
An official website of the United States government
The .gov means it’s official. Federal government websites often end in .gov or .mil. Before sharing sensitive information, make sure you’re on a federal government site.
The site is secure. The https:// ensures that you are connecting to the official website and that any information you provide is encrypted and transmitted securely.
- Publications
- Account settings
Preview improvements coming to the PMC website in October 2024. Learn More or Try it out now .
- Advanced Search
- Journal List
- Entropy (Basel)

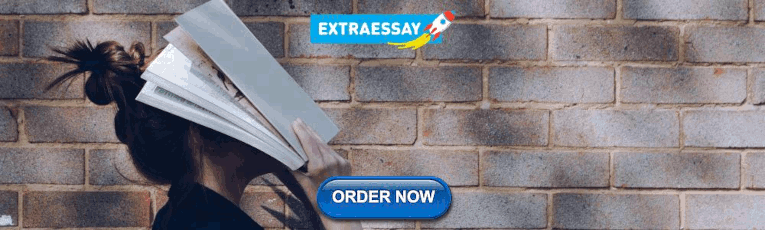
Stock Market Volatility and Return Analysis: A Systematic Literature Review
Roni bhowmik.
1 School of Economics and Management, Jiujiang University, Jiujiang 322227, China
2 Department of Business Administration, Daffodil International University, Dhaka 1207, Bangladesh
Shouyang Wang
3 Academy of Mathematics and Systems Science, Chinese Academy of Sciences, Beijing 100080, China; nc.ca.ssma@gnawys
In the field of business research method, a literature review is more relevant than ever. Even though there has been lack of integrity and inflexibility in traditional literature reviews with questions being raised about the quality and trustworthiness of these types of reviews. This research provides a literature review using a systematic database to examine and cross-reference snowballing. In this paper, previous studies featuring a generalized autoregressive conditional heteroskedastic (GARCH) family-based model stock market return and volatility have also been reviewed. The stock market plays a pivotal role in today’s world economic activities, named a “barometer” and “alarm” for economic and financial activities in a country or region. In order to prevent uncertainty and risk in the stock market, it is particularly important to measure effectively the volatility of stock index returns. However, the main purpose of this review is to examine effective GARCH models recommended for performing market returns and volatilities analysis. The secondary purpose of this review study is to conduct a content analysis of return and volatility literature reviews over a period of 12 years (2008–2019) and in 50 different papers. The study found that there has been a significant change in research work within the past 10 years and most of researchers have worked for developing stock markets.
1. Introduction
In the context of economic globalization, especially after the impact of the contemporary international financial crisis, the stock market has experienced unprecedented fluctuations. This volatility increases the uncertainty and risk of the stock market and is detrimental to the normal operation of the stock market. To reduce this uncertainty, it is particularly important to measure accurately the volatility of stock index returns. At the same time, due to the important position of the stock market in the global economy, the beneficial development of the stock market has become the focus. Therefore, the knowledge of theoretical and literature significance of volatility are needed to measure the volatility of stock index returns.
Volatility is a hot issue in economic and financial research. Volatility is one of the most important characteristics of financial markets. It is directly related to market uncertainty and affects the investment behavior of enterprises and individuals. A study of the volatility of financial asset returns is also one of the core issues in modern financial research and this volatility is often described and measured by the variance of the rate of return. However, forecasting perfect market volatility is difficult work and despite the availability of various models and techniques, not all of them work equally for all stock markets. It is for this reason that researchers and financial analysts face such a complexity in market returns and volatilities forecasting.
The traditional econometric model often assumes that the variance is constant, that is, the variance is kept constant at different times. An accurate measurement of the rate of return’s fluctuation is directly related to the correctness of portfolio selection, the effectiveness of risk management, and the rationality of asset pricing. However, with the development of financial theory and the deepening of empirical research, it was found that this assumption is not reasonable. Additionally, the volatility of asset prices is one of the most puzzling phenomena in financial economics. It is a great challenge for investors to get a pure understanding of volatility.
A literature reviews act as a significant part of all kinds of research work. Literature reviews serve as a foundation for knowledge progress, make guidelines for plan and practice, provide grounds of an effect, and, if well guided, have the capacity to create new ideas and directions for a particular area [ 1 ]. Similarly, they carry out as the basis for future research and theory work. This paper conducts a literature review of stock returns and volatility analysis based on generalized autoregressive conditional heteroskedastic (GARCH) family models. Volatility refers to the degree of dispersion of random variables.
Financial market volatility is mainly reflected in the deviation of the expected future value of assets. The possibility, that is, volatility, represents the uncertainty of the future price of an asset. This uncertainty is usually characterized by variance or standard deviation. There are currently two main explanations in the academic world for the relationship between these two: The leverage effect and the volatility feedback hypothesis. Leverage often means that unfavorable news appears, stock price falls, leading to an increase in the leverage factor, and thus the degree of stock volatility increases. Conversely, the degree of volatility weakens; volatility feedback can be simply described as unpredictable stock volatility that will inevitably lead to higher risk in the future.
There are many factors that affect price movements in the stock market. Firstly, there is the impact of monetary policy on the stock market, which is extremely substantial. If a loose monetary policy is implemented in a year, the probability of a stock market index rise will increase. On the other hand, if a relatively tight monetary policy is implemented in a year, the probability of a stock market index decline will increase. Secondly, there is the impact of interest rate liberalization on risk-free interest rates. Looking at the major global capital markets, the change in risk-free interest rates has a greater correlation with the current stock market. In general, when interest rates continue to rise, the risk-free interest rate will rise, and the cost of capital invested in the stock market will rise simultaneously. As a result, the economy is expected to gradually pick up during the release of the reform dividend, and the stock market is expected to achieve a higher return on investment.
Volatility is the tendency for prices to change unexpectedly [ 2 ], however, all kinds of volatility is not bad. At the same time, financial market volatility has also a direct impact on macroeconomic and financial stability. Important economic risk factors are generally highly valued by governments around the world. Therefore, research on the volatility of financial markets has always been the focus of financial economists and financial practitioners. Nowadays, a large part of the literature has studied some characteristics of the stock market, such as the leverage effect of volatility, the short-term memory of volatility, and the GARCH effect, etc., but some researchers show that when adopting short-term memory by the GARCH model, there is usually a confusing phenomenon, as the sampling interval tends to zero. The characterization of the tail of the yield generally assumes an ideal situation, that is, obeys the normal distribution, but this perfect situation is usually not established.
Researchers have proposed different distributed models in order to better describe the thick tail of the daily rate of return. Engle [ 3 ] first proposed an autoregressive conditional heteroscedasticity model (ARCH model) to characterize some possible correlations of the conditional variance of the prediction error. Bollerslev [ 4 ] has been extended it to form a generalized autoregressive conditional heteroskedastic model (GARCH model). Later, the GARCH model rapidly expanded and a GARCH family model was created.
When employing GARCH family models to analyze and forecast return volatility, selection of input variables for forecasting is crucial as the appropriate and essential condition will be given for the method to have a stationary solution and perfect matching [ 5 ]. It has been shown in several findings that the unchanged model can produce suggestively different results when it is consumed with different inputs. Thus, another key purpose of this literature review is to observe studies which use directional prediction accuracy model as a yardstick from a realistic point of understanding and has the core objective of the forecast of financial time series in stock market return. Researchers estimate little forecast error, namely measured as mean absolute deviation (MAD), root mean squared error (RMSE), mean absolute error (MAE), and mean squared error (MSE) which do not essentially interpret into capital gain [ 6 , 7 ]. Some others mention that the predictions are not required to be precise in terms of NMSE (normalized mean squared error) [ 8 ]. It means that finding the low rate of root mean squared error does not feed high returns, in another words, the relationship is not linear between two.
In this manuscript, it is proposed to categorize the studies not only by their model selection standards but also for the inputs used for the return volatility as well as how precise it is spending them in terms of return directions. In this investigation, the authors repute studies which use percentage of success trades benchmark procedures for analyzing the researchers’ proposed models. From this theme, this study’s authentic approach is compared with earlier models in the literature review for input variables used for forecasting volatility and how precise they are in analyzing the direction of the related time series. There are other review studies on return and volatility analysis and GARCH-family based financial forecasting methods done by a number of researchers [ 9 , 10 , 11 , 12 , 13 ]. Consequently, the aim of this manuscript is to put forward the importance of sufficient and necessary conditions for model selection and contribute for the better understanding of academic researchers and financial practitioners.
Systematic reviews have most notable been expanded by medical science as a way to synthesize research recognition in a systematic, transparent, and reproducible process. Despite the opportunity of this technique, its exercise has not been overly widespread in business research, but it is expanding day by day. In this paper, the authors have used the systematic review process because the target of a systematic review is to determine all empirical indication that fits the pre-decided inclusion criteria or standard of response to a certain research question. Researchers proved that GARCH is the most suitable model to use when one has to analysis the volatility of the returns of stocks with big volumes of observations [ 3 , 4 , 6 , 9 , 13 ]. Researchers observe keenly all the selected literature to answer the following research question: What are the effective GARCH models to recommend for performing market volatility and return analysis?
The main contribution of this paper is found in the following four aspects: (1) The best GARCH models can be recommended for stock market returns and volatilities evaluation. (2) The manuscript considers recent papers, 2008 to 2019, which have not been covered in previous studies. (3) In this study, both qualitative and quantitative processes have been used to examine the literature involving stock returns and volatilities. (4) The manuscript provides a study based on journals that will help academics and researchers recognize important journals that they can denote for a literature review, recognize factors motivating analysis stock returns and volatilities, and can publish their worth study manuscripts.
2. Methodology
A systematic literature examination of databases should recognize as complete a list as possible of relevant literature while keeping the number of irrelevant knocks small. The study is conducted by a systematic based literature review, following suggestions from scholars [ 14 , 15 ]. This manuscript was led by a systematic database search, surveyed by cross-reference snowballing, as demonstrated in Figure 1 , which was adapted from Geissdoerfer et al. [ 16 ]. Two databases were selected for the literature search: Scopus and Web-of-Science. These databases were preferred as they have some major depositories of research and are usually used in literature reviews for business research [ 17 ].

Literature review method.
At first stage, a systematic literature search is managed. The keywords that were too broad or likely to be recognized in literature-related keywords with other research areas are specified below. As shown in Table 1 , the search string “market return” in ‘Title‘ respectively “stock market return”, “stock market volatility”, “stock market return volatility”, “GARCH family model* for stock return”, “forecasting stock return”, and GARCH model*, “financial market return and volatility” in ‘Topic’ separately ‘Article title, Abstract, Keywords’ were used to search for reviews of articles in English on the Elsevier Scopus and Thomson Reuters Web-of-Science databases. The asterisk (*) is a commonly used wildcard symbol that broadens a search by finding words that start with the same letters.
Literature search strings for database.
Search String | Search Field | Number of Non-Exclusive Results | ||
---|---|---|---|---|
Scopus | Web-of-Science | Last Updated | ||
Market Return | Title/Article title | 1540 | 1148 | 17 January 2020 |
Market volatility | Topic/Article title, Abstract, Keywords | 13,892 | 13,767 | 17 January 2020 |
Stock market return | Topic/Article title, Abstract, Keywords | 11,567 | 13,440 | 17 January 2020 |
Stock market volatility | Topic/Article title, Abstract, Keywords | 5683 | 6853 | 17 January 2020 |
Market return and volatility | Topic/Article title, Abstract, Keywords | 3241 | 6632 | 17 January 2020 |
GARCH family model* for stock return | Topic/Article title, Abstract, Keywords | 53 | 41 | 17 January 2020 |
Forecasting stock return and GARCH model* | Topic/Article title, Abstract, Keywords | 227 | 349 | 17 January 2020 |
Financial market return and volatility | Topic/Article title, Abstract, Keywords | 2212 | 2638 | 17 January 2020 |
At second stage, suitable cross-references were recognized in this primary sample by first examining the publications’ title in the reference portion and their context and cited content in the text. The abstracts of the recognized further publications were examined to determine whether the paper was appropriate or not. Appropriate references were consequently added to the sample and analogously scanned for appropriate cross-references. This method was continual until no additional appropriate cross-references could be recognized.
At the third stage, the ultimate sample was assimilated, synthesized, and compiled into the literature review presented in the subsequent section. The method was revised a few days before the submission.
Additionally, the list of affiliation criteria in Table 2 , which is formed on discussions of the authors, with the summaries of all research papers were independently checked in a blind system method. Evaluations were established on the content of the abstract, with any extra information unseen, and were comprehensive rather than exclusive. In order to check for inter-coder dependability, an initial sample of 30 abstracts were studied for affiliation by the authors. If the abstract was not satisfactorily enough, the whole paper was studied. Simply, 4.61 percent of the abstract resulted in variance between the researchers. The above-mentioned stages reduced the subsequent number of full papers for examination and synthesis to 50. In order to recognize magnitudes, backgrounds, and moderators, these residual research papers were reviewed in two rounds of reading.
Affiliation criteria.
Affiliation Criteria | Rational Explanation |
---|---|
Abstract must express the stock market and GARCH model as the sharp object of this research work. | Since this kind of research is not restricted to any journals, research on other subjects than stock market maybe appears. |
Abstract must show clear indication of stock market volatility and return studies through GARCH model robustness. | The focus of the research is to study stock market return and volatility analysis by GARCH family model. |
Research paper must be written in English language. | English language is the leading research language in the arena of finance. |
3. Review of Different Studies
In this paper, a large amount of articles were studied but only a few were well thought out to gather the quality developed earlier. For every published article, three groups were specified. Those groups were considered as index and forecast time period, input elements, econometric models, and study results. The first group namely “index and forecast time period with input elements” was considered since market situation like emerging, frontier, and developed markets which are important parameters of forecast and also the length of evaluation is a necessary characteristic for examining the robustness of the model. Furthermore, input elements are comparatively essential parameters for a forecast model because the analytical and diagnostic ability of the model is mainly supported on the inputs that a variable uses. In the second group, “model” was considered forecast models proposed by authors and other models for assessment. The last group is important to our examination for comparing studies in relationships of proper guiding return and volatility, acquired by using recommended estimate models, named the “study results” group.
Measuring the stock market volatility is an incredibly complex job for researchers. Since volatility tends to cluster, if today’s volatility is high, it is likely to be high tomorrow but they have also had an attractive high hit rate with major disasters [ 4 , 7 , 11 , 12 ]. GARCH models have a strong background, recently having crossed 30 years of the fast progress of GARCH-type models for investigating the volatility of market data. Literature of eligible papers were clustered in two sub groups, the first group containing GARCH and its variations model, and the second group containing bivariate and other multivariate GARCH models, summarized in a table format for future studies. Table 3 explains the review of GARCH and its variations models. The univariate GARCH model is for a single time series. It is a statistical model that is used to analyze a number of different kinds of financial data. Financial institutions and researchers usually use this model to estimate the volatility of returns for stocks, bonds, and market indices. In the GARCH model, current volatility is influenced by past innovation to volatility. GARCH models are used to model for forecast volatility of one time series. The most widely used GARCH form is GARCH (1, 1) and this has some extensions.
Different literature studies based on generalized autoregressive conditional heteroskedastic (GARCH) and its variations models.
Authors | Data Set | Econometric Models | Study Results |
---|---|---|---|
[ ] | Daily returns data, TASE indices, the TA25 index period October 1992 to May 2005 and TA100 index period July 1997 to May 2005 | GARCH, EGARCH, and APARCH model | Findings suggest that one can improve overall estimation by using the asymmetric GARCH model and the EGARCH model is a better predictor than the other asymmetric models. |
[ ] | Daily returns over the period January 2004 to March 2009 | EGARCH in mean model | Nigerian stock market returns show that volatility is persistent and there is a leverage effect. The study found little evidence open the relationship between stock returns and risk as measures by its aim volatility. |
[ ] | Examine the interaction of volatility and volume in 79 traded companies in Cairo and Alexandria Stock Exchange | GARCH model | They found that information size and direction have a negligible effect on conditional volatility and, as a result, the presence of noise trading and speculative bubbles is suspected. |
[ ] | Six years’ data from March 2003 to March 2009 for four US stock indices i.e., Dow Jones, Nasdaq, NYSE, S&P500 | ARCH, GARCH (1,1), EGARCH (1,1) Multivariate volatility models | The study concludes that EGARCH model is that best fitted process for all the sample data based on AIC minimum criterion. It is observed that there are high volatility periods at the beginning and at the end of our estimation period for all stock indices. |
[ ] | Daily OHLC values of NSE index returns from 2005–2008 | Rolling window moving average estimator, EWMA, GARCH models, Extreme value indicators, and Volatility index (VIX) | A GARCH and VIX models, proved to be the best methods. Extreme value models fail to perform because of low frequency data. |
[ ] | Taiwanese stock index futures prices, daily data April 2001 to December 2008 | GARCH type models: GARCH, GJR-GARCH, QGARCH, EGARCH, IGARCH, CGARCH | They demonstrate that the EGARCH model provides the most accurate daily volatility forecasts, while the performances of the standard GARCH model and the GARCH models with highly persistent and long-memory characteristics are relatively poor. |
[ ] | Daily closing price from January 2005 to May 2009 | BDS Test, ARCH-LM test, and GARCH (1,1) model | Persistence of volatility is more than Indian stock market |
[ ] | Hong Kong stock market from 1984 to 2009 | GARCH family models | The EGARCH and AGARCH models can detect the asymmetric effect well in response to both good news and bad news. By comparing different GARCH models, they find that it is the EGARCH model that best fits the Hong Kong case. |
[ ] | Taiwan Stock Exchange (TAIEX), the S&P 500 Index, and the Nasdaq Composite Index for the period of January, 2000 to January, 2004 | GJR-GARCH model (1,1) | There is a significant price transmission effect and volatility asymmetry among the TAIEX, the US spot index, and US index futures. |
[ ] | Shanghai stock exchange Ten industries sector indices daily data ranging from January 2009 to June 2012 | Volatility estimation AR (1), EGARCH (1,1) | Time varying beta risk of industry sector indices in Shanghai stock results industries respond positively to rises in such non-diversifiable risk. Reports on the volatility persistence of the various industry sectors and identifies which industries have high and low persistence. |
[ ] | New York, London and Tokyo as well as those of Hong Kong, Shanghai and Shenzen the period of January 1993 to March 2010 | Granger causality test, VAR model, VEC model, variance decomposition, impulse response function, co-integration and GARCH models | Evidence shows that five stock markets are in the process of increasing integration. The periodic break down of co-integrating relationship is advantageous to foreign investors. |
[ ] | Saudi stock market by using (Tadawul All Share Index; TASI) over the period of January 2007 to November 2011 | GARCH (1,1) model, including both symmetric and asymmetric models | The results provide evidence of the existence of a positive risk premium, which supports the positive correlation hypothesis between volatility and the expected stock returns. |
[ ] | Daily closing price of BSE and NSE stock indices period of January 2008 to August 2011 | ARFIMA and FIGARCH models | Absence of long memory in return series of the Indian stock market. Strong evidence of long memory in conditional variance of stock indices. |
[ ] | China stock indices, six industry indexes, January 2006 to June 2012 | ARMA and GARCH family model, GARCH (1,1), TGARCH (1,1), EGARCH (1,1) | The paper examined the leverage effect and information symmetry. Both ARCH and GARCH models can explain volatility clustering phenomena and have been quite successful in modeling real data in various applications. |
[ ] | Daily closing prices of the SHCI and SZCI indices from January 1997 to August 2007 | GARCH family models | An asymmetric effect of negative news exists in the Chinese stock markets. The EGARCH and the GJR models tend to overestimate the volatility and returns in the high-volatility periods. |
[ ] | Daily closing data for November 2009 to March 2013, NIFTY and NIFTY Junior indices | ADF Test, Johansen’s co-integration test, and GARCH (1,1) model | Empirical results found that one-month futures do not bring volatility in the VIX. |
[ ] | Daily data of sectoral indices for the period of January 2001 to June 2014 | ARMA (1,1), and GARCH (1,1) models | Return of the BSE sectoral indices exhibit characteristics of normality, stationarity, and heteroscedasticity. |
[ ] | MENA stock market indices of daily observations for the period January 2007 to March 2012 | GARCH family models | MENA region’s markets are higher between extremes than between ordinary observations registered during normal periods, but they offer many opportunities to investors to diversify their portfolio and reduce their degree of risk aversion. Dependence between markets increases during volatile periods. |
[ ] | The daily closing prices of S&P CNX500 of National Stock Exchange for the period from January 2003 to December 2012 | GARCH, TGARCH, and EGARCH models | The result of that volatility varies over time and constant variance assumption is inconsistent. The empirical evidence indicated the presence of time varying volatility. |
[ ] | S&P500 market daily returns the sample period from July 1996 to May 2006 | GARCH family models | Results of ANN models will be compared with time series model using GARCH family models. The use of the novel model for conditional stock markets returns volatility can handle the vast amount of nonlinear data, simulate their relationship, and give a moderate solution for the hard problem. |
[ ] | The daily closing prices of S&P CNX Nifty Index for the period from January 2003 to December 2012 | Both symmetric and asymmetric models GARCH (1,1) | The result proves that GARCH and TGARCH estimations are found to be the most appropriate model to capture symmetric and asymmetric volatility respectively. |
[ ] | Central and Eastern Europe region for the period from October 2005 to December 2013 | Both symmetric and asymmetric GARCH models, i.e.,; GARCH, IGARCH, EGARCH, GJR, and PGARCH | Study indicate that existence of the leverage effect in case of stock markets from the CEE region, which indicates that negative shocks increase the volatility more than positive shocks. |
[ ] | Australian share markets data for the period of January 1988 to December 2004 | GARCH family models | Findings support asymmetric effects in the Australian share markets, and by incorporating them into the GARCH-M models yield better results in both financial and econometric terms. |
[ ] | Asian countries, i.e., Pakistan, India, Sri Lanka, China, Japan, and Hong Kong. The daily data was considered from the period January 1999 to January 2014 | GARCH model | Result revealed absence of any spillover effect of volatility across Indian and Chinese stock markets. However, bidirectional and unidirectional spillover effects have been established across other Asian markets. |
[ ] | CSI 300 index consider for the period of July 2013 to January 2016 | GARCH, EGARCH, APARCH, and PTTGARCH models | The PTTGARCH models both with single regime and Markov regime switching outperform other models in estimation and prediction of the volatilities of the return series within the sample and out-of-sample. |
[ ] | India stock market daily data for the period of April 2003 to March 2015 | GARCH, EGARCH, and TARCH models | The existence of volatility clustering and leverage effect in the market and the investment activities of foreign portfolio investment have had a significant impact on the volatility of stock market. |
[ ] | India NIFTY Volatility Index (IVIX) and CNX NIFTY Index (NIFTY), Australia S&P/ASX 200 Volatility Index (AVIX) and S&P/ASX 200 Index (ASX), and Hong Kong Hang Seng Volatility Index (VHSI) and HSI, consider the period of January 2008 to July 2016 | GARCH family models | The study finds that volatility index is a biased forecast but possesses relevant information in explaining future realized volatility. GARCH family models suggest that it contains relevant information in describing the volatility process. |
[ ] | The EUR/JPY exchange rate of daily prices and time period considered from 1999 to 2005 | GARCH model, Entropy, and VAR model | GARCH-based forecast is more stable whilst the entropy-based forecast reacts faster to new information. VAR model performs the worst failing the tests, whilst the normal GARCH model passes all tests. But the best results overall are obtained by the entropy-based forecast model. |
[ ] | Emerging six Asian stock markets daily stock market index data from January 2002 to December 2016 | GARCH model, Granger Causality Tests, and VAR model | The volatility and return spillovers behave very differently over time, during the pre-crisis, crisis, and post crisis periods. Importantly, the Asian emerging stock markets interaction was less before the global financial crisis period. |
[ ] | Daily negative returns of the Google’s stock price and Dow Jones index, November 2004 to November 2016 | PTTGARCH model | Article demonstrates its validity through a simulation study and real data analysis. The result indicates that for practical applications, the underlying innovation distribution should be modeled in a more refined manner. |
[ ] | NSE from the period of April 2003 to September 2015 | GARCH family models | The findings reported an evidence of volatility, which exhibited the clustering and persistence of stocks. The return series of the stocks selected for the study were found to react on good and bad news asymmetrically. |
[ ] | US stock return a daily frequency S&P500 index covering the period from January 2004 to December 2016 | GARCH family models | The SVI variable exhibits the best performance among all considered models and SVI variable offers the highest gains for investors. |
[ ] | BSE 30, SSE composite, DSEX, FBMKLCI, PSEi, KOSPI indices data of daily closing prices for the period of January 2007 to 2016 | GARCH family models and VAR model | The returns and volatility linkages exist between the emerging Asian markets and the developed stock markets. The volatilities to unexpected shocks in various markets, especially, come from neighboring country markets and more developed country markets. |
[ ] | Borsa Istanbul sector indices of daily data over the period of October 1987 to January 2017 | GARCH model | Model shows the existence of a positive and statistically significant relationships between trading volume and the number of information events makes the variability of the sector indices to increase. |
[ ] | High frequency data, stock market policies issued related news, January 2014 to August 2015 | GARCH-M and EGARCH-M models | The results show that China’s stock market was mainly driven by government policies rather than economic fundamentals, as measured by GDP, PPI, and PMI. |
[ ] | Nifty 50 and BSE Sensex daily data from both indices over the period of January 1995 to December 2015 | GARCH, TGARCH, and EGARCH models | The study indicates that symmetric information is not suitable for a certain period considered in this study. The TGARCH model outperformed all the models due to the availability of information. |
[ ] | The data consists of daily, weekly, and monthly closing prices of six emerging stock market indexes in Asian countries from the period of 2007 to 2016 | Unit root tests, serial correlation test, runs test, VR tests, ARMA, GARCH model, and BDS test | Study suggests that none of the sample Asian emerging stock markets follow Random-walk and hence all are weak-form efficient markets except South Korean Markets. Additionally, short-term variants of the technical trading rules have better predictive ability than long-term variants. |
[ ] | BSE and NSE daily data of the closing value from April 2011 to March 2017 | GARCH family models | The study suggested that the P-GARCH model is most suitable to predict and forecast the stock market volatility for BSE and NSE markets. |
[ ] | Brazil, India, Indonesia and Pakistan stock markets return of the average price (open, close, high, and low) for January 2014 to October 2018 | GARCH family models | The result confirms the presence of volatility clustering and leverage effect that is that good news affects the future stock market than bad news. |
Notes: APARCH (Asymmetric Power ARCH), AIC (Akaike Information Criterion), OHLC (Open-High-Low-Close Chart), NSE (National Stock Exchange of India), EWMA (Exponentially Weighted Moving Average), CGARCH (Component GARCH), BDS (Brock, Dechert & Scheinkman) Test, ARCH-LM (ARCH-Lagrange Multiplier) test, VAR (Vector Autoregression) model, VEC (Vector Error Correction) model, ARFIMA (Autoregressive Fractional Integral Moving Average), FIGARCH (Fractionally Integrated GARCH), SHCI (Shanghai Stock Exchange Composite Index), SZCI (Shenzhen Stock Exchange Component Index), ADF (Augmented Dickey–Fuller) test, BSE (Bombay Stock Exchange), and PGARCH (Periodic GARCH) are discussed.
In a simple GARCH model, the squared volatility σ t 2 is allowed to change on previous squared volatilities, as well as previous squared values of the process. The conditional variance satisfies the following form: σ t 2 = α 0 + α 1 ϵ t − 1 2 + … + α q ϵ t − q 2 + β 1 σ t − 1 2 + … + β p σ t − p 2 where, α i > 0 and β i > 0 . For the GARCH model, residuals’ lags can substitute by a limited number of lags of conditional variances, which abridges the lag structure and in addition the estimation method of coefficients. The most often used GARCH model is the GARCH (1, 1) model. The GARCH (1, 1) process is a covariance-stationary white noise process if and only if α 1 + β < 1 . The variance of the covariance-stationary process is given by α 1 / ( 1 − α 1 − β ) . It specifies that σ n 2 is based on the most recent observation of φ t 2 and the most recent variance rate σ n − 1 2 . The GARCH (1, 1) model can be written as σ n 2 = ω + α φ n − 1 2 + β σ n − 1 2 and this is usually used for the estimation of parameters in the univariate case.
Though, GARCH model is not a complete model, and thus could be developed, these developments are detected in the form of the alphabet soup that uses GARCH as its key component. There are various additions of the standard GARCH family models. Nonlinear GARCH (NGARCH) was proposed by Engle and Ng [ 18 ]. The conditional covariance equation is in the form: σ t 2 = γ + α ( ε t − 1 − ϑ σ t − 1 ) 2 + β σ t − 1 2 , where α , β , γ > 0 . The integrated GARCH (IGARCH) is a restricted version of the GARCH model, where the sum of all the parameters sum up to one and this model was introduced by Engle and Bollerslev [ 19 ]. Its phenomenon might be caused by random level shifts in volatility. The simple GARCH model fails in describing the “leverage effects” which are detected in the financial time series data. The exponential GARCH (EGARCH) introduced by Nelson [ 5 ] is to model the logarithm of the variance rather than the level and this model accounts for an asymmetric response to a shock. The GARCH-in-mean (GARCH-M) model adds a heteroskedasticity term into the mean equation and was introduced by Engle et al. [ 20 ]. The quadratic GARCH (QGARCH) model can handle asymmetric effects of positive and negative shocks and this model was introduced by Sentana [ 21 ]. The Glosten-Jagannathan-Runkle GARCH (GJR-GARCH) model was introduced by Glosten et al. [ 22 ], its opposite effects of negative and positive shocks taking into account the leverage fact. The threshold GARCH (TGARCH) model was introduced by Zakoian [ 23 ], this model is also commonly used to handle leverage effects of good news and bad news on volatility. The family GARCH (FGARCH) model was introduced by Hentschel [ 24 ] and is an omnibus model that is a mix of other symmetric or asymmetric GARCH models. The COGARCH model was introduced by Klüppelberg et al. [ 25 ] and is actually the stochastic volatility model, being an extension of the GARCH time series concept to continuous time. The power-transformed and threshold GARCH (PTTGARCH) model was introduced by Pan et al. [ 26 ], this model is a very flexible model and, under certain conditions, includes several ARCH/GARCH models.
Based on the researchers’ articles, the symmetric GARCH (1, 1) model has been used widely to forecast the unconditional volatility in the stock market and time series data, and has been able to simulate the asset yield structure and implied volatility structure. Most researchers show that GARCH (1, 1) with a generalized distribution of residual has more advantages in volatility assessment than other models. Conversely, the asymmetry influence in stock market volatility and return analysis was beyond the descriptive power of the asymmetric GARCH models, as the models could capture more specifics. Besides, the asymmetric GARCH models can incompletely measure the effect of positive or negative shocks in stock market return and volatility, and the GARCH (1, 1) comparatively failed to accomplish this fact. In asymmetric effect, the GJR-GARCH model performed better and produced a higher predictable conditional variance during the period of high volatility. In addition, among the asymmetric GARCH models, the reflection of EGARCH model appeared to be superior.
Table 4 has explained the review of bivariate and other multivariate GARCH models. Bivariate model analysis was used to find out if there is a relationship between two different variables. Bivariate model uses one dependent variable and one independent variable. Additionally, the Multivariate GARCH model is a model for two or more time series. Multivariate GARCH models are used to model for forecast volatility of several time series when there are some linkages between them. Multivariate model uses one dependent variable and more than one independent variable. In this case, the current volatility of one time series is influenced not only by its own past innovation, but also by past innovations to volatilities of other time series.
Different literature studies based on bivariate and other multivariate GARCH models.
Authors | Data Set | Econometric Models | Study Results |
---|---|---|---|
[ ] | 15 world indices for the period of January 2000 to February 2008 have been considered | AR-GARCH, bivariate VAR, Multivariate GARCH (BEKK) model | There is significant positive volatility spillover from other markets to Indian market, mainly from Hong Kong, Korea, Japan, and Singapore and US market. Indian market affects negatively the volatility of US and Pakistan. |
[ ] | Daily returns data from February 2003 to January 2006, Arabian Gulf Cooperation Council equity markets data | MGARCH and VAR models | Arabian Gulf Cooperation Council markets exhibit significant own and cross spillover of innovations and volatility spillover and persistence in these markets. |
[ ] | S&P 500, NIKKE 225, KSE 100, BSE 30, Hang Seng indices. Daily closing Index and data from January 1989 to December 2009 | ARCH, GARCH models, GARCH-BEKK model correlation, unit root tests, granger-causality test | Time varying correlation increases in bearish spells whereas bullish periods do not have a big “Statistical” impact on correlation. |
] | Daily returns of Prague stock exchange index and other 11 major stock indices during 1994 to 2009 | DCC-MVGARCH model | The study found the existence of an increasing trend in conditional correlations among a whole European region. Results show the unidirectional influence of foreign markets affecting Czech market. |
[ ] | Daily data of Japanese stock over the study period 1994–2007 | BEKK-GARCH model | They found that news shocks in the Japanese currency market account for volatility transmission in eight of the 10 industrial sectors considered. They also found that significant asymmetric effects in five of these industries. |
[ ] | Weekly stock market data of Australia, Singapore, UK, and the US for the period from Jan 1992 to June 2010 | M-GARCH Model, Diagonal BEKK model ARCH, and GARCH techniques | Positive return spillover effects are only unidirectional and run from both US and UK (the bigger markets) to Australia and Singapore (the smaller markets). Shocks arising from the US market can impact on all of the other markets in the sample. |
[ ] | Five sectors daily data covering period from January 2002 to October 2009 | VAR Framework one lag, BEKK (1,1) model | International financial markets are not integrated in all the sectors. Results find that the three highly integrated sectors are bank, real estate, and oil. |
[ ] | The weekly closing stock indexes and local currency and exchange rates used for four emerging markets, data from December 1994 to March 2009 | Markov-Switching-EGARCH model | Results provide strong evidence that the relationship between stock and foreign exchange market is regime dependent and stock price volatility responds asymmetrically to events in the foreign exchange market. |
] | Daily closing prices of six largest industrial sector composite total return indices during January 2002 to April 2013 | AR (1) model, MV-GARCH models, DCC models, VECH, and BEKK techniques, and GJR-GARCH model | The results show that global and domestic economic uncertainty as well as local asset market segment significantly influences both the short run dynamics and the aggregate level of co-movement between local sector pairs. |
[ ] | TAIEX and Nikkei from both indices over the period of January, 2000 to March, 2016 | Bi-EGARCH model | The past returns on NIKKEI influenced significantly current period returns of TAIEX, yet there was no such influence flowing from past returns of TAIEX to the current returns on NIKKEI index. Furthermore, the two stock markets are more sensitive to falling rather than rising trends of each other, implying that there is a mutual tendency between these markets to crash due to a retreat in the counterpart market. |
[ ] | GEM index china, daily return data over the period of January 2014 to June 2018 | DCC-MV-GARCH model, bivariate EGARCH model and VECM model | The network entropy indices increased in the period of the market crash. Equity market-trading activity and network entropy were informationally efficient in the long run and the more heterogeneous the stock network is, the higher market returns. |
The most recognizable use of multivariate GARCH models is the analysis of the relations between the volatilities and co-volatilities of several markets. A multivariate model would create a more dependable model than separate univariate models. The vector error correction (VEC) models is the first MGARCH model which was introduced by Bollerslev et al. [ 66 ]. This model is typically related to subsequent formulations. The model can be expressed in the following form: v e c h ( H t ) = ℂ + ∑ j = 1 q X j v e c h ( ϵ t − j ϵ t − j ' ) + ∑ j = 1 p Y j v e c h ( H t − j ) where v e c h is an operator that stacks the columns of the lower triangular part of its argument square matrix and H t is the covariance matrix of the residuals. The regulated version of the VEC model is the DVEC model and was also recommended by Bollerslev et al. [ 66 ]. Compared to the VEC model, the estimation method proceeded far more smoothly in the DVEC model. The Baba-Engle-Kraft-Kroner (BEKK) model was introduced by Baba et al. [ 67 ] and is an innovative parameterization of the conditional variance matrix H t . The BEKK model accomplishes the positive assurance of the conditional covariance by conveying the model in a way that this property is implied by the model structure. The Constant Conditional Correlation (CCC) model was recommended by Bollerslev [ 68 ], to primarily model the conditional covariance matrix circuitously by estimating the conditional correlation matrix. The Dynamic Conditional Correlation (DCC) model was introduced by Engle [ 69 ] and is a nonlinear mixture of univariate GARCH models and also a generalized variety of the CCC model. To overcome the inconveniency of huge number of parameters, the O-GARCH model was recommended by Alexander and Chibumba [ 70 ] and consequently developed by Alexander [ 71 , 72 ]. Furthermore, a multivariate GARCH model GO-GARCH model was introduced by Bauwens et al. [ 73 ].
The bivariate models showed achieve better in most cases, compared with the univariate models [ 85 ]. MGARCH models could be used for forecasting. Multivariate GARCH modeling delivered a realistic but parsimonious measurement of the variance matrix, confirming its positivity. However, by analyzing the relative forecasting accuracy of the two formulations, BEKK and DCC, it could be deduced that the forecasting performance of the MGARCH models was not always satisfactory. By comparing it with the other multivariate GARCH models, BEKK-GARCH model was comparatively better and flexible but it needed too many parameters for multiple time series. Conversely, for the area of forecasting, the DCC-GARCH model was more parsimonious. In this regard, it was significantly essential to balance parsimony and flexibility when modeling multivariate GARCH models.
The current systematic review has identified 50 research articles for studies on significant aspects of stock market return and volatility, review types, and GARCH model analysis. This paper noticed that all the studies in this review used an investigational research method. A literature review is necessary for scholars, academics, and practitioners. However, assessing various kinds of literature reviews can be challenging. There is no use for outstanding and demanding literature review articles, since if they do not provide a sufficient contribution and something that is recent, it will not be published. Too often, literature reviews are fairly descriptive overviews of research carried out among particular years that draw data on the number of articles published, subject matter covered, authors represented, and maybe methods used, without conducting a deeper investigation. However, conducting a literature review and examining its standard can be challenging, for this reason, this article provides some rigorous literature reviews and, in the long run, to provide better research.
4. Conclusions
Working on a literature review is a challenge. This paper presents a comprehensive literature which has mainly focused on studies on return and volatility of stock market using systematic review methods on various financial markets around the world. This review was driven by researchers’ available recommendations for accompanying systematic literature reviews to search, examine, and categorize all existing and accessible literature on market volatility and returns [ 16 ]. Out of the 435 initial research articles located in renowned electronic databases, 50 appropriate research articles were extracted through cross-reference snowballing. These research articles were evaluated for the quality of proof they produced and were further examined. The raw data were offered by the authors from the literature together with explanations of the data and key fundamental concepts. The outcomes, in this research, delivered future magnitudes to research experts for further work on the return and volatility of stock market.
Stock market return and volatility analysis is a relatively important and emerging field of research. There has been plenty of research on financial market volatility and return because of easily increasing accessibility and availability of researchable data and computing capability. The GARCH type models have a good model on stock market volatilities and returns investigation. The popularity of various GARCH family models has increased in recent times. Every model has its specific strengths and weaknesses and has at influence such a large number of GARCH models. To sum up the reviewed papers, many scholars suggest that the GARCH family model provides better results combined with another statistical technique. Based on the study, much of the research showed that with symmetric information, GARCH (1, 1) could precisely explain the volatilities and returns of the data and when under conditions of asymmetric information, the asymmetric GARCH models would be more appropriate [ 7 , 32 , 40 , 47 , 48 ]. Additionally, few researchers have used multivariate GARCH model statistical techniques for analyzing market volatility and returns to show that a more accurate and better results can be found by multivariate GARCH family models. Asymmetric GARCH models, for instance and like, EGARCH, GJR GARCH, and TGARCH, etc. have been introduced to capture the effect of bad news on the change in volatility of stock returns [ 42 , 58 , 62 ]. This study, although short and particular, attempted to give the scholar a concept of different methods found in this systematic literature review.
With respect to assessing scholars’ articles, the finding was that rankings and specifically only one GARCH model was sensitive to the different stock market volatilities and returns analysis, because the stock market does not have similar characteristics. For this reason, the stock market and model choice are little bit difficult and display little sensitivity to the ranking criterion and estimation methodology, additionally applying software is also another matter. The key challenge for researchers is finding the characteristics in stock market summarization using different kinds of local stock market returns, volatility detection, world stock market volatility, returns, and other data. Additional challenges are modeled by differences of expression between different languages. From an investigation perception, it has been detected that different authors and researchers use special datasets for the valuation of their methods, which may put boundary assessments between research papers.
Whenever there is assurance that scholars build on high accuracy, it will be easier to recognize genuine research gaps instead of merely conducting the same research again and again, so as to progress better and create more appropriate hypotheses and research questions, and, consequently, to raise the standard of research for future generation. This study will be beneficial for researchers, scholars, stock exchanges, regulators, governments, investors, and other concerned parties. The current study also contributes to the scope of further research in the area of stock volatility and returns. The content analysis can be executed taking the literature of the last few decades. It determined that a lot of methodologies like GARCH models, Johansen models, VECM, Impulse response functions, and Granger causality tests are practiced broadly in examining stock market volatility and return analysis across countries as well as among sectors with in a country.
Author Contributions
R.B. and S.W. proposed the research framework together. R.B. collected the data, and wrote the document. S.W. provided important guidance and advice during the process of this research. All authors have read and agreed to the published version of the manuscript.
This research received no external funding.
Conflicts of Interest
The authors declare no conflict of interest.
Competitive pricing on online markets: a literature review
- Research Article
- Open access
- Published: 14 June 2022
- Volume 21 , pages 596–622, ( 2022 )
Cite this article
You have full access to this open access article
- Torsten J. Gerpott 1 &
- Jan Berends 1
29k Accesses
9 Citations
Explore all metrics
Past reviews of studies concerning competitive pricing strategies lack a unifying approach to interdisciplinarily structure research across economics, marketing management, and operations. This academic void is especially unfortunate for online markets as they show much higher competitive dynamics compared to their offline counterparts. We review 132 articles on competitive posted goods pricing on either e-tail markets or markets in general. Our main contributions are (1) to develop an interdisciplinary framework structuring scholarly work on competitive pricing models and (2) to analyze in how far research on offline markets applies to online retail markets.
Similar content being viewed by others
Pricing Strategies in the Electronic Marketplace
Can online retailers escape the law of one price.
Platform Competition: Market Structure and Pricing
Avoid common mistakes on your manuscript.
Introduction
Setting prices relative to competitors, i.e., competitive pricing, Footnote 1 is a classical marketing problem which has been studied extensively before the emergence of e-commerce (Talluri and van Ryzin 2004 ; Vives 2001 ). Although literature on online pricing has been reviewed in the past (Ratchford 2009 ), interrelations between pricing and competition were rarely considered systematically (Li et al. 2017 ). As less than 2% of high-impact journal articles address pricing issues (Toni et al. 2017 ), pricing strategies do not receive proper research attention according to their practical relevance. This research gap holds even more for competitive pricing. In the past, the monopolistic assumption that demand for homogeneous goods mostly depends on prices set by a single firm may have been a viable simplification since price comparisons were difficult. Today, consumer search costs Footnote 2 shrink as the prices of most goods can be compared on relatively transparent online markets. Therefore, demand is increasingly influenced by prices of competitors which therefore should not be ignored (Lin and Sibdari 2009 ).
In the early 1990s, few people anticipated that business-to-consumer (B2C) online goods retail markets Footnote 3 would develop from a dubious alternative to conventional “brick-and-mortar” retail stores to an omnipresent distribution channel for all kinds of products in less than two decades (Balasubramanian 1998 ; Boardman and McCormick 2018 ). In 2000, e-commerce accounted for a mere 1% of overall retail sales. In 2025, e-retail sales are projected to account for nearly 25% of global retail sales (Lebow 2019 ). Traditional offline channels are nowadays typically complemented by online technologies (Gao and Su 2018 ). With digitization of various societal sectors in general and the COVID-19 pandemic in particular, the shift toward online channels is unlikely to stop in the future. Besides direct online shops, two-thirds of e-commerce sales are sold through online marketplaces/platforms like Alibaba, Amazon or eBay (Young 2022 ). The marketplace operator acts as an intermediary (two-sided platform) who matches demand (online consumers) with supply (retailers). Whereas the retailer retains control over product assortment and prices, he has to pay a commission to the marketplace operator (Hagiu 2007 ). However, these intermediaries often act as sellers themselves, thereby posing direct competition to retailers who have to decide between direct or marketplace channels (Ryan et al. 2012 ).
Online consumer markets fundamentally differ from offline settings (Chintagunta et al. 2012 ; Lee and Tan 2003 ; Scarpi et al. 2014 ; Smith and Brynjolfsson 2001 ). Factors which make competition even more prevalent for online than for offline markets are summarized in Table 1 .
To date, a number of scholarly articles reviews various aspects of pricing under competition or online pricing (Boer 2015a ; Chen and Chen 2015 ; Cheng 2017 ; Kopalle et al. 2009 ; Ratchford 2009 ; Vives 2001 ). Vives ( 2001 ) provides an overview of the history of pricing theory and its evolution from the early work of Bertrand ( 1883 ) who studied a duopoly with unconstrained capacity and identical products to Dudey ( 1992 ) who set the foundation for today’s dynamic pricing Footnote 4 research with constrained capacities and a finite sales horizon. Ratchford ( 2009 ) reviews the influence of online markets on pricing strategies. Although he depicts factors shaping the competitive environment of online markets and compares online versus offline channels, he does not include competitive strategies specifically. This also holds for review papers on dynamic pricing which treat competition rather novercally (Boer 2015a ; Gönsch et al. 2009 ). With emphasis on mobility barriers, multimarket contact and mutual forbearance, Cheng ( 2017 ) studies competition mechanisms across strategic groups. Kopalle et al. ( 2009 ) discuss competitive effects in retail focusing on different aspects such as manufacturer interaction and cross-channel competition. To the best of our knowledge, Chen and Chen ( 2015 ) are the only scholars who review existing competitive pricing research by classifying model characteristics along product uniqueness (identical vs. differentiated), type of customer (myopic vs. strategic), pricing policy (contingent vs. preannounced) and number of competitors (duopoly vs. oligopoly). However, competition is only one of three pricing problems they analyze forcing them to reduce scope and depth and to exclude online peculiarities. In addition, significant competitive pricing contributions were published since 2015 (chapter 2.2). Overall, given the limitations of previous reviews of the pricing literature makes revisiting the current state of research a worthwhile undertaking.
Most often, competitive pricing literature uses simplifying assumptions limiting the applicability of presented models. The simplifications are required to circumvent challenges like the curse of dimensionality (Harsha et al. 2019 ; Kastius and Schlosser 2022 ; Li et al. 2017 ; Schlosser and Boissier 2018 ), endogeneity problems (Cebollada et al. 2019 ; Chu et al. 2008 ; Fisher et al. 2018 ; Villas-Boas and Winer 1999 ), uncertain information (Adida and Perakis 2010 ; e.g., Bertsimas and Perakis 2006 ; Chung et al. 2012 ; Ferreira et al. 2016 ; Keskin and Zeevi 2017 ; Shugan 2002 ) and simultaneity bias (Li et al. 2017 ). As a consequence, early work on pricing strategies with competition was restricted to theoretical discussions (Caplin and Nalebuff 1991 ; Mizuno 2003 ; Perloff and Salop 1985 ). This holds especially true in combination with other practical circumstances such as capacity constraints, time-varying demand or a finite selling horizon (Gallego and Hu 2014 ).
Armstrong and Green ( 2007 ) find empirical evidence that competitive pricing, especially for the sake of gaining market share, harms profitability. Similarly, some researchers cursorily ascribe competitor-based pricing as a sign of a poor management because it signals a lack of capabilities to set prices independently (Larson 2019 ). Revenue management researchers therefore often assume that monopoly pricing models implicitly capture the dynamic effects of competition. The so-called market response hypothesis is the key rationale to neglect the effects of competition altogether (Phillips 2021 ; Talluri and van Ryzin 2004 ). According to this reasoning, competition does not have to be considered as all relevant effects are already included in historical sales data. However, this intuitive argument can be easily rebutted as Simon ( 1979 ) already showed that price elasticities change over time. Furthermore, Cooper et al. ( 2015 ) study the validity of the market response hypothesis and conclude that this monopolistic view is rarely adequate. Monopolistic pricing models can only be applied to stable markets with little time-varying demand and little expected competitive reactions.
Detrimental outcomes of ignoring competition in pricing strategies are shown by Anufriev et al. ( 2013 ), Bischi et al. ( 2004 ), Isler and Imhof ( 2008 ), Schinkel et al. ( 2002 ), and Tuinstra (2004). The negative effects are even more harmful in fierce competitive settings such as situations with a high number of competitors or price sensitive customers (van de Geer et al. 2019 ). Empirical evidence on the influence of competition on pricing decisions is provided by Richards and Hamilton ( 2006 ) who find that retailers compete on price and variety for market share. Li et al. ( 2017 ) observe that competition-based variables explained 30.2% of hotel price variations in New York—compared to 22.3% attributed to demand-side variables. Similarly, Hinterhuber ( 2008 ) assesses competitor-based pricing as a dominant strategy from a practical perspective. Li et al. ( 2008 ) argue that because of its relevance, competition should be considered in operational revenue management and not be treated stepmotherly as an abstract strategic constraint.
Although striving to simplify pricing models is desirable, researchers should thus not simply ignore effects of competition on price setting in a non-monopolistic (online) world. Blindly pegging pricing strategies to competitors or undercutting competitors to gain market share may favor detrimental price wars and not profit-maximizing market structures. Nevertheless, no significant market player can operate isolated on online markets—decisions made always affect competing firms and consumer demand (Chiang et al. 2007 ). In such dynamic markets (chapter 3.3), competition must be considered with time-varying attributes (Schlosser et al. 2016 ).
Against this background, we suggest a conceptual framework to structure research covering competitive online pricing. It can serve scholars as a map to direct future research on the one hand and provide practicing managers with a guide to locate relevant pricing contributions on the other hand. Although the framework can be applied to a variety of markets with competitive dynamics, we concentrate our review on research covering B2C online goods retail markets. Thus, related research with a focus on auction pricing, multichannel peculiarities, behavioral pricing and multi-dimensional pricing approaches such as Everything-as-a-Service (XaaS) or bundle pricing is only assessed when findings are crucial to the competition-related discussion. In the remainder, we proceed as follows. The next chapter provides a descriptive overview of the competitive pricing literature for the subsequent discussion. Chapter 3 puts the identified literature into the perspective of online retail markets considering product and environmental characteristics. Section 4 concludes with practical implications and directions for future research.
Overview of competitive pricing research
Initially, properties of the reviewed literature are briefly summarized. Besides (a) the journal representation, (b) the historical development of online market considerations and (c) research domains, we classify research according to (d) the geographical and industry context as well as (e) research design and empirical foundation.
We identified relevant references through a semi-structured multi-pronged search strategy. Following Tranfield et al. ( 2003 ), we firstly screened the literature reviews mentioned in chapter 1 to obtain an overview of existing research streams. Second, we created a set of potentially relevant contributions by searching multiple keywords in the journal databases EBSCO, Scopus and Web of Science (c.f. Baloglu and Assante 1999 ). Footnote 5 Third, high-impact journals (see Appendix 1) in the academic fields economics, marketing management, and operations were screened. With focus on highly cited (> 10 citations in Scopus), recent (published later than 2000) research, we identified an initial sample of 996 unique papers. Fourth, we studied the abstract and skimmed the text of all papers for relevance to competitive online pricing, reducing our initial set to 174 papers. Fifth, we screened the references of the papers and identified literature cited which we not already included in our set. Sixth, especially for research areas with limited coverage in peer-reviewed journals, we uncovered gray literature through searches with Google Scholar. As a result, this study concentrates on papers published between 2000 and 2022 and only sparsely utilizes literature from the pre-internet era. The final sample of the papers with relevance to competitive B2C online pricing encompasses 132 entries. A complete list of the papers reviewed in great depth is provided in Appendix 2. 94% are peer-reviewed articles. Book chapters, conference papers and preprint/working papers each account for 2%.
Journal representation
Competitive pricing literature is widely dispersed over a broad range of journals as roughly half of the articles considered are from journals with less than three articles in our review. Notably, journals with a higher density of competitive pricing contributions are from the fields of operations, economics or are interdisciplinary. Table 2 reports the distribution of articles among the journals with the highest representation. In addition, it provides the considered articles subject to a content analysis in chapter 3.
Online pricing contributions over time
Between 1976 and the end of the second millennium, the number of papers on competitive pricing in an internet context is naturally limited (Fig. 1 ). Parallel to the dissemination of online use among residential households, interest of researchers in online pricing in a competitive environment started to take off. 71.8% of the papers published from 2015 to 2022 consider online settings specifically. The corresponding statistic from 2000 to 2005 amounts to 43.8%.
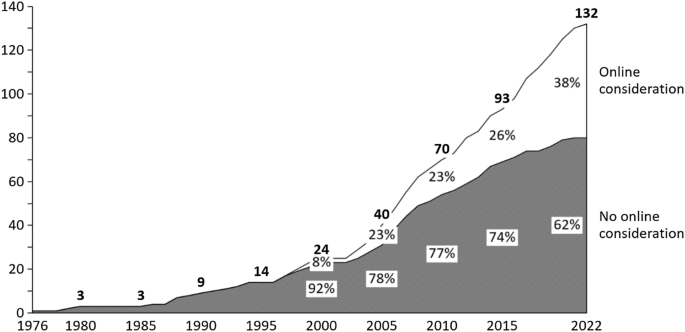
Competitive pricing literature and its consideration of online peculiarities accumulated by year
Development of research domains
Competitive pricing literature typically can be assigned to one of the following research domains:
The economics domain takes a market perspective across individual firms. It elaborates on the existence and uniqueness of competitive equilibria also including all subjects regarding econometrics.
The marketing management domain analyzes competitive pricing problems from the perspective of a single firm with a focus on customer reactions to pricing decisions. It includes all subjects linked to marketing, strategy, business, international, technology, innovation, and general management.
The operations domain considers quantitative pricing solutions for, among others, quantity planning, choice of distribution channels, and detection of algorithm driven price collusion. It includes all subjects regarding computer science, industrial and manufacturing engineering, and mathematics.
Separating the last 47 years of competitive pricing research into three intervals, all reviewed papers are assigned to their most affiliated research domain. Although the domains are similarly represented in our review (see Fig. 2 ), we see differences in their temporal change. Whereas rather theoretical economic subjects are covered relatively constant over time, more practice-oriented marketing management and operations subjects gained momentum since 2000. This suggests a shift from model conceptualization toward applicable research, frequently based on empirical data.
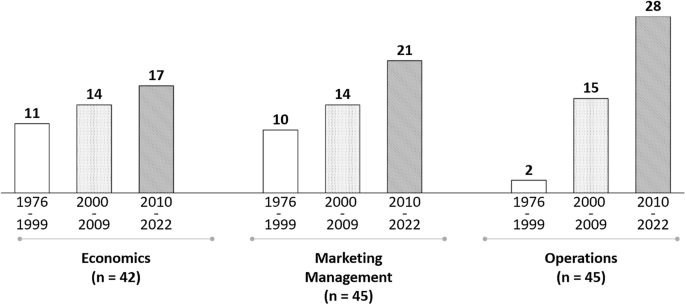
Distribution of competitive pricing literature over research domain and time interval
Geographical and industry context
As the origin of revenue management lies in transportation and hospitality optimization problems, one could expect that competitive pricing research also originates in these dynamic sectors. However, our analysis reveals a different picture: Almost half of the papers in our review do not concentrate on a specific industry. Besides, most industry-specific competitive pricing articles focus on retail, with 38% concentrating on the retail industry versus 8% and 4% on transportation and hospitality, respectively (see Fig. 3 ). This supports our proposition in chapter 1 that effects of competition on industry-specific pricing are particularly relevant for online markets.
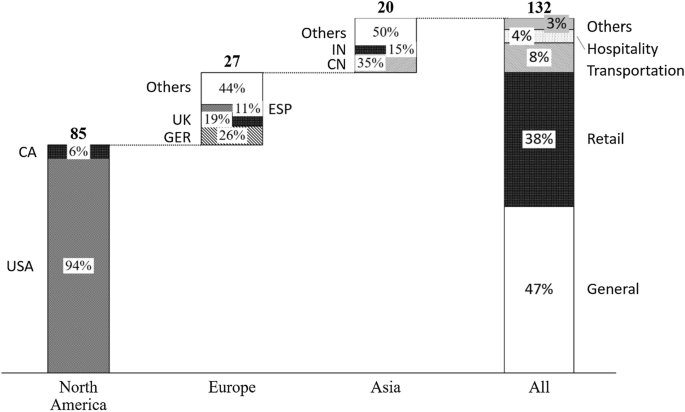
Competitive pricing research by focal industry and location of lead authors’ institution
Competitive pricing literature is predominantly driven by researchers employed by U.S. institutions (60%). The remaining 40% consist of Europe (19%), Asia (17%) and Canada (4%).
Research approach
A lack of empirical testing is an issue that hampers competitive pricing research. Liozu ( 2015 ) reported that only 15% of general pricing literature include empirical data. For competitive pricing, the situation appears even more aggravated. In addition to parameters such as price elasticities and stock levels of the company under study, comprehensive, real-time information of other market participants is crucial to add practical value.
For instance, to solve a simple Bertrand equilibrium, Footnote 6 full information of all competitors is needed, which is rarely available in real-life settings. Therefore, many problems covered in the literature are of a theoretical nature. In accordance with Liozu ( 2015 ), we find that only 18% of reviewed articles use empirical evidence to validate hypotheses. An additional 23% strive to ameliorate this shortage through simulation data and numerical examples. The remaining 59% fail to bring any empirical evidence or numerical examples.
As can be taken from Fig. 4 , missing empirical support is particularly prevalent for equilibrium models which use empirical data in only 7% of all papers.
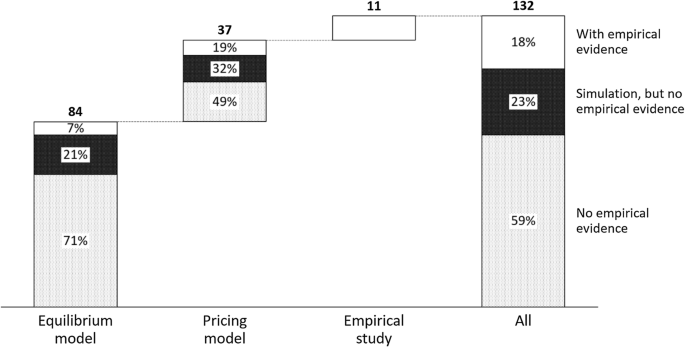
Competitive pricing research by research design and empirical validation
Competitive pricing on online markets
In this chapter, we assess the applicability of competitive pricing work to online markets. Typical characteristics of competitive B2C pricing models were derived from literature described in chapter 2. Competitive pricing literature can be classified along four characteristics depicted in Table 3 that form the market environment in which firms compete for consumer demand.
In the remainder of chapter 3, we discuss the four key questions in more depth and elaborate on their applicability to online retail markets.
Product similarity
In general, products in competitive pricing models are either identical (homogeneous) or differentiated by at least one quality parameter (heterogeneous). In case of homogeneous products, pricing is the only purchase decision variable—a perfectly competitive setting (Chen and Chen 2015 ). However, many firms strive to differentiate their products as this shifts the focus from the price as competitive lever to other product-related features (Afeche et al. 2011 ; Boyd and Bilegan 2003 ; Thomadsen 2007 ). According to Lancaster ( 1979 ), there are two types of product differentiation: vertical and horizontal differentiation. Vertical differentiation Footnote 7 encompasses all product distinctions which are objectively measurable and quantifiable regarding their quality level. Horizontal differentiation Footnote 8 can manifest in many variants and includes all product-related aspects which cannot be quantified according to their quality levels. Footnote 9 A key difference in the modeling of substitutable yet differentiated versus identical goods is that customers have heterogenous preferences among products. Footnote 10 A recent stream of literature approaches unknown differentiation criteria by assessing online consumer-generated content (DeSarbo and Grewal 2007 ; Lee and Bradlow 2011 ; Netzer et al. 2012 ; Ringel and Skiera 2016 ; Won et al. 2022 ).
Besides the chosen price level, Cachon and Harker ( 2002 ) argue that firms compete with the operational performance level offered and perceived, i.e., service level in online retail, to differentiate an otherwise homogenous offering. In situations, where resellers with comparable service and shipping policies offer similar products, price is a major decision variable for potential buyers (Yang et al. 2020 ). Often, e-tailers do not possess the right to exclusively distribute a certain product. For example, Samsung’s Galaxy S21 5G was offered by 69 resellers on the German price comparison website Idealo.de. Footnote 11 As some products in e-tail can be differentiated and others cannot, both identical and differentiated product research have their raison d’être for competitive online pricing.
Most competitive pricing models only address the effects of single-product settings. This simplification is reasonable if there is no interdependence between products of an e-tailer (Gönsch et al. 2009 ). Taking up on the smartphone example, the prices of close substitutes, such as Huawei’s P30 Pro, nonetheless have an impact on the demand of Samsung’s Galaxy S21 5G. To further extent product differentiation, price models have to incorporate multi-product pricing problems in non-cooperative settings (Chen and Chen 2015 ). Such models have to account not only for demand impact of directly competing products but also for synergies, cannibalization/substitution effects of (own) differentiated goods. Although there is a recent research stream regarding product assortment (Besbes and Sauré 2016 ; Federgruen and Hu 2015 ; Heese and Martínez-de-Albéniz 2018 ; Nip et al. 2020 ; Sun and Gilbert 2019 ), multi-product work is still underdeveloped. Thus, competitive multi-product pricing constitutes an area which should be addressed in future research.
Product durability
The durability of products is an important feature to differentiate between competitive pricing model types. Durable (non-perishable) products do not have an expiration date, for example consumer durables such as household appliances. Perishable products can only be sold for a limited time interval and have a finite sales horizon. After expiration date, unused capacity is lost or significantly devalued to a salvage value. Footnote 12 Combined with limited capacities, the firm objective is thus most often to maximize turnover under capacity constraints and finite sales horizon (Gallego and van Ryzin 1997 ; McGill and van Ryzin 1999 ; Weatherford and Bodily 1992 ).
Perishability can be of relevance for products with seasonality effects or short product life cycles (i.e., finite selling horizon) such as apparel, food groceries or winter sports equipment. This is especially relevant because online retailers of perishable products are severely restricted in their shipment, return handle policies and supply chain length (Cattani et al. 2007 ). Sellers cannot replenish their inventory after the planning phase and cannot retain goods for future sales periods (Perakis and Sood 2006 ). Some products like apparel—albeit reducing in value after a selling season—still have a certain salvage value and can be sold at reduced prices (Anand and Girotra 2007 ).
It depends on the type of product to decide whether perishability should be included in competitive pricing models. There is a fundamental distinction in the underlying optimization objective for models with or without perishability. Whereas models with perishable products tend to focus on revenue maximization over a definite short-term time horizon, models with durable products tend to focus on profit maximization over an indefinite or at least long-term time horizon by balancing current revenues of existing and future revenues of new customers. To account for this trade-off, models with durable products need to discount future cash flows incorporating time value of money, stock-keeping, opportunity and other costs related to prolonged sales (Farias et al. 2012 ). To conclude, perishability cannot be treated as an extension to durable models but rather as a separate class of pricing models. Depending on the product and/or setting in focus, both are relevant for online retailing. Further research could investigate the performance of models with and without consideration of perishability in various (online) settings to determine when it is appropriate to use which class of pricing models. Also, an interesting field of future studies arises around the question which instruments (e.g., service differentiations or price diffusion) are used by online retailers to differentiate otherwise homogeneous offerings.
Time dependence
A key differentiator of competitive pricing models is the consideration of either a static (time-independent) setup with definite equilibrium or a dynamic (time-dependent) constellation with changing environmental factors and equilibria. Albeit static pricing models have no time component, many consist of multiple stages to investigate the interplay of different factors. Footnote 13 In contrast, dynamic models allow for varying competitive (re-)actions over time. Footnote 14 Within the latter category, there are models with a finite (Afeche et al. 2011 ; Levin et al. 2008 ; Liu and Zhang 2013 ; Yang and Xia 2013 ) and an infinite (Anderson and Kumar 2007 ; Li et al. 2017 ; Schlosser and Richly 2019 ; Villas-Boas and Winer 1999 ; Weintraub et al. 2008 ) time horizon.
Historically, competitive pricing models assumed fixed prices over the considered time horizon. Limited computational power made it impossible to appropriately estimate models dynamically due to dimensionality issues (Schlosser and Boissier 2018 ). A lack of reliable demand information, high menu and investment costs to implement dynamic approaches were additional reasons why pricing models remained inherently static without incorporating changing competitive responses (Ferreira et al. 2016 ). The focus in retail has conventionally rather been on long-term profit optimization and to a lesser degree on dynamically changing price optimizations (Elmaghraby and Keskinocak 2003 ).
The literature disagrees on whether firms should opt for static or dynamic pricing strategies. A static environment allows to simplify and concentrate on a specific topic such as equilibrium discussions. For instance, Lal and Rao ( 1997 ) study success factors of everyday low pricing and derive conditions for a perfect Nash equilibrium between an everyday low price retailer and a retailer with promotional pricing. With Zara as an example for a company with a successful static pricing strategy, Liu and Zhang ( 2013 ) argue that with the presence of strategic customers who prolong sales in anticipation of price decreases, firms might even be better off to deploy static over dynamic price setting processes. Studying the time-variant pricing plans in electricity markets, Schlereth et al. ( 2018 ) suggest that consumers might prefer static over dynamic pricing because of factors like choice confusion, lack of trust in price fairness, perceived economical risk or perceived additional effort. Further support for a static pricing strategy is found in Cachon and Feldman ( 2010 ) and Hall et al. ( 2009 ).
Nevertheless, to generalize that static should strictly be preferred over dynamic pricing models could be short-sighted. Firms cannot generally infer future behavior of competitors from past observations to assess how competitive (re-) actions may influence the optimal pricing policy (Boer 2015b ). Corresponding to the surge of revenue management systems in the airline industry during the 70s and 80s, increased price and demand transparency, low menu costs and an abundance of decision support software created fierce competition among online retailers (Fisher et al. 2018 ). Taking up on the above mentioned example by Liu and Zhang ( 2013 ), Caro and Gallien ( 2012 ) show that even Zara does not solely rely on static pricing. They supported Zara’s pricing team in designing and implementing a dynamic clearance pricing optimization system—to generate a competitive advantage in addition to the fast-fashion retail model Zara mainly pursues (Caro 2012 ). Zhang et al. ( 2017 ) discuss various duopoly pricing models with static and dynamic pricing under advertising. They find that market surplus is highest when one firm prices dynamically, profiting from the static behavior of the other. Chung et al. ( 2012 ) provide numerical evidence that a dynamic pricing model with an appropriately specified demand estimation always outperforms static pricing strategies—also in settings with incomplete information. Xu and Hopp ( 2006 ) show that dynamic pricing outperforms preannounced pricing, especially with effective inventory management and elastic demand. Further support for advantages of dynamic pricing can be found by Popescu ( 2015 ), Wang and Sun ( 2019 ), and Zhang et al. ( 2018b ). Empirical evidence of the negative consequences of sticking to a static strategy in a changing environment is found in the cases of Nokia, Kodak, and Xerox.
While some scholars distinguish between discrete and continuous dynamic pricing systems (Vinod 2020 ), we suggest to classify dynamic pricing models according to their level of sophistication into two evolutionary stages: the (in e-commerce widely applied) manual rule-based pricing approach and the data-driven algorithmic optimization approach (Popescu 2015 ; Le Chen et al. 2016 ). Footnote 15 For the rule-based approach, “if-then-else rules” are defined and updated manually. Footnote 16 However, the mere number of stock-keeping units (SKUs) in today’s retailer offerings aggravate the initial setup and handling of rule-based pricing and make real-time adjustments unmanageable (Schlosser and Boissier 2018 ). In addition, rule-based approaches are rather subjective than sufficiently data-driven. Faced with a large range of SKUs, competitor responses and heterogeneous demand elasticities, canceling out the human decision-making process on an operational level is the next evolutionary step for competitive pricing systems (Calvano et al. 2020 ). Data-driven algorithmic pricing strategies use observable market Footnote 17 data to predict sales probabilities based on consumer demand and competitive responses (Schlosser and Richly 2019 ).
As online marketplaces benefit from an increased number of retailers on their platforms, they typically support sellers to establish automated dynamic pricing systems (Kachani et al. 2010 ). Footnote 18 However, Schlosser and Richly ( 2019 ) claim that current dynamic pricing systems are not able to deal with the complexity of competitor-based pricing and therefore most often ignore competition altogether or solely rely on manually adjusted rule-based mechanics. Challenges include the indefinite spectrum of changing competitor strategies, asymmetric access to competitor knowledge, a large solution space under limited information and the black-box character of dynamic systems, which exacerbates an intervention in case of a pricing system malfunction. Besides, researchers did not yet identify an algorithm which consistently outperforms other methodologies in competitive situations. Instead, it depends on the specific setting and other competitors’ pricing behavior to assess which pricing algorithm is optimal (van de Geer et al. 2019 ) exacerbating the application of such systems.
Reflecting the literature findings for both static and dynamic pricing strategies, we conclude that pricing managers should develop dynamic pricing models in most e-commerce situations. As long as demand and competitor price responses vary over time on online markets, dynamic models are naturally superior to time-independent approaches. Static models on the other hand are only appropriate in market constellations with little time-varying demand and competitor behavior. As static research can be expected to remain a vivid field of literature, further research with regard to the transferability of static models to dynamic settings is desirable. In addition, more research is needed that helps to better understand the implications of widely applied rule-based dynamic pricing methods and their transition toward algorithmic approaches (Boer 2015a ; van de Geer et al. 2019 ; Kastius and Schlosser 2022 ; Könönen 2006 ).
Market structure
The market structure describes the number of competing firms such as duopoly or oligopoly in a demand setting with an indefinite number of consumers. 60% of the reviewed papers studied duopolies, 49% oligopolies, 7% monopolistic competition, and 3% perfect competition. Footnote 19
Especially for research in the economics stream, many papers assume a perfectly competitive market. Pricing research with perfectly competitive markets (e.g., van Mieghem and Dada 1999 or Yang and Xia 2013 ) is likely to be of very limited value to online retailers. Building on the notion of Diamond ( 1971 ), Salop ( 1976 ) argues that if customers have positive information gathering costs, no perfect competition can occur as firms have room to slightly increase prices without losing demand. Christen ( 2005 ) found evidence that even with strong competition and low information costs, cost uncertainty could decrease the detrimental effect of competition for sellers and could increase prices above Bertrand levels. Similarly, Bryant ( 1980 ) showed that perfect competition is not possible in a market with uncertain demand, even if the number of firms is large and customers have no search costs. Rather, price dispersion reflects uncertain demand (Borenstein and Rose Nancy L. 1994 ; Cavallo 2018 ; Clemons et al. 2002 ; Obermeyer et al. 2013 ; Wang et al. 2021 ). Israeli et al. ( 2022 ) empirically show that the market power of individual firms does not only depend on the number and intensity of competitors but also on the firm’s ability to adjust prices in response to varying inventory levels of product substitutes, especially with low consumer search costs. This is of relevance for e-commerce as e-tailers could exploit this dependence by incorporating competitors’ stock levels into pricing decisions (Fisher et al. 2018 ).
Some papers discuss (quasi) monopolistic competition (e.g., Xu and Hopp 2006 ) in which small firms charge the (higher) monopoly price rather than the (lower) competitive price. From an empirical study in the U.S. airline industry, Chen (2018) concludes that, as firms can price discriminate late-arriving consumers, competition is softened, profits are increased, and the only single-price equilibrium could be at the monopoly price. This supports Lal and Sarvary ( 1999 ) who show that online retailers enjoy a certain amount of monopoly power in cases where buyers cannot switch suppliers for repeated purchases (e.g., technical incompatibility reasons). In such cases, switching costs could increase online prices (Chen and Riordan 2008 ). However, this contradicts Deck and Gu ( 2012 ) who empirically show that, although the distribution of buyer values of competing products might theoretically lead to higher prices through competition, intensity of competition rarely allows for an occurrence of this phenomenon in e-tail settings.
Although duopoly settings can serve to assess the relevant strength of pricing strategies, which is not directly possible for oligopoly markets due to the curse of dimensionality (Kastius and Schlosser 2022 ), they cannot be transferred to more competitive environments (van de Geer et al. 2019 ). In online retail, a duopoly market structure is a rare exemption. Like for perfectly competitive markets, findings of duopoly research must be carefully assessed in terms of their applicability to online retail oligopolies.
Bresnahan and Reiss ( 1991 ) found empirical evidence that markets with an increasing number of dealers have lower prices than in less competitive market structures such as monopolies or duopolies. Although applicable to many online retail markets, where retailers face dozens, if not hundreds of thousands of competitors (Schlosser and Boissier 2018 ), few research attention is currently given toward a structure with a large number of competitors in an imperfect market (cf. Li et al. 2017 ). A way to assess the current competitive structure of markets is the utilization of online consumer-generated content such as forum entries (Netzer et al. 2012 ; Won et al. 2022 ) or clickstream data (Ringel and Skiera 2016 ) and actual sales data (Kim et al. 2011 ).
In many countries with well-developed B2C online markets, one or few major retailers dominate on an oligopolistic market. For example, the top three online retailers in the United States accounted for over 50% of the revenue generated on the national e-commerce market in 2021. Footnote 20 Due to lower locational limitations in conjunction with substantial economies of scale and scope, online markets tend to become more concentrated than their offline counterparts (Borsenberger 2015 ). Although one could expect that increased market transparency leads to a higher intensity of competition (Cao and Gruca 2003 ), limiting the market power of established firms and leaving growth potential for smaller firms (Zhao et al. 2017 ), it appears reasonable to predict that most online markets will ultimately resemble an oligopoly setting with a with a relatively small number of players—enabling increased tacit pricing algorithm collusion in the future (Calvano et al. 2020 ). With few exceptions (e.g., Noel 2007 ), there is little research (Brown and Goolsbee 2002 ; Wang et al. 2021 ; Cavallo 2018 ) exploring what type of competitor-based pricing strategies are used and what competitive dynamics are found on e-tail markets. Thus, more research is needed to investigate the current state of market structure and intensity of competition in today’s e-commerce markets as drivers of the selection and the outcomes of pricing approaches.
Implications and directions
We contribute to the literature by providing an interdisciplinary review of competitive online retail research. Competitive pricing problems can most often be assigned to one of the academic fields of economics, marketing management or operations. In a first step, this review offered a descriptive portrayal of the relevant literature. Motivated by practical issues and common features in competitive pricing research, we then structured competitive pricing contributions along four properties of pricing models. First, do firms compete with identical or quality differentiated products? Second, are products to be considered as perishable or durable goods? Third, is the market setting to be regarded time-independent or not? Fourth, which market structure prevails on e-tail markets? The framework is derived from an analysis of pricing research not exclusively restricted to online retail settings. Therefore, it could be extended to other online or offline markets, with little loss of generalizability.
We focused on e-tail markets because the relevance of competition for pricing strategies is disproportionally higher in such environments. On e-tail online markets, products are rarely offered exclusively so that the likelihood of substitutive competition is high. Nevertheless, products can be differentiated through other factors than prices such as generous shipping, customer retention (e.g., loyalty reward programs) or return and issue handling policies. With a look on product similarities, accounting for product interdependencies and multi-product situations are important improvements of prevailing pricing models. Second, pricing models with both a focus on perishable and/or durable products are relevant on e-tail markets. However, further research is needed exploring which of the respective perishability considerations are appropriate for different settings. Third, we conclude that, albeit time-independent static models may occasionally serve to simplify pricing issues, dynamic models outperform their static counterparts in constantly changing market environments such as in e-commerce. Fourth, we show that in most practical settings, online markets resemble either an oligopolistic market structure or a structure with many firms under imperfect competition. Thus, future research should consider these two “real” competitive settings instead of further looking at simplifying market structures such as monopolistic or duopolistic competition. This should ease a transfer of theoretical insights into practical applications. To sum, firms should be able to improve their competitive position by developing a profit optimizing dynamic pricing strategy for identical products in an oligopolistic setting with a varying number and relevance of competitors.
Due to space limitations, we had to focus on competitive pricing model characteristics related to four overall product and market attributes. Thus, more work is needed on other characteristics of competitive pricing models, particularly firm- and consumer-related characteristics. Firm-related characteristics encompass various additional properties of interacting firms (e.g., similarity or capacity constraints). Similarly, consumer-related characteristics entail further properties of interacting buyers (e.g., certainty, discreteness, sophistication, and homogeneity of demand).
In the selection process of literature, this study only considered papers in peer-reviewed journals and conference proceedings in English. Subsequent research could complement our findings by including industry-funded, unpublished and non-peer-reviewed articles, also in other languages. In addition, we do not claim that our research captures all competitive pricing publications of the considered field. As our study spans almost 50 years of a frequently discussed topic in the domains of economics, marketing management, and operations, we had to constrain the scope to the most influential work. Although we mutually evaluated our selection decisions and consulted outside peers for validation and further input, we cannot eliminate the element of subjectivity. Consequently, other authors could have selected slightly different papers. However, this shortcoming is unlikely to significantly affect our results as our literature selection was derived from a broad array of competitive pricing research and would therefore be only marginally influenced by a few omitted articles.
Competitive pricing includes all activities and processes to price products with the consideration of competitors. This does not only include rigidly pegging prices to competitor prices but rather a comprehensive consideration of current and expected price (re-)actions of competing firms to sustainably ensure profit maximization. In this article, the terms competitive pricing, competitor-oriented pricing and competitor-based pricing are used synonymously.
Search costs are defined as the costs of time and resources to acquire information with respect to price, assortment, and quality characteristics of the goods provided by different sellers. The internet dramatically reduces search costs through price comparison websites such as Google Shopping, Shopzilla (USA) or Idealo (Germany).
Business-to-consumer (B2C) online retail sales encompass all forms of electronic commerce markets in which residential end customers can directly buy goods from a seller over the internet through a web browser or a mobile app. In this paper, the terms business-to-consumer (B2C) online goods retail, online retail, e-tail, e-retail and e-commerce markets are used synonymously.
In contrast to classical quantity-based revenue management, dynamic pricing, also known as surge pricing, is the practice of adjusting prices according to current market demand (Boer 2015a ). Revenue management, also known as yield management, is a type of price discrimination which originates from the airline and hospitality industries. Typically, revenue management models assume fixed capacities, low marginal cost, varying demand and highly perishable inventory (Talluri and van Ryzin 2004 ).
Keywords used for abstract, title and keyword screening were “competitive pricing”, “competitor-based pricing”, “competition” AND “pricing”. To find literature for online pricing in particular, the search was combined with the keywords “online”, “e-retail”, “ecommerce” and “e-commerce”. Whereas the combination was scanned in great depths, the three competitive keywords were screened for influential papers with implications for online markets.
Bertrand competition is a simplified model of competition to explain price competition among (at least) two firms for an identical product at equal unit cost of production. Prices are set simultaneously, and consumers buy without search costs from the firm with the lowest price. When all firms charge the same price, consumer demand is split evenly between firms. A firm is willing to supply unlimited amounts of quantities above the unit cost of production and is indifferent to supply at unit cost as it will earn zero profit. The only Bertrand equilibrium exists when prices are equal to unit cost (i.e., competitive price) as each firm otherwise would have an incentive to undercut all other competitors and thereby rake in the entire market demand. Therefore, there can be no equilibrium at prices above the competitive price and price dispersion cannot occur.
In vertical differentiation, consumer choice depends on specific quality levels of product attributes. At the same price, all consumers prefer one product over other products, for example because of superior design. In the simplest form, products differ in one attribute and customers are willing to pay marginal increments of this attribute.
In horizontal differentiation, consumer choice depends on preferences for products. At the same price, some customers would buy one product and others other products.
We consider product differentiation only to product-related differentiation attributes. However, in competitive pricing literature firm-related differences such as firm loyalty or distribution channels are occasionally attributed to differentiation. For example, Abhishek et al. ( 2016 ) differentiate online distribution channels of otherwise homogeneous products and firms.
Heterogeneous customer preferences are a key requirement for product differentiation, otherwise price constitutes the only driver of the buying decision (Li et al. 2017 ). Without heterogeneity in consumers’ marginal willingness to pay for different levels of product quality, there can be no product differentiation (Pigou 1920 ).
Accessed 14–03-2022.
Salvage value is defined as the residual cash-flow of a good after its expiration date.
For example, game settings on the foundation of Stackelberg games necessarily comprise ≥ 2 stages (Geng and Mallik 2007 ; Gupta et al.; Wang et al. 2020 ; Yao and Liu 2005 ). Another example would be Anand and Girotra ( 2007 ) who propose a 3-stage model in which they include the supply chain configuration and determination of production quantities in addition to the actual price setting.
As such, we classify n-stage models as dynamic models when not all individual stages serve a specific time-independent purpose.
For instance, the Brandenburg consumer advice center (Verbraucherzentrale Brandenburg) examined dynamic price differentiation in online retail and found that 15 of the 16 observed German online shops dynamically changed their prices in 2018 (Dautzenberg et al. 2018 ).
A typical rule would be to set prices always x% lower than competitor prices up to a certain profit threshold.
Observable market data include price and stock levels of competitors (Fisher et al. 2018 ) or clickstream and keyword data of customers (Li et al. 2017 ).
Examples for support programs by online marketplaces are Amazon’s Seller Central ( https://sellercentral.amazon.com/gp/help/external/G201994820?language=en_US&ref=efph_G201994820_cont_43381 ; Accessed 14–03-2022), eBay’s Seller Tools ( https://pages.ebay.com/sell/automation.html ; Accessed 14–03-2022) or Idealo’s Partner Program ( https://partner.idealo.com/de ; Accessed 14–03-2022).
Cumulatively, these values exceed 100% as some articles discussed more than one kind of market structure. Applied by economists to simplify real markets as the foundation of price theory, perfect competition relates to a market structure which is controlled entirely by market forces and not by individual firms. Instead, individual firms only act as price takers and cannot earn any economic profit. The conditions for a perfect competition, such as full information, homogeneous products, fully rational buyers, no scale, network or externality effects, no entry barriers, and no transaction costs, are rarely attainable in practical settings (Stigler 1957 ). If not all conditions for perfect competition are fulfilled, the market structure is imperfect which applies to most practical settings. Besides a monopoly with only one seller on the market, three market structures with competing firms exist: Monopolistic, duopolistic, and oligopolistic competition. An oligopoly is characterized by a small number of firms in which the behavior of one firm drives the actions of other firms. A duopoly is a particular case of an oligopoly in which two firms control the market. An extreme case of imperfect competition is (quasi) monopolistic competition in which products are differentiated and firms maintain a certain spare capacity giving them a certain degree of pricing power to maximize their (short-term) profits. In consequence, prices can be higher than corresponding the competitive (Bertrand) price (Vives 2001 ).
For an overview of the top 15 online shops in the United States in 2021, see Davidkhanian ( 2021 ).
Abhishek, Vibhanshu, Kinshuk Jerath, and Z. John Zhang. 2016. Agency selling or reselling? Channel structures in electronic retailing. Management Science 62 (8): 2259–2280. https://doi.org/10.1287/mnsc.2015.2230 .
Article Google Scholar
Adida, Elodie, and Georgia Perakis. 2010. Dynamic pricing and inventory control: Uncertainty and competition. Operations Research 58 (2): 289–302. https://doi.org/10.1287/opre.1090.0718?journalCode=opre .
Afeche, Philipp, Hu Ming, and Yang Li. 2011. Reorder flexibility and price competition for differentiated seasonal products with market size uncertainty. SSRN Electronic Journal . https://doi.org/10.2139/ssrn.1976886 .
Aggarwal, Praveen, and Taihoon Cha. 1998. Asymmetric price competition and store vs. national brand choice. Journal of Product & Brand Management 7 (3): 244–253. https://doi.org/10.1108/10610429810222877 .
Aksoy-Pierson, Margaret, Gad Allon, and Awi Federgruen. 2013. Price competition under mixed multinomial logit demand functions. Management Science 59 (8): 1817–1835. https://doi.org/10.1287/mnsc.1120.1664 .
Allen, Beth, and Martin Hellwig. 1986. Bertrand-Edgeworth oligopoly in large markets. Review of Economic Studies 53 (2): 175–204. https://doi.org/10.2307/2297646 .
Anand, Krishnan S., and Karan Girotra. 2007. The strategic perils of delayed differentiation. Management Science 53 (5): 697–712. https://doi.org/10.1287/mnsc.1060.0655 .
Anderson, Eric T., and Nanda Kumar. 2007. Price competition with repeat, loyal buyers. Quantitative Marketing and Economics 5 (4): 333–359. https://doi.org/10.1007/s11129-007-9023-7 .
Anderson, Chris K., Henning Rasmussen, and Leo MacDonald. 2005. Competitive pricing with dynamic asymmetric price effects. International Transactions in Operational Research 12 (5): 509–525. https://doi.org/10.1111/j.1475-3995.2005.00522.x .
Anton, James J., Gary Biglaiser, and Nikolaos Vettas. 2014. Dynamic price competition with capacity constraints and a strategic buyer. International Economic Review 55 (3): 943–958. https://doi.org/10.1111/iere.12077 .
Anufriev, Mikhail, Dávid Kopányi, and Jan Tuinstra. 2013. Learning cycles in bertrand competition with differentiated commodities and competing learning rules. Journal of Economic Dynamics and Control 37 (12): 2562–2581. https://doi.org/10.1016/j.jedc.2013.06.010 .
Armstrong, J. Scott, and Kesten C. Green. 2007. Competitor-oriented objectives: Myth of market share. International Journal of Business 12 (1): 117–136.
Google Scholar
Ba, Sulin, Jan Stallaert, and Zhongju Zhang. 2012. Research note—Online price dispersion: A game-theoretic perspective and empirical evidence. Information Systems Research 23 (2): 575–592. https://doi.org/10.1287/isre.1110.0353 .
Babaioff, Moshe, Renato Paes Leme, and Balasubramanian Sivan. 2015. Price competition, fluctuations, and welfare guarantees. In EC '15: Proceedings of the Sixteenth ACM Conference on Economics and Computation, Portland, USA. 15-Jun-15 - 19-Jun-15. https://doi.org/10.48550/arXiv.1411.2036
Balakrishnan, Anantaram, Shankar Sundaresan, and Bo. Zhang. 2014. Browse-and-switch: Retail-online competition under value uncertainty. Production and Operations Management 23 (7): 1129–1145. https://doi.org/10.1111/poms.12165 .
Balasubramanian, Sridhar. 1998. Mail versus mall: A strategic analysis of competition between direct marketers and conventional retailers. Marketing Science 17 (3): 181–195. https://doi.org/10.1287/mksc.17.3.181 .
Baloglu, Seyhmus, and Lisa Marie Assante. 1999. A content analysis of subject areas and research methods used in five hospitality management journals. Journal of Hospitality & Tourism Research 23 (1): 53–70. https://doi.org/10.1177/109634809902300105 .
Bernstein, Fernando, and Awi Federgruen. 2004. A general equilibrium model for industries with price and service competition. Operations Research 52 (6): 868–886. https://doi.org/10.1287/opre.1040.0149 .
Bernstein, Fernando, and Awi Federgruen. 2005. Decentralized supply chains with competing retailers under demand uncertainty. Management Science 51 (1): 18–29. https://doi.org/10.1287/mnsc.1040.0218 .
Bernstein, Fernando, Jing-Sheng Song, and Xiaona Zheng. 2008. “Bricks-and-mortar” vs. “clicks-and-mortar”: An equilibrium analysis. European Journal of Operational Research 187 (3): 671–690. https://doi.org/10.1016/j.ejor.2006.04.047 .
Bertrand, Joseph Louis François. 1883. Thèorie mathèmatique de la richesse sociale. Journal des Savants 499–508.
Bertsimas, Dimitris, and Georgia Perakis. 2006. Dynamic pricing: A learning approach. In Mathematical and computational models for congestion charging , ed. Siriphong Lawphongpanich, Donald W. Hearn, and Michael J. Smith, 45–79. Boston: Kluwer Academic Publishers.
Chapter Google Scholar
Besbes, Omar, and Denis Sauré. 2016. Product assortment and price competition under multinomial logit demand. Production and Operations Management 25 (1): 114–127. https://doi.org/10.1111/poms.12402 .
Bischi, Gian-Italo, Carl Chiarella, and Michael Kopel. 2004. The long run outcomes and global dynamics of a duopoly game with misspecified demand functions. International Game Theory Review 6 (3): 343–379. https://doi.org/10.1142/S0219198904000253 .
Blattberg, Robert C., and Kenneth J. Wisniewski. 1989. Price-induced patterns of competition. Marketing Science 8 (4): 291–309. https://doi.org/10.1287/mksc.8.4.291 .
Boardman, Rosy, and Helen McCormick. 2018. Shopping channel preference and usage motivations. Journal of Fashion Marketing and Management: An International Journal 22 (2): 270–284. https://doi.org/10.1108/JFMM-04-2017-0036 .
Boccard, Nicolas, and Xavier Wauthy. 2000. Bertrand competition and Cournot outcomes: Further results. Economics Letters 68 (3): 279–285. https://doi.org/10.1016/S0165-1765(00)00256-1 .
Borenstein, Severin, and L. Rose Nancy. 1994. Competition and price dispersion in the U.S. airline industry. Journal of Political Economy 102 (4): 653–683. https://doi.org/10.1086/261950 .
Borsenberger, Claire. 2015. The concentration phenomenon in e-commerce. In Postal and delivery innovation in the digital economy , ed. Michael A. Crew and Timothy J. Brennan, 31–41. Cham: Springer International Publishing.
Boyd, E. Andrew, and Ioana C. Bilegan. 2003. Revenue management and e-commerce. Management Science 49 (10): 1363–1386. https://doi.org/10.1287/mnsc.49.10.1363.17316 .
Bresnahan, Timothy F., and Peter C. Reiss. 1991. Entry and competition in concentrated markets. Journal of Political Economy 99 (5): 977–1009. https://doi.org/10.1086/261786 .
Brown, Jeffrey R., and Austan Goolsbee. 2002. Does the internet make markets more competitive? Evidence from the life insurance industry. Journal of Political Economy 110 (3): 481–507. https://doi.org/10.1086/339714 .
Bryant, John. 1980. Competitive equilibrium with price setting firms and stochastic demand. International Economic Review 21 (3): 619–626. https://doi.org/10.2307/2526357 .
Brynjolfsson, Erik, and Michael D. Smith. 2000. Frictionless commerce? A comparison of internet and conventional retailers. Management Science 46 (4): 563–585. https://doi.org/10.1287/mnsc.46.4.563.12061 .
Cachon, Gérard P., and Pnina Feldman. 2010. Dynamic versus static pricing in the presence of strategic consumers. The Wharton School, University of Pennsylvania (Working Paper).
Cachon, Gérard. P., and Patrick T. Harker. 2002. Competition and outsourcing with scale economies. Management Science 48 (10): 1314–1333. https://doi.org/10.1287/mnsc.48.10.1314.271 .
Caillaud, Bernard, and Romain de Nijs. 2014. Strategic loyalty reward in dynamic price discrimination. Marketing Science 33 (5): 725–742. https://doi.org/10.1287/mksc.2013.0840 .
Calvano, Emilio, Giacomo Calzolari, Vincenzo Denicolò, and Sergio Pastorello. 2020. Artificial intelligence, algorithmic pricing, and collusion. American Economic Review 110 (10): 3267–3297. https://doi.org/10.1257/aer.20190623 .
Campbell, Colin, Gautam Ray, and Waleed A. Muhanna. 2005. Search and collusion in electronic markets. Management Science 51 (3): 497–507. https://doi.org/10.1287/mnsc.1040.0327 .
Cao, Yong, and Thomas S. Gruca. 2003. The effect of stock market dynamics on internet price competition. Journal of Service Research 6 (1): 24–36. https://doi.org/10.1177/1094670503254272 .
Caplin, Andrew, and Barry Nalebuff. 1991. Aggregation and imperfect competition: On the existence of equilibrium. Econometrica 59 (1): 25–59. https://doi.org/10.2307/2938239 .
Caro, Felipe. 2012. Zara: Staying fast and fresh. The European Case Clearing House. ECCH Case study 612-006-1.
Caro, Felipe, and Jérémie. Gallien. 2012. Clearance pricing optimization for a fast-fashion retailer. Operations Research 60 (6): 1404–1422. https://doi.org/10.2139/ssrn.1731402 .
Cattani, Kyle, Olga Perdikaki, and Ann Marucheck. 2007. The perishability of online grocers. Decision Sciences 38 (2): 329–355. https://doi.org/10.1111/j.1540-5915.2007.00161.x .
Cavallo, Alberto. 2018. More Amazon effects: online competition and pricing behaviors. National Bureau of Economic Research (Working Papers 25138).
Cebollada, Javier, Yanlai Chu, and Zhiying Jiang. 2019. Online category pricing at a multichannel grocery retailer. Journal of Interactive Marketing 46 (1): 52–69. https://doi.org/10.1016/j.intmar.2018.12.004 .
Chen, Yongmin. 1997. Paying customers to switch. Journal of Economics Management Strategy 6 (4): 877–897. https://doi.org/10.1111/j.1430-9134.1997.00877.x .
Chen, Ming, and Zhi-Long. Chen. 2015. Recent developments in dynamic pricing research: Multiple products, competition, and limited demand information. Production and Operations Management 24 (5): 704–731. https://doi.org/10.1111/poms.12295 .
Chen, Yongmin, and Michael H. Riordan. 2008. Price-increasing competition. RAND Journal of Economics 39 (4): 1042–1058. https://doi.org/10.1111/j.1756-2171.2008.00049.x .
Chen, Ying-Ju, Yves Zenou, and Junjie Zhou. 2018. Competitive pricing strategies in social networks. RAND Journal of Economics 49 (3): 672–705. https://doi.org/10.1111/1756-2171.12249 .
Cheng, Kuangnen. 2017. Competitive dynamics across strategic groups: A literature review and validation by quantitative evidence of operation data. International Journal of Business Environment 9 (4): 301–323. https://doi.org/10.1504/IJBE.2017.092223 .
Chiang, Wen Chyuan, Jason C.H. Chen, and Xu Xiaojing. 2007. An overview of research on revenue management: Current issues and future research. International Journal of Revenue Management 1 (1): 97–128. https://doi.org/10.1504/IJRM.2007.011196 .
Chintagunta, Pradeep K., Junhong Chu, and Javier Cebollada. 2012. Quantifying transaction costs in online/offline grocery channel choice. Marketing Science 31 (1): 96–114. https://doi.org/10.1287/mksc.1110.0678 .
Chioveanu, Ioana. 2012. Price and quality competition. Journal of Economics 107 (1): 23–44. https://doi.org/10.1007/s00712-011-0259-z .
Chioveanu, Ioana, and Jidong Zhou. 2013. Price competition with consumer confusion. Management Science 59 (11): 2450–2469. https://doi.org/10.1287/mnsc.2013.1716 .
Choi, S. Chan. 1996. Price competition in a duopoly common retailer channel. Journal of Retailing 72 (2): 117–134. https://doi.org/10.1016/S0022-4359(96)90010-X .
Christen, Markus. 2005. Research note: Cost uncertainty is bliss: The effect of competition on the acquisition of cost information for pricing new products. Management Science 51 (4): 668–676. https://doi.org/10.1287/mnsc.1040.0320 .
Chu, Junhong, Pradeep Chintagunta, and Javier Cebollada. 2008. Research note: A comparison of within-household price sensitivity across online and offline channels. Marketing Science 27 (2): 283–299. https://doi.org/10.1287/mksc.1070.0288 .
Chung, Byung Sun, Jiahan Li, Tao Yao, Changhyun Kwon, and Terry L. Friesz. 2012. Demand learning and dynamic pricing under competition in a state-space framework. IEEE Transactions on Engineering Management 59 (2): 240–249.
Clemons, Eric K., Il-Horn Hann, and Lorin M. Hitt. 2002. Price dispersion and differentiation in online travel: An empirical investigation. Management Science 48 (4): 534–549. https://doi.org/10.1287/mnsc.48.4.534 .
Cooper, William L., Tito Homem-de-Mello, and Anton J. Kleywegt. 2015. Learning and pricing with models that do not explicitly incorporate competition. Operations Research 63 (1): 86–103. https://doi.org/10.1287/opre.2014.1341 .
Currie, Christine S. M., Russell C. H. Cheng, and Honora K. Smith. 2008. Dynamic pricing of airline tickets with competition. Journal of the Operational Research Society 59 (8): 1026–1037. https://doi.org/10.1057/palgrave.jors.2602425 .
Dana, James D., and Nicholas C. Petruzzi. 2001. Note: The newsvendor model with endogenous demand. Management Science 47 (11): 1488–1497. https://doi.org/10.1287/mnsc.47.11.1488.10252 .
Dana, James D., and Kevin R. Williams. 2022. Intertemporal price discrimination in sequential quantity-price games. Marketing Science . https://doi.org/10.1287/mksc.2021.1345 .
Dasci, Abdullah, and Mustafa Karakul. 2009. Two-period dynamic versus fixed-ratio pricing in a capacity constrained duopoly. European Journal of Operational Research 197 (3): 945–968. https://doi.org/10.1016/j.ejor.2007.12.039 .
Dautzenberg, Kirsti, Constanze Gaßmann, and Britta Groß. 2018. Online-Handel: Das Spiel mit dem dynamischen Preis. https://www.verbraucherzentrale-brandenburg.de/sites/default/files/2018-08/marktwaechter-untersuchung-dynamische-preisdifferenzierung.pdf . Accessed 14 March 2022.
Davidkhanian, Suzy. 2021. US retail spending jumped nearly 16% this year despite inflation, supply chain woes. https://www.emarketer.com/content/us-retail-spending-jumped-this-year-despite-inflation-supply-chain-woes . Accessed 14 March 2022.
de Toni, Deonir, Gabriel Sperandio Milan, Evandro Busata Saciloto, and Fabiano Larentis. 2017. Pricing strategies and levels and their impact on corporate profitability. Revista de Administração 52 (2): 120–133. https://doi.org/10.1016/j.rausp.2016.12.004 .
Deck, Cary, and Gu Jingping. 2012. Price increasing competition? Experimental evidence. Journal of Economic Behavior & Organization 84 (3): 730–740. https://doi.org/10.1016/j.jebo.2012.09.015 .
den Boer, Arnoud V. 2015a. Dynamic pricing and learning: Historical origins, current research, and new directions. Surveys in Operations Research and Management Science 20 (1): 1–18. https://doi.org/10.1016/j.sorms.2015.03.001 .
den Boer, Arnoud V. 2015b. Tracking the market: Dynamic pricing and learning in a changing environment. European Journal of Operational Research 247 (3): 914–927.
DeSarbo, Wayne S., and Rajdeep Grewal. 2007. An alternative efficient representation of demand-based competitive asymmetry. Strategic Management Journal 28 (7): 755–766. https://doi.org/10.1002/smj.601 .
Devaraj, Sarv, Ming Fan, and Rajiv Kohli. 2002. Antecedents of B2C channel satisfaction and preference: Validating e-commerce metrics. Information Systems Research 13 (3): 316–333. https://doi.org/10.1287/isre.13.3.316.77 .
Diamond, Peter A. 1971. A model of price adjustment. Journal of Economic Theory 3 (2): 156–168. https://doi.org/10.1016/0022-0531(71)90013-5 .
Dickson, Peter R., and Joel E. Urbany. 1994. Retailer reactions to competitive price changes. Journal of Retailing 70 (1): 1–21. https://doi.org/10.1016/0022-4359(94)90025-6 .
Dinerstein, Michael, Liran Einav, Jonathan Levin, and Neel Sundaresan. 2018. Consumer price search and platform design in internet commerce. American Economic Review 108 (7): 1820–1859. https://doi.org/10.1257/aer.20171218 .
Dong, James, A. Serdar Simsek, and Huseyin Topaloglu. 2019. Pricing problems under the Markov chain choice model. Production and Operations Management 28 (1): 157–175. https://doi.org/10.1111/poms.12903 .
Dudey, Marc. 1992. Dynamic Edgeworth-Bertrand competition. Quarterly Journal of Economics 107 (4): 1461–1477. https://doi.org/10.2307/2118397 .
Dzyabura, Daria, Srikanth Jagabathula, and Eitan Muller. 2019. Accounting for discrepancies between online and offline product evaluations. Marketing Science 38 (1): 88–106. https://doi.org/10.1287/mksc.2018.1124 .
Elmaghraby, Wedad, and Pınar Keskinocak. 2003. Dynamic pricing in the presence of inventory considerations: Research overview, current practices, and future directions. Management Science 49 (10): 1287–1309. https://doi.org/10.1287/mnsc.49.10.1287.17315 .
Farias, Vivek, Denis Saure, and Gabriel Y. Weintraub. 2012. An approximate dynamic programming approach to solving dynamic oligopoly models. RAND Journal of Economics 43 (2): 253–282. https://doi.org/10.1111/j.1756-2171.2012.00165.x .
Fay, Scott. 2008. Selling an opaque product through an intermediary: The case of disguising one’s product. Journal of Retailing 84 (1): 59–75. https://doi.org/10.1016/j.jretai.2008.01.005 .
Federgruen, Awi, and Hu Ming. 2015. Multi-product price and assortment competition. Operations Research 63 (3): 572–584. https://doi.org/10.1287/opre.2015.1380 .
Ferreira, Kris Johnson, Bin Hong Alex. Lee, and David Simchi-Levi. 2016. Analytics for an online retailer: Demand forecasting and price optimization. Manufacturing Service Operations Management 18 (1): 69–88. https://doi.org/10.1287/msom.2015.0561 .
Fisher, Marshall, Santiago Gallino, and Jun Li. 2018. Competition-based dynamic pricing in online retailing: A methodology validated with field experiments. Management Science 64 (6): 2473–2972. https://doi.org/10.1287/mnsc.2017.2753 .
Frambach, Ruud T., Henk C.A. Roest, and Trichy V. Krishnan. 2007. The impact of consumer internet experience on channel preference and usage intentions across the different stages of the buying process. Journal of Interactive Marketing 21 (2): 26–41. https://doi.org/10.1002/dir.20079 .
Gallego, Guillermo, and Hu Ming. 2014. Dynamic pricing of perishable assets under competition. Management Science 60 (5): 1241–1259. https://doi.org/10.1287/mnsc.2013.1821 .
Gallego, Guillermo, Woonghee Tim Huh, Wanmo Kang, and Robert Phillips. 2006. Price competition with the attraction demand model: Existence of unique equilibrium and its stability. Manufacturing & Service Operations Management 8 (4): 359–375. https://doi.org/10.1287/msom.1060.0115 .
Gallego, Guillermo, and Garrett J. van Ryzin. 1997. A multiproduct dynamic pricing problem and its applications to network yield management. Operations Research 45 (1): 24–41. https://doi.org/10.1287/opre.45.1.24 .
Gallego, Guillermo, and Ruxian Wang. 2014. Multi-product optimization and competition under the nested logit model with product-differentiated price sensitivities. Operations Research 62 (2): 450–461. https://doi.org/10.1287/opre.2013.1249 .
Gao, Fei, and Su Xuanming. 2018. Omnichannel service operations with online and offline self-order technologies. Management Science 64 (8): 3595–3608. https://doi.org/10.1287/mnsc.2017.2787 .
Geng, Qin, and Suman Mallik. 2007. Inventory competition and allocation in a multi-channel distribution system. European Journal of Operational Research 182 (2): 704–729. https://doi.org/10.1016/j.ejor.2006.08.041 .
Gönsch, Jochen, Robert Klein, and Claudius Steinhardt. 2009. Dynamic pricing - State-of-the-art. Journal of Business Economics 3 (2): 1–40.
Gupta, Varun, Dmitry Ivanov, and Tsan-Ming Choi. 2021. Competitive pricing of substitute products under supply disruption. Omega . https://doi.org/10.1016/j.omega.2020.102279 .
Hagiu, Andrei. 2007. Merchant or two-sided platform? Review of Network Economics 6 (2): 115–133. https://doi.org/10.2202/1446-9022.1113 .
Hall, Joseph M., Praveen K. Kopalle, and David F. Pyke. 2009. Static and dynamic pricing of excess capacity in a make-to-order environment. Production and Operations Management 18 (4): 411–425. https://doi.org/10.1111/j.1937-5956.2009.01044.x .
Hamilton, Jonathan H., and Steven M. Slutsky. 1990. Endogenous timing in duopoly games: Stackelberg or cournot equilibria. Games and Economic Behavior 2 (1): 29–46. https://doi.org/10.1016/0899-8256(90)90012-J .
Harsha, Pavithra, Shivaram Subramanian, and Joline Uichanco. 2019. Dynamic pricing of omnichannel inventories. Manufacturing & Service Operations Management 21 (1): 47–65. https://doi.org/10.1287/msom.2018.0737 .
Heese, H. Sebastian, and Victor Martínez-de-Albéniz. 2018. Effects of assortment breadth announcements on manufacturer competition. Manufacturing and Service Operations Management 20 (2): 302–316. https://doi.org/10.1287/msom.2017.0643 .
Hinterhuber, Andreas. 2008. Customer value-based pricing strategies: Why companies resist. Journal of Business Strategy 29 (4): 41–50. https://doi.org/10.1108/02756660810887079 .
Isler, Karl, and Henrik Imhof. 2008. A game theoretic model for airline revenue management and competitive pricing. Journal of Revenue and Pricing Management 7 (4): 384–396. https://doi.org/10.1057/rpm.2008.30 .
Israeli, Ayelet, Fiona Scott-Morton, Jorge Silva-Risso, and Florian Zettelmeyer. 2022. How market power affects dynamic pricing: Evidence from inventory fluctuations at car dealerships. Management Science 68 (2): 895–916. https://doi.org/10.1287/mnsc.2021.3967 .
Ittoo, Ashwin, and Nicolas Petit. 2017. Algorithmic pricing agents and tacit collusion: A technological perspective. SSRN Electronic Journal . https://doi.org/10.2139/ssrn.3046405 .
Janssen, Maarten C. W., and José Luis Moraga-González. 2004. Strategic pricing, consumer search and the number of firms. Review of Economic Studies 71 (4): 1089–1118. https://doi.org/10.1111/0034-6527.00315 .
Jerath, Kinshuk, Serguei Netessine, and Senthil K. Veeraraghavan. 2010. Revenue management with strategic customers: Last-minute selling and opaque selling. Management Science 56 (3): 430–448. https://doi.org/10.1287/mnsc.1090.1125 .
Kachani, Shmatov, Soulaymane Kachani, and Kyrylo Shmatov. 2010. Competitive pricing in a multi-product multi-attribute environment. Production and Operations Management 20 (5): 668–680. https://doi.org/10.1111/j.1937-5956.2010.01195.x .
Kastius, Alexander, and Rainer Schlosser. 2022. Dynamic pricing under competition using reinforcement learning. Journal of Revenue and Pricing Management 21 (1): 50–63. https://doi.org/10.1057/s41272-021-00285-3 .
Keskin, N. Bora, and Assaf Zeevi. 2017. Chasing demand: Learning and earning in a changing environment. Mathematics of Operations Research 42 (2): 277–307. https://doi.org/10.1287/moor.2016.0807 .
Kim, Jun B., Paulo Albuquerque, and Bart J. Bronnenberg. 2011. Mapping online consumer search. Journal of Marketing Research 48 (1): 13–27. https://doi.org/10.1509/jmkr.48.1.13 .
Koças, Cenk. 2005. A model of internet pricing under price-comparison shopping. International Journal of Electronic Commerce 10 (1): 111–134. https://doi.org/10.1080/10864415.2005.11043959 .
Könönen, Ville. 2006. Dynamic pricing based on asymmetric multiagent reinforcement learning. International Journal of Intelligent Systems 21 (1): 73–98. https://doi.org/10.1002/int.20121 .
Kopalle, Praveen, Dipayan Biswas, Pradeep K. Chintagunta, Jia Fan, Koen Pauwels, Brian T. Ratchford, and James A. Sills. 2009. Retailer pricing and competitive effects. Journal of Retailing 85 (1): 56–70. https://doi.org/10.1016/j.jretai.2008.11.005 .
Kutschinski, Erich, Thomas Uthmann, and Daniel Polani. 2003. Learning competitive pricing strategies by multi-agent reinforcement learning. Journal of Economic Dynamics and Control 27 (11): 2207–2218. https://doi.org/10.1016/S0165-1889(02)00122-7 .
Lal, Rajiv, and Ram Rao. 1997. Supermarket competition: The case of every day low pricing. Marketing Science 16 (1): 60–80. https://doi.org/10.1287/mksc.16.1.60 .
Lal, Rajiv, and Miklos Sarvary. 1999. When and how is the internet likely to decrease price competition? Marketing Science 18 (4): 485–503. https://doi.org/10.1287/mksc.18.4.485 .
Lancaster, Kelvin. 1979. Variety, equity, and efficiency: Product variety in an industrial society . New York: Columbia University Press.
Book Google Scholar
Larson, Ronald B. 2019. Promoting demand-based pricing. Journal of Revenue and Pricing Management 18 (1): 42–51. https://doi.org/10.1057/s41272-017-0126-9 .
Le Chen, Alan Mislove, and Christo Wilson. 2016. An empirical analysis of algorithmic pricing on Amazon Marketplace. In Proceedings of the 25th International Conference on World Wide Web - WWW '16 , 1339–1349, Montreal. 11-Apr-16 - 15-Apr-16. New York: ACM Press. https://doi.org/10.1145/2872427.2883089
Lebow, Sara. 2019. Worldwide ecommerce continues double-digit growth following pandemic push to online. https://www.emarketer.com/content/worldwide-ecommerce-continues-double-digit-growth-following-pandemic-push-online . Accessed 14 March 2022.
Lee, Khai Sheang, and Soo Jiuan Tan. 2003. E-retailing versus physical retailing. A theoretical model and empirical test of consumer choice. Journal of Business Research 56 (11): 877–885. https://doi.org/10.1016/S0148-2963(01)00274-0 .
Lee, Thomas Y., and Eric T. Bradlow. 2011. Automated marketing research using online customer reviews. Journal of Marketing Research 48 (5): 881–894. https://doi.org/10.1509/jmkr.48.5.881 .
Levin, Yuri, Jeff McGill, and Mikhail Nediak. 2008. Risk in revenue management and dynamic pricing. Operations Research 56 (2): 326–343. https://doi.org/10.1287/opre.1070.0438 .
Levin, Yuri, Jeff McGill, and Mikhail Nediak. 2009. Dynamic pricing in the presence of strategic consumers and oligopolistic competition. Management Science 55: 32–46. https://doi.org/10.1287/mnsc.1080.0936 .
Li, Jun, Serguei Netessine, and Sergei Koulayev. 2017. Price to compete… with many: How to identify price competition in high-dimensional space. Management Science 64 (9): 4118–4136. https://doi.org/10.1287/mnsc.2017.2820 .
Li, Michael Z. F., Anming Zhang, and Yimin Zhang. 2008. Airline seat allocation competition. International Transactions in Operational Research 15 (4): 439–459. https://doi.org/10.1111/j.1475-3995.2008.00642.x .
Lin, Yen-Ting, Ali K. Parlaktürk, and Jayashankar M. Swaminathan. 2014. Vertical integration under competition: Forward, backward, or no integration? Production and Operations Management 23 (1): 19–35. https://doi.org/10.1111/poms.12030 .
Lin, Kyle Y., and Soheil Y. Sibdari. 2009. Dynamic price competition with discrete customer choices. European Journal of Operational Research 197 (3): 969–980. https://doi.org/10.1016/j.ejor.2007.12.040 .
Liozu, Stephan. 2015. The pricing journey: The organizational transformation toward pricing excellence . Stanford: Stanford Business Books.
Lippman, Steven A., and Kevin F. McCardle. 1997. The competitive newsboy. Operations Research 45 (1): 54–65. https://doi.org/10.1287/opre.45.1.54 .
Liu, Qian, and Dan Zhang. 2013. Dynamic pricing competition with strategic customers under vertical product differentiation. Management Science 59 (1): 84–101. https://doi.org/10.1287/mnsc.1120.1564 .
Loginova, Oksana. 2021. Price competition online: Platforms versus branded websites. Journal of Economics and Management Strategy . https://doi.org/10.1111/jems.12461 .
Mantin, Benny, Daniel Granot, and Frieda Granot. 2011. Dynamic pricing under first order Markovian competition. Naval Research Logistics 58 (6): 608–617. https://doi.org/10.1002/nav.20470 .
Martínez-de-Albéniz, Victor, and Kalyan Talluri. 2011. Dynamic price competition with fixed capacities. Management Science 57 (6): 1078–1093. https://doi.org/10.1287/mnsc.1110.1337 .
Maskin, Eric, and Jean Tirole. 1988. A theory of dynamic oligopoly, II: Price competition, kinked demand curves, and edgeworth cycles. Econometrica 56 (3): 571–599. https://doi.org/10.2307/1911701 .
Matsubayashi, Nobuo, and Yoshiyasu Yamada. 2008. A note on price and quality competition between asymmetric firms. European Journal of Operational Research 187 (2): 571–581. https://doi.org/10.1016/j.ejor.2007.03.021 .
McGill, Jeffrey I., and Garrett J. van Ryzin. 1999. Revenue management: Research overview and prospects. Transportation Science 33 (2): 233–256. https://doi.org/10.1287/trsc.33.2.233 .
Miklós-Thal, Jeanine, and Catherine Tucker. 2019. Collusion by algorithm: Does better demand prediction facilitate coordination between sellers? Management Science 65 (4): 1552–1561. https://doi.org/10.1287/mnsc.2019.3287 .
Mitra, Subrata. 2021. Economic models of price competition between traditional and online retailing under showrooming. Decision . https://doi.org/10.1007/s40622-021-00293-7 .
Mizuno, Toshihide. 2003. On the existence of a unique price equilibrium for models of product differentiation. International Journal of Industrial Organization 21 (6): 761–793. https://doi.org/10.1016/S0167-7187(03)00017-1 .
Mookherjee, Reetabrata, and Terry L. Friesz. 2008. Pricing, allocation, and overbooking in dynamic service network competition when demand is uncertain. Production and Operations Management 17 (4): 455–474. https://doi.org/10.3401/poms.1080.0042 .
Moorthy, K. Sridhar. 1988. Product and price competition in a duopoly. Marketing Science 7 (2): 141–168. https://doi.org/10.1287/mksc.7.2.141 .
Motta, Massimo. 1993. Endogenous quality choice: Price vs. quantity competition. The Journal of Industrial Economics 41 (2): 113–131. https://doi.org/10.2307/2950431 .
Nalca, Arcan, Tamer Boyaci, and Saibal Ray. 2010. Competitive price-matching guarantees under imperfect store availability. Quantitative Marketing and Economics 8 (3): 275–300. https://doi.org/10.1007/s11129-010-9080-1 .
Netessine, Serguei, and Robert A. Shumsky. 2005. Revenue management games: Horizontal and vertical competition. Management Science 51 (5): 813–831. https://doi.org/10.1287/mnsc.1040.0356 .
Netzer, Oded, Ronen Feldman, Jacob Goldenberg, and Moshe Fresko. 2012. Mine your own business: Market-structure surveillance through text mining. Marketing Science 31 (3): 521–543. https://doi.org/10.1287/mksc.1120.0713 .
Nip, Kameng, Changjun Wang, and Zizhuo Wang. 2020. Competitive and cooperative assortment games under Markov chain choice model. SSRN Electronic Journal . https://doi.org/10.2139/ssrn.3607722 .
Noel, Michael D. 2007. Edgeworth price cycles, cost-based pricing, and sticky pricing in retail gasoline markets. Review of Economics and Statistics 89 (2): 324–334. https://doi.org/10.1162/rest.89.2.324 .
Obermeyer, Andy, Christos Evangelinos, and Ronny Püschel. 2013. Price dispersion and competition in European airline markets. Journal of Air Transport Management 26 (1): 31–34. https://doi.org/10.1016/j.jairtraman.2012.08.014 .
Olivares, Marcelo, and Gérard. P. Cachon. 2009. Competing retailers and inventory: An empirical investigation of General Motors’ dealerships in isolated U.S. markets. Management Science 55 (9): 1586–1604. https://doi.org/10.1287/mnsc.1090.1050 .
Parlar, Mahmut. 1988. Game theoretic analysis of the substitutable product inventory problem with random demands. Naval Research Logistics 35 (3): 397–409. https://doi.org/10.1002/1520-6750(198806)35:3%3c397:AID-NAV3220350308%3e3.0.CO;2-Z .
Penz, Elfriede, and Margaret K. Hogg. 2011. The role of mixed emotions in consumer behaviour. European Journal of Marketing 45 (1): 104–132. https://doi.org/10.1108/03090561111095612 .
Perakis, Georgia, and Anshul Sood. 2006. Competitive multi-period pricing for perishable products: A robust optimization approach. Mathematical Programming 107 (1–2): 295–335. https://doi.org/10.1007/s10107-005-0688-y .
Perloff, Jeffrey M., and Steven C. Salop. 1985. Equilibrium with product differentiation. Review of Economic Studies 52 (1): 107. https://doi.org/10.2307/2297473 .
Phillips, Robert L. 2021. Pricing and revenue optimization , 2nd ed. Stanford: Stanford Business Books.
Pigou, Arthur Cecil. 1920. The economics of welfare . London: Macmillan & co.
Popescu, Dana. 2015. Repricing algorithms in e-commerce. Technology and Operations Management (Working Paper No. 2015/75/TOM). https://doi.org/10.2139/ssrn.2669997 .
Putsis, William, and Ravi Dhar. 1998. The many faces of competition. Marketing Letters 9 (3): 269–284. https://doi.org/10.1023/A:1008072222645 .
Ratchford, Brian T. 2009. Online pricing: Review and directions for research. Journal of Interactive Marketing 23 (1): 82–90. https://doi.org/10.1016/j.intmar.2008.11.001 .
Richards, Timothy J., and Stephen F. Hamilton. 2006. Rivalry in price and variety among supermarket retailers. American Journal of Agricultural Economics 88 (3): 710–726. https://doi.org/10.1111/j.1467-8276.2006.00890.x .
Ringel, Daniel M., and Bernd Skiera. 2016. Visualizing asymmetric competition among more than 1,000 products using big search data. Marketing Science 35 (3): 511–534. https://doi.org/10.1287/mksc.2015.0950 .
Ryan, Jennifer K., Daewon Sun, and Xuying Zhao. 2012. Competition and coordination in online marketplaces. Production and Operations Management 21 (6): 997–1014. https://doi.org/10.1111/j.1937-5956.2012.01332.x .
Salop, Steven C. 1976. Information and monopolistic competition. American Economic Review 66 (2): 240–245.
Sarkar, Amit, and Brojeswar Pal. 2021. Competitive pricing strategies of multi channel supply chain under direct servicing by the manufacturer. RAIRO Operations Research 55 (1): 1849–1873. https://doi.org/10.1051/ro/2020063 .
Scarpi, Daniele, Gabriele Pizzi, and Marco Visentin. 2014. Shopping for fun or shopping to buy: Is it different online and offline? Journal of Retailing and Consumer Services 21 (3): 258–267. https://doi.org/10.1016/j.jretconser.2014.02.007 .
Schinkel, Maarten Pieter, Jan Tuinstra, and Dries Vermeulen. 2002. Convergence of Bayesian learning to general equilibrium in mis-specified models. Journal of Mathematical Economics 38 (4): 483–508. https://doi.org/10.1016/S0304-4068(02)00062-9 .
Schlereth, Christian, Bernd Skiera, and Fabian Schulz. 2018. Why do consumers prefer static instead of dynamic pricing plans? An empirical study for a better understanding of the low preferences for time-variant pricing plans. European Journal of Operational Research 269 (3): 1165–1179. https://doi.org/10.1016/j.ejor.2018.03.033 .
Schlosser, Rainer, and Martin Boissier. 2018. Dealing with the dimensionality curse in dynamic pricing competition: Using frequent repricing to compensate imperfect market anticipations. Computers and Operations Research 100: 26–42. https://doi.org/10.1016/j.cor.2018.07.011 .
Schlosser, Rainer, Martin Boissier, Andre Schober, and Matthias Uflacker. 2016. How to survive dynamic pricing competition in e-commerce. In Proceedings of the 10th ACM conference on recommender systems, Boston. 15-Sept-16 to 19-Sept-16. New York: Association for Computing Machinery.
Schlosser, Rainer, and Keven Richly. 2019. Dynamic pricing under competition with data-driven price anticipations and endogenous reference price effects. Journal of Revenue and Pricing Management 18: 451–464. https://doi.org/10.1057/s41272-019-00206-5 .
Serth, Sebastian, Nikolai Podlesney, Marvin Bornstein, Jan Lindemann, Johanna Lattt, Jan Selke, Rainer Schlosser, Martin Boissier, and Matthias Uflacker. 2017. An interactive platform to simulate dynamic pricing competition on online marketplaces. In IEEE 21st International Enterprise Distributed Object Computing Conference , 61–66, Quebec City, Canada. 10-Oct-17 to 13-Oct-17. https://doi.org/10.1109/EDOC.2017.17 .
Shugan, Steven M. 2002. Editorial: Marketing science, models, monopoly models, and why we need them. Marketing Science 21 (3): 223–228. https://doi.org/10.1287/mksc.21.3.223.145 .
Siegert, Caspar, and Robert Ulbricht. 2020. Dynamic oligopoly pricing: Evidence from the airline industry. International Journal of Industrial Organization 71: 102639. https://doi.org/10.1016/j.ijindorg.2020.102639 .
Simon, Hermann. 1979. Dynamics of price elasticity and brand life cycles: An empirical study. Journal of Marketing Research 16 (4): 439–452. https://doi.org/10.1177/002224377901600401 .
Smith, Michael D., and Erik Brynjolfsson. 2001. Consumer decision-making at an internet shopbot: Brand still matters. The Journal of Industrial Economics 49 (4): 541–558. https://doi.org/10.1111/1467-6451.00162 .
Stigler, George J. 1957. Perfect competition, historically contemplated. Journal of Political Economy 65 (1): 1–17. https://doi.org/10.1086/257878 .
Stiglitz, Joseph E. 1979. Equilibrium in product markets with imperfect information. American Economic Review 69 (2): 339–345.
Sun, Haoying, and Stephen M. Gilbert. 2019. Retail price competition with product fit uncertainty and assortment selection. Production and Operations Management 28 (7): 1658–1673. https://doi.org/10.1111/poms.13005 .
Talluri, Kalyan T., and Garrett J. van Ryzin. 2004. The theory and practice of revenue management . Boston: Springer.
Thomadsen, Raphael. 2007. Product positioning and competition: The role of location in the fast food industry. Marketing Science 26 (6): 792–804. https://doi.org/10.1287/mksc.1070.0296 .
Tranfield, David, David Denyer, and Palminder Smart. 2003. Towards a methodology for developing evidence-informed management knowledge by means of systematic review. British Journal of Management 14 (3): 207–222. https://doi.org/10.1111/1467-8551.00375 .
Tuinstra. 2004. A price adjustment process in a model of monopolistic competition. International Game Theory Review 6 (3): 417–442. https://doi.org/10.1142/S0219198904000289 .
van de Geer, Ruben, Arnoud V. den Boer, Christopher Bayliss, Christine S. M. Currie, Andria Ellina, Malte Esders, Alwin Haensel, Xiao Lei, Kyle D. S. Maclean, Antonio Martinez-Sykora, Asbjørn Nilsen Riseth, Fredrik Ødegaard, and Simos Zachariades. 2019. Dynamic pricing and learning with competition: Insights from the dynamic pricing challenge at the 2017 INFORMS RM and pricing conference. Journal of Revenue and Pricing Management 18 (3): 185–203. https://doi.org/10.1057/s41272-018-00164-4 .
van Mieghem, Jan A., and Maqbool Dada. 1999. Price versus production postponement: Capacity and competition. Management Science 45 (12): 1639–1649. https://doi.org/10.1287/mnsc.45.12.1631 .
Villas-Boas, J. Miguel. 1999. Dynamic competition with customer recognition. RAND Journal of Economics 30 (4): 604–631. https://doi.org/10.2307/2556067 .
Villas-Boas, J. Miguel. 2004. Consumer learning, brand loyalty, and competition. Marketing Science 23 (1): 134–145. https://doi.org/10.1287/mksc.1030.0044 .
Villas-Boas, J. Miguel. 2006. Dynamic competition with experience goods. Journal of Economics and Management Strategy 15 (1): 37–66. https://doi.org/10.1111/j.1530-9134.2006.00091.x .
Villas-Boas, J. Miguel, and Russell S. Winer. 1999. Endogeneity in brand choice models. Management Science 45 (10): 1324–1338. https://doi.org/10.1287/mnsc.45.10.1324 .
Vinod, Balakrishna. 2020. Advances in revenue management: The last frontier. Journal of Revenue and Pricing Management 20: 15–20. https://doi.org/10.1057/s41272-020-00264-0 .
Viswanathan, Siva. 2005. Competing across technology-differentiated channels: The impact of network externalities and switching costs. Management Science 51 (3): 483–496. https://doi.org/10.1287/mnsc.1040.0338 .
Vives, Xavier. 2001. Oligopoly pricing: Old ideas and new tools . London: MIT Press.
von Stackelberg, Heinrich. 2011. Market structure and equilibrium . Berlin: Springer.
Wang, Zizhuo, and Hu Ming. 2014. Committed versus contingent pricing under competition. Production and Operations Management 23 (11): 1919–1936. https://doi.org/10.1111/poms.12202 .
Wang, Sujuan, Hu Qiying, and Liu Weiqi. 2017. Price and quality-based competition and channel structure with consumer loyalty. European Journal of Operational Research 262 (2): 563–574. https://doi.org/10.1016/j.ejor.2017.03.052 .
Wang, Yongzhao, and Xiaojie Sun. 2019. Dynamic vs. static wholesale pricing strategies in a dual-channel green supply chain. Complexity 13: 1–14. https://doi.org/10.1155/2019/8497070 .
Wang, Ningning, Ting Zhang, Xiaowei Zhu, and Peimiao Li. 2020. Online-offline competitive pricing with reference price effect. Journal of the Operational Research Society 72 (3): 642–653. https://doi.org/10.1080/01605682.2019.1696154 .
Wang, Wenche, Fan Li, and Yujia Zhang. 2021. Price discount and price dispersion in online market: Do more firms still lead to more competition? Journal of Theoretical and Applied Electronic Commerce Research 16 (2): 164–178. https://doi.org/10.4067/S0718-18762021000200111 .
Weatherford, Lawrence R., and Samuel E. Bodily. 1992. A taxonomy and research overview of perishable-asset revenue management: Yield management, overbooking, and pricing. Operations Research 40 (5): 831–844. https://doi.org/10.1287/opre.40.5.831 .
Weintraub, Gabriel Y., C. Lanier Benkard, and Benjamin van Roy. 2008. Markov perfect industry dynamics with many firms. Econometrica 76 (6): 1375–1411. https://doi.org/10.3982/ECTA6158 .
Wenzelburger, J. 2004. Learning to play best response in duopoly games. International Game Theory Review 6 (3): 443–459. https://doi.org/10.1142/S0219198904000290 .
Won, Eugene J. S., Oh. Yun Kyung, and Joon Yeon Choeh. 2022. Analyzing competitive market structures based on online consumer-generated content and sales data. Asia Pacific Journal of Marketing and Logistics Advance Online Publication . https://doi.org/10.1108/APJML-08-2021-0616 .
Wu, Lin-Liang Bill, and Desheng Wu. 2016. Dynamic pricing and risk analytics under competition and stochastic reference price effects. IEEE Transactions on Industrial Informatics 12 (3): 1282–1293. https://doi.org/10.1109/TII.2015.2507141 .
Xu, Xiaowei, and Wallace J. Hopp. 2006. A monopolistic and oligopolistic stochastic flow revenue management model. Operations Research 54 (6): 1098–1109. https://doi.org/10.1287/opre.1060.0336 .
Yang, Jian, and Yusen Xia. 2013. A nonatomic-game approach to dynamic pricing under competition. Production and Operations Management 22 (1): 88–103. https://doi.org/10.1111/j.1937-5956.2012.01334.x .
Yang, Yongge, Yu-Ching Lee, and Po-An Chen. 2020. Competitive demand learning: a data-driven pricing algorithm. Cornell University (Working Paper). https://doi.org/10.48550/arxiv.2008.05195 .
Yano, Makoto, and Takashi Komatsubara. 2006. Endogenous price leadership and technological differences. International Journal of Economic Theory 2 (3–4): 365–383. https://doi.org/10.1111/j.1742-7363.2006.0041.x .
Yano, Makoto, and Takashi Komatsubara. 2018. Price competition or price leadership. Economic Theory 66 (4): 1023–1057. https://doi.org/10.1007/s00199-017-1080-x .
Yao, Dong-Qing, and John J. Liu. 2005. Competitive pricing of mixed retail and e-tail distribution channels. Omega 33 (3): 235–247. https://doi.org/10.1016/j.omega.2004.04.007 .
Young, Jessica. 2022. US ecommerce grows 14.2% in 2021. https://www.digitalcommerce360.com/article/us-ecommerce-sales/ . Accessed 14 March 2022.
Zhang, Jianxiong, Liyan Lei, Shichen Zhang, and Lijun Song. 2017. Dynamic vs. static pricing in a supply chain with advertising. Computers & Industrial Engineering 109 (1): 266–279. https://doi.org/10.1016/j.cie.2017.05.006 .
Zhang, Ting, Ling Ge, Qinglong Gou, and Li-Wen Chen. 2018a. Consumer showrooming, the sunk cost effect and online-offline competition. Journal of Electronic Commerce Research 19 (1): 55–74.
Zhang, Zhichao, Qing Zhang, Zhi Liu, and Xiaoxue Zheng. 2018b. Static and dynamic pricing strategies in a closed-loop supply chain with reference quality effects. Sustainability 10 (1): 157. https://doi.org/10.3390/su10010157 .
Zhao, Xuan, Derek Atkins, Hu Ming, and Wensi Zhang. 2017. Revenue management under joint pricing and capacity allocation competition. European Journal of Operational Research 257 (3): 957–970. https://doi.org/10.1016/j.ejor.2016.08.025 .
Download references
Open Access funding enabled and organized by Projekt DEAL.
Author information
Authors and affiliations.
University of Duisburg-Essen, Duisburg, Germany
Torsten J. Gerpott & Jan Berends
You can also search for this author in PubMed Google Scholar
Corresponding author
Correspondence to Torsten J. Gerpott .
Additional information
Publisher's note.
Springer Nature remains neutral with regard to jurisdictional claims in published maps and institutional affiliations.
See Table 4 .
See Table 5 .
Rights and permissions
Open Access This article is licensed under a Creative Commons Attribution 4.0 International License, which permits use, sharing, adaptation, distribution and reproduction in any medium or format, as long as you give appropriate credit to the original author(s) and the source, provide a link to the Creative Commons licence, and indicate if changes were made. The images or other third party material in this article are included in the article's Creative Commons licence, unless indicated otherwise in a credit line to the material. If material is not included in the article's Creative Commons licence and your intended use is not permitted by statutory regulation or exceeds the permitted use, you will need to obtain permission directly from the copyright holder. To view a copy of this licence, visit http://creativecommons.org/licenses/by/4.0/ .
Reprints and permissions
About this article
Gerpott, T.J., Berends, J. Competitive pricing on online markets: a literature review. J Revenue Pricing Manag 21 , 596–622 (2022). https://doi.org/10.1057/s41272-022-00390-x
Download citation
Received : 15 March 2022
Accepted : 01 May 2022
Published : 14 June 2022
Issue Date : December 2022
DOI : https://doi.org/10.1057/s41272-022-00390-x
Share this article
Anyone you share the following link with will be able to read this content:
Sorry, a shareable link is not currently available for this article.
Provided by the Springer Nature SharedIt content-sharing initiative
- Competition
- Competitive pricing
- Dynamic pricing
- Online markets
- Find a journal
- Publish with us
- Track your research
Have a language expert improve your writing
Run a free plagiarism check in 10 minutes, generate accurate citations for free.
- Knowledge Base
Methodology
- How to Write a Literature Review | Guide, Examples, & Templates
How to Write a Literature Review | Guide, Examples, & Templates
Published on January 2, 2023 by Shona McCombes . Revised on September 11, 2023.
What is a literature review? A literature review is a survey of scholarly sources on a specific topic. It provides an overview of current knowledge, allowing you to identify relevant theories, methods, and gaps in the existing research that you can later apply to your paper, thesis, or dissertation topic .
There are five key steps to writing a literature review:
- Search for relevant literature
- Evaluate sources
- Identify themes, debates, and gaps
- Outline the structure
- Write your literature review
A good literature review doesn’t just summarize sources—it analyzes, synthesizes , and critically evaluates to give a clear picture of the state of knowledge on the subject.
Instantly correct all language mistakes in your text
Upload your document to correct all your mistakes in minutes
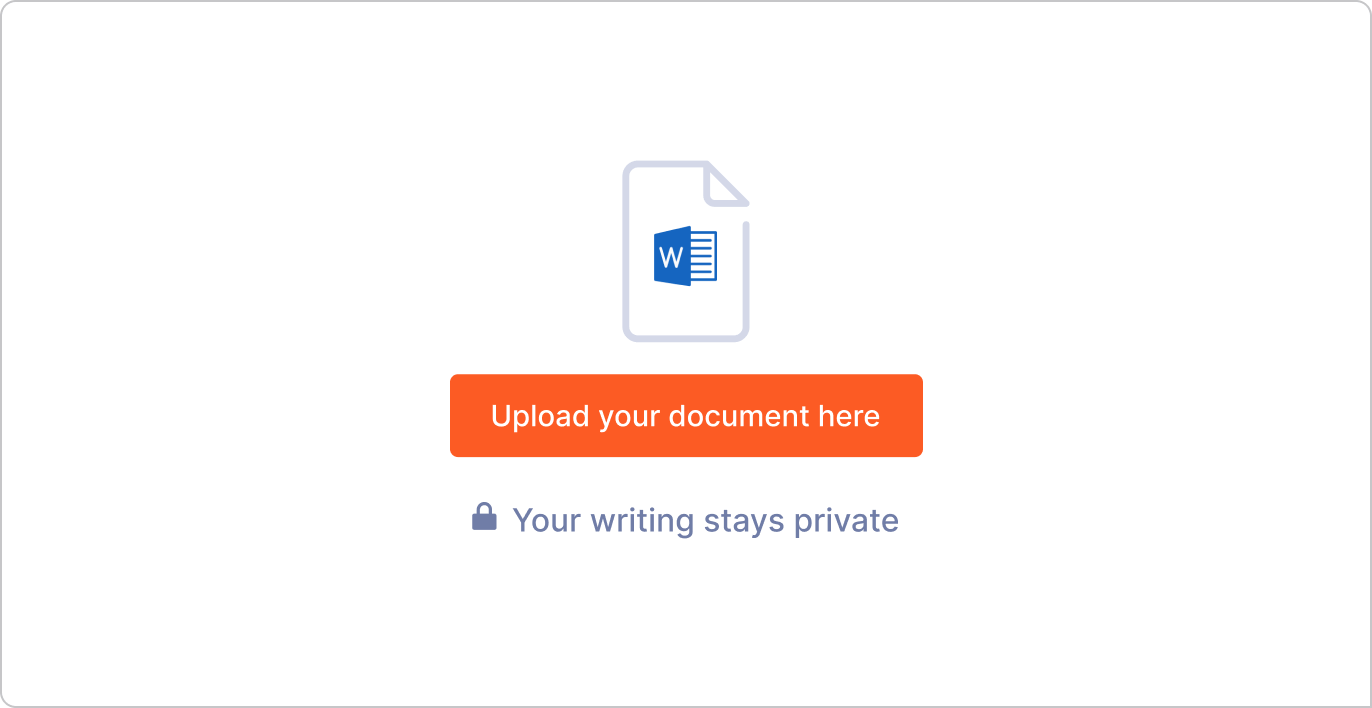
Table of contents
What is the purpose of a literature review, examples of literature reviews, step 1 – search for relevant literature, step 2 – evaluate and select sources, step 3 – identify themes, debates, and gaps, step 4 – outline your literature review’s structure, step 5 – write your literature review, free lecture slides, other interesting articles, frequently asked questions, introduction.
- Quick Run-through
- Step 1 & 2
When you write a thesis , dissertation , or research paper , you will likely have to conduct a literature review to situate your research within existing knowledge. The literature review gives you a chance to:
- Demonstrate your familiarity with the topic and its scholarly context
- Develop a theoretical framework and methodology for your research
- Position your work in relation to other researchers and theorists
- Show how your research addresses a gap or contributes to a debate
- Evaluate the current state of research and demonstrate your knowledge of the scholarly debates around your topic.
Writing literature reviews is a particularly important skill if you want to apply for graduate school or pursue a career in research. We’ve written a step-by-step guide that you can follow below.
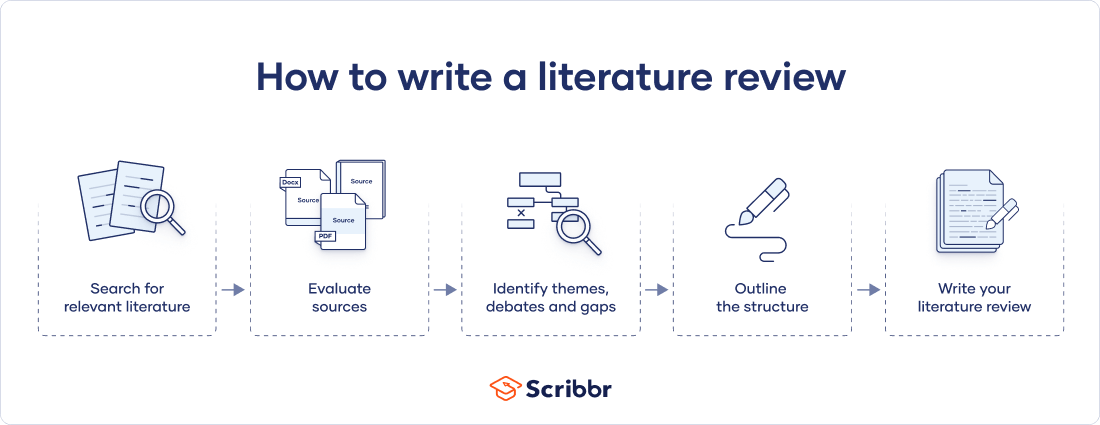
Receive feedback on language, structure, and formatting
Professional editors proofread and edit your paper by focusing on:
- Academic style
- Vague sentences
- Style consistency
See an example
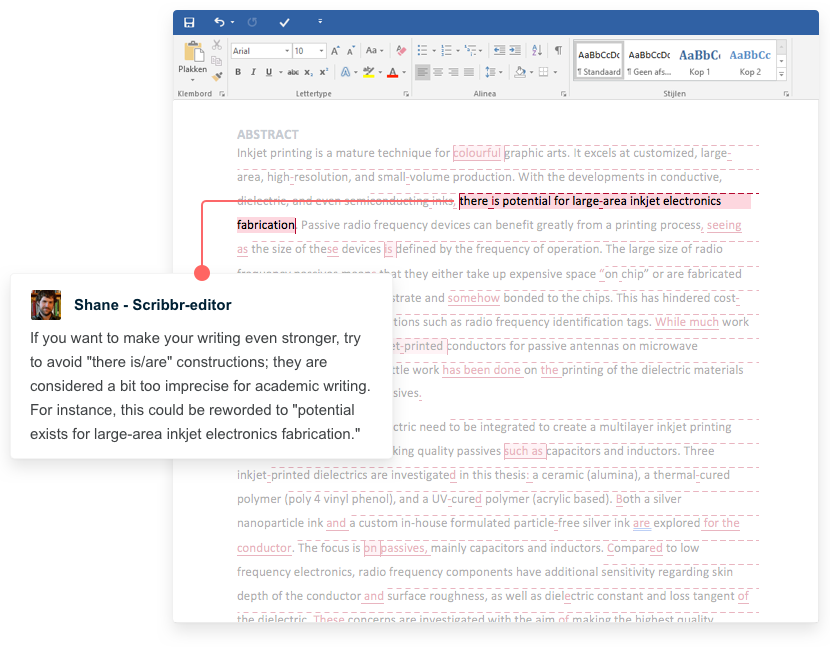
Writing literature reviews can be quite challenging! A good starting point could be to look at some examples, depending on what kind of literature review you’d like to write.
- Example literature review #1: “Why Do People Migrate? A Review of the Theoretical Literature” ( Theoretical literature review about the development of economic migration theory from the 1950s to today.)
- Example literature review #2: “Literature review as a research methodology: An overview and guidelines” ( Methodological literature review about interdisciplinary knowledge acquisition and production.)
- Example literature review #3: “The Use of Technology in English Language Learning: A Literature Review” ( Thematic literature review about the effects of technology on language acquisition.)
- Example literature review #4: “Learners’ Listening Comprehension Difficulties in English Language Learning: A Literature Review” ( Chronological literature review about how the concept of listening skills has changed over time.)
You can also check out our templates with literature review examples and sample outlines at the links below.
Download Word doc Download Google doc
Before you begin searching for literature, you need a clearly defined topic .
If you are writing the literature review section of a dissertation or research paper, you will search for literature related to your research problem and questions .
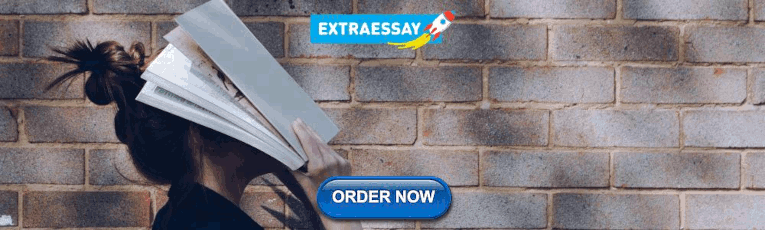
Make a list of keywords
Start by creating a list of keywords related to your research question. Include each of the key concepts or variables you’re interested in, and list any synonyms and related terms. You can add to this list as you discover new keywords in the process of your literature search.
- Social media, Facebook, Instagram, Twitter, Snapchat, TikTok
- Body image, self-perception, self-esteem, mental health
- Generation Z, teenagers, adolescents, youth
Search for relevant sources
Use your keywords to begin searching for sources. Some useful databases to search for journals and articles include:
- Your university’s library catalogue
- Google Scholar
- Project Muse (humanities and social sciences)
- Medline (life sciences and biomedicine)
- EconLit (economics)
- Inspec (physics, engineering and computer science)
You can also use boolean operators to help narrow down your search.
Make sure to read the abstract to find out whether an article is relevant to your question. When you find a useful book or article, you can check the bibliography to find other relevant sources.
You likely won’t be able to read absolutely everything that has been written on your topic, so it will be necessary to evaluate which sources are most relevant to your research question.
For each publication, ask yourself:
- What question or problem is the author addressing?
- What are the key concepts and how are they defined?
- What are the key theories, models, and methods?
- Does the research use established frameworks or take an innovative approach?
- What are the results and conclusions of the study?
- How does the publication relate to other literature in the field? Does it confirm, add to, or challenge established knowledge?
- What are the strengths and weaknesses of the research?
Make sure the sources you use are credible , and make sure you read any landmark studies and major theories in your field of research.
You can use our template to summarize and evaluate sources you’re thinking about using. Click on either button below to download.
Take notes and cite your sources
As you read, you should also begin the writing process. Take notes that you can later incorporate into the text of your literature review.
It is important to keep track of your sources with citations to avoid plagiarism . It can be helpful to make an annotated bibliography , where you compile full citation information and write a paragraph of summary and analysis for each source. This helps you remember what you read and saves time later in the process.
Don't submit your assignments before you do this
The academic proofreading tool has been trained on 1000s of academic texts. Making it the most accurate and reliable proofreading tool for students. Free citation check included.
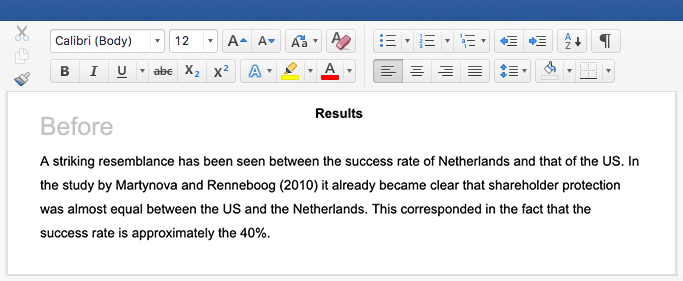
Try for free
To begin organizing your literature review’s argument and structure, be sure you understand the connections and relationships between the sources you’ve read. Based on your reading and notes, you can look for:
- Trends and patterns (in theory, method or results): do certain approaches become more or less popular over time?
- Themes: what questions or concepts recur across the literature?
- Debates, conflicts and contradictions: where do sources disagree?
- Pivotal publications: are there any influential theories or studies that changed the direction of the field?
- Gaps: what is missing from the literature? Are there weaknesses that need to be addressed?
This step will help you work out the structure of your literature review and (if applicable) show how your own research will contribute to existing knowledge.
- Most research has focused on young women.
- There is an increasing interest in the visual aspects of social media.
- But there is still a lack of robust research on highly visual platforms like Instagram and Snapchat—this is a gap that you could address in your own research.
There are various approaches to organizing the body of a literature review. Depending on the length of your literature review, you can combine several of these strategies (for example, your overall structure might be thematic, but each theme is discussed chronologically).
Chronological
The simplest approach is to trace the development of the topic over time. However, if you choose this strategy, be careful to avoid simply listing and summarizing sources in order.
Try to analyze patterns, turning points and key debates that have shaped the direction of the field. Give your interpretation of how and why certain developments occurred.
If you have found some recurring central themes, you can organize your literature review into subsections that address different aspects of the topic.
For example, if you are reviewing literature about inequalities in migrant health outcomes, key themes might include healthcare policy, language barriers, cultural attitudes, legal status, and economic access.
Methodological
If you draw your sources from different disciplines or fields that use a variety of research methods , you might want to compare the results and conclusions that emerge from different approaches. For example:
- Look at what results have emerged in qualitative versus quantitative research
- Discuss how the topic has been approached by empirical versus theoretical scholarship
- Divide the literature into sociological, historical, and cultural sources
Theoretical
A literature review is often the foundation for a theoretical framework . You can use it to discuss various theories, models, and definitions of key concepts.
You might argue for the relevance of a specific theoretical approach, or combine various theoretical concepts to create a framework for your research.
Like any other academic text , your literature review should have an introduction , a main body, and a conclusion . What you include in each depends on the objective of your literature review.
The introduction should clearly establish the focus and purpose of the literature review.
Depending on the length of your literature review, you might want to divide the body into subsections. You can use a subheading for each theme, time period, or methodological approach.
As you write, you can follow these tips:
- Summarize and synthesize: give an overview of the main points of each source and combine them into a coherent whole
- Analyze and interpret: don’t just paraphrase other researchers — add your own interpretations where possible, discussing the significance of findings in relation to the literature as a whole
- Critically evaluate: mention the strengths and weaknesses of your sources
- Write in well-structured paragraphs: use transition words and topic sentences to draw connections, comparisons and contrasts
In the conclusion, you should summarize the key findings you have taken from the literature and emphasize their significance.
When you’ve finished writing and revising your literature review, don’t forget to proofread thoroughly before submitting. Not a language expert? Check out Scribbr’s professional proofreading services !
This article has been adapted into lecture slides that you can use to teach your students about writing a literature review.
Scribbr slides are free to use, customize, and distribute for educational purposes.
Open Google Slides Download PowerPoint
If you want to know more about the research process , methodology , research bias , or statistics , make sure to check out some of our other articles with explanations and examples.
- Sampling methods
- Simple random sampling
- Stratified sampling
- Cluster sampling
- Likert scales
- Reproducibility
Statistics
- Null hypothesis
- Statistical power
- Probability distribution
- Effect size
- Poisson distribution
Research bias
- Optimism bias
- Cognitive bias
- Implicit bias
- Hawthorne effect
- Anchoring bias
- Explicit bias
A literature review is a survey of scholarly sources (such as books, journal articles, and theses) related to a specific topic or research question .
It is often written as part of a thesis, dissertation , or research paper , in order to situate your work in relation to existing knowledge.
There are several reasons to conduct a literature review at the beginning of a research project:
- To familiarize yourself with the current state of knowledge on your topic
- To ensure that you’re not just repeating what others have already done
- To identify gaps in knowledge and unresolved problems that your research can address
- To develop your theoretical framework and methodology
- To provide an overview of the key findings and debates on the topic
Writing the literature review shows your reader how your work relates to existing research and what new insights it will contribute.
The literature review usually comes near the beginning of your thesis or dissertation . After the introduction , it grounds your research in a scholarly field and leads directly to your theoretical framework or methodology .
A literature review is a survey of credible sources on a topic, often used in dissertations , theses, and research papers . Literature reviews give an overview of knowledge on a subject, helping you identify relevant theories and methods, as well as gaps in existing research. Literature reviews are set up similarly to other academic texts , with an introduction , a main body, and a conclusion .
An annotated bibliography is a list of source references that has a short description (called an annotation ) for each of the sources. It is often assigned as part of the research process for a paper .
Cite this Scribbr article
If you want to cite this source, you can copy and paste the citation or click the “Cite this Scribbr article” button to automatically add the citation to our free Citation Generator.
McCombes, S. (2023, September 11). How to Write a Literature Review | Guide, Examples, & Templates. Scribbr. Retrieved September 3, 2024, from https://www.scribbr.com/dissertation/literature-review/
Is this article helpful?
Shona McCombes
Other students also liked, what is a theoretical framework | guide to organizing, what is a research methodology | steps & tips, how to write a research proposal | examples & templates, "i thought ai proofreading was useless but..".
I've been using Scribbr for years now and I know it's a service that won't disappoint. It does a good job spotting mistakes”
Information
- Author Services
Initiatives
You are accessing a machine-readable page. In order to be human-readable, please install an RSS reader.
All articles published by MDPI are made immediately available worldwide under an open access license. No special permission is required to reuse all or part of the article published by MDPI, including figures and tables. For articles published under an open access Creative Common CC BY license, any part of the article may be reused without permission provided that the original article is clearly cited. For more information, please refer to https://www.mdpi.com/openaccess .
Feature papers represent the most advanced research with significant potential for high impact in the field. A Feature Paper should be a substantial original Article that involves several techniques or approaches, provides an outlook for future research directions and describes possible research applications.
Feature papers are submitted upon individual invitation or recommendation by the scientific editors and must receive positive feedback from the reviewers.
Editor’s Choice articles are based on recommendations by the scientific editors of MDPI journals from around the world. Editors select a small number of articles recently published in the journal that they believe will be particularly interesting to readers, or important in the respective research area. The aim is to provide a snapshot of some of the most exciting work published in the various research areas of the journal.
Original Submission Date Received: .
- Active Journals
- Find a Journal
- Proceedings Series
- For Authors
- For Reviewers
- For Editors
- For Librarians
- For Publishers
- For Societies
- For Conference Organizers
- Open Access Policy
- Institutional Open Access Program
- Special Issues Guidelines
- Editorial Process
- Research and Publication Ethics
- Article Processing Charges
- Testimonials
- Preprints.org
- SciProfiles
- Encyclopedia
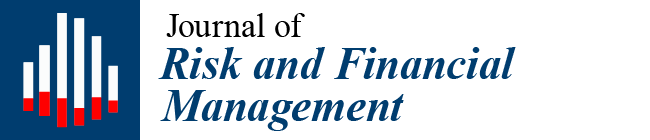
Article Menu
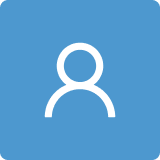
- Subscribe SciFeed
- Recommended Articles
- Google Scholar
- on Google Scholar
- Table of Contents
Find support for a specific problem in the support section of our website.
Please let us know what you think of our products and services.
Visit our dedicated information section to learn more about MDPI.
JSmol Viewer
Market orientation and hotel industry: literature review and implications for periods of market turmoil.
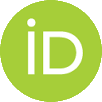
1. Introduction
2. literature review, 3. data and methods, 3.2. methods, 4.1. descriptive analysis, 4.2. co-citation analysis, 5. discussion, future research, and conclusions, 5.1. discussion, 5.2. future research proposals, 5.3. conclusions, author contributions, institutional review board statement, informed consent statement, data availability statement, conflicts of interest.
- Agarwal, Sanjeev, M. Krishna Erramilli, and Chekitan S. Dev. 2003. Market Orientation and Performance in Service Firms: Role of Innovation. Journal of Services Marketing 17: 68–82. [ Google Scholar ] [ CrossRef ]
- Alderson, W. 1957. Marketing Behaviour and Executive Action . Homewood: R. D. Irwin, Inc. [ Google Scholar ]
- Ali, Faizan, S. Mostafa Rasoolimanesh, Marko Sarstedt, Christian M. Ringle, and Kisang Ryu. 2018. An Assessment of the Use of Partial Least Squares Structural Equation Modeling (PLS-SEM) in Hospitality Research. International Journal of Contemporary Hospitality Management 30: 514–38. [ Google Scholar ] [ CrossRef ] [ Green Version ]
- Aliperti, Giuseppe, and Ana Maria Cruz. 2019. Investigating Tourists’ Risk Information Processing. Annals of Tourism Research 79: 102803. [ Google Scholar ] [ CrossRef ]
- Anderson, James C., and David W. Gerbing. 1988. Structural Equation Modeling in Practice: A Review and Recommended Two-Step Approach. Psychological Bulletin 103: 411–23. [ Google Scholar ] [ CrossRef ]
- Aria, Massimo, and Corrado Cuccurullo. 2017. Bibliometrix: An R-Tool for Comprehensive Science Mapping Analysis. Journal of Informetrics 11: 959–75. [ Google Scholar ] [ CrossRef ]
- Armstrong, J. Scott, and Terry S. Overton. 1977. Estimating Nonresponse Bias in Mail Surveys. Journal of Marketing Research 14: 396–402. [ Google Scholar ] [ CrossRef ] [ Green Version ]
- Arnedo, Esther González, Jesús Alberto Valero-Matas, and Antonio Sánchez-Bayón. 2021. Spanish Tourist Sector Sustainability: Recovery Plan, Green Jobs and Wellbeing Opportunity. Sustainability 13: 11447. [ Google Scholar ] [ CrossRef ]
- Bagozzi, Richard P., and Youjae Yi. 1991. Multitrait-Multimethod Matrices in Consumer Research. Journal of Consumer Research 17: 426–39. [ Google Scholar ] [ CrossRef ]
- Bagozzi, Richard P., and Youjae Yi. 2012. Specification, Evaluation, and Interpretation of Structural Equation Models. Journal of the Academy of Marketing Science 40: 8–34. [ Google Scholar ] [ CrossRef ]
- Baker, William E., and James M. Sinkula. 1999. The Synergistic Effect of Market Orientation and Learning Orientation on Organizational Performance. Journal of the Academy of Marketing Science 27: 411–27. [ Google Scholar ] [ CrossRef ]
- Barney, Jay. 1991. Firm Resources and Sustained Competitive Advantage. Journal of Management 17: 99–120. [ Google Scholar ] [ CrossRef ]
- Baron, R. M., and D. A. Kenny. 1986. The Moderator-Mediator Variable Distinction in Social Psychological Research: Conceptual, Strategic, and Statistical Considerations. Journal of Personality and Social Psychology 51: 1173–82. [ Google Scholar ] [ CrossRef ]
- Bearden, William O., and Jesse E. Teel. 1983. Selected Determinats of Consumer Satisfaction and Complaint Reports. Journal of Marketing Research 20: 21–28. [ Google Scholar ] [ CrossRef ]
- Chang, Sea Jin, Arjen Van Witteloostuijn, and Lorraine Eden. 2010. From the Editors: Common Method Variance in International Business Research. Journal of International Business Studies 41: 178–84. [ Google Scholar ] [ CrossRef ]
- Chang, Song, Yaping Gong, and Cass Shum. 2011. Promoting Innovation in Hospitality Companies through Human Resource Management Practices. International Journal of Hospitality Management 30: 812–18. [ Google Scholar ] [ CrossRef ] [ Green Version ]
- Chang, Tung-Zong, and Su-Jane Chen. 1998. Market Orientation, Service Quality and Business Profitability: A Conceptual Model and Empirical Evidence. Journal of Services Marketing 12: 246–64. [ Google Scholar ] [ CrossRef ]
- Chin, Wynne W. 1998. The Partial Least Squares Approach to Structural Equation Modeling. In Modern Methods for Business Research . Edited by G. A. Marcoulides. Mahwah: Lawrence Erlbaum Associates, pp. 295–336. [ Google Scholar ] [ CrossRef ] [ Green Version ]
- Cohen, Erik. 2012. Globalization, Global Crises and Tourism. Tourism Recreation Research 37: 103–11. [ Google Scholar ] [ CrossRef ]
- Covin, Jeffrey G., and Dennis P. Slevin. 1989. Strategic Management of Small Firms in Hostile and Benign Environments. Strategic Management Journal 10: 75–87. [ Google Scholar ] [ CrossRef ]
- Day, George S., and Prakash Nedungadi. 1994. Managerial Representations of Competitive Advantage. Journal of Marketing 58: 31–44. [ Google Scholar ] [ CrossRef ]
- Day, George S., and Robin Wensley. 1988. Assessing Advantage: A Framework for Diagnosing Competitive Superiority. Journal of Marketing 52: 1–20. [ Google Scholar ] [ CrossRef ]
- Deshpandé, Rohit, and John U. Farley. 1998. Measuring Market Orientation: Generalization and Synthesis. Journal of Market-Focused Management 2: 213–32. [ Google Scholar ] [ CrossRef ]
- Deshpande, Rohit, John U. Farley, and Frederick E. Webster Jr. 1993. Corporate Culture, Customer Orientation, and Innovativeness in Japanese Firms: A Quadrad Analysis. Journal of Marketing 57: 23–27. [ Google Scholar ] [ CrossRef ] [ Green Version ]
- Drucker, Peter F. 1954. The Practice of Management . New York: Harper & Row. [ Google Scholar ]
- Ellegaard, Ole, and Johan A. Wallin. 2015. The Bibliometric Analysis of Scholarly Production: How Great Is the Impact? Scientometrics 105: 1809–31. [ Google Scholar ] [ CrossRef ] [ PubMed ] [ Green Version ]
- Farmaki, Anna. 2021. Memory and Forgetfulness in Tourism Crisis Research. Tourism Management 83: 104210. [ Google Scholar ] [ CrossRef ]
- Fornell, Claes, and David F. Larker. 1981. Evaluating Structural Equation Models with Unobservable Variables and Measurement Error. Journal of Marketing Research 18: 39–50. [ Google Scholar ] [ CrossRef ]
- Fuller, Christie M., Marcia J. Simmering, Guclu Atinc, Yasemin Atinc, and Barry J. Babin. 2016. Common Methods Variance Detection in Business Research. Journal of Business Research 69: 3192–98. [ Google Scholar ] [ CrossRef ]
- Gheysari, Hamed, Amran Rasli, Parastoo Roghanian, and Nadhirah Norhalim. 2012. A Review on the Market Orientation Evolution. Procedia Social and Behavioral Sciences 40: 542–49. [ Google Scholar ] [ CrossRef ] [ Green Version ]
- Gössling, Stefan, Daniel Scott, and C. Michael Hall. 2021. Pandemics, Tourism and Global Change: A Rapid Assessment of COVID-19. Journal of Sustainable Tourism 29: 1–20. [ Google Scholar ] [ CrossRef ]
- Gray, Brendan J., Sheelagh Matear, and Philip K. Matheson. 2002. Improving Service Firm Performance. Journal of Services Marketing 16: 186–200. [ Google Scholar ] [ CrossRef ]
- Gray, Brendan, Sheelagh Matear, Christo Boshoff, and Phil Matheson. 1998. Developing a Better Measure of Market Orientation. European Journal of Marketing 32: 884–903. [ Google Scholar ] [ CrossRef ]
- Grissemann, Ursula, Andreas Plank, and Alexandra Brunner-Sperdin. 2013. Enhancing Business Performance of Hotels: The Role of Innovation and Customer Orientation. International Journal of Hospitality Management 33: 347–56. [ Google Scholar ] [ CrossRef ]
- Han, Jin K., Namwoon Kim, and Rajendra K. Srivastava. 1998. Market Orientation and Organizational Performance: Is Innovation a Missing Link? Journal of Marketing 62: 30. [ Google Scholar ] [ CrossRef ]
- Haugland, Sven A., Ingunn Myrtveit, and Arne Nygaard. 2007. Market Orientation and Performance in the Service Industry: A Data Envelopment Analysis. Journal of Business Research 60: 1191–97. [ Google Scholar ] [ CrossRef ]
- Hjalager, Anne Mette. 2010. A Review of Innovation Research in Tourism. Tourism Management 31: 1–12. [ Google Scholar ] [ CrossRef ]
- Hu, Li Tze, and Peter M. Bentler. 1999. Cutoff Criteria for Fit Indexes in Covariance Structure Analysis: Conventional Criteria versus New Alternatives. Structural Equation Modeling 6: 1–55. [ Google Scholar ] [ CrossRef ]
- Hult, G. Tomas M., and David J. Ketchen. 2001. Does Market Orientation Matter?: A Test of the Relationship between Positional Advantage and Performance. Strategic Management Journal 22: 899–906. [ Google Scholar ] [ CrossRef ]
- Hult, G. Tomas M., Robert F. Hurley, and Gary A. Knight. 2004. Innovativeness: Its Antecedents and Impact on Business Performance. Industrial Marketing Management 33: 429–38. [ Google Scholar ] [ CrossRef ]
- Hurley, Robert F., and G. Tomas M. Hult. 1998. Innovation, Market Orientation, and Organizational Learning: An Integration and Empirical Examination. Journal of Marketing 62: 42–54. [ Google Scholar ] [ CrossRef ]
- Jaworski, Bernard J., and Ajay K. Kohli. 1993. Market Orientation: Antecedents and Consequences. Journal of Marketing 57: 53–70. [ Google Scholar ] [ CrossRef ]
- Jogaratnam, Giri. 2017. The Effect of Market Orientation, Entrepreneurial Orientation and Human Capital on Positional Advantage: Evidence from the Restaurant Industry. International Journal of Hospitality Management 60: 104–13. [ Google Scholar ] [ CrossRef ]
- Jogaratnam, Giri, and Eliza Ching-Yick Tse. 2006. Entrepreneurial Orientation and the Structuring of Organizations: Performance Evidence from the Asian Hotel Industry. International Journal of Contemporary Hospitality Management 18: 454–68. [ Google Scholar ] [ CrossRef ]
- Johnston, Robert. 1997. Identifying the Critical Determinants of Service Quality in Retail Banking: Importance and Effect. International Journal of Bank Marketing 15: 111–16. [ Google Scholar ] [ CrossRef ] [ Green Version ]
- Kasim, Azilah, Yuksel Ekinci, Levent Altinay, and Kashif Hussain. 2018. Impact of Market Orientation, Organizational Learning and Market Conditions on Small and Medium-Size Hospitality Enterprises. Journal of Hospitality Marketing & Management 27: 855–75. [ Google Scholar ] [ CrossRef ] [ Green Version ]
- Kelleher, Suzanne Rowan. 2022. More Than 10,000 Flight Delays Today Worldwide—Here Are the Big Trouble Spots. Forbes.com. Available online: https://www.forbes.com/sites/suzannerowankelleher/2022/07/11/10000-flight-delays-worldwide/?sh=56b134e24a00 (accessed on 20 August 2022).
- Kirca, Ahmet H., Satish Jayachandran, and William O. Bearden. 2005. Market Orientation: A Meta-Analytic Review and Assessment of Its Antecedents and Impact on Performance. Journal of Marketing 69: 24–41. [ Google Scholar ] [ CrossRef ] [ Green Version ]
- Kock, Ned. 2015. Common Method Bias in PLS-SEM. International Journal of E-Collaboration 11: 1–10. [ Google Scholar ] [ CrossRef ] [ Green Version ]
- Kohli, Ajay K., and Bernard J. Jaworski. 1990. Market Orientation: The Construct, Research Propositions and Managerial Implications. Journal of Marketing 54: 1–18. [ Google Scholar ] [ CrossRef ] [ Green Version ]
- Kohli, Ajay K., Bernard J. Jaworski, and Ajith Kumar. 1993. MARKOR: A Measure of Market Orientation. Journal of Marketing Research 30: 467–77. [ Google Scholar ] [ CrossRef ] [ Green Version ]
- Kotler, Philip. 1972. A Generic Concept of Marketing. Journal of Marketing 36: 45–54. [ Google Scholar ] [ CrossRef ]
- Langerak, Fred. 2003. An Appraisal of Research on the Predictive Power of Market Orientation. European Management Journal 21: 447–64. [ Google Scholar ] [ CrossRef ]
- Lee, Jongkuk, and Young Bong Chang. 2014. Interplay between Internal Investment and Alliance Specialization in R&D and Marketing. Industrial Marketing Management 43: 813–25. [ Google Scholar ] [ CrossRef ]
- Lee, Yong-Ki, Soon-Ho Kim, Min-Kyo Seo, and S. Kyle Hight. 2015. Market Orientation and Business Performance: Evidence from Franchising Industry. International Journal of Hospitality Management 44: 28–37. [ Google Scholar ] [ CrossRef ]
- Levitt, Theodore. 1960. Marketing Myopia. Harvard Business Review 38: 21–40. [ Google Scholar ]
- Li, Jie, Judy F. Burnham, Trey Lemley, and Robert M. Britton. 2010. Citation Analysis: Comparison of Web of Science, Scopus, SciFinder, and Google Scholar. Journal of Electronic Resources in Medical Libraries 7: 196–217. [ Google Scholar ] [ CrossRef ]
- Liao, Shu-Hsien, Wen-Jung Chang, Chi-Huan Wu, and Jerome M. Katrichis. 2011. A Survey of Market Orientation Research (1995–2008). Industrial Marketing Management 40: 301–10. [ Google Scholar ] [ CrossRef ]
- Liu, Xianbing, Can Wang, Weishi Zhang, Sunhee Suk, and Kinichi Sudo. 2013. Awareness and Acceptability of Chinese Companies on Market-Based Instruments for Energy Saving: A Survey Analysis by Sectors. Energy for Sustainable Development 17: 228–39. [ Google Scholar ] [ CrossRef ]
- Lumpkin, G. T., and Gregory G. Dess. 1996. Clarifying the Entrepreneurial Orientation Construct and Linking It to Performance. Academy of Management Review 21: 135–72. [ Google Scholar ] [ CrossRef ]
- Lumpkin, G. T., and Gregory G. Dess. 2001. Linking Two Dimensions of Entrepreneurial Orientation to Firm Performance: The Moderating Role of Environment and Industry Life Cycle. Journal of Business Venturing 16: 429–51. [ Google Scholar ] [ CrossRef ]
- Marshakova-Shaikevich, Irena V. 1973. System of Document Connections Based on References. Scientific and Technical Information Serial of VINITI 2: 3–8. [ Google Scholar ]
- Martínez-Ros, Ester, and Francina Orfila-Sintes. 2009. Innovation Activity in the Hotel Industry. Technovation 29: 632–41. [ Google Scholar ] [ CrossRef ] [ Green Version ]
- McCain, Katherine W. 1990. Mapping Authors in Intellectual Space: A Technical Overview: Introduction. Journal of the American Society for Information Science (1986-1998) 41: 433. [ Google Scholar ] [ CrossRef ]
- McCain, Katherine W. 1991. Mapping Economics through the Journal Literature: An Experiment in Journal Cocitation Analysis. Journal of the American Society for Information Science (1986-1998) 42: 290. [ Google Scholar ] [ CrossRef ]
- McKitterick, J B. 1957. What Is the Marketing Management Concept? In The Frontiers of Marketing Thought and Science . Edited by Frank M. Bass. Chicago: AMA. [ Google Scholar ]
- Morgan, Neil A., and Lopo Leotte Rego. 2006. The Value of Different Customer Satisfaction and Loyalty Metrics in Predicting Business Performance. Marketing Science 25: 426–39. [ Google Scholar ] [ CrossRef ] [ Green Version ]
- Morris, Steven A., and Betsy der Veer Martens. 2008. Mapping Research Specialties. Annual Review of Information Science and Technology 42: 213–95. [ Google Scholar ] [ CrossRef ]
- Mullins, Laurie J. 1993. The Hotel and the Open System Model of Organizational Analysis. The Service Industries Journal 13: 1–16. [ Google Scholar ] [ CrossRef ]
- Narver, John C., and Stanley F. Slater. 1990. The Effect of a Market Orientation on Business Profitability. Journal of Marketing 54: 20–35. [ Google Scholar ] [ CrossRef ]
- Nicolau, Juan Luis, and María Jesús Santa-María. 2013. The Effect of Innovation on Hotel Market Value. International Journal of Hospitality Management 32: 71–79. [ Google Scholar ] [ CrossRef ] [ Green Version ]
- Nunnaly, Jim C. 1978. Psychometric Theory , 2nd ed. New York: McGraw-Hill. [ Google Scholar ]
- Nwokah, N. Gladson. 2008. Strategic Market Orientation and Business Performance. European Journal of Marketing 42: 279–86. [ Google Scholar ] [ CrossRef ]
- Orfila-Sintes, Francina, and Jan Mattsson. 2009. Innovation Behavior in the Hotel Industry. Omega 37: 380–94. [ Google Scholar ] [ CrossRef ]
- Orfila-Sintes, Francina, Rafel Crespí-Cladera, and Ester Martínez-Ros. 2005. Innovation Activity in the Hotel Industry: Evidence from Balearic Islands. Tourism Management 26: 851–65. [ Google Scholar ] [ CrossRef ] [ Green Version ]
- Ottenbacher, Michael C. 2007. Innovation Management in the Hospitality Industry: Different Strategies for Achieving Success. Journal of Hospitality & Tourism Research 31: 431–54. [ Google Scholar ] [ CrossRef ]
- Ottenbacher, Michael, and Juergen Gnoth. 2005. How to Develop Successful Hospitality Innovation. Cornell Hotel and Restaurant Administration Quarterly 46: 205–22. [ Google Scholar ] [ CrossRef ]
- Pawlyk, Oriana. 2022. Flying Is Painful. Climate Change Will Make It Even Worse. Politico.com. Available online: https://www.politico.com/news/2022/08/13/flying-climate-change-delays-cancellations-00050101 (accessed on 7 September 2022).
- Podsakoff, Philip M., and Dennis W. Organ. 1986. Self-Reports in Organizational Research: Problems and Prospects. Journal of Management 12: 531–44. [ Google Scholar ] [ CrossRef ]
- Podsakoff, Philip M., Scott B. MacKenzie, Jeong Yeon Lee, and Nathan P. Podsakoff. 2003. Common Method Biases in Behavioral Research: A Critical Review of the Literature and Recommended Remedies. Journal of Applied Psychology 88: 879–903. [ Google Scholar ] [ CrossRef ] [ PubMed ]
- Preacher, Kristopher J., and Andrew F. Hayes. 2008. Asymptotic and Resampling Strategies for Assessing and Comparing Indirect Effects in Multiple Mediator Models. Behavior Research Methods 40: 879–91. [ Google Scholar ] [ CrossRef ] [ PubMed ]
- R Core Team. 2020. R: A Language and Environment for Statistical Computing . Vienna: R Foundation for Statistical Computing. [ Google Scholar ]
- Ruekert, Robert W. 1992. Developing a Market Orientation: An Organizational Strategy Perspective. International Journal of Research in Marketing 9: 225–45. [ Google Scholar ] [ CrossRef ]
- Sampaio, Carlos A. F., Ricardo Gouveia Rodrigues, and José M. Hernández-Mogollón. 2021. Price Strategy, Market Orientation, and Business Performance in the Hotel Industry. Journal of Global Information Management 29: 85–102. [ Google Scholar ] [ CrossRef ]
- Sampaio, Carlos Alberto Fernandes, José Manuel Hernández-Mogollón, and Ricardo José de Ascensão Gouveia Rodrigues. 2020. The Relationship between Market Orientation, Customer Loyalty and Business Performance: A Sample from the Western Europe Hotel Industry. Tourism and Hospitality Research 20: 131–43. [ Google Scholar ] [ CrossRef ]
- Sandvik, Izabela Leskiewicz, and Kare Sandvik. 2003. The Impact of Market Orientation on Product Innovativeness and Business Performance. International Journal of Research in Marketing 20: 355–76. [ Google Scholar ] [ CrossRef ]
- Sargeant, A., and M. Mohamad. 1999. Business Performance in the UK Hotel Sector—Does It Pay to Be Market Oriented? The Service Industries Journal 19: 42–59. [ Google Scholar ] [ CrossRef ]
- Shapiro, Benson P. 1988. What the Hells Is “Market Oriented”? Harvard Business Review 66: 119–25. [ Google Scholar ]
- Sharma, Abhinav, Hakseung Shin, María Jesús Santa-María, and Juan Luis Nicolau. 2021. Hotels’ COVID-19 Innovation and Performance. Annals of Tourism Research 88: 103180. [ Google Scholar ] [ CrossRef ]
- Sin, Leo Y. M., Alan C. B. Tse, Vincent C. S. Heung, and Frederick H. K. Yim. 2005. An Analysis of the Relationship between Market Orientation and Business Performance in the Hotel Industry. International Journal of Hospitality Management 24: 555–77. [ Google Scholar ] [ CrossRef ]
- Slater, Stanley F., and John C. Narver. 1994a. Does Competitive Environment Moderate the Market Orientation-Performance Relationship? Journal of Marketing 58: 46–55. [ Google Scholar ] [ CrossRef ]
- Slater, Stanley F., and John C. Narver. 1994b. Market Orientation, Customer Value, and Superior Performance. Business Horizons 37: 22–28. [ Google Scholar ] [ CrossRef ]
- Slater, Stanley, and John C. Narver. 1995. Market Orientation and the Learning Organization. Journal of Marketing 59: 63–74. [ Google Scholar ] [ CrossRef ]
- Small, Henry. 1973. Co-Citation in the Scientific Literature: A New Measure of the Relationship between Two Documents. Journal of the American Society for Information Science 24: 265–69. [ Google Scholar ] [ CrossRef ]
- Small, Henry. 1999. Visualizing Science by Citation Mapping. Journal of the American Society for Information Science 50: 799–813. [ Google Scholar ] [ CrossRef ]
- Smeral, Egon. 2009. The Impact of the Financial and Economic Crisis on European Tourism. Journal of Travel Research 48: 3–13. [ Google Scholar ] [ CrossRef ]
- Smith, Wendell R. 1958. Marketing Strategy: What Preparations Should Precede the Marketing Concept? Printer’s Ink , 264. [ Google Scholar ]
- Sureshchandar, G. S., Rajendran Chandrasekharan, and R. N. Anantharaman. 2002. The Relationship between Service Quality and Customer Satisfaction—A Factor Specific Approach. Journal of Services Marketing 16: 363–79. [ Google Scholar ] [ CrossRef ] [ Green Version ]
- Tajeddini, Kayhan. 2010. Effect of Customer Orientation and Entrepreneurial Orientation on Innovativeness: Evidence from the Hotel Industry in Switzerland. Tourism Management 31: 221–31. [ Google Scholar ] [ CrossRef ]
- Tajeddini, Kayhan. 2011. Customer Orientation, Learning Orientation, and New Service Development: An Empirical Investigation of the Swiss Hotel Industry. Journal of Hospitality & Tourism Research 35: 437–68. [ Google Scholar ] [ CrossRef ]
- Tajeddini, Kayhan, and Myfanwy Trueman. 2012. Managing Swiss Hospitality: How Cultural Antecedents of Innovation and Customer-Oriented Value Systems Can Influence Performance in the Hotel Industry. International Journal of Hospitality Management 31: 1119–29. [ Google Scholar ] [ CrossRef ]
- van Eck, Nees Jan, and Ludo Waltman. 2020. VOSviewer Manual. 2020. Available online: http://www.vosviewer.com/documentation/Manual_VOSviewer_1.6.1.pdf (accessed on 20 August 2022).
- Vega-Vázquez, Manuela, Francisco José Cossío-Silva, and María Ángeles Revilla-Camacho. 2016. Entrepreneurial Orientation-Hotel Performance: Has Market Orientation Anything to Say? Journal of Business Research 69: 5089–94. [ Google Scholar ] [ CrossRef ]
- Wang, Cheng-Hua, Kuan-Yu Chen, and Shiu-Chun Chen. 2012. Total Quality Management, Market Orientation and Hotel Performance: The Moderating Effects of External Environmental Factors. International Journal of Hospitality Management 31: 119–29. [ Google Scholar ] [ CrossRef ]
- Webster, Frederick E., Jr. 1988. The Rediscovery of the Marketing Concept. Business Horizons 31: 29–39. [ Google Scholar ] [ CrossRef ]
- Williams, Paul, and Earl Naumann. 2011. Customer Satisfaction and Business Performance: A Firm-Level Analysis. Journal of Services Marketing 25: 20–32. [ Google Scholar ] [ CrossRef ]
- World Health Organization. 2020. COVID-19 Management in Hotels and Other Entities of the Accommodation Sector . Geneva: World Health Organization. [ Google Scholar ]
- World Trade Organization. 2021. Press Release/876—Trade Statistics and Outlook. Available online: https://www.wto.org/english/news_e/pres21_e/pr876_e.pdf (accessed on 20 August 2022).
- World Travel and Tourism Council. 2020. Recovery Scenarios 2020 & Economic Impact from COVID-19. Available online: https://wttc.org/Research/Economic-Impact/Recovery-Scenarios-2020-Economic-Impact-from-COVID-19 (accessed on 20 August 2022).
- Zhou, Kevin Zheng, James R. Brown, and Chekitan S. Dev. 2009. Market Orientation, Competitive Advantage, and Performance: A Demand-Based Perspective. Journal of Business Research 62: 1063–70. [ Google Scholar ] [ CrossRef ] [ Green Version ]
- Zupic, Ivan, and Tomaž Čater. 2015. Bibliometric Methods in Management and Organization. Organizational Research Methods 18: 429–72. [ Google Scholar ] [ CrossRef ]
Click here to enlarge figure
Sources | Articles |
---|---|
International Journal of Hospitality Management | 27 |
International Journal of Contemporary Hospitality Management | 16 |
Journal of Business Research | 6 |
Journal of Hospitality and Tourism Management | 5 |
Services Industries Journal | 4 |
European Journal of Marketing | 3 |
Journal of Hospitality & Tourism Research | 3 |
Service Business | 3 |
Cornell Hospitality Quarterly | 2 |
Current Issues in Tourism | 2 |
Nr. | Document | Short Description |
---|---|---|
1 | ( ) | Examines the relationship between market orientation and performance in hotel companies and its association with measures of service quality, customer satisfaction, and employee satisfaction. |
2 | ( ) | Provides an introduction to the Partial Least Squares (PLS) approach for structural equation modelling and contrasts it with the covariance-based approach. Provides further insights about the conditions in which the PLS technique is appropriate. |
3 | ( ) | Evaluates the organizing framework that clarifies the nature of competitive advantage and uses it to distinguish the sources of advantage from their consequences for relative competitive position and performance superiority. |
4 | ( ) | Examines the impact of culture, customer orientation (market orientation), and innovativeness on business performances, further proposing a construct to measure market orientation, labelled as “customer orientation”, based on a cultural perspective. |
5 | ( ) | Examines three main questions: “Why are some organizations more market-oriented than others?”, “What effect does a market orientation have on employees and business performance?”, and “Does the linkage between a market orientation and business performance depend on the environmental context?”. Uses the Kohli and Jaworski ( ) framework and proposes a new market orientation measurement scale. |
6 | ( ) | Conducts a meta-analysis on market orientation literature which aggregates the empirical findings from the research stream. |
7 | ( ) | Synthesizes the extant knowledge about market orientation, clarifies the construct’s domain, and provides the foundation for future research about market orientation. |
8 | ( ) | Departs from previous studies (i.e., ; ), defines market orientation, and develops the MARKOR measurement scale. |
9 | ( ) | The authors provide a report on the development of a measure of market orientation (MKTOR scale) and assesses its effect on business’ profitability. |
10 | ( ) | Delivers a summary of simple and multiple mediation and explores approaches that can be used to investigate indirect mediator effects, as well as methods for contrasting two or more mediators within a single model. |
11 | ( ) | Examines the variation in market orientation across strategic business units, the relationship between market orientation and the business unit’s organizational systems, and the relationship between market orientation, individual job attitudes, and business unit performance. |
12 | ( ) | Studies the impact of market orientation on business performance in the Norwegian hotel industry, testing if product innovativeness acts as mediator in the relationship between market orientation and business performance. |
13 | ( ) | Assesses the implementation of market orientation in the United Kingdom (UK) hotel industry. Finds that many organizations need to achieve a higher degree of market orientation and to focus on competitors. |
14 | ( ) | Investigates the link between market orientation and business performance in the hotel industry. Finds a positive relationship between market orientation and marketing performance, as well as with financial performance. |
15 | ( ) | Evaluates how a competitive environment affects the strength of the relationship between market orientation and business performance and if it produces a greater emphasis on customer analysis relative to competitor analysis, or vice versa, considering a given magnitude of market orientation. |
16 | ( ) | This study incorporates factors such as market and technological turbulence, and competitive intensity in a model to examine how total quality management (TQM) and market orientation affect hotel performance and if market orientation mediates relationship between TQM and hotel performance. |
17 | ( ) | Evaluates how customer value affects a firm’s market orientation, competitive advantage, and organizational performance in the hotel industry. |
Nr. | Document | Short Description |
---|---|---|
1 | ( ) | Provides guidelines for the use of structural equation models (SEM) for theory testing and development. |
2 | ( ) | Examines methods for nonresponse bias estimation, predictions of the direction of nonresponse bias, and estimation of the magnitude of the bias. |
3 | ( ) | Provides guidelines and standards to use and an interpretation of SEM by researchers. |
4 | ( ) | Focuses on the study of the relationship between learning orientation, market orientation, and organizational performance. |
5 | ( ) | Examines the relationship between firm resources and sustained competitive advantage. |
6 | ( ) | Provides a set of guidelines, namely analytics procedures on how to evaluate moderator and mediator effects, separately and in wider models including moderators and mediators. |
7 | ( ) | Assesses what are the most effective strategic responses to environmental hostility among small manufacturing firms and shows that business performance in hostile environments is positively correlated with companies’ organic structure, entrepreneurial strategic posture based in a long-term orientation, high product prices, and a concern for predicting industry trends. |
8 | ( ) | Focuses on the study of the different types of mental models or representations of competitive advantages according to the emphasis on customer or management judgements about where and how competitors differ. |
9 | ( ) | Examines the statistical tests used in the analysis of SEM with unobservable variables and measurement errors and develops a system based on measures of shared variance within the structural model, measurement model, and overall models. |
10 | ( ) | Examines the adequacy of the conventional rule-of-thumb cut-off criteria for various fit indexes used to evaluate model fit. |
11 | ( ) | Based on the resource-based view of the firm, the authors suggest that market orientation, entrepreneurship, innovativeness, and organizational learning contribute to the creation of positional advantages for some firms. |
12 | ( ) | Evaluates the link between entrepreneurial orientation organization structure and performance in the context of the Asian hotel industry. |
13 | ( ) | Evaluates the nature of the entrepreneurial orientation (EO) construct and proposes a contingency framework to investigate the relationship between EO and firm performance. |
14 | ( ) | Assesses how proactiveness and competitive approaches are related to each other, how they are linked to business performance, and if they differ according to the environment in which companies settle these approaches in strategy-making. |
15 | ( ) | Provides a comprehensive text and guidelines to help researchers in measurement, particularly to be used in graduate courses in psychology, education, and areas of business such as management and marketing. |
16 | ( ) | Discusses the problems related with self-report questionnaires, particularly common method variance, the consistency motif, and social desirability, further providing guidance for post hoc remedies and further procedural methods for dealing with artefactual bias. |
17 | ( ) | Examines the extent to which method bias influences behavioural research results and evaluates several procedural and statistic methods to control method bias, proposing statistical remedies for different types of research settings. |
18 | ( ) | Focuses on the process in which organizations develop and use new knowledge, further providing a set of organizational elements, including the learning organization to improve performance. |
Nr. | Document | Short Description |
---|---|---|
1 | ( ) | Evaluates how hospitality companies can promote incremental and radical innovation using human resource management practices such as selection and training. |
2 | ( ) | Assesses the relationship between customer orientation, innovation, and business performance in hotel companies. |
3 | ( ) | The study presents a framework for the chain relationship market orientation–innovation–performance. |
4 | ( ) | Settles an analysis of research contributions, specifically, innovation in the tourism industry and its implications for destinations and national economies. |
5 | ( ) | Evaluates why some firms are more innovative than others, what effect innovativeness has on business performance, and if the link between innovativeness and business performance depends on the environmental context, proposing that market orientation, entrepreneurial orientation, and learning orientation are key antecedents to innovativeness, as well as a direct relationship between innovativeness and business performance. |
6 | ( ) | The study presents a conceptual framework for incorporating innovation in market orientation research. |
7 | ( ) | Explores the influence of firms and market characteristics on radical and incremental innovations. |
8 | ( ) | Evaluates the effect of hotel innovations on firm value. The authors examine this effect through market value, distinguishing the potentially different impacts of distinct innovation types: product, process, organization, and marketing. |
9 | ( ) | Settles empirical evidence on technological activity in the Spanish service sector, specifically, the tourist accommodation in the Balearics Islands (Spain). |
10 | ( ) | Develops and tests models of innovation behaviour in the hotel industry, specifically, relating four types of innovation, namely: management, external communication, service scope, and back-office to key determinants such as service provider characteristics, customer competences, and the market drivers. |
11 | ( ) | Seeks to identify factors that promote successful service innovations and examines the link between success and performance dimensions. |
12 | ( ) | Evaluates innovation in the hospitality industry to find success factors in hospitality firms, further investigating how success factors relate themselves with market performance, financial performance, and employee and customer relationship enhancement. |
13 | ( ) | Examines the relationship between customer orientation, entrepreneurship, and innovativeness with business performance in the hotel industry. |
14 | ( ) | Examines the influence of customer orientation and learning orientation in new service development and their subsequent effect on performance (financial and perceptual) in the hotel industry. |
15 | ( ) | Evaluates the relationship between corporate culture, indicators of innovativeness, and business performance in the Swiss hotel industry. |
MDPI stays neutral with regard to jurisdictional claims in published maps and institutional affiliations. |
Share and Cite
Sampaio, C.; Régio, M. Market Orientation and Hotel Industry: Literature Review and Implications for Periods of Market Turmoil. J. Risk Financial Manag. 2022 , 15 , 497. https://doi.org/10.3390/jrfm15110497
Sampaio C, Régio M. Market Orientation and Hotel Industry: Literature Review and Implications for Periods of Market Turmoil. Journal of Risk and Financial Management . 2022; 15(11):497. https://doi.org/10.3390/jrfm15110497
Sampaio, Carlos, and Mónica Régio. 2022. "Market Orientation and Hotel Industry: Literature Review and Implications for Periods of Market Turmoil" Journal of Risk and Financial Management 15, no. 11: 497. https://doi.org/10.3390/jrfm15110497
Article Metrics
Article access statistics, further information, mdpi initiatives, follow mdpi.
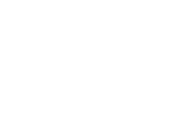
Subscribe to receive issue release notifications and newsletters from MDPI journals
Reference management. Clean and simple.
What is a literature review? [with examples]
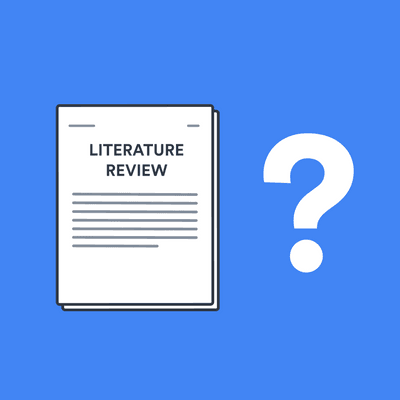
What is a literature review?
The purpose of a literature review, how to write a literature review, the format of a literature review, general formatting rules, the length of a literature review, literature review examples, frequently asked questions about literature reviews, related articles.
A literature review is an assessment of the sources in a chosen topic of research.
In a literature review, you’re expected to report on the existing scholarly conversation, without adding new contributions.
If you are currently writing one, you've come to the right place. In the following paragraphs, we will explain:
- the objective of a literature review
- how to write a literature review
- the basic format of a literature review
Tip: It’s not always mandatory to add a literature review in a paper. Theses and dissertations often include them, whereas research papers may not. Make sure to consult with your instructor for exact requirements.
The four main objectives of a literature review are:
- Studying the references of your research area
- Summarizing the main arguments
- Identifying current gaps, stances, and issues
- Presenting all of the above in a text
Ultimately, the main goal of a literature review is to provide the researcher with sufficient knowledge about the topic in question so that they can eventually make an intervention.
The format of a literature review is fairly standard. It includes an:
- introduction that briefly introduces the main topic
- body that includes the main discussion of the key arguments
- conclusion that highlights the gaps and issues of the literature
➡️ Take a look at our guide on how to write a literature review to learn more about how to structure a literature review.
First of all, a literature review should have its own labeled section. You should indicate clearly in the table of contents where the literature can be found, and you should label this section as “Literature Review.”
➡️ For more information on writing a thesis, visit our guide on how to structure a thesis .
There is no set amount of words for a literature review, so the length depends on the research. If you are working with a large amount of sources, it will be long. If your paper does not depend entirely on references, it will be short.
Take a look at these three theses featuring great literature reviews:
- School-Based Speech-Language Pathologist's Perceptions of Sensory Food Aversions in Children [ PDF , see page 20]
- Who's Writing What We Read: Authorship in Criminological Research [ PDF , see page 4]
- A Phenomenological Study of the Lived Experience of Online Instructors of Theological Reflection at Christian Institutions Accredited by the Association of Theological Schools [ PDF , see page 56]
Literature reviews are most commonly found in theses and dissertations. However, you find them in research papers as well.
There is no set amount of words for a literature review, so the length depends on the research. If you are working with a large amount of sources, then it will be long. If your paper does not depend entirely on references, then it will be short.
No. A literature review should have its own independent section. You should indicate clearly in the table of contents where the literature review can be found, and label this section as “Literature Review.”
The main goal of a literature review is to provide the researcher with sufficient knowledge about the topic in question so that they can eventually make an intervention.
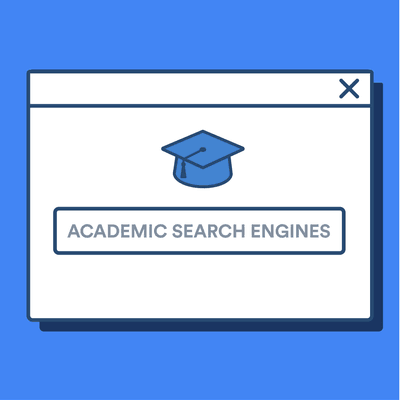

Use of Market Research Panels in Transit (2013)
Chapter: chapter two - literature review.
Below is the uncorrected machine-read text of this chapter, intended to provide our own search engines and external engines with highly rich, chapter-representative searchable text of each book. Because it is UNCORRECTED material, please consider the following text as a useful but insufficient proxy for the authoritative book pages.
8 accommodate new technologies, such as telephone sampling and computerization. Since Frankel and Frankel wrote their article in 1987, the Internet has become another technologi- cal advance, requiring innovations in sampling to accommo- date the technology. The Brick article notes that 10 years ago, no generally accepted method of sampling from the Internet had been established, and that as of the writing of the article in 2011, it was still the case. As developed by Neyman in 1934, probability survey sam- pling became the basis for virtually all sampling theory, with a very specific framework for making inferences. The frame- work assumes that all units in the population can be identified, that every unit has a probability of being selected, and that the probability can be computed for each unit. Once the population is selected, the framework assumes that all char- acteristics can be accurately measured. Non-response fac- tors and the inability to include portions of the population (coverage error) violate the pure assumptions of probability sampling. A variety of techniques has been developed to adjust for non-response and coverage error, such as model- based and design-based sampling methods. Technological advances that have changed sampling meth- ods include: the introduction of telephone surveying, which eventually replaced face-to-face interviews as the primary mode of household surveying; the shift from landline tele- phones to cell phones; and the advent of the Internet. Each of these not only affected methods of sampling, it is intertwined with the others, with changes in one leading to new develop- ments in the others. New Concerns with Traditional Quantitative Research The science of traditional quantitative market research rests on two fundamental assumptions: (1) the people who are surveyed approximate a random probability sample; and (2) the people who are surveyed are able and willing to answer the questions they are asked (Poynter 2010). In addition to these concerns with the theoretical underpinnings of market research, operational concerns are also putting pressure on traditional methodologies. Random Probability Sample The random probability sample is an essential ingredient of traditional market research. Without it, quantitative market This literature review consists of four sections: ⢠The first section provides a brief history of survey sam- pling and the theoretical basis for market research anal- ysis, providing context for what became the standard procedures and expectations of market research. This is followed by an overview of some of the issues facing market research today, and how they are impacting the statistical underpinning of market analysis. ⢠The second section introduces traditional panel surveys and panel survey techniques. A summary of a typical tra- ditional case example, the Puget Sound Transportation Panel Survey, is provided in Appendix A. ⢠The third section introduces relatively newer concepts of online panel research, with definitions particular to online panel surveys and techniques, issues with online panel research, and the special concerns of market research in the public sector. ⢠The fourth section circles back to the concerns raised in the first section, and looks at what lies ahead for the market research industry on these issues. MARKET RESEARCH CONTEXT A Brief History of Survey Sampling In a 2011 special edition of the Public Opinion Quarterly, Brick provides an article on the future of survey sampling (Brick 2011). For the purposes of the article, survey sampling is defined as the methodology for identifying a set of obser- vations from a population and making inferences about the population from those observations. Prior to 1934, full enu- meration was considered necessary to understanding char- acteristics of a population; everyone needed to be contacted. Neyman, in his 1934 article âOn the Two Different Aspects of the Representative Method: The Method of Stratified Sample and the Method of Purposive Selection,â planted the seeds that resulted in the overthrow of full enumeration and established the paradigm of survey sampling. The move to telephone surveying in the mid-20th century was another significant change in survey sampling methods. The pressures for timely and cost-efficient estimates were stimulants for change then, and are even more relevant today. The article by Brick draws from a 1987 article by Frankel and Frankel, âFifty Years of Survey Sampling in the United States.â In the 1987 article, sampling is described as having two phases: (1) the establishment of the basic methods of prob- ability sampling; and (2) innovations in the basic methods to chapter two LITERATURE REVIEW
9 research studies and analysis cannot be conducted. It is this underpinning of sampling theory that allows the calculation of sampling and expressing confidence in the results, such as results being ±3% with a confidence interval of 95%. As tele- phones became standard in every household, random-digit- dial techniques for landline telephones became the foundation of probabilistic sampling, with a solid theoretical basis. Results for a survey conducted between January and June 2011 by the National Center for Health Statistics found that 31.6% of American homes had only cell phones, and that an additional 16.4% of homes received all or almost all calls on wireless telephones despite also having a landline telephone (Blumbery 2011). Expert disagree, but it has been suggested that if more than 30% of the population has a 0% chance of being selected, then a random probabilistic sample cannot be selected (Brick 2011). The implication is profoundâthat with the incidence of landline phones declining, random-digit-dial telephone survey- ing, the mainstay of traditional quantitative market research, no longer provides a probabilistic sample. Cell phones are considered unsuitable for random-digit-dialing, for a variety of reasons, including the possibility of respondents having more than one cell phone resulting in duplication within the sample; respondent resistance; and legislation that prohibits the practice. Online recruitment is fast and economical, but does not provide a probabilistic sample, as is discussed later in this chapter. Willingness and Ability to Answer Research Questions Market researchers started out assuming that people could answer direct questions about their attitudes and behavior. Early on, it became clear that these questions were difficult to answer, so psychometrics and marketing science method- ologies were developed to facilitate responses and analysis of results. More recently even these techniques have been chal- lenged, as the industry realizes that respondents are unreliable witnesses about themselves. Operational Issues Other problems with the traditional market research process are operational. It is perceived as slow and costly, and increas- ingly, organizations are relying on techniques that may be providing quick results at the expense of quality. The cost of traditional survey research often is driven up by the decline in use of landline telephones, making it more difficult to obtain a traditional random probabilistic sample. In addition, more people have answering machines to screen calls or otherwise refuse to participate, again making it difficult to achieve a suf- ficient sample without additional time and expense. If cost is arguably the single most important factor in the search for new survey techniques, the Internet offers a potential solution, even though it doesnât provide a probabilistic sample. This shift from probability sampling to non-probability sam- pling is a paradigm change of the magnitude of the shift from enumeration to probability sampling theory in 1934âwhich, Brick notes in his article, was spurred by the cost of enumera- tion. Today researchers find themselves in a similar situation, driven by rising costs away from probabilistic sampling toward non-probabilistic sampling. The issues raised here may fundamentally change the way all market research is conducted. How the market research indus- try is responding and what may lie ahead is discussed in the last section of this chapter, âThe Future of Market Research.â TRADITIONAL MARKET RESEARCH PANELS Panel surveys have been conducted for many years, and have been used in the transportation industry for topics such as travel behavior changes and tracking customer satisfaction. The concepts discussed in this section are applicable to both traditional and online panel research. Definition of a Traditional Panel The meaning of a market research panel depends on the con- text, industry, and time period in which the term is being used. The AMA acknowledges this with the following distinctions: ⢠True panel: A sample of respondents who are measured repeatedly over time with respect to the same variables. ⢠Omnibus panel: A sample of respondents who are mea sured repeatedly over time but on variables that change from measurement to measurement [http:// www.marketing power.com/_layouts/Dictionary. aspx?dLetter=P (accessed Mar. 11, 2012)]. Traditional Panel Survey Techniques Traditional panel members were recruited through probabilis- tic sampling techniques so that survey results could be extra p- olated to the general population. Developing and maintaining a panel was an expensive proposition, made more difficult by the challenge of keeping track of people and households as they moved and changed phone numbers. Panel survey research was typically used to determine individual travel behavior changes over time, such as to understand the relation- ship between changes in household characteristics and choice of travel mode. Another use was for âbefore and afterâ studies to measure impacts of a change in policy or service; for exam- ple, adding a new light rail line or carpool lanes. These studies were often conducted by a MPO for the purpose of developing regional travel demand and forecasting models. Panels were rarely set up and maintained for the purpose of ad hoc, on-call market research (omnibus panels). Panel data collection is described as âa survey of a group of preselected respondents who agree to be panel members on a continuous basis for a given period of time and provide demographic data, allowing selection of special groups and
10 cally, each wave consists of the same core questions along with some new questions. In a travel behavior survey, the panel provides information on how the travel behavior of each participant evolves in response to changes in the travel environment, household background, or other factors. Rotating or revolving panel surveys are a combination of repeated and cross-sectional designs, in that they collect panel data on the same sample for a specified number of waves, after which portions of the panel are dropped and replaced with comparable members. The strength of this design is its abil- ity to allow for both short-term panel member analysis and long-term analysis of population and subgroup change. Like repeated cross-sectional designs, rotating panels periodically draw new members from the current population, obtaining similar measurements on them. Benefits of Panel Designs The most important benefit of a panel survey is that it directly measures changes at the individual level and can provide repeated measurements over time. This rich source of infor- mation on personal and household behavior is essential for determining causal relationships between travel behavior and the factors that influence personal travel decisions and developing predictive models for personal travel behavior. This same benefit applies to the ability to measure and under- stand trends in population behavior. Panel studies can be especially useful for before-and-after surveys that measure the impacts of transportation policy and service changes on travel behavior, rider attitudes, and safety. For example, a before-and-after study of the implementation of a new rail line (replacing existing bus service) shows that a shift in mode split occurred after the implementation of the new line. Results using a cross-sectional survey showed a shift from auto to train after opening of the rail line suggesting overall growth in transit use shifting car drivers to rail riders. A panel study measuring individual specific changes captured a shift from bus to car in addition to the shift from car to rail. This finding fundamentally changed the implications of the cross- sectional study: the new service attracted former car drivers, but also shifted former bus riders into cars. Additional benefits of the panel approach include statisti- cal efficiency (it requires a smaller sample size); lower cost (it requires fewer surveys); and speed (easy access to the panel allows faster survey implementation than when a fresh sample must be obtained). Limitations with Panel Designs Three primary limitations of panel surveys are identified: panel attrition, time-in-sample effects, and seam effects. 1. Panel attrition refers to panel member non-response in later waves of data collection. The Puget Sound Trans- portation Panel conducted its first wave of surveys in permitting the use of surveys to monitor responses over timeâ (Elmore-Yalch 1998). This maximizes the use of a sample in that the sampling need be done only once, after which the panel is accessible for future research efforts. Panel member attrition and replacement is an element of maintaining the panel, and is discussed elsewhere in this chapter. The remainder of this section is a summary of An Introduc- tion to Panel Surveys in Transportation Studies (Tourangeau et al. 1997), which provides a solid overview of the basics of traditional panel survey research, especially as applied to travel behavior studies. The report has a four-fold purpose for the development and implementation of travel behavior studies: (1) to highlight the differences between cross-sectional and panel surveys; (2) to discuss the limitations of both cross-sectional and panel sur- veys; (3) to identify situations where panel surveys are the preferred method; and (4) to provide guidelines for design- ing a panel survey and maintaining the panel. A panel survey approach is recommended when the purpose of the survey is to develop travel demand models and forecast future demand; to measure and understand trends in behavior; to assess the impact of a change in transportation policies or services; or to collect timely information on emerging travel issues. Definition of Cross-Sectional and Panel Designs There are two broad types of surveys, cross-sectional and panel surveys. A cross-sectional survey uses a fresh sample each time, whereas a panel survey samples the same persons (or households) over time. In addition, the questions may be the same or change with each survey. This creates four basic approaches to travel behavior surveys. One-time cross-sectional surveys provide a âsnapshotâ of travel behavior at a particular point in time, and show how behavior differs among members of the population, but pro- vide no direct information on how it changes over time. This type of survey makes no attempt to replicate conditions or questions from previous studies, and as a result is not well suited for assessing trends in population behavior. Repeated cross-sectional surveys measure travel behav- ior by repeating the same survey on two or more occasions. In addition to repeating the questions, the sampling is conducted in a similar manner to allow comparisons between or among separate survey efforts. Repeated cross-sectional surveys are sometimes referred to as a âlongitudinal survey designâ because they measure variations in the population over time. A more restrictive definition of a longitudinal survey design is where survey questions are repeated with the same sample over time. Longitudinal panel designs collect information on the same set of variables from the same sample members at two or more points in time. Each time the panel is surveyed, it provides what is called a âwaveâ of data collection. Typi-
11 1989. The fourth round of surveying in 1993 had a par- ticipation rate from the original panel member of about 55%, meaning 45% of the panel had left and needed to be replaced. 2. The time-in-sample effect refers to reporting errors or bias as a result of participants remaining in the panel over time. This is also called condition, rotation bias, or panel fatigue; and generally refers to respondents reporting fewer trips or fewer purchases in later rounds of a panel survey than in earlier ones. 3. Seam effects are another type of reporting error and refer to reporting changes at the beginning or ending of the interval between rounds rather than in other times covered by the interview. Design Issues in Conducting a Panel Survey There are four design issues that need to be considered in conducting a panel survey: definition of the sample unit; the number and spacing of rounds; method of data collection; and sample size. 1. Most traditional travel surveys conducted by MPOs use households as the sampling unit; however, sampling individuals is another option. When a household is the sampling unit, the panel survey sample can become com- plicated as household members are born, die, divorce, or mature and move out. For travel surveys, the report sug- gests using the household as the sampling unit, follow- ing initial respondents to new households, and adding any additional household members to the panel. 2. The number and spacing of survey rounds depends on factors such as the rate of changes in travel behavior and the need for up-to-date information. If changes in travel behavior are the result of external factors, such as rap- idly increasing gas prices, or if administrative reporting requires monthly or quarterly updates, this may shorten the intervals between survey waves. Panel travel surveys are collected at six-month or annual intervals, balancing the potential for respondent burden with the desire for regular data collection. The report recommends annual data collection for travel behavior studies. 3. Data collection methods differ in terms of cost, cover- age of the population, response rates, and data quality (inconsistent or missing data). In-person data collec- tion is typically the most expensive, but produces the highest percentage of coverage, highest response rates, and potentially most accurate data, as the interviewer can assist the respondent. Telephone data collection tends to be the next most expensive methodology, and eliminates the population without a telephone. This used to be limited since almost all households had a landline phone, but since the report was written the per- centage of mobile phone-only households has grown significantly. Data collection by mail is the cheapest of the three traditional modes, but has the lowest response rates and poorest data quality. [Since the report was written, Internet surveying has become another inex- pensive alternative method of data collection. Online surveying is covered in other portions of the literature review.] The report recommends using the telephone for data collection in the first wave of a travel behavior panel study and considering less expensive methods for successive waves, if necessary. 4. Selecting the sample size requires specifying the desired level of precision for the survey estimates. The preci- sion level is determined by the requirements for ana- lyzing the goals and objectives of the survey, typically rates of change in travel behavior at the household or sub-regional level. After the level of precision is deter- mined, traditional statistical formulas can be applied to determine the sample size, which is then adjusted for anticipated non-response, attrition, and eligibility rates. Issues with Maintaining the Panel The report points up three issues that need to be considered in terms of maintaining a panel: freshening the sample, main- taining high response rates across waves, and modifying the questionnaires across rounds. 1. âFreshening the sampleâ is the process of adding new panel members over time to ensure that the sample accurately reflects changes in the population from newly formed households or those who have recently moved to the study area. The longer the panel is con- tinued, the less likely it is to represent the study area. The report suggests that, if a panel continues for more than five years or there is significant in-migration to the study area, a supplemental sample be implemented. Another reason for freshening the sample is to off- set attrition, recruiting new panel members compa- rable to those who drop out and thereby maintaining the panel make-up and sample size for the duration of the panel effort. The report suggests that the initial sample size be large enough to accommodate antici- pated attrition in later waves, and that steps are taken to minimize attrition. Replacement of panel members should only be done as a last resort. 2. There are three techniques for maintaining high response rates: tracing people or households who move; main- taining contact with panel members between rounds; and providing incentives for participation. Methods of tracing panel members who move include mailing a let- ter several months in advance of the next wave request- ing updated contact information; and asking the post office to provide new addresses rather than forwarding the mail, to ensure that the contact files get updated. If new contact information is not provided, researchers may attempt a manual search through existing databases. The report suggests that a protocol be developed at the outset of the survey effort to track respondents between waves and reduce attrition. Another way of reducing attrition is to maintain respondent interest and contact information between
12 waves by sending postcards, holiday greetings, and sur- vey results. Incentives such as small amounts of cash can also be helpful. Cross-sectional surveys have shown that a small prepaid incentive (for example, a $2 bill) is effective in increasing participation rates and reducing attrition. Unfortunately, there was limited research at the time as to the effect of incentives on panel surveys over time. It is noted that non-respondents in one wave may still participate in the next, so that only those who refuse to respond to more than one round of the study would be dropped from the panel. 3. A defining element of a traditional panel survey is the ability to administer the same questions to panel mem- bers over time, which is what provides the direct mea- surement of change that is so valuable to travel behavior studies. Two situations arise that may make it neces- sary to modify the questionnaire across waves. First, a new issue may arise that can be advantageously posed to the panel. This then becomes a cross-sectional sur- vey, where the data are collected once. If the question is repeated in later waves, it becomes part of the panel effort. Although this is easy, fast, and less expensive than conducting a separate study, it can add to respon- dent fatigue by making the questionnaire longer. For this reason, it is suggested that new questions be kept to a minimum. The second reason for changing a question that there is a problem with the question itself (e.g., it is poorly worded, yields unreliable results, or becomes irrelevant). In this instance, it is important to revise the question as soon as possible. The report recommends that a calibration study be done to determine the effect of any core changes. Weighting the Panel Data The final section of the report deals with how to weight panel survey data. Weighting is done to account for differences in the probability of being selected, to compensate for differences in response rates across subgroups, and to adjust for random or systematic departures from the composition of the popula- tion. Weighting is done at two points: after the initial wave, following the procedures for standard cross-sectional surveys; and then after each wave to account for changes in the panel membership. Although weighting is fairly straightforward for the first wave, subsequent waves can be complicated if the sampling unit is a household, as is typical of travel behav- ior panel studies. Elements that must be taken into account are how to treat households who add or lose members over the course of the panel; and how to define a ârespondingâ or ânon-respondingâ household, for example, whether all survey waves are completed by all household members or only cer- tain household members. It is sometimes necessary to gener- ate different weights for different survey analyses. Detailed guidelines for developing panel survey weights are provided in the report appendices. ONLINE MARKET RESEARCH PANELS This section will discuss the types of online panels, sampling strategies, and issues and concerns with using the Internet for market research purposes. The current literature reviewed in this synthesis discusses sampling and recruitment for online panels using the Internet, e-mail, or other new technologies, such as quick response (QR) codes scanned by a smart phone. Multi-frame sampling, where a mix of sampling techniques is used for developing the panel, poses additional issues which are only now being explored and disseminated within the market research industry. Because this is an emerging area of research, this literature review does not include multi-frame sampling. Types of Online Panels Three types of panels are discussed by Poynter in his 2010 book, The Handbook of Online and Social Media Research: Tools and Techniques for Market Researchers. The first is a traditional panel, typically called a client panel or in-house panel, developed to meet specific criteria and recruited either in-house by the agency or through the assistance of a vendor. The panel can be recruited through a variety of techniques, including telephone; in-person intercepts (on a vehicle, or on the street); through existing agency customer databases; or online, through the agency website or pop-up invitations to join the panel. The critical elements of this type of panel are the definition and control that is exercised by the agency, and the intention for the agency to maintain the panel over time. An online access panel, also referred to as an access panel or online panel, is developed by independent market research firms and can provide samples for most markets that have a significant volume of research activity. The researcher provides the panel company with the desired sample speci- fication, and then either the researcher provides a link to the online survey, or the panel company scripts and hosts the online survey. The third type of panel survey is an online research com- munity, also known as a market research online community or MROC, which combines attributes of panel research with elements of a social media community. Although it is sometimes grouped with social media techniques, the online research community has been included here because it meets the definition of âa group of persons selected for the purpose of collecting data for the analysis of some aspect of the group or area.â In-House Panels As the name implies, in-house panels are owned by the research department of the agency, and are not purchased from a vendorâs existing panel. The in-house panel is used
13 for market research, not public relations, marketing or sales; and panel members are aware that they will be contacted for research, insight, and advice. The primary advantages of in-house panels are cost savings, speed of feedback, and control over the panel. Disadvantages include the workload required to manage a panel and that the possibility that panel members may become sensitized to the research approaches. In-house panels can be conducted simply from a list of people and an off-the-shelf survey program using e-mail and a way to unsubscribe from the panel. For small-budget projects or a low-key exploratory concept, a simple approach may be the most appropriate. More sophisticated panel management may require methods to prevent people from signing up mul- tiple times, the ability to draw sub-samples, protocols for han- dling and managing incentives, panel member replacement strategies, quotas on survey responses, online focus groups or bulletin board groups, and rules for creating an online panel community. The more sophisticated the approach, the more advanta- geous it is to contract with a vendor to run the panel. Using internal staff may make the research more responsive to man- agement needs while saving in consultant fees. A vendor, how- ever, can handle more work without overburdening agency staff, using employees familiar with the latest thinking and best practices. These different strengths often lead to a strong partnership between the vendor and staff. Traditionally, panel research was done with standard ques- tionnaires, implemented by means of mail or telephone. New developments in technology and the Internet have made it easy to expand the activities of a panel even further, creating online focus groups, photo projects where panel members take pictures with their cell phones and upload them to an agency website, brainstorming through collaborative systems such as âwikiâ sites, and quick âfun pollsâ that encourage participa- tion, generate panel engagement, and provide almost instant answers to questions of the moment. Tips for using an in-house panel include: 1. Manage the expectations of panel members by letting them know at the outset how many surveys/activities they should expect. 2. Let panel members know you value their participation and that they are making a difference. 3. Recognize that panels will usually be skewed toward members who are knowledgeable about the product or service, and that they may not represent the opinion of the general public. 4. Complement conventional incentives (such as cash) with intrinsic rewards, such as information about upcoming events or new products before it hits the general market. Online Access Panels Online access panels have fundamentally changed how mar- ket research is conducted. An online access panel âis a col- lection of potential respondents who have signed up with a vendor which provides people for market research surveys.â These respondents are aware that they are joining a market research panel, and that they will be receiving invitations to online surveys. The vendor keeps some information on the panel members so that it can draw samples, if requested, but does not share this information with the client. Panel mainte- nance, including the provision of incentives, is the vendorâs responsibility. In selecting a panel vendor, six factors need to be considered: 1. Does the vendor provide only the sample, or will it also host surveys? If so, can the brand image on the survey maintain the agencyâs brand, or does it become folded into the vendorâs survey branding? 2. What is the quality of the panel? Not all panels are created equal, and the results can vary based on the panel used. ESOMAR formulated â26 Questionsâ (later, â28 Questionsâ) for agencies to ask vendors in order to understand their procedures and the potential quality of the survey results. The questions can be found at: http://www.esomar.org/index.php/ 26-questions.html. 3. In looking at vendor costs, caution must be exercised to ensure that price quotes are on similar services so they can be correctly compared. 4. Make sure that the vendor has the capacity to complete the study, including any potential future waves of the study. It is common practice for panel survey vendors to outsource a portion of or even the entire project to another firm if they do not have the resources to com- plete it as scheduled. Outsourcing to another panel sur- vey firm can result in double-sampling people who are members of both panels. More importantly, because dif- ferent panels often have varying results, this can lead to confusion as to whether an apparent change is real or a reflection of the panel used. 5. The more data a vendor has on its panel members, the more closely a survey can be targeted to the appropriate respondents. This results in fewer respondents being screened out and a shorter survey with fewer necessary questions. 6. As with any service, it is helpful to have a supportive vendor who is willing to stay late if needed, help clean up errors, and respond quickly to issues and concerns. After selecting a vendor, it is essential to ensure a good working relationship. This can be facilitated by: ⢠Clarifying the quote for the project to make sure it includes all work needed;
14 ⢠Booking the fielding time for the job as soon as the ven- dor is selected so there is flexibility if dates need to be changed for holidays, computer maintenance, etc.; and ⢠Developing and agreeing on the timeline, including final- izing the sample specification, scripting the survey or sending the link to the survey, having a soft launch to test the survey, agreeing on the full implementation and end date, and specifying the frequency of communica- tion with the panel company, especially regarding prob- lems that may occur. Once the survey is in the field, it is important to monitor progress and report any issues immediately to the panel ven- dor, including problems reaching the target quotas for com- pleted surveys. The sooner action is taken, the easier it will be to rectify the issue. It is advisable to work closely with the vendor supplying the panel to take advantage of its experi- ence with data issues with long surveys and improving the survey experience. Online Research Communities Using social media to create online research communities or MROCs for research purposes is a relatively new field. Research communities have been offered by third-party ven- dors since about 2000, but did not become widely used until about 2006. Online research communities typically have a few hundred members, and straddle the divide between quantita- tive and qualitative research. The communities can be short- term, developed for one research question and then dissolved; or can be a long-term resource, allowing research on a wide variety of topics over a period of six months or more. The benefits of online research communities are that they provide access to the authentic voice of the customer; go beyond the numbers to provide qualitative discussion; provide quick turnaround at a low marginal cost because the sampling and recruitment is complete; and create an active dialogue with the customers, letting them feel they âmake a difference.â These communities can either be open to anyone who wishes to join (within the requirements of screening criteria, such as age or geographic location), or closed, in which case panel members are invited to participate. It is important to note that open communities tend to be more about involvement, advo- cacy, and transparency rather than insight and research. Incentives are important to maintaining a high level of par- ticipation for all types of research panels; however, several issues are to be considered when structuring an incentive pro- gram. (It should be noted that it is illegal for some public agen- cies to use incentives.) The argument for using incentives is that they represent a small payment for the time and contributions of the panel members, and may be necessary to obtain the level of engage- ment needed to make the community succeed. The type of incentive (cash versus intrinsic rewards) must also be con- sidered. A chance to win a transit pass or seeing the results immediately upon completing an instant poll are examples of incentives. Finally, the agency must decide how to allocate the incentives. Options include giving all members an incen- tive regardless of participation levels; giving members who participate in a specified time frame the incentive; offering a chance to win a prize; and awarding a prize to the âbestâ contribution in a specified time frame. Agencies should avoid starting with a high-value incentive, because lowering the incentive later will seem to panel members that the agency is taking away a benefit, resulting in a loss of participation. As with all research techniques, the online community can be developed and maintained either in-house or through a vendor. Online research communities require significant and continuous management. Even if the community is maintained by a vendor, significant input by staff is needed to ensure that the community is addressing issues of concern to the agency. The advantages of a having a research-only community are that it can be much smaller than broader-topic communities, and members may be more open if they know they will not be âsold toâ another interest. Opening the community up to other department managers may result in too many surveys and e-mails being sent to members, with research being pushed aside in favor of other topics. Likewise, it is important not to allow community members to usurp the purpose of the research community for their own agendas. Part of managing the community is monitoring and ending any member activ- ity that begins to create an agenda separate from that of the agency, even removing a panel member if necessary. The steps to and guidelines for setting up an online com- munity include determining: ⢠What type of community is best (short versus long term, open versus closed, and the number of members); ⢠The âlook and feelâ (i.e., makeup) of the community; ⢠Community tools; ⢠Methods of recruiting members; ⢠Terms and conditions (including intellectual prop- erty, member expectations, restricted activities, anti- community behavior, privacy and safety, incentive rules, eligibility, data protection and privacy), and the ability to change terms and conditions; ⢠Methods of moderating and managing communities (moderator function, community plan, dealing with neg- ativity, creating member engagement); and ⢠Requirements for finding and delivering insights. The rapid pace of change among social media makes it difficult to project how this type of research activity will be conducted in the future. Four considerations are identified in Poyntonâs book: 1. Market research organizations typically do not allow activities that would influence the outcome of the
15 research. Because interaction and relationships built between community members and the sponsoring com- munity agency may sensitize panel members to organi- zational issues, MROCs may be declared ânot research.â 2. Currently, online research communities are used for more qualitative work rather than large-scale quantita- tive work. The ability to expand online research to larger projects (e.g., international research) will increase this as a mainstream research tool. 3. Respondent fatigue may set in, resulting in a less engaged community. This may be especially true if panel mem- bers belong to more than one community. 4. Alternative (not research-based) methods may be more successful, such as having a very large community that can serve both marketing and research functions, or tap- ping into other existing communities to conduct research rather than establishing one specific to the organization. One of the primary concerns with online research commu- nities has been that the relationship with the organization may cause heightened brand awareness and affinity, and that this will lead to a positive bias in research results. However, Aus- tin notes in an article in Quirkâs Marketing Research Media (Austin 2012) that while engagement builds a relationship with the company, community members remain candid and critical despite their relationship with the brand. If anything, members became slightly more critical as their tenure length- ened, not less. The article recommends that in moving to a new research paradigm, organizations make two changes from the traditional research approach to take advantage of this finding: trade anonymity for transparency because transparency builds engagement; and trade distance for relationship because rela- tionship creates candor. Together, the community members âwork harder, they share more and they stay engaged in the research longer.â Online Panel Sampling Techniques A few online panels employ traditional random sampling tech- niques, such as random-digit-dialing, and then conduct the research online; but the majority of panels are recruited using a non-probability approach online, such as pop-up or web ban- ner ads. The AAPOR Report on Online Panels (Baker 2010) covers both types of panels. This review will cover probability and non-probability sampling techniques as they relate to panels; it also discusses âriver sampling,â although it is not a panel sampling technique, per se. Lastly, it provides an over- view of strategies for adjusting non-probability samples to represent a population. Probability sampling techniques for online survey research have been slow to be adopted, despite being around for more than 20 years. The recruitment is similar to voluntary, non- probabilistic samples, except that the initial contact is based on probabilistic sampling techniques such as random-digit- dialing, or other techniques for which the population is known. Computers may sometimes be provided to persons with no online access to remove bias that might exist from only includ- ing persons or households with Internet access. Once the sample is determined, panels are built and maintained in the same way, regardless of whether they are probability- or non- probability-based. A probability-based sample is more expen- sive to develop than a non-probabilistic sample. Consequently, systematic replacement or the replacement of panel members lost through attrition is also more costly. The benefit is that a panel can be built that represents the general population and allows analysis of results based on probability theory. Non-probability and volunteer online panel members are recruited through a variety of techniques, all of which involve self-selection. The invitations to join a panel can be delivered online (through pop-up or banner advertisements), in maga- zines, on television, or through any other medium where the target population is likely to see the advertisement. The recruit- ment entices respondents by offering an incentive, talking about the fun of taking surveys, or other proven techniques. A common practice in the industry for developing online panels is through co-registration agreements. An organization will compile e-mail lists of its website visitors and ask if they would like to receive offers from partner agencies. The e-mail list is then sold to a research panel company. Off-line recruit- ment strategies include purchasing an organizationâs cus- tomer contact database and asking participants in a telephone survey if they would like to become part of an online panel for future surveys. A technique used for both online and off-line recruitment is to ask existing panel members to refer their friends and relatives, sometimes offering a reward for each new panel member recruited. No two panels are recruited the same way, and the panel research companies carefully guard their methodologies for recruiting panel members. River sampling is an online technique that uses pop-up sur- veys, banner ads, or other methods to attract survey respon- dents when they are needed. In river sampling, the ad presents a survey invitation to site visitors and then directs or âdown- streamsâ them to another, unrelated website to complete the survey. (Using this analogy, a panel would be a pond or reser- voir sample.) Knowing on which websites to place the ads is critical to the success of river sampling. This technique is not related to developing a panel, although sometimes the respon- dent is invited to join a panel at the completion of the sur- vey. There is generally a reward of some kind for completing the survey, such as cash, online merchant gift cards, frequent flyer miles, etc. This type of sampling may be on the rise as researchers seek larger and more diverse sample pools, and to get respondents who are less frequently surveyed than those provided through online access panels. The AAPOR report provides an overview of strategies for adjusting self-selected (non-probability-based) online panels, and reviews complex weighting, quotas, benchmarking, and modeling methodologies for creating a more representative
16 sample. Complex weighting uses detailed information about the population to balance respondents so that they mirror the population. Quotas, which match key demographics of the respondents with the demographics of the target population, are the most common technique. Benchmarking keeps the sample specifications the same over multiple waves, under the assumption that any changes are the result of changes in the element being measured, regardless of whether the sample is representative of the population. Modeling refers to linking the benchmark results to the real world to model what a sur- vey score of X means in terms of actual outcomes. When applying statistical significance testing to the panel sample, it is important to recognize that the significance is not how representative it is of the population, but of the panel. âThe error statistics indicate how likely it is that another sample from the same panel will be different, which is a valid and rele- vant measure of reliabilityâ (Poynter, p. 74). It is not, however, an estimate of the population sampling error, as is commonly understood with traditional random (probabilistic) sampling. Response rates for online access panels have little impact on how representative the research is, but do provide a measure of the quality of the panel. Issues and Concerns with Online Panel Surveys: AAPOR Report on Online Panels Online surveys have grown rapidly because of the lower cost, faster turnaround time, and greater reliability in building tar- geted samples, at the same time that traditional survey research methods are plagued by increasing costs, higher non-response rates, and coverage concerns. The quality of online access panel survey data came into focus in 2006 when the VP of Proctor and Gambleâs Global Consumer Market Knowledge gave a presentation on the range of problems P&G had faced with online access panel reliability. It fielded a survey twice with the same panel, two weeks apart, with results that pointed to two different business conclusions. This focused the market research industryâs attention on the need to provide understand- ing, guidance, and research on the topic of online research. The traditional probabilistic sample, such as random-digit- dialing, is the underpinning of market research. Probabilistic samples are based on the probability of being selected out of a specified population (such as households within the city lim- its). Based on probability theory, the results can be projected to the population with a statistical level of certainty. Online panel surveys typically use non-probability samples, which are a significant departure from traditional methods. The AAPOR Report on Online Panels, produced by the AAPOR task force on opt-in online panels, is a seminal work on concerns and issues with online panel (i.e., non-probability sample) survey research. The scope was to âprovide key infor- mation and recommendations about whether and when opt-in panels might be best utilized and how best to judge their qualityâ (Baker 2010). Sampling Error, Coverage Error, and Non-Response Bias A sample is, by definition, a subset of a population. All sur- veys, regardless of sampling method, have some level of imprecision owing to variation in the sample. This is known as sampling error. A probabilistic sample is one where sam- pling theory provides the probability by which the member of the sample is selected from the total population. In traditional sampling methods, such as random-digit-dialing of house- holds within a geographic area, the total population of home telephone numbers is known. With address-based sampling, the total number of addresses in a specific area is known. Thus the total population is known and the probability of selecting any one phone number (or address) is known. This allows the data to be projected to the population as a whole. The difficulty with online sampling is that the population is unknown. Typically an e-mail address is used as the sampling unit (rather than a home telephone, as in the earlier example). The issues with e-mail addresses include duplication problems, in that one person may have more than one e-mail address; and clustering problems, where an e-mail address represents more than one person. As a result, online sampling differs from tra- ditional sampling in three significant ways: (1) the concept of a sampling frame is discarded and the focus is shifted to recruit- ing as large and diverse a group as possible; (2) instead of a representative sample of all households, a diverse group of persons with the attributes of interest for the panel is recruited; (3) the panel membership is rarely rotated, with panel mem- bers being retained as long as they keep completing surveys. Over time, this can lead to a very different panel membership than the initial profile of the panel. Coverage error occurs when persons, or groups of persons, have zero chance of being selected to participate in the sur- vey. Lack of access to the Internet creates significant cover- age bias. The AAPOR report includes data from 2008 stating that although 85% of the households in the continental United States have some level of Internet service, those without Inter- net access differ significantly from those who do. Those with- out access are more than twice as likely to be over the age of 65 as the general population. They are also more likely to be members of a minority group, to have incomes less than $25,000, to have a high school education (or less), to be unem- ployed, not to own a home, and to live in rural counties or the South Census Region. It can also be noted that having access to the Internet does not necessarily make for active users of the Internet. In 1970, household telephone coverage estimates of 88% led to the acceptability of using telephone surveys in place of in person interviewing. Coverage estimates of Inter- net usage are currently lower than 88%, indicating that it has not yet reached a level where it can be used to represent the general population. Commercial online access panels are even more problem- atic, in that a person has to have Internet access, receive an invitation to become a panel member, sign up for the panel,
17 and then participate in the surveys. Current estimates are that less than 5% of the population has signed up for an online panel, meaning that more than 95% of the population has a 0% chance of being selected. Non-response bias is when some of the persons in the sam- ple choose not to respond to the survey, or some of the ques- tions within the survey. Four stages of panel development are discussed, and how online panel survey development is affected by non-response bias: Stage 1: Recruitment of panel members. The previous discussion on coverage error points out issues with Internet access. In addition, there is bias regarding which Internet users are likely to join a panel. The report cites several studies that found online panels are more likely to be comprised of white, active Internet users with high education levels who are considerably more involved in civic and political activities; and who place less importance on religion and traditional gender roles and more importance on environmental issues. Stage 2: Joining and profiling the respondents. Most panels require a respondent to click through from the online ad to the companyâs website to register for the panel and complete some profile information, including an e-mail address. An e-mail is sent to the prospective panel mem- ber, who must respond in order to join the panel. A study by Alvarez et al. (2003) reported that just over 6% of those who clicked on the banner ad completed all of the steps to become a panel member. Stage 3: Completing the questionnaire. This is similar to random-digit dialing when a person refuses to par- ticipate in the survey or does not meet the eligibility requirements. Online surveys have an additional non- response bias from technical problems that can prevent delivery of the e-mail invitation or completion of the survey itself. Some panels will oversample groups that are known to have low response rates in order to have a representative sample after data collection is complete. Although this may result in a balanced sample on that particular dimension, it does not ensure that the sample is representative on other points. Stage 4: Panel maintenance. Attrition can be ânormal,â when people opt out for whatever reasons; or can be forced, when panel members are automatically dropped from the panel after a set period of time to keep the panel fresh. Many strategies are used to reduce panel attrition, but little research exists on reducing or determining the most âdesirableâ attrition rate to balance the costs of adding panel members with the potential concerns of long-term membership, such as panel conditioning. Measurement Error Measurement error is defined as the difference between an observed response and the underlying true response. This can be random error, as when a respondent picks an answer other than the true response, without any systematic direc- tion in the choice made. Systematic measurement error, or bias, occurs when the responses are more often skewed in one direction. Much of the literature regarding measurement error is related to the benefits and potential biases of personal interviewers and self-administered surveys, including paper and online surveys. Because this is an issue that is related to data collection methodology for any survey, not specific to panel surveys, it is beyond the scope of this project and will not be covered in this literature review. However, this is an important issue for all survey efforts, and researchers are encouraged to look at the issues related to both interviewers and self-administered surveys. One measurement issue directly related to panel surveys is that of panel conditioning. Repeatedly taking surveys on a par- ticular topic is known to make respondents more aware of that topic, pay more attention to it in their daily lives, and therefore have different responses on future surveys than if they had not been on the panel. The research on panel conditioning with online panels has mixed findings. Some studies have shown a marked bias towards an increased likelihood to purchase; other studies show that this effect can be mitigated by vary- ing topics from survey to survey. Other research studies have shown no difference in attitudinal responses between infre- quent and very experienced panel survey members. There are two theories on the effects of taking large numbers of surveys: Experienced survey-takers may be more likely to answer in a way that they believe is best for themselves (e.g., it will earn them more incentives, or get more surveys to complete; alter- natively, experienced survey takers will understand the pro- cess better, resulting in more accurate and complete responses. So far, there is no definitive research on the effects of panel members completing large numbers of surveys regarding the accuracy of the survey results. Sample Adjustments to Reduce Error and Bias It is agreed by most researchers that online panels are not representative of the general population, and that techniques are needed to correct for this if the results are used. Four tech- niques have been used to attempt to correct for the known biases with a goal of making the sample representative of the population: sampling to represent a population; modeling; post-survey adjustment; and propensity weighting. 1. The most common form of sampling to represent a certain population is quota sampling, with the quotas often being demographics to match the census. Other elements can be factored in by, for example, balancing members by political affiliation. There does not appear to be any research on the reliability or validity of this type of sampling applied to panel surveys. 2. Models are frequently used in the physical sciences and in epidemiological studies to reduce error and bias. Online panels are much more complex than epidemio- logical studies, however, making it more difficult to apply model-based techniques.
18 3. The most common post-survey adjustment is the weight- ing of survey data. The difference between the sample and sampling frame with probability samples is handled through probability theory principles. Because there is rarely a sampling frame in an online sample, the census and other sources are typically used to adjust the results for under-representation of certain groups of respon- dents. Work conducted by Dever et al. (2008) found that inclusion of enough variables could eliminate coverage bias, but did not address problems associated with being a non-probability sample. 4. To apply propensity weighting, a second âreferenceâ survey with a probability-based sample is conducted at the same time as the online panel survey, using the same questions. A model is built that can be used to weight future online surveys to better represent the target pop- ulation. Although this technique can be used success- fully, it can also increase other types of error, leading to erroneous conclusions from the resulting data. The AAPOR report (Baker 2010) provides an extensive discussion of and guidance on applying these techniques. The reader is encouraged to review the report before applying a sampling adjustment technique. Panel Data Quality Panel data cleaning is an important step in delivering results from respondents who are real, unique, and engaged in the sur- vey. Three areas of cleaning panel data are discussed: eliminat- ing fraudulent respondents, identifying duplicate respondents, and measuring engagement. Fraudulent respondents are those who sign up for a panel multiple times under false names and lie on the qualifying questionnaire to maximize their chances of participation. Duplicate responses occur when respondents answer the questionnaire more than once from the same invita- tion or when they are invited to complete the survey more than once because they belong to more than one panel. Measuring engagement is the most controversial technique. Four basic cleaning strategies are used to weed out respon- dents who may not be engaged with completing the survey, but are simply answering to earn the incentives: recognizing respondents with very short survey times (compared with all surveys); identifying respondents who answer all questions in a matrix format (usually scaled questions) the same way; recording an excessive selection of non-substantive answers, such as âdonât knowâ; and noting nonsense answers or identi- cal answers provided for all open-ended questions. Although there was no research at the time that demon- strated the effects of using cleaned data on the sample or final results, it is generally accepted that negative respondent behavior is detrimental to data quality. Industry Focus on Quality The market research industry has been focused on panel data quality, with virtually every national and international asso- ciation incorporating principles and guidelines for conducting online and panel research. Four key efforts are highlighted in the report: 1. The Council of American Survey Research Organiza- tion (CASRO) revised its Code of Standards and Eth- ics for Survey Research in 2007 to include specific clauses related to online panels. 2. ESOMAR developed comprehensive guidelines titled âConducting Market and Opinion Research Using the Internet.â This was supplemented by its â26 Questions to Help Research Buyers of Online Samples.â 3. The International Organization for Standardization technical committee that developed ISO 20252â Market, Opinion and Social Research also developed ISO 26362âAccess Panels in Market, Opinion and Social Research. The standard defines key terms and concepts in an attempt to create a common vocabu- lary for online panels, and details the specific kinds of information that a research panel is expected to make available to a client at the conclusion of every project. 4. The Advertising Research Foundation established the Online Research Quality Council, which in turn designed and executed the Foundations of Quality project. Work was in progress as of the writing of the AAPOR report, and as of the writing of this synthesis, results of the effort were just being made public. Recommendations The AAPOR Report on Online Panels makes the following recommendations to market researchers who are considering using online access panels: ⢠A non-probability online panel is appropriate when precise estimates of population values are not required, such as when testing the receptivity to product concepts and features. ⢠Avoid using non-probability online access panels when the research is to be used to estimate population values. There is no theoretical basis for making projections or estimates from this type of sample. ⢠The accuracy of self-administered computer surveys is undermined because it is a non-probability sample. A random-digit-dial telephone survey is more accurate than an online survey because it is a probability sam- ple, despite the coverage error arising from households without a landline phone. ⢠It has not yet been demonstrated that weighting the results from online access panel surveys is consistently effective and can be used to adjust for panel bias.
19 ⢠There are significant differences in the composition and practices of various online access panels, which can affect survey results. Different panels may yield significantly different results on the same questionnaire. Market Research by the Public Sector Poynterâs book devotes a section to issues specific to pub- lic sector research. Although most of the marketing research principles apply equally to the private and public sectors, there are a few areas where the public sector researcher needs to be particularly attentive, because public funds are being used to conduct the research and the results may determine how public funds are expended. Areas for particular attention are identified as: operating in the public eye, ârepresentativ- ity,â geographical limitations, social media and the public sector, and ethics. In the Public Eye Public sector research is subject to audit, inspection, and reporting in the media. Freedom of information laws ensure that the public has a right to see how public funds are being spent. Poorly conducted research could be brought to light in a public forum, creating public relations problems for a per- ceived waste of taxpayer money and jeopardizing the ability to conduct future research. As a result, care must be taken to ensure public sector research is conducted to the highest qual- ity and ethical standards. Representativity Having a representative sample is always important, but is of special concern for public agencies. Many public services, such as public transportation, target specific groups which may have multiple challenges. Much of the target population may not have Internet access, and those that do may not be typical of the market segment they are expected to represent. For each study, the researcher must carefully assess whether an online survey is appropriate for that market and research purpose, and whether the sampling and recruitment strategies provide survey results that can be defended in public. Geographical Limitations Public agencies have strict geographic boundaries from which a sample population can be drawn. Face-to-face or telephone surveys are often simplified by these restrictions. Surveys using an online access panel, however, can be problematic, as there may not be an adequate sample of persons from the target area. This is further exacerbated when the sample is also required to be representative of the population within a speci- fied geographical area. Social Media and the Public Sector There are several ways in which social media are being used for research in the public sector. Online communities engage in a range of activities, including information-sharing, research, community-building, and engagement. Online research com- munities are typically closed communities, operated by a ven- dor, with membership by invitation only as part of an overall sampling plan (see the MnDOT case example of an online research community). Twitter, blogs, and public discussions are resources for passive research, using data mining tools to monitor trends in what people are saying about the agency. Although useful information can be elicited from these sources, it should be noted that they do not provide a representative sample, and should be considered public comment rather than research. Social media is often used to reach out to groups that are otherwise hard to reach, such as young adults. It should be noted that using a variety of social media techniques, such as Facebook, YouTube, and Twitter, is likely to reach the same people multiple times. If multiple social media channels are used to recruit online survey participants, for example, the researcher must be prepared for the potential duplication of survey responses. Ethics There is an expectation that research will be reliable, and can be used by a decision-making body in a public forum. First and foremost, the researcher must provide unbiased market research. Often a vendor is used to conduct the research so as to provide a wall between the agency and the research and avoid the appearance of leading the respondents, or âspinningâ the results. The second concern is that quantitative research based on random probability sampling has been the standard method for achieving that level of reliability expected of a pub- lic agency. Since online research is typically not from a proba- bilistic sample, the researcher should recognize the potential lack of statistical reliability inherent in the research design and ensure that decision makers understand the limitations of the data. FUTURE OF MARKET RESEARCH Technology has fundamentally changed how society com- municates and how it does business. Whereas people used to communicate by means of the telephone at home, cell phones make communication possible virtually anywhere. Cell phone numbers do not represent a physical address; they have become a moving, real-time âpersonalâ address. The Internet provides instant access to information and communication through e-mail, websites and social media. The smart phone combines mobile communication with the Internet, creating
20 a completely new, technology-based world. Panels can now be developed online, quickly and easily. Household and per- sonal contact information is no longer tied to a home address, but exists outside of the personâs geographic location. This technology has led to a revolution in market research. Recruiting survey respondents is easier; developing a panel is faster; and surveys are online, resulting in automation of survey tabulation and reporting. As a result, recruiting and maintaining research panels is simpler, less expensive, and very attractive to decision makers who want results ânow.â But these changes have created a myriad of concerns, pri- marily related to using non-probabilistic sampling practices. The history of sampling theory provides some insights into what may occur in the future. In 1934, although sam- pling theory had not yet been developed, Anders Kaier con- vinced an international audience that representative samples could be used to represent a population. Morris Hansen of the U.S. Census Bureau greatly expanded the theory and prac- tice of sampling and helped convince the bureau to accept sampling and quality control methods in the 1940 Census. Through the leadership of these two important individuals, the practice was adopted. From there, sampling theory was developedâit followed the practice, rather than the theory creating the practice. Brick states that data collection costs will continue to put pressure on agencies to use non-probability samples from online recruitment. If this cannot be done within design-based probability sampling theory, he suggests two potential out- comes: A new paradigm that accommodates online surveys is introduced, which replaces or supplements traditional prob- ability sampling; or online surveys using non-probabilistic sampling are restricted to specific applications as a result of the weak theoretical basis. One potential solution is the use of multiple-frame sam- pling to reduce coverage error, a fundamental concern with online panel research. For example, to reach transit riders, an online survey could be placed on the agency website and be supplemented with paper surveys on board vehicles. Stat- isticians are working on establishing a theoretical basis for conducting sampling using the multiple-frame technique (Brick 2011). In addition to the changes in survey practice that led to the historical development of sampling theory, two additional factors are cited as creating the paradigm shift from popula- tion surveying to representative sampling in 1934. The first was the wealth of scientific development and statistical ideas, not necessarily related to survey sampling, which neverthe- less supported the growth and change in methods. The sec- ond factor was societyâs demand for information on a wide range of topics that made population sampling cumbersome and expensive. This desire for faster, cheaper research drove the development of probability sampling and our current market research paradigm. These characteristics are in place today, almost 80 years after probabilistic sampling made its debut. With the rapid changes in technology and societyâs insatiable thirst for more information, more quickly, and for less cost, a new research paradigm with a theoretical founda- tion to support non-probabilistic online surveying may be on the horizon.
TRB’s Transit Cooperative Research Program (TCRP) Synthesis 105: Use of Market Research Panels in Transit describes the various types of market research panels, identifies issues that researchers should be aware of when engaging in market research and panel surveys, and provides examples of successful market research panel programs.
The report also provides information about common pitfalls to be avoided and successful techniques that may help maximize research dollars without jeopardizing the quality of the data or validity of the results.
READ FREE ONLINE
Welcome to OpenBook!
You're looking at OpenBook, NAP.edu's online reading room since 1999. Based on feedback from you, our users, we've made some improvements that make it easier than ever to read thousands of publications on our website.
Do you want to take a quick tour of the OpenBook's features?
Show this book's table of contents , where you can jump to any chapter by name.
...or use these buttons to go back to the previous chapter or skip to the next one.
Jump up to the previous page or down to the next one. Also, you can type in a page number and press Enter to go directly to that page in the book.
To search the entire text of this book, type in your search term here and press Enter .
Share a link to this book page on your preferred social network or via email.
View our suggested citation for this chapter.
Ready to take your reading offline? Click here to buy this book in print or download it as a free PDF, if available.
Get Email Updates
Do you enjoy reading reports from the Academies online for free ? Sign up for email notifications and we'll let you know about new publications in your areas of interest when they're released.
A Comprehensive Literature Review on Marketing Strategies Adopting by Various Industries
9 Pages Posted: 30 Dec 2022
Rashini Hansika
Uva Wellassa University, Faculty of Management
Date Written: December 20, 2022
Marketing is the process of promoting and selling products and services, including market research and advertising. Today, marketing is an essential part of any organization's growth strategy. Many firms use marketing methods unknowingly to promote themselves and increase sales of their products and services. Marketing is one of the most crucial elements of organizations nowadays. Any strategy used to assist a group, company, or individual in accomplishing their objectives falls under the very broad category known as marketing. The goals of marketing are to raise brand awareness among the target market, increase market share, introduce the firm to new domestic or international markets, boost ROI, increase business profit, optimize the conversion funnel, acquire new leads, foster customer loyalty, and boost sales. A marketing strategy is a company's overarching plan for connecting with potential customers and persuading them to purchase its products or services. A marketing plan typically includes the value proposition of the company, key brand messages, data on target customer demographics, and other significant elements.
Keywords: Marketing Strategy, Premium pricing
Suggested Citation: Suggested Citation
Rashini Hansika (Contact Author)
Uva wellassa university, faculty of management ( email ), do you have a job opening that you would like to promote on ssrn, paper statistics.
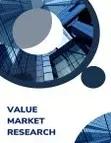
Global Literature Review Software Market Report By Type (Cloud-Based, On-Premise), By Application (Large Enterprises, Small and Medium-sized Enterprises (SMEs) And By Regions - Industry Trends, Size, Share, Growth, Estimation and Forecast, 2023-2032
The global demand for Literature Review Software Market is presumed to reach the valuation of nearly USD XX MN by 2030 from USD XX MN in 2022 with a CAGR of XX% during the period of 2023-2030. A literature review talks about the published material in a specific subject area, and at times within a certain time period. It can be just a humble synopsis of the sources, but typically a literature review includes structural patterns and blends both synopsis and synthesis. A synopsis is an outline of the important information drawn from the source; however, synthesis is a re-establishment of the same information. It may offer a newly analyzed version of old material or blend new with old analysis. A literature review software places each work in the context of its role and impact to have an insight into the research problem being examined in an automated way. The key focus of a literature review software is to outline, decode and synthesize the assertions and inklings of others without reinforcing new contributions in an automated way. Market Dynamics The ever-changing industry trends generate favorable prospects as well as complications with it. Literature Review Software helps to decode and analyze current industry trends and helps businesses to stay ahead of competitors and take the right business decisions at the right time. With the rise in industrialization and scientific research, the literature review software market is expected to flourish. The report covers Porter's Five Forces Model, Market Attractiveness Analysis and Value Chain analysis. These tools help to get a clear picture of the industry's structure and evaluate the competition attractiveness at a global level. Additionally, these tools also give inclusive assessment of each application/product segment in the global market of literature review software. Market Segmentation The entire literature review software market has been sub-categorized into type, application. The report provides an analysis of these subsets with respect to the geographical segmentation. This research study will keep marketer informed and helps to identify the target demographics for a product or service. By Type
- Cloud-Based
By Application
- Large Enterprises
- Small and Medium-sized Enterprises (SMEs)
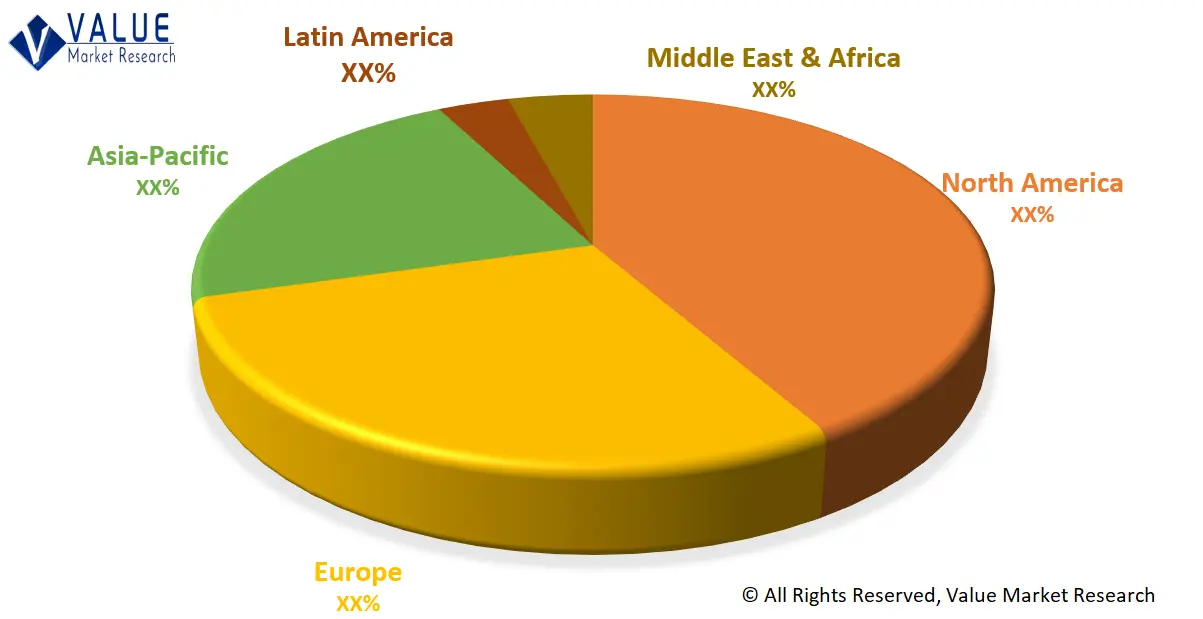
Frequently Asked Questions (FAQs) about this Report
What is the Global Literature Review Software Market segmentation covered in the report?
Who are the leading Global Literature Review Software Market manufacturers profiled in the report?
- Single User License: $3,920.00
- Upto 10 Users License: $4,730.00
- Corporate User License: $7,430.00
- DataPack License: $2,120.00
Avail customized purchase options to meet your exact research needs:
- Buy sections of this report
- Buy country level reports
- Request for historical data
- Request discounts available for Start-Ups & Universities
- Define and measure the global market
- Volume or revenue forecast of the global market and its various sub-segments with respect to main geographies
- Analyze and identify major market trends along with the factors driving or inhibiting the market growth
- Study the company profiles of the major market players with their market share
- Analyze competitive developments
- Client First Policy
- Excellent Quality
- After Sales Support
- 24/7 Email Support
Key questions answered by the report
- What is the current market size and trends?
- What will be the market size during the forecast period?
- How various market factors such as a driver, restraints, and opportunity impact the market?
- What are the dominating segment and region in the market and why
Need specific market information?
- Ask for free product review call with the author
- Share your specific research requirments for a customized report
- Request for due diligence and consumer centric studies
- Request for study updates, segment specific and country level reports
USEFUL LINKS
- Upcoming Reports
- Testimonials
- How To Order
- Research Methodology
FIND ASSISTANCE
- Press Release
- Privacy Policy
- Refund Policy
- Terms & Conditions
UG-203, Gera Imperium Rise, Wipro Circle Metro Station, Hinjawadi, Pune - 411057
- [email protected]
- +1-888-294-1147
BUSINESS HOURS
Monday to Friday : 9 A.M IST to 6 P.M IST
Saturday-Sunday : Closed
Email Support : 24 x 7
© , All Rights Reserved, Value Market Research
Baazar Style Retail IPO allotment date likely today: Latest GMP, how to check status in 4 steps
Baazar style retail's ipo, backed by rekha jhunjhunwala, raised ₹ 834.68 crore and was oversubscribed 40.6 times. share allotment is expected on september 4, with listing on bse and nse set for september 6..

Step 3: enter your PAN or application number or DP/Client ID or Account No.
Step 4: Click on Search
Your Baazar Style Retail IPO allotment status will be displayed on the screen.
How to check Baazar Style Retail IPO share allotment status on the BSE website
Step 1: Go to the BSE's allotment page through this link: https://www.bseindia.com/static/investors/application_statuschecksystem.aspx
Step 2: Select 'Equity' in the 'Issue Type' section.
Step 3: From the drop-down menu, pick the IPO under 'Issue Name'.
Step 4: Enter your application number or PAN.
How to check Baazar Style Retail IPO share allotment status on the NSE website
Step 1: Go to the official website of NSE using the following link- https://www1.nseindia.com/products/dynaContent/equities/ipos/ipo_login.jsp
Step 2: To register, click on the 'Click here to sign up' option and provide your PAN on the NSE website.
Step 3: Enter your username, password, and captcha code.
Step 4: After you are redirected to the next page, verify the status of your IPO allocation.
Baazar Style Retail IPO GMP today
Baazar Style Retail IPO GMP (grey market premium) today is +65. This indicates Baazar Style Retail share price was trading at a premium of ₹ 65 in the grey market, according to investorgain.com.
Based on the upper end of the IPO price range and the current premium in the grey market, Baazar Style Retail's estimated listing price is predicted to be ₹ 454 per share, representing a 16.71 per cent increase from the IPO price of ₹ 389.
Read all market-related news here
Disclaimer: The views and recommendations above are those of individual analysts, experts, and brokerage firms, not Mint. We advise investors to consult certified experts before making any investment decisions.
Catch all the Business News , Market News , Breaking News Events and Latest News Updates on Live Mint. Download The Mint News App to get Daily Market Updates.
- HDFC Bank Share Price
- Reliance Industries Share Price
- TCS Share Price
- Infosys Share Price
- HUL Share Price
Most Active Stocks
Bharat electronics, oil & natural gas corporation, market snapshot.
- Top Gainers
- 52 Week High
Jubilant Ingrevia
Piramal pharma, mazagon dock shipbuilders, deepak fertilisers & petrochemicals corporation, trending in market.
- Orient Technologies IPO
- Chandan Taparia recommendations
- NSE new circular
Recommended For You
Gold prices, popular in markets, ecos mobility ipo allotment finalised. gmp, steps to check status, baazar style retail ipo day 3: gmp, subscription status to review. apply or not, wait for it….
Log in to our website to save your bookmarks. It'll just take a moment.
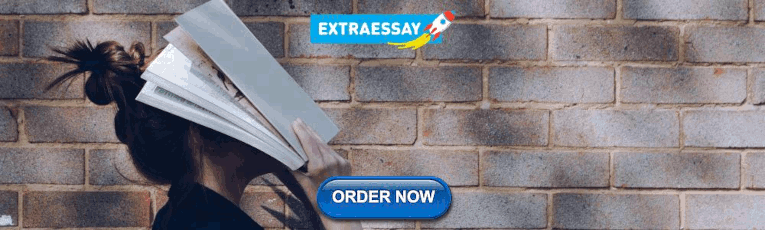
IMAGES
VIDEO
COMMENTS
A more careful analysis of the conceptualizations in Table 1 helps us discern several recurring themes that reflect the three central elements of market innovation. First, most conceptualizations employ a structural notion of market. For example, they refer to product-market structures (Darroch & Miles, 2011), exchange structures (Giesler, 2012), market norms and market representations ...
The impact of market share on financial firm performance is one of the most widely studied relationships in marketing strategy research. However, since the meta-analysis by Szymanski, Bharadwaj ...
A literature review can broadly be described as a more or less systematic way of collecting and synthesizing previous research (Baumeister & Leary, 1997; Tranfield ... New empirical generalizations from a meta-analysis of the market share-performance relationship. Journal of Marketing, 82 (2018), pp. 1-24, 10.1509/jm.16.0250. View in Scopus ...
A stock exchange, also called a securities exchange or. bourse is the name given to the facility for engaging in buying and selling of shares of. stock or bonds or other financial instruments. For ...
Market Share as a Performance Measure: A Conceptual Framework Tommy Hsu Tarleton State University, U.S.A. Received 17 January 2022 Accepted 16 March 2022 ABSTRACT In this article, the author provides an overview of the literature in the market share research and proposes a comprehensive model which can empirically investigate the relationship ...
Many firms use market share to set marketing goals and monitor performance. ... "Metrics that Marketers Muddle," MIT Sloan Management Review, 57 (3), 73-82. Google Scholar. Bhattacharya Abhi, Misra Shekhar, Sardashti Hanieh (2019), "Strategic ... (2000), "No Pain, No Gain: A Critical Review of the Literature on Signaling Unobservable ...
Market Share as a Performance Measure: A Conceptual Framework. Tommy Hsu. Published in European Journal of Studies… 1 May 2022. Business, Economics. European Journal of Studies in Management and Business. In this article, the author provides an overview of the literature in the market share research and proposes a comprehensive model which ...
However, the main purpose of this review is to examine effective GARCH models recommended for performing market returns and volatilities analysis. The secondary purpose of this review study is to conduct a content analysis of return and volatility literature reviews over a period of 12 years (2008-2019) and in 50 different papers.
A formal literature review is an evidence-based, in-depth analysis of a subject. There are many reasons for writing one and these will influence the length and style of your review, but in essence a literature review is a critical appraisal of the current collective knowledge on a subject. Rather than just being an exhaustive list of all that ...
Past reviews of studies concerning competitive pricing strategies lack a unifying approach to interdisciplinarily structure research across economics, marketing management, and operations. This academic void is especially unfortunate for online markets as they show much higher competitive dynamics compared to their offline counterparts. We review 132 articles on competitive posted goods ...
This article is a practical guide to conducting data analysis in general literature reviews. The general literature review is a synthesis and analysis of published research on a relevant clinical issue, and is a common format for academic theses at the bachelor's and master's levels in nursing, physiotherapy, occupational therapy, public health and other related fields.
The Literature Review about Brand Image . According to ... Market share and advertising efficiency effects are elevated when the extension is composed primarily of experience attributes and ...
Examples of literature reviews. Step 1 - Search for relevant literature. Step 2 - Evaluate and select sources. Step 3 - Identify themes, debates, and gaps. Step 4 - Outline your literature review's structure. Step 5 - Write your literature review.
We are not allowed to display external PDFs yet. You will be redirected to the full text document in the repository in a few seconds, if not click here.click here.
This study seeks to evaluate the market orientation literature within the scope of the hotel industry. It uses bibliometric methods, particularly a co-citation analysis combined with a literature review. Data were obtained from the Web of Science database and 136 unique documents were used in the analysis. The results show that market orientation literature using data from the hotel industry ...
The purpose of a literature review. The four main objectives of a literature review are:. Studying the references of your research area; Summarizing the main arguments; Identifying current gaps, stances, and issues; Presenting all of the above in a text; Ultimately, the main goal of a literature review is to provide the researcher with sufficient knowledge about the topic in question so that ...
Without it, quantitative market This literature review consists of four sections: â ¢ The first section provides a brief history of survey sam- pling and the theoretical basis for market research anal- ysis, providing context for what became the standard procedures and expectations of market research. ... Share a link to this book page on ...
The earlier literature review papers on stock market liquidity have considered an inconsistent number of studies from various research databases while some have examined a few studies that highlight different perspectives of stock market liquidity. ... Canadas N., Rodrigues A. M. (2015). Determinants of share price and share liquidity: An ...
A Comprehensive Literature Review on Marketing Strategies Adopting by Various Industries. 9 Pages Posted: 30 Dec 2022. See all articles by Rashini Hansika ... The goals of marketing are to raise brand awareness among the target market, increase market share, introduce the firm to new domestic or international markets, boost ROI, increase ...
Literature Review Software Market is anticipated to reach USD XX.X MN by 2032, this market report provides the growth, trends, key players & forecast of the market based on in-depth research by industry experts. The global market size, share along with dynamics are covered in the literature review software market report
The purpose of the literature review is to summarize the previous studies about marketing-financial performance nexus. Studies that were published between 1969 and 2019 could be reached from the ...
Literature reviews establish the foundation of academic inquires. However, in the planning field, we lack rigorous systematic reviews. In this article, through a systematic search on the methodology of literature review, we categorize a typology of literature reviews, discuss steps in conducting a systematic literature review, and provide suggestions on how to enhance rigor in literature ...
On Tuesday, during the second day of bidding, Gala Precision Engineering IPO received a subscription of 52.21 times, as per BSE data. Non-institutional investors subscribed to the quota 132.93 ...
Baazar Style Retail's IPO, backed by Rekha Jhunjhunwala, raised ₹834.68 crore and was oversubscribed 40.6 times. Share allotment is expected on September 4, with listing on BSE and NSE set for ...
Kumar and Misra (2015) evaluated 95 articles and presented a review of literature on various aspects of stock market liquidity like measurement of liquidity, determinants of liquidity, intraday movements, and liquidity effects on firm value. Recently, Díaz and Escribano (2020) reviewed 177 articles and discussed the dimensional liquidity ...
Mixed neuroendocrine and non-neuroendocrine neoplasms (MiNEN), formerly known as mixed adenoneuroendocrine carcinoma (MANEC), has been described in the WHO classification as a rare tumor in various gastrointestinal sites. 1 Historically, the nomenclature of these tumors was proposed by Lewin more than 30 years ago. He divided them into three separate groups, mixed or composite tumors ...