodríguez E. Dad’s snoring may have left molecular scars in your DNA: the emerging role of epigenetics in sleep disorders. Mol Neurobiol. 2018;55(4):2713–24. https://doi.org/10.1007/s12035-017-0409-6 .
Morin C. Contributions of cognitive-behavioral approaches to the clinical management of insomnia. Prim Care Companion J Clin Psychiatry. 2002;4(1):21–6.
Morin C, Vallières A, Ivers H. Dysfunctional Beliefs and Attitudes about Sleep (DBAS): validation of a brief version (DBAS-16). Sleep. 2007;30(11):1547–54. https://doi.org/10.1093/sleep/30.11.1547 .
Ong J, Manber R, Segal Z, Xia Y, Shapiro S, Wyatt J. A randomized controlled trial of mindfulness meditation for chronic insomnia. Sleep. 2014;37(9):1553–63. https://doi.org/10.5665/sleep.4010 .
Owen M, Cardno A, O’Donovan M. Psychiatric genetics: back to the future. Mol Psychiatry. 2000;5(1):22–31. https://doi.org/10.1038/sj.mp.4000702 .
Päivi L, Sitwat L, Harri O-K, Joona M, Raimo L. ACT for sleep—internet-delivered self-help ACT for sub-clinical and clinical insomnia: a randomized controlled trial. J Context Behav Sci. 2019;12:119–27. https://doi.org/10.1016/j.jcbs.2019.04.001 .
Palagini L, Biber K, Riemann D. The genetics of insomnia–evidence for epigenetic mechanisms? Sleep Med Rev. 2014;18(3):225–35. https://doi.org/10.1016/j.smrv.2013.05.002 .
Perlis M, Ellis J, Kloss J, Riemann D. Etiology and pathophysiology of insomnia. In: Kryger M, Roth T, Dement W, editors. Principles and practices of sleep medicine. 6th ed. Philadelphia: Elsevier; 2017. p. 769–784.
Perlis M, Shaw P, Cano G, Espie C. Models of insomnia. In: Kryger M, Roth T, Dement W, editors. Principles and practice of sleep medicine. 5th ed. Missouri: Elsevier Saunders; 2011. p. 850–865.
Qureshi I, Mehler M. Epigenetics of sleep and chronobiology. Curr Neurol Neurosci Rep. 2014;14(3):432. https://doi.org/10.1007/s11910-013-0432-6 .
Riemann D, Baglioni C, Bassetti C, Bjorvatn B, Dolenc Groselj L, Ellis J, Spiegelhalder K. European guideline for the diagnosis and treatment of insomnia. J Sleep Res. 2017;26(6):675–700. https://doi.org/10.1111/jsr.12594 .
Riemann D, Spiegelhalder K, Feige B, Voderholzer U, Berger M, Perlis M, Nissen C. The hyperarousal model of insomnia: a review of the concept and its evidence. Sleep Med Rev. 2010;14(1):19–311. https://doi.org/10.1016/j.smrv.2009.04.002 .
Riemann D, Spiegelhalder K, Nissen C, Hirscher V, Baglioni C, Feige B. REM sleep instability: a new pathway for insomnia? Pharmacopsychiatry. 2012;45(5):167–76. https://doi.org/10.1055/s-0031-1299721 .
Rodriguez M, Maeda Y. Meta-analysis of coefficient alpha. Psychol Methods. 2006;11(3):306–22. https://doi.org/10.1037/1082-989X.11.3.306 .
Schulz H, Salzarulo P. The development of sleep medicine: a historical sketch. J Clin Sleep Med. 2016;12(7):1041–52. https://doi.org/10.5664/jcsm.5946 .
Siddaway A, Wood A, Hedges L. How to do a systematic review: a best practice guide for conducting and reporting narrative reviews, meta-analyses, and meta-syntheses. Annu Rev Psychol. 2019;70:747–70. https://doi.org/10.1146/annurev-psych-010418-102803 .
Sirois F, Nauts S, Molnar D. Self-compassion and bedtime procrastination: an emotion regulation perspective. Mindfulness. 2019;10(3):434–45. https://doi.org/10.1007/s12671-018-0983-3 .
Spiegelhalder K, Regen W, Baglioni C, Nissen C, Riemann D, Kyle S. Neuroimaging insights into insomnia. Curr Neurol Neurosci Rep. 2015;15(3):9. https://doi.org/10.1007/s11910-015-0527-3 .
Stein M, McCarthy M, Chen C, Jain S, Gelernter J, He F, Ursano R. Genome-wide analysis of insomnia disorder. Mol Psychiatry. 2018;23(11):2238–50. https://doi.org/10.1038/s41380-018-0033-5 .
Stepanski E. Behavioral sleep medicine: a historical perspective. Behav Sleep Med. 2003;1(1):4–21. https://doi.org/10.1207/S15402010BSM0101_3 .
Tam V, Patel N, Turcotte M, Bossé Y, Paré G, Meyre D. Benefits and limitations of genome-wide association studies. Nat Rev Genet. 2019;20(8):467–84. https://doi.org/10.1038/s41576-019-0127 .
Tolin D, McKay D, Forman E, Klonsky E, Thombs B. Empirically supported treatment: recommendations for a new model. Clin Psychol Sci Pract. 2015;22(4):317–38. https://doi.org/10.1111/cpsp.12122 .
Trauer J, Qian M, Doyle J, Rajaratnam S, Cunnington D. Cognitive behavioral therapy for chronic insomnia: a systematic review and meta-analysis. Ann Intern Med. 2015;163(3):191–204. https://doi.org/10.7326/M14-2841 .
van Dalfsen J, Markus C. The involvement of sleep in the relationship between the serotonin transporter gene-linked polymorphic region (5-HTTLPR) and depression: a systematic review. J Affect Disord. 2019;256:205–12. https://doi.org/10.1016/j.jad.2019.05.047 .
van Straten A, van der Zweerde T, Kleiboer A, Cuijpers P, Morin C, Lancee J. Cognitive and behavioral therapies in the treatment of insomnia: a meta-analysis. Sleep Med Rev. 2018;38:3–16. https://doi.org/10.1016/j.smrv.2017.02.001 .
Walsh J, Roth T. Pharmacologic treatment of insomnia: benzodiazepine receptor agonists. In: Kryger M, Roth T, Dement W, editors. Principles and practices of sleep medicine. 6th ed. Philadelphia: Elsevier; 2017. p. 832–841.
Wang Y, Wang F, Zheng W, Zhang L, Ng C, Unqvari G, Xiang Y. Mindfulness-based interventions for insomnia: A meta-analysis of randomized controlled trials. Behav Sleep Med. 2018. https://doi.org/10.1080/15402002.2018.1518228 [Epub ahead of print] .
Whitfield-Gabrieli S, Ford J. Default mode network activity and connectivity in psychopathology. Annu Rev Clin Psychol. 2012;8:49–76. https://doi.org/10.1146/annurev-clinpsy-032511-143049 .
Wilson B. Cutting edge developments in neuropsychological rehabilitation and possible future directions. Brain Impair. 2011;12(1):33–42. https://doi.org/10.1375/brim.12.1.33 .
Wilson B. Neuropsychological rehabilitation: state of the science. S Afr J Psychol. 2013;43(3):267–77. https://doi.org/10.1177/0081246313494156 .
Winkler A, Rief W. Effect of placebo conditions on polysomnographic parameters in primary insomnia: a meta-analysis. Sleep. 2015;38(6):925–31. https://doi.org/10.5665/sleep.4742 .
Wittchen H, Jacobi F, Rehm J, Gustavsson A, Svensson M, Jönsson B, Steinhausen H. The size and burden of mental disorders and other disorders of the brain in Europe. Eur Neuropsychopharmacol. 2011;21(9):655–79. https://doi.org/10.1016/j.euroneuro.2011.07.018 .
Download references
Acknowledgements
The authors would like to express their gratitude to the reviewers for their important comments and suggestions.
Author information
Authors and affiliations.
Department of Education and Psychology, University of Aveiro, Campus Universitário de Santiago, 3810-193, Aveiro, Portugal
Daniel Ruivo Marques
Faculty of Psychology and Educational Sciences, CINEICC-Center for Research in Neuropsychology and Cognitive Behavioral Intervention, University of Coimbra, Coimbra, Portugal
Daniel Ruivo Marques, Ana Allen Gomes & Vanda Clemente
Faculty of Psychology and Educational Sciences, University of Coimbra, Rua Do Colégio Novo, 3000-115, Coimbra, Portugal
Ana Allen Gomes
Sleep Medicine Centre, Coimbra University Hospital Centre (CHUC), Coimbra, Portugal
Vanda Clemente, José Moutinho dos Santos & Joana Serra
Faculty of Medicine, University of Coimbra, Rua Larga, 3004-504, Coimbra, Portugal
Maria Helena Pinto de Azevedo
You can also search for this author in PubMed Google Scholar
Corresponding author
Correspondence to Daniel Ruivo Marques .
Ethics declarations
Conflict of interest.
The authors declare that they have no conflict of interest.
Additional information
Publisher's note.
Springer Nature remains neutral with regard to jurisdictional claims in published maps and institutional affiliations.
Rights and permissions
Reprints and permissions
About this article
Marques, D.R., Gomes, A.A., Clemente, V. et al. Trends in insomnia research for the next decade: a narrative review. Sleep Biol. Rhythms 18 , 199–207 (2020). https://doi.org/10.1007/s41105-020-00269-7
Download citation
Received : 20 August 2019
Accepted : 28 March 2020
Published : 06 April 2020
Issue Date : July 2020
DOI : https://doi.org/10.1007/s41105-020-00269-7
Share this article
Anyone you share the following link with will be able to read this content:
Sorry, a shareable link is not currently available for this article.
Provided by the Springer Nature SharedIt content-sharing initiative
- Sleep disorder
- Find a journal
- Publish with us
- Track your research
ORIGINAL RESEARCH article
The different faces of insomnia.
- 1 Department of Internal Medicine and Dermatology, Interdisciplinary Center of Sleep Medicine, Charité - Universitätsmedizin Berlin, Berlin, Germany
- 2 Department of Behavioral Therapy and Psychosomatic Medicine, Rehabilitation Center Seehof, Federal German Pension Agency, Seehof, Germany
- 3 Department of Biology, Saratov State University, Saratov, Russia
Objectives: The identification of clinically relevant subtypes of insomnia is important. Including a comprehensive literature review, this study also introduces new phenotypical relevant parameters by describing a specific insomnia cohort.
Methods: Patients visiting the sleep center and indicating self-reported signs of insomnia were examined by a sleep specialist who confirmed an insomnia diagnosis. A 14-item insomnia questionnaire on symptoms, progression, sleep history and treatment, was part of the clinical routine.
Results: A cohort of 456 insomnia patients was described (56% women, mean age 52 ± 16 years). They had suffered from symptoms for about 12 ± 11 years before seeing a sleep specialist. About 40–50% mentioned a trigger (most frequently psychological triggers), a history of being bad sleepers to begin with, a family history of sleep problems, and a negative progression of insomnia. Over one third were not able to fall asleep during the day. SMI (sleep maintenance insomnia) symptoms were most frequent, but only prevalence of EMA (early morning awakening) symptoms significantly increased from 40 to 45% over time. Alternative non-medical treatments were effective in fewer than 10% of cases.
Conclusion: Our specific cohort displayed a long history of suffering and the sleep specialist is usually not the first point of contact. We aimed to describe specific characteristics of insomnia with a simple questionnaire, containing questions (e.g., ability to fall asleep during the day, effects of non-medical therapy methods, symptom stability) not yet commonly asked and of unknown clinical relevance as yet. We suggest adding them to anamnesis to help differentiate the severity of insomnia and initiate further research, leading to a better understanding of the severity of insomnia and individualized therapy. This study is part of a specific Research Topic introduced by Frontiers on the heterogeneity of insomnia and its comorbidity and will hopefully inspire more research in this area.
Introduction
Insomnia is one of the most frequent sleep disorders with continuously increasing prevalence. About 30–50% of the US adult population exhibit insomnia symptoms, 15–20% display a short-term insomnia of <3 months, and 5–15% display a chronic insomnia of >3 months ( 1 – 3 ). Common diagnostic manuals include the ICSD-3 (International Classification of Sleep Disorders, 3 rd Edition, American Academy of Sleep Medicine 2014) and the DSM-5 (Diagnostic and Statistical Manual of Mental Disorders, 5 th Edition, American Psychiatric Association 2013) ( 4 , 5 ). Main characteristics of insomnia include dissatisfaction with sleep quantity and quality with one or more of the following symptoms: difficulties initiating sleep, difficulties maintaining sleep (frequent or prolonged awakenings with problems returning to sleep again), and early morning awakening (occurring earlier than desired after a total sleep time of only 3–5 h with the inability to return to sleep). The disturbed sleep is associated with stress, psychological strain and suffering, as well as impairment in social, occupational, and other important areas of functioning. Complaints include fatigue, exhaustion, lack of energy, daytime sleepiness, cognitive impairment (e.g., attention, concentration, and memory), mood swings (e.g., irritability, dysphoria), impaired occupational functioning and impaired social functioning. The symptoms occur for at least 3 nights per week for at least 3 months and occur despite an adequate sleep environment.
Previous dichotomization of insomnia in primary and secondary (or comorbid) insomnia has been abandoned with the new editions of the DSM-5 and ICSD-3. Currently, insomnia is mostly characterized by the common phenotypes of sleep onset insomnia (SOI insomnia, difficulty falling asleep), sleep maintenance insomnia (SMI insomnia, difficulty staying asleep), early morning awakenings insomnia (EMA insomnia), and a combination of those. Another categorization follows the timeframe of being an acute (<1 month), subacute (1–3 months), and chronic insomnia (>3 months) ( 4 , 5 ). While other sleep disorders (e.g., sleep apnea) are categorized by severity into mild, moderate, or severe, which has important implications for the choice of therapy, insomnia still lacks such a classification. The Insomnia Severity Index (ISI) is the only instrument currently in use that allows for severity classification: no insomnia (score 0–7), subclinical insomnia (score 8–14), or moderate to severe insomnia (score 15–28) ( 6 ).
The characterization of different phenotypes is important to establish clinically relevant subtypes of insomnia. It may help to reduce the heterogeneity of insomnia and facilitate cause identification and personalized treatments. Yet there are not many standardized instruments of insomnia diagnosis allowing for phenotyping. However, there has been evidence that insomniacs with a total sleep time of <6 h suffer a more severe insomnia than insomniacs with a total sleep time of 6 h or more ( 7 ). They display mental and psychological impairment compared to patients with average or longer than average sleep. However, mortality is increased for insomniacs with longer total sleep time ( 8 ). The sleep duration with the 6-h distinction also influences the therapy outcome, success of cognitive-behavioral therapy (CBT), and the relation to comorbid bipolar disorder ( 9 , 10 ). Recently, a study investigated subtypes of insomnia according to psychological stress ( 11 ). Questioning 2,224 volunteers with an ISI score of at least 10 and a control group of 2,098 volunteers with an ISI score below 10, five insomnia subtypes were identified: highly distressed, moderately distressed but sensitive to positive reinforcement (accepting of positive emotions), moderately distressed insensitive to positive reinforcement, slightly distressed with a high reactivity to their environment and life circumstances, and slightly distressed with low reactivity. The results showed a high stability of the classification over the 5-year investigation. The psychological categorization is clinically relevant as there were clear differences identified between the subtypes regarding development, therapy success, presence of electroencephalogram (EEG) biomarker, and the risk for depression. This was a first approach to subtyping insomnia patients according to psychological health. The exact effect of psychological health, family history, comorbidity, personality, environment and sleep quality on insomnia is still unclear. Similar symptom clusters have been discussed for other disorders including depression ( 12 ).
Our study is part of the specific Research Topic introduced by Frontiers on the heterogeneity of insomnia and its comorbidity. We aim to encourage and further the discussion on insomnia heterogeneity and the need for possible phenotyping, we do not intend to provide a complete list of phenotypes or possible clusters. The study picked up the approach of subtyping insomnia by collecting a short questionnaire during anamnesis on possible related symptoms, onset and course of insomnia. We described phenotypical traits of insomniacs with a cohort of sleep disturbed patients from a specialized outpatient clinic for sleep disorders.
Participants and Recruitment
Since 2018, a specialized 14-item insomnia questionnaire has formed part of the clinical routine at the outpatient clinic of the Interdisciplinary Center of Sleep Medicine, Charité—Universitätsmedizin Berlin ( Figure 1 ). The questionnaire is the result of literature research, clinical experience, and consensus of psychologists, neurologist, psychiatrists, and sleep physicians within the sleep center. Patients who visited the outpatient clinic between 01/2019 and 02/2020 and indicated self-reported symptoms presenting a suspicion of insomnia (e.g., difficulties initiating sleep, maintaining sleep, or early morning awakening) according to ICSD-3 criteria were recruited and completed the questionnaire. In total, 486 patients were examined by a physician specializing in sleep disorders and insomnia who confirmed an insomnia diagnosis. The questionnaire did not contain any identifying information. As the questionnaire is part of the clinical routine and the de-identified data has been analyzed retrospectively, ethical review and approval was not required in accordance with the local legislation and institutional requirements. As part of the clinical routine, patients signed informed consent forms allowing de-identified data of their patient file, including the insomnia questionnaire, to be used for research purposes.
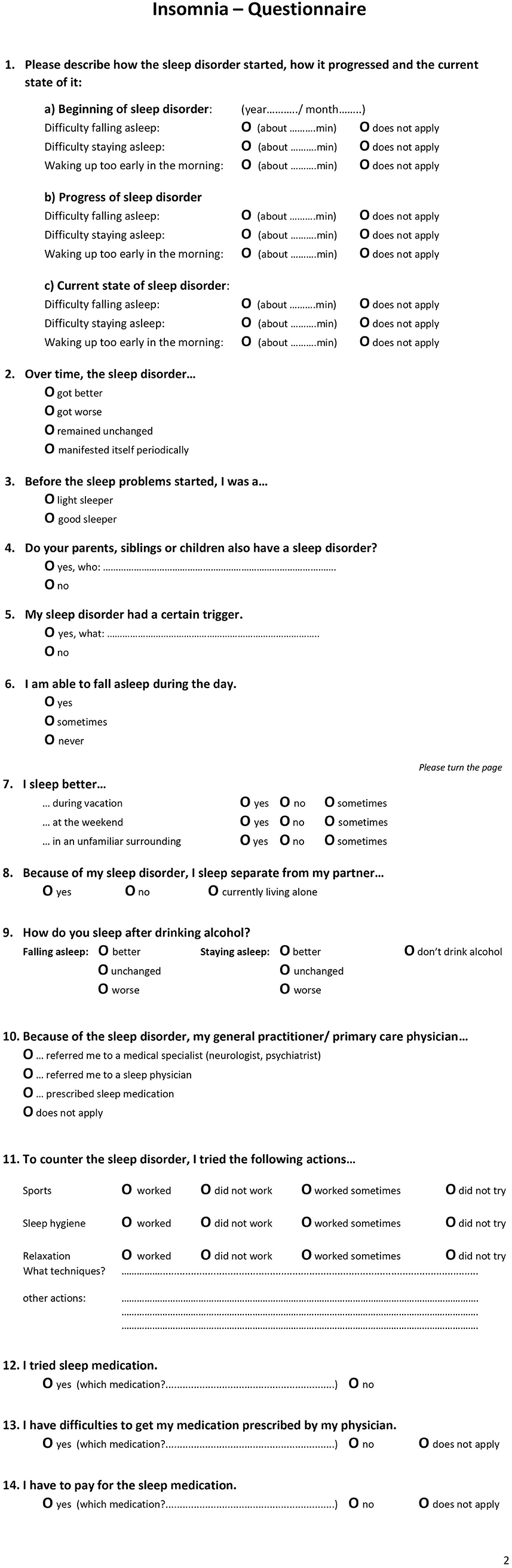
Figure 1 . The English translation of the 14-item Insomnia Questionnaire with page 1 and page 2.
Questionnaire
The insomnia questionnaire consisted of 14 items ( Figure 1 presents an English translation of the questionnaire). These included questions related to (1) type of insomnia (SOI—sleep onset insomnia, SMI—sleep maintenance insomnia, EMA—early awakening insomnia, multiple answers possible) at three points in time (start of disorder, progression, current state), (2) progression of insomnia, (3) sleep history of being a light or good sleeper, (4) relatives with sleep disorder, (5) triggers, (6) daytime sleep, (7) sleeping in different environments, (8) sleeping arrangement with partner, (9) alcohol as a sleep aid, (10) referral/ recommendation of general practitioner (multiple answer options), (11) alternative sleep treatments, and (12–14) sleep medication.
Procedure of the examination was standardized and performed by the same physician: On arrival, patients received several sleep questionnaires including the 14-item insomnia questionnaire. They were asked to complete these questionnaires before seeing the physician. During the following in-person consultation, the physician completed a full anamnesis (a patient-reported medical history) and confirmed a diagnosis of a primary insomnia according to ICSD-3 criteria. Next, the questions of the insomnia questionnaire were evaluated. Certain questions were clarified, and missing information added. For example, for question 3, light sleeper was defined. Light sleeper includes patients with a regular bedtime but whose sleep is sensitive to light, temperature, and noise. They need a specific degree of sleep comfort and sleep worse in an unfamiliar environment. These patients can nap during the day and sleep better during vacation and time off (e.g., weekends). They perceive their sleep as non-restorative. They also do not meet the diagnostic criteria of insomnia. The question refers to the time before the insomnia started, mostly referring to childhood / adolescence. For question 6, it was clarified that daytime napping included a daytime situation that explicitly allows for napping. For question 7, it was explained that “weekend” also included the days off work.
Sample size was calculated based on prevalence data and the estimated number of insomnia patients: ca. 30–50% of 328.2 million people (US population estimate 2019) result in about 98.5–164.1 million patients ( 13 ). With an accepted error rate of maximum 5% and a confidence interval of 95%, the sample size was set to at least 400 questionnaires in order to detect sufficiently powerful effects. Statistical analysis was performed using SPSS (IBM SPSS Statistics, Version 20). The patient cohort was described using a descriptive analysis with numbers and percentages ( Table 1 ). In order to investigate possible insomnia subgroups based on phenotypes/characteristics, we compared items with dichotomous answers. Item 7 (sleeping in different environments), item 9 (alcohol as a sleep aid), and item 11 (alternative sleep treatments) each had several subcategories which were consolidated into one overall category. For the text answer of item 5 (trigger) we performed a qualitative data analysis by subjectively grouping the text data and visually presenting the categories. A t-test was used for group comparisons of continuous variables (e.g., age), the chi-square test for dichotomous variables. Significance level was set at 0.05.
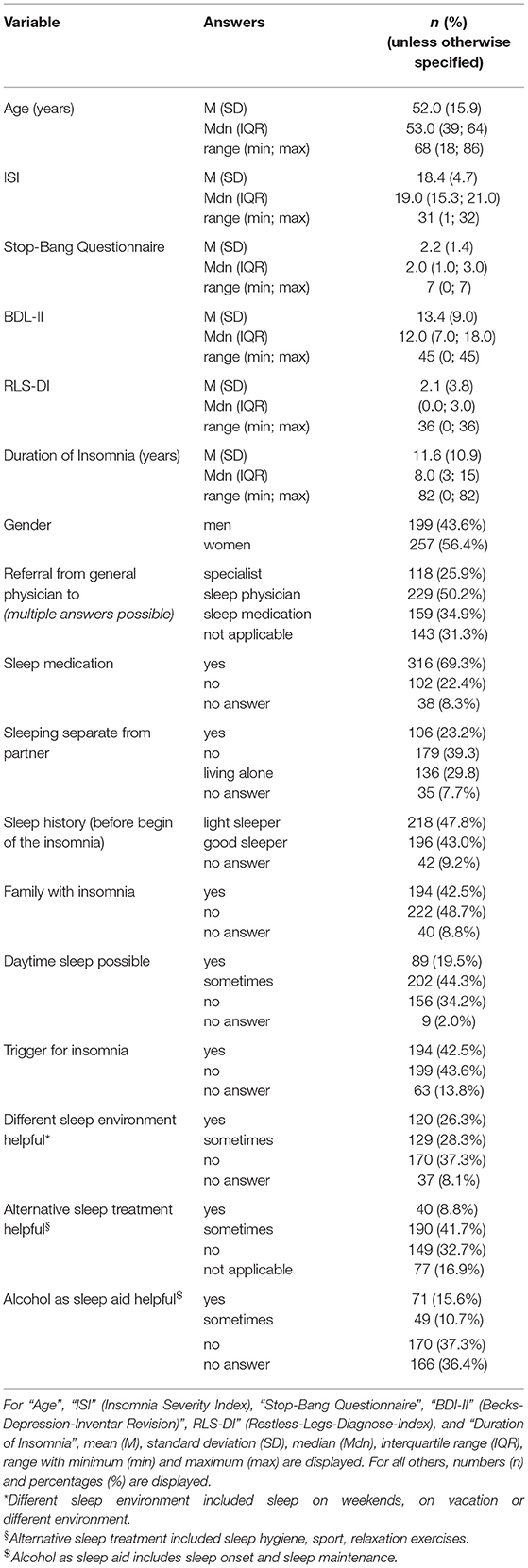
Table 1 . Sample description ( n = 456 patients).
Patient Description
Due to missing information that could also not be completed during the in-person consultation with the physician, 30 questionnaires were removed from analysis. The remaining 456 questionnaires were de-identified and analyzed. The patient cohort ( Table 1 ) reported having sleep problems for an average of 11.6 ± 10.9 years (range: 0–82 years, where 0 means the symptoms just started within the past month). The cohort consisted of slightly more female insomniacs (56%) and had an average age of 52.0 ± 15.9 years (range: 18–86 years). More than half of the patients reported having a partner and not living alone (63%), and of those 37% slept in a separate room due to the sleep disorder. If the patient went to a general physician first, 50% were referred to a sleep specialist and 26% to another specialist (neurologist, psychiatrist etc.). In 35% of those cases, the general physician initiated a therapy with sleep medication. In general, 69% of the patients reported having used sleep medication, 23% indicated that they had not. Only 9% mentioned that it was difficult to get sleep medication. While 26% stated they had to pay for sleep medication, 37% said they did not. In Germany, sleep medication for primary insomnia covered by insurance only includes melatonin (only for patients over 55 years) and z-drugs (only for the acute therapy of 4 weeks).
Sleep Characteristics
About 43% of the patients indicated that they had a history of being good sleepers before the insomnia onset, while 48% mentioned that they have always been light sleepers. Forty-three percent reported having a family member with sleep problems. Despite insomnia symptoms, 20% of patients indicated that they were able to fall asleep during the day and 44% sometimes. While 43% of patients reported a trigger for the sleep problems, 42% reported no trigger ( Table 1 ). Figure 2 presents a categorization of the reported triggers. The most frequent triggers were of psychological nature (22%) including depression, anxiety, post-traumatic stress disorder, death of a family member, trauma, rape, psychotherapy etc. Stress was listed as a separate category but is to be considered as a subcategory of psychological triggers (additional 11%). Work related triggers including change or loss of job, freelance work, work problems, shift work, long work hours, workload, mobbing/ bulling etc. accounted for 15%.
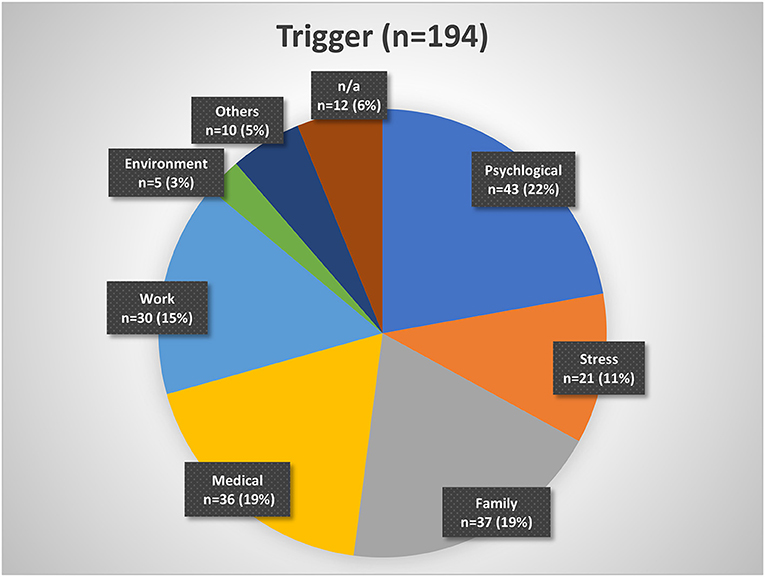
Figure 2 . Insomnia triggers organized by categories. Psychological triggers include depression, fear, trauma, etc. Stress may be considered a subgroup of psychological triggers. Family triggers include birth, children, marriage, divorce, etc. Medical triggers include sickness, operations, etc. Work triggers include mobbing, loss of job, change of job, workload, etc. Environment triggers include noise, lighting, neighborhood, etc. Other triggers include smoking, attitude, etc. n/a, not available.
The question about sleep in a different environment (item 7 of the questionnaire) included three subcategories: sleep during vacation, sleep at weekends, and sleep in unfamiliar surroundings. Sleep during vacation was perceived as better by 21% ( n = 84), sometimes better by 30% ( n = 121), and not at all better by 49% ( n = 198). Sleep at the weekend was perceived as better by 18% ( n = 70), sometimes better by 26% ( n = 103), and not at all better by 56% ( n = 224). Sleep in unfamiliar surroundings was perceived as better by 5% ( n = 19), sometimes better by 17% ( n = 68), and not at all better by 78% ( n = 304). We consolidated the subcategories in one general environment variable. First, sleep in a different environment (in general) was considered better if a patient answered “yes (sleep better)” to at least one of the subgroups. The remaining patients were categorized into the sometimes group if they answered “sometimes” to at least one of the subcategories. Then, the remaining patients were categorized into the “no (do not sleep better)” or “no answer” category. In general, 26% indicated that they sleep better in different environments, 28% sometimes, and 37% not at all ( Table 1 ).
The question for alternative non-medical treatments (item 11) also included three subcategories: sport, sleep hygiene, and relaxation techniques. Sport only helped in 7% ( n = 26), helped sometimes in 32% ( n = 130), and did not help in 46% ( n = 185). Sleep hygiene helped in 5% ( n = 18), helped sometimes in 29% ( n = 103), and did not help in 43% ( n = 154). Relaxation techniques helped in 5% ( n = 19), helped sometimes in 32% ( n = 117), and did not help in 38% ( n = 142). We combined the subcategories into one overall variable of non-medical treatment in the same way as for item 7. In general, 9% of the patients indicated that an alternative treatment helps, 42% mentioned it helped sometimes, and 33% reported it did not help at all ( Table 1 ).
Alcohol as a sleep aid (item 9) included two subcategories: alcohol as a sleep aid for sleep onset and alcohol as a sleep aid for sleep maintenance. While 40% ( n = 112) indicated alcohol helps with SOI symptoms, it did not change sleep onset in 41% ( n = 116) and symptoms got worse in 19% ( n = 54). Alcohol helped with SMI symptoms in 11% ( n = 31), did nothing in 46% ( n = 123), and got worse in 43% ( n = 116). We also consolidated this variable. Alcohol as a sleep aid in general helped, if a patient answered “sleep got better” to at least one of the two subcategories (without a “sleep got worse” for the other category). Alcohol worsened sleep if a patient answered at least once “got worse” (without a “got better” for the other category). We added the answer option “alcohol helps sometimes” for patients that answered “got better” to one of the categories and “got worse” to the other. The remaining patients were categorized as “no change” or “no answer.” In general, alcohol helped in 16%, helped sometimes in 11%, and did not help (or even got worse) in 37% ( Table 1 ).
Table 2 presents a further description of insomnia subtypes based on these sleep characteristics. We dichotomized the answers into yes/no in order to create a more equal group distribution for comparison. Patients with a sleep history of being light sleepers even before insomnia onset, had significantly longer insomnia symptoms than patients with a sleep history of being good sleepers ( p < 0.05). Patients with a family history of sleep problems were significantly more frequently female ( p < 0.05), had suffered from insomnia symptoms significantly longer ( p < 0.01), and presented significantly more EMA symptoms ( p < 0.05) than patients without a family history of sleep problems. Patients who were able to sleep during the day were significantly more frequently male ( p = 0.001) and displayed fewer SOI ( p < 0.001) and fewer EMA symptoms ( p < 0.01) than patients who could not sleep during the day. Patients with no trigger displayed a tendency to having a longer insomnia duration than patients with a trigger ( p = 0.05). Patients who were able to sleep better in different environments were significantly younger ( p < 0.001) and showed a tendency to shorter insomnia duration ( p = 0.05) than patients who did not sleep better in another environment. Patients for whom alcohol helped as a sleep aid were significantly younger ( p < 0.001) and presented significantly more SOI symptoms ( p < 0.001).
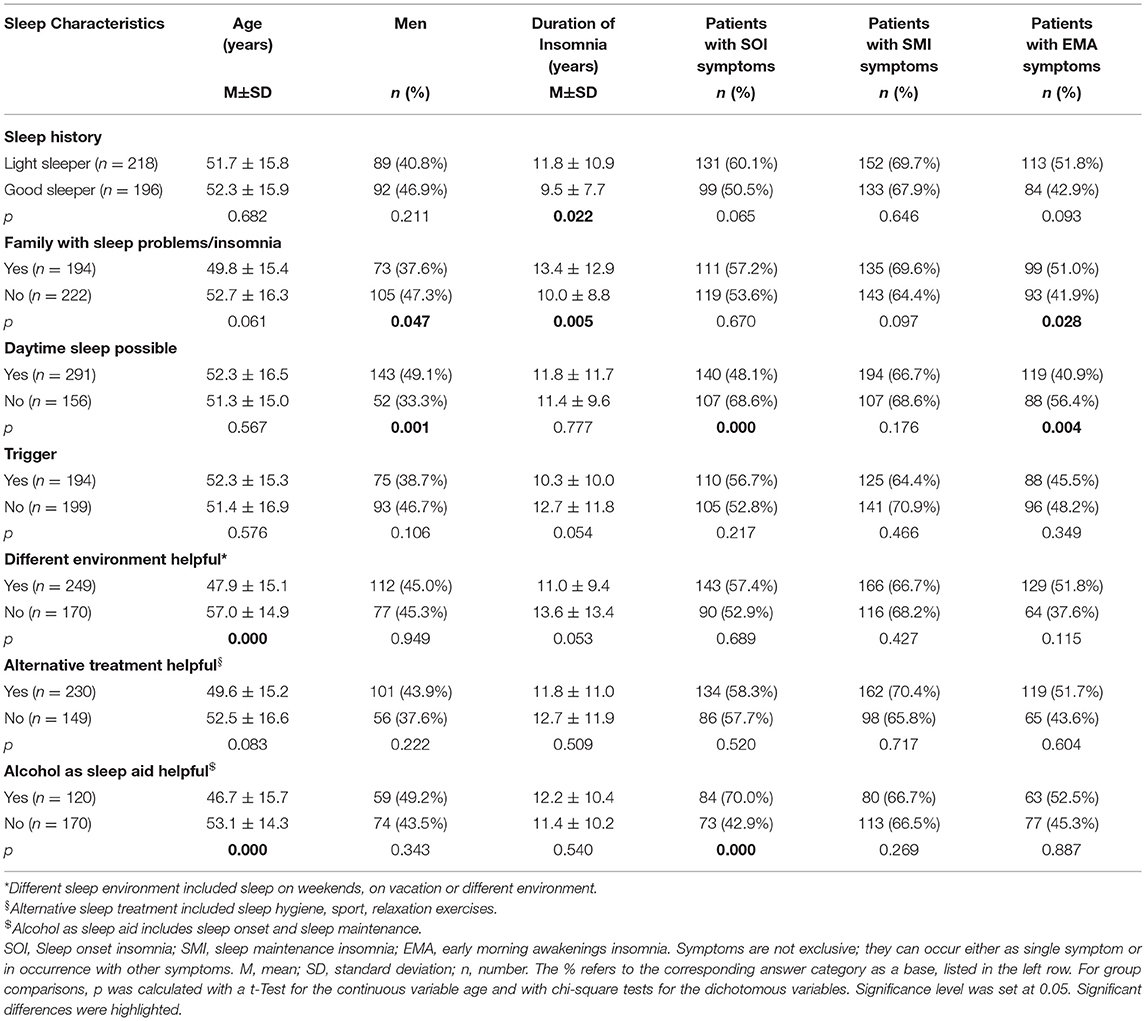
Table 2 . Description of possible insomnia phenotype subgroups based on sleep characteristics.
Insomnia Symptom Subtypes and Progression
At time of visit, 54% of patients presented SOI symptoms, 66% SMI symptoms, and 45% EMA symptoms ( Table 3 ). In 57% of the patients, there was a combination of those symptoms. Patients with SOI symptoms reported on average that they needed 85.6 ± 55.0 min to fall asleep. Patients with SMI symptoms reported waking up for about 79.0 ± 58.2 min after sleep onset. And patients with EMA symptoms reported that they woke up on average 79.0 ± 56.5 min too early in the morning. Patients with EMA symptoms (not exclusively, combination of symptoms possible) had the shortest history of sleep problems (10.2 ± 9.1 years, range: 0–44 years) compared to patients with SOI symptoms (12.0 ± 9.8 years, range: 0–82 years) and patients with SMI symptoms (11.5 ± 10.6 years, range: 0–82 years). Differences were not significant.
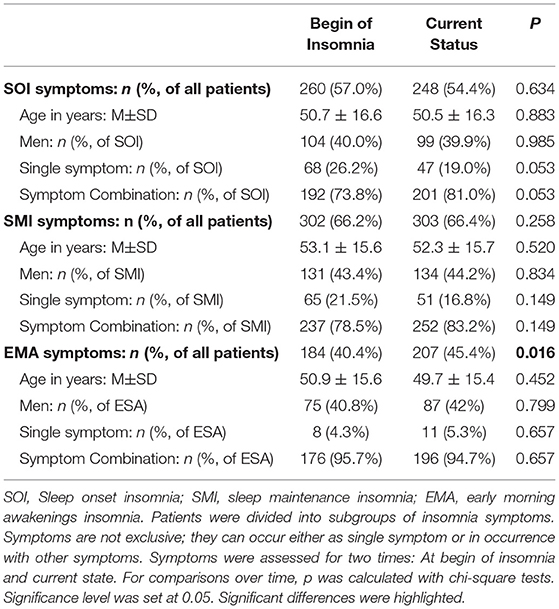
Table 3 . Patient description by insomnia subgroups based on symptoms over time.
Table 3 presents the possible change of sleep symptoms over time by type of sleep symptoms. There was no significant change in SOI or SMI symptoms. Only EMA symptoms significantly increased over time ( p = 0.016). Figure 3 presents the progression in severity of the sleep disorder. Fewer than 10% reported an improvement of symptoms, while in 41% the sleep disorder got worse. In 20% the symptoms showed a periodic pattern. The progression was independent of current symptoms.
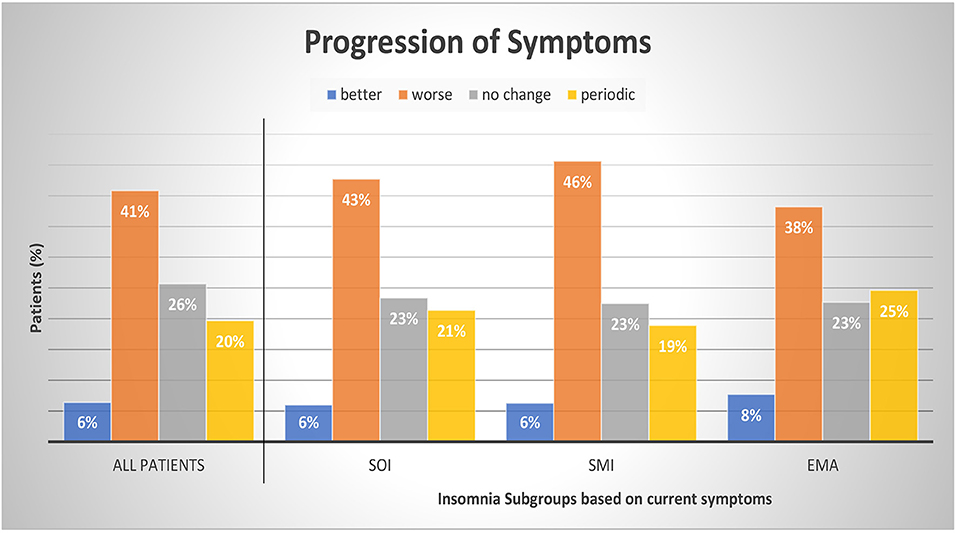
Figure 3 . Progression of symptoms by insomnia subgroups. Patients were divided into subgroups of current insomnia symptom. Symptoms are not exclusive, they can occur either as single symptom or in occurrence with other symptoms. SOI, Sleep onset insomnia; SMI, sleep maintenance insomnia; EMA, early morning awakenings insomnia. A patient with a periodic pattern of insomnia experiences weeks or months long periods with insomnia symptoms alternating with symptom free periods. For comparisons between symptom groups, p was calculated with chi-square tests. Results were not significant at a 0.05 level. The sum of the subcategories does not add up to 100% as we refrained from displaying the category “missing data and multiple answers” (7% All patients, 7% SOI, 6% SMI, and 7% EMA).
A distinct cohort of insomnia patients that reported to a special outpatient clinic for sleep disorders revealed that about 40–50% of the patients mentioned a trigger for the sleep problems, were not good sleepers to begin with (light sleepers), had a family history of sleep problems, and had a progressive course of insomnia. Over one third were not able to fall asleep during the day. Insomnia with SMI symptoms was most frequent, as well as a psychological trigger. Over time, EMA symptoms increased. Alternative non-medical treatments were only lastingly effective in fewer than 10%. Over two thirds of the patients (69%) had tried sleep medication. One of the unique traits of our cohort is the duration of the sleep problem before the visit to a specialist (over 11 years). For most, the sleep specialist/clinic is not the first point of contact. Thus, our patient cohort is not comparable to one from a general physician or population-based cohort.
Our results emphasize the insomnia heterogeneity and the need for phenotyping. Following, we will first discuss the characteristics assessed with our questionnaire starting with some new aspects that are currently not commonly asked (history of being a light sleeper, daytime sleep, effects of alternative treatments, alcohol, temporal stability/change of insomnia symptoms). Then, we will review the current literature for further possible phenotypes. Table 4 presents an overview.
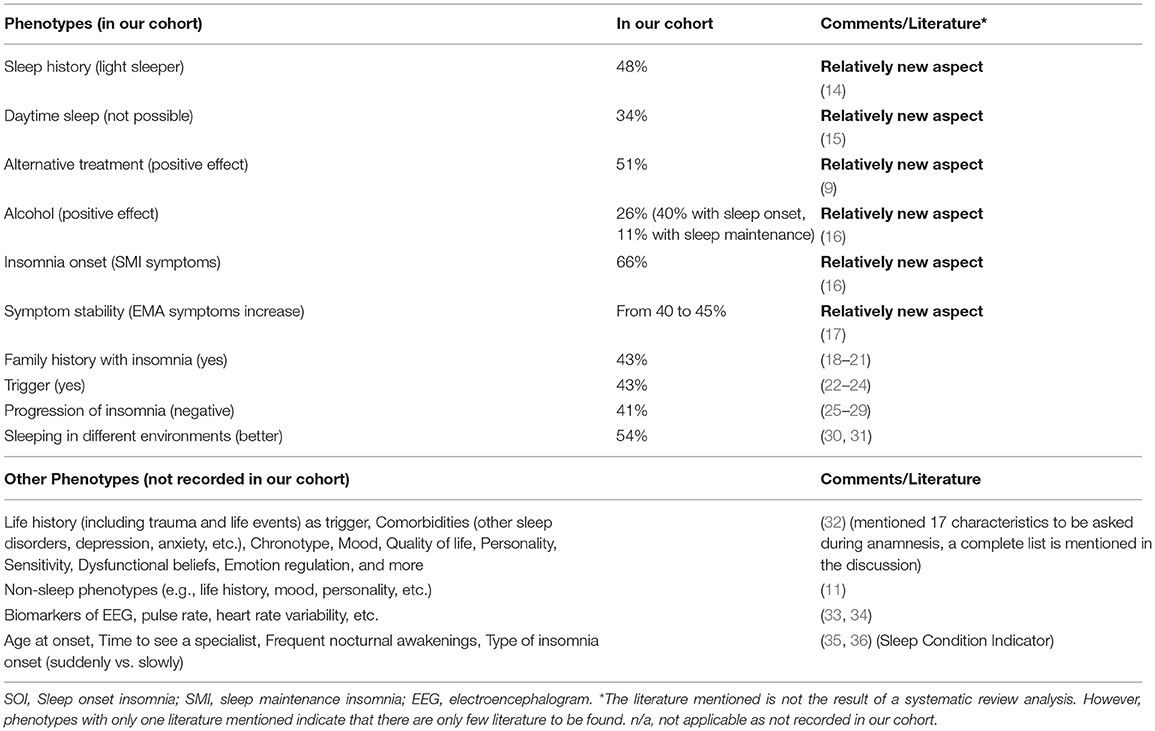
Table 4 . Overview of discussed phenotypes.
Phenotypes—Based on Our Cohort
Sleep history.
Almost half of our cohort (48%) presented a bad sleep history, indicative of an idiopathic insomnia.
There are no clear biomarkers or diagnostic criteria to distinguish between psychophysiological and idiopathic (chronic) insomnia ( 14 ). In order to identify idiopathic insomnia, we ask the patient for their sleep history, specifically before insomnia onset. Did the patient always experience poor (light) sleep, or were they a fairly good sleeper? We assume that light sleep is the pre-stage of insomnia, but not every light sleeper needs to develop insomnia, indicating that these variables are not predictors for differentiating between psychophysiological and idiopathic insomnia. Whether this distinction of good and bad sleep before developing insomnia influences therapy will need to be further investigated. Also, the term “light (bad)” sleep needs to be clearly defined and standardized.
Daytime Sleep
Using our questionnaire, we found in our cohort that 34% of patients reported not being able to take a nap during the daytime despite being tired and despite having the explicit opportunity of taking a nap. Those patients were predominantly women with more SOI and more EMA symptoms compared to patients who were able to fall asleep during the day. They did not differ regarding the duration of their insomnia symptoms.
Currently, it is not common during insomnia diagnosis to ask whether a patient is able to fall asleep during the day or to conduct a Multiple Sleep Latency Test (MSLT) for objective assessment. Our own experience with insomnia patients, however, showed how important this question is. We experienced that patients who sleep poorly at night and are tired during the day, but cannot sleep in the day either, usually have a higher degree of insomnia. They tend to suffer for more nights a week and are more resistant to therapy. In contrast, the possibility of falling asleep during the day, in front of the television, in the car, on public transport, in a meeting, or in other quiet surroundings, seems to be a sign of a lower degree of insomnia.
The ability to nap during the day has also been a criterion for other indications in the literature. The Hyperarousal Scale by Regestein et al. ( 37 ) provides indirectly a reference to the degree of alertness during the day and thus to the inability to fall asleep. Khassawneh et al. ( 38 ) used the scale together with the patient's subjective statement that they cannot nap during the day and found that patients with hyperarousal and short sleep duration have more cognitive deficits in memory tests. Li et al. ( 39 ) used the MSLT with a threshold value of 14 min to define hyperarousal. Drake et al. ( 40 ) also used the MSLT and investigated sleep disturbances due to commonly experienced stressful situations to identify factors representing the construct of “stress-related” vulnerability to sleep disturbance. Subjects with a high Ford Insomnia Response to Stress Test (FIRST) score had poorer sleep quality at night and higher latencies of sleep in the MSLT. Roehrs et al. ( 15 ) performed the MSLT in 95 patients with primary insomnia (32–64 years) and in 55 healthy sleepers and found a higher sleep latency in insomniacs (13.2 ± 4.65 min vs. 11.0 ± 4.93 min). However, the difference is small and the variability among insomniacs is high (between 2 and 20 min). The MSLT is still a questionable method for diagnosing insomnia, but it may be a possible tool for subtyping insomnia with regard to the ability to fall asleep during daytime. Espie et al. ( 41 ) examined daytime symptoms of 11,129 participants with ( n = 5,083) and without insomnia, coming from different backgrounds. Of the analyzed items (energy, concentration, relationships, ability to stay awake, mood, and ability to get through work), the items “energy” and “mood” turned out to be the two most important parameters for insomniacs, but not the item “ability to stay awake.” The importance of the criterion daytime sleepiness and/or ability to stay awake seems therefore recognized, but not yet uniformly defined and requires further research.
Alternative Treatment (Behavioral Therapy)
In our cohort, about 83% of the patients have tried at least one of these alternative non-medical behavioral treatments: sport, sleep hygiene, and/or relaxation techniques. In one third of the patients (33%) these techniques did not help. There were no significant age, gender, or symptom differences between patients with effective alternative treatments and patients where it was not effective. However, we did not investigate the severity of insomnia and it may be possible that patients where the alternative treatments did not show a positive effect may be patients with more severe insomnia.
Therapy recommendations for insomnia include a multi-modal behavioral therapy including psychological elements (e.g., CBT) as the first therapeutic step which many patients do complete, most commonly even before they arrange a visit to a specialist ( 42 ). This is also what we found in our cohort. Most of our patients have tried to educate themselves on their sleep problems, have tried to improve their sleep hygiene, have tried alternative non-medical treatments (e.g., sport, relaxation, etc.), and already went to either a natural health practitioner, homeopath, psychologist or psychotherapist. Currently, CBT is not yet good enough established in Germany as a definite treatment for insomnia. Studies have shown that CBT had less of an effect on insomniacs with short sleep duration ( 9 ). We assume that this also applies to patients with a more severe insomnia. However, severity has yet to been clearly defined. Patients will most likely show a similar reaction to phytopharmacology or alternative “smart” therapy (e.g., acoustic or electrical stimulation). A future quality check and standardization of CBT methods may be helpful in order to use the success of alternative treatment/behavioral therapy as a phenotypical criterion. We hypothesize that successful CBT is mainly linked to mild insomnia. For moderate to severe insomnia, CBT should be a necessary concomitant therapy.
In our cohort, only about 26% mentioned that alcohol helps with sleep problems in general. Patients for whom alcohol helped were significantly younger and presented more SOI symptoms. A more detailed analysis showed that alcohol helped especially with sleep onset (40%), less with sleep maintenance (only 11%). In 43% of our patients, alcohol even worsened sleep maintenance, which other studies confirmed ( 16 ). However, in almost half of our patients, alcohol showed no change.
Alcohol is a widely used sleep aid. Asking for the soporific effect of alcohol should become standard during insomnia anamnesis, as well as asking for the soporific effect of drugs (CBD, cannabis, etc.) which have become more and more a topic of sleep research ( 43 ). It is surprising that in our cohort many patients reported a lack of positive effect of alcohol as a sleep aid. It may be that the alcohol amount consumed was not high enough, as we did not ask for specifics.
Symptoms at Time of Insomnia Onset
In our cohort, 57% had SOI symptoms when the insomnia started (in 74% as a combination with other symptoms), 66% had SMI symptoms at the beginning (in 79% as a combination of symptoms), and 40% started with EMA symptoms (in 96% with other symptoms). The majority had a combination of several symptoms. Hence, in most cases of insomnia the sleep disorder started with SMI symptoms (either as single symptom or in combination). We found that patients with single SOI or single EMA were significantly younger than patients with a SOI combination (single: age 47 ± 17 years, combination: age 52 ± 16 years; p < 0.01) or EMA combination (single: age 39 ± 13 years, combination: age 51 ± 15 years; p < 0.01), respectively.
Bjorøy et al. ( 16 ) also investigated subtypes of insomnia in an extensive web-based survey with 64,503 patients who had displayed insomnia for >6 months. Here, 60% of the younger insomniacs (on average 37 years) showed SOI symptoms, either as a combination with SMI and/or EMA symptoms or as a single symptom. Confirming our own results, Bjorøy et al. ( 44 ) also found that SOI as a single symptom was more frequent in younger insomniacs, a SOI symptom combination more frequent in older insomniacs. They revealed further predictors for a symptom combination including female gender, evening chronotype, less education, and being single. While we do not assess aspects such as chronotype, they are important. Literature has shown that there is a higher insomnia prevalence in general in people with an evening chronotype. Insomniacs with a symptom combination also showed a higher comorbidity with depression, anxiety, and a higher use of alcohol and sleeping pills ( 16 ).
Symptom Stability Over Time
Not just the severity, but also the symptoms can change over time. In our cohort, prevalence of SOI and SMI symptoms did not change; EMA symptoms, however, significantly increased from 40 to 45% from first noticing those symptoms to the present (visit to a sleep specialist). Patients with SOI symptoms showed a tendency of an increase of SOI in symptom combination instead of as a single symptom (from 74 to 81%).
An early study of Hohagen et al. ( 17 ) also investigated the progression of insomnia symptoms and possible temporal stability of different patterns in 328 patients (18–65 years). In only 4 months, they discovered a >50% change in SOI, SMI, and EMA symptoms. Only in rare cases did a specific and single symptom insomnia (either SOI, SMI, or EMA) change from one to another single symptom. However, in many single symptom insomnia cases another symptom occurred over time while the first symptom stayed dominant. This tendency was also seen in our cohort regarding the SOI symptoms.
Family History
Almost half of our patient cohort (43%) reported a family history of disturbed sleep/insomnia. These patients were foremost female and presented more EMA symptoms than patients without a family history present.
A specific gene for insomnia is not known but a genetic predisposition cannot be completely ruled out ( 18 , 19 ). A twin study of children revealed a moderate inheritability of insomnia, and another study reported 35% inheritability ( 20 , 21 ).
In our cohort, almost every second patient (43%) reported a trigger. Patients with or without a trigger in our cohort did not differ regarding age, gender, and insomnia symptoms. However, those patients with no triggers showed a tendency to longer insomnia duration then the ones with a trigger. Here, it may be possible that the start of the trigger (whether sudden or slowly, unconsciously developing) may have an impact on the perception of insomnia as a chronic condition. Within our cohort, most frequently named were psychological triggers (e.g., depression, anxiety, trauma, burnout), family triggers (e.g., birth, divorce, custody battles), and medical/biological triggers including surgery and other illnesses. Work triggers (e.g., mobbing/ bulling, job loss) and stress as a separate psychological trigger came next.
Triggers are part of Spielman's theoretical model (1987) of factors causing chronic insomnia. The 3Ps consist of predisposing factors, precipitating factors which trigger acute insomnia, and perpetuating factors ( 22 , 23 ). Triggers would belong to the precipitating factors and may lead to a chronic insomnia. For a working patient, work related stress and job strain may play a bigger role as a trigger and moderator of the insomnia than for those patients that are not working ( 24 ). However, whether the existence of a trigger influences the progression or therapy of insomnia still needs to be further investigated.
Progression of Insomnia
Our patients reported most frequently a negative progression of insomnia (41%); in 26% there were no changes, and only in 7% was there an improvement. On average, the patients suffered from insomnia symptoms for about 11.6 years (range 0–82 years) before seeing a sleep specialist. Patients with predominantly EMA symptoms showed the shortest sleep problem history with 10.2 years (range 0–44 years) compared to patients with SOI or SMI symptoms. About 20% of our patients reported a periodic pattern of symptom severity.
The periodic pattern may be indicative of a non-24 h disorder ( 25 ). A patient with a periodic pattern of insomnia experiences weeks or months long periods with insomnia symptoms alternating with symptom free periods. Green et al. ( 26 ) also investigated the progression of insomnia for over 20 years in 5-year intervals. Patterns included: healthy pattern, episodic pattern, chronic pattern, and a pattern with the development of symptoms in the follow-up period. Chronic insomnia was linked to older women and the working class. It showed that social factors do affect the progression of a sleep disorder, a fact also indicated by Patel et al. ( 27 ) and Arber et al. ( 28 ). There is another distinction of insomnia subtypes by progression introduced by Wu et al. ( 29 ): persistent insomnia, remission, or relapse.
Sleep in Different Environments
Over half of our patients (54%) reported sleeping better in a different environment, including weekends/days with time off from work (51%), vacation (44%), and unfamiliar surroundings in general (22%). The category “unfamiliar surroundings” received the lowest number. Patients may have included job related hotel stays and therefore increased stress level, which may account for the lower number. Patients stating they slept better in a different environment were predominantly younger members of our cohort.
If patients reported sleeping better at weekends or on vacation, this may be an indication that the sleep disorder was caused by work stress or daily routine. In the literature, this is called behavioral induced insufficient sleep ( 30 , 31 ). As only few insomniacs are able to quit their job or family, this category may represent a specific insomnia phenotype. For those, specific interventions are possible including the end of shift work, change to home office work, change from full-time to part-time work, etc.
Further Discussion of Phenotypes
Studies suggest that insomnia is a heterogenic disorder and the identification of different phenotypes or comorbidities is important for personalized treatments ( 45 ). In our study, we presented some new aspects on what insomniacs should be asked during anamnesis and what should be considered during phenotyping. Benjamin et al. ( 32 ) already proposed the following characteristics: (1) life history including demographics, mental and physical health, trauma and life events. This study showed that more women than men and more older people than younger people suffer from insomnia and life events are usually triggers. Such triggers are mostly to be found at home, in health or at work/school, as could also be confirmed with our patients. But who reacts to such a negative trigger with insomnia and why, when, at what age, is not yet known and may possibly have a genetic reason. Further characteristics included (2) subjective sleep quality, (3) fatigue, sleepiness, hyperarousal in the daytime, (4) other sleep disorders, (5) lifetime sleep history, (6) chronotype, (7) depression, anxiety, mood, (8) quality of life, (9) personality, (10) worry, rumination, self-consciousness, sensitivity, (11) dysfunctional beliefs, (12) self-conscious emotion regulation and coping, (13) nocturnal mentation, (14) wake resting state mentation, (15) lifestyle including physical activity and food intake, (16) body temperature, and (17) hedonic evaluation. Other possible non-sleep phenotypes included: MRI, cognition, mood, traits, history of life events, family history, PSG, sleep microstructure, genetics. Blanken et al. ( 11 ) distinguished insomnia subtypes according to the so-called non-sleep categories of life history, mood perception, and personality. Miller et al. ( 33 ) presented an insomnia cluster analysis based on neurocognitive performance, sleep-onset measures of qualitative EEG, and heart rate variability (HRV). They identified two main clusters, depending on duration of sleep (<6 h vs. >6 h). The HRV changes during falling asleep may also play a role, as may the spectral power of the sleep EEG, and parameters from the sleep hypnogram such as sleep onset latency and wake after sleep onset. In one of our own studies, we were able to demonstrate that the increased nocturnal pulse rate and vascular stiffness in insomniacs with low sleep efficiency (<80%) represented an early sign of elevated cardiovascular risk, and thus presented a useful tool for phenotyping insomnia ( 34 ). In the future, other objective characteristics may include biomarkers or radiological features ( 46 , 47 ).
Further characteristics that may play a role but have not yet been mentioned or investigated are the age of the patient during insomnia onset, frequent nocturnal awakenings, the time it takes to see a specialist, and the kind of insomnia onset, slowly progressing or suddenly unexpected. There is no defined age at which the likelihood of insomnia increases, but we know that menopause is a major trigger for women. Grandner et al. ( 35 ) were able to show that getting older alone is not a predictor of insomnia, it rather includes multifactorial events. The question of how long it takes to see a specialist is also part of the Sleep Condition Indicator (SCI) by Espie et al. ( 36 ). They asked whether the insomnia had lasted longer than a year, 1–2, 3–6, or 7–12 months. We can easily agree with such a classification in terms of content. Many patients who wake up frequently at night consider this an insomnia with SMI symptoms. Frequent nocturnal awakenings, but with the ability to fall asleep again straight away, are according to the definition not considered a SMI insomnia. We did not address this in the present study, which presents a limitation. While it is mentioned in the DSM-5 as an independent sign of insomnia, patients affected by frequent nocturnal but subjectively normal sleep lengths and still restful sleep do not (yet) have insomnia. Whether it is an independent phenotype or a preliminary stage of a SMI insomnia should be further examined and defined. It also needs to be clarified whether devices for sleep registration help us with phenotyping. Polysomnography is certainly a very strong phenotypic feature when sleep time is very short, wake times after sleep onset is high and deep and/or dream sleep and sleep efficiency are not optimal. However, the current status is such that it is not suitable for diagnosis ( 48 ). In the near future, technical advances will help to provide objective, long-term sleep data, which are important for diagnosis, subtyping, and therapy for different types of insomnia.
Currently, questionnaires have been used to assess insomnia. The most known questionnaires include the ISI and the Pittsburgh Sleep Quality Index (PSQI). These are valid instruments ( 6 , 49 ). However, there are a number of other questionnaires used for insomnia such as the Amsterdam Resting-State Questionnaire (ARSQ), Dysfunctional Beliefs and Attitudes About Sleep Scale (DBAS), Sleep-Related Behaviors Questionnaire (SRBQ), Sleep Functional Impact Scale (SFIS), Leeds Sleep Evaluation Questionnaire (LSEQ), Glasgow Sleep Effort Scale (GSES) ( 50 – 55 ). In 2014, Espie et al. ( 36 ) introduced the SCI which presented a good instrument for identifying the presence of insomnia and also allowed for time differentiation. Also, the short version with only 2 questions seems valid, where questions are asked about the number of nights in the past month with poor sleep and about the trouble in general caused by sleep ( 56 ). Kalmbach et al. ( 57 ) presented a differentiation between good and bad sleepers based on the Presleep Arousal Scale—Cognitive (PSAS-C) and—Somatic (PSAS-S). People with a high PSAS-C have higher sleep latency and wake times after sleep onset, as well as higher MSLT latency and lower sleep efficiency and total sleep time. The PSAS-C in particular seems to be a good measure of the hyperarousal state. Research and official expert recommendations will show which questionnaires should be favored in clinical practice.
Limitations
Our study intended to encourage and further the discussion on insomnia heterogeneity and the need for possible phenotyping. While we introduced some new aspects of phenotyping, we neither provided a complete list of possible phenotypes nor defined specific clusters. Limitations of our study include the fact that further important aspects (e.g., comorbidity, employment, having children, chronotype, employment etc.) may need consideration. Also, some aspects of the questionnaire will need a more precise definition (e.g., light sleeper, daytime napping, weekend/vacation, alternative treatment, alcohol use), patients were not differentiated regarding sleep duration (<6 h vs. >6 h), and the progression of insomnia was observed retrospectively and not investigated prospectively. While our study was performed with patients of a sleep center, there is also need for phenotyping and thorough assessment of those phenotype characteristics in patients of a primary care setting.
As part of a specific Research Topic introduced by Frontiers on the heterogeneity of insomnia, our study provides further ideas on the already existing approaches to phenotyping insomnia patients. The aim of our study was not to examine all conceivable phenotypic features of insomnia, but to help document specific characteristics with simple questions about the onset and course of insomnia during anamnesis. While the clinical relevance of some of those possible phenotypes is not yet clear (e.g., sleep history, trigger, daytime sleep, sleep in a different environment, alternative treatment, insomnia progression/symptom stability etc.), they should play a role in future research and medical care of insomnia patients. We would like to give an impulse for further research in this area, in order to better differentiate insomnia, thus leading to more effective individualized therapy.
Data Availability Statement
The raw data supporting the conclusions of this article will be made available by the authors, without undue reservation.
Ethics Statement
Ethical review and approval was not required for the study on human participants in accordance with the local legislation and institutional requirements. The patients/participants provided their written informed consent to participate in this study.
Author Contributions
IF, TP, and VK had the role of supervision and conceptualized the study. IF was responsible for data collection. NL performed data analysis. All authors were involved in visualization and writing including data interpretation, result discussion, and drafting and reviewing the manuscript.
This was not an industry supported study. The study was initiated and funded by the Charité—Universitätsmedizin Berlin owned funding.
Conflict of Interest
The authors declare that the research was conducted in the absence of any commercial or financial relationships that could be construed as a potential conflict of interest.
Acknowledgments
We would like to thank all the patients that participated, and Hendrik Straße and Sandra Zimmermann involved in data entry and processing.
1. Ohayon MM. Epidemiology of insomnia: what we know and what we still need to learn. Sleep Med Rev. (2002) 6:97–111. doi: 10.1053/smrv.2002.0186
PubMed Abstract | CrossRef Full Text | Google Scholar
2. Morin CM, LeBlanc M, Daley M, Gregoire JP, Merette C. Epidemiology of insomnia: prevalence, self-help treatments, consultations, and determinants of help-seeking behaviors. Sleep Med. (2006) 7:123–30. doi: 10.1016/j.sleep.2005.08.008
3. Krystal AD, Prather AA, Ashbrook LH. The assessment and management of insomnia: an update. World Psychiatry. (2019) 18:337–52. doi: 10.1002/wps.20674
CrossRef Full Text | Google Scholar
4. American Academy of Sleep Medicine. International Classification of Sleep Disorders . 3rd ed. Darien, IL: American Academy of Sleep Medicine (2014).
Google Scholar
5. American Psychiatric Association. Diagnostic and Statistical Manual of Mental Disorders – Section II: Diagnostic Criteria and Codes: Sleep-Wake Disorders . 5th ed. Arlingten, VA: American Psychiatric Association (2013).
6. Bastien CH, Vallières A, Morin CM. Validation of the nsomnia Severity Index as an outcome measure for insomnia research. Sleep Med. (2001) 2:297–307. doi: 10.1016/S1389-9457(00)00065-4
7. Vgontzas AN, Fernandez-Mendoza J, Liao D, Bixler EO. Insomnia with objective short sleep duration: the most biologically severe phenotype of the disorder. Sleep Med Rev. (2013) 17:241–54. doi: 10.1016/j.smrv.2012.09.005
8. Wallace ML, Lee S, Hall MH, Stone KL, Langsetmo L, Redline S, et al. Heightened sleep propensity: a novel and high-risk sleep health phenotype in older adults. Sleep Health. (2019) 5:630–8. doi: 10.1016/j.sleh.2019.08.001
9. Bathgate CJ, Edinger JD, Krystal AD. Insomnia patients with objective short sleep duration have a blunted response to cognitive behavioral therapy for insomnia. Sleep. (2017) 40: zsw012. doi: 10.1093/sleepj/zsx050.334
10. Lewis KJS, Richards A, Karlsson R, Leonenko G, Jones SE, Jones HJ, et al. Comparison of genetic liability for sleep traits among individuals with bipolar disorder I or II and control participants. JAMA Psychiatry. (2019) 77:303–10. doi: 10.1001/jamapsychiatry.2019.4079
11. Blanken TF, Benjamins JS, Borsboom D, Vermunt JK, Paquola C, Ramautar J, et al. Insomnia disorder subtypes derived from life history and traits of affect and personality. Lancet Psychiatry. (2019) 6:151–63. doi: 10.1016/S2215-0366(18)30464-4
12. Bondar J, Caye A, Chekroud AM, Kieling C. Symptom clusters in adolescent depression and differential response to treatment: a secondary analysis of the treatment for adolescents with depression study randomised trial. Lancet Psychiatry. (2020) 7:337–43. doi: 10.1016/S2215-0366(20)30060-2
13. United States Census Bureau. Source 2019 Population Estimates . (2020). Available online at: https://data.census.gov/cedsci/all?q=Population%20estimates/ (accessed November 2, 2020).
14. Passarella S, Duong MT. Diagnosis and treatment of insomnia. Am J Health Syst Pharm. (2008) 65:927–34. doi: 10.2146/ajhp060640
15. Roehrs TA, Randall S, Harris E, Maan R, Roth T. MSLT in primary insomnia: stability and relation to nocturnal sleep. Sleep. (2011) 34:1657–52. doi: 10.5665/sleep.1426
16. Bjorøy I, Jørgensen VA, Pallesen S, Bjorvatn B. The prevalence of insomnia subtypes in relation to demographic characteristics, anxiety, depression, alcohol consumption and use of hypnotics. Front Psychol. (2020) 11:527. doi: 10.3389/fpsyg.2020.00527
17. Hohagen F, Käppler C, Schramm E, Riemann D, Weyerer S, Berger M. Sleep onset insomnia, sleep maintaining insomnia and insomnia with early morning awakening–temporal stability of subtypes in a longitudinal study on general practice attenders. Sleep. (1994) 17:551–4.
PubMed Abstract | Google Scholar
18. Jansen PR, Watanabe K, Stringer S, Skene N, Bryois J, Hammerschlag AR, et al. Genome-wide analysis of insomnia in 1,331,010 individuals identifies new risk loci and functional pathways. Nat Genet. (2019) 51:394–403. doi: 10.1038/s41588-018-0333-3
19. Stein MB, McCarthy MJ, Chen CY. Genome-wide analysis of insomnia disorder. Mol Psychiatry. (2018) 23:2238–50. doi: 10.1038/s41380-018-0033-5
20. Barclay NL, Gehrman PR, Gregory AM, Eaves LJ, Silberg JL. The heritability of insomnia progression during childhood/adolescence: results from a longitudinal twin study. Sleep. (2015) 38:109–18. doi: 10.5665/sleep.4334
21. Beaulieu-Bonneau S, LeBlanc M, Mérette C, Dauvilliers Y, Morin CM. Family history of insomnia in a population-based sample. Sleep. (2007) 30:1739– 45. doi: 10.1093/sleep/30.12.1739
22. Spielman AJ, Caruso LS, Glovinsky PB. A behavioral-perspective on insomnia treatment. Psychiatr Clin North Am. (1987) 10:541–53. doi: 10.1016/S0193-953X(18)30532-X
23. Perlis ML, Corbitt CB, Kloss JD. Insomnia research: 3Ps and beyond. Sleep Med Rev. (2014) 18:191–93. doi: 10.1016/j.smrv.2014.01.003
24. Halonen JI, Lallukka T, Pentti J, Stenholm S, Rod NH, Virtanen M, et al. Change in job strain as a predictor of change in insomnia symptoms: analyzing observational data as a non-randomized pseudo-trial. Sleep. (2017) 40:zsw007. doi: 10.1093/sleep/zsw007
25. Solaiman SS, Agrawal R. Non-24-hour sleep-wake circadian rhythm disorder in a sighted male with normal functioning. J Clin Sleep Med. (2018) 14:483–4. doi: 10.5664/jcsm.7008
26. Green MJ, Espie CA, Hunt K, Benzeval M. The longitudinal course of insomnia symptoms: inequalities by sex and occupational class among two different age cohorts followed for 20 years in the west of Scotland. Sleep. (2012) 35:815–23. doi: 10.5665/sleep.1882
27. Patel NP, Grandner MA, Xie D, Branas CC, Gooneratne N. “Sleep disparity” in the population: poor sleep quality is strongly associated with poverty and ethnicity. BMC Public Health. (2010) 10:475. doi: 10.1186/1471-2458-10-475
28. Arber S, Bote M, Meadows R. Gender and socio-economic patterning of self-reported sleep problems in Britain. Soc Sci Med. (2009) 68:281–9. doi: 10.1016/j.socscimed.2008.10.016
29. Wu MP, Lin HJ, Weng SF, Ho CH, Wang JJ, Hsu YW. Insomnia subtypes and the subsequent risks of stroke: report from a nationally representative cohort. Stroke. (2014) 45:1349–54. doi: 10.1161/STROKEAHA.113.003675
30. Hublin C, Sallinen M. Behaviorally induced insufficient sleep. Sleep Med Clin. (2012) 7:313–23. doi: 10.1016/j.jsmc.2012.03.008
31. Williams AB, Dzierzewski JM, Griffin SC, Lind MJ, Dick D, Rybarczyk BD. Insomnia disorder and behaviorally induced insufficient sleep syndrome: prevalence and relationship to depression in college students. Behav Sleep Med. (2020) 18:275–86. doi: 10.1080/15402002.2019.1578772
32. Benjamins JS, Migliorati F, Dekker K, Wassing R, Moens S, Blanken TF, et al. Insomnia heterogeneity: characteristics to consider for data-driven multivariate subtyping. Sleep Med Rev. (2017) 36:71–81. doi: 10.1016/j.smrv.2016.10.005
33. Miller CB, Bartlett DJ, Mullins AE, Dodds KL, Gordon CJ, Kyle SD, et al. Clusters of insomnia disorder: an exploratory cluster analysis of objective sleep parameters reveals differences in neurocognitive functioning, quantitative EEG, and heart rate variability. Sleep. (2016) 39:1993–2004. doi: 10.5665/sleep.6230
34. Laharnar N, Grote L, Zou D, Hedner J, Sommermeyer D, Straßenburger C, et al. Overnight pulse wave analysis to assess autonomic changes during sleep in insomnia patients and healthy sleepers. PLoS ONE. (2020) 15:e0232589. doi: 10.1371/journal.pone.0232589
35. Grandner MA. Sleep duration across the lifespan: implications for health. Sleep Med Rev. (2012) 16:199–201. doi: 10.1016/j.smrv.2012.02.001
36. Espie CA, Kyle SD, Hames P, Gardani M, Fleming L, Cape J. The sleep condition indicator: a clinical screening tool to evaluate insomnia disorder. BMJ Open. (2014) 4:e004183. doi: 10.1136/bmjopen-2013-004183
37. Regestein QR, Bambrosia J, Hallett M, Murawski B, Paine M. Daytime alertness in patients with insomnia. Am J Psychiatry. (1993) 150:1529–34. doi: 10.1176/ajp.150.10.1529
38. Khassawneh BY, Bathgate CJ, Tsai SC, Edinger JD. Neurocognitive performance in insomnia disorder: the impact of hyperarousal and short sleep duration. J Sleep Res. (2018) 27:e12747. doi: 10.1111/jsr.12747
39. Li Y, Vgontzas AN, Fernandez-Mendoza J, Bixler EO, Sun Y, Zhou J, et al. Insomnia with physiological hyperarousal is associated with hypertension. Hypertension. (2015) 65:644–50. doi: 10.1161/HYPERTENSIONAHA.114.04604
40. Drake C, Richardson G, Roehrs T, Scofield H, Roth T. Vulnerability to stress-related sleep disturbance and hyperarousal. Sleep. (2004) 27:285–91. doi: 10.1093/sleep/27.2.285
41. Espie CA, Kyle SD, Hames P, Cyhlarova E, Benzeval M. The daytime impact of DSM-5 insomnia disorder: comparative analysis of insomnia subtypes from the Great British Sleep Survey. J Clin Psychiatry. (2012) 73:e1478–84. doi: 10.4088/JCP.12m07954
42. Baglioni C, Altena E, Bjorvatn B, Blom K, Bothelius K, Devoto A, et al. The European academy for cognitive behavioural therapy for insomnia: an initiative of the European insomnia network to promote implementation and dissemination of treatment. J Sleep Res. (2020) 29:e12967. doi: 10.1111/jsr.12967
43. Suraev A, Grunstein RR, Marshall NS, D'Rozario AL, Gordon CJ, Bartlett DJ, et al. Cannabidiol (CBD) and Δ(9)-tetrahydrocannabinol (THC) for chronic insomnia disorder ('CANSLEEP' trial): protocol for a randomised, placebo-controlled, double-blinded, proof-of-concept trial. BMJ Open. (2020) 10:e034421. doi: 10.1136/bmjopen-2019-034421
44. Merikanto I, Kronholm E, Peltonen M, Laatikainen T, Lahti T, Partonen T. Relation of chronotype to sleep complaints in the general Finnish population. Chronobiol Int . (2012) 29:311–7. doi: 10.3109/07420528.2012.655870
45. Bjorvatn B, Jernelöv S, Pallesen S. Insomnia – A heterogenic disorder often comorbid with psychological and somatic disorders and diseases: a narrative review with focus on diagnostic and treatment challenges. Front Psychol. (2021) 12:639198. doi: 10.3389/fpsyg.2021.639198
46. Mikoteit T, Brand S, Eckert A, Holsboer-Trachsler E, Beck J. Brain-derived neurotrophic factor is a biomarker for subjective insomnia but not objectively assessable poor sleep continuity. J Psychiatr Res. (2019) 110:103–9. doi: 10.1016/j.jpsychires.2018.12.020
47. Neumann N, Lotze M, Domin M. Sex-specific association of poor sleep quality with gray matter volume. Sleep. (2020) : zsaa035. doi: 10.1093/sleep/zsaa035
48. Collen J, York CM. Wrist wearables: more questions than answers? J Clin Sleep Med. (2019) 15:1077–78. doi: 10.5664/jcsm.7858
49. Buysse DJ, Reynolds CF, Monk TH, Berman SR, Kupfer DJ. The pittsburgh sleep quality index – a new instrument for psychiatric practice and research. Psychiat Res. (1989) 28:193–213. doi: 10.1016/0165-1781(89)90047-4
50. Espie CA, Inglis SJ, Harvey L, Tessier S. Insomniacs' attributions: psychometric properties of the dysfunctional beliefs and attitudes about sleep scale and the sleep disturbance questionnaire. J Psychosom Res. (2009) 48:141–8. doi: 10.1016/s0022-3999(99)00090-2
51. Palagini L, Cellini N, Mauri M, Mazzei I, Simprage S, dell'Osso L, et al. Multiple phenotypes of resting-state cognition are altered in insomnia disorder. Sleep Health. (2016) 2:239–45. doi: 10.1016/j.sleh.2016.05.003
52. Ree MJ, Harvey AG. Investigating safety behaviours in insomnia: the development of the sleep-related behaviours questionnaire (SRBQ). Behav Change. (2004) 21:26–36. doi: 10.1375/bech.21.1.26.35971
53. Bell C, McLeod LD, Nelson LM, Fehnel SE, Zografos LJ, Bowers B. Development and psychometric evaluation of a new patient-reported outcome instrument measuring the functional impact of insomnia. Qual Life Res. (2011) 20:1457–68. doi: 10.1007/s11136-011-9885-8
54. Tarrasch R1, Laudon M, Zisapel N. Cross-cultural validation of the Leeds Sleep Evaluation Questionnaire (LSEQ) in insomnia patients. Hum Psychopharmacol. (2003) 18:603–10. doi: 10.1002/hup.534
55. Broomfield NM, Espie CA. Towards a valid, reliable measure of sleep effort. J Sleep Res. (2005) 14:401–7. doi: 10.1111/j.1365-2869.2005.00481.x
56. Luik AI, Machado PF, Siriwardena N, Espie CA. Screening for insomnia in primary care: using a two-item version of the Sleep Condition Indicator. Br J Gen Pract. (2019) 69:79–80. doi: 10.3399/bjgp19X701045
57. Kalmbach DA, Cheng P, O'Brien LM, Swanson LM, Sangha R, Sen S, et al. A randomized controlled trial of digital cognitive behavioral therapy for insomnia in pregnant women. Sleep Med. (2020) 72:82–92. doi: 10.1016/j.sleep.2020.03.016
Keywords: insomnia, phenotypes, subtypes, heterogeneity, symptom, progression, questionnaire, screening
Citation: Fietze I, Laharnar N, Koellner V and Penzel T (2021) The Different Faces of Insomnia. Front. Psychiatry 12:683943. doi: 10.3389/fpsyt.2021.683943
Received: 22 March 2021; Accepted: 24 May 2021; Published: 29 June 2021.
Reviewed by:
Copyright © 2021 Fietze, Laharnar, Koellner and Penzel. This is an open-access article distributed under the terms of the Creative Commons Attribution License (CC BY) . The use, distribution or reproduction in other forums is permitted, provided the original author(s) and the copyright owner(s) are credited and that the original publication in this journal is cited, in accordance with accepted academic practice. No use, distribution or reproduction is permitted which does not comply with these terms.
*Correspondence: Naima Laharnar, naima.laharnar@charite.de
Disclaimer: All claims expressed in this article are solely those of the authors and do not necessarily represent those of their affiliated organizations, or those of the publisher, the editors and the reviewers. Any product that may be evaluated in this article or claim that may be made by its manufacturer is not guaranteed or endorsed by the publisher.
- Research article
- Open access
- Published: 01 May 2020
The effect of yoga on sleep quality and insomnia in women with sleep problems: a systematic review and meta-analysis
- Wei-Li Wang 1 ,
- Kuang-Huei Chen 1 ,
- Ying-Chieh Pan 1 ,
- Szu-Nian Yang 2 &
- Yuan-Yu Chan 1 , 3
BMC Psychiatry volume 20 , Article number: 195 ( 2020 ) Cite this article
27k Accesses
76 Citations
198 Altmetric
Metrics details
To examine the effectiveness and safety of yoga of women with sleep problems by performing a systematic review and meta-analysis.
Medline/PubMed, ClinicalKey, ScienceDirect, Embase, PsycINFO, and the Cochrane Library were searched throughout the month of June, 2019. Randomized controlled trials comparing yoga groups with control groups in women with sleep problems were included. Two reviewers independently evaluated risk of bias by using the risk of bias tool suggested by the Cochrane Collaboration for programming and conducting systematic reviews and meta-analyses. The main outcome measure was sleep quality or the severity of insomnia, which was measured using subjective instruments, such as the Pittsburgh Sleep Quality Index (PSQI), Insomnia Severity Index (ISI), or objective instruments such as polysomnography, actigraphy, and safety of the intervention. For each outcome, a standardized mean difference (SMD) and confidence intervals (CIs) of 95% were determined.
Nineteen studies in this systematic review included 1832 participants. The meta-analysis of the combined data conducted according to Comprehensive Meta-Analysis showed a significant improvement in sleep (SMD = − 0.327, 95% CI = − 0.506 to − 0.148, P < 0.001). Meta-analyses revealed positive effects of yoga using PSQI scores in 16 randomized control trials (RCTs), compared with the control group in improving sleep quality among women using PSQI (SMD = − 0.54; 95% CI = − 0.89 to − 0.19; P = 0.003). However, three RCTs revealed no effects of yoga compared to the control group in reducing insomnia among women using ISI (SMD = − 0.13; 95% CI = − 0.74 to 0.48; P = 0.69). Seven RCTs revealed no evidence for effects of yoga compared with the control group in improving sleep quality for women with breast cancer using PSQI (SMD = − 0.15; 95% CI = − 0.31 to 0.01; P = 0.5). Four RCTs revealed no evidence for the effects of yoga compared with the control group in improving the sleep quality for peri/postmenopausal women using PSQI (SMD = − 0.31; 95% CI = − 0.95 to 0.33; P = 0.34). Yoga was not associated with any serious adverse events.
This systematic review and meta-analysis demonstrated that yoga intervention in women can be beneficial when compared to non-active control conditions in term of managing sleep problems. The moderator analyses suggest that participants in the non-breast cancer subgroup and participants in the non-peri/postmenopausal subgroup were associated with greater benefits, with a direct correlation of total class time with quality of sleep among other related benefits.
Peer Review reports
Sleep problems are one of the most common medical complaints. Lack of sleep is associated with significantly decreased work performance, impaired daytime function, and increased health care costs [ 1 ]. Sex-based differences in sleep problems have been widely published and discussed across sleep articles. Insomnia is approximately 1.41 times more common in women than in men [ 2 ]. Female populations at certain stages in their life span may be more vulnerable to insomnia. In these stages, hormonal changes associated with hormones, such as follicle-stimulating hormones (FSHs), luteinizing hormones (LHs), and progesterone, may play an important role in influencing women’s sleep construction [ 3 ] during adolescence [ 4 ], pregnancy and postpartum [ 5 ] or menopause [ 6 ]. Several behavioral, psychological and pharmacological treatments are available for insomnia, however, their efficacy varies considerably. The evidence of efficacy for cognitive behavior therapy is now well established in many reviews [ 7 , 8 ], but availability remains poor. Pharmacotherapy remains the most common treatment [ 9 ], although hypnotics have been associated with many side effects, such as drowsiness, cognitive impairment, dependence, tolerance and poor long term efficacy [ 10 ].
Yoga has been widely adapted in the modern Eastern and Western hemispheres in a variety of ways. Yoga is an ancient form of exercise that focuses on strength, flexibility, and breathing to boost physical, mental and spiritual health [ 11 ]. There are many different styles of yoga, such as Tibetan, Iyengar, and Hatha Yoga. Some styles are more vigorous than others, whereas some may have different areas of emphasis, such as posture or breathing. The main components of yoga in Europe or America are mostly associated with physical posture ( Asana ) and breathing control ( Pranayama ) and meditation ( Dhyana ) [ 11 ]. A trial in yoga for persistent fatigue breast cancer survivors showed yoga is safe and effective at improving fatigue severity, depressive moods, and sleep quality [ 12 ]. Yoga is also characterized as a mindful mode of physical activity. Mindfulness, as an important component of yoga, improves sleep disturbance by increasing melatonin levels, reducing hyperarousal, and addressing stress related cardiac and respiratory abnormalities [ 13 ].
The term “sleep quality” is commonly used in sleep medicine. In 1989, Buysse et al. referred to sleep quality as a “complex phenomenon that is difficult to define and measure objectively” [ 14 ]. Good sleep quality is associated with better health, less daytime sleepiness, greater well-being and better psychological functioning [ 15 ]. Recently, sleep quality is defined as one’s satisfaction of the sleep experience, integrating aspects of sleep initiation, sleep maintenance, sleep quantity, and refreshment upon awakening [ 16 ]. The National Sleep Foundation (NSF) released the key indicators of good sleep quality, as established by a panel of experts. They include increase in sleeping time while in bed (at least 85% of the total time), falling asleep in 30 min or less, waking up no more than once per night and being awake for 20 min or less after initially falling asleep. However, there was less or no consensus regarding sleep architecture or nap-related variables as elements of good sleep quality [ 17 ]. Poor sleep quality is one of the defining features of chronic insomnia [ 18 ]. Although recent systematic reviews and meta-analyses have assessed the efficacy and safety of yoga in specific groups of women, such as those with prenatal depression [ 19 ] and primary dysmenorrhea [ 20 ] in different stages, evidence for the efficacy of yoga in improving sleep quality and insomnia of women has not yet been systematically assessed. Thus, the aim of this review was to systematically evaluate and perform a meta-analysis of the available data on the efficacy and safety of yoga in terms of improving sleep quality and insomnia in women.
Before beginning the review, we followed the checklist for systematic reviews in concurrence with the preferred reporting items for systematic reviews and meta-analyses (PRISMA) guidelines [ 21 ] and suggestions by the Cochrane Collaboration for programming and conducting systematic reviews and meta-analyses [ 22 ].
Eligibility criteria
Types of studies.
Randomized controlled trials (RCTs), randomized crossover studies, and cluster randomized trials were all eligible for this meta-analysis. No restrictions in terms of language and countries were applied.
Types of participants
Studies that included women (aged ≥18 years) with sleep problems were eligible. No restrictions on the ethnicity and comorbidity of participants were applied.
Types of interventions
No restrictions regarding yoga type, form, structure, frequency, duration or length of intervention programs were applied. Studies on cointerventions that included yoga as a part of multicomponent interventions were excluded because it would be difficult to distinguish the effects of yoga from additional modalities. Studies in control interventions that compared yoga treatments with nontreatment, usual care, wait-lists, and education without active physical exercise programs were all eligible.
Types of outcome measures
The primary outcome of this study was sleep quality. To be included in this review, studies had to assess at least one of the sleep quality measures by using standardized instruments and provide outcomes both at the baseline and follow-up for primary outcomes. In particular, instruments in question include subjective measurements, such as the Pittsburgh Sleep Quality Index (PSQI) and Insomnia Severity Index (ISI), or objective measurements, such as polysomnography (PSG) and actigraphy. The PSQI score have been recommended as a reliable, valid and standardized instrument to measure and to identify quality of sleep. The widely employed Pittsburgh Sleep Quality Index (PSQI), provides a measure of global sleep quality, including sleep latency, sleep duration, habitual sleep efficiency, sleep disturbances, use of sleeping medication, and daytime dysfunction [ 14 ]. The seven components of the PSQI are standardized of areas routinely assessed sleep complaints with possible range of 0–21 points. A global PSQI score of 5 or higher provided a sensitive and specific measure for poor sleep quality [ 14 ]. The ISI score is a reliable and valid instrument to quantify perceived insomnia severity. A global ISI score of 8 or higher is indicative of some degree of insomnia, while moderate insomnia has a score of 15–21 and severe insomnia with a score of 22–28 [ 23 ]. PSG or actigraphy reports the most complete and precise information on the construction and distribution of sleep periods, such as total sleep time (TST), sleep efficiency (SE), and wake time after sleep onset (WASO) [ 24 ]. Sleep quality is also sometimes measured from PSG and actigraphy. Among these objective indices are measures such as sleep onset latency, total sleep time, wake time after sleep onset, sleep efficiency, and number of awakenings [ 25 ].
Secondary outcomes : The secondary outcome included in this study was the safety of the intervention, which was assessed as number of patients with adverse events (AEs), including serious adverse events or nonserious events. Serious adverse events referred to those events that caused death, life-threatening situations, hospitalization, disability or permanent damage, congenital anomaly/birth defect, or the need for medical or surgical intervention to prevent any of the aforementioned outcomes [ 26 ]. All other adverse events were regarded as nonserious.
Search methods
The search strategy comprised four electronic databases from their inception through June 01, 2019: Medline/PubMed, ClinicalKey, ScienceDirect, Embase, PsycINFO, and the Cochrane Library. The literature search was constructed around search terms for “yoga,” “women,” and “sleep” and was adapted for each database as necessary. The complete search strategy for PubMed was as follows: (“yoga” OR “asana” OR “pranayama” OR “dhyana”) AND (“women” OR “female”) AND (“sleep” OR “sleep quality” OR “sleep disturbance” OR “insomnia”). Additional reference lists of identified original articles or reviews, the table of the contents of the Journal of Yoga and Physical Therapy , and Journal of National Taiwan Sports University were searched manually.
Retrieved articles were scanned independently to verify their eligibility, and the entire text was assessed by two reviewers. A conflict of reviewers’ opinions on inclusion or exclusion of any article was discussed with a third reviewer to reach a consensus.
Data extraction and management
Two reviewers independently extracted data on design (e.g., article setting, author/year, country of studies, and sampling strategy), participants (e.g., age, body max index, clinical characteristics, comorbid condition, and overall sample size), interventions (e.g., yoga type, frequency of sessions per week, duration of yoga intervention, and total length of intervention time), control interventions (e.g., type, frequency, length, and duration), and outcomes (e.g., outcome measures with sleep quality and safety-related events). A conflict of reviewers’ opinions was discussed with a third reviewer until consensus was reached.
Risk of bias in individual studies
Two reviewers independently assessed the risk of bias in each study. There were seven domains of assessment for the risk of bias include in the following: (1) random sequence generation, (2) allocation concealment, (3) blinding of participants and personnel, (4) blinding of outcome assessment, (5) incomplete outcome data, (6) selective reporting, and (7) other biases using the Cochrane Systematic Review Manual risk of bias assessment tool [ 22 ]. All domains were scored as low risk, high risk, or unclear risk of bias and assessed individually. A risk of bias table was completed for each included study. A conflict of reviewers’ opinions was discussed with a third reviewer until consensus was reached.
Data assessment of overall effect size
A meta-analysis was conducted with Review Manager 5 software (Version 5.3, The Nordic Cochrane Centre, Copenhagen) and Comprehensive Meta-Analysis Software using a random effects model if at least two studies assessing this specific outcome were obtainable. For continuous outcomes, standardized mean differences (SMDs) with 95% confidence intervals (CIs) were calculated as the difference in means between groups divided by the pooled standard deviation. For studies that did not report data with standard deviations, we calculated these values from standard errors, confidence intervals, or t -values. If adequate information was available, we would plan to perform subgroup analysis. The p value of the summary effect < 0.05 were regarded as indicating statistical significance.
A negative SMD was provided a definition to display the beneficial effects of yoga intervention compared with the control intervention for sleep quality outcomes. Cohen’s categories were used to assess the significance of the overall effect size, with SMD = 0.2–0.5: small effect size; SMD = 0.5–0.8: medium effect size; and SMD > 0.8: large effect size [ 27 ].
Assessment of heterogeneity
Statistical heterogeneity between studies was analyzed using the I 2 statistics and the Cochrane chi-square. The variance between studies was measured using the tau-square (Tau 2 ). The level of heterogeneity was classified as I 2 = 0–24%: low heterogeneity; I 2 = 25–49%: moderate heterogeneity; I 2 = 50–74%: substantial heterogeneity; and I 2 = 75–100%: considerable heterogeneity. Given the low power of this test when only few studies or studies with a low sample size are included in a meta-analysis, a P value of ≤0.1 for the chi-square test was regarded as indicating significant heterogeneity [ 22 ].
Moderator analyses
Moderator and meta-regression analyses were further performed to identify possible reasons for interstudy heterogeneity. The subgroup analysis produced prespecified covariates, including outcome measurement tools, participant type, study quality, study region, participant age, intervention duration and study sample size.
Risk of publication bias
Risk of publication bias was evaluated for each meta-analysis that included at least 10 studies. Funnel plots generated using Review Manager 5 software was estimated from individual studies against each study’s standard error. Publication bias was evaluated through visual analysis, in which roughly the symmetrical funnel plot signifies no evidence of high risk of publication bias [ 28 ]. Potential publication bias was evaluated using the Egger’s Intercept Test, with p values < 0.05 signifying significant bias.
Literature search
The results of the literature search and screening process are summarized in Fig. 1 . The literature search totaled 1338 records; one additional record was retrieved from the Journal of National Taiwan Sports University in the Chinese language database [ 29 ]. In all, 1295 records were excluded because they did not meet all predefined inclusion criteria or were duplicated. Forty-four full-text articles were assessed for eligibility. Twenty-five were excluded because they were not randomized [ 29 , 30 ], did not include relevant outcomes [ 31 , 32 , 33 , 34 , 35 , 36 , 37 ], did not include only female participants [ 38 , 39 , 40 , 41 , 42 , 43 , 44 , 45 , 46 ], included yoga as a part of a multimodal intervention (or combined with other intervention) [ 47 , 48 , 49 , 50 ], lacked adequate control [ 51 ], and did not include a form of yoga intervention [ 52 , 53 ]. Nineteen full-text articles with 1832 participants were included in the qualitative synthesis and were included in the meta-analysis. All articles were published in English.
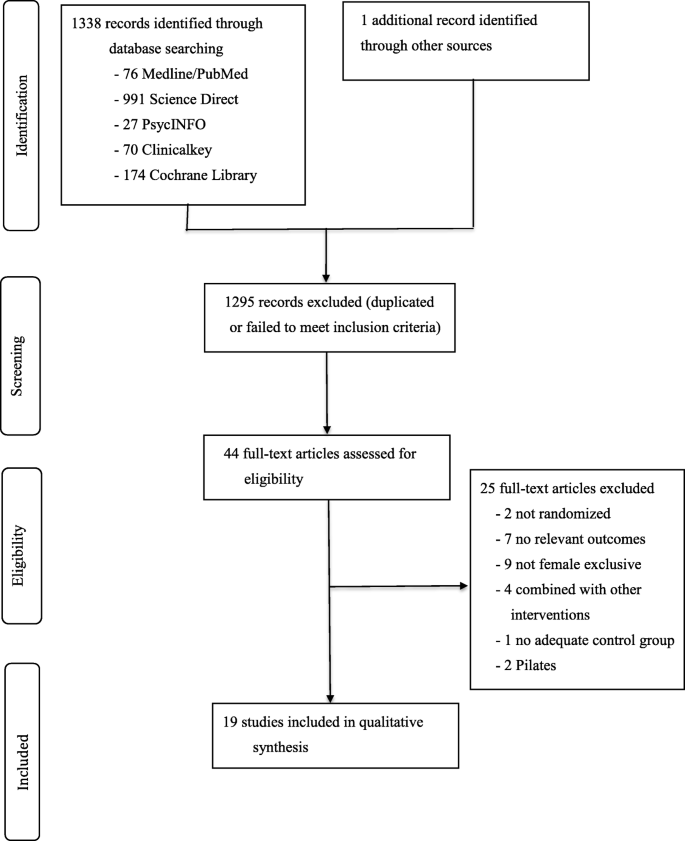
Flowchart of the results of the literature search
Study characteristics
A total of 19 studies were considered eligible for systematic reviews. Information regarding the characteristics of the sample, yoga or control group interventions, outcome measures, and results are listed in Tables 1 and 2 .
Study and participant characteristics
Of the 19 RCTs that were included in Table 1 , six RCTs included healthy participants [ 60 , 61 , 62 , 63 , 67 , 69 ], including nurses [ 67 ], teachers [ 69 ], and women in the menopausal transition period or postmenopausal period [ 60 , 61 , 62 , 63 ]. The other 13 RCTs included breast cancer patients undergoing treatment [ 55 , 57 , 58 , 59 ], breast cancer patients who had completed treatment [ 12 , 54 , 56 , 71 ], type 2 diabetes mellitus patients [ 68 ], fibromyalgia patients [ 64 ], knee osteoarthritis patients [ 66 ], restless leg syndrome patients [ 65 ], and patients experiencing dysfunctional uterine bleeding [ 70 ].
Overall, the 19 RCTs included were conducted in the United States [ 12 , 54 , 55 , 56 , 57 , 58 , 59 , 60 , 62 , 63 , 65 , 66 , 71 ], Brazil [ 61 , 64 ], India [ 69 , 70 ], Iran [ 68 ], and China [ 67 ]. Study participants were recruited from hospitals [ 54 , 55 , 57 , 58 , 67 , 68 , 70 , 71 ], outpatient clinics [ 59 , 61 ] and schools [ 69 ]. The process of recruitment also included using purchased lists and health-plan enrollment files [ 62 , 63 ] and multiple other mechanisms, including flyers, newspaper advertisements, web-based announcements, brochures, public health departments, tumor registry systems, and doctor referrals [ 12 , 56 , 60 , 65 , 66 ]. One study did not reveal the source from which participants were recruited [ 64 ]. Nineteen studies included in the systematic review displayed a baseline of PSQI higher than 5 or ISI higher than 8, indicating poor sleep quality or insomnia. The only exceptions were two studies, with individual control groups in each study displaying a baseline of PSQI lower than 5 [ 69 ] or ISI lower than 8 [ 71 ]. The sample size ranged from 20 to 249 with a median of 96. Participant’s mean age ranged from 29.8 to 71.9 years, with a median of 50.1 years. All participants were women.
Intervention characteristics
Of the 19 included studies in Table 1 , three reported using Iyengar Yoga [ 12 , 60 , 65 ]; two reported using Hatha Yoga [ 56 , 66 ]; two reported using Tibetan Yoga [ 58 , 61 ]; two reported using Restorative Yoga [ 54 , 71 ]; one reported using Vini Yoga [ 63 ]; one reported using Yoga Relaxation with MindSound Resonance Technique [ 69 ]; one reported using yoga breathing exercise in warm water [ 64 ]; and only seven RCTs revealed yoga programs with postures, breathing, relaxation or mediation, without defining a specific style of yoga [ 55 , 57 , 59 , 62 , 67 , 68 , 70 ]. All RCTs included yoga postures in their yoga intervention; 16 RCTs included yoga breathing; 15 RCTs included yoga relaxation; 12 RCTs included meditation; and 7 RCTs included all contents with postures, breathing, relaxation, and meditation for the yoga intervention group [ 55 , 57 , 62 , 67 , 68 , 70 , 71 ]. The duration of yoga interventions ranged from 1 week to 24 weeks, with a median of 10 weeks; the frequency of yoga interventions ranged from one to five weekly sessions of 45 to 120 min. Sixteen studies compared the yoga group with waitlist control groups with no specific treatment; three studies compared the yoga group with the control group, including two studies for education groups [ 12 , 65 ] and one study for social support groups [ 59 ].
Outcome measures
All studies evaluated outcomes directly at the end of interventions. All studies assessed the subjective or objective measurements of sleep quality: 16 RCTs used the PSQI; three RCTs used the ISI [ 61 , 62 , 71 ]; one RCT used PSG [ 61 ]; and two RCTs used actigraphy [ 58 , 63 ]. Safety-related events were reported in only two RCTs [ 56 , 62 ].
Risk of bias
Risk of bias in individual assessments.
Graphical representation of the risk-of-bias assessment is represented in Fig. 2 . All studies had a high or unclear risk of bias in at least one domain. All studies claimed to be randomized; however, three studies did not reveal their content and method of random sequence [ 54 , 61 , 68 ]. Twelve studies did not report methods applied to perform adequate allocation [ 54 , 55 , 57 , 58 , 59 , 60 , 61 , 63 , 67 , 68 , 69 , 70 ]. Most studies offered no data material on blinding. Three studies clearly reported that participants and personnel were blinded [ 12 , 59 , 66 ]. Four studies clearly reported that researchers and outcome assessments were blinded [ 12 , 56 , 59 , 66 ]. Six studies had insufficient data on attrition rates [ 60 , 61 , 62 , 64 , 65 , 68 ]. Twelve studies had a low risk of selection reporting; only two studies had a high risk of selective reporting due to several reported outcome parameters not being revealed in study protocol or duplicate publications reporting different results of the same trial [ 61 , 62 ]. Six studies had a high risk of other potential sources of bias due to poor participant compliance, intervention length, sample size or baseline differences [ 60 , 64 , 65 , 66 , 70 , 71 ].
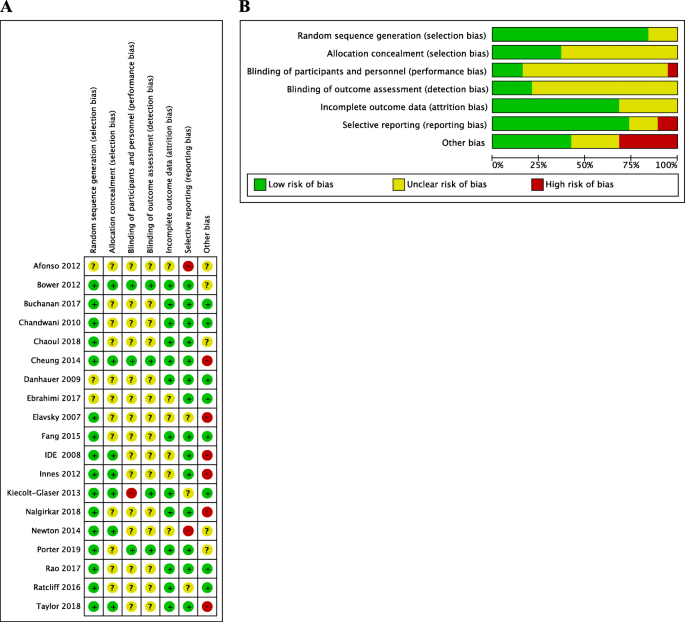
Risk of bias in individual studies. +, low risk of bias;?, unclear risk of bias; −, high risk of bias (a). Risk of bias for each criterion presented as percentages across all included studies (b)
Publication bias
The meta-analysis of the effect of yoga on the sleep quality of women that involved yoga groups compared with control groups included 16 studies. The asymmetrical shape of the funnel plot indicated that subjective publication bias was detected (Fig. 3 ). Objective publication bias was analyzed using Egger’s Regression Test. Egger’s Test consists of the regression between the accuracy of the studies and standardized effects, which are weighted by the inverse of variance. Borderline findings ( P = 0.05) show objective evidence on publication bias between precision and standardized effects of studies in the present study, specifically suggesting need for future studies to expound on the issue.
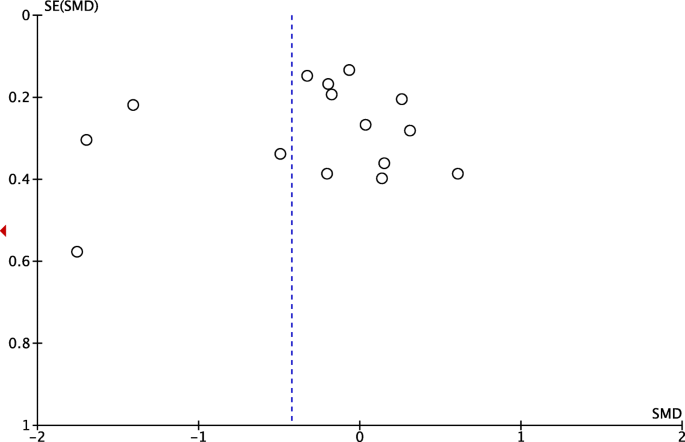
Funnel plot of estimate of publication bias in meta-analysis of the effects of yoga on women’s sleep quality compared to control groups (PSQI). SE: standard error; SMD: standardized mean difference
Analysis of overall effects
Primary outcomes.
The random effects model was applied to analyze the 19 RCTs outcomes by different sleep outcome measurement tools. The meta-analysis of combined data conducted with Comprehensive Meta-Analysis, showed a significant improvement in sleep problems (SMD = -0.327, 95% CI = − 0.506 to − 0.148, P < 0.001). However, significant heterogeneity existed among all the studies (Q = 43.152, I 2 = 58.287%, P = 0.001). Therefore, moderator and meta-regression analyses were conducted to further explore the determinants of the heterogeneity.
The meta-analysis revealed the effects of yoga compared with the control group on the sleep quality and insomnia of women using the PSQI or ISI, as displayed in Fig. 4 . Sixteen RCTs revealed evidence for effects of yoga compared with the control group in improving sleep quality in women using the PSQI (SMD = − 0.54; 95% CI = − 0.89 to − 0.19; P = 0.003). However, three RCTs revealed no effects of yoga compared with the control group in reducing the severity of insomnia in women using ISI (SMD = − 0.13; 95% CI = − 0.74 to 0.48; P = 0.69). Two RCTs revealed no effects of yoga compared with control group in improving sleep efficiency (SMD = 0.85; 95% CI = − 0.56 to 2.26; P = 0.26) or total sleep time (SMD = − 0.06; 95% CI = − 0.26 to 0.13; P = − 0.59) in women using actigraphy.
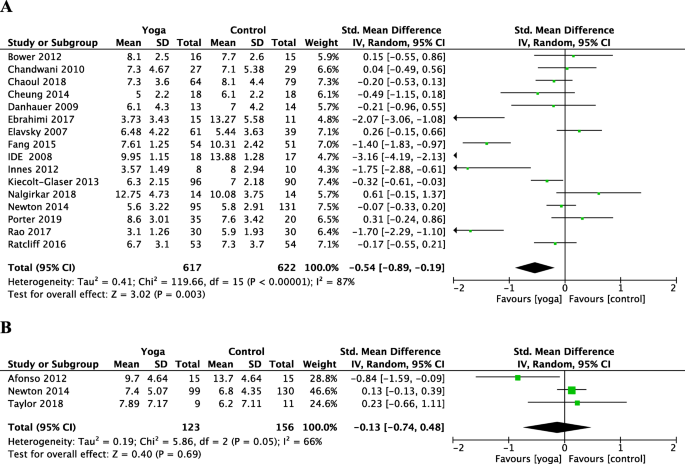
Forest plots for the effects of yoga on sleep quality in women versus control groups. a the global score of the Pittsburgh Sleep Quality Index (PSQI) b the global score of the Insomnia Severity Index (ISI). CI, confidence interval; IV, inverse variance; SD, standard deviation
Secondary outcomes (safety)
Only two studies reported safety-related events. Two events revealed in one study could potentially be attributed to yoga intervention: two women reported the recurrence of chronic back and/or shoulder problems [ 56 ]. In another study, adverse events reported did not differ between the yoga intervention group and the control group ( P = 0.41). These adverse events included muscle aches and strains (6.7%, yoga group; 10.3%, control group), low back pain (4.2%, yoga group; 3.1%, control group), or changes in strength or sensation in arms and legs (5.5% yoga group; 8.9% control group). Dropouts were not regarded as being adverse events because they did not explicitly show a possible reason or explanation for dropout in the original study. No serious adverse effects were reported in the included studies.
Subgroup analyses
Participants were divided into two separate subgroups. Meta-analyses revealed the effects of yoga compared with the control group for women with breast cancer in Fig. 5 . Seven RCTs revealed no evidence for the effect of yoga compared with the control group in improving sleep quality for women with breast cancer using the PSQI (SMD = − 0.15; 95% CI = − 0.31 to 0.01; P = 0.5). Four RCTs revealed no evidence for effects of yoga compared with the control group in improving sleep quality for women undergoing treatment for breast cancer (SMD = − 0.08; 95% CI = − 0.29 to 0.13; P = 0.45). Three RCTs revealed no evidence for positive effects of yoga in terms of improving sleep quality for women with breast cancer who had completed treatment compared with the control group (SMD = − 0.25; 95% CI = − 0.50 to 0.00; P = 0.05).
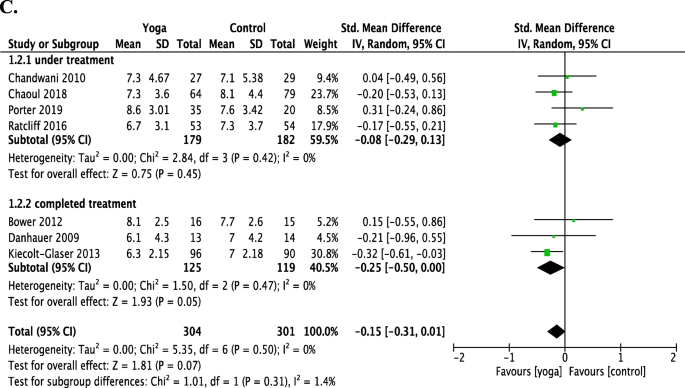
c Forest plots of the effects of yoga on the sleep quality of women with breast cancer (including women under treatment and women who had completed treatment) versus a control group using the global score of the Pittsburgh Sleep Quality Index (PSQI). CI, confidence interval; IV, inverse variance; SD, standard deviation
The meta-analysis showed evidence of the positive effects of yoga on sleep quality compared with control groups for peri/postmenopausal women as displayed in Fig. 6 . Four RCTs revealed no evidence for effects of yoga compared with control groups in improving sleep quality in peri/postmenopausal women using the PSQI (SMD = − 0.31; 95% CI = − 0.95 to 0.33; P = 0.34). Two RCTs revealed no evidence for effects of yoga compared with the control group in reducing severity of insomnia in peri/postmenopausal using ISI (SMD = − 0.29; 95% CI = − 1.23 to 0.65; P = 0.55).
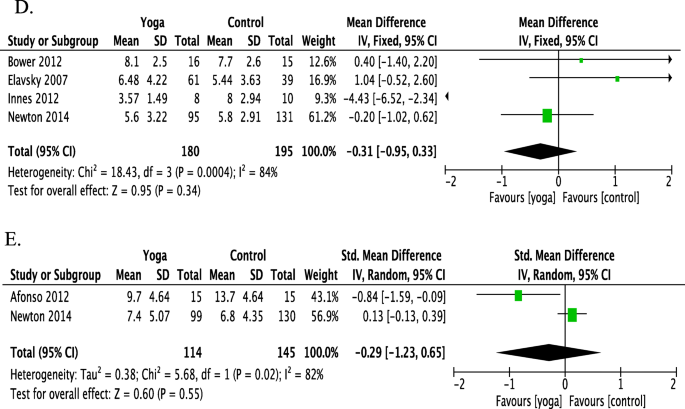
Forest plots displaying the effects of yoga versus control groups on sleep quality in peri/postmenopausal women in ( d ) the global score of the Pittsburgh Sleep Quality Index (PSQI) ( e ) the global score of Insomnia Severity Index (ISI). CI, confidence interval; IV, inverse variance; SD, standard deviation
Moderator analyses, meta-regression
Moderator analyses and meta-regression are presented in Table 3 . Significant factors in observed heterogeneity were identified in yoga on sleep quality and insomnia in women with sleep problems. Studies that used PSQI as outcome measurement tool showed a greater reduction in sleep problems than other studies that used other instruments as outcome measurement tools ( Hedges ’ g = − 0.369 vs. 0.031, P = 0.002). Participants without breast cancer showed more improvement in sleep problems than participants with breast cancer ( Hedges ’ g = − 0.522 vs. -0.148, P = 0.001). Studies without peri/postmenopausal women showed more improvement in sleep problems than studies with peri/postmenopausal women ( Hedges ’ g = − 0.419 vs. -0.084, P = 0.003). Regression analyses revealed a positive correlation with total length of class hours ( p = 0.003), indicating that more total class hours, increased the chance to have significant results. Regression analyses revealed a negative correlation with mean age ( p = 0.003) and sample size ( p = 0.032) of study, indicating that the younger, and smaller sample sizes were more likely to have significant results.
Sensitivity analyses
In the included studies with low risk of selection bias, reporting bias, and other bias, the effect of yoga group compared to control group on women sleep PSQI did not change substantially, including random sequence generation bias (SMD = − 0.45; 95% CI = − 0.84 to − 0.11; P = 0.01; heterogeneity: I 2 = 88%; χ 2 = 107.43, P < 0.00001), allocation concealment bias (SMD = − 0.77; 95% CI = − 1.37 to − 0.16; P = 0.01; heterogeneity: I 2 = 88%; χ 2 = 40.95, P < 0.00001), selective reporting bias (standard mean difference = − 0.59; 95% CI = − 1.10 to − 0.08; P = 0.02; heterogeneity: I 2 = 88%; χ 2 = 93.11, P < 0.00001) and other bias (standard mean difference = − 0.53; 95% CI = − 1.03 to − 0.04; P = 0.03; heterogeneity: I 2 = 86%; χ 2 = 44.03, P < 0.00001). The effect compared with the control group remained significant in terms of sensitivity analyses of performance bias, detection bias, or attrition bias after eliminating high risk bias or uncertain risk bias of the studies.
Summary of evidence
In this systematic review of 19 studies for yoga’s effect on improving women’s sleep quality and severity of insomnia, 19 RCTs revealed evidence for yoga improving sleep problems in women (SMD = − 0.327, 95% CI = − 0.506 to − 0.148, P < 0.001). As shown in Fig. 4 , 16 RCTs meta-analysis suggests yoga can bring 1.2 points improvement in PSQI score (SMD = − 0.54; 95% CI = − 0.89 to − 0.19; P = 0.003). However, seven RCTs revealed no evidence for yoga improving sleep quality in women with breast cancer (Fig. 5 , SMD = − 0.15; 95% CI = − 0.31 to 0.01; P = 0.5). Four RCTs revealed no evidence for improving PSQI in peri/postmenopausal women (Fig. 6 , SMD = − 0.31; 95% CI = − 0.95 to 0.33; P = 0.34). Two RCTs revealed no evidence for improving ISI in peri/postmenopausal women (Fig. 6 , SMD = − 0.29; 95% CI = − 1.23 to 0.65; P = 0.55).
However, heterogeneity of effects were high across all studies. In Table 3 , our moderator analyses yielded statistically significant differences, the effect of yoga for improving sleep problems in non-breast cancer subgroup, non peri/postmenopausal subgroup are superior to breast cancer subgroup, peri/postmenopausal subgroup.
Overall, the application of yoga was not associated with worsening of sleep problems or increased adverse effects. Only two studies explicitly assessed safety-related nonserious adverse events. Yoga is most likely a comparatively safe intervention in this population. However, future RCTs should take more measures to ensure stricter reporting of adverse events and reasons for dropouts.
Comparison with prior reviews
There was no systematic review available that explicitly focused on yoga for improving sleep quality and insomnia in a specific gender. Ours is the first systematic review and meta-analysis with 19 RCTs that to focus on the effects of yoga on women with sleep problems. A previous review published until February 2019 included subgroup analysis of yoga on mind-body therapies on insomnia [ 72 ]. This recent review illustrated that yoga had beneficial effects on subjective sleep quality in participants in all gender groups. Our meta-analysis with 16 RCTs uncovered evidence for the effects of yoga on the sleep quality in women. Only six RCTs were found to have overlapped with this previous review [ 58 , 61 , 62 , 65 , 66 , 67 ]. Our meta-analysis also examined the potential effect on specific subgroups, such as breast cancer and peri/postmenopausal subgroups, with these subgroups serving as potential factors in sleep quality effects (although the result did not show any clear difference). Significant subgroup differences were identified for the following participants types: (peri/postmenopausal vs. non peri/postmenopausal, breast cancer vs. non-breast cancer). Results from the peri/postmenopausal subgroup of women in our systematic review also agreed with previous published reports that suggested that yoga had no significant effect on the severity of insomnia in middle-aged women [ 73 ]. There were baseline differences between participants based on intervention assignment in PSQI scores [ 62 , 65 ]. This may have contributed to results displaying no significant effect in sleep quality in the peri/postmenopausal subgroup of women. Yoga seems to be effective for reducing total menopausal symptoms including psychological, somatic, vasomotor and in previous systematic review and meta-analysis [ 74 ], but there is no direct answer in the study focusing on reducing sleep problems. Future research should ensure more rigorous methodology and adequate sample size concerning the effects of yoga on quality of sleep improvement among the subgroup of peri/postmenopausal women.
Compared to yoga intervention, previous systematic reviews also indicate that programmed exercise improved sleep quality in middle-aged women [ 73 ]. However, these reviews are also limited to high heterogeneity of clinical evidence and failed to provide any specific suggestions for exercise dosages or formats. Additionally, other reviews included an overly wide range of nonpharmacological interventions ranging from walking [ 75 ], tai chi [ 76 ], qigong exercise [ 72 ] showing evidence of beneficial effect in improving self-rated sleep quality. However, despite this, heterogeneity remained high due to difference of interventions and target populations. Our meta-analysis conducted to further explore the determinants of the heterogeneity with subgroup analysis for categorical moderators and continuous moderators to find significant factors for observed heterogeneity.
External and internal validity
Major threats to external validity included the specificity of variables of sampled participants and multiple yoga types or styles. The majority of RCTs included participants from North America, South America, and Asia; lacking studies from Europe and Africa. It might not be as universally transferable to other areas.
There were several other limitations in this review: the wide variety of diagnoses included; the inclusion of only certain types of people or professions, such as nurses, teachers, and peri/postmenopausal women; and patients with breast cancer, type 2 diabetes mellitus, fibromyalgia syndrome, osteoarthritis of the knee, restless leg syndrome, and primary dysfunctional uterine bleeding. The heterogeneity of interventions with different types or styles of yoga (postures, breathing, relaxation, or mediation), and potential bias were included in this systematic review.
Other threat to internal validity was study bias. Only few effects were robust against all potential bias. All of our studies claimed to have applied randomization methods; however, three RCTs failed to provide the design protocol of randomization. Some of the included studies may not have been truly randomized. Erroneous allocation concealment has been empirically revealed to be a significant source of bias in RCTs [ 77 ]. Our included studies only had a low risk or an unclear risk of detection bias without high risk detection bias. The results of meta-analysis did no changed when studies excluded high risk or unclear risk reviews on selection bias or reporting bias. The internal validity of the review appeared to be limited but acceptable.
Strengths and weaknesses
This is the first and latest systematic review and meta-analysis available on yoga for sleep quality and insomnia in women. A large number of RCTs on female population-related physiological and physiological comorbidities and risk factors in insomnia were included. There were five primary limitations of this review. First, subjective publication bias revealed in this review may have been due to selective reporting bias, which means that articles with negative findings may have not been published or poor methodological quality of including articles. We have applied Egger’s Test for objective publication bias in our review. Second, the participant characteristics included in the review were heterogeneous; subgroups were included to analyze the effectiveness of different participant groups; and the small number of RCTs limited data presentation. Third, the severity of the sleep complaints and health status of participants was not considered or individually listed in each study. Baseline differences in PSQI scores were found between intervention and control groups in three studies [ 56 , 62 , 65 ]. This may have led to heterogeneity. The fourth limitation was the intensity, frequency, and duration of yoga interventions were heterogeneous; short term applications of less than 1 month yoga intervention were found in some studies [ 58 , 64 , 69 ]. Only four reviews reported long-term follow up effects, ranging from 3 months to 12 months [ 55 , 56 , 57 , 58 ]. Lastly, lack of safety issue evaluation including serious adverse events or nonserious events in each study.
Implications for further research
This systematic review and meta-analysis was limited by the low methodological quality of included studies. Further RCTs should ensure rigorous methodology and reporting, which would mean adequate sample size, adequate randomization, allocation concealment, intention-to-treat analysis, and blinding of at least outcome assessors [ 78 ]. Researchers for study interventions may need to apply a standard protocol. Adequate reporting of safety issues with yoga intervention should be discussed in future randomized controlled trials. Evidence was limited because few studies report safety-related adverse effects. Most of the included studies failed to report this aspect.
This systematic review and meta-analysis demonstrated that yoga intervention in women has benefits compared to non-active control conditions in term of managing sleep problems. The moderator analyses suggested that participants in the non-breast cancer subgroup or participants in the non-peri/postmenopausal subgroup were associated with greater benefits, with the longer total length of class time, the more beneficial these practices were.
Availability of data and materials
All data analyzed during this study are included in this published article and the original studies’ publications.
Abbreviations
Adverse events
Body max index
Confidence interval
Diagnostic and Statistical Manual of Mental Disorders, Fourth Edition criteria
Dysfunctional uterine dysfunction
Eastern Cooperative Oncology Group Performance Status
Follicle-stimulating hormone
Insomnia Severity Index
Inverse variance
Luteinizing hormones
Mean differences
Osteoarthritis
Onset latency
Preferred reporting items for systematic reviews and meta- analyses
Pittsburgh Sleep Quality Index
Randomized controlled trial
Standard deviation
Sleep efficiency
Standard error
Standardized mean differences
Slow- wave sleep
Total sleep time
Wake time after sleep onset
Radiotherapy treatment
Kessler RC, Berglund PA, Coulouvrat C, Hajak G, Roth T, Shahly V, et al. Insomnia and the performance of US workers: results from the America insomnia survey. Sleep. 2011;34:1161–71.
PubMed PubMed Central Google Scholar
Zhang B, Wing YK. Sex differences in insomnia: a meta-analysis. Sleep. 2006;29:85–93.
PubMed Google Scholar
Kische H, Ewert R, Fietze I, Gross S, Wallaschofski H, Völzke H, et al. Sex hormones and sleep in men and women from the general population: a cross-sectional observational study. J Clin Endocrinol Metab. 2016;101:3968–77.
CAS PubMed Google Scholar
Calhoun SL, Fernandez-Mendoza J, Vgontzas AN, Liao D, Bixler EO. Prevalence of insomnia symptoms in a general population sample of young children and preadolescents: gender effects. Sleep Med. 2014;15:91–5.
Kizilirmak A, Timur S, Kartal B. Insomnia in pregnancy and factors related to insomnia. Sci World J. 2012;2012:197093.
Google Scholar
Kravitz HM, Ganz PA, Bromberger J, Powell LH, Sutton-Tyrrell K, Meyer PM. Sleep difficulty in women at midlife: a community survey of sleep and the menopausal transition. Menopause. 2003;10:19–28.
van Straten A, van der Zweerde T, Kleiboer A, Cuijpers P, Morin CM, Lancee J. Cognitive and behavioral therapies in the treatment of insomnia: a meta-analysis. Sleep Med Rev. 2018;38:3–16.
Riemann D, Baglioni C, Bassetti C, Bjorvatn B, Dolenc Groselj L, et al. European guideline for the diagnosis and treatment of insomnia. J Sleep Res. 2017;26(6):675–700.
Winkler A, Auer C, Doering BK, Rief W. Drug treatment of primary insomnia: a meta-analysis of polysomnographic randomized controlled trials. CNS Drugs. 2014;28:799–816.
Schroeck JL, Ford J, Conway EL, Kurtzhalts KE, Gee ME, Vollmer KA, et al. Review of safety and efficacy of sleep medicines in older adults. Clin Ther. 2016;38(11):2340–72.
Feuerstein G. The Yoga Tradition: Its History, Literature, Philosophy, and Practice. Prescott: Hohm Press. 2008.
Bower JE, Garet D, Sternlieb B, Ganz PA, Irwin MR, Olmstead R, et al. Yoga for persistent fatigue in breast cancer survivors: a randomized controlled trial. Cancer. 2012;118:3766–75.
Zeichner SB, Zeichner RL, Gogineni K, Shatil S, Ioachimescu O. Cognitive behavioral therapy for insomnia, mindfulness, and yoga in patients with breast cancer with sleep disturbance: a literature review. Breast Cancer (Auckl). 2017;11:1178223417745564.
Buysse DJ, Reynolds CF 3rd, Monk TH, Berman SR, Kupfer DJ. The Pittsburgh sleep quality index: a new instrument for psychiatric practice and research. Psychiatry Res. 1989;28(2):193–213.
Hyyppa MT, Kronholm E. Quality of sleep and chronic illnesses. J Clin Epidemiol. 1989;42(7):633–8.
Gellman MD, Turner JR. Sleep quality. In: Encyclopedia of behavioral medicine; 2013.
Ohayon M, Wickwire EM, Hirshkowitz M, Albert SM, Avidan A, Daly FJ, et al. National Sleep Foundation’s sleep quality recommendations: first report. Sleep Health. 2017;3(1):6–19.
Edinger JD, Bonnet MH, Bootzin RR, Doghramji K, Dorsey CM, Espie CA, et al. Derivation of research diagnostic criteria for insomnia: report of an American Academy of Sleep Medicine work group. Sleep. 2004;27(8):1567–96.
Gong H, Ni C, Shen X, Wu T, Jiang C. Yoga for prenatal depression: a systematic review and meta-analysis. BMC Psychiatry. 2015;15:14.
McGovern CE, Cheung C. Yoga and quality of life in women with primary dysmenorrhea: a systematic review. J Midwifery Womens Health. 2018;63:470–82.
Moher D, Liberati A, Tetzlaff J, Altman DG, PRISMA Group. Reprint--preferred reporting items for systematic reviews and meta-analyses: the PRISMA statement. Phys Ther. 2009;89:873–80.
Higgins JPT, Green S, Cochrane Collaboration. Cochrane handbook for systematic reviews of interventions, Cochrane Book Series, vol. xxi. Chichester; Hoboken: Wiley-Blackwell; 2008. p. 649.
Bastien CH, Vallieres A, Morin CM. Validation of the insomnia severity index as an outcome measure for insomnia research. Sleep Med. 2001;2:297–307.
Ibanez V, Silva J, Cauli O. A survey on sleep assessment methods. Peer J. 2018;6:e4849.
Buysse DJ, Ancoli-Israel S, Edinger JD, Lichstein KL, Morin CM. Recommendations for a standard research assessment of insomnia. Sleep. 2006;29(9):1155–73.
Administration UFaD. What is a serious adverse event? 2019. http://www.fda.gov/safety/reporting-serious-problems-fda/what-serious-adverse-event .
Cohen J. Statistical power analysis for the behavioral science. 2nd ed. Hillsdale: L. Erlbaum Associates; 1988.
Egger M, Davey Smith G, Schneider M, Minder C. Bias in meta-analysis detected by a simple, graphical test. BMJ. 1997;315:629–34.
CAS PubMed PubMed Central Google Scholar
Yen CY. Effect of body balance sport training on depressive tendencies and sleep quality of female college students. J Natl Taiwan Sports Univ. 2008;19:37–50.
Sun YC, Hung YC, Chang Y, Kuo SC. Effects of a prenatal yoga programme on the discomforts of pregnancy and maternal childbirth self-efficacy in Taiwan. Midwifery. 2010;26:e31–6.
Lengacher CA, Reich RR, Ramesar S, Alinat CB, Moscoso M, Cousin L, et al. Feasibility of the mobile mindfulness-based stress reduction for breast cancer (mMBSR(BC)) program for symptom improvement among breast cancer survivors. Psychooncology. 2018;27:524–31.
Carson JW, Carson KM, Porter LS, Keefe FJ, Seewaldt VL. Yoga of awareness program for menopausal symptoms in breast cancer survivors: results from a randomized trial. Support Care Cancer. 2009;17:1301–9.
Chattha R, Nagarathna R, Padmalatha V, Nagendra HR. Effect of yoga on cognitive functions in climacteric syndrome: a randomised control study. BJOG. 2008;115:991–1000.
Vadiraja SH, Rao MR, Nagendra RH, Nagarathna R, Rekha M, Vanitha N, et al. Effects of yoga on symptom management in breast cancer patients: a randomized controlled trial. Int J Yoga. 2009;2:73–9.
Witek Janusek L, Tell D, Mathews HL. Mindfulness based stress reduction provides psychological benefit and restores immune function of women newly diagnosed with breast cancer: a randomized trial with active control. Brain Behav Immun. 2019;80:358–73.
Lotzke D, Wiedemann F, Rodrigues Recchia D, Ostermann T, Sattler D, Ettl J, et al. Iyengar-yoga compared to exercise as a therapeutic intervention during (neo)adjuvant therapy in women with stage I-III breast cancer: health-related quality of life, mindfulness, spirituality, life satisfaction, and cancer-related fatigue. Evid Based Complement Alternat Med. 2016;2016:5931816.
Ghaffarilaleh G, Ghaffarilaleh V, Sanamno Z, Kamalifard M. Yoga positively affected depression and blood pressure in women with premenstrual syndrome in a randomized controlled clinical trial. Complement Ther Clin Pract. 2019;34:87–92.
Mustian KM, Sprod LK, Janelsins M, Peppone LJ, Palesh OG, Chandwani K, et al. Multicenter, randomized controlled trial of yoga for sleep quality among cancer survivors. J Clin Oncol. 2013;31:3233–41.
Kohn M, Persson Lundholm U, Bryngelsson IL, Anderzén-Carlsson A, Westerdahl E. Medical yoga for patients with stress-related symptoms and diagnoses in primary health care: a randomized controlled trial. Evid Based Complement Alternat Med. 2013;2013:215348.
Sakuma Y, Sasaki-Otomaru A, Ishida S, Kanoya Y, Arakawa C, Mochizuki Y, et al. Effect of a home-based simple yoga program in child-care workers: a randomized controlled trial. J Altern Complement Med. 2012;18:769–76.
Chen KM, Chen MH, Chao HC, Hung HM, Lin HS, Li CH. Sleep quality, depression state, and health status of older adults after silver yoga exercises: cluster randomized trial. Int J Nurs Stud. 2009;46:154–63.
Jain M, Tripathy PR, Manik R, Tripathy S, Behera B, Barman A. Short term effect of yoga asana - an adjunct therapy to conventional treatment in frozen shoulder. J Ayurveda Integr Med. 2019.
Carson JW, Carson KM, Jones KD, Bennett RM, Wright CL, Mist SD. A pilot randomized controlled trial of the yoga of awareness program in the management of fibromyalgia. Pain. 2010;151:530–9.
Zhang JX, Liu XH, Xie XH, Zhao D, Shan MS, Zhang XL, et al. Mindfulness-based stress reduction for chronic insomnia in adults older than 75 years: a randomized, controlled, single-blind clinical trial. Explore. 2015;11:180–5.
Yurtkuran M, Alp A, Yurtkuran M, Dilek K. A modified yoga-based exercise program in hemodialysis patients: a randomized controlled study. Complement Ther Med. 2007;15:164–71.
Ward L, Stebbings S, Athens J, Cherkin D, David Baxter G. Yoga for the management of pain and sleep in rheumatoid arthritis: a pilot randomized controlled trial. Musculoskeletal Care. 2018;16:39–47.
Field T, Diego M, Delgado J, Medina L. Tai chi/yoga reduces prenatal depression, anxiety and sleep disturbances. Complement Ther Clin Pract. 2013;19:6–10.
Andersen SR, Würtzen H, Steding-Jessen M, Christensen J, Andersen KK, Flyger H, et al. Effect of mindfulness-based stress reduction on sleep quality: results of a randomized trial among Danish breast cancer patients. Acta Oncol. 2013;52:336–44.
Vardar Yagli N, Şener G, Arıkan H, Sağlam M, İnal İnce D, Savcı S, et al. Do yoga and aerobic exercise training have impact on functional capacity, fatigue, peripheral muscle strength, and quality of life in breast cancer survivors? Integr Cancer Ther. 2015;14:125–32.
Guthrie KA, Larson JC, Ensrud KE, Anderson GL, Carpenter JS, Freeman EW, et al. Effects of pharmacologic and nonpharmacologic interventions on insomnia symptoms and self-reported sleep quality in women with hot flashes: a pooled analysis of individual participant data from four MsFLASH trials. Sleep. 2018;41(1). https://doi.org/10.1093/sleep/zsx190 .
Reed SD, Guthrie KA, Newton KM, Anderson GL, Booth-LaForce C, Caan B, et al. Menopausal quality of life: RCT of yoga, exercise, and omega-3 supplements. Am J Obstet Gynecol. 2014;210:244.e1–11.
CAS Google Scholar
Ashrafinia F, Mirmohammadali M, Rajabi H, Kazemnejad A, Sadeghniiathaghighi K, Amelvalizadeh M, et al. The effects of Pilates exercise on sleep quality in postpartum women. J Bodyw Mov Ther. 2014;18:190–9.
Aibar-Almazána A, Hita-Contreras F, Cruz-Díaz D, de la Torre-Cruz M, Jiménez-García JD, Martínez-Amat A. Effects of Pilates training on sleep quality, anxiety, depression and fatigue in postmenopausal women: a randomized controlled trial. Maturitas. 2019;124:62–7.
Danhauer SC, Mihalko SL, Russell GB, Campbell CR, Felder L, Daley K, et al. Restorative yoga for women with breast cancer: findings from a randomized pilot study. Psychooncology. 2009;18:360–8.
Chandwani KD, Thornton B, Perkins GH, Arun B, Raghuram NV, Nagendra HR, et al. Yoga improves quality of life and benefit finding in women undergoing radiotherapy for breast cancer. J Soc Integr Oncol. 2010;8:43–55.
Kiecolt-Glaser JK, Barrow J, Makambi K, Sheppard V, Wallington SF, Martin C, et al. Yoga’s impact on inflammation, mood, and fatigue in breast cancer survivors: a randomized controlled trial. J Clin Oncol. 2014;32:1040–9.
Ratcliff CG, Milbury K, Chandwani KD, Chaoul A, Perkins G, Nagarathna R, et al. Examining mediators and moderators of yoga for women with breast cancer undergoing radiotherapy. Integr Cancer Ther. 2016;15:250–62.
Chaoul A, Milbury K, Spelman A, Basen-Engquist K, Hall MH, Wei Q, et al. Randomized trial of Tibetan yoga in patients with breast cancer undergoing chemotherapy. Cancer. 2018;124:36–45.
Porter LS, et al., Feasibility of a mindful yoga program for women with metastatic breast cancer: results of a randomized pilot study. Support Care Cancer, 2019;27(11):4307–16.
Elavsky S, McAuley E. Lack of perceived sleep improvement after 4-month structured exercise programs. Menopause. 2007;14:535–40.
Afonso RF, Hachul H, Kozasa EH, Oliveira Dde S, Goto V, Rodrigues D, et al. Yoga decreases insomnia in postmenopausal women: a randomized clinical trial. Menopause. 2012;19:186–93.
Newton KM, Reed SD, Guthrie KA, Sherman KJ, Booth-LaForce C, Caan B, et al. Efficacy of yoga for vasomotor symptoms: a randomized controlled trial. Menopause. 2014;21:339–46.
Buchanan DT, Landis CA, Hohensee C, Guthrie KA, Otte JL, Paudel M, et al. Effects of yoga and aerobic exercise on actigraphic sleep parameters in menopausal women with hot flashes. J Clin Sleep Med. 2017;13:11–8.
Ide MR, Laurindo LMM, Rodrigues-Junior AL, Tanaka C. Effect of aquatic-respiratory exercise-based program in patients with fibromyalgia. Int J Rheum Dis. 2008;11:131–40.
Innes KE, Selfe TK. The effects of a gentle yoga program on sleep, mood, and blood pressure in older women with restless legs syndrome (RLS): a preliminary randomized controlled trial. Evid Based Complement Alternat Med. 2012;2012:294058.
Cheung C, Wyman JF, Resnick B, Savik K. Yoga for managing knee osteoarthritis in older women: a pilot randomized controlled trial. BMC Complement Altern Med. 2014;14:160.
Fang R, Li X. A regular yoga intervention for staff nurse sleep quality and work stress: a randomised controlled trial. J Clin Nurs. 2015;24:3374–9.
Ebrahimi M, Guilan-Nejad TN, Pordanjani AF. Effect of yoga and aerobics exercise on sleep quality in women with type 2 diabetes: a randomized controlled trial. Sleep Sci. 2017;10:68–72.
Rao M, Metri KG, Raghuram N, Hongasandra NR. Effects of mind sound resonance technique (yogic relaxation) on psychological states, sleep quality, and cognitive functions in female teachers: a randomized, controlled trial. Adv Mind Body Med. 2017;31:4–9.
Nalgirkar SP, Vinchurkar SA, Saoji AA, Mohanty S. Yoga as a therapeutic intervention in the management of dysfunctional uterine bleeding: a controlled pilot study. J Midlife Health. 2018;9(1):8–13.
Taylor TR, Barrow J, Makambi K, Sheppard V, Wallington SF, Martin C, et al. A restorative yoga intervention for African-American breast cancer survivors: a pilot study. J Racial Ethn Health Disparities. 2018;5:62–72.
Wang X, Li P, Pan C, Dai L, Wu Y, Deng Y. The effect of mind-body therapies on insomnia: a systematic review and meta-analysis. Evid Based Complement Alternat Med. 2019;2019:9359807.
Rubio-Arias JA, Marín-Cascales E, Ramos-Campo DJ, Hernandez AV, Pérez-López FR. Effect of exercise on sleep quality and insomnia in middle-aged women: a systematic review and meta-analysis of randomized controlled trials. Maturitas. 2017;100:49–56.
Cramer H, Peng W, Lauche R. Yoga for menopausal symptoms-a systematic review and meta-analysis. Maturitas. 2018;109:13–25.
Tang MF, Chiu HY, Xu X, Kwok JY, Cheung DST, Chen CY, et al. Walking is more effective than yoga at reducing sleep disturbance in cancer patients: a systematic review and meta-analysis of randomized controlled trials. Sleep Med Rev. 2019;47:1–8.
Du S, Dong J, Zhang H, Jin S, Xu G, Liu Z, et al. Taichi exercise for self-rated sleep quality in older people: a systematic review and meta-analysis. Int J Nurs Stud. 2015;52(1):368–79.
Schulz KF, Chalmers I, Hayes RJ, Altman DG. Empirical evidence of bias. Dimensions of methodological quality associated with estimates of treatment effects in controlled trials. JAMA. 1995;273:408–12.
Schulz KF, Altman DG, Moher D. CONSORT 2010 statement: updated guidelines for reporting parallel group randomised trials. J Pharmacol Pharmacother. 2010;1:100–7.
Download references
Acknowledgements
Not applicable.
The authors thank to the editor’s valuable suggestion. This work was supported by the Taoyuan Armed Forces General Hospital (AFTYGH-10831 and TYAFGH-D-109026). The funder had no involvement in the design of this study and will not have any role during its execution, analyses or interpretation of data, writing the manuscript, and decision to submit the manuscript for publication.
Author information
Authors and affiliations.
Department of Psychiatry, Taoyuan Armed Forces General Hospital, Taoyuan, Taiwan
Wei-Li Wang, Kuang-Huei Chen, Ying-Chieh Pan & Yuan-Yu Chan
Department of Psychiatry, Beitou Branch, Tri-Service General Hospital, National Defense Medical Center, Taipei, Taiwan
Szu-Nian Yang
Department of Psychology, Chung Yuan Christian University, Taoyuan, Taiwan
Yuan-Yu Chan
You can also search for this author in PubMed Google Scholar
Contributions
WLW, KHC, YCP, SNY and YYC designed the study. WLW conducted the literature searches. Selection of studies and data extraction: KHC and YCP. Disagreements were resolved by discussion or arbitration by YCP and SNY. Statistical analysis: WLW, KHC, YCP, SNY and YYC. FC drafted the manuscript. WLW, KHC, YCP, SNY and YYC critically revised the manuscript and approved the final version.
Corresponding author
Correspondence to Yuan-Yu Chan .
Ethics declarations
Ethics approval and consent to participate, consent for publication, competing interests.
The authors declare that they have no competing interests.
Additional information
Publisher’s note.
Springer Nature remains neutral with regard to jurisdictional claims in published maps and institutional affiliations.
Rights and permissions
Open Access This article is licensed under a Creative Commons Attribution 4.0 International License, which permits use, sharing, adaptation, distribution and reproduction in any medium or format, as long as you give appropriate credit to the original author(s) and the source, provide a link to the Creative Commons licence, and indicate if changes were made. The images or other third party material in this article are included in the article's Creative Commons licence, unless indicated otherwise in a credit line to the material. If material is not included in the article's Creative Commons licence and your intended use is not permitted by statutory regulation or exceeds the permitted use, you will need to obtain permission directly from the copyright holder. To view a copy of this licence, visit http://creativecommons.org/licenses/by/4.0/ . The Creative Commons Public Domain Dedication waiver ( http://creativecommons.org/publicdomain/zero/1.0/ ) applies to the data made available in this article, unless otherwise stated in a credit line to the data.
Reprints and permissions
About this article
Cite this article.
Wang, WL., Chen, KH., Pan, YC. et al. The effect of yoga on sleep quality and insomnia in women with sleep problems: a systematic review and meta-analysis. BMC Psychiatry 20 , 195 (2020). https://doi.org/10.1186/s12888-020-02566-4
Download citation
Received : 31 July 2019
Accepted : 24 March 2020
Published : 01 May 2020
DOI : https://doi.org/10.1186/s12888-020-02566-4
Share this article
Anyone you share the following link with will be able to read this content:
Sorry, a shareable link is not currently available for this article.
Provided by the Springer Nature SharedIt content-sharing initiative
- Sleep quality
- Complementary and alternative medicine
- Meta-analysis
BMC Psychiatry
ISSN: 1471-244X
- General enquiries: [email protected]
Featured Topics
Featured series.
A series of random questions answered by Harvard experts.
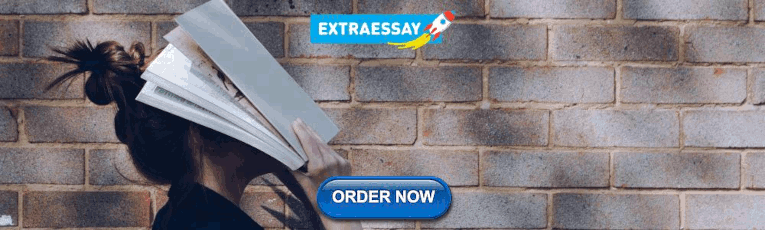
Explore the Gazette
Read the latest.
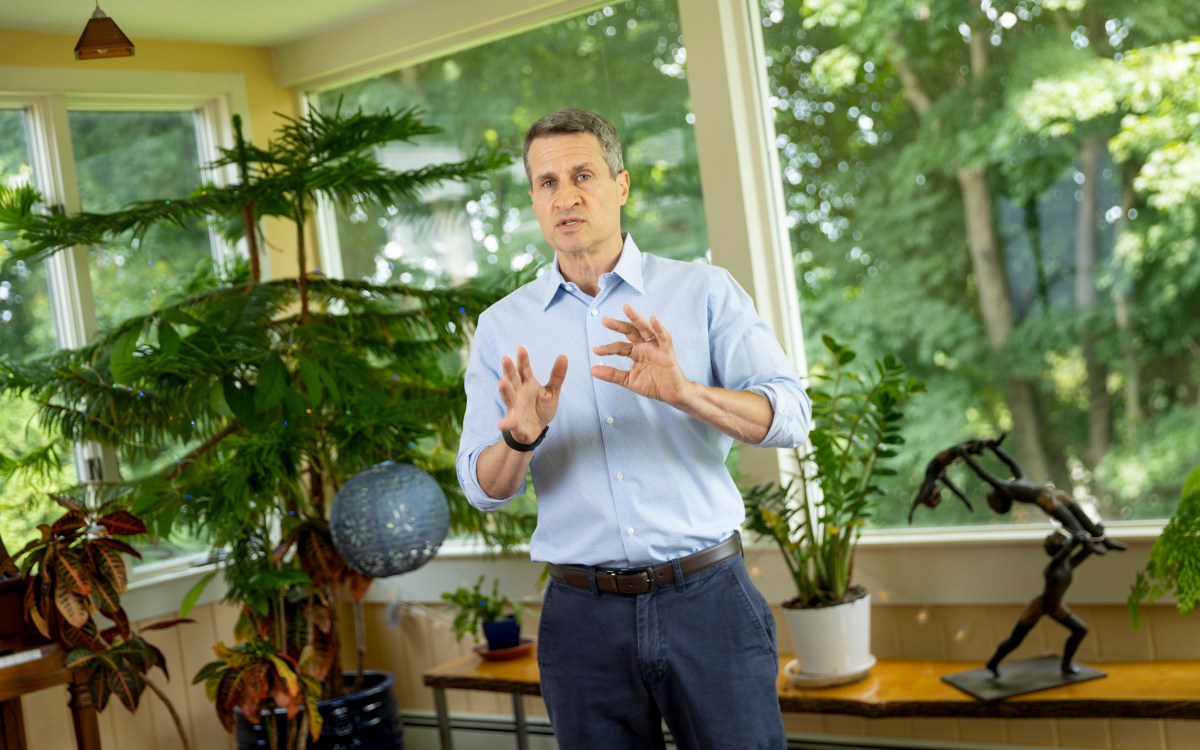
Beginning of end of HIV epidemic?
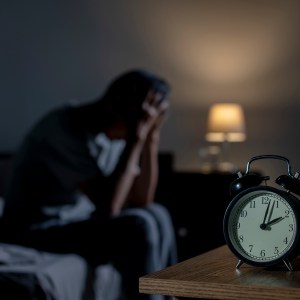
Can good sleep help prevent diabetes?
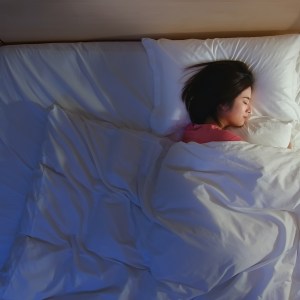
Between bright light and a good mood, plenty of sleep
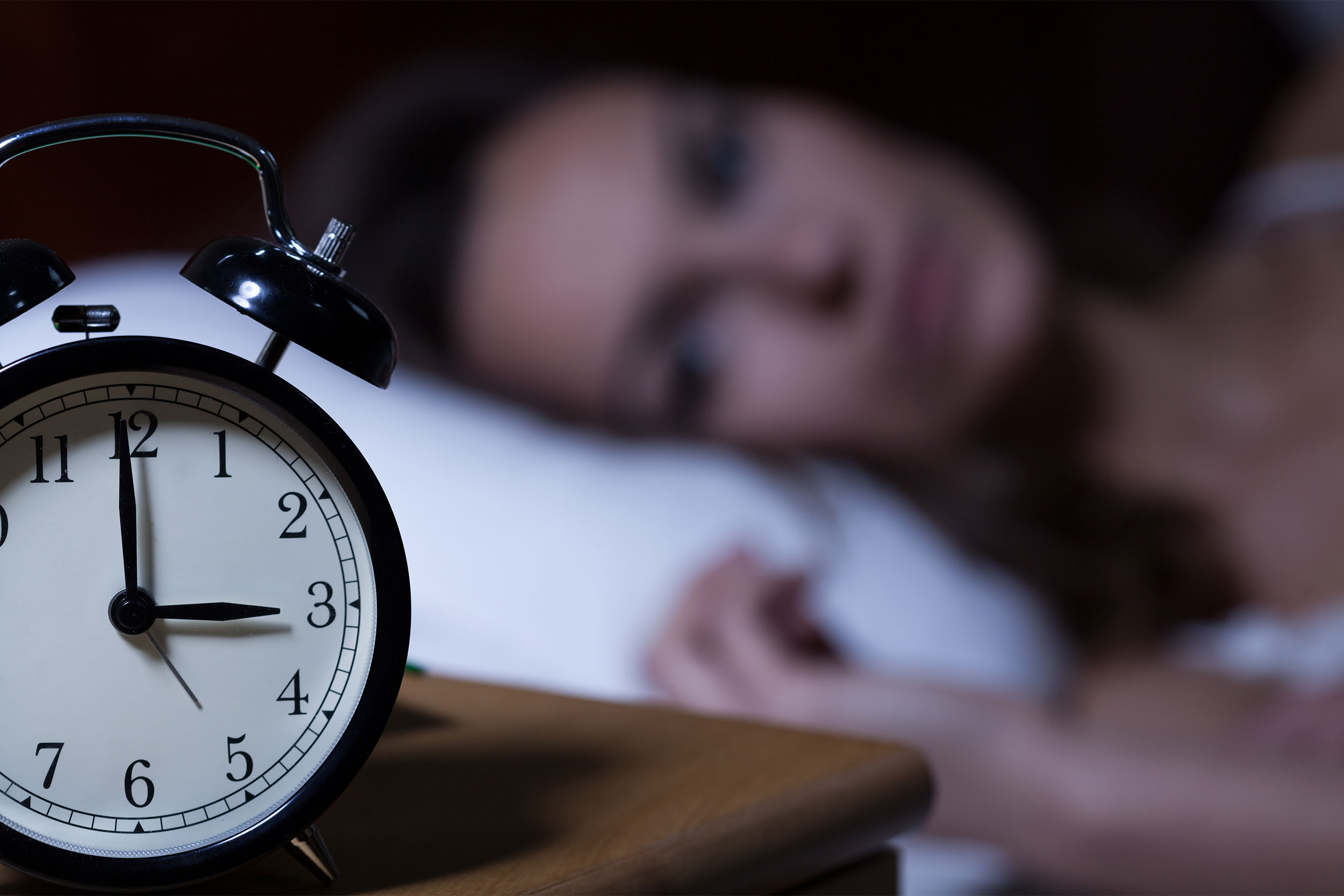
Less rest, more stress for the weary
Kira Sampson
BWH Communications
As women’s sleep goes down, their blood pressure goes up, study finds
Women who sleep less than seven to eight hours at night are at greater risk of developing hypertension, or high blood pressure, finds a new study by the Channing Division of Network Medicine at Brigham and Women’s Hospital. Results are published in the journal Hypertension .
From 2001-2017, researchers followed 66,122 participants between 25 and 42 years of age in the Nurses’ Health Study II (NHS2) cohort, all without hypertension at the study’s onset. Shahab Haghayegh , a research fellow at the Brigham and Harvard Medical School, and his colleagues collected information on participants’ age, race, body mass index (BMI), diet, lifestyle, physical activity, history of sleep apnea, and family history of hypertension and assessed the incidence of hypertension among the group every two years.
“This study highlights yet another reason why getting a good night’s sleep is so important.” Shahab Haghayegh, BWH and Harvard Medical School
Data analyses revealed that women with sleeping difficulties had higher BMIs, lower physical activity, and poorer diets, on average. Researchers also found that those who struggled with sleep were likelier to smoke and drink alcohol and have previously gone through menopause.
Among the 25,987 cases of hypertension documented over the follow-up, women who slept less than seven to eight hours a night had a significantly higher risk of developing hypertension, according to the data collected. Similarly, women who had trouble falling asleep and staying asleep were also likelier to develop hypertension. Waking up early in the morning was not associated with this increased risk. Notably, these associations, remained significant after controlling for participant shift work schedules (night versus day shifts) and chronotype (the tendency to keep early bird or night owl hours).
More like this
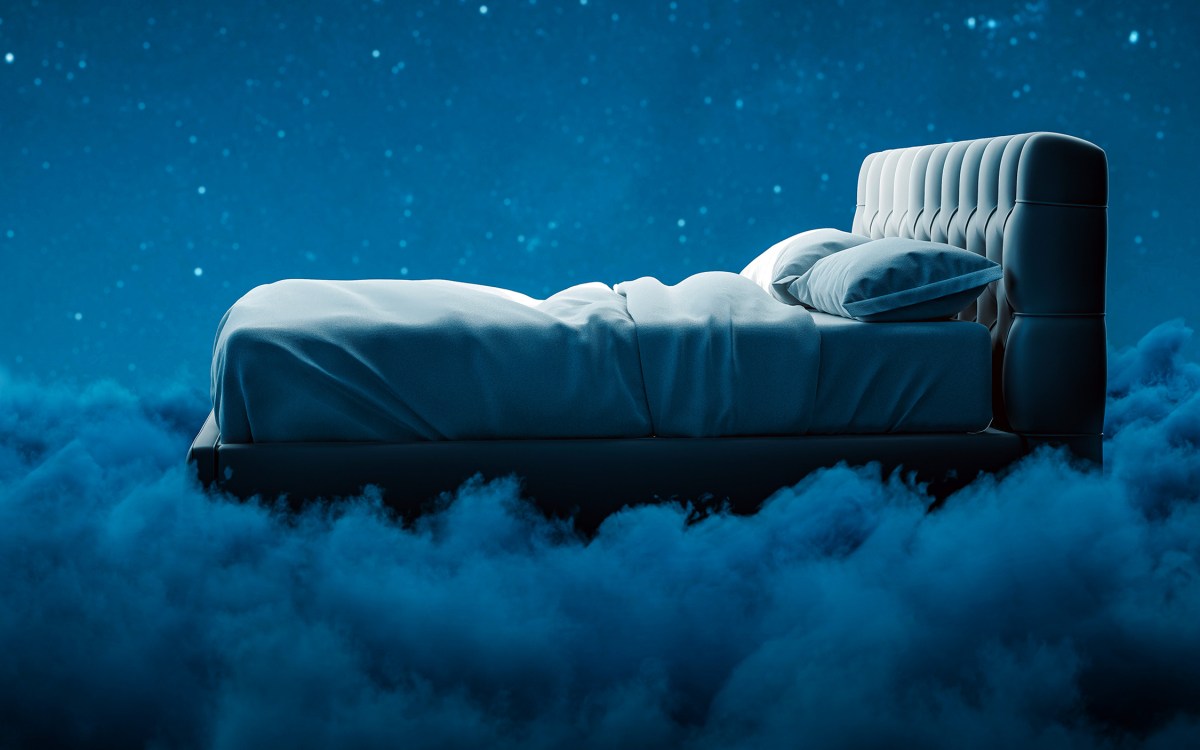
Not getting enough sleep? That’s only half the battle.
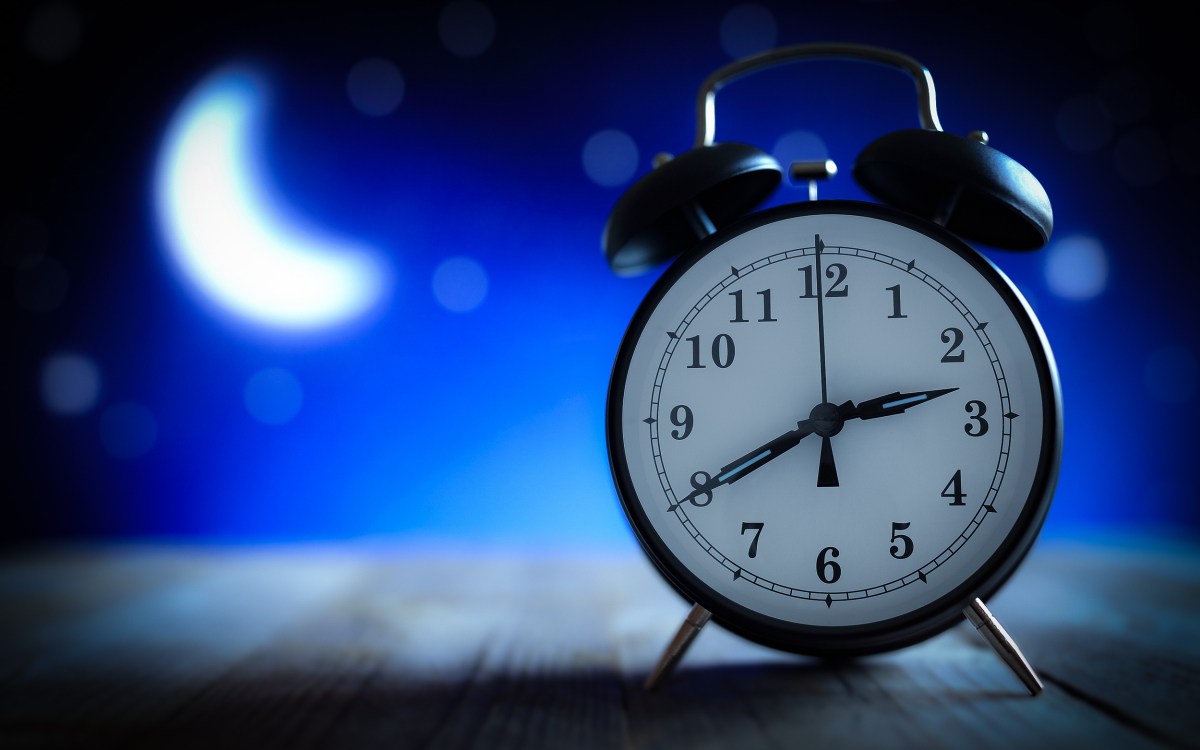
The night owl’s disease problem
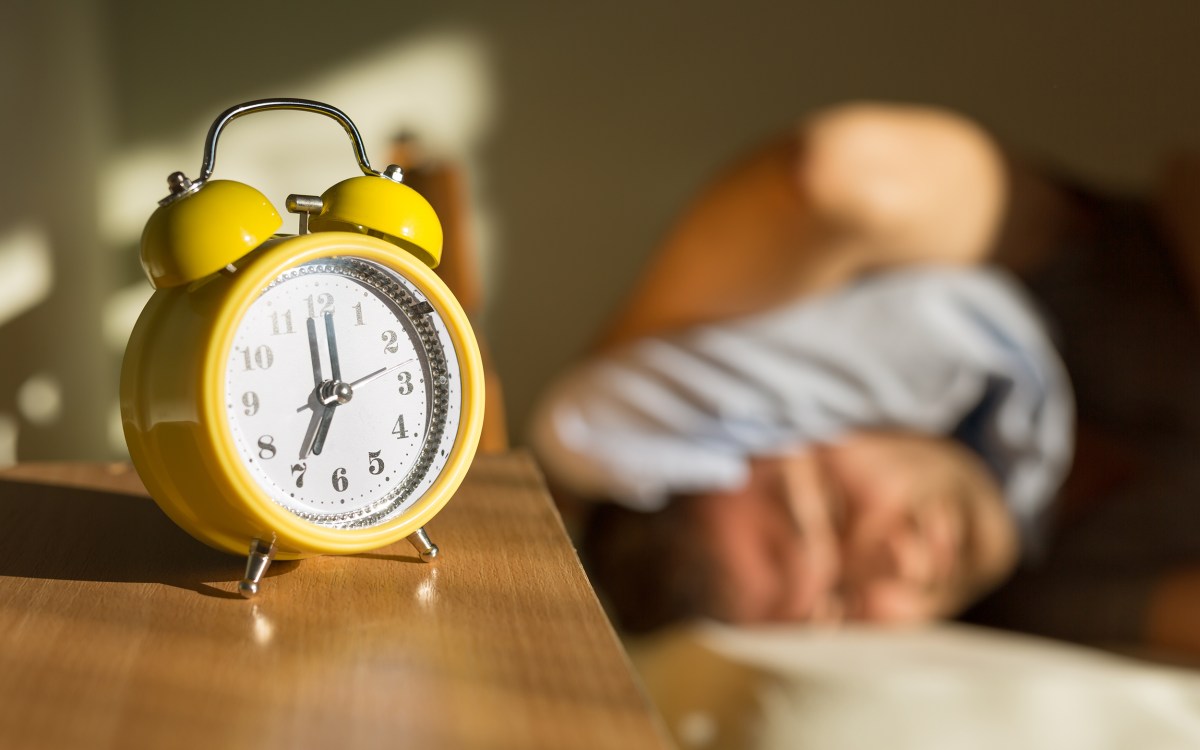
Lack of sleep will catch up to you in more ways than one
While the exact nature of the relationship between sleep and risk of hypertension is unknown, Haghayegh said that sleep difficulties can lead to a chain of events that can increase sodium retention, arterial stiffness, and cardiac output, potentially leading to hypertension. Disruptions to the sleep/wake cycle can also influence blood vessel constriction/relaxation and the function of cells that regulate the vascular tone.
While this study only looked at the association between sleep and hypertension in women, researchers hope to expand their work to include men and nonbinary participants.
A second limitation is that researchers could only collect data on sleep quality at select time points throughout the study. Some of the study’s strengths include the larger number of participants and length of follow-up duration.
Haghayegh emphasizes that these findings do not indicate causality. He wants to understand why this association exists and how treating one condition may also treat the other. In future clinical studies, he aims to investigate whether sleep medications could have a beneficial effect on blood pressure.
“I hope these findings further underscore the crucial role of quality sleep in our overall well-being. The American Academy of Sleep Medicine recommends sleeping seven or more hours a night, and if you cannot fall or stay asleep, it might be worth exploring why that is,” said Haghayegh. “This study highlights yet another reason why getting a good night’s sleep is so important.”
Co-authors on the paper include Susanne Strohmaier, Rikuta Hamaya, A. Heather Eliassen, Walter C. Willet, and Eric B. Rimm. The senior author of the paper is Eva Schernhammer of the Channing Division of Network Medicine.
This study was supported by the National Institutes of Health (grant numbers UM1CA186107, U01 CA176726, P01CA87969, U01 HL145386, P30 ES000002, R01 CA163451, and R01 HD101101) and by funding from the European Research Council under the European Union’s Horizon 2020 research and innovation program (grant agreement numbers 101053225).
Share this article
You might like.
Scientists cautiously optimistic about trial results of new preventative treatment, prospects for new phase in battle with deadly virus
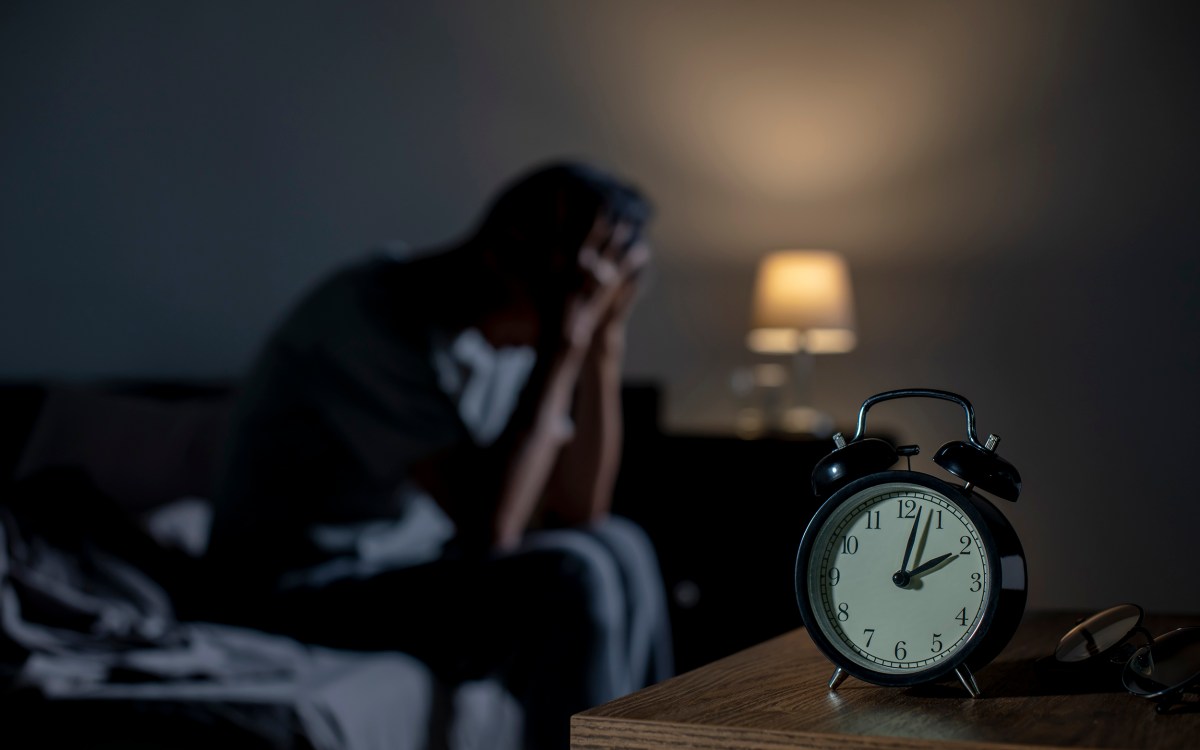
Study links irregular sleep patterns with higher disease risk
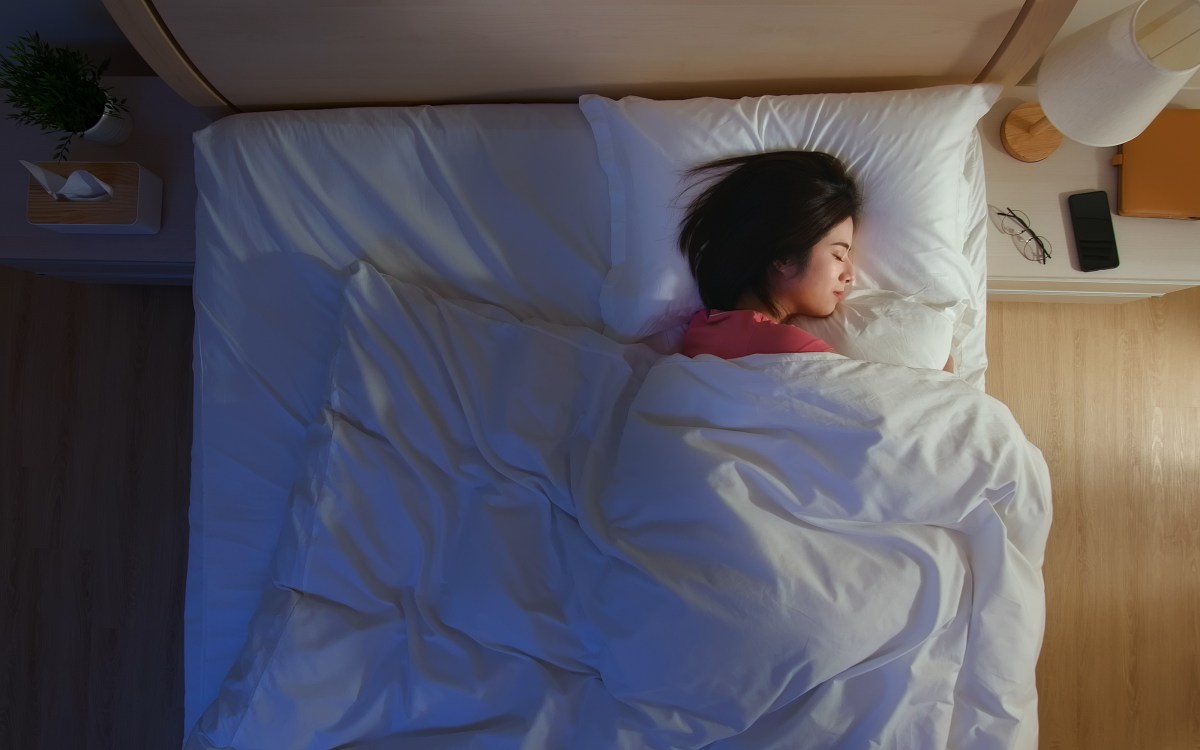
Researchers outline path to lower risk of depression
The way forward for Democrats — and the country
Danielle Allen is more worried about identity politics and gaps in civic education than the power of delegates
Good genes are nice, but joy is better
Harvard study, almost 80 years old, has proved that embracing community helps us live longer, and be happier
- How to Fall Asleep Fast: Tips to Get to Sleep Quickly
- How to Sleep Better: Tips to Improve Sleep Quality
- Sleep and Aging: Sleep Tips for Older Adults
- Parasomnias: Types, Causes, and Symptoms
Sleep Statistics
- Sleep Deprivation: Symptoms, Causes, and Effects
The Stages of Sleep: REM and Non-REM Sleep Cycles
Medical causes of sleep problems.
- Online Therapy: Is it Right for You?
- Mental Health
- Health & Wellness
- Children & Family
- Relationships
Are you or someone you know in crisis?
- Bipolar Disorder
- Eating Disorders
- Grief & Loss
- Personality Disorders
- PTSD & Trauma
- Schizophrenia
- Therapy & Medication
- Exercise & Fitness
- Healthy Eating
- Well-being & Happiness
- Weight Loss
- Work & Career
- Illness & Disability
- Heart Health
- Learning Disabilities
- Family Caregiving
- Teen Issues
- Communication
- Emotional Intelligence
- Love & Friendship
- Domestic Abuse
- Healthy Aging
- Alzheimer’s Disease & Dementia
- End of Life
- Meet Our Team
What is insomnia?
Symptoms of insomnia.
- Why can't I sleep? Causes of insomnia
Habits that cause insomnia and disrupt sleep
Treating insomnia with a better sleep environment and routine, insomnia and anxiety, what to do when insomnia wakes you up in the middle of the night, sleep supplements and medication for insomnia, when to see a doctor about insomnia, insomnia causes, symptoms & cures what to do when you can't sleep.
Can’t sleep? If you struggle to fall asleep or stay asleep at night, it can take a huge toll on your health. Here’s how to beat insomnia and end the sleepless nights.
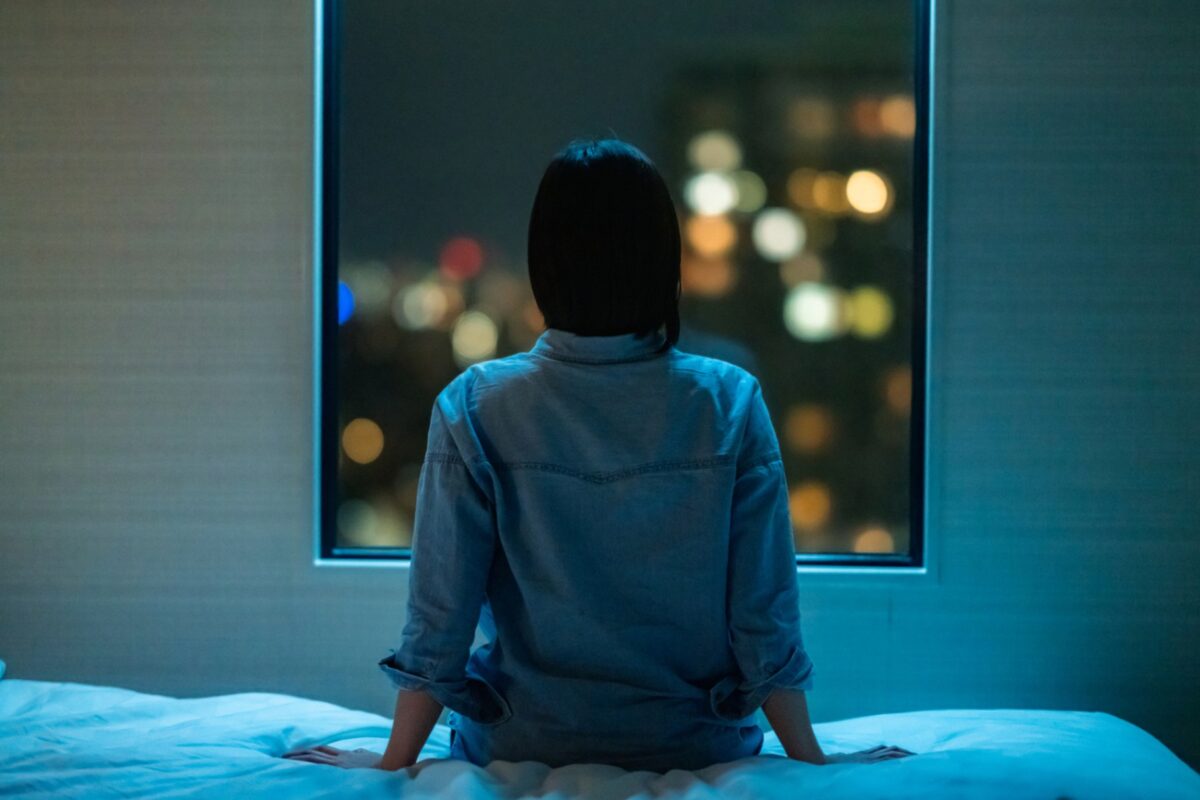
Insomnia is the inability to fall asleep or stay asleep at night, resulting in unrefreshing or non-restorative sleep. And it’s a very common problem, one that takes a toll on your energy, mood, and ability to function during the day. Chronic insomnia can even contribute to serious health problems.
Some people struggle to get to sleep no matter how tired they are. Others wake up in the middle of the night and lie awake for hours, anxiously watching the clock. But, because different people need different amounts of sleep, insomnia is defined by the quality of your sleep and how you feel after sleeping—not the number of hours you sleep or how quickly you doze off. Even if you’re spending eight hours a night in bed, if you feel drowsy and fatigued during the day, you may be experiencing insomnia.
Although insomnia is the most common sleep complaint, it is not a single sleep disorder . It’s more accurate to think of it as a symptom of another problem, whether it’s something as simple as drinking too much caffeine during the day or something more complex like feeling overloaded with stress.
The good news is that most cases of insomnia can be cured with changes you can make on your own—without relying on sleep specialists or turning to prescription or over-the-counter sleeping pills. By addressing the underlying causes and making simple changes to your daily habits and sleep environment , you can put a stop to the frustration of insomnia and finally get a good night’s sleep.
If you’re having trouble sleeping right now …
Try listening to HelpGuide’s Sleep Meditation Using Guided Imagery or Deep Breathing Meditation .
These include:
- Difficulty falling asleep despite being tired.
- Waking up frequently during the night.
- Trouble getting back to sleep when awakened.
- Unrefreshing sleep.
- Relying on sleeping pills or alcohol to fall asleep.
- Waking up too early in the morning.
- Daytime drowsiness, fatigue, or irritability.
- Difficulty concentrating during the day.
Why can’t I sleep? Causes of insomnia
In order to properly treat and cure your insomnia, you need to become a sleep detective. Emotional issues such as stress, anxiety, and depression cause half of all insomnia cases. But your daytime habits, sleep routine, and physical health may also play a role. Try to identify all possible causes of your insomnia. Once you figure out the root cause, you can tailor treatment accordingly.
What’s causing your insomnia?
- Are you under a lot of stress ?
- Are you depressed ? Do you feel emotionally flat or hopeless?
- Do you struggle with chronic feelings of anxiety or worry ?
- Have you recently gone through a traumatic experience ?
- Are you taking any medications that might be affecting your sleep?
- Do you have any health problems that may be interfering with sleep ?
- Is your bedroom quiet and comfortable?
- Do you try to go to bed and get up around the same time every day?
Common psychological and medical causes of insomnia
Sometimes, insomnia only lasts a few days and goes away on its own, especially when it is tied to an obviously temporary cause, such as stress over an upcoming presentation, a painful breakup, or jet lag. Other times, insomnia is stubbornly persistent. Chronic insomnia is usually tied to an underlying mental or physical issue.
Anxiety, stress, and depression are some of the most common causes of chronic insomnia. Having difficulty sleeping can also make anxiety , stress, and depression symptoms worse. Other common emotional and psychological causes include anger , worry, grief , bipolar disorder , and trauma . Treating these underlying problems is essential to resolving your insomnia.
Speak to a Licensed Therapist
BetterHelp is an online therapy service that matches you to licensed, accredited therapists who can help with depression, anxiety, relationships, and more. Take the assessment and get matched with a therapist in as little as 48 hours.
Medical problems or illness. Many medical conditions and diseases can contribute to insomnia , including asthma, allergies, Parkinson’s disease , hyperthyroidism, acid reflux, kidney disease, and cancer. Chronic pain is also a common cause of insomnia.
Medications. Many prescription drugs can interfere with sleep, including antidepressants , stimulants for ADHD , corticosteroids, thyroid hormone, high blood pressure medications, and some contraceptives. Common over-the-counter culprits include cold and flu medications that contain alcohol, pain relievers that contain caffeine (Midol, Excedrin), diuretics, and slimming pills.
Sleep disorders. Insomnia is itself a sleep disorder, but it can also be a symptom of other sleep disorders , including sleep apnea , restless legs syndrome , and circadian rhythm disturbances tied to jet lag or late-night shift work.
While treating underlying physical and mental issues is a good first step, it may not be enough to cure your insomnia. You also need to look at your daily habits. Some of the things you’re doing to cope with insomnia may actually be making the problem worse.
For example, maybe you’re using sleeping pills or alcohol to fall asleep , which disrupts sleep even more over the long-term. Or maybe you drink excessive amounts of coffee during the day, making it harder to fall asleep later. Other daytime habits that can negatively impact your ability to sleep at night include having an irregular sleep schedule, napping, eating sugary foods or heavy meals too close to bedtime, and not getting enough exercise or exercising too late in the day.
Not only can poor daytime habits contribute to insomnia, but a poor night’s sleep can make these habits harder to correct, creating a vicious cycle of unrefreshing sleep:
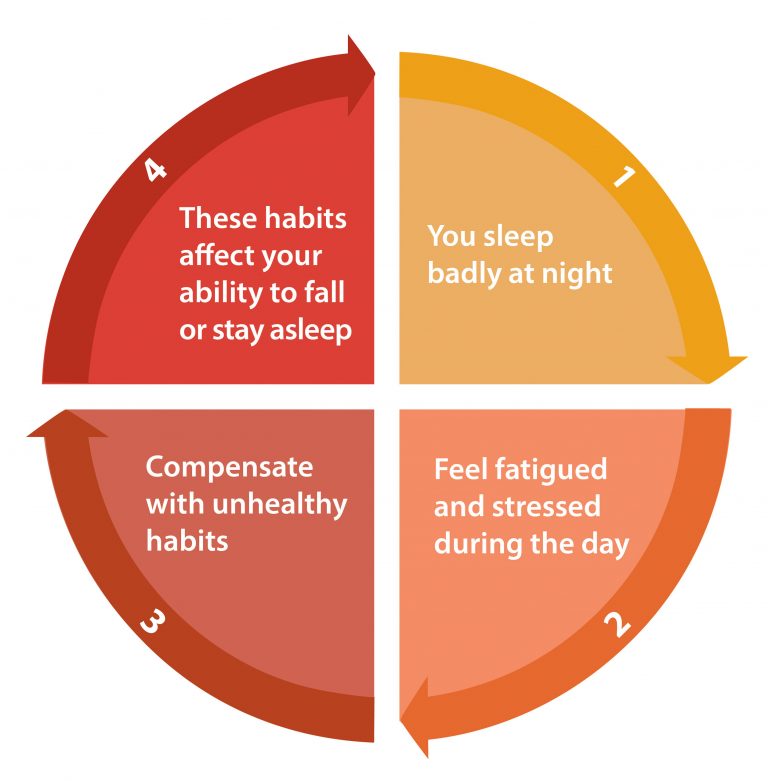
Oftentimes, changing the habits that are reinforcing sleeplessness is enough to overcome the insomnia altogether. It may take a few days for your body to get used to the change, but once you do, you’ll sleep better.
If you’re having trouble identifying insomnia-causing habits
Some habits are so ingrained that you may overlook them as a possible contributor to your insomnia. Maybe your Starbucks habit affects your sleep more than you realize. Or maybe you’ve never made the connection between that late-night glass of wine and your sleep difficulties.
Keeping a sleep diary (or using a sleep tracking app) is a helpful way to pinpoint habits and behaviors contributing to your insomnia.
Two powerful weapons in the fight against insomnia are a quiet, comfortable bedroom and a relaxing bedtime routine. Both can make a big difference in improving the quality of your sleep .
Make sure your bedroom is quiet, dark, and cool. Noise, light, a bedroom that’s too hot or cold, or an uncomfortable mattress or pillow can all interfere with sleep. Try using a sound machine or earplugs to mask outside noise, an open window or fan to keep the room cool, and blackout curtains or an eye mask to block out light. Experiment with different levels of mattress firmness , foam toppers, and pillows that provide the support you need to sleep comfortably.
[Read: How to Sleep Better]
Stick to a regular sleep schedule. Support your biological clock by going to bed and getting up at the same time every day, including weekends. Get up at your usual time in the morning even if you’re tired. This will help you get back in a regular sleep rhythm.
Turn off all screens at least an hour before bed. Electronic screens emit a blue light that disrupts your body’s production of melatonin and combats sleepiness. So instead of watching TV or spending time on your phone, tablet, or computer, choose another relaxing activity, such as reading a book or listening to soft music.
Avoid stimulating activity and stressful situations before bedtime. This includes checking messages on social media , big discussions or arguments with your spouse or family, or catching up on work. Postpone these things until the morning.
Avoid naps. Napping during the day can make it more difficult to sleep at night. If you feel like you have to take a nap, limit it to 30 minutes before 3 p.m.
Things to avoid before bed:
Drinking too many liquids. Waking up at night to go to the bathroom becomes a bigger problem as we age. By not drinking anything an hour before sleep and going to the bathroom several times as you get ready for bed, you can reduce the frequency you’ll wake up to go during the night.
Alcohol . While a nightcap may help you to relax and fall asleep, it interferes with your sleep cycle once you’re out, causing you to wake up during the night.
Big evening meals . Try to eat dinner earlier in the evening, and avoid heavy, rich foods within two hours of going to bed. Spicy or acidic foods can cause stomach trouble and heartburn which can wake you during the night.
Caffeine . The American Academy of Sleep Medicine recommends that you stop drinking caffeinated beverages at least six hours before bedtime. People who are sensitive to caffeine may need to stop even earlier.
The more trouble you have with sleep, the more it starts to invade your thoughts. You may dread going to sleep because you just know that you’re going to toss and turn for hours or wake up at 2 a.m. again. Or maybe you’re worried because you have a big day tomorrow, and if you don’t get a solid 8 hours, you’re sure you’ll blow it?
Agonizing and expecting sleep difficulties only makes insomnia worse. Worrying about getting to sleep or how tired you’re going to be floods your body with adrenaline, and before you know it, you’re wide-awake.
Relieving anxiety that keeps you from falling or staying asleep
If sleep worries are getting in the way of your ability to unwind at night, the following strategies may help. The goal is to train your body to associate the bed with sleep and nothing else—especially not frustration and anxiety.
Use the bedroom only for sleeping and sex. With many of us working from home now, it can be difficult to avoid, but if possible don’t work, use your computer, or watch TV in your bedroom. The goal is to associate the bedroom with sleep alone, so that your brain and body get a strong signal that it’s time to nod off when you get into bed.
Move bedroom clocks out of view. Anxiously watching the minutes tick by when you can’t sleep—knowing that you’re going to be exhausted when the alarm goes off—is a surefire recipe for insomnia. You can use an alarm, but make sure you can’t see the time when you’re in bed.
Get out of bed when you can’t sleep. Don’t try to force yourself to sleep. Tossing and turning only amps up your anxiety. Get up, leave the bedroom, and do something relaxing, such as reading, meditating , or taking a bath. When you’re sleepy, go back to bed.
Challenging the worries and thoughts that fuel insomnia
It’s also helpful to challenge the negative attitudes about sleep and your insomnia problem that you’ve developed over time. The key is to recognize self-defeating thoughts and replace them with more realistic ones.
[Read: How to Stop Worrying]
Challenging self-defeating thoughts that fuel insomnia
Self-defeating thought: | Sleep-promoting comeback: |
---|---|
I should be able to sleep well every night like a normal person. I shouldn’t have a problem! | Lots of people struggle with sleep from time to time. I will be able to sleep with the right techniques. |
It’s the same every single night, another night of sleepless misery. | Not every night is the same. Some nights I do sleep better than others. |
If I don’t get some sleep, I’ll tank my presentation and jeopardize my job. | I can get through the presentation even if I’m tired. I can still rest and relax tonight, even if I can’t sleep. |
I’m never going to be able to sleep well. It’s out of my control. | Insomnia can be cured. If I stop worrying so much and focus on positive solutions, I can beat it. |
It’s going to take me at least an hour to get to sleep tonight. I just know it. | I don’t know what will happen tonight. Maybe I’ll get to sleep quickly if I use the strategies I’ve learned. |
Remember, learning how to stop worrying takes time and practice. You may find it helpful to jot down your own list, taking note of the negative thoughts that pop up and how you can dispute them. You may be surprised at how often these negative thoughts run through your head. Be patient and ask for support if you need it.
Many people with insomnia are able to fall asleep fast at bedtime, but then wake up in the middle of the night. They then struggle to get back to sleep, often lying awake for hours. If this describes you, the following tips may help.
Stay out of your head. Hard as it may be, try not to stress over your inability to fall back to sleep, because that stress only encourages your body to stay awake. To stay out of your head, focus on the feelings in your body or practice breathing exercises. Take a breath in, then breathe out slowly while saying or thinking the word, “Ahhh.” Take another breath and repeat.
Make relaxation your goal, not sleep. If you find it hard to fall back to sleep, try a relaxation technique such as visualization, progressive muscle relaxation, or meditation, which can be done without even getting out of bed. Even though it’s not a replacement for sleep, relaxation can still help rejuvenate your mind and body.
[Listen: Progressive Muscle Relaxation]
Do a quiet, non-stimulating activity. If you’ve been awake for more than 20 minutes, get out of bed and do a quiet, non-stimulating activity, such as reading a book. Keep the lights dim and avoid screens so as not to cue your body that it’s time to wake up.
Postpone worrying and brainstorming. If you wake during the night feeling anxious about something, make a brief note of it on paper and postpone worrying about it until the next day when it will be easier to resolve. Similarly, if a great idea is keeping you awake, make a note of it on paper and fall back to sleep knowing you’ll be much more productive after a good night’s rest.
Relaxation techniques that can help you get back to sleep
Abdominal breathing. Breathing deeply and fully, involving not only the chest, but also the belly, lower back, and ribcage, can help relaxation. Close your eyes and take deep, slow breaths, making each breath even deeper than the last. Breathe in through your nose and out through your mouth.
Progressive muscle relaxation . Make yourself comfortable. Starting with your feet, tense the muscles as tightly as you can. Hold for a count of 10, and then relax. Continue to do this for every muscle group in your body, working your way up from your feet to the top of your head.
Mindfulness meditation . Sit or lie quietly and focus on your natural breathing and how your body feels in the moment. Allow thoughts and emotions to come and go without judgment, always returning to focus on breath and your body.
These audio meditations can help.
When you’re tossing and turning at night, it can be tempting to turn to sleep aids for relief. But before you do, here’s what you need to know.
Dietary supplements for insomnia
There are many dietary and herbal supplements marketed for their sleep-promoting effects. Although they may be described as “natural,” be aware that sleep remedies can still have side effects and interfere with other medications or vitamins you’re taking. For more information, talk to your doctor or pharmacist.
[Read: Sleeping Pills and Natural Sleep Aids]
While scientific evidence is still being gathered for alternative sleep remedies, you might find that some of them work wonderfully for you. The two supplements with the most evidence supporting their effectiveness for insomnia are melatonin and valerian.
- Melatonin. Melatonin is a naturally occurring hormone that your body produces at night. Melatonin helps regulate your sleep-wake cycle. Melatonin is also available as an over-the-counter supplement. While melatonin doesn’t work for everyone, it may be an effective insomnia treatment for you—especially if you’re an extreme “night owl” with a natural tendency to go to bed and get up much later than others.
- Valerian. Valerian is an herb with mild sedative effects that may help you sleep better. However, the quality of valerian supplements varies widely.
Prescription sleeping pills
While prescription sleeping pills can provide temporary relief, it’s important to understand that sleep medications are not a cure for insomnia. And if not used carefully, they actually make insomnia worse in the long run. It’s best to use medication only as a last resort, and then, only on a very limited, as-needed basis.
First, try changing your sleep habits, your daily routine, and your attitudes about sleep. Evidence shows that lifestyle and behavioral changes make the largest and most lasting difference when it comes to insomnia.
If you’ve tried a variety of self-help techniques without success, schedule an appointment with a sleep specialist, especially if insomnia is taking a heavy toll on your mood and health. Provide the doctor with as much supporting information as possible, including information from your sleep diary.
Therapy vs. sleeping pills for insomnia
In general, sleeping pills and sleep aids are most effective when used sparingly for short-term situations, such as traveling across time zones or recovering from a medical procedure. Your insomnia won’t be cured by sleeping pills—in fact, over the long-term they can actually make insomnia worse.
Since many people complain that frustrating, negative thoughts and worries prevent them from sleeping at night, cognitive-behavioral therapy (CBT) can be much more effective in addressing insomnia. CBT is a form of psychotherapy that treats problems by modifying negative thoughts, emotions, and patterns of behavior. It can be conducted individually, in a group, or even online . A study at Harvard Medical School found that CBT was more effective at treating chronic insomnia than prescription sleep medication—but without the risks or side effects.
More Information
- Sleep-Wake Disorders. (2013). In Diagnostic and Statistical Manual of Mental Disorders . American Psychiatric Association. Link
- Blue light has a dark side—Harvard Health . (n.d.). Retrieved April 7, 2022, from Link
- Britton, A., Fat, L. N., & Neligan, A. (2020). The association between alcohol consumption and sleep disorders among older people in the general population. Scientific Reports , 10(1), 5275. Link
- Drake, C., Roehrs, T., Shambroom, J., & Roth, T. (2013). Caffeine Effects on Sleep Taken 0, 3, or 6 Hours before Going to Bed. Journal of Clinical Sleep Medicine , 09(11), 1195–1200. Link
- Ferracioli-Oda, E., Qawasmi, A., & Bloch, M. H. (2013). Meta-Analysis: Melatonin for the Treatment of Primary Sleep Disorders. PLoS ONE , 8(5), e63773. Link
- Fitzgerald, T., & Vietri, J. (2015). Residual Effects of Sleep Medications Are Commonly Reported and Associated with Impaired Patient-Reported Outcomes among Insomnia Patients in the United States. Sleep Disorders , 2015, 1–9. Link
- Green, A., Cohen-Zion, M., Haim, A., & Dagan, Y. (2017). Evening light exposure to computer screens disrupts human sleep, biological rhythms, and attention abilities. Chronobiology International , 34(7), 855–865. Link
- Mitchell, M. D., Gehrman, P., Perlis, M., & Umscheid, C. A. (2012). Comparative effectiveness of cognitive behavioral therapy for insomnia: A systematic review. BMC Family Practice , 13(1), 40. Link
- Ohayon, M. M., & Roth, T. (2001). What are the contributing factors for insomnia in the general population? Journal of Psychosomatic Research , 51(6), 745–755. Link
- Valerian—Health Professional Fact Sheet . (n.d.). Retrieved April 7, 2022, from Link
More in Sleep
How to fall asleep fast.
Tips for getting to sleep quickly
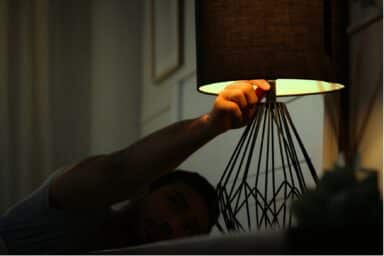
How to Sleep Better
Tips to improve sleep quality
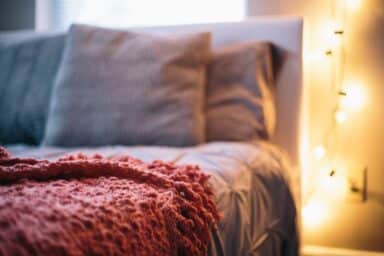
Sleep and Aging
Tips for overcoming insomnia and other age-related sleep problems
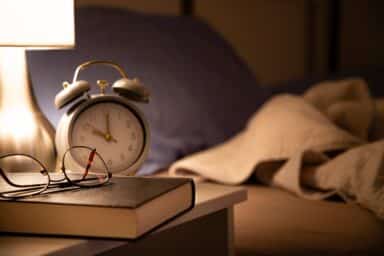
Parasomnias
Types, causes, and symptoms
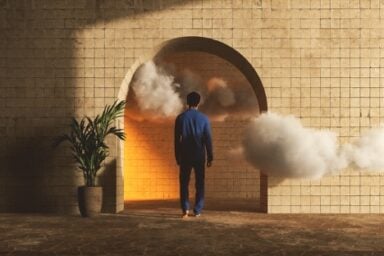
How sleep impacts health and well-being
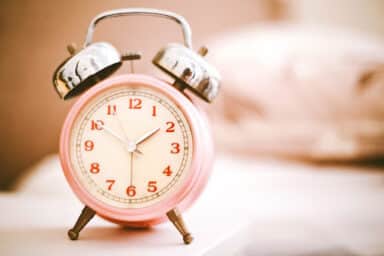
Sleep Deprivation
How lack of sleep can damage your health
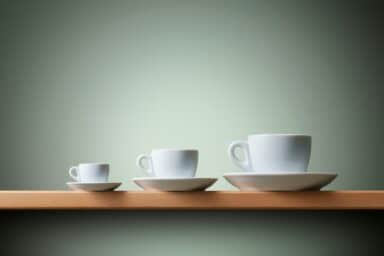
Circadian rhythms, sleep stages, and sleep architecture
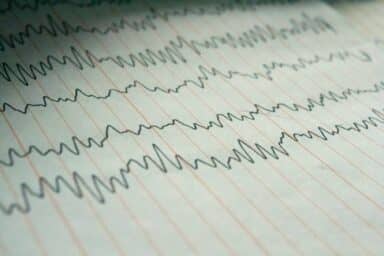
There may be a medical reason for your sleepless nights
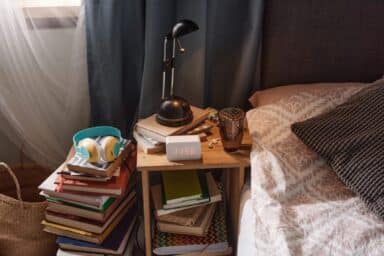
Professional therapy, done online
BetterHelp makes starting therapy easy. Take the assessment and get matched with a professional, licensed therapist.
Help us help others
Millions of readers rely on HelpGuide.org for free, evidence-based resources to understand and navigate mental health challenges. Please donate today to help us save, support, and change lives.
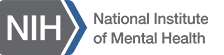
Transforming the understanding and treatment of mental illnesses.
Información en español
Celebrating 75 Years! Learn More >>
- Science News
- Meetings and Events
- Social Media
- Press Resources
- Email Updates
- Innovation Speaker Series
Day Two: Placebo Workshop: Translational Research Domains and Key Questions
July 11, 2024
July 12, 2024
Day 1 Recap and Day 2 Overview
ERIN KING: All right. It is 12:01 so we'll go ahead and get started. And so on behalf of the Co-Chairs and the NIMH Planning Committee, I'd like to welcome you back to day two of the NIMH Placebo Workshop, Translational Research Domains and Key Questions. Before we begin, I will just go over our housekeeping items again. So attendees have been entered into the workshop in listen-only mode with cameras disabled. You can submit your questions via the Q&A box at any time during the presentation. And be sure to address your question to the speaker that you would like to respond.
For more information on today's speakers, their biographies can be found on the event registration website. If you have technical difficulties hearing or viewing the workshop, please note these in the Q&A box and our technicians will work to fix the problem. And you can also send an e-mail to [email protected]. And we'll put that e-mail address in the chat box for you. This workshop will be recorded and posted to the NIMH event web page for later viewing.
Now I would like to turn it over to our workshop Co-Chair, Dr. Cristina Cusin, for today's introduction.
CRISTINA CUSIN: Thank you so much, Erin. Welcome, everybody. It's very exciting to be here for this event.
My job is to provide you a brief recap of day one and to introduce you to the speakers of day two. Let me share my slides.
Again, thank you to the amazing Planning Committee. Thanks to their effort, we think this is going to be a success. I learned a lot of new information and a lot of ideas for research proposals and research projects from day one. Very briefly, please go and watch the videos. They are going to be uploaded in a couple of weeks if you missed them.
But we had an introduction from Tor, my Co-Chair. We had an historic perspective on clinical trials from the industry regulatory perspective. We had the current state from the FDA on placebo.
We had an overview of how hard it is to sham, to provide the right sham for device-based trials, and the challenges for TMS. We have seen some new data on the current state of placebo in psychosocial trials and what is the equivalent of a placebo pill for psychosocial trials. And some social neuroscience approach to placebo analgesia. We have come a long way from snake oil and we are trying to figure out what is placebo.
Tor, my Co-Chair, presented some data on the neurocircuitry underlying placebo effect and the questions that how placebo is a mixture of different elements including regression to the mean, sampling bias, selective attrition for human studies, the natural history of illness, the placebo effect per se that can be related to expectations, context, learning, interpretation.
We have seen a little bit of how is the impact on clinical trial design and how do we know that something, it really works. Or whatever this "it" is. And why do even placebo effect exists? It's fascinating idea that placebo exists as a predictive control to anticipate threats and the opportunity to respond in advance and to provide causal inference, a construct perception to infer the underlying state of body and of world.
We have seen historical perspective. And Ni Aye Khin and Mike Detke provided some overview of 25 years of randomized control trials from the data mining in major depressive disorders, schizophrenia trials and the lessons we have learned.
We have seen some strategies, both historical strategies and novel strategies to decrease placebo response in clinical trials and the results. Start from trial design, SPCD, lead-in, placebo phase and flexible dosing. Use of different scales. The use of statistical approaches like last observation carried forward or MMRM. Centralized ratings, self-rating, computer rating for different assessments. And more issues in clinical trials related to patient selection and professional patients.
Last, but not least, the dream of finding biomarkers for psychiatric conditions and tying response, clinical response to biomarkers. And we have seen how difficult it is to compare more recent studies with studies that were started in the '90s.
We have the FDA perspective with Tiffany Farchione in this placebo being a huge issue from the FDA. Especially the discussion towards the end of the day was on how to blind psychedelics.
We have seen an increasing placebo response rate in randomized controlled trials, also in adolescents, that is. And the considerations from the FDA of novel design models in collaboration with industry. We had examples of drugs approved for other disorders, not psychiatric condition, and realized -- made me realize how little we know about the true pathophysiology of psychiatric disorders, likely also heterogeneous conditions.
It made me very jealous of other fields because they have objective measures. They have biology, they have histology, they have imaging, they have lab values. While we are -- we are far behind, and we are not really able to explain to our patients why our mitigations are supposed to work or how they really work.
We heard from Holly Lisanby and Zhi-De Deng. The sham, the difficulty in producing the right sham for each type of device because most of them have auxiliary effects that are separate from the clinical effect like the noise or the scalp stimulation for TMS.
And it's critical to obtain a true blinding and separating sham from verum. We have seen how in clinical trials for devices expectancy from the patient, high tech environment and prolonged contact with clinicians and staff may play a role. And we have seen how difficult it is to develop the best possible sham for TMS studies in tDCS. It's really complicated and it's so difficult also to compare published studies in meta-analysis because they've used very different type of sham. Not all sham are created equal. And some of them could have been biologically active, so therefore invalidating the result or making the study uninformative.
Then we moved on to another fascinating topic with Dr. Rief and Dr. Atlas. What is the impact of psychological factors when you're studying a psychological intervention. Expectations, specific or nonspecific factors in clinical trials and what is interaction between those factors.
More, we learned about the potential nocebo effect of standard medical care or being on a wait list versus being in the active arm of a psychotherapy trial. And the fact that we are not accurately measuring the side effect of psychotherapy trial itself. And we heard more a fascinating talk about the neurocircuitry mediating placebo effect -- salience, affective value, cognitive control. And how perception of provider, perception of his or her warmth and competence and other social factors can affect response and placebo response, induce bias in evaluation of acute pain of others. Another very interesting field of study.
From a clinician perspective, this is -- and from someone who conduct clinical trials, all this was extremely informative because in many case in our patient situation no matter how good the treatment is, they have severe psychosocial stressors. They have difficulties to accessing food, to access treatment, transportation, or they live in an extremely stressful environment. So to disentangle other psychosocial factors from the treatment, from the biology is going to be critical to figure out how to treat best our patients.
And there is so much more work to do. Each of us approach the placebo topic for research from a different perspective. And like the blind man trying to understand what is an elephant, we have to endure it, we have to talk to each other, we have to collaborate and understand better the underlying biology, understand different aspect of the placebo phenomena.
And this lead us to the overview for day two. We are going to hear more about other topic that are so exciting. The placebo, the nocebo effect and other predictive factors in laboratory setting. We are going to hear about genetic of the placebo response to clinical trials. More physiological and psychological and neuromechanism for analgesia. And after a brief break around 1:30, we are going to hear about novel biological and behavioral approaches for the placebo effect.
We are going to hear about brain mapping. We are going to hear about other findings from imaging. And we're going to hear about preclinical modeling. There were some questions yesterday about animal models of placebo. And last, but not least, please stay around because in the panel discussion, we are going to tackle some of your questions. And we are going to have two wonderful moderators, Ted Kaptchuk and Matthew Rudorfer. So please stay with us and ask questions. We love to see more challenges for our speakers. And we're going to be all of the panelists from yesterday, from today are going to be present. Thank you so much.
Now we're going to move on to our first speaker of the day. If I am correct according to the last -- Luana.
Measuring & Mitigating the Placebo Effect
LUANA COLLOCA: Thank you very much, Cristina. First, I would love to thank the organizer. This is a very exciting opportunity to place our awareness about this important phenomenon for clinical trials and the clinical practice.
And today, I wish to give you a very brief overview of the psychoneurobiological mechanism of a placebo and nocebo, the description of some pharmacological studies, and a little bit of information on social learning. That is a topic that has been mentioned a little bit yesterday. And finally, the translational part. Can we translate what we learn from mechanistic approach to placebo and nocebo in terms of a disease and symptomatology and eventually predictors is the bigger question.
So we learned yesterday that placebo effects are generated by verbal suggestion, this medication has strong antidepressant effects. Therapeutic prior experience, merely taking a medication weeks, days before being substitute with a simulation of placebo sham treatment. Observation of a benefit in other people, contextual and treatment cue, and interpersonal interactions.
Especially in the fields of pain where we can simulate nociception, painful experience in laboratory setting, we learn a lot about the modulation related to placebo. In particular, expectation can provide a reaction and activation of parts of the brain like frontal area, nucleus accumbens, ventral striatum. And this kind of mechanism can generate a descending stimulation to make the painful nociceptive stimulus less intense.
The experience of analgesia at the level of a pain mechanism translate into a modulation reduction of a pain intensity. But most important, pain unpleasantness and the effective components of the pain. I will show today some information about the psychological factor, the demographic factor as well as genetic factors that can be predictive of placebo effects in the context of a pain.
On the other hand, a growing interest is related to nocebo effects, the negative counter sides of this phenomenon. When we talk about nocebo effects, we refer to increase in worsening of outcome in symptoms related to negative expectation, prior negative therapeutic experience, observing a negative outcome in others, or even mass psychogenic modeling such as some nocebo-related response during the pandemic. Treatment leaflets, the description of all side effects related to a medication. Patient-clinician communication. The informed consent where we list all of the side effects of a procedure or medication as well as contextual cues in clinical encounters.
And importantly, internal factor like emotion, mood, maladaptive cognitive appraisal, negative valence, personality traits, somatosensory features and omics can be predictive of negative worsening of symptom and outcome related to placebo and nocebo effects. In terms of a nocebo very briefly, there is a lot of attention again related to brain imaging with beautiful data show that the brainstem, the spinal cord, the hippocampus play a critical role during nocebo hyperalgesic effects.
And importantly, we learn that about placebo and nocebo through different approach including brain imaging, as we saw yesterday, but also pharmacological approach. We start from realizing that placebo effects are really neurobiological effects with the use of agonist or antagonist.
In other words, we can use a drug to mimic the action of that drug when we replace the drug with a saline solution, for example. In the cartoon here, you can see a brief pharmacological conditioning with apomorphine. Apomorphine is a dopamine agonist. And after three days of administration, apomorphine was replaced with saline solution in the intraoperative room to allow us to understand if we can mimic at the level of neuronal response the effects of apomorphine.
So in brief these are patients undergoing subthalamic EEG installation of deep brain stimulation. You can see here reaching the subthalamic nucleus. So after crossing the thalamus, the zona incerta, the STN, and the substantia nigra, the surgeon localized the area of stimulation. Because we have two subthalamic nuclei, we can use one as control and the other one as target to study in this case the effects of saline solution given after three days of apomorphine.
What we found was in those people who respond, there was consistency in reduction of clinical symptoms. As you can see here, the UPDRS, a common scale to measure rigidity in Parkinson, the frequency of a discharge at the level of neurons and the self-perception, patients with sentences like I feel like after Levodopa, I feel good. This feeling good translate in less rigidity, less tremor in the surgical room.
On the other hand, some participants didn't respond. Consistently we found no clinical improvement, no difference in preference over this drug at the level of a single unit and no set perception of a benefit. This kind of effects started to trigger the questions what is the reason why some people who responded to placebo and pharmacological conditioning and some other people don't. I will try to address this question in the second part of my talk.
On the other hand, we learn a lot about the endogenous modulation of pain and true placebo effects by using in this case an antagonist. The goal in this experiment was to create a painful sensation through a tourniquet. Week one with no treatment. Week two we pre-inject healthy participant with morphine. Week three the same morphine. And week four we replace morphine with placebo.
And you can see that a placebo increase the pain tolerance in terms of imminent. And this was not carryover effects. In fact, the control at week five showed no differences. Part of the participants were pre-injected with an antagonist Naloxone that when we use Naloxone at high dose, we can block the opioids delta and K receptors. You can see that by pre-injecting Naloxone there is a blockage of placebo analgesia, and I would say this morphine-like effects related to placebo given after morphine.
We start to then consider this phenomenon. Is this a way for tapering opioids. And we called this sort of drug-like effects as dose-extending placebo. The idea is that if we use a pharmacological treatment, morphine, apomorphine, as I showed to you, and then we replace the treatment with a placebo, we can create a pharmacological memory, and this can translate into a clinical benefit. Therefore, the dose-extending placebo can be used to extend the benefit of the drug, but also to reduce side effects related to the active drug.
In particular for placebo given after morphine, you can see on this graph, the effects is similarly strong if we do the repetition of a morphine one day apart or one week apart. Interestingly, this is the best model to be used in animal research.
Here at University of Maryland in collaboration with Todd Degotte, we create a model of anhedonia in mice and we condition animals with Ketamine. The goal was to replace Ketamine with a placebo. There are several control as you can see. But what is important for us, we condition animal with Ketamine week one, three and five. And then we substitute Ketamine with saline along with the CS. The condition of the stimulus was a light, a low light. And we want to compare this with an injection of Ketamine given at week seven.
So as you can see here, of course Ketamine was inducing a benefit as compared to saline and the Ketamine. But what is seen testing when we compare Ketamine week seven with saline replacing the Ketamine, we found no difference; suggesting that even in animals, in mice we were able to create drug-related effects. In this case, a Ketamine antidepressant-like placebo effects. These effects also add dimorphic effects in the sense that we observed this is in males but not in females.
So another approach to use agonist, like I mentioned for apomorphine in Parkinson patient, was to use vasopressin and oxytocin to increase placebo effects. In this case, we used verbal suggestion that in our experience especially with healthy participants tended to create very small sample size in terms of placebo analgesic effects. So we knew that from the literature that there is a dimorphic effects for this hormone. So we inject people with intranasal vasopressin, saline, oxytocin in low dose and no treatment. You can see there was a drug effects in women whereby vasopressin boost placebo analgesic effects, but not in men where yet we found many effects of manipulation but not drug effects.
Importantly, vasopressin affect dispositional anxiety as well as cortisol. And there is a negative correlation between anxiety and cortisol in relationship to vasopressin-induced placebo analgesia.
Another was, can we use medication to study placebo in laboratory setting or can we study placebo and nocebo without any medication? One example is to use a manipulation of the intensity of the painful stimulations. We use a thermal stimulation tailored at three different levels. 80 out of 100 with a visual analog scale, 50 or 20, as you can see from the thermometer.
We also combined the level of pain with a face. So first to emphasize there is three level of pain, participants will see an anticipatory cue just before the thermal stimulation. Ten seconds of the thermal stimulation to provide the experience of analgesia with the green and the hyperalgesia with the red as compared to the control, the yellow condition.
Therefore, the next day we move in the fMRI. And the goal was to try to understand to what extent expectation is relevant in placebo and nocebo effects. We mismatch what they anticipate, and they learn the day before. But also you can see we tailored the intensity at the same identical level. 50 for each participant.
We found that when expectation matched the level of the cues, anticipatory cue and face, we found a strong nocebo effects and placebo effects. You can see in red that despite the level of pain were identical, the perceived red-related stimuli as higher in terms of intensity, and the green related the stimuli as lower when compared to the control. By mismatching what they expect with what they saw, we blocked completely placebo effects and still nocebo persist.
So then I showed to you that we can use conditioning in animals and in humans to create placebo effects. But also by suggestion, the example of vasopressin. Another important model to study placebo effects in laboratory setting is social observation. We see something in other people, we are not told what we are seeing and we don't experience the thermal stimulation. That is the setting. A demonstrator receiving painful or no painful stimulation and someone observing this stimulation.
When we tested the observers, you can see the level of pain were tailored at the same identical intensity. And these were the effects. In 2009, when we first launched this line of research, this was quite surprising. We didn't anticipate that merely observing someone else could boost the expectations and probably creating this long-lasting analgesic effect. This drove our attention to the brain mechanism of what is so important during this transfer of placebo analgesia.
So we scanned participants when they were observing a video this time. And a demonstrator receiving control and placebo cream. We counterbalance the color. We controlled for many variables. So during the observation of another person when they were not stimulated, they didn't receive the cream, there is an activation of the left and right temporoparietal junction and a different activation of the amygdala with the two creams. And importantly, an activation of the periaqueductal gray that I show to you is critical in modulating placebo analgesia.
Afterwards we put both the placebo creams with the two different color. We tailored the level of pain at the identical level of intensity. And we saw how placebo effects through observation are generated. They create strong different expectation and anxiety. And importantly, we found that the functional connectivity between the dorsolateral prefrontal cortex and temporoparietal junction that was active during the observation mediate the behavior results. Suggesting that there is some mechanism here that may be relevant to exploit in clinical trials and clinical practice.
From this, I wish to switch to a more translational approach. Can we replicate these results observed in health participant for nociception in people suffering from chronic pain. So we chose as population of facial pain that is an orphan disease that has no consensus on how to treat it, but also it affects the youngest including children.
So participants were coming to the lab. And thus you can see we used the same identical thermal stimulation, the same electrodes, the same conditioning that I showed to you. We measured expectation before and after the manipulation. The very first question was can we achieve similar monitored distribution of placebo analgesia in people suffering chronically from pain and comorbidities. You can see that we found no difference between temporo parenthala, between TMD and controls. Also, we observed that some people responded to the placebo manipulation with hyperalgesia. We call this nocebo effect.
Importantly, these affects are less relevant than the benefit that sometime can be extremely strong show that both health control and TMD. Because we run experiment in a very beautiful ecological environment where we are diverse, the lab, the experimenters as well as the population we recruit in the lab has a very good distribution of race, ethnicity.
So the very first question was we need to control for this factor. And this turned out to be a beautiful model to study race, ethnicity in the lab. So when chronic pain patient were studied by same experimenter race, dark blue, we observe a larger placebo effect. And this tell us about the disparity in medicine. In fact, we didn't see these effects in our controls.
In chronic pain patient, we also saw a sex concordance influence. But in the opposite sense in women studied by a man experimenter placebo effects are larger. Such an effect was not seen in men.
The other question that we had was what about the contribution of psychological factors. At that stage, there were many different survey used by different labs. Some are based on the different area of, you know, the states of the world, there were trends where in some people in some study they observe an effects of neurodisease, more positive and negative set, that refer to the words. Instead of progressing on single survey, and now we have a beautiful meta-analysis today that is not worth in the sense that it is not predictive of placebo effects.
We use the rogue model suggested by the NIMH. And by doing a sophisticated approach we were able to combine this into four balances. Emotional distress, reward-seeking, pain related fear catastrophizing, empathy and openness. These four valences then were interrelated to predict placebo effects. And you can see that emotional distress is associated with lower magnitude of placebo effects extinguishing over time and lower proportion of placebo responsivity.
Also people who tend to catastrophizing display lower magnitude of placebo effects. In terms of expectation, it is also interesting patients expect to benefit, they have this desire for a reward. But also those people who are more open and characterized by empathy tend for the larger expectations. But this doesn't translate necessarily in larger placebo effects, somehow hinting that the two phenomenon can be not necessarily linked.
Because we study chronic pain patients they come with their own baggage of disease comorbidities. And Dr. Wang in his department look at insomnia. Those people suffering from insomnia tends to have lower placebo analgesic effects along with those who have a poor pattern of sleep, suggesting that clinical factor can be relevant when we wish to predict placebo effects.
Another question that we address how simple SNPs, single nucleotide polymorphism variants in three regions that have been published can be predictive of placebo effects. In particular, I'm referring to OPRM1 that is linked to the gene for endogenous opioids. COMT linked to endogenous dopamine. And FAAH linked to endogenous cannabinoids. And we will learn about that more with the next talk.
And you can see that there is a prediction. These are rogue codes that can be interesting. We model all participants with verbal suggestion alone, the conditioning. There isn't really a huge difference between using one SNP versus two or three. What is truly impact and was stronger in terms of prediction was accounting for the procedure we used to study placebo. Whether by suggestion alone versus condition. When we added the manipulation, the prediction becomes stronger.
More recently, we started gene expression transcriptomic profile associated with placebo effects. We select from the 402 participants randomly 54. And we extract their transcriptomic profiles. Also we select a validation cohort to see if we can't replicate what we discover in terms of mRNA sequencing. But we found over 600 genes associated with the discovered cohort. In blue are the genes downregulated and in red upregulated.
We chose the top 20 genes and did the PCA to validate the top 20. And we found that six of them were replicated and they include all these genes that you see here. The Selenom for us was particularly interesting, as well as the PI3, the CCDC85B, FBXL15, HAGHL and the TNFRSF4. So with this --
LUANA COLLOCA: Yes, I'm done. With this, that is the goal probably one day with AI and other approach to combine clinical psychological brain imaging and so on, characteristic and behavior to predict a level of transitory response to placebo. That may guide us in clinical trials and clinical path to tailor the treatment. Therefore, the placebo and nocebo biological response can be to some extent predicted. And identify those who responded to placebo can help tailoring drug development and symptom management.
Thank you to my lab. All of you, the funding agencies. And finally, for those who like to read more about placebo, this book is available for free to be downloaded. And they include many of the speakers from this two-day event as contributors to this book. Thank you very much.
CRISTINA CUSIN: Thank you so much, Luana. It was a wonderful presentation. We have one question in the Q&A.
Elegant studies demonstrating powerful phenomena. Two questions. Is it possible to extend or sustain placebo-boosting effect? And what is the dose response relationship with placebo or nocebo?
LUANA COLLOCA: Great questions. The goal is to boost a placebo effects. And one way, as I showed was, for example, using intranasal vasopressin. But also extending relationship with placebo we know that we need the minimum of a three or four other administration before boosting this sort of pharmacological memory. And the longer is the administration of the active drug before we replace with placebo, the larger the placebo effects.
For nocebo, we show similar relationship with the collaborators. So again, the longer we condition, the stronger the placebo or nocebo effects. Thank you so much.
CRISTINA CUSIN: I wanted to ask, do you have any theory or interpretation about the potential for transmit to person a placebo response between the observer or such, do you have any interpretation of this phenomenon?
LUANA COLLOCA: It is not completely new in the literature. There is a lot of studies show that we can transfer pain in both animal models and humans.
So transfer analgesia is a natural continuation of that line of research. And the fact that we mimic things that we see in some other people, this is the very most basic form of learning when we grow up. But also from a revolutionary point of view protect us from predators and animals and us as human beings observing is a very good mechanism to boost behaviors and in this case placebo effects. Thank you.
CRISTINA CUSIN: Okay. We will have more time to ask questions.
We are going to move on to the next speaker. Dr. Kathryn Hall.
KATHRYN HALL: Thank you. Can you see my screen okay? Great.
So I'm going to build on Dr. Colloca's talk to really kind of give us a deeper dive into the genetics of the placebo response in clinical trials.
So I have no disclosures. So as we heard and as we have been hearing over the last two days, there is -- there are physiological drivers of placebo effects, whether they are opioid signaling or dopamine signaling. And these are potentiated by the administration or can be potentiated by saline pills, saline injections, sugar pills. And what's really interesting here, I think, is this discussion about how drugs impact the drivers of placebo response. In particular we heard about Naloxone yesterday and proglumide.
What I really want to do today is think about the next layer. Like how do the genes that shape our biology and really drive or influence that -- those physiological drivers of placebo response, how do the genes, A, modify our placebo response? But also, how are they modifying the effect of the drugs and the placebos on this basic -- this network?
And if you think about it, we really don't know much about all of the many interactions that are happening here. And I would actually argue that it goes even beyond genetic variation to other factors that lead to heterogeneity in clinical trials. Today I'm going to really focus on genes and variations in the genome.
So let's go back so we have the same terminology. I'm going to be talking about placebo-responsing trials. And so we saw this graph or a version of this graph yesterday where in clinical trials when we want to assess the effect of a drug, we subtract the outcomes in the placebo arm from the outcomes in the drug treatment arm. And there is a basic assumption here that the placebo response is additive to the drug response.
And what I want to do today is to really challenge that assumption. I want to challenge that expectation. Because I think we have enough literature and enough studies that have already been done that demonstrate that things are not as simple as that and that we might be missing a lot from this basic averaging and subtracting that we are doing.
So the placebo response is that -- is the bold lines there which includes placebo effects which we have been focusing on here. But it also includes a natural history of the disease or the condition, phenomenon such as statistical regression not mean, blinding and bias and Hawthorn effects. So we lump all of those together in the placebo arm of the trial and subtract the placebo response from the drug response to really understand the drug effect.
So one way to ask about, well, how do genes affect this is to look at candidate genes. And as Dr. Colloca pointed out and has done some very elegant studies in this area, genes like COMT, opioid receptors, genes like OPRM1, the FAAH endocannabinoid signaling genes are all candidate genes that we can look at in clinical trials and ask did these genes modify what we see in the placebo arm of trials?
We did some studies in COMT. And I want to just show you those to get a -- so you can get a sense of how genes can influence placebo outcomes. So COMT is catacholamethyl transferase. And it's a protein, an enzyme that metabolizes dopamine which as you saw is important in mediating the placebo response. COMT also metabolizes epinephrin, norepinephrine and catecholest estrogen. So the fact that COMT might be involved in placebo response is really interesting because it might be doing more than just metabolizing dopamine.
So we asked the question what happens if we look at COMT genetic variation in clinical trials of irritable bowel syndrome? And working with Ted Kaptchuk and Tony Lembo at Beth Israel Deaconess Medical Center, we did just that. We looked at COMT effects in a randomized clinical trial of irritable bowel syndrome. And what we did see was that for the gene polymorphism RS46AD we saw that people who had the weak version of the COMT enzyme actually had more placebo response. These are the met/met people here shown on this, in this -- by this arrow. And that the people who had less dopamine because that enzyme didn't work as well for this polymorphism, they had less of a placebo response in one of the treatment arms. And we would later replicate this study in another clinical trial that was recently concluded in 2021.
So to get a sense, as you can see, we are somewhat -- we started off being somewhat limited by what was available in the literature. And so we wanted to expand on that to say more about genes that might be associated with placebo response. So we went back, and we found 48 studies in the literature where there was a gene that was looked at that modified the placebo response.
And when we mapped those to the interactome, which is this constellation of all gene products and their interactions, their physical interactions, we saw that the placebome or the placebo module had certain very interesting characteristics. Two of those characteristics that I think are relevant here today are that they overlapped with the targets of drugs, whether they were analgesics, antidepressive drugs, anti-Parkinson's agents, placebo genes putatively overlapped with drug treatment genes or targets.
They also overlapped with disease-related genes. And so what that suggests is that when we were looking at the outcomes of clinical trial there might be a lot more going on that we are missing.
And let's just think about that for a minute. On the left is what we expect. We expect that we are going to see an effect in the drug, it's going to be greater than the effect of the placebo and that difference is what we want, that drug effect. But what we often see is on the right here where there is really no difference between drug and placebo. And so we are left to scratch our heads. Many companies go out of business. Many sections of companies close. And, quite frankly, patients are left in need. Money is left on the table because we can't discern between drug and placebo.
And I think what is interesting is that's been a theme that's kind of arisen since yesterday where oh, if only we had better physiological markers or better genes that targeted physiology then maybe we could see a difference and we can, you know, move forward with our clinical trials.
But what I'm going to argue today is actually what we need to do is to think about what is happening in the placebo arm, what is contributing to the heterogeneity in the placebo arm, and I'm going to argue that when we start to look at that compared to what is happening in the drug treatment arm, oftentimes -- and I'm going to give you demonstration after demonstration. And believe me, this is just the tip of the iceberg.
What we are seeing is there are differential effects by genotype in the drug treatment arm and the placebo treatment arm such that if you average out what's happening in these -- in these drug and placebo arms, you would basically see that there is no difference. But actually there's some people that are benefiting from the drug but not placebo. And conversely, benefiting from placebo but not drug. Average out to no difference.
Let me give you some examples. We had this hypothesis and we started to look around to see if we could get partners who had already done clinical trials that had happened to have genotyped COMT. And what we saw in this clinical trial for chronic fatigue syndrome where adolescents were treated with clonidine was that when we looked in the placebo arm, we saw that the val/val patients, so this is the COMT genotype. The low activity -- sorry, that is high activity genotype. They had the largest number increase in the number of steps they were taking per week. In contrast, the met/met people, the people with the weaker COMT had fewer, almost no change in the number of steps they were taking per week.
So you would look at this and you would say, oh, the val/val people were the placebo responders and the met/met people didn't respond to placebo. But what we saw when we looked into the drug treatment arm was very surprising. We saw that clonidine literally erased the effect that we were seeing in placebo for the val/val participants in this trial. And clonidine basically was having no effect on the heterozygotes, the val/mets or on the met/mets. And so this trial rightly concluded that there was no benefit for clonidine.
But if they hadn't taken this deeper look at what was happening, they would have missed that clonidine may potentially be harmful to people with chronic fatigue in this particular situation. What we really need to do I think is look not just in the placebo or not just in the drug treatment arm but in both arms to understand what is happening there.
And I'm going to give you another example. And, like I said, the literature is replete with these examples. On the left is an example from a drug that was used to test cognitive -- in cognitive scales, Tolcupone, which actually targets COMT. And what you can see here again on the left is differential outcomes in the placebo arm and in the drug treatment arm that if you were to just average these two you would not see the differences.
On the right is a really interesting study looking at alcohol among people with alcohol disorder, number of percent drinking days. And they looked at both COMT and OPRM1. And this is what Dr. Colloca was just talking about there seemed to be not just gene-placebo drug interactions but gene-gene drug placebo interactions. This is a complicated space. And I know we like things to be very simple. But I think what these data are showing is we need to pay more attention.
So let me give you another example because these -- you know, you could argue, okay, those are objective outcomes -- sorry, subjective outcomes. Let's take a look at the Women's Health Study. Arguably, one of the largest studies on aspirin versus placebo in history. 30,000 women were randomized to aspirin or placebo. And lo and behold, after 10 years of following them the p value was nonsignificant. There was no difference between drug and placebo.
So we went to this team, and we asked them, could we look at COMT because we had a hypothesis that COMT might modify the outcomes in the placebo arm and potentially differentially modify the treatments in the drug treatment arm. You might be saying that can't have anything to do with the placebo effect and we completely agree. This if we did find it would suggest that there might be something to do with the placebo response that is related to natural history. And I'm going to show you the data that -- what we found.
So when we compared the outcomes in the placebo arm to the aspirin arm, what we found was the met/met women randomized to placebo had the highest of everybody rates of cardiovascular disease. Which means the highest rates of myocardial infarction, stroke, revascularization and death from a cardiovascular disease cause. In contrast, the met/met women on aspirin had benefit, had a statistically significant reduction in these rates.
Conversely, the val/val women on placebo did the best, but the val/val women on aspirin had the highest rates, had significantly higher rates than the val/val women on placebo. What does this tell us? Well, we can't argue that this is a placebo effect because we don't have the control for placebo effects, which is a no treatment control.
But we can say that these are striking differences that, like I said before, if you don't pay attention to them, you miss the point that there are subpopulations for benefit or harm because of differential outcomes in the drug and placebo arms of the trial.
And so I'm going to keep going. There are other examples of this. We also partnered with a group at Brigham and Women's Hospital that had done the CAMP study, the Childhood Asthma Management Study. And in this study, they randomized patients to placebo, Budesonide or Nedocromil for five years and study asthma outcomes.
Now what I was showing you previously was candidate gene analyses. What this was, was a GWAS. We wanted to be agnostic and ask are there genes that modify the placebo outcomes and are these outcomes different in the -- when we look in the drug treatment arm. And so that little inset is a picture of all of the genes that were looked at in the GWAS. And we had a borderline genome Y significant hit called BBS9. And when we looked at BBS9 in the placebo arm, those white boxes at the top are the baseline levels of coughing and wheezing among these children. And in the gray are at the end of the treatment their level of coughing and wheezing.
And what you can see here is that participants with the AA genotype were the ones that benefited from the Bedenoside -- from placebo, whereas the GG, the patients with the GG genotype really there was no significant change.
Now, when we looked in the drug treatment arms, we were surprised to see that the outcomes were the same, of course, at baseline. There is no -- everybody is kind of the same. But you can see the differential responses depending on the genotype. And so, again, not paying attention to these gene drug/placebo interactions we miss another story that is happening here among our patients.
Now, I just want to -- I added this one because it is important just to realize that this is not just about gene-drug placebo. But these are also about epigenetic effects. And so here is the same study that I showed earlier on alcohol use disorder. They didn't just stop at looking at the polymorphisms or the genetic variants. This team also went so far as to look at methylation of OPRM1 and COMT.
So methylation is basically when the promoter region of a gene is basically blocked because it has a methyl group. It has methylation on some of the nucleotides in that region. So you can't make the protein as efficiently. And if you look on the right, what you can see in the three models that they looked at, they looked at other genes. They also looked at SLC6A3 that's involved in dopamine transport. And what you can see here is that there is significant gene by group by time interactions for all these three genes, these are candidate genes that they looked at.
And even more fascinating is their gene-by-gene interactions. Basically it is saying that you cannot say what the outcome is going to be unless you know the patient's or the participant's COMT or OPRM genotype A and also how methylated the promoter region of that -- of these genes are. So this makes for a very complicated story. And I know we like very simple stories.
But I want to say that I'm just adding to that picture that we had before to say that it's not just in terms of the gene's polymorphisms, but as Dr. Colloca just elegantly showed it is transcription as well as methylation that might be modifying what is happening in the drug treatment arm and the placebo treatment arm. And to add to this it might also be about the natural history of the condition.
So BBS9 is actually a gene that is involved in the cilia, the activity of the formation of the cilia which is really important in breathing in the nasal canal. And so, you can see that it is not just about what's happening in the moment when you are doing the placebo or drug or the clinical trial, it also might -- the genes might also be modifying where the patient starts out and how the patient might develop over time. So, in essence, we have a very complicated playground here.
But I think I have shown you that genetic variation, whether it is polymorphisms in the gene, gene-gene interactions or epigenetics or all of the above can modify the outcomes in placebo arms of clinical trials. And that this might be due to the genetic effects on placebo effects or the genetic effects on natural history. And this is something I think we need to understand and really pay attention to.
And I also think I've showed you, and these are just a few examples, there are many more. But genetic variation can differentially modify drugs and placebos and that these potential interactive effects really challenge this basic assumption of additivity that I would argue we have had for far too long and we really need to rethink.
TED KAPTCHUK: (Laughing) Very cool.
KATHRYN HALL: Hi, Ted.
TED KAPTCHUK: Oh, I didn't know I was on.
KATHRYN HALL: Yeah, that was great. That's great.
So in summary, can we use these gene-placebo drug interactions to improve clinical trials. Can we change our expectations about what is happening. And perhaps as we have been saying for the last two days, we don't need new drugs with clear physiological effects, what we need is to understand drug and placebo interactions and how they impact subpopulations and can reveal who benefits or is harmed by therapies.
And finally, as we started to talk about in the last talk, can we use drugs to boost placebo responses? Perhaps some drugs already do. Conversely, can we use drugs to block placebo responses? And perhaps some drugs already do.
So I just want to thank my collaborators. There was Ted Kaptchuk, one of my very close mentors and collaborators. And really, thank you for your time.
CRISTINA CUSIN: Thank you so much. It was a terrific presentation. And definitely Ted's captured laugh, it was just one of the best spontaneous laughs.
We have a couple of questions coming through the chat. One is about the heterogeneity of response in placebo arms. It is not uncommon to see quite a dispersion of responses at trials. Was that thought experiment, if one looks at the fraction of high responders in the placebo arms, would one expect to see, enrich for some of the genetic marker for and as placebo response?
KATHRYN HALL: I absolutely think so. We haven't done that. And I would argue that, you know, we have been having kind of quiet conversation here about Naloxone because I think as Lauren said yesterday that the findings of Naloxone is variable. Sometimes it looks like Naloxone is blocking placebo response and sometimes it isn't.
We need to know more about who is in that trial, right? Is this -- I could have gone on and showed you that there is differences by gender, right. And so this heterogeneity that is coming into clinical trials is not just coming from the genetics. It's coming from race, ethnicity, gender, population. Like are you in Russia or are you in China or are you in the U.S. when you're conducting your clinical trial? We really need to start unpacking this and paying attention to it. I think because we are not paying attention to it, we are wasting a lot of money.
CRISTINA CUSIN: And epigenetic is another way to consider traumatic experiences, adverse event learning. There is another component that we are not tracking accurately in clinical trials. I don't think this is a one of the elements routinely collected. Especially in antidepressant clinical trials it is just now coming to the surface.
KATHRYN HALL: Thank you.
CRISTINA CUSIN: Another question comes, it says the different approaches, one is GWAS versus candidate gene approach.
How do you start to think about genes that have a potential implication in neurophysiological pathways and choosing candidates to test versus a more agnostic U.S. approach?
KATHRYN HALL: I believe you have to do both because you don't know what you're going to find if you do a GWAS and it's important to know what is there.
At the same time, I think it's also good to test our assumptions and to replicate our findings, right? So once you do the GWAS and you have a finding -- for instance, our BBS9 finding would be amazing to replicate or to try and test in another cohort. But, of course, it is really difficult to do a whole clinical trial again. These are very expensive, and they last many years.
And so, you know, I think replication is something that is tough to do in this space, but it is really important. And I would do both.
CRISTINA CUSIN: Thank you. We got a little short on time. We are going to move on to the next speaker. Thank you so much.
FADEL ZEIDAN: Good morning. It's me, I imagine. Or good afternoon.
Let me share my screen. Yeah, so good morning. This is going to be a tough act to follow. Dr. Colloca and Dr. Hall's presentations were really elegant. So manage your expectations for mine. And, Ted, please feel free to unmute yourself because I think your laugh is incredibly contagious, and I think we were all were laughing as well.
So my name is Fadel Zeidan, I'm at UC San Diego. And I'll be discussing mostly unpublished data that we have that's under review examining if and how mindfulness meditation assuages pain and if the mechanism supporting mindfulness meditation-based analgesia are distinct from placebo.
And so, you know, this is kind of like a household slide that we all are here because we all appreciate how much of an epidemic chronic pain is and, you know, how significant it is, how much it impacts our society and the world. And it is considered a silent epidemic because of the catastrophic and staggering cost to our society. And that is largely due to the fact that the subjective experience of pain is modulated and constructed by a constellation of interactions between sensory, cognitive, emotional dimensions, genetics, I mean I can -- the list can go on.
And so what we've been really focused on for the last 20 years or so is to appreciate if there is a non-pharmacological approach, a self-regulated approach that can be used to directly assuage the experience of pain to acutely modify exacerbated pain.
And to that extent, we've been studying meditation, mindfulness-based meditation. And mindfulness is a very nebulous construct. If you go from one lab to another lab to another lab, you are going to get a different definition of what it is. But obviously my lab's definition is the correct one. And so the way that we define it is awareness of arising sensory events without reaction, without judgment.
And we could develop this construct, this disposition by practicing mindfulness-based meditation, which I'll talk about here in a minute. And we've seen a lot of -- and this is an old slide -- a lot of new evidence, converging evidence demonstrating that eight weeks of manualized mindfulness-based interventions can produce pretty robust improvements in chronic pain and opiate misuse. These are mindfulness-based stress reduction programs, mindfulness-oriented recovery enhancement, mindfulness-based cognitive therapy which are about eight weeks long, two hours of formalized didactics a week, 45 minutes a day of homework.
There is yoga, there is mental imagery, breathing meditation, walking meditation, a silent retreat and about a $600 tab. Which may not be -- I mean although they are incredibly effective, may not be targeting demographics and folks that may not have the time and resources to participate in such an intense program.
And to that extent and, you know, as an immigrant to this country I've noticed that we are kind of like this drive-thru society where, you know, we have a tendency to eat our lunches and our dinners in our cars. We're attracted to really brief interventions for exercise or anything really, pharmaceuticals, like ":08 Abs" and "Buns of Steel." And we even have things called like the military diet that promise that you'll lose ten pounds in three days without dying.
So we seemingly are attracted to these fast-acting interventions. And so to this extent we've worked for quite some time to develop a very user friendly, very brief mindfulness-based intervention. So this is an intervention that is about four sessions, 20 minutes each session. And participants are -- we remove all religious aspects, all spiritual aspects. And we really don't even call it meditation, we call it mindfulness-based mental training.
And our participants are taught to sit in a straight posture, close their eyes, and to focus on the changing sensations of the breath as they arise. And what we've seen is this repetitive practice enhances cognitive flexibility and the ability to -- flexibility and the ability to sustain attention. And when individual's minds drift away from focusing on the breath, they are taught to acknowledge distractive thoughts, feelings, emotions without judging themselves or the experience. Doing so by returning their attention back to the breath.
So there is really a one-two punch here where, A, you're focusing on the breath and enhancing cognitive flexibility; and, B, you're training yourself to not judge discursive events. And that we believe enhances emotion regulation. So quite malleable to physical training we would say mental training. Now that we have the advent of imaging, we can actually see that there are changes in the brain related to this.
But as many of you know, mindfulness is kind of like a household term now. It's all over our mainstream media. You know, we have, you know, Lebron meditating courtside. Oprah meditating with her Oprah blanket. Anderson Cooper is meditating on TV. And Time Magazine puts, you know, people on the cover meditating. And it's just all over the place.
And so these types of images and these types of, I guess, insinuations could elicit nonspecific effects related to meditation. And for quite some time I've been trying to really appreciate not is meditation more effective than placebo, although that's interesting, but does mindfulness meditation engage mechanisms that also are shared by placebo? So beliefs that you are meditating could elicit analgesic responses.
The majority of the manualized interventions in their manuals they use terms like the power of meditation, which I guarantee you is analgesic. To focus on the breath, we need to slow the breath down. Not implicit -- not explicitly, but it just happens naturally. And slow breathing can also reduce pain. Facilitator attention, social support, conditioning, all factors that are shared with other therapies and interventions but in particular are also part of meditation training.
So the question is because of all this, is mindfulness meditation merely -- or not merely after these two rich days of dialogue -- but is mindfulness meditation engaging processes that are also shared by placebo.
So if I apply a placebo cream to someone's calf and then throw them in the scanner versus asking someone to meditate, the chances are very high that the brain processes are going to be distinct. So we wanted to create a -- and validate an operationally matched mindfulness meditation intervention that we coined as sham mindfulness meditation. It's not sham meditation because it is meditation. It's a type of meditative practice called Pranayama.
But here in this intervention we randomize folks, we tell folks that they've been randomized to a genuine mindfulness meditation intervention. Straight posture, eyes closed. And every two to three minutes they are instructed to, quote-unquote, take a deep breath as we sit here in mindfulness meditation. We even match the time giving instructions between the genuine and the sham mindfulness meditation intervention.
So the only difference between the sham mindfulness and the genuine mindfulness is that the genuine mindfulness is taught to explicitly focus on the changing sensations of the breath without judgment. The sham mindfulness group is just taking repetitive deep, slow breaths. So if the magic part of mindfulness, if the active component of mindfulness is this nonjudgmental awareness, then we should be able to see disparate mechanisms between these.
And we also use a third arm, a book listening control group called the "Natural History of Selborne" where it's a very boring, arguably emotionally pain-evocating book for four days. And this is meant to control for facilitator time and -- sorry, facilitator attention and the time elapsed in the other group's interventions.
So we use a very high level of noxious heat to the back of the calf. And we do so because imaging is quite expensive, and we want to ensure that we can see pain-related processing within the brain. Here and across all of our studies, we use ten 12-second plateaus of 49 degrees to the calf, which is pretty painful.
And then we assess pain intensity and pain unpleasantness using a visual analog scale, where here the participants just see red the more they pull on the algometer the more in pain they are. But on the back, the numbers fluoresce where 0 is no pain and 10 is the worst pain imaginable.
So pain intensity can be considered like sensory dimension of pain, and pain unpleasantness could be more like I don't want to say pain affect but more like the bothersome component of pain, pain unpleasantness. So what we did was we combined all of our studies that have used the mindfulness, sham mindfulness in this book listing control, to see does mindfulness meditation engage is mindfulness meditation more effective than sham mindfulness meditation at reducing pain.
We also combined two different fMRI techniques: Blood oxygen dependent level signalling, bold, which allows us a higher temporal resolution and signal to noise ratio than, say, perfusion imaging technique and allows us to look at connectivity. However, meditation is also predicated on changes in respiration rate which could elicit pretty dramatic artifacts in the brain, breathing related artifacts explicitly related to CO2 output.
So using the perfusion based fMRI technique like arterial spin labeling is really advantageous as well, although it's not as temporally resolute as bold, it provides us a direct quantifiable measurement of cerebral blood flow.
So straight to the results. On the Y axis we have the pain ratings, and on the X axis are book listening controls sham mindfulness meditation, mindfulness meditation. Here are large sample sizes. Blue is intensity and red is unpleasantness. This is the post intervention fMRI scans where we see the first half of the scan to the second half of the scan our controlled participants are simply resting and pain just increases because of pain sensitization and being in a claustrophobic MRI environment.
And you can see here that sham mindfulness meditation does produce pretty significant reduction in pain intensity and unpleasantness, more than the control book. But mindfulness meditation is more effective than sham mindfulness and the controls at reducing pain intensity and pain unpleasantness.
There does seem to be some kind of additive component to the genuine intervention, although this is a really easy practice, the sham techniques.
So for folks that have maybe fatigue or cognitive deficits or just aren't into doing mindfulness technique, I highly recommend this technique, which is just a slow breathing approach, and it's dead easy to do.
Anyone that's practiced mindfulness for the first time or a few times can state that it can be quite difficult and what's the word? -- involving, right?
So what happened in the brain? These are our CBF maps from two studies that we replicated in 2011 and '15 where we found that higher activity, higher CBF in the right anterior insula, which is ipsilateral to the stimulation site and higher rostral anterior cingulate cortex subgenual ACC was associated with greater pain relief, pain intensity, and in the context of pain unpleasantness, higher over the frontal cortical activity was associated with lower pain, and this is very reproducible where we see greater thalamic deactivation predicts greater analgesia on the unpleasantness side.
These areas, obviously right entry insula in conjunction with other areas is associated with interoceptive processing awareness of somatic sensations. And then the ACC and the OFC are associated with higher order cognitive flexibility, emotional regulation processes. And the thalamus is really the gatekeeper from the brain -- I'm sorry, from the body to the brain. Nothing can enter the brain except unless it goes through the thalamus, except if it's the sense of smell.
So it's really like this gatekeeper of arising nociceptive information.
So the takehome here is that mindfulness is engaging multiple neural processes to assuage pain. It's not just one singular pathway.
Our gold studies were also pretty insightful. Here we ran a PPI analysis, psychophysiologic interaction analysis and this was whole brain to see what brain regions are associated with pain relief on the context of using the bold technique, and we find that greater ventral medial prefrontal cortical activity deactivation I'm sorry is associated with lower pain, and the vmPFC is a super evolved area that's associated with, like, higher order processes relating to self. It's one of the central nodes of the so called default mode network, a network supporting self referential processing. But in the context of the vmPFC, I like the way that Tor and Mathieu reflect the vmPFC as being more related to affective meaning and has a really nice paper showing that vmPFC is uniquely involved in, quote/unquote, self ownership or subjective value, which is particularly interesting for the context of pain because pain is a very personal experience that's directly related to the interpretation of arising sensations and what they mean to us.
And seemingly -- I apologize for the reverse inferencing here -- but seemingly mindfulness meditation based on our qualitative assessments as well is reducing the ownership or the intrinsic value, the contextual value of those painful sensations, i.e., they don't feel like they bother -- that pain is there but it doesn't bother our participants as much, which is quite interesting as a manipulation.
We also ran our connectivity analysis between the contralateral thalamus and the whole brain, and we found that greater decoupling between the contralateral thalamus and the precuneus, another central node of the default mode network predicted greater analgesia.
This is a really cool, I think, together mechanism showing that two separate analyses are indicating that the default mode network could be an analgesic system which we haven't seen before. We have seen the DMN involved in chronic pain and pain related exacerbations, but I don't think we've seen it as being a part of an analgesic, like being a pain relieving mechanism. Interestingly, the thalamus and precuneus together are the first two nodes to go offline when we lose consciousness, and they're the first two nodes to come back online when we recover from consciousness, suggesting that these two -- that the thalamus and precuneus are involved in self referential awareness, consciousness of self, things of this nature.
Again, multiple processes involved in meditation based pain relief which maybe gives rise to why we are seeing consistently that meditation could elicit long lasting improvements in pain unpleasantness, in particular, as compared to sensory pain. Although it does that as well.
And also the data gods were quite kind on this because these mechanisms are also quite consistent with the primary premises of Buddhist and contemplative scriptures saying that the primary principle is that your experiences are not you.
Not that there is no self, but that the processes that arise in our moment to moment experience are merely reflections and interpretations in judgments, and that may not be the true inherent nature of mind.
And so before I get into more philosophical discourse, I'm going to keep going for the sake of time. Okay.
So what happened with the sham mindfulness meditation intervention?
We did not find any neural processes predicted analgesia significantly and during sham mindfulness meditation. What did predict analgesia during sham mindfulness was slower breathing rate, which we've never seen before with mindfulness. We've never seen a significant or even close to significant relationship between mindfulness based meditation analgesia and slow breathing. But over and over we see that sham mindfulness based analgesia is related to slower breathing which provides us this really cool distinct process where kind of this perspective where mindfulness is engaging higher order top down type processes to assuage pain while sham mindfulness may be engaging this more bottom up type response to assuage pain.
I'm going to move on to some other new work, and this is in great collaboration with the lovely Tor Wager, and he's developed, with Marta and Woo, these wonderful signatures, these machine learned multivariate pattern signatures that are remarkably accurate at predicting pain over I think like 98, 99 percent.
His seminal paper, the Neurological Pain Signature, was published in the New England Journal of Medicine that showed that these signatures can predict nociceptive specific, in particular, for this particular, thermal heat pain with incredible accuracy.
And it's not modulated by placebo or affective components, per se. And then the SIIPS is a machine learned signature that is, as they put it, associated with cerebral contributions to pain. But if you look at it closely, these are markers that are highly responsive to the placebo response.
So the SIIPS can be used -- he has this beautiful pre print out, showing that it does respond with incredible accuracy to placebo, varieties of placebo.
So we used this MVPA to see if meditation engages signature supporting placebo responses.
And then Marta Ceko's latest paper with Tor published in Nature and Neuro found that the negative affect of signature predicts pain responses above and beyond nociceptive related processes. So this is pain related to negative affect, which again contributes to the multimodal processing of pain and how now we could use these elegant signatures to kind of disentangle which components of pain meditation and other techniques assuage. Here's the design.
We had 40 -- we combined two studies. One with bold and one with ASL. So this would be the first ASL study with signatures, with these MVPA signatures.
And we had the mindfulness interventions that I described before, the book listing interventions I described before and a placebo cream intervention which I'll describe now, all in response to 49 degrees thermal stimuli.
So across again all of our studies we use the same methods. And the placebo group -- I'll try to be quick about this -- this is kind of a combination of Luana Colloca, Don Price and Tor's placebo conditioning interventions where we administer 49 degrees -- we tell our participants that we're testing a new form of lidocaine, and the reason that it's new is that the more applications of this cream, the stronger the analgesia.
And so in the conditioning sessions, they come in, administer 49 degrees, apply and remove this cream, which is just petroleum jelly after 10 minutes, and then we covertly reduce the temperature to 48.
And then they come back in in session two and three, after 49 degrees and removing the cream, we lower the temperature to 47. And then on the last conditioning session, after we remove the cream, we lower the temperature to 46.5, which is a qualitatively completely different experience than 49.
And we do this to lead our participants to believe that the cream is actually working.
And then in a post intervention MRI session, after we remove the cream, we don't modulate the temperature, we just keep it at 49, and that's how we measured placebo in these studies. And then so here, again -- oops -- John Dean and Gabe are coleading this project.
Here, pain intensity on this axis, pain unpleasantness on that axis, controls from the beginning of the scan to the end of the scan significantly go up in pain.
Placebo cream was effective at reducing intensity and unpleasantness, but we see mindfulness meditation was more effective than all the conditions at reducing pain. The signatures, we see that the nociceptive specific signature, the controls go up in pain here.
No change in the placebo and mindfulness meditation you can see here produces a pretty dramatic reduction in the nociceptive specific signature.
The same is true for the negative affective pain signature. Mindfulness meditation uniquely modifies this signature as well which I believe this is one of the first studies to show something like this.
But it does not modulate the placebo signature. What does modulate the placebo signature is our placebo cream, which is a really nice manipulation check for these signatures.
So here, taken together, we show that mindfulness meditation, again, is engaging multiple processes and is reducing pain by directly assuaging nociceptive specific markers as well as markers supporting negative affect but not modulating placebo related signatures, providing further credence that it's not a placebo type response, and we're also demonstrating this granularity between a placebo mechanism that's not being shared by another active mechanism. While we all assume that active therapies and techniques are using a shared subset of mechanisms or processes with placebo, here we're providing accruing evidence that mindfulness is separate from a placebo.
I'll try to be very quick on this last part. This is all not technically related placebo, but I would love to hear everyone's thoughts on these new data we have.
So as we've seen elegantly that pain relief by placebo, distraction, acupuncture, transcranial magnetic stimulation, prayer, are largely driven by endogenous opioidergic release. And, yes, there are other systems. A prime other system is the (indiscernible) system, serotonergic system, dopamine. The list can go on. But it's considered by most of us that the endogenous opioidergic system is this central pain modulatory system.
And the way we do this is by antagonizing endogenous opioids by employing incredibly high administration dosage of naloxone.
And I think this wonderful paper by Ciril Etnes's (phonetic) group provides a nice primer on the appropriate dosages for naloxone to antagonize opiates. And I think a lot of the discussions here where we see differences in naloxone responses are really actually reflective of differences in dosages of naloxone.
It metabolizes so quickly that I would highly recommend a super large bolus with a maintenance infusion IV.
And we've seen this to be a quite effective way to block endogenous opioids. And across four studies now, we've seen that mindfulness based pain relief is not mediated by endogenous opioids. It's something else. We don't know what that something else is but we don't think it's endogenous opioids. But what if it's sex differences that could be driving these opioidergic versus non opioid opioidergic differences?
We've seen that females require -- exhibit higher rates of chronic pain than males. They are prescribed opiates at a higher rate than men. And when you control for weight, they require higher dosages than men. Why?
Well, there's excellent literature in rodent models and preclinical models that demonstrate that male rodents versus female -- male rodents engage endogenous opioids to reduce pain but female rodents do not.
And this is a wonderful study by Ann Murphy that basically shows that males, in response to morphine, have a greater latency and paw withdrawal when coupled with morphine and not so much with females.
But when you add naloxone to the picture, with morphine, the latency goes down. It basically blocks the analgesia in male rodents but enhances analgesia in female rodents.
We basically asked -- we basically -- Michaela, an undergraduate student doing an odyssey thesis asked this question: Are males and females in humans engaging in distinct systems to assuage pain?
She really took off with this and here's the design. We had heat, noxious heat in the baseline.
CRISTINA CUSIN: Doctor, you have one minute left. Can you wrap up?
FADEL ZEIDAN: Yep. Basically we asked, are there sex differences between males and females during meditation in response to noxious heat? And there are.
Baseline, just change in pain. Green is saline. Red is naloxone. You can see that with naloxone onboard, there's greater analgesia in females, and we reversed the analgesia. Largely, there's no differences between baseline in naloxone in males, and the males are reducing pain during saline.
We believe this is the first study to show something like this in humans. Super exciting. It also blocked the stress reduction response in males but not so much in females. Let me just acknowledge our funders. Some of our team. And I apologize for the fast presentation. Thank you.
CRISTINA CUSIN: Thank you so much. That was awesome.
We're a little bit on short on time.
I suggest we go into a short break, ten minute, until 1:40. Please continue to add your questions in Q&A. Our speakers are going to answer or we'll bring some of those questions directly to the discussion panel at the end of the session today. Thank you so much.
Measuring & Mitigating the Placebo Effect (continued)
CRISTINA CUSIN: Hello, welcome back. I'm really honored to introduce our next speaker, Dr. Marta Pecina. And she's going to talk about mapping expectancy-mood interactions in antidepressant placebo effects. Thank you so much.
MARTA PECINA: Thank you, Cristina. It is my great pleasure to be here. And just I'm going to switch gears a little bit to talk about antidepressant placebo effects. And in particular, I'm going to talk about the relationship between acute expectancy-mood neural dynamics and long-term antidepressant placebo effects.
So while we all know that depression is a very prevalent disorder, and just in 2020, Major Depressive Disorder affected 21 million adults in the U.S. and 280 million adults worldwide. And current projections indicate that by the year 2030 it will be the leading cause of disease burden globally.
Now, response rates to first-line treatments, antidepressant treatments are approximately 50%. And complete remission is only achieved in 30 to 35% of individuals. Also, depression tends to be a chronic disorder with 50% of those recovering from a first episode having an additional episode. And 80% of those with two or more episodes having another recurrence.
And so for patients who are nonresponsive to two intervention, remission rates with subsequent therapy drop significantly to 10 to 25%. And so, in summary, we're facing a disorder that is very resistant or becomes resistant very easily. And in this context, one would expect that antidepressant placebo effects would actually be low. But we all know that this is not the case. The response rate to placebos is approximately 40% compared to 50% response rates to antidepressants. And obviously this varies across studies.
But what we do know and learned yesterday as well is that response rates to placebos have increased approximately 7% over the last 40 years. And so these high prevalence of placebo response in depressions have significantly contributed to the current psychopharmacology crisis where large pharma companies have reduced at least in half the number of clinical trials devoted to CNS disorders.
Now, antidepressant placebo response rates among individuals with depression are higher than in any other psychiatric condition. And this was recently published again in this meta-analysis of approximately 10,000 psychiatric patients. Now, other disorders where placebo response rates are also prevalent are generalized anxiety disorder, panic disorders, HDHC or PTSD. And maybe less frequent, although still there, in schizophrenia or OCD.
Now, importantly, placebo effects appear not only in response to pills but also surgical interventions or devices, as it was also mentioned yesterday. And this is particularly important today where there is a very large development of device-based interventions for psychiatric conditions. So, for example, in this study that also was mentioned yesterday of deep brain stimulation, patients with resistant depression were assigned to six months of either active or some pseudo level DBS. And this was followed by open level DBS.
As you can see here in this table, patients from both groups improved significantly compared to baseline, but there were no significant differences between the two groups. And for this reason, DBS has not yet been approved by the FDA for depression, even though it's been approved for OCD or Parkinson's disease as we all know.
Now what is a placebo effect, that's one of the main questions of this workshop, and how does it work from a clinical neuroscience perspective? Well, as it's been mentioned already, most of what we know about the placebo effect comes from the field of placebo analgesia. And in summary, classical theories of the placebo effect have consistently argued that placebo effects results from either positive expectancies regarding the potential beneficial effects of a drug or classical conditioning where the pairing of a neutral stimulus, in this case the placebo pill, with an unconditioned stimulus, in this case the active drug, results in a conditioned response.
Now more recently, theories of the placebo effect have used computational models to predict placebo effects. And these theories posit that individuals update their expectancies as new sensory evidence is accumulated by signaling the response between what is expected and what is perceived. And this information is then used to refine future expectancies. Now these conceptual models have been incorporated into a trial-by-trial manipulation of both expectancies of pain relief and pain sensory experience. And this has rapidly advanced our understanding of the neural and molecular mechanisms of placebo analgesia.
And so, for example, in these meta analytic studies using these experiments they have revealed really two patterns of distinct activations with decreases in brain activity in regions involving brain processing such as the dorsal medial prefrontal cortex, the amygdala and the thalamus; and increases in brain activity in regions involving effective appraisal, such as the vmDFC, the nucleus accumbens, and the PAG.
Now what happens in depression? Well, in the field of antidepressant placebo effects, the long-term dynamics of mood and antidepressant responses have not allowed us to have such trial-by-trial manipulation of expectancies. And so instead researchers have used broad brain changes in the context of a randomized control trial or a placebo lead-in phase which has, to some extent, limited the progress of the field.
Now despite these methodological limitations of these studies, they provide important insights about the neural correlates of antidepressant placebo effects. In particular, from studies -- two early on studies we can see the placebo was associated with increased activations broadly in cortical regions and decreased activations in subcortical regions. And these deactivations in subcortical regions were actually larger in patients who were assigned to an SSRI drug treatment.
We also demonstrated that there is similar to pain, antidepressant placebo effects were associated with enhanced endogenous opiate release during placebo administration, predicting the response to open label treatment after ten weeks. And we have also -- we and others have demonstrated that increased connectivity between the salience network and the rostral anterior cingulate during antidepressant placebo effects can actually predict short-term and long-term placebo effects.
Now an important limitation, and as I already mentioned, is that this study is basically the delay mechanism of action of common antidepressant and this low dynamics of mood which really limit the possibility of actively manipulating antidepressant expectancies.
So to address this important gap, we develop a trial-by-trial manipulation of antidepressant expectancies to be used inside of the scanner. And the purpose was really to be able to further disassociate expectancy and mood dynamics during antidepressant placebo effects.
And so the basic structure of this test involved an expectancy condition where subjects are presented with a four-second infusion cue followed by an expectancy rating cue, and a reinforcement condition which consist of 20 seconds of some neurofeedback followed by a mood rating cue. Now the expectancy and the reinforcement condition map onto the classical theories of the placebo effect that I explained earlier.
During the expectancy condition, the antidepressant infusions are compared to periods of calibration where no drug is administered. And during the reinforcement condition, on the other hand, some neurofeedback of positive sign 80% of the time as compared to some neurofeedback of baseline sign 80% of the time. And so this two-by-two study design results in four different conditions. The antidepressant reinforced, the antidepressant not reinforced, the calibration reinforced, and the calibration not reinforced.
And so the cover story is that we tell participants that we are testing the effects of a new fast-acting antidepressant compared to a conventional antidepressant, but in reality, they are both saline. And then we tell them that they will receive multiple infusions of these drugs inside of the scanner while we record their brain activity which we call neurofeedback. So then patients learn that positive neurofeedback compared to baseline is more likely to cause mood improvement. But they are not told that the neurofeedback is simulated.
Then we place an intravenous line for the administration of the saline infusion, and we bring them inside of the scanner. For these kind of experiments we recruit individuals who are 18 through 55 with or without anxiety disorders and have a HAMD depression rating scale greater than 16, consistent with moderate depression. They're antidepressant medication free for at least 25 -- 21 days and then we use consenting procedures that involve authorized deception.
Now, as suspected, behavioral results during this test consistently show that antidepressant expectancies are higher during the antidepressant infusions compared to the calibration, especially when they are reinforced by positive sham neurofeedback. Now mood responses also are significantly higher during positive sham neurofeedback compared to baseline. But this is also enhanced during the administration of the antidepressant infusions.
Now interestingly, these effects are moderated by the present severity such that the effects of the test conditions and the expectancies and mood ratings are weaker in more severe depression even though their overall expectancies are higher, and their overall mood are lower.
Now at a neuron level, what we see is that the presentation of the infusion cue is associated with an increased activation in the occipital cortex and the dorsal attention network suggesting greater attention processing engaged during the presentation of the treatment cue. And similarly, the reinforcement condition revealed increased activations in the dorsal attention network with additional responses in the ventral striatum suggesting that individuals processed the sham positive neurofeedback cue as rewarding.
Now an important question for us was now that we can manipulate acute placebo -- antidepressant placebo responses, can we use this experiment to understand the mechanisms implicated in short-term and long-term antidepressant placebo effects. And so as I mentioned earlier, there was emerging evidence suggesting that placebo analgesic could be explained by computational models, in particular reinforcement learning.
And so we tested the hypothesis that antidepressant placebo effects could be explained by similar models. So as you know, under these theories, learning occurs when an experienced outcome differs from what is expected. And this is called the prediction error. And then the expected value of the next possible outcome is updated with a portion of this prediction error as reflected in this cue learning rule.
Now in the context of our experiment, model predicted expectancies for each of the four trial conditions would be updated every time the antidepressant or the calibration infusion cue is presented and an outcome, whether positive or baseline neurofeedback, is observed based on a similar learning rule.
Now this basic model was then compared against two alternative models. One which included differential learning rates to account for the possibility that learning would depend on whether participants were updating expectancies for the placebo or the calibration. And then an additional model to account for the possibility that subjects were incorporating positive mood responses as mood rewards.
And then finally, we constructed this additional model to allow the possibility of the combination of models two and three. And so using patient model comparison, we found that the model -- the fourth model, model four which included a placebo bias learning in our reinforcement by mood dominated all the other alternatives after correction for patient omnibus risk.
Now we then map the expected value and reward predictions error signals from our reinforcement learning models into our raw data. And what we found was that expected value signals map into the salience network raw responses; whereas reward prediction errors map onto the dorsal attention network raw responses. And so all together, the combination of our model-free and model-based results reveal that the processing of the antidepressant in patient cue increase activation in the dorsal attention network; whereas, the encoding of the expectancies took place in the salience network once salience had been attributed to the cue.
And then furthermore, we demonstrated that the reinforcement learning model predicted expectancies in coding the salience network triggered mood changes that are perceived as reward signals. And then these mood reward signals further reinforce antidepressant expectancies through the information of expectancy mood dynamics defined by models of reinforcement learning, an idea that could possibly contribute to the formation of long-lasting antidepressant placebo effects.
And so the second aim was really -- was going to look at these in particular how to use behavioral neuroresponses of placebo effects to predict long-term placebo effects in the context of a clinical trial. And so our hypothesis was that during placebo administration greater salient attribution to the contextual cue in the salience network would transfer to regions involved in mood regulation to induce mood changes. So in particular we hypothesized that the DMN would play a key role in belief-induced mood regulation.
And why the DMN? Well, we knew that activity in the rostral anterior cingulate, which is a key node of the DMN, is a robust predictor of mood responses to both active antidepressant and placebos, implying its involvement in nonspecific treatment response mechanisms. We also knew that the rostral anterior cingulate is a robust predicter of placebo analgesia consistent with its role in cognitive appraisals, predictions and evaluation. And we also had evidence that the SN to DMN functional connectivity appears to be a predictor of placebo and antidepressant responses over ten weeks of treatment.
And so in our clinical trial, which you can see the cartoon diagram here, we randomized six individuals to placebo or escitalopram 20 milligrams. And this table is just to say there were no significant differences between the two groups in regard to the gender, race, age, or depression severity. But what we found interesting is that there were also no significant differences in the correct belief assignment with 60% of subjects in each group approximately guessing that they were receiving escitalopram.
Now as you can see here, participants showed lower MADR scores at eight weeks in both groups. But there was no significant differences between the two groups. However, when split in the two groups by the last drug assignment belief, subjects with the drug assignment belief improved significantly compared to those with a placebo assignment belief.
And so the next question was can we use neuroimaging to predict these responses? And what we found was at a neural level during expectancy process the salience network had an increased pattern of functional connectivity with the DMN as well as with other regions of the brainstem including the thalamus. Now at the end -- we also found that increased SN to DMN functional connectivity predicted expectancy ratings during the antidepressant placebo fMRI task such that higher connectivity was associated with greater modulation of the task conditions on expectancy ratings.
Now we also found that enhanced functional connectivity between the SN and the DMN predicted the response to eight weeks of treatment, especially on individuals who believed that they were of the antidepressant group. Now this data supports that during placebo administration, greater salient attributions to the contextual cue is encoded in the salience network; whereas belief-induced mood regulation is associated with an increased functional connectivity between the SN and DMN and altogether this data suggest that enhancements to DMN connectivity enables the switch from greater salient attribution to the treatment cue to DMN-mediated mood regulation.
And so finally, and this is going to be brief, but the next question for us was can we modulate these networks to actually enhance placebo-related activity. And in particular, we decided to use theta burst stimulation which can potentiate or depotentiate brain activity in response to brief periods of stimulation. And so in this study participants undergo three counterbalance sessions of TBS with either continuous, intermittent, or sham known to depotentiate, potentiate, and have no effect.
So each TBS is followed by an fMRI session during the antidepressant placebo effect task which happens approximately an hour after stimulation. The inclusive criteria are very similar to all of our other studies. And our pattern of stimulation is pretty straightforward. We do two blocks of TBS. And during the first block stimulation intensity is gradually escalated in 5% increments in order to enhance tolerability. And during the second session the stimulation is maintained constant at 80% of the moderate threshold.
Then we use the modified cTBS session consisting of three stimuli applied at intervals of 30 hertz. We first repeat it at 6 hertz for a total of 600 stimuli in a continuous train of 33.3 seconds. Then we did the iTBS session consist of a burst of three stimuli applied at intervals of 50 hertz with bursts repeated at 5 hertz for a total of 600 stimulus during 192 seconds. We also use a sham condition where 50% of subjects are assigned to sham TBS simulating the iTBS stimulus pattern, and 50% are assigned to sham TBS simulating the cTBS pattern.
Now our target is the DMN which is the cortical target for the dorsal medial -- the cortical target for the DMN -- sorry, the dmPFC which is the cortical target for the DMN. And this corresponds to the -- and we found these effects based on the results from the antidepressant placebo fMRI task.
And so this target corresponds to our neurosynth scalp which is located 30% of the distance from the nasion-to-inion forward from the vertex and 5% left which corresponds to an EEG location of F1. And the connectivity map of these regions actually result in activation of the DMN. Now we can also show here the E-Field map of this target which basically demonstrates supports a nice coverage of the DMN.
And so what we found here is that the iTBS compared to sham and cTBS enhances the effect of the reinforcement condition of mood responses. And we also found that at a neural level iTBS compared to cTBS shows significant greater bold responses during expectancy processing within the DMN with sham responses in the middle but really not significantly different from iTBS. Now, increased bold responses in the ventral medial prefrontal cortex were associated with a greater effect of the task conditions of mood responses.
And so all together our results suggest that first trial-by-trial modulation of antidepressant expectancies effectively disassociates expectancy mood dynamics. Antidepressant expectancies are predicted by models of reinforcement learning and they're encoded in the salience network. We also showed that enhanced SN to DMN connectivity enables the switch from greater salient attribution to treatment cues to DMN-mediated mood regulation, contributing to the formation of acute expectancy-mood interactions and long-term antidepressant placebo effects. And iTBS potentiation of the DMN enhances placebo-induced mood responses and expectancy processing.
With this, I would just like to thank my collaborators that started this work with me at the University of Michigan and mostly the people in my lab and collaborators at the University of Pittsburgh as well as the funding agencies.
CRISTINA CUSIN: Wonderful presentation. Really terrific way of trying to untangle different mechanism in placebo response in depression, which is not an easy feat.
There are no specific questions in the Q&A. I would encourage everybody attending the workshop to please post your question to the Q&A. Every panelist can answer in writing. And then we will answer more questions during the discussion, but please don't hesitate.
I think I will move on to the next speaker. We have only a couple of minutes so we're just going to move on to Dr. Schmidt. Thank you so much. We can see your slides. We cannot hear you.
LIANE SCHMIDT: Can you hear me now?
CRISTINA CUSIN: Yes, thank you.
LIANE SCHMIDT: Thank you. So I'm Liane Schmidt. I'm an INSERM researcher and team leader at the Paris Brain Institute. And I'm working on placebo effects but understanding the appetitive side of placebo effects. And what I mean by that I will try to explain to you in the next couple of slides.
NIMH Staff: Can you turn on your video?
LIANE SCHMIDT: Sorry?
NIMH Staff: Can you please turn on your video, Dr. Schmidt?
LIANE SCHMIDT: Yeah, yes, yes, sorry about that.
So it's about the appetitive side of placebo effects because actually placebo effects on cognitive processes such as motivation and biases and belief updating because these processes actually play also a role when patients respond to treatment. And when we measure placebo effects, basically when placebo effects matter in the clinical setting.
And this is done at the Paris Brain Institute. And I'm working also in collaboration with the Pitie-Salpetriere Hospital Psychiatry department to get access to patients with depression, for example.
So my talk will be organized around three parts. On the first part, I will show you some data about appetitive placebo effects on taste pleasantness, hunger sensations and reward learning. And this will make the bridge to the second part where I will show you some evidence for asymmetrical learning biases that are more tied to reward learning and that could contribute actually or can emerge after fast-acting antidepressant treatment effects in depression.
And why is this important? I will try to link these two different parts, the first and second part, in the third part to elaborate some perspectives on the synergies between expectations, expectation updating through learning mechanisms, motivational mechanisms, motivational processes and drug experiences and which we can -- might harness actually by using computational models such as, for example, risk-reward Wagner models as Marta just showed you all the evidence for this in her work.
The appetitive side of placebo effects is actually known very well from the field of research in consumer psychology and marketing research where price labels, for example, or quality labels can affect decision-making processes and also experiences like taste pleasantness experience. And since we are in France, one of the most salient examples for these kind of effects comes from wine tasting. And many people have shown -- many studies have shown that basically the price of wine can influence how pleasant it tastes.
And we and other people have shown that this is mediated by activation in what is called the brain valuation systems or regions that encode expected and experienced reward. And one of the most prominent hubs in this brain valuation system is the ventral medial prefrontal cortex, what you see here on the SPM on the slide. That can explain, that basically translates these price label effects on taste pleasantness liking. And what is interesting is also that its sensitivity to monetary reward, for example, obtaining by surprise a monetary reward. It activates, basically the vmPFC activates when you obtain such a reward surprisingly.
And the more in participants who activate the vmPFC more in these kind of positive surprises, these are also the participants in which the vmPFC encoded more strongly the difference between expensive and cheap wines, which makes a nice parallel to what we know from placebo hyperalgesia where it has also been shown that the sensitivity of the reward system in the brain can moderate placebo analgesia with participants with higher reward sensitivity in the ventral striatum, for example, another region showing stronger placebo analgesia.
So this is to basically hope to let you appreciate that these effects parallel nicely what we know from placebo effects in the pain and also in disease. So we went further beyond actually, so beyond just taste liking which is basically experiencing rewards such as wine. But what could be -- could placebos also affect motivational processes per se? So when we, for example, want something more.
And one way to study is to study basic motivation such as, for example, hunger. It is long thought, for instance, eating behavior that is conceptualized to be driven by homeostatic markers, hormone markers such as Ghrelin and Leptin that signal satiety and energy stores. And as a function of these different hormonal markers in our blood, we're going to go and look for food and eat food. But we also know from the placebo effects on taste pleasantness that there is a possibility that our higher order beliefs about our internal states not our hormones can influence whether we want to eat food, whether we engage in these types of very basic motivations. And that we tested that, and other people also, that's a replication.
In the study where we gave healthy participants who came into the lab in a fasted state a glass of water. And we told them well, water sometimes can stimulate hunger by stimulating the receptors in your mouth. And sometimes you can also drink a glass of water to kill your hunger. And a third group, a control group was given a glass of water and told it's just water; it does nothing to hunger. And then we asked them to rate how hungry they feel over the course of the experiment. And it's a three-hour experiment. Everybody has fasted. And they have to do this food choice task in an fMRI scanner so they get -- everybody gets hungry over this three hours.
But what was interesting and what you see here on this rain cloud plot is that participants who believed or drank the water suggested to be a hunger killer increased in their hunger rating less than participants who believed the water will enhance their hunger. So this is a nice replication what we already know from the field; other people have shown this, too.
And the interesting thing is that it also affected this food wanting, this motivational process how much you want to eat food. So when people laid there in the fMRI scanner, they saw different food items, and they were asked whether they want to eat it or not for real at the end of the experiment. So it's incentive compatible. And what you see here is basically what we call stimulus value. So how much do you want to eat this food.
And the hunger sensation ratings that I just showed you before parallel what we find here. The people in the decreased hunger suggestion group wanted to eat the food less than in the increased hunger suggestion group, showing that it is not only an effect on subjective self-reports or how you feel your body signals about hunger. It's also about what you would actually prefer, what your subjective preference of food that is influenced by the placebo manipulation. And it's also influencing how your brain valuation system again encodes the value for your food preference. And that's what you see on this slide.
Slide two, you see the ventral medial prefrontal cortex. The yellow boxes that the more yellow they are, the stronger they correlate to your food wanting. And you see on the right side with the temporal time courses of the vmPFC that that food wanting encoding is stronger when people were on the increased hunger suggestion group than in the decreased hunger suggestion group.
So basically what I've showed you here is three placebo effects. Placebo effects on subjective hunger ratings, placebo effects on food choices, and placebo effects on how the brain encodes food preference and food choices. And you could wonder -- these are readouts. So these are behavioral readouts, neural readouts. But you could wonder what is the mechanism behind? Basically what is in between the placebo intervention here and basically the behavior feed and neural readout of this effect.
And one snippet of the answer to this question is when you look at the expectation ratings. For example, expectations have long been shown to be one of the mediators, the cognitive mediators of placebo effects across domains. And that's what we see here, too. Especially in the hunger killer suggestion group. The participants who believed that the hunger -- that the drug will kill their hunger more strongly were also those whose hunger increased less over the course of the experiment experience.
And this moderated activity in the region that you see here, which is called the medial prefrontal cortex, that basically activated when people saw food on the screen and thought about whether they want to eat it or not. And this region activated by that activity was positively moderated by the strength of the expectancy about the glass of water to decrease their hunger. So the more you expect that the water will decrease your hunger, the more the mPFC activates when you see food on the screen.
It's an interesting brain region because it's right between the ventral medial prefrontal cortex that encodes the value, the food preference, and the dorsal lateral prefrontal cortex. And it has been shown by past research to connect to the vmPFC when participants self-control, especially during food decision-making paradigms.
But another mechanism or another way to answer the question about the mechanism of how the placebo intervention can affect this behavior in neural effects is to use computational modelings to better understand the preference formation -- the preference formation basically. And one way is that -- is drift diffusion modeling. So these drift diffusion models come from perceptual research for understanding perception. And they are recently also used to better understand preference formation. And they assume that your preference for a yes or no food choice, for example, is a noisy accumulation of evidence.
And there are two types of evidence you accumulate in these two -- in these decision-making paradigms is basically how tasty and how healthy food is. How much you like the taste, how much you consider the health. And this could influence this loop of your evidence accumulation how rapidly basically you reach a threshold towards yes or no.
It could also be that the placebo and the placebo manipulation could influence this loop. But the model loops test several other hypotheses. It could be that the placebo intervention basically affected also just the threshold like that reflects how carefully you made the decision towards a yes or no choice. It could be your initial bias; that is, basically initially you were biased towards a yes or a no response. Or it could be the nondecision time which reflects more sensory motor integration.
And the answer to this question is basically that three parameters were influenced by the placebo manipulation. Basically how much you integrated healthiness and tastiness in your initial starting bias. So you paid more attention to the healthiness when you believed that you were on a hunger killer. And more the tastiness when you believed that you were on a hunger enhancer. And similarly, you were initially biased towards accepting food more when participants believed they were on a hunger enhancer than on a hunger killer.
Interestingly, so this basically shows that this decision-making process is biased by the placebo intervention and basically also how much you filter information that is most relevant. When you are hungry, basically taste is very relevant for your choices. When you are believing you are less hungry, then you have more actually space or you pay less attention to taste, but you can also pay attention more to healthiness of food.
And so the example that shows that this might be a filtering of expectation-relevant information is to use psychophysiologic interaction analyzers that look basically at the brain activity in the vmPFC, that's our seed region. Where in the brain does it connect when people, when participants see food on a computer screen and have to think about whether they want to eat this food or not?
And what we observed there that's connected to the dlPFC, the dorsal lateral prefrontal cortex region. And it's a region of interest that we localized first to be sure it is actually a region that is inter -- activating through an interference resolution basically when we filter -- have to filter information that is most relevant to a task in a separate Stroop localizer task.
So the vmPFC connects stronger to this dlPFC interference resolution region and this is moderated especially in the decreased hunger suggestion group by how much participants considered the healthiness against the tastiness of food.
To wrap this part up, it's basically that we replicated findings from previous studies about appetitive placebo effects by showing that expectancies about efficiency of a drink can affect hunger sensations. How participants make -- form their food preferences, make food choices. And value encoding in the ventral medial prefrontal cortex.
But we also provided evidence for underlying neurocognitive mechanisms that involve the medial prefrontal cortex that is moderated by the strengths of the hunger expectation. That the food choice formation is biased in the form of attention-filtering mechanism toward expectancy congruent information that is taste for an increased hunger suggestion group, and healthiness for a decreased hunger suggestion group. And this is implemented by regions that are linked to interference resolution but also to valuation preference encoding.
And so why should we care? In the real world, it is not very relevant to provide people with deceptive information about hunger-influencing ingredients of drinks. But studies like this one provide insights into cognitive mechanisms of beliefs about internal states and how these beliefs can affect the interoceptive sensations and also associated motivations such as economic choices, for example.
And this can actually also give us insights into the synergy between drug experiences and outcome expectations. And that could be harnessed via motivational processes. So translated basically via motivational processes. And then through it maybe lead us to better understand active treatment susceptibility.
And I'm going to elaborate on this in the next part of the talk by -- I'm going a little bit far, coming a little bit far, I'm not talking about or showing evidence about placebo effects. But yes -- before that, yes, so basically it is.
Links to these motivational processes have long been suggested actually to be also part of placebo effects or mechanisms of placebo effect. And that is called the placebo-reward hypothesis. And that's based on findings in Parkinson's disease that has shown that when you give Parkinson's patients a placebo but tell them it's a dopaminergic drug, then you can measure dopamine in the brain. And the dopamine -- especially the marker for dopamine, its binding potential decreases. That is what you see here on this PET screen -- PET scan results.
And that suggests that the brain must have released endogenous dopamine. And dopamine is very important for expectations and learning. Basically learning from reward. And clinical benefit is the kind of reward that patients expect. So it might -- it is possible that basically when a patient expects reward clinical benefit, its brain -- their brain releases dopamine in remodulating that region such as the vmPFC or the ventral striatum.
And we have shown this in the past that the behavioral consequence of such a nucleus dopamine release under placebo could be linked to reward learning, indeed. And what we know is that, for example, that Parkinson patients have a deficit in learning from reward when they are off dopaminergic medication. But this normalizes when they are under active dopaminergic medication.
So we wondered if based on these PET studies under placebo, the brain releases dopamine, does this also have behavior consequences on their reward learning ability. And that is what you see here on the screen on the right side on the screen is that the Parkinson patients basically tested on placebo shows similar reward learning abilities as under active drug.
And this again was also underpinned by increased correlation of the ventral medial prefrontal cortex. Again, this hub of the brain valuation system to the learned reward value. That was stronger in the placebo and active drug condition compared to baseline of drug condition.
And I want to make now this -- a link to another type of disease where also the motivation is deficitary, and which is depression. And depression is known to be maintained or is sympathized to be maintained by this triad of very negative beliefs about the world, the future and one's self. Which is very insensitive to belief disconfirming information, especially if the belief disconfirming information is positive, so favorable. And this has been shown by cognitive neuroscience studies to be reflected by a thought of like of good news/bad news bias or optimism biases and belief updating in depression. And this good news/bad news bias is basically a bias healthy people have to consider favorable information that contradicts initial negative beliefs more than negative information.
And this is healthy because it avoids reversing of beliefs. And it also includes a form of motivational process because good news have motivational salience. So it should be more motivating to update beliefs about the future, especially if these beliefs are negative, then when we learn that our beliefs are way too negative and get information about that disconfirms this initial belief. But depressed patients, they like this good news/bad news bias. So we wonder what happens when patients respond to antidepressant treatments that give immediate sensory evidence about being on an antidepressant.
And these new fast-acting antidepressants such as Ketamine, these types of antidepressants that patients know right away whether they got the treatment through dissociative experiences. And so could it be that this effect or is it a cognitive model of depression. So this was the main question of the study. And then we wondered again what is the computational mechanism. And is it linked again also, as shown in the previous studies, to reward learning mechanisms, so biased updating of beliefs. And is it linked to clinical antidepressant effects and also potentially outcome expectations makes the link to placebo effects.
So patients were given the -- were performing a belief updating task three times before receiving Ketamine infusions. And then after first infusion and then one week after the third infusion, each time, testing time we measured the depression with the Montgomery-Asberg Depression Rating Scale. And patients performed this belief updating task where they were presented with different negative life events like, for example, getting a disease, losing a wallet here, for example.
And they were asked to estimate their probability of experiencing this life event in the near future. And they were presented with evidence about the contingencies of this event in the general population, what we call the base rate. And then they had the possibility to update their belief knowing now the base rate.
And this is, for example, a good news trial where participants initially overestimated the chance for losing a wallet and then learn it's much less frequent than they initially thought. Updates, for example, 15%. And in a bad news trial, it's you initially underestimated your probability of experiencing this adverse life event. And if you have a good news/bad news bias, well, you're going to consider this information to a lesser degree than in a good news trial.
And that's what -- exactly what happens in the healthy controls that you see on the left most part of the screen. I don't know whether you can see the models, but basically we have the belief updating Y axis. And this is healthy age-matched controls to patients. And you can see updating of the good news. Updating of the bad news. We tested the participants more than two times within a week. You can see the bias. There is a bias that decreases a little bit with more sequential testing in the healthy controls. But importantly, in the patients the bias is there although before Ketamine treatment.
But it becomes much more stronger after Ketamine treatment. It emerged basically. So patients become more optimistically biased after Ketamine treatment. And this correlates to the MADRS scores. Patients who improve more with treatment are also those who show a stronger good news/bad news bias after one week of treatment.
And we wondered again about the computational mechanisms. So one way to get at this using a Rescoria-Wagner model reward reinforcement learning model that basically assumes that updating is proportional to your surprise which is called the estimation error.
The difference between the initial estimate and the base rate. And this is weighted by learning rate. And the important thing here is the learning rate has got two components, a scaling parameter and an asymmetry parameter. And the asymmetry parameter basically weighs in how much the learning rate varies after good news, after positive estimation error, than after negative estimation errors.
And what we can see that in healthy controls, there is a stronger learning rate for positive estimation errors and less stronger for negative estimation errors translating this good news/bad news bias. It's basically an asymmetrical learning mechanism. And in the patients, the asymmetrical learning is non-asymmetrical before Ketamine treatment. And it becomes asymmetrical as reflected in the learning rates after Ketamine treatment.
So what we take from that is basically that Ketamine induced an optimism bias. But an interesting question is whether -- basically what comes first. Is it basically the improvement in the depression that we measured with the Montgomery-Asberg Depression Rating Scale, or is it the optimism bias that emerged and that triggered basically. Since it's a correlation, we don't know what comes first.
And an interesting side effect or aside we put in the supplement was that in 16 patients, it's a very low sample size, the expectancy about getting better also correlated to the clinical improvement after Ketamine treatment. We have two expectancy ratings here about the efficiency about Ketamine and also what patients expect their intensity of depression will be after Ketamine treatment.
And so that suggested the clinical benefit is kind of in part or synergistically seems to interact with the drug experience that emerges that generates an optimism bias. And to test this more, we continued data collection just on the expectancy ratings. And basically wondered how the clinical improvement after first infusion links to the clinical improvement after third infusion.
And we know from here that patients improve after first infusion are also those that improved after a third infusion. But is it mediated by their expectancy about the Ketamine treatment? And that's what we indeed found is that basically the more patients expected to get better, the more they got better after one week of treatment. But it mediated this link between the first drug experience and the later drug experiences and suggested there might not be an additive effect as other panelist members today already put forward today, it might be synergetic link.
And one way to get at these synergies is basically again use computational models. And this idea has been around although yesterday that basically there could be self-fulfilling prophesies that could contribute to the treatment responsiveness and treatment adherence. And these self-fulfilling prophesies are biased symmetrically learning mechanisms that are more biased when you have positive treatment experiences, initial positive treatment experiences, and then might contribute how you adhere to the treatment in the long term and also how much you benefit from it in the long term. So it's both drug experience and an expectancy.
And so this is nonpublished work where we played with this idea basically using a reinforcement learning model. This is also very inspired by we know from placebo analgesia. Tor and Luana Kuven, they have a paper on showing that self-fulfilling prophecies can be harnessed with biased patient and reinforcement learning models. And the idea of these models is that there are two learning rates, alpha plus and alpha minus. And these learning rates rate differently into the updating of your expectation after drug experience.
LIANE SCHMIDT: Okay, yeah, I'm almost done.
So rate differently on these drug experiences and expectations as a function of whether the initial experience was congruent to your expectations. So a positive experience, then a negative one. And here are some simulations of this model. I'm showing this basically that your expectation is getting more updated the more bias, positively biased you are. Then when you are negatively biased. And these are some predictions of the model concerning depression improvement.
To wrap this up, the conclusion about this is that there seems to be asymmetrical learning that can capture self-fulfilling prophesies and could be a mechanism that translates expectations and drug experiences potentially across domains from placebo hypoalgesia to antidepressant treatment responsiveness. And the open question is obviously to challenge these predictions of these models more with empirical data in pain but also in mood disorders as Marta does and as we do also currently at Cypitria where we test the mechanisms of belief updating biases in depression with fMRI and these mathematical models.
And this has a direct link implication because it could help us to better understand how these fast-acting antidepressants work and what makes patients adhere to them and get responses to them. Thank you for your attention. We are the control-interoception-attention team. And thank you to all the funders.
CRISTINA CUSIN: Fantastic presentation. Thank you so much. Without further ado, let's move on to the next speaker. Dr. Greg Corder.
GREG CORDER: Did that work? Is it showing?
GREG CORDER: Awesome, all right. One second. Let me just move this other screen. Perfect. All right.
Hi, everyone. My name is Greg Corder. I'm an Assistant Professor at the University of Pennsylvania. I guess I get to be the final scientific speaker in this session over what has been an amazing two-day event. So thank you to the organizers for also having me get the honor of representing the entire field of preclinical placebo research as well.
And so I'm going to give a bit of an overview, some of my friends and colleagues over the last few years and then tell you a bit about how we're leveraging a lot of current neuroscience technologies to really identify the cell types and circuits building from, you know, the human fMRI literature that's really honed in on these key circuits for expectations, belief systems as well as endogenous antinociceptive symptoms, in particular opioid cell types.
So the work I'm going to show from my lab has really been driven by these two amazing scientists. Dr. Blake Kimmey, an amazing post-doc in the lab. As well as Lindsay Ejoh, who recently last week just received her D-SPAN F99/K00 on placebo circuitry. And we think this might be one of the first NIH-funded animal projects on placebo. So congratulations, Lindsay, if you are listening.
Okay. So why use animals, right? We've heard an amazing set of stories really nailing down the specific circuits in humans leveraging MRI, fMRI, EEG and PET imaging that give us this really nice roadmap and idea of how beliefs in analgesia might be encoded within different brain circuits and how those might change over times with different types of patient modeling or updating of different experiences.
And we love this literature. We -- in the lab we read it in depth as best as we can. And we use this as a roadmap in our animal studies because we can take advantage of animal models that really allow us to dive deep into the very specific circuits using techniques like that on the screen here from RNA sequencing, electrophysiology really showing that those functional measurements in fMRI are truly existent with the axons projecting from one region to another.
And then we can manipulate those connections and projections using things like optogenetics and chemogenetics that allow us really tight temporal coupling to turn cells on and off. And we can see the effects of that intervention in real time on animal behavior. And that's really the tricky part is we don't get to ask the animals do you feel pain? Do you feel less pain? It's hard to give verbal suggestions to animals.
And so we have to rely on a lot of different tricks and really get into the heads of what it's like to be a small prey animal existing in a world with a lot of large monster human beings around them. So we really have to be very careful about how we design our experiments. And it's hard. Placebo in animals is not an easy subject to get into. And this is reflected in the fact that as far as we can tell, there is only 24 published studies to date on placebo analgesia in animal models.
However, I think this is an excellent opportunity now to really take advantage of what has been the golden age of neuroscience technologies exploding in the last 10-15 years to revisit a lot of these open questions about when are opioids released, are they released? Can animals have expectations? Can they have something like a belief structure and violations of those expectations that lead to different types of predictions errors that can be encoded in different neural circuits. So we have a chance to really do that.
But I think the most critical first thing is how do we begin to behaviorally model placebo in these preclinical models. So I want to touch on a couple of things from some of my colleagues. So on the left here, this is a graph that has been shown on several different presentations over the past two days from Benedetti using these tourniquet pain models where you can provide pharmacological conditioning with an analgesic drug like morphine to increase this pain tolerance.
And then if it is covertly switched out for saline, you can see that there is an elevation in that pain tolerance reflective of something like a placebo analgesic response overall. And this is sensitive to Naloxone, the new opioid receptor antagonist, suggesting endogenous opioids are indeed involved in this type of a placebo-like response.
And my colleague, Dr. Matt Banghart, at UCSD has basically done a fantastic job of recapitulating this exact model in mice where you can basically use morphine and other analgesics to condition them. And so if I just kind of dive in a little bit into Matt's model here.
You can have a mouse that will sit on a noxious hot plate. You know, it's an environment that's unpleasant. You can have contextual cues like different types of patterns on the wall. And you can test the pain behavior responses like how much does the animal flick and flinch and lick and bite and protect itself to the noxious hot plate.
And then you can switch the contextual cues, provide an analgesic drug like morphine, see reductions in those pain behaviors. And then do the same thing in the Benedetti studies, you switch out the morphine for saline, but you keep the contextual cues. So the animal has effectively created a belief that when I am in this environment, when I'm in this doctor's office, I'm going to receive something that is going to reduce my perceptions of pain.
And, indeed, Matt sees a quite robust effect here where this sort of placebo response is -- shows this elevated paw withdrawal latency indicating that there is endogenous nociception occurring with this protocol. And it happens, again, pretty robustly. I mean most of the animals going through this conditioning protocol demonstrate this type of antinociceptive behavioral response. This is a perfect example of how we can leverage what we learn from human studies into rodent studies for acute pain.
And this is also really great to probe the effects of placebo in chronic neuropathic pain models. And so here this is Dr. Damien Boorman who was with Professor Kevin Key in Australia, now with Lauren Martin in Toronto.
And here Damien really amped up the contextual cues here. So this is an animal who has had an injury to the sciatic nerve with this chronic constriction injury. So now this animal is experiencing something like a tonic chronic neuropathic pain state. And then once you let the pain develop, you can have the animals enter into this sort of placebo pharmacological conditioning paradigm where animals will go onto these thermal plates, either hot or cool, in these rooms that have a large amount of visual tactile as well as odorant cues. And they are paired with either morphine or a controlled saline.
Again, the morphine is switched for saline on that last day. And what Damien has observed is that in a subset of the animals, about 30%, you can have these responder populations that show decreased pain behavior which we interpret as something like analgesia overall. So overall you can use these types of pharmacological conditionings for both acute and chronic pain.
So now what we're going to do in our lab is a bit different. And I'm really curious to hear the field's thoughts because all -- everything I'm about to show is completely unpublished. Here we're going to use an experimenter-free, drug-free paradigm of instrumental conditioning to instill something like a placebo effect.
And so this is what Blake and Lindsay have been working on since about 2020. And this is our setup in one of our behavior rooms here. Our apparatus is this tiny little device down here. And everything else are all the computers and optogenetics and calcium imaging techniques that we use to record the activity of what's going on inside the mouse's brain.
But simply, this is just two hot plates that we can control the temperature of. And we allow a mouse to freely explore this apparatus. And we can with a series of cameras and tracking devices plot the place preference of an animal within the apparatus. And we can also record with high speed videography these highly conserved sort of protective recuperative pain-like behaviors that we think are indicative of the negative affect of pain.
So let me walk you through our little model here real quick. Okay. So we call this the placebo analgesia conditioning assay or PAC assay. So here is our two-plate apparatus here. So plate number one, plate number two. And the animal can always explore whichever plate it wants. It's never restricted to one side. And so we have a habituation day, let the animal familiarize itself. Like oh, this is a nice office, I don't know what's about to happen.
And then we have a pretest. And in this pretest, importantly, we make both of these plates, both environments a noxious 45-degree centigrade. So this will allow the animal to form an initial expectation that the entire environment is noxious and it's going to hurt. So both sides are noxious. Then for our conditioning, this is where we actually make one side of the chamber non-noxious. So it's just room temperature. But we keep one side noxious. So now there is a new expectation for the animal that it learns that it can instrumentally move its body from one side to the other side to avoid and escape feeling pain.
And so we'll do this over three days, twice per day. And then on our post tester placebo day we make both environments hot again. So now we'll start the animal off over here and the animals will get to freely choose do they want to go to the side that they expect should be non-noxious? Or what happens? So what happens?
Actually, if you just look at the place preference for this, over the course of conditioning we can see that the animals will, unsurprisingly, choose the environment that is non-noxious. And they spend 100% of their time there basically. But when we flip the plates or flip the conditions such that everything is noxious on the post test day, the animals will still spend a significant amount of time on the expected analgesia side. So I'm going to show you some videos here now and you are all going to become mouse pain behavior experts by the end of this.
So what I'm going to show you are both side by side examples of conditioned and unconditioned animals. And try to follow along with me as you can see what the effect looks like. So on this post test day. Oh, gosh, let's see if this is going to -- here we go. All right. So on the top we have the control animal running back and forth. The bottom is our conditioned animal.
And you'll notice we start the animal over here and it's going to go to the side that it expects it to not hurt. Notice the posture of the animals. This animal is sitting very calm. It's putting its entire body down on the hot plate. This animal, posture up, tail up. It's running around a little bit frantically. You'll notice it start to lick and bite and shake its paws. This animal down here might have a couple of flinches so it's letting you know that some nociception is getting into the nervous system overall.
But over the course of this three-minute test, the animals will rightly choose to spend more time over here. And if we start to quantify these types of behaviors that the animals are doing in both conditions, what we find is that there is actually a pretty significant reduction in these nociceptive behaviors. But it's not across the entire duration of this placebo day or post test day.
So this trial is three minutes long. And what we see is that this antinociceptive and preference choice only exists for about the first 90 seconds of this assay. So this is when the video I just showed, the animal goes to the placebo side, it spends a lot of its time there, does not seem to be displaying pain-like behaviors.
And then around 90 seconds, the animal -- it's like -- it's almost like the belief or the expectation breaks. And at some point, the animal realizes oh, no, this is actually quite hot. It starts to then run around and starts to show some of the more typical nociceptive-like behaviors. And we really like this design because this is really, really amenable to doing different types of calcium imaging, electrophysiology, optogenetics because now we have a really tight timeline that we can observe the changing of neural dynamics at speeds that we can correlate with some type of behavior.
Okay. So what are those circuits that we're interested in overall that could be related to this form of placebo? Again, we like to use the human findings as a wonderful roadmap. And Tor has demonstrated, and many other people have demonstrated this interconnected distributed network involving prefrontal cortex, nucleus accumbens, insula, thalamus, as well as the periaqueductal gray.
And so today I'm going to talk about just the periaqueductal gray. Because there is evidence that there is also release of endogenous opioids within this system here. And so we tend to think that the placebo process and the encoding, whatever that is, the placebo itself is likely not encoded in the PAG. The PAG is kind of the end of the road. It's the thing that gets turned on during placebo and we think is driving the antinociceptive or analgesic effects of the placebo itself.
So the PAG, for anyone who's not as familiar, we like it because it's conserved across species. We look at in a mouse. There's one in a human. So potentially it's really good for translational studies as well. It has a very storied past where it's been demonstrated that the PAG subarchitecture has these beautiful anterior to posterior columns that if you electrically stimulate different parts of PAG, you can produce active versus passive coping mechanisms as well as analgesia that's dependent on opioids as well as endocannabinoids.
And then the PAG is highly connected. Both from ascending nociception from the spinal cord as well as descending control systems from prefrontal cortex as well as the amygdala. So with regard to opioid analgesia. If you micro infuse morphine into the posterior part of the PAG, you can produce an analgesic effect in rodents that is across the entire body. So it's super robust analgesia from this very specific part of the PAG.
If you look at the PAG back there and you do some of these techniques to look for histological indications that the mu opioid receptor is there, it is indeed there. There is a large amount of mu opioid receptors, it's OPRM1. And it's largely on glutamatergic neurons. So the excitatory cells, not the inhibitory cells. They are on some of them.
And as far as E-phys data goes as well, we can see that the mu opioid receptor is there. So DAMGOs and opioid agonist. We can see activation of inhibitory GIRK currents in those cells. So the system is wired up for placebo analgesia to happen in that location. Okay. So how are we actually going to start to tease this out? By finding these cells where they go throughout the brain and then understanding their dynamics during placebo analgesia.
So last year we teamed up with Karl Deisseroth's lab at Stanford to develop a new toolkit that leverages the genetics of the opioid system, in particular the promoter for the mu opioid receptor. And we were able to take the genetic sequence for this promoter and package it into adeno associated viruses along with a range of different tools that allow us to turn on or turn off cells or record their activity. And so we can use this mu opioid receptor promoter to gain genetic access throughout the brain or the nervous system for where the mu opioid receptors are. And we can do so with high fidelity.
This is just an example of our mu opioid virus in the central amygdala which is a highly mu opioid specific area. But so Blake used this tool using the promoter to drive a range of different trans genes within the periaqueductal gray. And right here, this is the G camp. So this is a calcium indicator that allows us to in real time assess the calcium activity of PAG mu opioid cells.
And so what Blake did was he took a mouse, and he recorded the nociceptive responses within that cell type and found that the mu opioid cell types are actually nociceptive. They respond to pain, and they do so with increasing activity to stronger and stronger and more salient and intense noxious stimuli. So these cells are actually nociceptive.
And if we look at a ramping hot plate, we can see that those same mu opioid cell types in the PAG increase the activity as this temperature on this hot plate increases. Those cells can decrease that activity if we infuse morphine.
Unsurprisingly, they express the mu opioid receptor and they're indeed sensitive to morphine. If we give naltrexone to block the mu opioid receptors, we can see greater activity to the noxious stimuli, suggesting that there could be an opioid tone or some type of an endogenous opioid system that's keeping this system in check, that it's repressing its activity. So when we block it, we actually enhance that activity. So it's going to be really important here. The activity of these mu opioid PAG cells correlates with affective measures of pain.
When animals are licking, shaking, biting, when it wants to escape away from noxious stimuli, that's when we see activity within those cells. So this is just correlating different types of behavior when we see peak amplitudes within those cell types. So let me skip that real quick.
Okay. So we have this ability to look and peek into the activity of mu opioid cell types. Let's go back to that placebo assay, our PAC assay I mentioned before. If we record from the PAG on that post test day in an animal that has not undergone conditioning, when the plates are super hot, we see a lot of nocioceptive activity in these cells here. They're bouncing up and down.
But if we look at the activity of the nociception in an animal undergoing placebo, what we see is there's a suppression of neural activity within that first 90 seconds. And this actually does seem to extinguish within the lighter 90 seconds. So kind of tracks along with the behavior of those animals. When they're showing anti nocioceptive behavior, that's when those cells are quiet.
When the pain behavior comes back, that's when those cell types are ramping up. But what about the opioids too? Mu opioid receptor cell type's decreasing activity. What about the opioids themselves here? The way to do this in animals has been to use microdialysis, fantastic technique but it's got some limitations to it. This is a way of sampling peptides in real time and then using liquid chromatography to tell if the protein was present. However, the sampling rate is about 10 minutes.
And in terms of the brain processing, 10 minutes might as well be an eternity. If we're talking about milliseconds here. But we want to know what these cells here and these red dots are doing. These are the enkephaliner cells in the PAG. We needed revolution in technologies. One of those came several years ago from Dr. Lin Tian, who developed some of the first sensors for dopamine. Some of you may have heard of it. It's called D-Light.
This is a version of D-Light. But it's actually an enkephalin opioid sensor. What Lin did to genetically engineer this is to take the delta opioid receptor, highly select it for enkephalin, and then link it with this GFP molecule here such that when enkephalin binds to the sensor it will fluoresce.
We can capture that florescence with microscopes that we implant over the PAG and we can see when enkephalin is being released with subsecond resolution. And so what we did for that is we want to see if enkephalin is indeed being released onto those mu opioid receptor expressing pain encoding neurons in the PAG. What I showed you before is that those PAG neurons, they ramp up their activity as the nociception increases, a mouse standing on a hot plate. We see nociception ramp up. What do you all think happened with the opoids?
It wasn't what we expected. It actually drops. So what we can tell is that there's a basal opioid tone within the PAG, but that as nociception increases, acute nociception, we see a decrease suppression of opioid peptide release.
We think this has to do with stuff that Tor has published on previously that the PAG is more likely involved in updating prediction errors. And this acute pain phenomenon we think is reflective of the need to experience pain to update your priors about feeling pain and to bias the selection of the appropriate behaviors, like affect related things to avoid pain. However, what happens in our placebo assay?
We actually see the opposite. So if we condition animals to expect pain relief within that PAC assay, we actually see an increase from the deltoid sensor suggesting that there is an increase in enkephalin release post conditioning. So there can be differential control of the opioid system within this brain region. So this next part is the fun thing you can do with animals. What if we just bypassed the need to do the placebo assay?
If we know that we just need to cause release of enkephalin within the PAG to produce pain relief, we could just directly do that with optigenetics. So we tried to us this animal that allows us to put a red light sensitive opsin protein into the enkephalinergic interneurons into the PAG.
When we shine red light on top of these cells, they turn on and they start to release their neurotransmitters. These are GABAergic and enkephalinergic. So they're dumping out GABA and now dumping out enkephalin into the ERG. We can visualize that using the Delta Light sensor from Lin Tien.
So here is an example of optogenetically released enkephalin within the PAG over 10 minutes. The weird thing that we still don't fully understand is that this signal continues after the optogenetic stimulation. So can we harness the placebo effect in mice? At least it seems we can. So if we turn on these cells strongly, cause them to release enkephalin and put animals back on these ramping hot plate tests we don't see any changes in the latency to detect pain, but we see specific ablation or reductions in these affective motivational pain like behaviors overall. Moderator: You have one minute remaining.
GREGORY CORDER: Cool. In this last minutes, people are skeptical. Can we actually test these higher order cognitive processes in animals? And for anyone who is not a behavioral preclinical neural scientist, you might not be aware there's an absolute revolution happening in behavior with the use of deep learning modules that can precisely and accurately quantify animal behavior. So this is an example of a deep learning tracking system.
We've built the Light Automated Pain Evaluator that can capture a range of different pain related behaviors fully automated without human intervention whatsoever that can be paired with brain reporting techniques like calcium imaging, that allow us to fit a lot of different computational models to understand what the activity of single neurons might be doing, let's say, in the cingulate cortex that might be driving that placebo response.
We can really start to tie now in at single cell resolution the activity of prefrontal cortex to drive these placebo effects and see if that alters anti nocioceptive behavior endogenously. I'll stop there and thank all the amazing people, Blake, Greg, and Lindsay, who did this work, as well as all of our funders and the numerous collaborators who have helped us do this. So thank you.
CRISTINA CUSIN: Terrific talk. Thank you so much. We're blown away. I'll leave the discussion to our two moderators. They're going to gather some of the questions from the chat and some of their own questions for all the presenters from today and from yesterday as well.
TED KAPTCHUK: Matt, you start gathering questions. I got permission to say a few moments of comments. I wanted to say this is fantastic. I actually learned an amazing amount of things. The amount of light that was brought forward about what we know about placebos and how we can possibly control placebo effects, how we can possibly harness placebo effects.
There was so much light and new information. What I want to do in my four minutes of comments is look to the future. What I mean by that is -- I want to give my comments and you can take them or leave them but I've got a few minutes.
What I want to say is we got the light, but we didn't put them together. There's no way we could have. We needed to be more in the same room. How does this fit in with your model? It's hard to do. What I mean by putting things together is I'll give you an example. In terms of how do we control placebo effects in clinical trials. I not infrequently get asked by the pharmaceutical industry, when you look at our placebo data -- we just blew it. Placebo was good as or always as good as the drug.
And the first thing I say is I want to talk to experts in that disease. I want to know the natural history. I want to know how you made your entry criteria so I can understand regression to the mean.
I want to know what's the relationship of the objective markers and subjective markers so I can begin to think about how much is the placebo response. I always tell them I don't know. If I knew how to reduce -- increase the difference between drug and placebo I'd be a rich man, I wouldn't be an academic. What I usually wind up saying is, get a new drug. And they pay me pretty well for that. And the reason is that they don't know anything about natural history. We're trying to harness something, and I just want to say -- I've done a lot of natural history controls, and that's more interesting than the rest of the experiments because they're unbelievable, the amount of improvement people show entering the trial without any treatment.
I just want to say we need to look at other things besides the placebo effect. We want to control the placebo response in a randomized control trial. I want to say that going forward. But I also want to say that we need a little bit of darkness. We need to be able to say, you know, I disagree with you. I think this other data, and one of the things I've learned doing placebo reach there's a paper that contradicts your paper real quickly and there's lots of contradictory information. It's very easy to say you're wrong, and we don't say it enough.
I want to take one example -- please forgive me -- I know that my research could be said that, Ted, you're wrong. But I just want to say something. Consistently in the two days of talk everyone talks about the increase of the placebo response over time. No one refers to the article published in 2022 in BMJ, first author was Mark Stone and senior author was Irving Kirsch. And they analyzed all FDA Mark Stone is in the Division of Psychiatry at CDER at the FDA. They analyzed all data of placebo controlled trials in major depressive disorder. They had over 230 trials, way more than 70,000 patients, and they analyzed the trend over time, in 1979 to the present, the publication. There was no increase in the placebo effect.
Are they right or are other people right? Nothing is one hundred percent clear right now and we need to be able to contradict each other when we get together personally and say, I don't think that's right, maybe that's right. I think that would help us. And the last thing I want to say is that some things were missing from the conference that we need to include in the future. We need to have ethics. Placebo is about ethics. If you're a placebo researcher in placebo controlled trials, that's an important question:
What are we talking about in terms of compromising ethics? There's no discussion that we didn't have time but in the future, let's do that.
And the last thing I would say is, we need to ask patients what their experience is. I've got to say I've been around for a long time. But the first time I started asking patients what their experiences were, they were in double blind placebo or open label placebo, I did it way after they finished the trial, the trial was over, and I actually took notes and went back and talked to people. They told me things I didn't even know about. And we need to have that in conferences. What I want to say, along those lines, is I feel so much healthier because I'm an older person, and I feel with this younger crowd here is significantly younger than me.
Maybe Matt and I are the same age, I don't know, but I think this is really one of the best conferences I ever went to. It was real clear data. We need to do lots of other things in the future. So with that, Matt, feed me some questions.
MATTHEW RUDORFER: Okay. Thanks. I didn't realize you were also 35. But okay. [LAUGHTER].
MATTHEW RUDORFER: I'll start off with a question of mine. The recent emergence of intravenous ketamine for resistant depression has introduced an interesting methodologic approach that we have not seen in a long time and that is the active placebo. So where the early trials just used saline, more recently we have seen benzodiazapine midazolam, while not mimicking really the full dissociative effect that many people get from ketamine, but the idea is for people to feel something, some kind of buzz so that they might believe that they're on some active compound and not just saline. And I wonder if the panel has any thoughts about the merits of using an active placebo and is that something that the field should be looking into more?
TED KAPTCHUK: I'm going to say something. Irving Kirsch published a meta analysis of H studies that used atropine as a control in depression studies. He felt that it made it difficult to detect a placebo drug difference. But in other meta analysis said that was not true. That was common in the '80s. People started thinking about that. But I have no idea how to answer your question.
MICHAEL DETKE: I think that's a great question. And I think in the presentations yesterday about devices, Dr. Lisanby was talking about the ideal sham. And I think it's very similar, the ideal active placebo would have none of the axia of the drug, of the drug in question, but would have, you know, exactly the same side effects and all other features, and of course that's attractive, but of course we probably would never have a drug that's exactly like that. I think midazolam was a great thing to try with ketamine. It's still not exactly the same. But I'd also add that it's not black and white. It's not like we need to do this with ketamine and ignore it for all of our other drugs. All of our drugs have side effects.
Arguably, if you do really big chunks, like classes of relatively modern antidepressants, antipsychotics and the psychostimulants, those are in order of bigger effect sizes in clinical trials, psychostimulants versus anti psychotics, versus -- and they're also in the order of roughly, I would argue, of unblinding, of functional unblinding. And in terms of more magnitude, Zyprexa will make you hungry. And also speed of onset of some of the adverse effects, stimulants and some of the Type II -- the second generation and beyond -- anti psychotics, they have pretty noticeable side effects for many subjects and relatively rapidly. So I think those are all important features to consider.
CRISTINA CUSIN: Dr. Schmidt?
LIANE SCHMIDT: I think using midazolam could give, like, some sensory sensations so the patients actually can say there's some effect on the body like immediately. But this raises actually a question whether these dissociations we observe in some patients of ketamine infusions we know have, will play a role for the antidepressant response. It's still an open question. So I don't have the answer to that question. And I think with midazolam doesn't really induce dissociations. I don't know, maybe you can isolate the dissociations you get on ketamine. But maybe even patients might be educated, expecting scientific reaction experiences and basically when they don't have -- so they make the midazolam experience something negative. So yeah, just self fulfilling prophesies might come into play.
CRISTINA CUSIN: I want to add for five seconds. Because I ran a large ketamine clinic. We know very little about cyto placebo maintaining an antidepressant response while the dissociation often wears off over time. It's completely separate from the anti depressant effect. We don't have long term placebo studies. The studies are extremely short lived and we study the acute effect. But we don't know how to sustain or how to maintain, what's the role of placebo effect in long term treatments. So that's another field that really is open to investigations. Dr. Rief.
WINFRIED RIEF: Following up on the issue of active placebos. I just want to mention that we did a study comparing active placebos to passive placebos and showing that active placebos are really more powerful. And I think the really disappointing part of this news is that it questions the blinding of our typical RCTs comparing antidepressants versus placebos because many patients who are in the active group or the tracked group, they perceive these onset effects and this will further boost the placebo mechanisms in the track group that are not existing in the passive placebo group. This is a challenge that further questions the validity of our typical RCTs.
CRISTINA CUSIN: Marta.
MARTA PECINA : Just a quick follow up to what Cristina was saying, too, that we need to clarify whether we want to find an active control for the dissociative effects or for the antidepressive effects. I think the approach will be very different. And this applies to ketamine but also psychodelics because we're having this discussion as well. So when thinking about how to control for or how to blind or how we just -- these treatments are very complicated. They have multiple effects. We just need to have the discussion of what are we trying to blind because the mechanism of action of the blinding drug will be very different.
TED KAPTCHUK: Can I say something about blinding? Robertson, who is the author of the 1970 -- no -- 1993 New England Journal paper saying that there's no that the placebo effect is a myth.
In 2022, published in BMJ, the largest -- he called it a mega meta analysis on blinding. And he took 144 randomized control trials that included nonblinded evidence on the drug versus blinded evidence of the drug. I'm not going to tell you the conclusion because it's unbelievable. But you should read it because it really influences -- it would influence what we think about blinding. That study was just recently replicated on a different set of patients with procedures in JAMA Surgery three months ago. And blinding like placebo is more complicated than we think. That's what I wanted to say.
MATTHEW RUDORFER: Another clinical factor that's come up during our discussion has been the relationship of the patient to the provider that we saw data showing that a warm relationship seemed to enhance therapeutic response, I believe, to most interventions. And I wonder what the panel thinks about the rise on the one hand of shortened clinical visits now that, for example, antidepressants are mostly given by busy primary care physicians and not specialists and the so called med check is a really, kind of, quickie visit, and especially since the pandemic, the rise of telehealth where a person might not ever even meet their provider in person, and is it possible we're on our way to where a clinical trial could involve, say, mailing medication every week to a patient, having them do their weekly ratings online and eliminating a provider altogether and just looking at the pharmacologic effect?
I mean, that probably isn't how we want to actually treat people clinically, but in terms of research, say, early phase efficacy, is there merit to that kind of approach?
LUANA COLLOCA: I'll comment on this, Dr. Rudorfer. We're very interested to see how the telemedicine or virtual reality can affect placebo effects, and we're modeling in the lab placebo effects induced via, you know, in person interaction.
There's an Avatar and virtual reality. And actually we found placebo effects with both the settings. Or whether, when we look at empathy, the Avatar doesn't elicit any empathy in the relationship. We truly need the in person connection to have empathy. So that suggests that our outcome that are affected by having in person versus telemedicine/para remote interactions, but yet the placebo effects persist in both the settings. The empathy is differently modulated and the empathy mediated, interestingly in our data, placebo effects only in the in person interactions. There is still a value in telemedicine. Effects that bypass empathy completely in competence.
MATTHEW RUDORFER: Dr. Hall.
KATHRYN HALL: Several of the large studies, like the Women's Health Study, Physicians' Health Study and, more recently, Vital, they did exactly that, where they mail these pill packs. And I mean, the population, obviously, is clinicians. So they are very well trained and well behaved. And they follow them for years but there's very little contact with the providers, and you still have these giant -- I don't know if you can call them placebo effects -- but certainly many of these trials have not proven to be more effective, the drugs they're studying, than placebo.
MATTHEW RUDORFER: Dr. Atlas.
LAUREN ATLAS: I wanted to chime in briefly on this important question. I think that the data that was presented yesterday in terms of first impressions of providers is relevant for this because it suggests that even when we use things like soft dot (phonetic) to select physicians and we have head shots (phonetic), that really we're making these decisions about who to see based on these kinds of just first impressions and facial features and having the actual interactions by providers is critical for sort of getting beyond that kind of factor that may drive selection. So I think if we have situations where there's reduced chances to interact, first of all, people are bringing expectations to the table based on what they know about the provider and then you don't really have the chance to build on that without the actual kind of therapeutic alliance. That's why I think, even though our study was done in an artificial setting, it really does show how we make these choices when there are bios for physicians and things available for patients to select from. I think there's a really important expectation being brought to the table before the treatment even occurs.
MATTHEW RUDORFER: Thanks. Dr. Lisanby.
SARAH “HOLLY” LISANBY: Thanks for raising this great question, Matt. I have a little bit of a different take on it. Equity in access to mental health care is a challenge. And the more that we can leverage technology to provide and extend the reach of mental health care the better. And so telemedicine and telepsychiatry, we've been thrust into this era by the pandemic but it existed before the pandemic as well. And it's not just about telepsychotherapy or teleprescription from monitoring pharmacotherapy, but digital remote neuromodulation is also a thing now. There are neuromodulation interventions that can be done at home that are being studied, and so there have been trials on transcranial direct current stimulation at home with remote monitoring. There are challenges in those studies differentiating between active and sham. But I think you're right in that we may have to rethink how do we control remote studies when the intensity of the clinician contact is very different, but I do think that we should explore these technologies so that we can extend the reach and extend access to research and to care for people who are not able to come into the research lab setting.
TED KAPTCHUK: May I add something on this? It's also criticizing myself. In 2008, I did this very nice study showing you could increase the doctor/patient relationship. And as you increase it, the placebo effect got bigger and bigger, like a dose response. A team in Korea that I worked with replicated that. I just published that replication.
The replication came out with the exact opposite results. The less doctor/patient relationship, the less intrusive, the less empathy got better effects. We're dealing with very complicated culturally constructed issues, and I just want to put it out there, the sand is soft. I'm really glad that somebody contradicted a major study that I did.
LUANA COLLOCA: Exactly. The central conference is so critical, what we observed in one context in one country, but even within the same in group or out group can be completely different in Japan, China or somewhere else. So the Americas, South Africa. So we need larger studies and more across country collaborations.
MATTHEW RUDORFER: Dr. Schmidt.
LIANE SCHMIDT: I just wanted to raise a point not really like -- it's more like a comment, like there's also very interesting research going on in the interactions between humans and robots, and usually humans treat robots very badly. And so I wonder what could be like -- here we focus on very human traits, like empathy, competence, what we look at. But when it comes to artificial intelligence, for example, and when we have to interact with algorithms, basically, like all these social interactions might completely turn out completely different, actually, and all have different effects on placebo effects. Just a thought.
MATTHEW RUDORFER: Dr. Rief.
WINFRIED RIEF: Yesterday, I expressed a belief for showing more warmth and competence, but I'll modify it a little bit today because I think the real truth became quite visible today, and that is that there is an interaction between these non specific effect placebo effects and the track effect. In many cases, at least. We don't know whether there are exceptions from this rule, but in many cases we have an interaction. And to learn about the interaction, we instead need study designs that modulate track intake versus placebo intake, but they also modulate the placebo mechanisms, the expectation mechanisms, the context of the treatment. And only if we have these 2 by 2 designs, modulating track intake and modulating context and psychological factors, then we learn about the interaction. You cannot learn about the interaction if you modulate only one factor.
And, therefore, I think what Luana and others have said that interact can be quite powerful and effective in one context but maybe even misleading in another context. I think this is proven. We have to learn more about that. And all the studies that have been shown from basic science to application that there could be an interaction, they're all indicating this line and to this necessity that we use more complex designs to learn about the interaction.
MATTHEW RUDORFER: Yes. And the rodent studies we've seen, I think, have a powerful message for us just in terms of being able to control a lot of variables that are just totally beyond our control in our usual human studies. It always seemed to me, for example, if you're doing just an antidepressant versus placebo trial in patients, well, for some people going into the clinic once a week to get ratings, that might be the only day of the week that they get up and take a shower, get dressed, have somebody ask them how they're doing, have some human interaction. And so showing up for your Hamilton rating could be a therapeutic intervention that, of course, we usually don't account for in the pharmacotherapy trial. And the number of variables really can escalate in a hurry when we look at our trials closely.
TED KAPTCHUK: Tor wants to say something.
TOR WAGER: Thanks, Ted.
I wanted to add on to the interaction issue, which came up yesterday, which Winfried and others just commented on, because it seems like it's really a crux issue. If the psychosocial or expectation effects and other things like that are entangled with specific effects so that one can influence the other and they might interact, then, yeah, we need more studies that independently manipulate specific drug or device effects and other kinds of psychological effects independently. And I wanted to bring this back up again because this is an idea that's been out here for a long time. I think the first review on this was in the '70s, like '76 or something, and it hasn't really been picked up for a couple of reasons. One, it's hard to do the studies. But second, when I talk to people who are in industry and pharma, they are very concerned about changing the study designs at all for FDA approval.
And since we had some, you know, FDA and regulatory perspectives here yesterday, I wanted to bring that up and see what people think, because I think that's been a big obstacle. And if it is, then that may be something that would be great for NIH to fund instead of pharma companies because then there's a whole space of drugs, psychological or neurostimulation psychological interactions, that can be explored.
MATTHEW RUDORFER: We also had a question. Yesterday there was discussion in a naloxone trial in sex differences in placebo response. And wonder if there's any further thoughts on studies of sex differences or diversity in general in placebo trials. Yes.
LUANA COLLOCA: We definitely see sex differences in placebo effect, and I show also, for example, women responded to arginine vasopressin in a way that we don't observe in men.
But also you asked about diversity. Currently actually in our paper just accepted today where we look at where people are living, the Maryland states, and even the location where they are based make a difference in placebo effects. So people who live in the most distressed, either the greatest Baltimore area, tended to have lower placebo effects as compared to a not distressful location. And we define that the radius of the criteria and immediately it's a race but we take into account the education, the income and so on. So it is interesting because across studies consistently we see an impact of diversity. And in that sense, I echo, listen to the comment that we need to find a way to reach out to these people and truly improve access and the opportunity for diversity. Thank you for asking.
MATTHEW RUDORFER: Thank you. Another issue that came up yesterday had to do with the pharmacogenomics. And there was a question or a question/comment about using candidate approaches and are they problematic.
KATHRYN HALL: What approaches.
MATTHEW RUDORFER: Candidate genes.
KATHRYN HALL: I think we have to start where we are. I think that the psychiatric field has had a really tough time with genetics. They've invested a lot and, sadly, don't have as much to show for it as they would like to. And I think that that has really tainted this quest for genetic markers of placebo and related studies, these interaction factors. But it's really important to not, I think, to use that to stop us from looking forward and identifying what's there. Because when you start to scratch the surface, there are interactions. You can see them. They're replete in the literature. And what's really fascinating is everybody who finds them, they don't see them when they report their study. And even some of these vasopressin studies, not obviously, Tor, yours, but I was reading one the other day where they had seen tremendous differences by genetics in response to arginine vasopressin. And they totally ignored what they were seeing in placebo and talked about who responds to drug. And so I think that not only do we need to start looking for what's happening, we need to start being more open minded and paying attention to what we're seeing in the placebo arm and accounting for that, taking that into account to understand what we're seeing across a trial in total.
CRISTINA CUSIN: I'll take a second to comment on sufficient selection and trying to figure out, depending on the site who are the patients who went there, treatment and depression clinical trial. If we eliminate from the discussion professional patient and we think about the patients who are more desperate, patients who don't have access to care, patients who are more likely to have psychosocial stressors or the other extreme, there are patients who are highly educated. The trials above and they search out, but they're certainly not representative of the general populations we see in the clinical setting.
They are somewhat different. And then if you think about the psychedelics trial, they go from 5,000 patients applying for a study and the study ends up recruiting 20, 30. So absolutely not representative of the general population we see in terms of diversity, in terms of comorbidities, in terms of psychosocial situations. So that's another factor that adds to the complexity of differentiating what happens in the clinical setting versus artificial setting like a research study. Tor.
MATTHEW RUDORFER: The question of who enters trials and I think the larger issue of diagnosis in general has, I think, really been a challenge to the field for many years. Ted and I go back a ways, and just looking at depression, of course, has dominated a lot of our discussion these last couple of days, with good reason. Now I realize the good database, my understanding is that the good database of placebo controlled trials go back to the late '90s, is what we heard yesterday. And if you go back further, the tricyclic era not only dealt with different medications, which we don't want to go back to, but if you think about practice patterns then, on the one hand, the tricyclics, most nonspecialists steered clear of, they required a lot of hands on. They required titration slowly up. They had some concerning toxicities, and so it was typical that psychiatrists would prescribe them but family docs would not. And that also had the effect of a naturalistic screening, that is, people would have to reach a certain level of severity before they were referred to a psychiatrist to get a prescription for medication.
More mildly ill people either wound up, probably inappropriately, on tranquilizers or no treatment at all and moderately to severely ill people wound up on tricyclics, and of course inpatient stays were common in those days, which again was another kind of screening. So it was the sort of thing, I mean, in the old days I heard of people talk about, well, you could, if you go to the inpatient board, you could easily collect people to be in clinical trial and you kind of knew that they were vetted already. That they had severe depression, the general sense was that the placebo response would be low. Though there's no real evidence for that. But the thing is, once we had the SSRIs on the one hand, the market vastly expanded because they're considered more broad spectrum. People with milder illness and anxiety disorders now are appropriate candidates and they're easier to dispense. The concern about overdose is much less, and so they're mostly prescribed by nonspecialists. So it's the sort of thing where we've seen a lot of large clinical trials where it doesn't take much to reach the threshold for entry, being if I go way back and this is just one of my personal concerns over many years the finer criteria, which I think were the first good set of diagnostic criteria based on data, based on literature, those were published in 1972 to have a diagnosis of major depression, called for four weeks of symptoms. Actually, literally, I think it said one month.
DSM III came out in 1980 and it called for two weeks of symptoms. I don't know -- I've not been able to find any documentation of how the one month went to two weeks, except that the DSM, of course, is the manual that's used in clinical practice. And you can understand, well, you might not want to have too high a bar to treat people who are seeking help. But I think one of the challenges of DSM, it was not meant as a research manual. Though that's often how it's used. So ever since that time, those two weeks have gotten reified, and so my point is it doesn't take much to reach diagnostic criteria for DSM, now, 5TR, major depression. So if someone is doing a clinical trial of an antidepressant, it is tempting to enroll people who meet, honestly meet those criteria but the criteria are not very strict. So I wonder whether that contributes to the larger placebo effect that we see today.
End of soapbox. The question -- I'd like to revisit an excellent point that Dr. Lisanby raised yesterday which has to do with the research domain criteria, the RDOC criteria. I don't know if anyone on the panel has had experience in using that in any trials and whether you see any merit there. Could RDOC criteria essentially enrich the usual DSM type clinical criteria in terms of trying to more finely differentiate subtypes of depression, might respond differently to different treatments.
MODERATOR: I think Tor has been patient on the hand off. Maybe next question, Tor, I'm not sure if you had comments on previous discussion.
TOR WAGER: Sure, thanks. I wanted to make a comment on the candidate gene issue. And I think it links to what you were just saying as well, doctor, in a sense. I think it relates to the issue of predicting individual differences in placebo effects and using that to enhance clinical trials, which has been really sort of a difficult issue. And in genetics, I think what's happened, as many of us know, is that there were many findings on particular candidate genes, especially comped and other particular set of genes in Science and Nature, and none of those really replicated when larger GWA studies started being done. And the field of genetics really focused in on reproducibility and replicability in one of our sample sizes. So I think my genetics colleagues tell me something like 5,000 is a minimum for even making it into their database of genetic associations. And so that makes it really difficult to study placebo effects in sample sizes like that. And at the same time, there's been this trend in psychology and in science, really, in general, towards reproducibility and replicability that probably in part are sort of evoked by John Ioannidis's provocative claims that most findings are false, but there's something really there.
There's been many teams of people who have tried to pull together, like Brian Nosek's work with Open Science Foundation, and many lab studies to replicate effects in psychology with much higher power. So there's this sort of increasing effort to pull together consortia to really test these things vigorously. And I wonder if -- we might not have a GWA study of placebo effects in 100,000 people or something, which is what would convince a geneticist that there's some kind of association. I'm wondering what the ways forward are, and I think one way is to increasingly come together to pull studies or do larger studies that are pre registered and even registered reports which are reviewed before they're published so that we can test some of these associations that have emerged in these what we call early studies of placebo effects.
And I think if we preregister and found something in sufficiently large and diverse samples, that might make a dent in convincing the wider world that essentially there is something that we can use going forward in clinical trials. And pharma might be interested in, for example, as well. That's my take on that. And wondering what people think.
KATHRYN HALL: My two cents. I completely agree with you. I think the way forward is to pull our resources to look at this and not simply stop -- I think when things don't replicate, I think we need to understand why they don't replicate. I think there's a taboo on looking beyond, if you prespecified it and you don't see it, then it should be over. I think in at least this early stage, when we're trying to understand what's happening, I think we need to allow ourselves deeper dives not for action but for understanding.
So I agree with you. Let's pull our resources and start looking at this. The other thing I would like to point out that's interesting is when we've looked at some of these clinical trials at the placebo arm, we actually learn a lot about natural history. We just did one in Alzheimer's disease and in the placebo arm the genome wide significant hit was CETP, which is now a clinical target in Alzheimer's disease. You can learn a lot by looking at the placebo arms of these studies not just about whether or not the drug is working or how the drug is working, but what's happening in the natural history of these patients that might change the effect of the drug.
TED KAPTCHUK: Marta, did you have something to say; you had your hand up.
MARTA PECINA: Just a follow up to what everybody is saying. I do think the issue of individualability is important. I think that one thing that maybe kind of explains some of the things that was also saying at the beginning that there's a little bit of lack of consistency or a way to put all of these findings together. The fact that we think about it as a one single placebo effect and we do know that there's not one single placebo effect, but even within differing clinical conditions is the newer value placebo effect the same in depression as it is in pain?
Or are there aspects that are the same, for example, expectancy processing, but there's some other things that are very specific to the clinical condition, whether it's pain processing, mood or some others. So I think we face the reality of use from a neurobiology perspective that a lot of the research has been done in pain and still there's very little being done at least in psychiatry across many other clinical conditions that we just don't know. And we don't really even know if the placebo how does the placebo effect look when you have both pain and depression, for example?
And so those are still very open questions that kind of reflect our state, right, that we're making progress but there's a lot to do.
TED KAPTCHUK: Winfried, did you want to say something? You have your hand up.
WINFRIED RIEF: I wanted to come back to the question of whether we really understand this increase of placebo effects. I don't know whether you have (indiscernible) for that. But I'm more like a scientist I can't believe that people are nowadays more reacting to placebos than they did 20 years ago. So there might be other explanations for this effect, like we changed the trial designs. We have more control visits maybe nowadays compared to 30 years ago, but there could be also other factors like publication bias which was maybe more frequent, more often 30 years ago than it is nowadays with the need for greater registration. So there are a lot of methodological issues that could explain this increase of placebo effects or of responses in the placebo groups. I would be interested whether you think that this increase is well explained or what your explanations are for this increase.
TED KAPTCHUK: Winfried, I want to give my opinion. I did think about this issue. I remember the first time it was reported in scientists in Cleveland, 40, 50 patients, and I said, oh, my God, okay, and the newspapers had it all over: The placebo effect is increasing. There's this boogie man around, and everyone started believing it. I've been consistently finding as many papers saying there's no -- I've been collecting them. There's no change over time there are changes over time. When I read the original article, I said, of course there's differences. The patients that got recruited in 1980 were different than the patients in 1990 or 2010. They were either more chronic, less chronic.
They were recruited in different ways, and that's really an easy explanation of why things change. Natural history changes. People's health problems are different, and I actually think that the Stone's meta analysis with 70,033 patients says it very clearly. It's a flat line from 1979. And the more data you have, the more you have to believe it. That's all. That's my personal opinion. And I think we actually are very deeply influenced by the media. I mean, I can't believe this:
The mystery of the placebo. We know more about placebo effects at least compared to many drugs on the market. Thanks my opinion. Thanks, Winfried, for letting me say it.
MATTHEW RUDORFER: Thanks, Ted.
We have a question for Greg. The question is, I wonder what the magic of 90 seconds is? Is there a physiologic basis to the turning point when the mouse changes behavior?
GREGORY CORDER: I think I addressed it in a written post somewhere. We don't know. We see a lot of variability in those animals. So like in this putative placebo phase, some mice will remain on that condition side for 40 seconds, 45 seconds, 60 seconds. Or they'll stay there the entire three minutes of the test. We're not exactly sure what's driving the difference in those different animals. These are both male and females. We see the effect in both male and female C57 black six mice, a genetically inbred animal. We always try to restrict the time of day of testing. We do reverse light testing. This is the animal wake cycle.
And there are things like dominance hierarchies within the cages, alpha versus betas. They may have different levels of pain thresholds. But the breaking of whatever the anti nocioceptive effect is they're standing on a hot plate for quite a long time. At some point those nociceptors in the periphery are going to become sensitized and signal. And to some point it's to the animal's advantage to pay attention to pain. You don't want to necessarily go around not paying attention to something that's potentially very dangerous or harmful to you. We would have to scale up the number of animals substantially I think, to really start parse out what the difference is that would account for that. But that's an excellent point, though.
MATTHEW RUDORFER: Carolyn.
CAROLYN RODRIGUEZ: I want to thank all today's speakers and wonderful presentations today. I just wanted to just go back for a second to Dr. Pecina's point about thinking about a placebo effect is not a monolith and also thinking about individual disorders.
And so I'm a clinical trialist and do research in obsessive compulsive disorder, and a lot of the things that are written in the literature meta analysis is that OCD has one of the lowest placebo rates. And so, you know, from what we gathered today, I guess to turn the question on its head is, is why is that, is that the case, why is that the case, and does that say something about OCD pathology, and what about it? Right? How can we really get more refined in terms of different domains and really thinking about the placebo effect.
So just want to say thank you again and to really having a lot of food for thought.
MATTHEW RUDORFER: Thanks. As we're winding down, one of the looming questions on the table remains what are research gaps and where do you think the next set of studies should go. And I think if anyone wants to put some ideas on the table, they'd be welcome.
MICHAEL DETKE: One of the areas that I mentioned in my talk that is hard for industry to study, or there's a big incentive, which is I talked about having third party reviewers review source documents and videos or audios of the HAM D, MADRS, whatever, and that there's not much controlled evidence.
And, you know, it's a fairly simple design, you know, within our largest controlled trial, do this with half the sites and don't do it with the other half.
Blinding isn't perfect. I haven't thought about this, and it can probably be improved upon a lot, but imagine you're the sponsor who's paying the $20 million in three years to run this clinical trial. You want to test your drug as fast as you possibly can. You don't want to really be paying for this methodology.
So that might be -- earlier on Tor or someone mentioned there might be some specific areas where this might be something for NIH to consider picking up. Because that methodology is being used in hundreds of trials, I think, today, the third party remote reviewer. So there's an area to think about.
MATTHEW RUDORFER: Thanks. Holly.
SARAH “HOLLY” LISANBY: Yeah. Carolyn just mentioned one of the gap areas, really trying to understand why some disorders are more amenable to the placebo response than others and what can that teach us. That sounds like a research gap area to me.
Also, throughout these two days we've heard a number of research gap areas having to do with methodology, how to do placebos or shams, how to assess outcome, how to protect the blind, how do you select what your outcome measures should be.
And then also today my mind was going very much towards what can preclinical models teach us and the genetics, the biology of a placebo response, the biogender line, individual differences in placebo response.
There may be clues there. Carolyn, to your point to placebo response being lower in OCD, and yet there are some OCD patients who respond, what's different about them that makes them responders?
And so studies that just look at within a placebo response versus nonresponse or gradation response or durability response and the mechanisms behind that.
These are questions that I think may ultimately facilitate getting drugs and devices to market, but certainly are questions that might be helpful to answer at the research stage, particularly at the translational research stage, in order to inform the design of pivotal trials that you would ultimately do to get things to market.
So it seems like there are many stages before getting to the ideal pivotal trial. So I really appreciate everyone's input. Let me stop talking because I really want to hear what Dr. Hall has to say.
KATHRYN HALL: I wanted to just come back for one of my favorite gaps to this question increasing the placebo effect. I think it's an important one because so many trials are failing these days. And I think it's not all trials are the same.
And what's really fascinating to me is that you see in Phase II clinical trials really great results, and then what's the first thing you do as a pharma company when you got a good result? You get to put out a press release.
And what's the first thing you're going to go do when you enroll in a clinical trial? You're going to read a press release. You're going to read as much as you can about the drug or the trial you're enrolling in. And how placebo boosting is it going to be to see that this trial had amazing effects on this condition you're struggling with.
If lo and behold we go to Phase III, and you can -- we're actually writing a paper on this, how many times we see the words "unexpected results," and I think we saw them here today, today or yesterday. Like, this should not be unexpected. When your Phase III trial fails, you should not be surprised because this is what's happening time and time again.
And I think some of the -- yeah, I agree, Ted, it's like this is a modern time, but there's so much information out there, so much information to sway us towards placebo responses that I think that's a piece of the problem. And finding out what the problem is I think is a really critical gap.
MATTHEW RUDORFER: Winfried.
WINFRIED RIEF: Yeah. May I follow up in that I think it fits quite nicely to what has been said before, and I want to direct I want to answer directly to Michael Detke.
On first glance, it seems less expensive to do the trials the way we do it with one placebo group and one drug arm, and we try to keep the context constant. But this is the problem. We have a constant context without any variation, so we don't learn under which context conditions is this drug really effective and what are the context conditions the drug might not be effective at all.
And therefore I think the current strategy is more like a lottery. It's really by chance it can happen that you are in this little window where the drug can show the most positive effectivity, but it can also be that you are in this little window or the big window where the drug is not able to show its effectivity.
And therefore I think, on second glance, it's a very expensive strategy only to use one single context to evaluate a drug.
MATTHEW RUDORFER: If I have time for--
TED KAPTCHUK: Marta speak, and then Liane should speak.
MARTA PECINA: I just wanted to add kind of a minor comment here, which is this idea that we're going to have to move on from the idea that giving someone a placebo is enough to induce positive expectancies and the fact that expectancies evolve over time.
So at least in some of the data that we've shown, and it's a small sample, but still we see that 50% of those subjects who are given a placebo don't have drug assignment beliefs. And so that is a very large amount of variability there that we are getting confused with everything else.
And so I do think that it is really important, whether in clinical trials, in research, to really come up with very and really develop new ways of measuring expectancies and allow expectancies to be measured over time. Because they do change. We have some prior expectancies, and then we have some expectancies that are learned based on experience. And I do think that this is an area of improvement that the field could improve relatively easily, you know, assess expectancies better, measure expectancies better.
TED KAPTCHUK: Liane, why don't you say something, and Luana, and then Cristina.
LIANE SCHMIDT: So I wanted to -- maybe one -- another open gap is like about the cognition, like what studying placebo, how can it help us to better understand human reasoning, like, and vice versa, actually, all the biases we have, these cognitive processes like motivation, for example, or memory, and yet all the good news about optimism biases, how do they contribute to placebo effects on the patient side but also on the clinician side when the clinicians have to make diagnosis or judge, actually, treatment efficiency based on some clinical scale.
So basically using like tools from cognition, like psychology or cognitive neuroscience, to better understand the processes, the cognitive processes that intervene when we have an expectation and behavior reach out, a symptom or neural activation, what comes in between, like how is it translated, basically, from cognitive predictability.
LUANA COLLOCA: I think we tended to consider expectation as static measurement when in reality we know that what we expect at the beginning of this workshop is slightly different by the end of what we are hearing and, you know, learning.
So expectation is a dynamic phenomenon, and the assumption that we can predict placebo effects with our measurement of expectation can be very limiting in terms of, you know, applications. Rather, it is important to measure expectation over time and also realize that there are so many nuance, like Liane just mentioned, of expectations, you know.
There are people who say I don't expect anything, I try everything, or people who say, oh, I truly want, I will be I truly want to feel better. And these also problematic patients because having an unrealistic expectation can often destroy, as I show, with a violation of expectancies of placebo effects.
TED KAPTCHUK: Are we getting close? Do you want to summarize? Or who's supposed to do that? I don't know.
CRISTINA CUSIN: I think I have a couple of minutes for remarks. There's so much going on, and more questions than answers, of course.
That has been a fantastic symposium, and I was trying to pitch some idea about possibly organizing a summit with all the panelists, all the presenters, and everyone else who wants to join us, because I think that with a coffee or a tea in our hands and talking not through a Zoom video, we could actually come up with some great idea and some collaboration projects.
Anyone who wants to email us, we'll be happy to answer. And we're always open to collaborating and starting a new study, bouncing off each other new ideas. This is what we do for a living. So we're very enthusiastic about people asking difficult questions.
And some of the questions that are ongoing and I think would be future areas is what we were talking a few minutes ago, we don't know if a placebo responder in a migraine study, for example, would be a placebo responder of depression study or IBS study. We don't know if this person is going to be universal placebo responder or is the context include the type of disease they're suffering from so it's going to be fairly different, and why some disorders have lower placebo response rate overall compared to others. Is that a chronicity, a relaxing, remitting disorder, has higher chance of placebo because the system can be modulated, versus a disorder that is considered more chronic and stable? A lot of this information is not known in the natural history.
Also comes to mind the exact trial it is because we almost never have a threshold for number of prior episodes of depression to enter a trial or how chronic has it been or years of depression or other factors that can clearly change our probability of responding to a treatment.
We heard about methodology for clinical trial design and how patients could be responsive to placebo responses or sham, responsive to drug. How about patients who could respond to both? We have no idea how many of those patients are undergoing a trial, universal responders, unless we do a crossover. And we know that crossover is not a popular design for drug trials.
So we need to figure out also aspects of methodology, how to assess outcome, what's the best way to assess the outcome that we want, is it clinically relevant, how to protect the blind aspect, assess expectations and how expectations change over time.
We didn't hear much during the discussion about the role of mindfulness in pain management, and I would like to hear much more about how we're doing in identifying the areas and can we actually intervene on those areas with devices to help with pain management. That's one of the biggest problems we have in terms of clinical care.
In the eating disorder aspect, creating computational models to influence food choices. And, again, with devices or treatments specifically changing the balance about making healthier food choices, I can see an entire field developing. Because most of the medications we prescribe for psychiatric disorders affect food choices and there's weight gain, potentially leading to obesity and cardiovascular complications. So there's an entire field of research we have not touched on.
And the role of animal models in translational results, I don't know if animal researchers, like Greg, talk much with clinical trialists. I think that would be a cross fertilization that is much needed, and we can definitely learn from each other.
And just fantastic. I thank all the panelists for their willingness to work with us and their time, dedication, and just so many meetings to discuss to agree on the program and to divide and conquer different topics. Has been a phenomenal experience, and I'm very, very grateful.
And the NIMH staff has been also amazing, having to collaborate with all of them, and they were so organized. And just a fantastic panel. Thank you, everybody.
MATTHEW RUDORFER: Thank you.
TOR WAGER: Thank you.
NIMH TEAM: Thanks from the NIMH team to all of our participants here.
(Meeting adjourned)
Olympic medalist Lindsey Vonn addresses struggles after retirement, knee replacement
Lindsey Vonn held up her hands with fingers extended when asked how many surgeries she’s had.
"It’s more than two hands," said Vonn, 38, the downhill skier who won an Olympic gold medal, two Olympic bronze medals and four World Cup overall championships before injuries prompted her to retire in 2019. "I just had a surgery three weeks ago.
"Yeah, my right knee is in a constant state of pain pretty much, so I’m just trying to manage that. I definitely paid a heavy price for the success I had in my skiing career. But I have no regrets."
Vonn talked about a variety of subjects during a recent Zoom interview while in Los Angeles for business purposes. The conversation included the ongoing impact of countless crashes on the ski slopes.
In a few months, Vonn said, she will undergo knee replacement surgery − which says as much about her hopes for the future as her past.
"My goal is for that to allow me to ski with my kids one day," she said.
The kids she hopes to have.
Vonn, who famously dated Tiger Woods and retired NHL defenseman P.K. Subban, has been dating tequila founder Diego Osorio since 2021. She has avoided talking publicly about the relationship, but she discussed with USA TODAY Sports her desire to be a mother.
"I’m the oldest of five kids," she said. "The youngest three were triplets, and I changed a lot of diapers and I took them to school and I made them lunches.
"I think my parents did a really great job. I’d love to add things that I’ve learned in my life kind of in that process of raising a child."
How multiple surgeries have affected Lindsey Vonn
In 2013, Vonn said, her first knee surgery left more than scars. It also coincided with the onset of insomnia.
"I really started to struggle with my sleep and it became this downward spiral where I just had a lot of anxiety and it just kept getting worse and worse," she said. "And obviously I’ve had my share of surgeries in my life. So the compounding effect of all those things really was not helpful for me and my sleep patterns."
She said the problem lingered nine more years before she consulted her doctor. According to Vonn, her doctor prescribed QUVIVIQ, a drug used to treat insomnia.
"I don’t know if I look like I’ve been sleeping better," Vonn said, with a self-deprecating smile. It "has been such a game changer for me. I’m finally getting the sleep that I so desperately needed."
Vonn said she has partnered with Idorsia, the Swiss pharmaceutical research company that makes the drug.
Challenges Lindsey Vonn faced beyond insomnia
The problems Vonn said she faced after retirement at 34 went beyond insomnia. They surfaced when she was writing a draft of her memoir, “Rise: My Story,’’ according to Vonn.
"I was still very upset and I think resentful that I had to retire because I didn’t want to," she said. "I had to because of my injuries. So I rewrote it and I think was in a much better place the second time around."
In addition to what she described as the therapeutic process of writing the book, Vonn also credits her work with Armando Gonzalez, a therapist who has worked with professional athletes and Olympians.
One approach Gonzalez uses is brainspotting, a psychotherapy that involves identifying how a patient’s body responds when they’re describing a traumatic event, according to a paper co-authored by brainspotting’s founder, David Grand.
Vonn said it’s helped her figure out “who I am and what makes me tick."
"It’s not skiing, it’s what skiing made me feel," she said. "And so kind of figuring out how I can find that same emotion in my life now, which again, I don’t have the adrenaline as much anymore obviously. But I have no shortage of adventure as I am always trying new things and I enjoy the feeling of setting my mind to something to do it. And I don’t always succeed but I think for me it’s that challenge that really excites me and makes me feel similarly to when I was racing."
Lindsey Vonn reflects on her mental health journey
Vonn said her current state of mind is a departure from what she felt during her career.
"Being on the road when I was skiing was really difficult for me," she said. "I’ve struggled with depression also since I was a teenager.
"Journaling has been something that really has helped me. Now mental health is something that everyone talks about. But when I was racing it was absolutely not and it was a sign of weakness if you were struggling with something.
"Talking to someone was not an option. So for me I just kind of internalized it, I wrote it down in my journal and that was my way of coping."
Journaling no longer was enough and so she sought therapy, Vonn said.
She credits athletes such as Michael Phelps, Kevin Love, Naomi Osaka and Simone Biles for being “really important players in that conversation’’ and also noted she addressed it publicly in 2012 .
"Because it was something that I was weighing on me for a long time," he said. "I felt like I needed to share that part of myself to kind of get the weight off my back and it definitely did help me a lot in my journey."

An official website of the United States government
The .gov means it’s official. Federal government websites often end in .gov or .mil. Before sharing sensitive information, make sure you’re on a federal government site.
The site is secure. The https:// ensures that you are connecting to the official website and that any information you provide is encrypted and transmitted securely.
- Publications
- Account settings
- My Bibliography
- Collections
- Citation manager
Save citation to file
Email citation, add to collections.
- Create a new collection
- Add to an existing collection
Add to My Bibliography
Your saved search, create a file for external citation management software, your rss feed.
- Search in PubMed
- Search in NLM Catalog
- Add to Search
Associations between insomnia and cardiovascular diseases: a meta-review and meta-analysis of observational and Mendelian randomization studies
Affiliations.
- 1 Department of Cardiology, Heart Center, The First Affiliated Hospital of Xinxiang Medical University, Weihui, China.
- 2 Center for Sleep and Circadian Medicine, The Affiliated Brain Hospital of Guangzhou Medical University, Guangzhou, Guangdong, China.
- 3 School of Cardiovascular and Metabolic Medicine and Sciences, King's College London British Heart Foundation Centre of Research Excellence, London, UK.
- PMID: 39167428
- DOI: 10.5664/jcsm.11326
Study objectives: Observational studies suggest associations between insomnia and cardiovascular diseases (CVDs), but the causal mechanism remains unclear. We investigated the potential causal associations between insomnia and CVDs by a combined systematic meta-review and meta-analysis of observational and Mendelian randomization (MR) studies.
Methods: We searched PubMed, Web of Science, and Embase for English-language articles from inception to 7/11/2023. Two reviewers independently screened the articles to minimize potential bias. We summarized the current evidence on the associations of insomnia with coronary artery disease (CAD), atrial fibrillation (AF), heart failure (HF), myocardial infarction (MI), hypertension (HTN), and stroke risk by combining meta-analyses of observational and MR studies.
Results: Four meta-analyses of observational studies and 9 MR studies were included in the final data analysis. A systematic meta-review of observational studies provided strong evidence that insomnia is an independent risk factor for many CVDs, including AF, MI, and HTN. A meta-analysis of MR studies revealed that insomnia may be potentially causally related to CAD (odds ratio (OR)=1.14, 95% confidence interval (CI)=1.10-1.19, I 2 =97%), AF (OR=1.02, 95% CI=1.01-1.04, I 2 =94%), HF (OR=1.04, 95% CI=1.03-1.06, I 2 =97%), HTN (OR=1.16, 95% CI=1.13-1.18, I 2 =28%), large artery stroke (OR=1.14, 95% CI=1.05-1.24, I 2 =0%), any ischemic stroke (OR=1.09, 95% CI=1.03-1.14, I 2 =60%), and primary intracranial hemorrhage (OR=1.16, 95% CI=1.05-1.27, I 2 =0%). No evidence suggested that insomnia is causally associated with cardioembolic or small vessel stroke.
Conclusions: Our results provide strong evidence supporting a possible causal association between insomnia and CVD risk. Strategies to treat insomnia may be promising targets for preventing CVDs.
Keywords: cardiovascular; insomnia; mendelian randomization; meta-review; observational study.
© 2024 American Academy of Sleep Medicine.
PubMed Disclaimer
Related information
Linkout - more resources, full text sources.
- American Academy of Sleep Medicine
Research Materials
- NCI CPTC Antibody Characterization Program
Miscellaneous
- NCI CPTAC Assay Portal
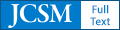
- Citation Manager
NCBI Literature Resources
MeSH PMC Bookshelf Disclaimer
The PubMed wordmark and PubMed logo are registered trademarks of the U.S. Department of Health and Human Services (HHS). Unauthorized use of these marks is strictly prohibited.

An official website of the United States government
The .gov means it’s official. Federal government websites often end in .gov or .mil. Before sharing sensitive information, make sure you’re on a federal government site.
The site is secure. The https:// ensures that you are connecting to the official website and that any information you provide is encrypted and transmitted securely.
- Publications
- Account settings
Preview improvements coming to the PMC website in October 2024. Learn More or Try it out now .
- Advanced Search
- Journal List
- Ann Med Surg (Lond)
- v.85(6); 2023 Jun
- PMC10289604

Exploring the relationship between insomnia and depression: a cross-sectional prospective study
Ahmed mishaal mohammed salih.
a Mental Health Nursing, College of Nursing, University of Mosul
Zeyad Tariq Madallah
Radhwan h ibrahim.
b Community Health Nursing, College of Nursing
Mahfoodh Suliaman Hasan Alseadn
c Mental Health Medicine
Osama Ismael Almushhadany
d College of Medicine, Ninevah University, Iraq
Associated Data
The data that support the findings of this study are available from the corresponding author upon reasonable request. Access to the data is subject to a data use agreement and restrictions on reuse of the data.
Objectives:
This is a cross-sectional prospective study aimed to examine the association between insomnia and depression among individuals attending an outpatient psychiatric clinic.
A total of 416 participants were recruited from the psychiatric and mental health outpatient clinic using a cross-sectional design. The participants completed the Pittsburgh Sleep Quality Index (PSQI) and the Beck Depression Inventory (BDI) to measure sleep disturbances and depressive symptoms, respectively. Ethical approval was obtained from the Institutional Review Board at Ninevah University. Descriptive statistics, bivariate correlations, and multivariate regression analysis were used to analyze the data. The data collected in the study was analyzed using SPSS v0.26.
A total of 416 participants were recruited from the psychiatric and mental health outpatient clinic in Iraq through convenience sampling. The sample included 227 (54.6%) females and 189 (45.4%) males, with a mean age of 35.4 years (SD=9.8, range=18–65). Participants had a mean PSQI score of 11.6 (SD=3.7, range=2–21) and a mean BDI score of 21.8 (SD=11.1, range=0–54). Bivariate correlations showed a significant positive relationship between sleep disturbances (as measured by the PSQI) and depressive symptoms (as measured by the BDI) (r=0.67, P <0.001).
Conclusions:
The study provides valuable insights into the relationship between insomnia and depression in a sample of individuals from Iraq. These findings could contribute to the development of interventions to improve mental health outcomes among this population.
Introduction
- The study investigated the relationship between insomnia and depression in a sample of adults living in Iraq.
- A cross-sectional study was conducted on 416 adults aged 18 years and above, who were recruited from various regions in Iraq.
- Data on demographic characteristics, insomnia symptoms, and depression symptoms were collected using validated questionnaires.
- The results of the study suggest a significant association between insomnia and depression in the Iraqi population, with individuals reporting insomnia being more likely to have symptoms of depression. The findings have important implications for the screening, prevention, and treatment of these two conditions.
- The manuscript adds to the growing body of literature on the relationship between insomnia and depression, particularly in the Middle Eastern population.
- The study highlights the importance of addressing mental health issues in the Iraqi population, and the need for further research in this area.
The prevalence of sleep disturbances in individuals with depression is indeed high, with estimates suggesting that up to 75% of depressed patients experience difficulty initiating or maintaining sleep, as well as early morning awakenings 1 . These sleep disturbances can have a significant impact on an individual’s overall well-being, exacerbating depressive symptoms, and contributing to a range of negative health outcomes 2 . It is therefore crucial that clinicians and researchers alike pay close attention to sleep disturbances in patients with depression, and work to identify effective strategies for improving sleep quality in this population 3 . These may include a combination of pharmacological and nonpharmacological approaches, such as cognitive-behavioral therapy for insomnia, sleep hygiene education, and the use of sleep-promoting medications. By addressing sleep disturbances as a key component of depression treatment, we may be able to improve outcomes and ultimately reduce the burden of depressive disorders 4 .
Insomnia and depression are two common and often co-occurring mental health conditions that can have a significant impact on an individual’s overall well-being 5 – 7 . Previous research 8 – 10 has suggested a bidirectional relationship between insomnia and depression, with poor sleep quality contributing to the development and maintenance of depressive symptoms, and depression exacerbating sleep disturbances. However, the underlying mechanisms of this relationship remain unclear, and further research is needed to fully understand the link between insomnia and depression. The current study aims to investigate the relationship between insomnia and depression using a cross-sectional design.
Study design
This study employed a cross-sectional prospective design to investigate the relationship between sleep disturbances and depression. The work has been reported in line with the strengthening the reporting of cohort, cross-sectional and case-control studies in surgery (STROCSS) criteria 11 .
Study setting
The research was conducted in the city of Mosul, Iraq. Participants were recruited from the outpatient department of psychiatric and mental health at Al Salam teaching Hospital is a tertiary care center affiliated with the Ministry of Health. It provides a wide range of medical services, including psychiatric and mental health services, to the people of Mosul and the surrounding areas. The study was conducted over a period of 3 months.
Study sample
The study recruited a total of 416 participants using convenience sampling methods. The inclusion criteria for the study were patients aged 18 years or older, who had a diagnosis of a sleep disorder, including sleep apnea, narcolepsy, restless legs syndrome, periodic limb movement, circadian rhythm disorder, sleepwalking, or insomnia, and who agreed to participate in the study. The exclusion criteria were patients with a history of a major medical or psychiatric disorder, a substance use disorder, or any medication that may affect sleep.
Study outcome
The outcomes of the study were as follows:
Primary outcome
Sleep disturbances measured by the pittsburgh sleep quality index (psqi).
The PSQI is a self-report questionnaire that assesses sleep quality over the past month. It consists of 19 items that measure various aspects of sleep, including sleep quality, latency, duration, efficiency, disturbances, use of sleep medication, and daytime dysfunction. The total score ranges from 0 to 21, with higher scores indicating worse sleep quality.
The validation of the PSQI has been well-established in previous research, and it has been shown to have good reliability and validity.
Depressive symptoms measured by the Beck Depression Inventory (BDI)
The BDI is a self-assessment tool consisting of 21 questions designed to evaluate depression symptoms and attitudes. It has been developed in numerous formats, such as computerized versions, card form, a 13-item short form, and the more recent BDI-II. The inventory takes approximately 10 min to complete and requires a fifth to sixth grade reading level for comprehension.
The BDI exhibits good internal consistency, with values ranging from .73 to .92 and an average of .86. The 13-item short form also shows comparable reliability. The alpha coefficients of the BDI are .86 and .81 for psychiatric and nonpsychiatric populations, respectively, indicating high internal consistency.
Based on the total score, individuals are categorized into different levels of depression severity, as follows:
- 0–13: minimal depression.
- 14–19: mild depression.
- 20–28: moderate depression.
- 29–63: severe depression.
Pilot study
A pilot study was conducted with a convenience sample of 20 participants to test the feasibility and acceptability of the study procedures, including the recruitment process, study measures, and data collection procedures. Based on the results of the pilot study, no major revisions to the study procedures were deemed necessary.
Data collection
Data collection occurred over a period of 6 months. Participants were invited to the research laboratory where they provided informed consent and completed a battery of self-report questionnaires, including the PSQI and BDI. The questionnaires were completed in a private room with no distractions. Participants were also asked to provide demographic information, including age, sex, education level, and employment status.
Data analysis
The data collected in this study were analyzed using SPSS v.26. Descriptive statistics, including means and SD, were used to characterize the sample and study measures. Bivariate correlations were calculated to examine the relationship between sleep disturbances and depression. A multivariate regression analysis was conducted to examine the unique contribution of sleep disturbances to depression, after controlling for relevant demographic variables. In the multivariate regression analysis, age and sex were included as covariates, and their effects on the relationship between sleep disturbances and depression were examined. The level of statistical significance used in the study was set at P <0.05, and adjustments were made for multiple comparisons using the Bonferroni correction. Additionally, a sensitivity analysis was conducted to examine the robustness of the study’s findings. In this analysis, the sample was stratified by age and sex, and the relationship between sleep disturbances and depression was examined within each stratum.
Ethical approval
In this study, ethical approval was obtained from the Institutional Review Board at Ninevah University. The approval number was #85, and it was granted on 18 October 2021.
Based on the information provided in the tables, we can make some observations
The Table Table1 1 shows that the study included both male and female participants, with females comprising the majority (58.6%). The age of the participants ranged from 18 to 80 years, with a mean age of 41.10 years and a SD of 12.16. The number of children ranged from 0 to 12, with a mean of 4.80 and a standard deviation of 3.52. In terms of socioeconomic features, the table reports on the educational level and residence of the participants. The educational level was categorized as illiterate, primary, secondary, and college, with the majority of participants having a primary education (41.4%) or college education (34.5%). The residence was categorized as rural, suburban, or urban, with the majority of participants living in a suburban area (51.7%). Regarding the prognostic features, the study assessed the severity of depression based on the Beck scores and sleep disturbances. The Beck scores ranged from borderline (scores of 10–16) to severe (scores of 30–40), with the majority of participants having mild (41.4%) or moderate (27.6%) depression Table Table1. 1 . The mean insomnia score was 18.10, indicating moderate sleep disturbance overall, but with significant differences in severity between the depression categories. Among the study participants, 13.8% had mild insomnia, 69% had moderate insomnia, and 17.2% had severe insomnia. The comparison between groups showed a statistically significant difference in the severity of insomnia between groups ( P =0.03). Of the participants with mild insomnia, 50% had a history of previous illness, compared to 31% of participants with moderate insomnia and 7.7% of participants with severe insomnia. Regarding previous illness, 82.8% of study participants reported having had an illness before, while 17.2% reported no previous illness. The comparison between groups showed a statistically significant difference in the prevalence of previous illnesses between groups ( P =0.004). Among the participants with a history of previous illness, the most common illnesses reported were respiratory diseases (41.4%) and gastrointestinal diseases (17.2%) Table Table2. 2 . Sleep disturbance symptoms are common among participants, with 60% reporting difficulty falling asleep, 50% waking up frequently, and 40% waking up too early. The majority of participants (70%) reported experiencing sleep disturbance symptoms for more than 6 months. Participants with sleep disturbance symptoms had lower scores on QOL measures compared to those without such symptoms, particularly in physical health, psychological health, social relationships, and environmental factors. Medication was the most common type of treatment for sleep disturbance symptoms (40%), followed by relaxation techniques (24%) and therapy/counseling (20%) Table Table3. 3 . The analysis shows that insomnia and sleep apnea are positively associated with sleep disturbance symptoms, with standardized regression coefficients (β) of 0.452 and 0.168, respectively. This suggests that individuals with insomnia and sleep apnea are more likely to report sleep disturbance symptoms. On the other hand, sleepwalking is negatively associated with sleep disturbance symptoms, with a β of -0.055. Sex, age, and circadian rhythm disorder were not found to be significantly associated with sleep disturbance symptoms Table Table4 4 .
Severity of depression based on Beck scores and sleep disturbances among study participants
Factors | Beck | Borderline | Mild | Moderate | Severe | |
---|---|---|---|---|---|---|
Age, mean±SD | 41.10±12.16 | 42.00±10.23 | 45.24±13.62 | 44.23±17.96 | 0.7 | |
No. of children, mean±SD | 4.80±3.52 | 7.25±4.64 | 5.48±2.66 | 4.30±2.58 | 0.4 | |
Insomnia score, mean±SD | 18.10±2.88 | 14.00±3.74 | 17.48±4.911 | 20.54±3.20 | 0.02 | |
Sex, No. (%) | 0.6 | |||||
Female | 17 (58.6%) | 3 (75.0%) | 21 (72.4%) | 8 (61.5%) | ||
Male | 12 (41.4%) | 1 (25.0%) | 8 (27.6%) | 5 (38.5%) | ||
Marital status, No. (%) | 0.6 | |||||
Divorced | – | 1 (25.0%) | – | 1 (7.7%) | ||
Married | 23 (79.3%) | 3 (75.0%) | 22 (75.9%) | 8 (61.5%) | ||
Single | 4 (13.8%) | 2 (6.9%) | 3 (10.3%) | 3 (23.1%) | 1 (7.7%) | |
Widow | 1 (7.7%) | |||||
Educational level, No. (%) | 0.05 | |||||
College | 10 (34.5%) | – | 10 (34.5%) | 2 (15.4%) | ||
Illiterate | 4 (13.8%) | – | 12 (41.4%) | 5 (38.5%) | ||
Primary | 9 (31.0%) | 3 (75.0%) | 4 (13.8%) | 4 (30.8%) | ||
Secondary | 6 (20.7%) | 1 (25.0%) | 3 (10.3%) | 2 (15.4%) | ||
Residence, No. (%) | 0.1 | |||||
Rural | 1 (3.4%) | 1 (25.0%) | 7 (24.1%) | 1 (7.7%) | ||
Suburban | 15 (51.7%) | 1 (25.0%) | 7 (24.1%) |
Comparison of severity of insomnia and previous illness among study participants
Variable | Mild | Moderate | Severe | |
---|---|---|---|---|
Severity of insomnia | 4 (13.8%) | 20 (69%) | 5 (17.2%) | 0.03 |
2 (50%) | 2 (50%) | – | ||
9 (31%) | 10 (34.5%) | 10 (34.5%) | ||
1 (7.7%) | 6 (46.2%) | 6 (46.2%) | ||
Previous illness | 24 (82.8%) | 5 (17.2%) | 0.004 | |
1 (25%) | 3 (75%) | |||
12 (41.4%) | 17 (58.6%) | |||
7 (53.8%) | 6 (46.2%) |
Sleep disturbance symptoms, duration, qol measures, and type of treatment among participants
Sleep disturbance symptom | Number of participants reporting symptom | Percentage of participants reporting symptom |
---|---|---|
Difficulty falling asleep | 150 | 60% |
Waking up frequently | 125 | 50% |
Waking up too early | 100 | 40% |
Nightmares | 75 | 30% |
Snoring | 60 | 24% |
Sleepwalking | 50 | 20% |
Duration of Symptoms | Number of participants | Percentage of participants |
Less than 3 months | 25 | 10% |
3–6 months | 37 | 15% |
More than 6 months | 175 | 70% |
Unsure | 13 | 5% |
QOL measure domain | Participants with sleep disturbance symptoms | Participants without sleep disturbance symptoms |
Physical health | 40.5 (SD=5.2) | 62.3 (SD=6.8) |
Psychological health | 42.8 (SD=5.5) | 65.1 (SD=7.1) |
Social relationships | 43.6 (SD=6.0) | 66.5 (SD=8.2) |
Environmental factors | 42.5 (SD=5.8) | 64.8 (SD=7.5) |
Overall QOL score | 45.2 (SD=7.8) | 68.7 (SD=8.1) |
Type of treatment | Number of participants | Percentage of participants |
Medication | 100 | 40% |
Relaxation techniques | 60 | 24% |
Therapy/Counseling | 50 | 20% |
Natural/Alternative | 25 | 10% |
No Treatment | 25 | 10% |
Multiple regression analysis of factors associated with sleep disturbance symptoms
Factors | B | β | 95% CIs for B | R |
---|---|---|---|---|
Sex | −0.174 | −0.030 | −0.789–0.503 | 36.2% |
Age | 0.050 | 0.062 | −0.016–0.089 | |
CRD | −0.018 | −0.012 | −0.149–0.119 | |
Sleepwalking | −0.172 | −0.055 | −0.386–0.115 | |
Insomnia | 0.249 | 0.452 | 0.187–0.299 | |
Sleep apnea | 0.169 | 0.168 | 0.069–0.301 | |
Narcolepsy | 0.116 | 0.059 | −0.041–0.264 | |
RLS/PLM | 0.152 | 0.087 | 0.014–0.302 |
The results of this study provide valuable insights into the demographic characteristics and sleep disturbance symptoms of individuals with different levels of depression severity. The study also examines the factors associated with sleep disturbance symptoms, which can inform future interventions to improve sleep quality in individuals with depression.
Numerous longitudinal studies have discovered that insomnia is an independent risk factor for developing new or recurring depression in young, middle-aged, and older adults 12 – 17 . This two-way link between sleep disruption and depression has led to a new understanding that sleep issues are not merely a side effect of depression, but rather a predictive early warning sign. People are most likely to experience their first depressive episode between the ages of 30 and 40, followed by a secondary, smaller peak between the ages of 50 and 60. The average age for depression onset is roughly 27 years. However, our research found that most participants were in their mid-40s, which can be explained by approximately one-third of them having prior symptoms. This implies that they may have had their first episode in their 20s, with mild symptoms that faded naturally. Conversely, the remaining two-thirds without previous symptoms might have had their first episodes during early middle age due to sociocultural or economic factors. Those in their young adulthood may overlook their mental well-being while focusing on their early marital or occupational lives. As one gets older, both depressive symptoms and insomnia scores increase in severity, with insomnia becoming the primary concern driving patients to seek medical help. Nonetheless, sleep quality is generally believed to decline as adults age.
Our study found that sleep problems were twice as prevalent in women compared to men, with a female-to-male ratio of ~2:1. This sex difference could be attributed, in part, to differences in symptom perception between men and women. Sleep disturbances are commonly reported by middle-aged women, with age and the menopausal transition being significant contributing factors. Previous research 18 - 20 has focused on the relationship between sleep, sex hormones, and body temperature. For instance, the study of women’s health across the nation found that 35% of women aged 40–55 years experienced difficulty with sleep 21 . It remains unclear whether vasomotor symptoms initiate arousal or whether hot flashes occur after arousal 22 . Consequently, older women who spend the same amount of time in bed as their younger counterparts sleep ~2 h less 23 . Perimenopausal women’s daytime levels of LH were found to correlate with the number of nocturnal awakenings 24 . Although sleep quality may not change during the menopausal transition, sleep quantity, which is affected by specific symptoms such as hot flashes and depression, has been linked to menopause 25 - 27 .
In our study, approximately three-quarters of the participants were married and primarily responsible for childcare. Sleep quality and quantity may be affected by factors such as marital conflicts, financial concerns, and childcare duties. Despite a lower socioeconomic status in rural areas, only 13.3% of patients reported sleep issues at our clinic, which may be attributed to the simpler lifestyle in rural regions compared to urban or suburban areas. Of the participants, 36 (48%) came from urban and 29 (38.7%) from suburban locations. Urbanization and modern societies could potentially increase the risk of depression and contribute to chronic sleep deprivation among their inhabitants.
There tends to be a negative correlation between education level and depression as well as sleep issues. Our sample supports this observation, with a significant number of illiterate individuals experiencing sleep disturbances and moderate to severe depression. However, this trend does not align with college graduates, who comprise around 30% of our sample. These individuals may experience hopelessness due to job market difficulties or stress related to work and personal expectations, which could contribute to their mental health challenges.
In our study, ~80% of participants were identified as having borderline or moderate depression according to the beck depression scale. Almost 39% exhibited borderline symptoms and an equal percentage demonstrated moderate depressive symptoms. Borderline individuals, comprising more than a third of the sample, experienced significant sleep issues despite lacking severe depressive symptoms. Sleep problems were observed in various forms such as interrupted or unsatisfactory sleep (about 28%), and early insomnia (around 21%). This could be due to the distress caused by sleep issues, or the stigma surrounding mental illness in our community, causing patients to downplay their depression symptoms and focus more on sleep-related complaints. Alternatively, sleep problems could be early indicators of depression. Furthermore, participants classified as having borderline depression who were taking antidepressants showed a fair response to their treatments; however, they reported experiencing insomnia, which might be a side effect of the medication.
In our sample, all patients presented with anxiety symptoms, which may explain why early insomnia and other sleep disturbances, such as interrupted and unsatisfactory sleep, were the most common sleep problems reported among those with depressive symptoms. Late insomnia, which is typically associated with severe depression, was less frequently reported. These findings suggest that anxiety may play a significant role in the development of sleep disturbances in patients with depressive symptoms. It is important for clinicians to address both anxiety and sleep problems when treating patients with depression to improve overall outcomes.
There is a positive association between depression and insomnia. As the severity of depression increases from moderate to severe, the severity of insomnia also increases. Approximately 70% of individuals with borderline depression have moderate severity insomnia, measured as 18 by insomnia scores. About 70% of those with moderate depression and 90% of those with severe depression have moderate to severe insomnia. This relationship confirms the connection between depression and insomnia.
Hypersomnia is a less common feature of atypical depression and is more prevalent in young individuals, with only 12% of patients experiencing the symptom, particularly those with moderate to severe depression. Although sleep apnea cases are few, studies have noted a strong correlation between obstructive sleep apnea and depression symptoms. Furthermore, mood and circadian rhythms show a bidirectional relation and share similarities in epidemiology, clinical presentation, neurobiology, and treatment implications, although only 6.9% of the sample experienced such issues.
Strengths and implication
The findings of this study have important implications for clinical practice, particularly in the treatment of sleep disturbance symptoms. The study found a significant relationship between sleep disturbances and depression, suggesting that addressing sleep problems may be an important part of treating depression in individuals attending an outpatient psychiatric clinic. The most common type of treatment reported by the participants in this study was medication, but the study suggests that nonpharmacological interventions such as relaxation techniques and therapy/counseling may also be beneficial in improving quality of life. Clinicians should consider incorporating these types of interventions into their treatment plans for individuals with sleep disturbances and depression. In addition, future research should focus on longitudinal studies to establish causal relationships between sleep disturbances and depression, as well as randomized controlled trials to evaluate the effectiveness of nonpharmacological interventions. This will help to build a more comprehensive understanding of the relationship between sleep disturbances and depression and inform the development of effective interventions to improve mental health outcomes.
Weaknesses and limitations
There are several limitations of this study that should be considered when interpreting the findings. These limitations include the sample was recruited through convenience sampling from a single outpatient psychiatric clinic, which may limit the generalizability of the findings to other populations. The study utilized a cross-sectional design, which limits the ability to establish causality between sleep disturbances and depression. Longitudinal studies are needed to establish temporal relationships between these variables. The study relied on self-report measures to assess sleep disturbances and depression, which may be subject to bias or inaccuracies. The study did not include objective measures of sleep quality, such as polysomnography or actigraphy, which may provide a more accurate assessment of sleep disturbances. The study did not include detailed demographic information on participants, such as socioeconomic status, education level, or employment status, which may have influenced the study findings. The study did not assess the effectiveness of treatments received by participants for sleep disturbances and depression, which may have influenced the study results.
Assessment and management of bias
The study attempted to minimize selection bias by using a convenient sampling method and including all eligible participants who consented to participate. The study attempted to minimize response bias by using validated measures and ensuring participants understood the questions. The study did not report any attempts to minimize observer or measurement bias, as it relied solely on self-report measures.
In this study, ethical approval was obtained from the Institutional Review Board at Ninevah University. The approval number was #85, and it was granted on 18 October 2021. (UIN no. researchregistry8823).
Written informed consent was obtained from the patient for publication and any accompanying images. A copy of the written consent is available for review by the Editor-in-Chief of this journal on request.
Sources of funding
No funding was received for the study.
Author contribution
A.M.M.S. and R.H.I.: contributed to the study design, data collection, data analysis, and interpretation, and writing of the paper; Z.T.M. and M.S.H.A.: contributed to the study design, data analysis, and interpretation, and writing of the paper. All authors have approved the final version of the manuscript and have agreed to be accountable for all aspects of the work.
Conflicts of interest disclosure
Authors have no conflict of interest to declare.
Dr. Radhwan Hussein Ibrahim, Nursing College, Ninevah University, Mosul City, Iraq. E-mail [email protected]
Data availability statement
Provenance and peer review.
Not commissioned, externally peer reviewed.
Acknowledgments
The authors would like to express their gratitude to the Ethical Committee at Ninevah University, city of Mosul, Iraq, for approving and monitoring the ethical considerations of this study. The authors also extend their sincere appreciation to the study participants who generously gave their time and cooperation to make this research possible. The authors thank the staff and administration of the Psychiatric and Mental Health outpatient clinic at Al Salam Teaching Hospital for their support and assistance in facilitating the recruitment of participants for this study. Their contributions are greatly appreciated.
Sponsorships or competing interests that may be relevant to content are disclosed at the end of this article
Published online 8 May 2023
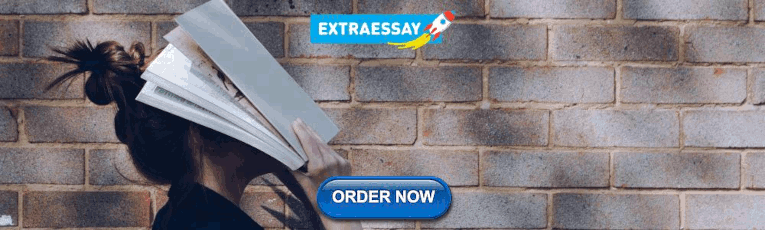
IMAGES
VIDEO
COMMENTS
This paper reviews the state of the art for optimally diagnosing and treating insomnia based on the available research evidence. DIAGNOSTIC CRITERIA FOR INSOMNIA The clinical diagnosis of insomnia is based on the complaint of trouble falling asleep, trouble staying asleep, or early morning awakening, and resultant daytime dysfunction 1 , 2 .
Introduction. The prevalence of insomnia in the general population ranges between 8-40%, depending on the definition used. While 20-30% of the general population has poor sleep (i.e., insomnia symptoms of difficulty initiating or maintaining sleep, early morning awakening, or non-restorative sleep at any given time), another 8-10% of the population suffers from chronic insomnia. 1,2 Also ...
Indeed, recent insights gained from research into the pathophysiology of insomnia have facilitated development of newer treatment approaches with more efficacious outcomes. This narrative review provides a summary of the diagnostic criteria and pathophysiology of insomnia and its subtypes. Further, this review emphasizes new and emerging ...
Background and purpose: Insomnia is a common and debilitating disorder that is frequently associated with important consequences for physical health and well-being. Methods: An international expert group considered the current state of knowledge based on the most relevant publications in the previous 5 years, discussed the current challenges in the field of insomnia and identified future ...
Insomnia. Dear readers of the Journal of Sleep Research, This virtual issue of JSR brings together ten articles published in 2019 and 2020 dealing with different aspects of insomnia. Insomnia, especially in its chronic form, is a frequent sleep disorder, probably the most frequent sleep disorder of all, afflicting approximately 10% of the adult ...
Insomnia is highly prevalent in clinical practice, occurring in up to 50% of primary care patients. Insomnia can present independently or alongside other medical conditions or mental health disorders and is a risk factor for the development and exacerbation of these other disorders if not treated. In 2016, the American College of Physicians recommended that insomnia be specifically targeted ...
This article summarizes epidemiologic evidence on insomnia, including the prevalence, incidence, and risk factors, as well as its course and consequences. Approximately 10% of the adult population suffers from an insomnia disorder and another 20% experiences occasional insomnia symptoms. Women, older adults, and people with socioeconomic ...
Insomnia disorder has known striking developments over the last few years. Partly due to advances in neuroimaging techniques and brain sciences, our understanding of insomnia disorder has become more fine-tuned. Besides, developments within psychological and psychiatric fields have contributed to improve conceptualization, assessment, and treatment of insomnia. In this paper, we present a list ...
Recent research suggests that insomnia and depression share common pathological processes that make individuals vulnerable to both conditions—specifically, abnormal regulation of CRF. ... Real, misreported, and backfilled adherence with paper sleep diaries Clegg-Kraynok M, Barnovsky L and Zhou E Sleep Medicine, 10.1016/j.sleep.2023.04.011, , ...
When defined as a sleep disorder, insomnia is characterise d by a. difficulty in falling asleep or remaining asleep, which may represe nt problems with sleep. mainte nance or early morning ...
Insomnia-the unwelcome experience of difficulty sleeping-is common and can be acute, intermittent, or chronic. Insomnia can be the presenting symptom for several common sleep disorders, but it also often occurs comorbidly with mental and physical health conditions. Evaluating the symptom of insomnia requires assessing-largely by history-whether ...
REVIEW. Introduction. Sleep is an essential biological function, which is crucial for neural. development, learning and memory, emotional regulation, cardiovascular and metabolic function, as well ...
Introduction. Insomnia is one of the most frequent sleep disorders with continuously increasing prevalence. About 30-50% of the US adult population exhibit insomnia symptoms, 15-20% display a short-term insomnia of <3 months, and 5-15% display a chronic insomnia of >3 months (1-3).Common diagnostic manuals include the ICSD-3 (International Classification of Sleep Disorders, 3 rd ...
3.1. Current models of insomnia. Insomnia symptoms may be acute, transient and chronic. Chronic insomnia, also currently referred to as "insomnia disorder", now has similar diagnostic criteria in the American Psychiatric Association's Diagnostic and Statistical Manual of Mental Disorders, Fifth Edition (DSM-5), and confirmed in the recent text revised version DSM-TR (American Psychiatric ...
Recent research suggests that insomnia and depression share common pathological processes that make individuals vulnerable to both conditions—specifically, abnormal regulation of CRF. CRF regulation has been extensively implicated in the pathogenesis of depression, and hyperactivity of the HPA axis and CRF neurons could account for the ...
Strategic opportunities in sleep and circadian research: report of the Joint Task Force of the Sleep Research Society and American Academy of Sleep Medicine. Sleep. 2014;37(2):219-227. Crossref Google Scholar; 74. Jackson CL, Walker JR, Brown MK, Das R, Jones NL. A workshop report on the causes and consequences of sleep health disparities. Sleep.
Insomnia disorder is a conditio n characterized by both. nocturnal and diurnal symptoms. I t involves a p redomi. nant complain t of dissatisfaction with sleep quality or. duration and is accom ...
In conclusion, to our knowledge, this is the first bibliometric study to identify the 100 most cited papers in insomnia research. Our results suggest that the outbreak of the COVID-19 epidemic is strongly associated with the onset of insomnia and stimulates the researcher's interest. The key words suggest "COVID-19;" "anxiety ...
Background To examine the effectiveness and safety of yoga of women with sleep problems by performing a systematic review and meta-analysis. Methods Medline/PubMed, ClinicalKey, ScienceDirect, Embase, PsycINFO, and the Cochrane Library were searched throughout the month of June, 2019. Randomized controlled trials comparing yoga groups with control groups in women with sleep problems were ...
Another important research avenue is the study of chrono-medical timing of sleep deprivation and light exposure for their positive effects on mood in depression. Present day research takes the view on insomnia, i.e., prolonged sleep latency, problems to maintain sleep, and early morning awakening, as a transdiagnostic symptom for many mental ...
Co-authors on the paper include Susanne Strohmaier, Rikuta Hamaya, A. Heather Eliassen, Walter C. Willet, and Eric B. Rimm. ... P30 ES000002, R01 CA163451, and R01 HD101101) and by funding from the European Research Council under the European Union's Horizon 2020 research and innovation program (grant agreement numbers 101053225).
Medical problems or illness. Many medical conditions and diseases can contribute to insomnia, including asthma, allergies, Parkinson's disease, hyperthyroidism, acid reflux, kidney disease, and cancer. Chronic pain is also a common cause of insomnia.. Medications. Many prescription drugs can interfere with sleep, including antidepressants, stimulants for ADHD, corticosteroids, thyroid ...
The National Institute of Mental Health (NIMH) hosted a virtual workshop on the placebo effect. The purpose of this workshop was to bring together experts in neurobiology, clinical trials, and regulatory science to examine placebo effects in drug, device, and psychosocial interventions for mental health conditions. Topics included interpretability of placebo signals within the context of ...
Research shows that genetics, familial history of insomnia, female gender and environmental stress influence how the sleep system responds to stress. Further work has identified neurobiological underpinnings for sleep reactivity involving disrupted cortical networks and dysregulation in the autonomic nervous system and hypothalamic-pituitary ...
Present day research takes the view on insomnia, i.e., prolonged sleep latency, problems to maintain sleep, and early morning awakening, as a transdiagnostic symptom for many mental disorders, being most closely related to depression. Studying insomnia from different angles as a transdiagnostic phenotype has opened many new perspectives for ...
Vonn said she has partnered with Idorsia, the Swiss pharmaceutical research company that makes the drug. Challenges Lindsey Vonn faced beyond insomnia The problems Vonn said she faced after ...
Strategies to treat insomnia may be promising targets for preventing CVDs. Associations between insomnia and cardiovascular diseases: a meta-review and meta-analysis of observational and Mendelian randomization studies ... King's College London British Heart Foundation Centre of Research Excellence, London, UK. PMID: 39167428 DOI: 10.5664/jcsm ...
Previous research 8-10 has suggested a bidirectional relationship between insomnia and depression, with poor sleep quality contributing to the development and maintenance of depressive symptoms, and depression exacerbating sleep disturbances. However, the underlying mechanisms of this relationship remain unclear, and further research is ...