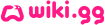
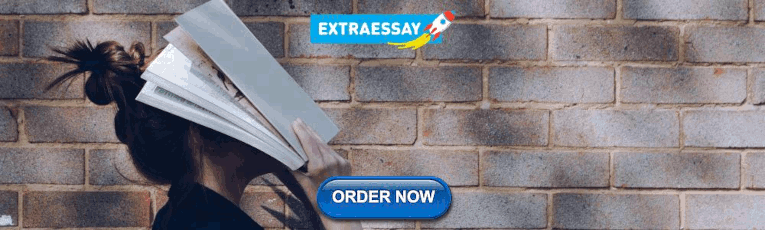
Personal tools
- Create account
Navigation menu
- Sign up to edit
- View source
- View history
- Purge cache
- Community portal
- Recent changes
- Random page
- Editors' Manual
- Official forums
- Admin noticeboard
External Resources
- Oxygen Not Included Database
- ONI Assistant
- BlueprintNotIncluded
- Professor Oakshell's Calculators
More KLEI Wikis
- Don't Starve
- Invisible Inc.
- What links here
- Related changes
- Special pages
- Printable version
- Permanent link
- Page information
- Cite this page
Data Analysis Research

Data Analysis Research is a tertiary tier of Research points. It is required for all tier 6 and higher research categories.
Data Analysis Research Points are obtainable by a Duplicant by processing Data Banks at the Virtual Planetarium . Data Banks can be generated in a number of ways, including using the Orbital Data Collection Lab , studying Geysers , analyzing Artifacts , and searching Crashed Satellites and inspectable Points of Interests.
- U37-483944 : Orbital Research rethemed to Data Analysis Research, which was generated by processing data disks at the Virtual Planetarium .
- Orbital Data Collection Lab
- Novice Research
- Advanced Research
- Applied Sciences Research
- Version Controlled Pages
GUIDES NOT INCLUDED
Keep calm and press the space bar
The Complete Beginner's Completely Incomplete Guide to Oxygen Not Included
Spaced out research: radbolts, data banks and you, introduction.
There is a bunch of useful stuff in the research tree that is locked behind the third and fourth kinds of research. (The first two kinds are covered earlier in this guide .)
For the third kind of research you will need Data Banks, which in turn means you will have to send a dupe (and some plastic) into orbit. (Don't worry - you get the dupe back.) For the fourth kind of research you will need to generate radbolts.
You will probably need the fourth kind of research before the third kind, so let's be confusing and look at them in reverse order.
Applied Sciences Research (the one with the radbolts)
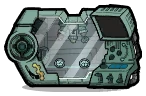
Materials Study Terminal. Aim radbolts at the circle on the left.

Manual Radbolt Generator

Radbolt Generator
Spaced Out introduces radiation into the game. You can see radiation levels with the radiation overlay (the radiation image top right).
The fourth kind of research is called Applied Sciences Research . It's the one with the orange icon in the research tree.
The way you conduct applied sciences research is by shooting radbolts into a Materials Study Terminal (a kind of research station). This generates the points used for research. The research is then conducted on the materials study terminal (the same one you shoot the radbolts into).
There are two ways to generate radbolts:
A Manual Radbolt Generator uses Uranium Ore and dupe labour to generate radbolts
A Radbolt Generator gathers radiation from its surroundings to generate radbolts
Note: both generators let you decide what direction you want them to shoot the radbolts.
Another note: shooting radbolts into a materials study terminal is a good thing. Shooting them into a dupe is a bad thing. So make sure to check the direction thing mentioned in the previous note.
A radbolt shot from the radbolt generator will be worth (or contain) 50 radbolt points
A radbolt shot from the manual generator will be worth (or contain) 5 radbolt points
The materials study terminal can store 100 points worth of radiation. If the materials study terminal is full and you shoot more radbolts at it, they will spin around a bit in the terminal and then continue on in the direction they were heading when entering the machine.
Note: A radbolts rad value decreases slightly as it travels, so the actual value you get out of one radbolt depends on how far it has to travel to get to the research terminal.
Another note: according to the wiki you can also use enriched uranium ore for the manual radbolt generator, which would generate a radbolt worth 25 rabdolt points.
One way to keep a researching dupe out of harms way when firing radbolts into a materials study terminal is to fire them at an angle. For instance by placing your radbolt generator below and to the left of your materials study terminal. (You can also research a tile that lets radbolts pass through it, making it possible to shoot them from beneath the terminal.)
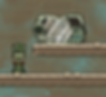
Diagonal setup. Radbolts won't hit the dupe doing research. (But be mindful of what you have above and to the right of the terminal.)
If you are using a manual radbolt generator then you will need Uranium Ore to generate radbolts. (Some maps have it, if yours doesn't you can check the planet you can teleport to.)
Digging up a few tiles of uranium ore is enough if you just want to get some of the early things researched. Once dug up, uranium ore is rad-free and fine for dupes to handle. (And even before you dig it up it only emits small amounts of radiation.)
You can of course research lots of stuff with the manual radbolt generator if you want, it just ties up a dupe and uses uranium ore. I usually do lots of the early research on the manual generator (partly because the more advanced setup needs a lot of power and partly because setting it up takes a while and I'm lazy.) I switch over the the radbolt generator at the latest when research starts requiring hundreds of points per unlock.
If you will be using a radbolt generator (not the manual one) then you need to place it in an area with a lot of rads. Or, more likely, you will place a lot of rads around your radbolt generator - commonly with the help of one or several Wheezeworts.

Wheezewort and radbolt generator. More wheezeworts produce more radiation. So stick in some extras if you have them.

I use us a design like this. (Note: Oops - the auto-sweeper can't reach the wheezewort on the bottom. So if you use something like this, modify it a bit. Have a second auto-sweeper below and place the phosphorite storage bin so both auto-sweepers can reach it. Or have a way for dupes to fertilize the bottom wheezewort. Or move the auto-sweeper to below the farm tiles.)
Wheezeworts need to be fertilized with phosphorite. You can find it in the hot, purple (aka caustic) biome. Also, Dreckos and Glossy Dreckos drop phosphorite.
Wheezeworts produce a lot of radiation. A lot of Wheezeworts produce a lot of a lot of radiation. You can save your dupes a bunch of radiation exposure by setting up a storage bin and an auto-sweeper so the auto-sweeper handles the fertilization. (I need to check this but I think phosphorite is under "agriculture" in the storage bin selection list.)
When planning your layout, keep in mind that it is the lower, green part of the radbolt generator that collects rads. Also, particularly if you are manually fertilizing the wheezeworts, make sure dupes can't be hit by radbolts.
A radbolt generator will generate 1/10th of the rads in its surroundings. (So 1000 rads per cycle would generate 100 radbolt points per cycle.)
A final note: The radbolt generator is a power-hungry little thing (480W). If there are brownouts - if you run out of power - it begins losing its radiation charge. So put some thought into power and how you will make sure you have a steady supply when setting up this research build.
(And, if all of this seems a bit much to deal with, you can always use the manual generator until you feel ready to go for a wheezewort setup.)
Interstellar Research (the one with the data banks)
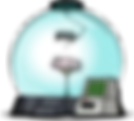
Virtual Planetarium

Orbital Collection Lab
Interstellar research is conducted in a Virtual Planetarium using Data Banks .
You will come across some data banks on your map. Whenever you find a structure of any kind, click on it and see if you can examine it. Often you can, and it will result in some data banks. Analyzing geysers and the like also gives some data banks.
The data banks you come across won't be anywhere near enough to unlock the entire research tree. To get additional data banks you will need an Orbital Collection Lab .
The orbital collection lab uses dupe labour and plastic to generate data banks. However, you can only operate orbital collection labs when in space. Blasting off and heading one tile in any direction is enough.
( Getting to space is covered later in the guide.)
Use of the orbital collection lab is affected by the machinery skill. But its use is not locked behind any particular skill - any dupe can use it.
To unlock the entire research tree you will need a lot of plastic - upwards of 10K. So give some thought to your plastic production. (Later in this guide we'll cover the low-tech option of ranching Glossy Dreckos and also refining petroleum .)

Oxygen Not Included

4,141 | Unique Visitors |
192 | Current Favorites |

- Use a high pressure vent on your pump's oxygen output
- Store any oxylite high in the cabin, as far from CO2 as possible
- Run a long snake of piping off the pump output before the oxygen return vent, so that if the vent does overpressurize you have lots of extra gas storage in the pipes.
- Run a long snake of piping off the pump output before the CO2 output vent, so that while in space you have lots of extra gas storage in the pipes.
- Uses the solo spacefarer nosecone with a data collection lab to get the first bits of space research started before you can build your first "real" rocket.
- Uses a standard spacefarer module with a data collection lab and standard telescope. Can sustain two dupes in space for long periods of research. In place of the standard telescope a second data collection lab can be installed to double the research output.
- Same as the regular research rocket, except it uses the large (enclosed) telescope. Yes, it's possible :)
- The gray tiles near the ceiling can be either plastic tile made of plastic or a metal tile made of lead. Their purpose is to block radiation, and these two materials have the best shielding properties at 68%. Use whichever material your colony can spare.
- The light brownish speckled tiles are carpet tiles and I use them by default for any surface a dupe could stand on. These tiles are fantastic for decor and also give the dupes a "tickled tootsies" stress debuff when walked on. If you're limited on reed fiber then you want to at least try to have a carpet tile under beds, workstations and mess tables, where the dupes will spend long periods of time.
- The light blue semi-transparent tiles are window tiles. These can be made of either glass or diamond, and the purpose is to allow light through. I only use these where a carpet tile would block the well lit bonus on a station below.
- The darker brown tile that looks like a brick texture is just a standard tile. It only exists to save the special materials above when there would be no benefit from using another tile type. In other words if a dupe can't walk on it and I don't need to block radiation or allow light below it, then a standard tile works.
- Finally the wire mesh looking tiles are airflow tiles. I typically don't use these unless I'm installing a pump and need to block it off from another room to preserve the morale bonus, or along the floor to ensure CO2 won't get trapped on one side.
- 4 (3) Mess Tables
- 1 (2) Party Line Phone
- Refrigerator
- Power Outlet
- Gas Output Fitting
- Ceiling Light
- 1 telescope (or a second orbital data lab)
- 2 mess tables
- 2 party line phones
- 1 hand washing station
- 1 ceiling light
- 1 plastic/lead tile left of the fridge (plus the upper left corner tile if you skipped building it)
- Add a power outlet and wire power inside the rocket
- Add a gas vent and gas output fitting (only if using the mini pump and gas cargo canister as your CO2/O2 solution)
- Optionally add a starmap location sensor
- 2 plastic/lead shielding tiles + 1 airflow tile (to enclose the pump)
- First of all, if you want to keep things how they are but add the well lit bonus to the pilot station, you can replace the left carpet tile above it with a window tile. The tradeoff is the dupe eating at the mess table will not receive the tickled tootsies stress debuff.
- If you would prefer that the telescope receive the well lit bonus *instead of* the orbital data lab, you can swap the positions of the power outlet and ceiling light. This has the added bonus of providing the well lit bonus to the pilot station without having to replace the carpet tile with a window.
- If you would like both the telescope *and* the orbital data lab to receive the well lit bonus (and the pilot station too) you can keep everything as shown and construct a second ceiling light to the left of the aeropot.

- Remember me Not recommended on shared computers
Forgot your password?
Or sign in with one of these services
- [Oxygen Not Included: Spaced Out!] - General Discussion
The first and last research rocket you'll ever need [guide]
By n_t_p , April 17, 2021 in [Oxygen Not Included: Spaced Out!] - General Discussion
Recommended Posts

Link to comment
Share on other sites.
35 minutes ago, n_t_p said: Hi. Here's my rocket: Oxygen is provided from storage module. CO2 is pumped back in. On the floor you'll need non-perishable food, metal (preferably copper or gold), glass, ore, and 6.1t of plastic. That's how much plastic it takes to do all research (as of this post). Deconstruct the rocket control station after going up into orbit. You don't need it. Deconstructing and rebuilding the manual generator each day gets you the +1 morale from the barracks. Idk if this is worth it. First thing to research is the pixel packs. That will get you enough morale to not worry about stress, and you can stop rebuilding the manual generator each day if you were doing that. Second thing to research is the small gas pump. It will be in place well before CO2 takes over the cabin. Duplicant +1 Barracks +1 Mess hall +3 Four shift break +3 Grizzly meal -1 Advanced Research skill -1 Astronomy skill -2 Orbital Researcher skill -3 Rocket piloting skill -1 Total morale = 0 If rebuilding the manual generator is too much micro management you can probably ignore it. -1 morale is manageable, and will take a long time to stress a dupe out to the point of having a stress reaction. After researching and building the Pixel Packs you should be at +1 or +3 or so which should give enough stress relief to negate the bathroom loss and soggy feet stress. For extra wiggle room you can send a dupe with an interest in science, or a dupe with the sunny disposition neural vacillator buff, or send up berry sludge instead of nutrition bars. Reveal hidden contents
Why, thank you! This is an excellent design! Don't mind if I use it...
SharraShimada
On 4/17/2021 at 1:26 PM, SharraShimada said: Okay, nice design, but it will only work until the devs disable deconstruction of the control station mid flight
There's a simple yet fancy solution for that. Replace the cot with a rocket control station.
On 4/17/2021 at 9:26 AM, SharraShimada said: Okay, nice design, but it will only work until the devs disable deconstruction of the control station mid flight
I don't think they will. It's only needed for launch and the speed bonus.
This topic is now archived and is closed to further replies. Please be aware that the content of this thread may be outdated and no longer applicable.
- Existing user? Sign In
- Game Updates
- Klei Bug Tracker
- All Activity
My Activity Streams
- Unread Content
- Content I Started
- Klei Ambassadors
- Service Status
- Create New...
| |||||||||||||||||||||||||||||||||||||||||||||||||||||||||||||||||||||||||||||||||||||||||||||||||||||||||||||||||||||||||||||||||||||||||||||||||||||||||||||||||||||||||||||||||||||||||||||||||||||||||||||||||||||||||||||||||||||||||||||||||||||||||||||||||||||||||||||||||||||||||||||||||||||||||||||||||||||||||||||||||||||||||||||||||||||||||||||||||||||||||||||||||||||||||||||||||||||||||||||||||||||||||||||||||||||||||||||||||||||||||||||||||||||||||||||||||||||||||||||||||||||||||||||||||||||||||||||||||||||||||||||||||||||||||||||||||||||||||||||||||||||||||||||||||||||||||||||||||||||||||||||||||||||||||||||||||||||||||||||||||||||||||||||||||||||||||||||||||||||||||||||||||||||||||||||||||||||||||||||||||||||||||||||||||||||||||||||||||||||||||||||||||||||||||||||||||||||||||||||||||||||||||||||||||||||||||||||||||||||||||||||||||||||||||||||||||||||||||||||||||||||||||||||||||||||||||||||||||||||||||||||||||||||||||||||||||||||||||||||||||||||||||||||||||||||||||||||||||||||||
| |||||||||||||||||||||||||||||||||||||||||||||||||||||||||||||||||||||||||||||||||||||||||||||||||||||||||||||||||||||||||||||||||||||||||||||||||||||||||||||||||||||||||||||||||||||||||||||||||||||||||||||||||||||||||||||||||||||||||||||||||||||||||||||||||||||||||||||||||||||||||||||||||||||||||||||||||||||||||||||||||||||||||||||||||||||||||||||||||||||||||||||||||||||||||||||||||||||||||||||||||||||||||||||||||||||||||||||||||||||||||||||||||||||||||||||||||||||||||||||||||||||||||||||||||||||||||||||||||||||||||||||||||||||||||||||||||||||||||||||||||||||||||||||||||||||||||||||||||||||||||||||||||||||||||||||||||||||||||||||||||||||||||||||||||||||||||||||||||||||||||||||||||||||||||||||||||||||||||||||||||||||||||||||||||||||||||||||||||||||||||||||||||||||||||||||||||||||||||||||||||||||||||||||||||||||||||||||||||||||||||||||||||||||||||||||||||||||||||||||||||||||||||||||||||||||||||||||||||||||||||||||||||||||||||||||||||||||||||||||||||||||||||||||||||||||||||||||||||||||||
![]() Saunders’ Research OnionPlain-Language Explainer (With Examples) By: David Phair (PhD) and Kerryn Warren (PhD) | January 2021 ![]() I f you’re learning about research skills and methodologies, you may have heard the term “ research onion ”. Specifically, the research onion developed by Saunders et al in 2007 . But what exactly is this elusive onion? In this post, we’ll break Saunders’ research onion down into bite-sized chunks to make it a little more digestible. What (exactly) is the research onion?At the simplest level, Saunders’ (2007)research onion describes the different decisions you’ll need to make when developing a research methodology – whether that’s for your dissertation, thesis or any other formal research project. As you work from the outside of the onion inwards , you’ll face a range of choices that progress from high-level and philosophical to tactical and practical in nature. This also mimics the general structure for the methodology chapter . While Saunders’ research onion is certainly not perfect, it’s a useful tool for thinking holistically about methodology. At a minimum, it helps you understand what decisions you need to make in terms of your research design and methodology. The layers of Saunders’ research onionThe onion is made up of six layers, which we’ll peel back one at a time:
Onion Layer 1: Research PhilosophyThe very first layer of the onion is the research philosophy . But what does that mean? Well, the research philosophy is the foundation of any study as it describes the set of beliefs the research is built upon . Research philosophy can be described from either an ontological or epistemological point of view. “A what?!”, you ask? In simple terms, ontology is the “what” and “how” of what we know – in other words, what is the nature of reality and what are we really able to know and understand. For example, does reality exist as a single objective thing, or is it different for each person? Think about the simulated reality in the film The Matrix. Epistemology , on the other hand, is about “how” we can obtain knowledge and come to understand things – in other words, how can we figure out what reality is, and what the limits of this knowledge are. This is a gross oversimplification, but it’s a useful starting point (we’ll cover ontology and epistemology another post). With that fluffy stuff out the way, let’s look at three of the main research philosophies that operate on different ontological and epistemological assumptions:
These certainly aren’t the only research philosophies, but they are very common and provide a good starting point for understanding the spectrum of philosophies. ![]() Research Philosophy 1: PositivismPositivist research takes the view that knowledge exists outside of what’s being studied . In other words, what is being studied can only be done so objectively , and it cannot include opinions or personal viewpoints – the researcher doesn’t interpret, they only observe. Positivism states that there is only one reality and that all meaning is consistent between subjects. In the positivist’s view, knowledge can only be acquired through empirical research , which is based on measurement and observation. In other words, all knowledge is viewed as a posteriori knowledge – knowledge that is not reliant on human reasoning but instead is gained from research. For the positivist, knowledge can only be true, false, or meaningless . Basically, if something is not found to be true or false, it no longer holds any ground and is thus dismissed. Let’s look at an example, based on the question of whether God exists or not. Since positivism takes the stance that knowledge has to be empirically vigorous, the knowledge of whether God exists or not is irrelevant. This topic cannot be proven to be true or false, and thus this knowledge is seen as meaningless. Kinda harsh, right? Well, that’s the one end of the spectrum – let’s look at the other end. ![]() Research Philosophy 2: InterpretivismOn the other side of the spectrum, interpretivism emphasises the influence that social and cultural factors can have on an individual. This view focuses on people’s thoughts and ideas , in light of the socio-cultural backdrop. With the interpretivist philosophy, the researcher plays an active role in the study, as it’s necessary to draw a holistic view of the participant and their actions, thoughts and meanings. Let’s look at an example. If you were studying psychology, you may make use of a case study in your research which investigates an individual with a proposed diagnosis of schizophrenia. The interpretivist view would come into play here as social and cultural factors may influence the outcome of this diagnosis. Through your research, you may find that the individual originates from India, where schizophrenic symptoms like hallucinations are viewed positively, as they are thought to indicate that the person is a spirit medium. This example illustrates an interpretivist approach since you, as a researcher, would make use of the patient’s point of view, as well as your own interpretation when assessing the case study. Research Philosophy 3: PragmatismPragmatism highlights the importance of using the best tools possible to investigate phenomena. The main aim of pragmatism is to approach research from a practical point of view , where knowledge is not fixed, but instead is constantly questioned and interpreted. For this reason, pragmatism consists of an element of researcher involvement and subjectivity, specifically when drawing conclusions based on participants’ responses and decisions. In other words, pragmatism is not committed to (or limited by) one specific philosophy. Let’s look at an example in the form of the trolley problem, which is a set of ethical and psychological thought experiments. In these, participants have to decide on either killing one person to save multiple people or allowing multiple people to die to avoid killing one person. This experiment can be altered, including details such as the one person or the group of people being family members or loved ones. The fact that the experiment can be altered to suit the researcher’s needs is an example of pragmatism – in other words, the outcome of the person doing the thought experiment is more important than the philosophical ideas behind the experiment. ![]() Onion Layer 2: Research ApproachLet’s peel off another layer and take a look at the research approach . Your research approach is the broader method you’ll use for your research – inductive or deductive . It’s important to clearly identify your research approach as it will inform the decisions you take in terms of data collection and analysis in your study (we’ll get to that layer soon). Inductive approaches entail generating theories from research , rather than starting a project with a theory as a foundation. Deductive approaches, on the other hand, begin with a theory and aim to build on it (or test it) through research. Sounds a bit fluffy? Let’s look at two examples: An inductive approach could be used in the study of an otherwise unknown isolated community. There is very little knowledge about this community, and therefore, research would have to be conducted to gain information on the community, thus leading to the formation of theories. On the other hand, a deductive approach would be taken when investigating changes in the physical properties of animals over time, as this would likely be rooted in the theory of evolution. In other words, the starting point is a well-established pre-existing body of research. ![]() What’s the relevance of qualitative and quantitative data to research approaches? Well, inductive approaches are usually used within qualitative research, while quantitative research tends to reflect a deductive approach, usually informed by positivist philosophy. The reason for using a deductive approach here is that quantitative research typically begins with theory as a foundation, where progress is made through hypothesis testing. In other words, a wider theory is applied to a particular context, event, or observation to see whether these fit in with the theory, as with our example of evolution above. Need a helping hand?Onion Layer 3: Research StrategySo far, we’ve looked at pretty conceptual and intangible aspects of the onion. Now, it’s time to peel another layer off that onion and get a little more practical – introducing research strategy . This layer of the research onion details how, based on the aims of the study, research can be conducted. Note that outside of the onion, these strategies are referred to as research designs. There are several strategies you can take, so let’s have a look at some of them.
Strategy 1: Experimental researchExperimental research involves manipulating one variable (the independent variable ) to observe a change in another variable (the dependent variable ) – in other words, to assess the relationship between variables. The purpose of experimental research is to support, refute or validate a research hypothesis . This research strategy follows the principles of the scientific method and is conducted within a controlled environment or setting (for example, a laboratory). Experimental research aims to test existing theories rather than create new ones, and as such, is deductive in nature. Experimental research aligns with the positivist research philosophy, as it assumes that knowledge can only be studied objectively and in isolation from external factors such as context or culture. Let’s look at an example of experimental research. If you had a hypothesis that a certain brand of dog food can raise a dogs’ protein levels, you could make use of experimental research to compare the effects of the specific brand to a “regular” diet. In other words, you could test your hypothesis. In this example, you would have two groups, where one group consists of dogs with no changes to their diet (this is called the control group) and the other group consists of dogs being fed the specific brand that you aim to investigate (this is called the experimental/treatment group). You would then test your hypothesis by comparing the protein levels in both groups. ![]() Strategy 2: Action researchNext, we have action research . The simplest way of describing action research is by saying that it involves learning through… wait for it… action. Action research is conducted in practical settings such as a classroom, a hospital, a workspace, etc – as opposed to controlled environments like a lab. Action research helps to inform researchers of problems or weaknesses related to interactions within the real-world . With action research, there’s a strong focus on the participants (the people involved in the issue being studied, which is why it’s sometimes referred to as “participant action research” or PAR. An example of PAR is a community intervention (for therapy, farming, education, whatever). The researcher comes with an idea and it is implemented with the help of the community (i.e. the participants). The findings are then discussed with the community to see how to better the intervention. The process is repeated until the intervention works just right for the community. In this way, a practical solution is given to a problem and it is generated by the combination of researcher and community (participant) feedback. This kind of research is generally applied in the social sciences , specifically in professions where individuals aim to improve on themselves and the work that they are doing. Action research is most commonly adopted in qualitative studies and is rarely seen in quantitative studies. This is because, as you can see in the above examples, action research makes use of language and interactions rather than statistics and numbers. ![]() Strategy 3: Case study researchA case study is a detailed, in-depth study of a single subject – for example, a person, a group or an institution, or an event, phenomenon or issue. In this type of research, the subject is analysed to gain an in-depth understanding of issues in a real-life setting. The objective here is to gain an in-depth understanding within the context of the study – not (necessarily) to generalise the findings. It is vital that, when conducting case study research, you take the social context and culture into account, which means that this type of research is (more often than not) qualitative in nature and tends to be inductive. Also, since the researcher’s assumptions and understanding play a role in case study research, it is typically informed by an interpretivist philosophy. For example, a study on political views of a specific group of people needs to take into account the current political situation within a country and factors that could contribute towards participants taking a certain view. ![]() Strategy 4: Grounded theoryNext up, grounded theory. Grounded theory is all about “letting the data speak for itself”. In other words, in grounded theory, you let the data inform the development of a new theory, model or framework. True to the name, the theory you develop is “ grounded ” in the data. Ground theory is therefore very useful for research into issues that are completely new or under-researched. Grounded theory research is typically qualitative (although it can also use quantitative data) and takes an inductive approach. Typically, this form of research involves identifying commonalities between sets of data, and results are then drawn from completed research without the aim of fitting the findings in with a pre-existing theory or framework. For example, if you were to study the mythology of an unknown culture through artefacts, you’d enter your research without any hypotheses or theories, and rather work from the knowledge you gain from your study to develop these. ![]() Strategy 5: EthnographyEthnography involves observing people in their natural environments and drawing meaning from their cultural interactions. The objective with ethnography is to capture the subjective experiences of participants, to see the world through their eyes. Creswell (2013) says it best: “Ethnographers study the meaning of the behaviour, the language, and the interaction among members of the culture-sharing group.” For example, if you were interested in studying interactions on a mental health discussion board, you could use ethnography to analyse interactions and draw an understanding of the participants’ subjective experiences. For example, if you wanted to explore the behaviour, language, and beliefs of an isolated Amazonian tribe, ethnography could allow you to develop a complex, complete description of the social behaviours of the group by immersing yourself into the community, rather than just observing from the outside. Given the nature of ethnography, it generally reflects an interpretivist research philosophy and involves an inductive , qualitative research approach. However, there are exceptions to this – for example, quantitative ethnography as proposed by David Shafer. ![]() Strategy 6: Archival researchLast but not least is archival research. An archival research strategy draws from materials that already exist, and meaning is then established through a review of this existing data. This method is particularly well-suited to historical research and can make use of materials such as manuscripts and records. For example, if you were interested in people’s beliefs about so-called supernatural phenomena in the medieval period, you could consult manuscripts and records from the time, and use those as your core data set. As you can see, there is a wide range of choices in terms of research strategy. The right choice for your project will depend largely on your research aims and objectives, as well as the choices you make in terms of research philosophy and approach. Onion Layer 4: ChoicesThe next layer of the research onion is simply called “choices” – they could have been a little more specific, right? In any case, this layer is simply about deciding how many data types (qualitative or quantitative) you’ll use in your research. There are three options – mono , mixed , and multi-method . Let’s take a look at them. Choosing to use a mono method means that you’ll only make use of one data type – either qualitative or quantitative. For example, if you were to conduct a study investigating a community’s opinions on a specific pizza restaurant, you could make use of a qualitative approach only, so that you can analyse participants’ views and opinions of the restaurant. If you were to make use of both quantitative and qualitative data, you’d be taking a mixed-methods approach. Keeping with the previous example, you may also want to assess how many people in a community eat specific types of pizza. For this, you could make use of a survey to collect quantitative data and then analyse the results statistically, producing quantitative results in addition to your qualitative ones. Lastly, there’s multi-method . With a multi-method approach, you’d make use of a wider range of approaches, with more than just a one quantitative and one qualitative approach. For example, if you conduct a study looking at archives from a specific culture, you could make use of two qualitative methods (such as thematic analysis and content analysis ), and then additionally make use of quantitative methods to analyse numerical data. ![]() Onion Layer 5: Time horizonWhat’s that far in the distance? It’s the time horizon. But what exactly is it? Thankfully, this one’s pretty straightforward. The time horizon simply describes how many points in time you plan to collect your data at . Two options exist – the cross-sectional and longitudinal time horizon. Imagine that you’re wasting time on social media and think, “Ooh! I want to study the language of memes and how this language evolves over time”. For this study, you’d need to collect data over multiple points in time – perhaps over a few weeks, months, or even years. Therefore, you’d make use of a longitudinal time horizon. This option is highly beneficial when studying changes and progressions over time. If instead, you wanted to study the language used in memes at a certain point in time (for example, in 2020), you’d make use of a cross-sectional time horizon. This is where data is collected at one point in time, so you wouldn’t be gathering data to see how language changes, but rather what language exists at a snapshot point in time. The type of data collected could be qualitative, quantitative or a mix of both, as the focus is on the time of collection, not the data type. ![]() Onion Layer 6: Techniques and ProceduresFinally, we reach the centre of the onion – this is where you get down to the real practicalities of your research to make choices regarding specific techniques and procedures . Specifically, this is where you’ll:
What’s important to note here is that these techniques and procedures need to align with all the other layers of the research onion – i.e., research philosophy, research approaches, research strategy, choices, and time horizon. For example, you if you’re adopting a deductive, quantitative research approach, it’s unlikely that you’ll use interviews to collect your data, as you’ll want high-volume, numerical data (which surveys are far better suited to). So, you need to ensure that the decisions at each layer of your onion align with the rest, and most importantly, that they align with your research aims and objectives. ![]() Let’s Recap: Research Onion 101Saunder’s (2007) research onion details the many interrelated choices you’ll need to make when you’re crafting your research methodology. These include:
![]() Learn More About Methodology![]() Triangulation: The Ultimate Credibility EnhancerTriangulation is one of the best ways to enhance the credibility of your research. Learn about the different options here. ![]() Research Limitations 101: What You Need To KnowLearn everything you need to know about research limitations (AKA limitations of the study). Includes practical examples from real studies. ![]() In Vivo Coding 101: Full Explainer With ExamplesLearn about in vivo coding, a popular qualitative coding technique ideal for studies where the nuances of language are central to the aims. ![]() Process Coding 101: Full Explainer With ExamplesLearn about process coding, a popular qualitative coding technique ideal for studies exploring processes, actions and changes over time. ![]() Qualitative Coding 101: Inductive, Deductive & Hybrid CodingInductive, Deductive & Abductive Coding Qualitative Coding Approaches Explained... 📄 FREE TEMPLATES Research Topic Ideation Proposal Writing Literature Review Methodology & Analysis Academic Writing Referencing & Citing Apps, Tools & Tricks The Grad Coach Podcast 64 CommentsThis is good Wow this was sooo helpful. I don’t feel so blank about my research anymore. With this information I can conquer my research. Going ‘write’ into it. Get it write not right hahahaha Very good article . Thanks a million Do you use each of these as headings for your methodology section I am doing research with Bolton University so i would like to empower myself. Really thoughtful presentation and preparation. I learnt too much to teach my students in a very simple and understandable way Very useful, thank you. You’re most welcome. Good luck with your research! thanks alot for your brief and brilliant notes I am a Student at Malawi Institute of Management, pursuing a Masters’ degree in Business Administration. I find this to be very helpful Extremely useful, well explained. Thank you so much I would like to download this file… I can’t find the attachment file. Thanks Thank you so much for explaining it in the most simple and precise manner! Very thoughtful and well expained, thanks. This is good for upgrade my research knowledge I have enjoying your videos on YouTube, they are very educative and useful. I have learned a lot. Thanks Thank you this has really helped me with writing my dissertation methodology ! Thanks so much for this piece. Just to be clear, which layer do interviews fit in? well explained i found it to be very engaging. now i’m going to pass my research methods course. thank you. Thank you so much this has really helped as I can’t get this insight from uni due to covid well explained with more clarity! this is an excellent piece i find it super helpful Beautiful, thank you! Am from Malawi @ LAMAU University. I think this is so helpful and the info is applicable and simple to understand. Beautiful and helpful. Thank you! This is well done! A complex but useful approach to research simplified! I would like to learn more from the team. A very simplified version of a complex topic. I found it really helpful. I would like to know if this publication can be cited for academic research. Thank you You’re welcome to cite this page, but it would be better to cite the original work of Saunders. Thirteen odd years since my MSc in HRM & HRD at UoL. I’d like to say thank you for the effort to produce such an insightful discussion of a rather complex topic. I am a PhD in Media Studies student. I found this enormously helpful when stringing together the methodology chapter, especially the research philosophy section. Hello there. Thank you for summarising the work on the onion. A more recent version of the onion (Saunders et al., 2019) refers to ‘methodological choices’ rather than choices. This can be downloaded, along with the chapter dealing with research philosophies at: https://www.researchgate.net/publication/330760964_Research_Methods_for_Business_Students_Chapter_4_Understanding_research_philosophy_and_approaches_to_theory_development or https://www.academia.edu/42304065/Research_Methods_for_Business_Students_Chapter_4_Understanding_research_philosophy_and_approaches_to_theory_development_8th_edition Thank you Mark Saunders. Your work is very insightful Thank you for the update and additional reading Mark, very helpful indeed. THROUGHLY AND SIMPLY BRIEFED TO MAKE SENSE AND A CLEAR INSIGHT. THANK YOU, VERY MUCH. Thank you for the sharing the recent version of the Onion! I want to keep it in my reference of my assignment. May I?? Great summary, thank you taking the time to put this together. I’m sure it’s been a big help to lots of people. It definitely was to me. I love the analysis… some people do not recognize qualitative or quantitative as an approach but rather have inductive, abductive, and deductive. This has been helpful in the understanding of research . Thank you for this valuable information. Great summary. Well explained. Thank you, guys. This makes my fears on methodology go away. I confidently look forward to working on my methodology now. Thank you so much I ma doing a PhD with UNIMA, School of Education simple and clear Simple guide to crafting a research methodology. Quite impactful. Thank you Thank you for this, this makes things very clear. Now I’m off to conquer my research proposal. Thanks again. Thank you for this very informative and valuable information. What would the best approach be to take if you are using secondary data to form a qualitative study and relying on industry reports and peer journals to distinguish what factors influence the use of say cryptocurrency ? Thanks for providing the whole idea/knowledge in the simplest way with essential factors which made my entire research process more efficient as well as valuable. what is about research design such as descriptive, causal-comparative, correlation, developmental where these fall in the research onion? This is very helpful. Thank you for this wonderful piece. However, it would be nicer to have References to the knowledge provided here. My suggestion This material is very important for researchers, particularly for PhD scholars to conduct further study. This was insightful. Thank you for the knowledge. Thank you for the wonderful knowledge !Easy to understand and grasp. thanks very much very simple. will need a coach Hi this is a great article giving much help to my research. I just wanted to mention here that the example where you mentioned that ” schizophrenic symptoms like hallucinations are viewed positively, as they are thought to indicate the person is a spirit medium” is completely false as those are different cases and a bit out of context here. We are medically and psychologically well versed and obviously understand the difference between the two. As much as I am grateful to this article I would like to suggest you to give proper examples. Thank you very much, sincerely I appreciate your efforts, it is insightful information. Once again I’m grateful . In short, a complete insight of and for writing research methodology. This information was very helpful, I was having difficulties in writing my methodology now I can say I have the full knowledge to write a more informative research methodology. Thank you so much for this amazing explanation. As a person who hasn’t ever done a research project, this video helped me to clear my doubts and approach my research in a clear and concise manner. Great work very well explained , after going through this there is no need any material to study . a very concise and to the point. I have one small query. If I choose mixed -methods (quantitative and qualitative techniques), Then, my research Philosophy will also change to both Positivists and Interpretivist. Isn’t? well explained and thank you Thanks for this presentation. Quite simple and easy to understand, and to teach others. Hello! Having made a decision to use a particular research philosophy, how then do we go about justifying that choice with references? Thank you. Submit a Comment Cancel replyYour email address will not be published. Required fields are marked * Save my name, email, and website in this browser for the next time I comment. Submit Comment
BrainSTORM Week : Register now for our upcoming dSTORM webinar series! learn more Empowering curious minds, transforming discoveryYour super-resolution microscopy journey made simple ![]() Where are you in your super-resolution journey?ONI is here to support you along your super-resolution journey Answer a few simple questions to discover key resources that can help you make the most of single-molecule imaging technology across a wide range of applications I’m new to super-resolution microscopyWelcome to the world of super-res, how can you use super-resolution for your application or biological question, want to learn more about its uses, i’m planning my experiment, find what technique might be more suitable for your biological question, key considerations for sample preparation, want more technical and step-by-step details, choose your technique, what biological information do you need, what type of sample do you have, what's the size of your structure of interest, do you need multicolor imaging, your technique. Within the Nanoimager, TIRF imaging might provide you with the necessary information within a diffraction-limited context. SIM or Airyscan might also be sufficient in this case, you can get resolution down to 250 nm (SIM) and 350 nm (Airyscan). For smaller structures, single-molecule localization techniques (SMLM) like dSTORM or PAINT would be highly recommended to obtain high precision results. If advanced microscopy is not available, expansion Microscopy could be used in this case. For smaller structures, PALM imaging could be used here. PAINT is recommended in live cell mostly to quantify outer membrane structures via protein-protein interactions or weak interactions (uPAINT, glyco-PAINT, peptide-PAINT) dSTORM imaging is the most suitable option in this case. It can achieve resolutions under 20 nm, allowing the visualization of structures and localization of molecules with great detail. It is compatible with multicolor imaging (up to 3) if needed. PALM imaging is the most suitable option in this case. It can achieve resolution under 20 nm in both live or fixed samples. DNA-PAINT might be the most suitable option in this case. It can achieve resolutions under 20 nm in both fixed or live sample, and is compatible with multicolor imaging. The lack of photobleaching due to continuously replenishing of fluorophores is also a benefit from PAINT. Single Particle Tracking (SPT) , usually combined with PALM , is the best option to follow the live dynamics of single molecules. Two-color SPT is also an available option. STED for live cell imaging or in vivo STED for in vivo imaging can be helpful here. STED can reach temporal resolutions of < 50 ms SIM for live cell imaging or in vivo SIM for in vivo imaging can be helpful here. SIm can reach temporal resolution of < 100 ns smFRET seems the best option in this case, it enables distances between single molecules to be measured at a scale of 1-10 nm. Intra- and inter-molecular distances and stoichiometries can measured PALM imaging could be an alternative to follow live molecular interactions, below 100 nm in axial resolution. For quantitative results, smFRET would be more suitable. PALM imaging combined with Single Particle Tracking (SPT) could be an alternative to follow live molecular interactions, below 100 nm in axial resolution and for single molecules. For quantitative results, smFRET would be more suitable. Unfortunately, we cannot find the technology you need. Please contact us for details I’m ready to imageDouble check you have everything you need, master imaging techniques within the nanoimager, i want to analyze my data, what are you looking to do. Most single-molecule localization microscopy techniques, such as dSTORM , PAINT or PALM , allow the acquisition of high-resolution images (~20 nm) that provide morphological information of structures and the organization of proteins within them. For instance, to assess the distribution of a specific protein within a structure, a simple density profile measurement can be performed. 3D imaging and visualization further enhances the nanoscale information of structure morphology and protein organization. One of the most powerful applications of single molecule localization microscopy (SMLM) is investigating the spatial distribution of features down to the nanometer scale. The raw data obtained from SMLM experiments, such as dSTORM imaging, is a point cloud of localizations. This serves as the basis of nanoscale morphological analysis. We can use different clustering algorithms to detect the features of interest in the data. On ONI’s cloud-based CODI software, we offer different clustering analyses including DBSCAN, HDBSCAN and our in-house extension of HDBSCAN called Constrained clustering. Clustering on localization data allows researchers to find biological structures and quantify their morphological properties (size and shape), as well as determine presence/absence, even down to the level of expression, for specific markers of interest. You can find out more about clustering on this blog post. The nanoscale resolution and clustering-based identification of structures allows investigation on the spatial relationship between structures and proteins. This powerful information can be extracted with different algorithms, such as K-Nearest Neighbors (KNN), which uses proximity to classify clusters. Please reach out to our team to find out more about using this and similar tools on our platform. A critical part of SMLM imaging is to remove false positive localizations or noise to obtain accurate information and sensitivity for the analysis. To this end, the CODI analysis workflow includes a single-molecule filtering step that enables real-time visualization adaptation as users tune the filtering parameters. You can use the SPT tracking functions within NimOS to detect fluorescent signals that form tracks. This allows users to follow biomolecule movement and extract particle speed and size distributions. For advanced analysis, Trackmate is a popular tool used by researchers. PALM imaging coupled with Single Particle Tracking informs us how two molecules interact and behave over time in a live-cell environment. The NimOS tracking analysis allows a quick and in-depth analysis for the dynamic behavior of the biomolecules. There are different ways to understand interactions between biomolecules. smFRET enables distance-based kinetic measurements between single molecules within a distance of 1-10 nm each, as well as intra- and inter-molecular distances and stoichiometries. The Nanoimager software NimOS enables smFRET analysis as soon as the acquisition has finished, allowing a quick evaluation to make a quick and informative decision. The EV Profiling App on CODI is the perfect analysis workflow for EV samples with various subpopulations. The App can quantify size and biomarker distributions from a collection of single EVs. The App includes a range of tools from drift correction, single-molecule filtering, clustering, cluster filtering, and counting of biomarker distribution. In addition, the batch analysis tool allows analysis automation by applying the same analysis settings to multiple datasets. Our cloud-based software platform called CODI stands for Collaborative Discovery. We are always happy to hear about features or tools that you would like to use for your applications. Our CODI platform allows you to download images directly for publications with changeable color schemes, localisation sizes and contrast along with a scale bar. High-resolution image export is also available from NimOS, with file formats compatible with several third party softwares, such as FIJI ImageJ, Adobe Illustrator, and Affinity Designer. Our CODI platform provides researchers with the possibility of sharing datasets, with different degrees of privacy (from close collaborators to fully public). This enables others to not only visualize datasets but also how they have been processed to reach the scientific conclusions. You can also explore our publications section on the website, with over 200 papers published with ONI products. Our CODI platform provides researchers with the possibility of sharing datasets and analyses, and setting different levels of privacy as desired; thereby providing a one-end collaborative platform for the super-resolution community. ![]() The NanoimagerSeveral super-resolution imaging modalities in one compact benchtop microscope. dSTORM, PALM, PAINT, Single-Particle Tracking, smFRET, TIRF, HILO… explore its possibilities for your research! ![]() Application-specific and customizable sample preparation with fully automated fluidic control and the most user-centric, reliable, and reproducible end-to-end SMLM fluidics solution on the market today. ![]() Start your complete super-resolution experience using our sample preparation kits to help you fix and label your samples: from cells to nanoparticles. ![]() CODI is your cloud-based analysis platform, where you can collaborate with colleagues by sharing data and analysis workflows from anywhere. ![]() The Nanoimager is a class 1 laser product that can be used in a standard lab, without specific laser training or the need for a dark room. Simply plug it in and image anywhere! No, you can do a lot more. The Nanoimager allows you to investigate your sample using different imaging technologies on both fixed or live samples. Yes. Our consumable kits largely simplify sample preparation and these can be compatible with other imaging systems with equivalent specifications. However, all our kits are optimized for use with the Nanoimager. Subscribe to our mailing list
Register for the NCI Genomic Data Commons Analysis Tool ChallengeDon’t miss the chance to mix collaboration, competition, and tool development in the NCI Genomic Data Commons (GDC) Analysis Tool Challenge . By participating in the challenge, you will:
The GDC Data Portal Analysis Center will feature the winning tools and make them available to a broad audience of cancer researchers. SUBSCRIBE TO UPDATES![]() Diet Research Data:Effects of Quick Fat Diet on Male and Female ICR Mice ![]() 1. Body Weight 2. Food and Water Intake 3. Fasting Blood Glucose Levels 4. Oral Glucose Tolerance Test 5. Insulin Tolerance Test 6. Blood Analysis at Biopsy 7. Major Organ Weights at Biopsy Related CLEA Japan product: Quick Fat![]() 1.Objective2.materials and methods. (1)Experimental Site The experiment was conducted in the mouse breeding room (conventional) of the Central Research Institute of Nippon Compound Feed Co., Ltd. (currently Feed One Co., Ltd.).
Male and female Jcl:ICR mice were introduced at 3 weeks of age and acclimatized for 1 week. After that, they were divided into two groups (control group: fed CE-2, experimental group: fed QF) based on body weight, and fed their respective diets ad libitum from 4 to 25 weeks of age. The number of mice in each group was 8 for both males and females.
(5)Experimental Procedures During the feeding period, body weight, food intake, and water intake were measured weekly. Blood samples were collected from the tail vein after a 20-hour fast at 4, 6, 8, 10, 14, 18, and 22 weeks of age. At the end of the feeding period (25 weeks of age), an oral glucose tolerance test (glucose dose = 2 g/kg body weight) was performed, followed by Biopsy of 6 mice per group. After a 20-hour fast under ether anesthesia, blood was collected from the posterior vena cava, and major organs and peritoneal adipose tissue were removed. Blood was stored as serum for the measurement of lipid metabolism-related parameters (triglycerides, free fatty acids, total cholesterol, esterified cholesterol, free cholesterol, and phospholipids). Student's t-test was used for statistical analysis. 1.Body WeightDifferences in Body Weight Changes Between Sexes Body weight was measured weekly. The points and vertical lines in the figure represent the mean ± standard error. Statistical analysis was performed between groups within the same sex at each age, and * indicates a significant difference (p < 0.05). ![]() 2.Food and Water IntakeDifferences in Food Intake Between Sexes The points and vertical lines in the figure represent the mean ± standard error. ![]() Differences in Water Intake Between Sexes ![]() 3.Fasting Blood Glucose LevelsDifferences in Fasting Blood Glucose Levels Between Sexes Blood samples were collected from the tail vein every 2 weeks at 1 pm, 4 hours after lights on. Whole blood was measured using a whole blood glucose meter (Glucotest PROR / Sanwa Kagaku Kenkyusho Co., Ltd.). The vertical bars and lines in the figure represent the mean ± standard error. Statistical analysis was performed between groups within the same sex at each age, and * indicates a significant difference (p < 0.05). ![]() 4.Oral Glucose Tolerance TestResults of Oral Glucose Tolerance Test in ICR Mice at 25 Weeks of Age - 1 After an overnight fast (20 hours), a glucose solution (2g/kg body weight) was orally administered via gastric gavage, and blood was collected from the tail vein before administration (0 min) and 30, 60, and 120 min after administration. The obtained blood was stored as plasma at -40°C. Blood glucose levels were measured using the Glucose CⅡ - Test Wako (Wako Pure Chemical Industries, Ltd.). The points and vertical lines in the figure represent the mean ± standard error. Statistical analysis was performed between groups at each time point, and * indicates a significant difference (p < 0.05). ![]() Results of Oral Glucose Tolerance Test in ICR Mice at 25 Weeks of Age – 2 【Sum of Blood Glucose Levels and Blood Glucose Increment Area】 The sum of blood glucose levels was calculated for each time point during the oral glucose tolerance test. The blood glucose increment area was calculated using the following formula: Blood glucose increment area (mg/dl・h) = {(a + 2b + 3c + 2d) × 1/4} - 2a (where a, b, c, and d are the blood glucose levels at 0, 30, 60, and 120 minutes, respectively) The vertical bars and lines in the figure represent the mean ± standard error. Statistical analysis was performed between groups, and * indicates a significant difference (p < 0.05). ![]() 5.Insulin Tolerance TestResults of Insulin Tolerance Test in ICR Mice at 25 Weeks of Age An insulin solution (0.75 IU/kg body weight) was intraperitoneally injected, and blood was collected from the tail vein before administration (0 min) and 20, 40, 60, 80, and 120 min after administration. Whole blood glucose levels were measured using a whole blood glucose meter (Glucotest PROR / Sanwa Kagaku Kenkyusho Co., Ltd.). The points and vertical lines in the figure represent the mean ± standard error. Statistical analysis was performed between groups at each time point, and * indicates a significant difference (p < 0.05). ![]() 6.Blood Analysis at BiopsySerum and Plasma Analysis at Biopsy The values in the table represent the mean ± standard error. At 25 weeks of age, blood was collected after a 20-hour fast under ether anesthesia. Blood was collected from the posterior vena cava and stored as plasma and serum. Statistical analysis was performed between groups for each item, and * indicates a significant difference (p < 0.05). ![]() 7.Major Organ Weights at BiopsyOrgan Weights The values in the table represent the mean ± standard error. Statistical analysis was performed between groups for each item, and * indicates a significant difference (p < 0.05). ![]() おすすめ記事 / Recommended articles![]() Diet Research Data:Effects of Quick Fat Diet on Female C57BL/6J Mice ![]() Diet Research Data:Effects of Quick Fat Diet on Male C57BL/6J Mice アーカイブ / Archives
カテゴリー / Categories
実験動物や関連の飼料、器材、受託業務に関するご質問やご相談を承ります。どうぞ、お気軽にお問い合わせください。 Federal health transfers outpace provincial spending despite premiers' criticisms, analysis showsFindings contrast the rhetoric that has punctuated federal and provincial health negotiations. ![]() Social SharingDespite castigation from provincial premiers over lagging federal contributions to health spending, an analysis of 20 years of health funding data shows that federal transfers have mostly outpaced increases to provincial health budgets. In 2023, federal health transfers amounted to $47.1 billion, a 212 per cent increase over 2005, when the transfers were $15.1 billion. Total spending by all 10 provinces grew in that time to $221.9 billion up from $86.2 billion, an increase of 158 per cent. The Canadian Press, in partnership with Humber College StoryLab, collected data on provincial health budgets and federal health transfers from 2004 to 2023 to track annual spending from the launch of the 2004 federal-provincial health accord under former Liberal prime minister Paul Martin. The findings stand in stark contrast to the rhetoric that has punctuated federal and provincial health negotiations over the last several years, as health systems struggled in the aftermath of the COVID-19 pandemic. Two years ago, a shortage of health workers led to emergency room closures and extreme backlogs for services across the country and premiers demanded the federal government pay a greater share of the health spending bill. ![]() Ontario opposition leader concerned over 1.3% increase in health-care budgetFormer Manitoba premier Heather Stefanson, after a meeting with her fellow provincial leaders at the end of 2022, said health spending used to be split evenly but the federal share had slowly dwindled over time. Governments originally envisioned that health-care costs would be divided evenly between Ottawa and provincial governments in 1959, before most provinces even had medicare. But the funding model shifted drastically in the 1970s and has changed again many times since. Rather than slowly dropping off over the last two decades as the premiers suggested, the data shows federal transfers actually grew at a slightly faster pace than provincial health spending since the Martin health accord in 2004. In 2005-06, federal health transfers grew 39 per cent in one year while provincial health spending grew by six per cent. That meant the federal share of total health spending jumped to 20.7 per cent from 17.5 per cent. Federal health-care spending was far higher during the COVID-19 pandemic because of specific transfers. Those extra funds stopped flowing in 2022-23, by which time the federal share of total provincial spending had grown just slightly to 21.2 per cent. ![]() Covering cost of prescriptions can lower health-care costs, research suggestsThat reality wasn't acknowledged when premiers were clamouring for more federal money after the pandemic, federal Health Minister Mark Holland said in a recent interview. It was also not acknowledged in his recent negotiations with provinces as part of Prime Minister Justin Trudeau's proposed $196-billion health deal, which involved signing one-on-one agreements with each province. "I understand the position of the provinces — huge demands on them — but we have been ensuring that we're providing the dollars that are necessary and required to help them in their health systems," Holland said. "Now what we need to do is to begin to transform how our system functions. We need to move from a crisis-based system where we wait until people are really sick and then we deal with it, to being upstream and avoiding illness and being engaged in prevention." Ontario Premier Doug Ford declined The Canadian Press's interview request as chair of the Council of the Federation, the official organization of the premiers.
A written statement said premiers "continue to urge the federal government to provide adequate and sustainable health-care funding," also reiterating their concerns that the agreements have an end date. The premiers call that the "funding cliff," fearing they can't plan for long-term stability when federal offers all have expiration dates. In February 2023, about 10 days after Trudeau tabled the latest health funding offer, the premiers issued a joint statement to reluctantly accept it. "While this first step marks a positive development, the federal approach will clearly not address structural health care funding needs, nor long-term sustainability challenges we face in our health-care systems across the country," they wrote. ![]() Who is contributing, and how much?Getting a clear view of who is paying the growing cost of Canada's health care isn't straightforward. No government is collecting health spending data on a national scale, and federal contributions are difficult to pin down. It's important to know how much each government is contributing so that voters can hold them accountable, said Haizhen Mou, a professor with the University of Saskatchewan's graduate school of public policy. "They have certain expectations on the quality and quantity of health care they receive, however, they cannot hold either level of government accountable, because there's no clear division of responsibility," said Mou, who studies health funding and politics. "There's no clear, no transparent contribution ratio or expectation for this contribution from either government in the system so far." ![]() The Canadian Press and Humber College StoryLab combed through decades of provincial public accounts and federal transfers to compile the data manually. Territories were not included because health spending records couldn't be verified in some cases. The territories also receive additional support from the federal government to fund necessary travel and accommodations for some patients that can't be treated near their homes. The analysis did not account for equalization payments and other federal contributions to provincial general revenues that could ultimately be spent on health. Nor did it look at tax points transfers, which the federal government includes when it assesses how much money it is giving the provinces for health care. That dates back to 1977, when the federal government lowered its tax rates for personal and corporate income and the provinces could increase their provincial tax rates and take that revenue instead. In 2023, after the latest health funding offer to the premiers was made public, Ottawa said tax point transfers amounted to $25 billion. However, the provinces do not include tax point transfers when discussing the federal share of health-care spending. Holland says he's open to finding a way to make information about health spending more available as a way to cut through the political rhetoric. "I think anything that provides transparency and allows us to get to talking about the material, consequential things that we have to be doing, as opposed to debating over dollar values, I think is helpful," he said. ![]() Why Nova Scotia’s budget doesn’t skimp on health-care spendingThe new health deals call on provinces to improve the collection of national health data, but makes no specific mention of tracking federal and provincial spending. The one thing that is clear is that health care spending is growing. Per capita, Canada's transfers for health grew six times faster than population growth, amounting to $1,115 per person in 2023, up from $427 per person in 2005. Those figures have not been adjusted for inflation. Among the provinces, per capita spending grew at massively different rates, with Newfoundland's budget soaring 19 times faster than its population, while spending in Nova Scotia and Alberta grew less than two times as fast as the population. Related Stories![]() NASA’s Mini BurstCube Mission Detects Mega BlastThe shoebox-sized BurstCube satellite has observed its first gamma-ray burst, the most powerful kind of explosion in the universe, according to a recent analysis of observations collected over the last several months. “We’re excited to collect science data,” said Sean Semper, BurstCube’s lead engineer at NASA’s Goddard Space Flight Center in Greenbelt, Maryland. “It’s an important milestone for the team and for the many early career engineers and scientists that have been part of the mission.” The event, called GRB 240629A, occurred on June 29 in the southern constellation Microscopium. The team announced the discovery in a GCN (General Coordinates Network) circular on August 29. ![]() BurstCube deployed into orbit April 18 from the International Space Station, following a March 21 launch . The mission was designed to detect, locate, and study short gamma-ray bursts , brief flashes of high-energy light created when superdense objects like neutron stars collide. These collisions also produce heavy elements like gold and iodine, an essential ingredient for life as we know it. BurstCube is the first CubeSat to use NASA’s TDRS (Tracking and Data Relay Satellite) system, a constellation of specialized communications spacecraft. Data relayed by TDRS (pronounced “tee-driss”) help coordinate rapid follow-up measurements by other observatories in space and on the ground through NASA’s GCN . BurstCube also regularly beams data back to Earth using the Direct to Earth system — both it and TDRS are part of NASA’s Near Space Network . After BurstCube deployed from the space station, the team discovered that one of the two solar panels failed to fully extend. It obscures the view of the mission’s star tracker, which hinders orienting the spacecraft in a way that minimizes drag. The team originally hoped to operate BurstCube for 12-18 months, but now estimates the increased drag will cause the satellite to re-enter the atmosphere in September. “I’m proud of how the team responded to the situation and is making the best use of the time we have in orbit,” said Jeremy Perkins, BurstCube’s principal investigator at Goddard. “Small missions like BurstCube not only provide an opportunity to do great science and test new technologies, like our mission’s gamma-ray detector, but also important learning opportunities for the up-and-coming members of the astrophysics community.” BurstCube is led by Goddard. It’s funded by the Science Mission Directorate’s Astrophysics Division at NASA Headquarters. The BurstCube collaboration includes: the University of Alabama in Huntsville; the University of Maryland, College Park; the Universities Space Research Association in Washington; the Naval Research Laboratory in Washington; and NASA’s Marshall Space Flight Center in Huntsville. By Jeanette Kazmierczak NASA’s Goddard Space Flight Center , Greenbelt, Md. Media Contact: Claire Andreoli 301-286-1940 [email protected] NASA’s Goddard Space Flight Center, Greenbelt, Md. Related Terms
IEEE Account
Purchase Details
Profile Information
A not-for-profit organization, IEEE is the world's largest technical professional organization dedicated to advancing technology for the benefit of humanity. © Copyright 2024 IEEE - All rights reserved. Use of this web site signifies your agreement to the terms and conditions. Thank you for visiting nature.com. You are using a browser version with limited support for CSS. To obtain the best experience, we recommend you use a more up to date browser (or turn off compatibility mode in Internet Explorer). In the meantime, to ensure continued support, we are displaying the site without styles and JavaScript.
Knowledge mapping and evolution of research on older adults’ technology acceptance: a bibliometric study from 2013 to 2023
Humanities and Social Sciences Communications volume 11 , Article number: 1115 ( 2024 ) Cite this article Metrics details
The rapid expansion of information technology and the intensification of population aging are two prominent features of contemporary societal development. Investigating older adults’ acceptance and use of technology is key to facilitating their integration into an information-driven society. Given this context, the technology acceptance of older adults has emerged as a prioritized research topic, attracting widespread attention in the academic community. However, existing research remains fragmented and lacks a systematic framework. To address this gap, we employed bibliometric methods, utilizing the Web of Science Core Collection to conduct a comprehensive review of literature on older adults’ technology acceptance from 2013 to 2023. Utilizing VOSviewer and CiteSpace for data assessment and visualization, we created knowledge mappings of research on older adults’ technology acceptance. Our study employed multidimensional methods such as co-occurrence analysis, clustering, and burst analysis to: (1) reveal research dynamics, key journals, and domains in this field; (2) identify leading countries, their collaborative networks, and core research institutions and authors; (3) recognize the foundational knowledge system centered on theoretical model deepening, emerging technology applications, and research methods and evaluation, uncovering seminal literature and observing a shift from early theoretical and influential factor analyses to empirical studies focusing on individual factors and emerging technologies; (4) moreover, current research hotspots are primarily in the areas of factors influencing technology adoption, human-robot interaction experiences, mobile health management, and aging-in-place technology, highlighting the evolutionary context and quality distribution of research themes. Finally, we recommend that future research should deeply explore improvements in theoretical models, long-term usage, and user experience evaluation. Overall, this study presents a clear framework of existing research in the field of older adults’ technology acceptance, providing an important reference for future theoretical exploration and innovative applications. Similar content being viewed by others![]() Research progress and intellectual structure of design for digital equity (DDE): A bibliometric analysis based on citespace![]() Exploring the role of interaction in older-adult service innovation: insights from the testing stage![]() Smart device interest, perceived usefulness, and preferences in rural Alabama seniorsIntroduction. In contemporary society, the rapid development of information technology has been intricately intertwined with the intensifying trend of population aging. According to the latest United Nations forecast, by 2050, the global population aged 65 and above is expected to reach 1.6 billion, representing about 16% of the total global population (UN 2023 ). Given the significant challenges of global aging, there is increasing evidence that emerging technologies have significant potential to maintain health and independence for older adults in their home and healthcare environments (Barnard et al. 2013 ; Soar 2010 ; Vancea and Solé-Casals 2016 ). This includes, but is not limited to, enhancing residential safety with smart home technologies (Touqeer et al. 2021 ; Wang et al. 2022 ), improving living independence through wearable technologies (Perez et al. 2023 ), and increasing medical accessibility via telehealth services (Kruse et al. 2020 ). Technological innovations are redefining the lifestyles of older adults, encouraging a shift from passive to active participation (González et al. 2012 ; Mostaghel 2016 ). Nevertheless, the effective application and dissemination of technology still depends on user acceptance and usage intentions (Naseri et al. 2023 ; Wang et al. 2023a ; Xia et al. 2024 ; Yu et al. 2023 ). Particularly, older adults face numerous challenges in accepting and using new technologies. These challenges include not only physical and cognitive limitations but also a lack of technological experience, along with the influences of social and economic factors (Valk et al. 2018 ; Wilson et al. 2021 ). User acceptance of technology is a significant focus within information systems (IS) research (Dai et al. 2024 ), with several models developed to explain and predict user behavior towards technology usage, including the Technology Acceptance Model (TAM) (Davis 1989 ), TAM2, TAM3, and the Unified Theory of Acceptance and Use of Technology (UTAUT) (Venkatesh et al. 2003 ). Older adults, as a group with unique needs, exhibit different behavioral patterns during technology acceptance than other user groups, and these uniquenesses include changes in cognitive abilities, as well as motivations, attitudes, and perceptions of the use of new technologies (Chen and Chan 2011 ). The continual expansion of technology introduces considerable challenges for older adults, rendering the understanding of their technology acceptance a research priority. Thus, conducting in-depth research into older adults’ acceptance of technology is critically important for enhancing their integration into the information society and improving their quality of life through technological advancements. Reviewing relevant literature to identify research gaps helps further solidify the theoretical foundation of the research topic. However, many existing literature reviews primarily focus on the factors influencing older adults’ acceptance or intentions to use technology. For instance, Ma et al. ( 2021 ) conducted a comprehensive analysis of the determinants of older adults’ behavioral intentions to use technology; Liu et al. ( 2022 ) categorized key variables in studies of older adults’ technology acceptance, noting a shift in focus towards social and emotional factors; Yap et al. ( 2022 ) identified seven categories of antecedents affecting older adults’ use of technology from an analysis of 26 articles, including technological, psychological, social, personal, cost, behavioral, and environmental factors; Schroeder et al. ( 2023 ) extracted 119 influencing factors from 59 articles and further categorized these into six themes covering demographics, health status, and emotional awareness. Additionally, some studies focus on the application of specific technologies, such as Ferguson et al. ( 2021 ), who explored barriers and facilitators to older adults using wearable devices for heart monitoring, and He et al. ( 2022 ) and Baer et al. ( 2022 ), who each conducted in-depth investigations into the acceptance of social assistive robots and mobile nutrition and fitness apps, respectively. In summary, current literature reviews on older adults’ technology acceptance exhibit certain limitations. Due to the interdisciplinary nature and complex knowledge structure of this field, traditional literature reviews often rely on qualitative analysis, based on literature analysis and periodic summaries, which lack sufficient objectivity and comprehensiveness. Additionally, systematic research is relatively limited, lacking a macroscopic description of the research trajectory from a holistic perspective. Over the past decade, research on older adults’ technology acceptance has experienced rapid growth, with a significant increase in literature, necessitating the adoption of new methods to review and examine the developmental trends in this field (Chen 2006 ; Van Eck and Waltman 2010 ). Bibliometric analysis, as an effective quantitative research method, analyzes published literature through visualization, offering a viable approach to extracting patterns and insights from a large volume of papers, and has been widely applied in numerous scientific research fields (Achuthan et al. 2023 ; Liu and Duffy 2023 ). Therefore, this study will employ bibliometric methods to systematically analyze research articles related to older adults’ technology acceptance published in the Web of Science Core Collection from 2013 to 2023, aiming to understand the core issues and evolutionary trends in the field, and to provide valuable references for future related research. Specifically, this study aims to explore and answer the following questions: RQ1: What are the research dynamics in the field of older adults’ technology acceptance over the past decade? What are the main academic journals and fields that publish studies related to older adults’ technology acceptance? RQ2: How is the productivity in older adults’ technology acceptance research distributed among countries, institutions, and authors? RQ3: What are the knowledge base and seminal literature in older adults’ technology acceptance research? How has the research theme progressed? RQ4: What are the current hot topics and their evolutionary trajectories in older adults’ technology acceptance research? How is the quality of research distributed? Methodology and materialsResearch method. In recent years, bibliometrics has become one of the crucial methods for analyzing literature reviews and is widely used in disciplinary and industrial intelligence analysis (Jing et al. 2023 ; Lin and Yu 2024a ; Wang et al. 2024a ; Xu et al. 2021 ). Bibliometric software facilitates the visualization analysis of extensive literature data, intuitively displaying the network relationships and evolutionary processes between knowledge units, and revealing the underlying knowledge structure and potential information (Chen et al. 2024 ; López-Robles et al. 2018 ; Wang et al. 2024c ). This method provides new insights into the current status and trends of specific research areas, along with quantitative evidence, thereby enhancing the objectivity and scientific validity of the research conclusions (Chen et al. 2023 ; Geng et al. 2024 ). VOSviewer and CiteSpace are two widely used bibliometric software tools in academia (Pan et al. 2018 ), recognized for their robust functionalities based on the JAVA platform. Although each has its unique features, combining these two software tools effectively constructs mapping relationships between literature knowledge units and clearly displays the macrostructure of the knowledge domains. Particularly, VOSviewer, with its excellent graphical representation capabilities, serves as an ideal tool for handling large datasets and precisely identifying the focal points and hotspots of research topics. Therefore, this study utilizes VOSviewer (version 1.6.19) and CiteSpace (version 6.1.R6), combined with in-depth literature analysis, to comprehensively examine and interpret the research theme of older adults’ technology acceptance through an integrated application of quantitative and qualitative methods. Data sourceWeb of Science is a comprehensively recognized database in academia, featuring literature that has undergone rigorous peer review and editorial scrutiny (Lin and Yu 2024b ; Mongeon and Paul-Hus 2016 ; Pranckutė 2021 ). This study utilizes the Web of Science Core Collection as its data source, specifically including three major citation indices: Science Citation Index Expanded (SCIE), Social Sciences Citation Index (SSCI), and Arts & Humanities Citation Index (A&HCI). These indices encompass high-quality research literature in the fields of science, social sciences, and arts and humanities, ensuring the comprehensiveness and reliability of the data. We combined “older adults” with “technology acceptance” through thematic search, with the specific search strategy being: TS = (elder OR elderly OR aging OR ageing OR senile OR senior OR old people OR “older adult*”) AND TS = (“technology acceptance” OR “user acceptance” OR “consumer acceptance”). The time span of literature search is from 2013 to 2023, with the types limited to “Article” and “Review” and the language to “English”. Additionally, the search was completed by October 27, 2023, to avoid data discrepancies caused by database updates. The initial search yielded 764 journal articles. Given that searches often retrieve articles that are superficially relevant but actually non-compliant, manual screening post-search was essential to ensure the relevance of the literature (Chen et al. 2024 ). Through manual screening, articles significantly deviating from the research theme were eliminated and rigorously reviewed. Ultimately, this study obtained 500 valid sample articles from the Web of Science Core Collection. The complete PRISMA screening process is illustrated in Fig. 1 . ![]() Presentation of the data culling process in detail. Data standardizationRaw data exported from databases often contain multiple expressions of the same terminology (Nguyen and Hallinger 2020 ). To ensure the accuracy and consistency of data, it is necessary to standardize the raw data (Strotmann and Zhao 2012 ). This study follows the data standardization process proposed by Taskin and Al ( 2019 ), mainly executing the following operations: (1) Standardization of author and institution names is conducted to address different name expressions for the same author. For instance, “Chan, Alan Hoi Shou” and “Chan, Alan H. S.” are considered the same author, and distinct authors with the same name are differentiated by adding identifiers. Diverse forms of institutional names are unified to address variations caused by name changes or abbreviations, such as standardizing “FRANKFURT UNIV APPL SCI” and “Frankfurt University of Applied Sciences,” as well as “Chinese University of Hong Kong” and “University of Hong Kong” to consistent names. (2) Different expressions of journal names are unified. For example, “International Journal of Human-Computer Interaction” and “Int J Hum Comput Interact” are standardized to a single name. This ensures consistency in journal names and prevents misclassification of literature due to differing journal names. Additionally, it involves checking if the journals have undergone name changes in the past decade to prevent any impact on the analysis due to such changes. (3) Keywords data are cleansed by removing words that do not directly pertain to specific research content (e.g., people, review), merging synonyms (e.g., “UX” and “User Experience,” “aging-in-place” and “aging in place”), and standardizing plural forms of keywords (e.g., “assistive technologies” and “assistive technology,” “social robots” and “social robot”). This reduces redundant information in knowledge mapping. Bibliometric results and analysisDistribution power (rq1), literature descriptive statistical analysis. Table 1 presents a detailed descriptive statistical overview of the literature in the field of older adults’ technology acceptance. After deduplication using the CiteSpace software, this study confirmed a valid sample size of 500 articles. Authored by 1839 researchers, the documents encompass 792 research institutions across 54 countries and are published in 217 different academic journals. As of the search cutoff date, these articles have accumulated 13,829 citations, with an annual average of 1156 citations, and an average of 27.66 citations per article. The h-index, a composite metric of quantity and quality of scientific output (Kamrani et al. 2021 ), reached 60 in this study. Trends in publications and disciplinary distributionThe number of publications and citations are significant indicators of the research field’s development, reflecting its continuity, attention, and impact (Ale Ebrahim et al. 2014 ). The ranking of annual publications and citations in the field of older adults’ technology acceptance studies is presented chronologically in Fig. 2A . The figure shows a clear upward trend in the amount of literature in this field. Between 2013 and 2017, the number of publications increased slowly and decreased in 2018. However, in 2019, the number of publications increased rapidly to 52 and reached a peak of 108 in 2022, which is 6.75 times higher than in 2013. In 2022, the frequency of document citations reached its highest point with 3466 citations, reflecting the widespread recognition and citation of research in this field. Moreover, the curve of the annual number of publications fits a quadratic function, with a goodness-of-fit R 2 of 0.9661, indicating that the number of future publications is expected to increase even more rapidly. ![]() A Trends in trends in annual publications and citations (2013–2023). B Overlay analysis of the distribution of discipline fields. Figure 2B shows that research on older adults’ technology acceptance involves the integration of multidisciplinary knowledge. According to Web of Science Categories, these 500 articles are distributed across 85 different disciplines. We have tabulated the top ten disciplines by publication volume (Table 2 ), which include Medical Informatics (75 articles, 15.00%), Health Care Sciences & Services (71 articles, 14.20%), Gerontology (61 articles, 12.20%), Public Environmental & Occupational Health (57 articles, 11.40%), and Geriatrics & Gerontology (52 articles, 10.40%), among others. The high output in these disciplines reflects the concentrated global academic interest in this comprehensive research topic. Additionally, interdisciplinary research approaches provide diverse perspectives and a solid theoretical foundation for studies on older adults’ technology acceptance, also paving the way for new research directions. Knowledge flow analysisA dual-map overlay is a CiteSpace map superimposed on top of a base map, which shows the interrelationships between journals in different domains, representing the publication and citation activities in each domain (Chen and Leydesdorff 2014 ). The overlay map reveals the link between the citing domain (on the left side) and the cited domain (on the right side), reflecting the knowledge flow of the discipline at the journal level (Leydesdorff and Rafols 2012 ). We utilize the in-built Z-score algorithm of the software to cluster the graph, as shown in Fig. 3 . ![]() The left side shows the citing journal, and the right side shows the cited journal. Figure 3 shows the distribution of citing journals clusters for older adults’ technology acceptance on the left side, while the right side refers to the main cited journals clusters. Two knowledge flow citation trajectories were obtained; they are presented by the color of the cited regions, and the thickness of these trajectories is proportional to the Z-score scaled frequency of citations (Chen et al. 2014 ). Within the cited regions, the most popular fields with the most records covered are “HEALTH, NURSING, MEDICINE” and “PSYCHOLOGY, EDUCATION, SOCIAL”, and the elliptical aspect ratio of these two fields stands out. Fields have prominent elliptical aspect ratios, highlighting their significant influence on older adults’ technology acceptance research. Additionally, the major citation trajectories originate in these two areas and progress to the frontier research area of “PSYCHOLOGY, EDUCATION, HEALTH”. It is worth noting that the citation trajectory from “PSYCHOLOGY, EDUCATION, SOCIAL” has a significant Z-value (z = 6.81), emphasizing the significance and impact of this development path. In the future, “MATHEMATICS, SYSTEMS, MATHEMATICAL”, “MOLECULAR, BIOLOGY, IMMUNOLOGY”, and “NEUROLOGY, SPORTS, OPHTHALMOLOGY” may become emerging fields. The fields of “MEDICINE, MEDICAL, CLINICAL” may be emerging areas of cutting-edge research. Main research journals analysisTable 3 provides statistics for the top ten journals by publication volume in the field of older adults’ technology acceptance. Together, these journals have published 137 articles, accounting for 27.40% of the total publications, indicating that there is no highly concentrated core group of journals in this field, with publications being relatively dispersed. Notably, Computers in Human Behavior , Journal of Medical Internet Research , and International Journal of Human-Computer Interaction each lead with 15 publications. In terms of citation metrics, International Journal of Medical Informatics and Computers in Human Behavior stand out significantly, with the former accumulating a total of 1,904 citations, averaging 211.56 citations per article, and the latter totaling 1,449 citations, with an average of 96.60 citations per article. These figures emphasize the academic authority and widespread impact of these journals within the research field. Research power (RQ2)Countries and collaborations analysis. The analysis revealed the global research pattern for country distribution and collaboration (Chen et al. 2019 ). Figure 4A shows the network of national collaborations on older adults’ technology acceptance research. The size of the bubbles represents the amount of publications in each country, while the thickness of the connecting lines expresses the closeness of the collaboration among countries. Generally, this research subject has received extensive international attention, with China and the USA publishing far more than any other countries. China has established notable research collaborations with the USA, UK and Malaysia in this field, while other countries have collaborations, but the closeness is relatively low and scattered. Figure 4B shows the annual publication volume dynamics of the top ten countries in terms of total publications. Since 2017, China has consistently increased its annual publications, while the USA has remained relatively stable. In 2019, the volume of publications in each country increased significantly, this was largely due to the global outbreak of the COVID-19 pandemic, which has led to increased reliance on information technology among the elderly for medical consultations, online socialization, and health management (Sinha et al. 2021 ). This phenomenon has led to research advances in technology acceptance among older adults in various countries. Table 4 shows that the top ten countries account for 93.20% of the total cumulative number of publications, with each country having published more than 20 papers. Among these ten countries, all of them except China are developed countries, indicating that the research field of older adults’ technology acceptance has received general attention from developed countries. Currently, China and the USA were the leading countries in terms of publications with 111 and 104 respectively, accounting for 22.20% and 20.80%. The UK, Germany, Italy, and the Netherlands also made significant contributions. The USA and China ranked first and second in terms of the number of citations, while the Netherlands had the highest average citations, indicating the high impact and quality of its research. The UK has shown outstanding performance in international cooperation, while the USA highlights its significant academic influence in this field with the highest h-index value. ![]() A National collaboration network. B Annual volume of publications in the top 10 countries. Institutions and authors analysisAnalyzing the number of publications and citations can reveal an institution’s or author’s research strength and influence in a particular research area (Kwiek 2021 ). Tables 5 and 6 show the statistics of the institutions and authors whose publication counts are in the top ten, respectively. As shown in Table 5 , higher education institutions hold the main position in this research field. Among the top ten institutions, City University of Hong Kong and The University of Hong Kong from China lead with 14 and 9 publications, respectively. City University of Hong Kong has the highest h-index, highlighting its significant influence in the field. It is worth noting that Tilburg University in the Netherlands is not among the top five in terms of publications, but the high average citation count (130.14) of its literature demonstrates the high quality of its research. After analyzing the authors’ output using Price’s Law (Redner 1998 ), the highest number of publications among the authors counted ( n = 10) defines a publication threshold of 3 for core authors in this research area. As a result of quantitative screening, a total of 63 core authors were identified. Table 6 shows that Chen from Zhejiang University, China, Ziefle from RWTH Aachen University, Germany, and Rogers from Macquarie University, Australia, were the top three authors in terms of the number of publications, with 10, 9, and 8 articles, respectively. In terms of average citation rate, Peek and Wouters, both scholars from the Netherlands, have significantly higher rates than other scholars, with 183.2 and 152.67 respectively. This suggests that their research is of high quality and widely recognized. Additionally, Chen and Rogers have high h-indices in this field. Knowledge base and theme progress (RQ3)Research knowledge base. Co-citation relationships occur when two documents are cited together (Zhang and Zhu 2022 ). Co-citation mapping uses references as nodes to represent the knowledge base of a subject area (Min et al. 2021). Figure 5A illustrates co-occurrence mapping in older adults’ technology acceptance research, where larger nodes signify higher co-citation frequencies. Co-citation cluster analysis can be used to explore knowledge structure and research boundaries (Hota et al. 2020 ; Shiau et al. 2023 ). The co-citation clustering mapping of older adults’ technology acceptance research literature (Fig. 5B ) shows that the Q value of the clustering result is 0.8129 (>0.3), and the average value of the weight S is 0.9391 (>0.7), indicating that the clusters are uniformly distributed with a significant and credible structure. This further proves that the boundaries of the research field are clear and there is significant differentiation in the field. The figure features 18 cluster labels, each associated with thematic color blocks corresponding to different time slices. Highlighted emerging research themes include #2 Smart Home Technology, #7 Social Live, and #10 Customer Service. Furthermore, the clustering labels extracted are primarily classified into three categories: theoretical model deepening, emerging technology applications, research methods and evaluation, as detailed in Table 7 . ![]() A Co-citation analysis of references. B Clustering network analysis of references. Seminal literature analysisThe top ten nodes in terms of co-citation frequency were selected for further analysis. Table 8 displays the corresponding node information. Studies were categorized into four main groups based on content analysis. (1) Research focusing on specific technology usage by older adults includes studies by Peek et al. ( 2014 ), Ma et al. ( 2016 ), Hoque and Sorwar ( 2017 ), and Li et al. ( 2019 ), who investigated the factors influencing the use of e-technology, smartphones, mHealth, and smart wearables, respectively. (2) Concerning the development of theoretical models of technology acceptance, Chen and Chan ( 2014 ) introduced the Senior Technology Acceptance Model (STAM), and Macedo ( 2017 ) analyzed the predictive power of UTAUT2 in explaining older adults’ intentional behaviors and information technology usage. (3) In exploring older adults’ information technology adoption and behavior, Lee and Coughlin ( 2015 ) emphasized that the adoption of technology by older adults is a multifactorial process that includes performance, price, value, usability, affordability, accessibility, technical support, social support, emotion, independence, experience, and confidence. Yusif et al. ( 2016 ) conducted a literature review examining the key barriers affecting older adults’ adoption of assistive technology, including factors such as privacy, trust, functionality/added value, cost, and stigma. (4) From the perspective of research into older adults’ technology acceptance, Mitzner et al. ( 2019 ) assessed the long-term usage of computer systems designed for the elderly, whereas Guner and Acarturk ( 2020 ) compared information technology usage and acceptance between older and younger adults. The breadth and prevalence of this literature make it a vital reference for researchers in the field, also providing new perspectives and inspiration for future research directions. Research thematic progressBurst citation is a node of literature that guides the sudden change in dosage, which usually represents a prominent development or major change in a particular field, with innovative and forward-looking qualities. By analyzing the emergent literature, it is often easy to understand the dynamics of the subject area, mapping the emerging thematic change (Chen et al. 2022 ). Figure 6 shows the burst citation mapping in the field of older adults’ technology acceptance research, with burst citations represented by red nodes (Fig. 6A ). For the ten papers with the highest burst intensity (Fig. 6B ), this study will conduct further analysis in conjunction with literature review. ![]() A Burst detection of co-citation. B The top 10 references with the strongest citation bursts. As shown in Fig. 6 , Mitzner et al. ( 2010 ) broke the stereotype that older adults are fearful of technology, found that they actually have positive attitudes toward technology, and emphasized the centrality of ease of use and usefulness in the process of technology acceptance. This finding provides an important foundation for subsequent research. During the same period, Wagner et al. ( 2010 ) conducted theory-deepening and applied research on technology acceptance among older adults. The research focused on older adults’ interactions with computers from the perspective of Social Cognitive Theory (SCT). This expanded the understanding of technology acceptance, particularly regarding the relationship between behavior, environment, and other SCT elements. In addition, Pan and Jordan-Marsh ( 2010 ) extended the TAM to examine the interactions among predictors of perceived usefulness, perceived ease of use, subjective norm, and convenience conditions when older adults use the Internet, taking into account the moderating roles of gender and age. Heerink et al. ( 2010 ) adapted and extended the UTAUT, constructed a technology acceptance model specifically designed for older users’ acceptance of assistive social agents, and validated it using controlled experiments and longitudinal data, explaining intention to use by combining functional assessment and social interaction variables. Then the research theme shifted to an in-depth analysis of the factors influencing technology acceptance among older adults. Two papers with high burst strengths emerged during this period: Peek et al. ( 2014 ) (Strength = 12.04), Chen and Chan ( 2014 ) (Strength = 9.81). Through a systematic literature review and empirical study, Peek STM and Chen K, among others, identified multidimensional factors that influence older adults’ technology acceptance. Peek et al. ( 2014 ) analyzed literature on the acceptance of in-home care technology among older adults and identified six factors that influence their acceptance: concerns about technology, expected benefits, technology needs, technology alternatives, social influences, and older adult characteristics, with a focus on differences between pre- and post-implementation factors. Chen and Chan ( 2014 ) constructed the STAM by administering a questionnaire to 1012 older adults and adding eight important factors, including technology anxiety, self-efficacy, cognitive ability, and physical function, based on the TAM. This enriches the theoretical foundation of the field. In addition, Braun ( 2013 ) highlighted the role of perceived usefulness, trust in social networks, and frequency of Internet use in older adults’ use of social networks, while ease of use and social pressure were not significant influences. These findings contribute to the study of older adults’ technology acceptance within specific technology application domains. Recent research has focused on empirical studies of personal factors and emerging technologies. Ma et al. ( 2016 ) identified key personal factors affecting smartphone acceptance among older adults through structured questionnaires and face-to-face interviews with 120 participants. The study found that cost, self-satisfaction, and convenience were important factors influencing perceived usefulness and ease of use. This study offers empirical evidence to comprehend the main factors that drive smartphone acceptance among Chinese older adults. Additionally, Yusif et al. ( 2016 ) presented an overview of the obstacles that hinder older adults’ acceptance of assistive technologies, focusing on privacy, trust, and functionality. In summary, research on older adults’ technology acceptance has shifted from early theoretical deepening and analysis of influencing factors to empirical studies in the areas of personal factors and emerging technologies, which have greatly enriched the theoretical basis of older adults’ technology acceptance and provided practical guidance for the design of emerging technology products. Research hotspots, evolutionary trends, and quality distribution (RQ4)Core keywords analysis. Keywords concise the main idea and core of the literature, and are a refined summary of the research content (Huang et al. 2021 ). In CiteSpace, nodes with a centrality value greater than 0.1 are considered to be critical nodes. Analyzing keywords with high frequency and centrality helps to visualize the hot topics in the research field (Park et al. 2018 ). The merged keywords were imported into CiteSpace, and the top 10 keywords were counted and sorted by frequency and centrality respectively, as shown in Table 9 . The results show that the keyword “TAM” has the highest frequency (92), followed by “UTAUT” (24), which reflects that the in-depth study of the existing technology acceptance model and its theoretical expansion occupy a central position in research related to older adults’ technology acceptance. Furthermore, the terms ‘assistive technology’ and ‘virtual reality’ are both high-frequency and high-centrality terms (frequency = 17, centrality = 0.10), indicating that the research on assistive technology and virtual reality for older adults is the focus of current academic attention. Research hotspots analysisUsing VOSviewer for keyword co-occurrence analysis organizes keywords into groups or clusters based on their intrinsic connections and frequencies, clearly highlighting the research field’s hot topics. The connectivity among keywords reveals correlations between different topics. To ensure accuracy, the analysis only considered the authors’ keywords. Subsequently, the keywords were filtered by setting the keyword frequency to 5 to obtain the keyword clustering map of the research on older adults’ technology acceptance research keyword clustering mapping (Fig. 7 ), combined with the keyword co-occurrence clustering network (Fig. 7A ) and the corresponding density situation (Fig. 7B ) to make a detailed analysis of the following four groups of clustered themes. ![]() A Co-occurrence clustering network. B Keyword density. Cluster #1—Research on the factors influencing technology adoption among older adults is a prominent topic, covering age, gender, self-efficacy, attitude, and and intention to use (Berkowsky et al. 2017 ; Wang et al. 2017 ). It also examined older adults’ attitudes towards and acceptance of digital health technologies (Ahmad and Mozelius, 2022 ). Moreover, the COVID-19 pandemic, significantly impacting older adults’ technology attitudes and usage, has underscored the study’s importance and urgency. Therefore, it is crucial to conduct in-depth studies on how older adults accept, adopt, and effectively use new technologies, to address their needs and help them overcome the digital divide within digital inclusion. This will improve their quality of life and healthcare experiences. Cluster #2—Research focuses on how older adults interact with assistive technologies, especially assistive robots and health monitoring devices, emphasizing trust, usability, and user experience as crucial factors (Halim et al. 2022 ). Moreover, health monitoring technologies effectively track and manage health issues common in older adults, like dementia and mild cognitive impairment (Lussier et al. 2018 ; Piau et al. 2019 ). Interactive exercise games and virtual reality have been deployed to encourage more physical and cognitive engagement among older adults (Campo-Prieto et al. 2021 ). Personalized and innovative technology significantly enhances older adults’ participation, improving their health and well-being. Cluster #3—Optimizing health management for older adults using mobile technology. With the development of mobile health (mHealth) and health information technology, mobile applications, smartphones, and smart wearable devices have become effective tools to help older users better manage chronic conditions, conduct real-time health monitoring, and even receive telehealth services (Dupuis and Tsotsos 2018 ; Olmedo-Aguirre et al. 2022 ; Kim et al. 2014 ). Additionally, these technologies can mitigate the problem of healthcare resource inequality, especially in developing countries. Older adults’ acceptance and use of these technologies are significantly influenced by their behavioral intentions, motivational factors, and self-management skills. These internal motivational factors, along with external factors, jointly affect older adults’ performance in health management and quality of life. Cluster #4—Research on technology-assisted home care for older adults is gaining popularity. Environmentally assisted living enhances older adults’ independence and comfort at home, offering essential support and security. This has a crucial impact on promoting healthy aging (Friesen et al. 2016 ; Wahlroos et al. 2023 ). The smart home is a core application in this field, providing a range of solutions that facilitate independent living for the elderly in a highly integrated and user-friendly manner. This fulfills different dimensions of living and health needs (Majumder et al. 2017 ). Moreover, eHealth offers accurate and personalized health management and healthcare services for older adults (Delmastro et al. 2018 ), ensuring their needs are met at home. Research in this field often employs qualitative methods and structural equation modeling to fully understand older adults’ needs and experiences at home and analyze factors influencing technology adoption. Evolutionary trends analysisTo gain a deeper understanding of the evolutionary trends in research hotspots within the field of older adults’ technology acceptance, we conducted a statistical analysis of the average appearance times of keywords, using CiteSpace to generate the time-zone evolution mapping (Fig. 8 ) and burst keywords. The time-zone mapping visually displays the evolution of keywords over time, intuitively reflecting the frequency and initial appearance of keywords in research, commonly used to identify trends in research topics (Jing et al. 2024a ; Kumar et al. 2021 ). Table 10 lists the top 15 keywords by burst strength, with the red sections indicating high-frequency citations and their burst strength in specific years. These burst keywords reveal the focus and trends of research themes over different periods (Kleinberg 2002 ). Combining insights from the time-zone mapping and burst keywords provides more objective and accurate research insights (Wang et al. 2023b ). ![]() Reflecting the frequency and time of first appearance of keywords in the study. An integrated analysis of Fig. 8 and Table 10 shows that early research on older adults’ technology acceptance primarily focused on factors such as perceived usefulness, ease of use, and attitudes towards information technology, including their use of computers and the internet (Pan and Jordan-Marsh 2010 ), as well as differences in technology use between older adults and other age groups (Guner and Acarturk 2020 ). Subsequently, the research focus expanded to improving the quality of life for older adults, exploring how technology can optimize health management and enhance the possibility of independent living, emphasizing the significant role of technology in improving the quality of life for the elderly. With ongoing technological advancements, recent research has shifted towards areas such as “virtual reality,” “telehealth,” and “human-robot interaction,” with a focus on the user experience of older adults (Halim et al. 2022 ). The appearance of keywords such as “physical activity” and “exercise” highlights the value of technology in promoting physical activity and health among older adults. This phase of research tends to make cutting-edge technology genuinely serve the practical needs of older adults, achieving its widespread application in daily life. Additionally, research has focused on expanding and quantifying theoretical models of older adults’ technology acceptance, involving keywords such as “perceived risk”, “validation” and “UTAUT”. In summary, from 2013 to 2023, the field of older adults’ technology acceptance has evolved from initial explorations of influencing factors, to comprehensive enhancements in quality of life and health management, and further to the application and deepening of theoretical models and cutting-edge technologies. This research not only reflects the diversity and complexity of the field but also demonstrates a comprehensive and in-depth understanding of older adults’ interactions with technology across various life scenarios and needs. Research quality distributionTo reveal the distribution of research quality in the field of older adults’ technology acceptance, a strategic diagram analysis is employed to calculate and illustrate the internal development and interrelationships among various research themes (Xie et al. 2020 ). The strategic diagram uses Centrality as the X-axis and Density as the Y-axis to divide into four quadrants, where the X-axis represents the strength of the connection between thematic clusters and other themes, with higher values indicating a central position in the research field; the Y-axis indicates the level of development within the thematic clusters, with higher values denoting a more mature and widely recognized field (Li and Zhou 2020 ). Through cluster analysis and manual verification, this study categorized 61 core keywords (Frequency ≥5) into 11 thematic clusters. Subsequently, based on the keywords covered by each thematic cluster, the research themes and their directions for each cluster were summarized (Table 11 ), and the centrality and density coordinates for each cluster were precisely calculated (Table 12 ). Finally, a strategic diagram of the older adults’ technology acceptance research field was constructed (Fig. 9 ). Based on the distribution of thematic clusters across the quadrants in the strategic diagram, the structure and developmental trends of the field were interpreted. ![]() Classification and visualization of theme clusters based on density and centrality. As illustrated in Fig. 9 , (1) the theme clusters of #3 Usage Experience and #4 Assisted Living Technology are in the first quadrant, characterized by high centrality and density. Their internal cohesion and close links with other themes indicate their mature development, systematic research content or directions have been formed, and they have a significant influence on other themes. These themes play a central role in the field of older adults’ technology acceptance and have promising prospects. (2) The theme clusters of #6 Smart Devices, #9 Theoretical Models, and #10 Mobile Health Applications are in the second quadrant, with higher density but lower centrality. These themes have strong internal connections but weaker external links, indicating that these three themes have received widespread attention from researchers and have been the subject of related research, but more as self-contained systems and exhibit independence. Therefore, future research should further explore in-depth cooperation and cross-application with other themes. (3) The theme clusters of #7 Human-Robot Interaction, #8 Characteristics of the Elderly, and #11 Research Methods are in the third quadrant, with lower centrality and density. These themes are loosely connected internally and have weak links with others, indicating their developmental immaturity. Compared to other topics, they belong to the lower attention edge and niche themes, and there is a need for further investigation. (4) The theme clusters of #1 Digital Healthcare Technology, #2 Psychological Factors, and #5 Socio-Cultural Factors are located in the fourth quadrant, with high centrality but low density. Although closely associated with other research themes, the internal cohesion within these clusters is relatively weak. This suggests that while these themes are closely linked to other research areas, their own development remains underdeveloped, indicating a core immaturity. Nevertheless, these themes are crucial within the research domain of elderly technology acceptance and possess significant potential for future exploration. Discussion on distribution power (RQ1)Over the past decade, academic interest and influence in the area of older adults’ technology acceptance have significantly increased. This trend is evidenced by a quantitative analysis of publication and citation volumes, particularly noticeable in 2019 and 2022, where there was a substantial rise in both metrics. The rise is closely linked to the widespread adoption of emerging technologies such as smart homes, wearable devices, and telemedicine among older adults. While these technologies have enhanced their quality of life, they also pose numerous challenges, sparking extensive research into their acceptance, usage behaviors, and influencing factors among the older adults (Pirzada et al. 2022 ; Garcia Reyes et al. 2023 ). Furthermore, the COVID-19 pandemic led to a surge in technology demand among older adults, especially in areas like medical consultation, online socialization, and health management, further highlighting the importance and challenges of technology. Health risks and social isolation have compelled older adults to rely on technology for daily activities, accelerating its adoption and application within this demographic. This phenomenon has made technology acceptance a critical issue, driving societal and academic focus on the study of technology acceptance among older adults. The flow of knowledge at the level of high-output disciplines and journals, along with the primary publishing outlets, indicates the highly interdisciplinary nature of research into older adults’ technology acceptance. This reflects the complexity and breadth of issues related to older adults’ technology acceptance, necessitating the integration of multidisciplinary knowledge and approaches. Currently, research is primarily focused on medical health and human-computer interaction, demonstrating academic interest in improving health and quality of life for older adults and addressing the urgent needs related to their interactions with technology. In the field of medical health, research aims to provide advanced and innovative healthcare technologies and services to meet the challenges of an aging population while improving the quality of life for older adults (Abdi et al. 2020 ; Wilson et al. 2021 ). In the field of human-computer interaction, research is focused on developing smarter and more user-friendly interaction models to meet the needs of older adults in the digital age, enabling them to actively participate in social activities and enjoy a higher quality of life (Sayago, 2019 ). These studies are crucial for addressing the challenges faced by aging societies, providing increased support and opportunities for the health, welfare, and social participation of older adults. Discussion on research power (RQ2)This study analyzes leading countries and collaboration networks, core institutions and authors, revealing the global research landscape and distribution of research strength in the field of older adults’ technology acceptance, and presents quantitative data on global research trends. From the analysis of country distribution and collaborations, China and the USA hold dominant positions in this field, with developed countries like the UK, Germany, Italy, and the Netherlands also excelling in international cooperation and research influence. The significant investment in technological research and the focus on the technological needs of older adults by many developed countries reflect their rapidly aging societies, policy support, and resource allocation. China is the only developing country that has become a major contributor in this field, indicating its growing research capabilities and high priority given to aging societies and technological innovation. Additionally, China has close collaborations with countries such as USA, the UK, and Malaysia, driven not only by technological research needs but also by shared challenges and complementarities in aging issues among these nations. For instance, the UK has extensive experience in social welfare and aging research, providing valuable theoretical guidance and practical experience. International collaborations, aimed at addressing the challenges of aging, integrate the strengths of various countries, advancing in-depth and widespread development in the research of technology acceptance among older adults. At the institutional and author level, City University of Hong Kong leads in publication volume, with research teams led by Chan and Chen demonstrating significant academic activity and contributions. Their research primarily focuses on older adults’ acceptance and usage behaviors of various technologies, including smartphones, smart wearables, and social robots (Chen et al. 2015 ; Li et al. 2019 ; Ma et al. 2016 ). These studies, targeting specific needs and product characteristics of older adults, have developed new models of technology acceptance based on existing frameworks, enhancing the integration of these technologies into their daily lives and laying a foundation for further advancements in the field. Although Tilburg University has a smaller publication output, it holds significant influence in the field of older adults’ technology acceptance. Particularly, the high citation rate of Peek’s studies highlights their excellence in research. Peek extensively explored older adults’ acceptance and usage of home care technologies, revealing the complexity and dynamics of their technology use behaviors. His research spans from identifying systemic influencing factors (Peek et al. 2014 ; Peek et al. 2016 ), emphasizing familial impacts (Luijkx et al. 2015 ), to constructing comprehensive models (Peek et al. 2017 ), and examining the dynamics of long-term usage (Peek et al. 2019 ), fully reflecting the evolving technology landscape and the changing needs of older adults. Additionally, the ongoing contributions of researchers like Ziefle, Rogers, and Wouters in the field of older adults’ technology acceptance demonstrate their research influence and leadership. These researchers have significantly enriched the knowledge base in this area with their diverse perspectives. For instance, Ziefle has uncovered the complex attitudes of older adults towards technology usage, especially the trade-offs between privacy and security, and how different types of activities affect their privacy needs (Maidhof et al. 2023 ; Mujirishvili et al. 2023 ; Schomakers and Ziefle 2023 ; Wilkowska et al. 2022 ), reflecting a deep exploration and ongoing innovation in the field of older adults’ technology acceptance. Discussion on knowledge base and thematic progress (RQ3)Through co-citation analysis and systematic review of seminal literature, this study reveals the knowledge foundation and thematic progress in the field of older adults’ technology acceptance. Co-citation networks and cluster analyses illustrate the structural themes of the research, delineating the differentiation and boundaries within this field. Additionally, burst detection analysis offers a valuable perspective for understanding the thematic evolution in the field of technology acceptance among older adults. The development and innovation of theoretical models are foundational to this research. Researchers enhance the explanatory power of constructed models by deepening and expanding existing technology acceptance theories to address theoretical limitations. For instance, Heerink et al. ( 2010 ) modified and expanded the UTAUT model by integrating functional assessment and social interaction variables to create the almere model. This model significantly enhances the ability to explain the intentions of older users in utilizing assistive social agents and improves the explanation of actual usage behaviors. Additionally, Chen and Chan ( 2014 ) extended the TAM to include age-related health and capability features of older adults, creating the STAM, which substantially improves predictions of older adults’ technology usage behaviors. Personal attributes, health and capability features, and facilitating conditions have a direct impact on technology acceptance. These factors more effectively predict older adults’ technology usage behaviors than traditional attitudinal factors. With the advancement of technology and the application of emerging technologies, new research topics have emerged, increasingly focusing on older adults’ acceptance and use of these technologies. Prior to this, the study by Mitzner et al. ( 2010 ) challenged the stereotype of older adults’ conservative attitudes towards technology, highlighting the central roles of usability and usefulness in the technology acceptance process. This discovery laid an important foundation for subsequent research. Research fields such as “smart home technology,” “social life,” and “customer service” are emerging, indicating a shift in focus towards the practical and social applications of technology in older adults’ lives. Research not only focuses on the technology itself but also on how these technologies integrate into older adults’ daily lives and how they can improve the quality of life through technology. For instance, studies such as those by Ma et al. ( 2016 ), Hoque and Sorwar ( 2017 ), and Li et al. ( 2019 ) have explored factors influencing older adults’ use of smartphones, mHealth, and smart wearable devices. Furthermore, the diversification of research methodologies and innovation in evaluation techniques, such as the use of mixed methods, structural equation modeling (SEM), and neural network (NN) approaches, have enhanced the rigor and reliability of the findings, enabling more precise identification of the factors and mechanisms influencing technology acceptance. Talukder et al. ( 2020 ) employed an effective multimethodological strategy by integrating SEM and NN to leverage the complementary strengths of both approaches, thus overcoming their individual limitations and more accurately analyzing and predicting older adults’ acceptance of wearable health technologies (WHT). SEM is utilized to assess the determinants’ impact on the adoption of WHT, while neural network models validate SEM outcomes and predict the significance of key determinants. This combined approach not only boosts the models’ reliability and explanatory power but also provides a nuanced understanding of the motivations and barriers behind older adults’ acceptance of WHT, offering deep research insights. Overall, co-citation analysis of the literature in the field of older adults’ technology acceptance has uncovered deeper theoretical modeling and empirical studies on emerging technologies, while emphasizing the importance of research methodological and evaluation innovations in understanding complex social science issues. These findings are crucial for guiding the design and marketing strategies of future technology products, especially in the rapidly growing market of older adults. Discussion on research hotspots and evolutionary trends (RQ4)By analyzing core keywords, we can gain deep insights into the hot topics, evolutionary trends, and quality distribution of research in the field of older adults’ technology acceptance. The frequent occurrence of the keywords “TAM” and “UTAUT” indicates that the applicability and theoretical extension of existing technology acceptance models among older adults remain a focal point in academia. This phenomenon underscores the enduring influence of the studies by Davis ( 1989 ) and Venkatesh et al. ( 2003 ), whose models provide a robust theoretical framework for explaining and predicting older adults’ acceptance and usage of emerging technologies. With the widespread application of artificial intelligence (AI) and big data technologies, these theoretical models have incorporated new variables such as perceived risk, trust, and privacy issues (Amin et al. 2024 ; Chen et al. 2024 ; Jing et al. 2024b ; Seibert et al. 2021 ; Wang et al. 2024b ), advancing the theoretical depth and empirical research in this field. Keyword co-occurrence cluster analysis has revealed multiple research hotspots in the field, including factors influencing technology adoption, interactive experiences between older adults and assistive technologies, the application of mobile health technology in health management, and technology-assisted home care. These studies primarily focus on enhancing the quality of life and health management of older adults through emerging technologies, particularly in the areas of ambient assisted living, smart health monitoring, and intelligent medical care. In these domains, the role of AI technology is increasingly significant (Qian et al. 2021 ; Ho 2020 ). With the evolution of next-generation information technologies, AI is increasingly integrated into elder care systems, offering intelligent, efficient, and personalized service solutions by analyzing the lifestyles and health conditions of older adults. This integration aims to enhance older adults’ quality of life in aspects such as health monitoring and alerts, rehabilitation assistance, daily health management, and emotional support (Lee et al. 2023 ). A survey indicates that 83% of older adults prefer AI-driven solutions when selecting smart products, demonstrating the increasing acceptance of AI in elder care (Zhao and Li 2024 ). Integrating AI into elder care presents both opportunities and challenges, particularly in terms of user acceptance, trust, and long-term usage effects, which warrant further exploration (Mhlanga 2023 ). These studies will help better understand the profound impact of AI technology on the lifestyles of older adults and provide critical references for optimizing AI-driven elder care services. The Time-zone evolution mapping and burst keyword analysis further reveal the evolutionary trends of research hotspots. Early studies focused on basic technology acceptance models and user perceptions, later expanding to include quality of life and health management. In recent years, research has increasingly focused on cutting-edge technologies such as virtual reality, telehealth, and human-robot interaction, with a concurrent emphasis on the user experience of older adults. This evolutionary process demonstrates a deepening shift from theoretical models to practical applications, underscoring the significant role of technology in enhancing the quality of life for older adults. Furthermore, the strategic coordinate mapping analysis clearly demonstrates the development and mutual influence of different research themes. High centrality and density in the themes of Usage Experience and Assisted Living Technology indicate their mature research status and significant impact on other themes. The themes of Smart Devices, Theoretical Models, and Mobile Health Applications demonstrate self-contained research trends. The themes of Human-Robot Interaction, Characteristics of the Elderly, and Research Methods are not yet mature, but they hold potential for development. Themes of Digital Healthcare Technology, Psychological Factors, and Socio-Cultural Factors are closely related to other themes, displaying core immaturity but significant potential. In summary, the research hotspots in the field of older adults’ technology acceptance are diverse and dynamic, demonstrating the academic community’s profound understanding of how older adults interact with technology across various life contexts and needs. Under the influence of AI and big data, research should continue to focus on the application of emerging technologies among older adults, exploring in depth how they adapt to and effectively use these technologies. This not only enhances the quality of life and healthcare experiences for older adults but also drives ongoing innovation and development in this field. Research agendaBased on the above research findings, to further understand and promote technology acceptance and usage among older adults, we recommend future studies focus on refining theoretical models, exploring long-term usage, and assessing user experience in the following detailed aspects: Refinement and validation of specific technology acceptance models for older adults: Future research should focus on developing and validating technology acceptance models based on individual characteristics, particularly considering variations in technology acceptance among older adults across different educational levels and cultural backgrounds. This includes factors such as age, gender, educational background, and cultural differences. Additionally, research should examine how well specific technologies, such as wearable devices and mobile health applications, meet the needs of older adults. Building on existing theoretical models, this research should integrate insights from multiple disciplines such as psychology, sociology, design, and engineering through interdisciplinary collaboration to create more accurate and comprehensive models, which should then be validated in relevant contexts. Deepening the exploration of the relationship between long-term technology use and quality of life among older adults: The acceptance and use of technology by users is a complex and dynamic process (Seuwou et al. 2016 ). Existing research predominantly focuses on older adults’ initial acceptance or short-term use of new technologies; however, the impact of long-term use on their quality of life and health is more significant. Future research should focus on the evolution of older adults’ experiences and needs during long-term technology usage, and the enduring effects of technology on their social interactions, mental health, and life satisfaction. Through longitudinal studies and qualitative analysis, this research reveals the specific needs and challenges of older adults in long-term technology use, providing a basis for developing technologies and strategies that better meet their requirements. This understanding aids in comprehensively assessing the impact of technology on older adults’ quality of life and guiding the optimization and improvement of technological products. Evaluating the Importance of User Experience in Research on Older Adults’ Technology Acceptance: Understanding the mechanisms of information technology acceptance and use is central to human-computer interaction research. Although technology acceptance models and user experience models differ in objectives, they share many potential intersections. Technology acceptance research focuses on structured prediction and assessment, while user experience research concentrates on interpreting design impacts and new frameworks. Integrating user experience to assess older adults’ acceptance of technology products and systems is crucial (Codfrey et al. 2022 ; Wang et al. 2019 ), particularly for older users, where specific product designs should emphasize practicality and usability (Fisk et al. 2020 ). Researchers need to explore innovative age-appropriate design methods to enhance older adults’ usage experience. This includes studying older users’ actual usage preferences and behaviors, optimizing user interfaces, and interaction designs. Integrating feedback from older adults to tailor products to their needs can further promote their acceptance and continued use of technology products. ConclusionsThis study conducted a systematic review of the literature on older adults’ technology acceptance over the past decade through bibliometric analysis, focusing on the distribution power, research power, knowledge base and theme progress, research hotspots, evolutionary trends, and quality distribution. Using a combination of quantitative and qualitative methods, this study has reached the following conclusions: Technology acceptance among older adults has become a hot topic in the international academic community, involving the integration of knowledge across multiple disciplines, including Medical Informatics, Health Care Sciences Services, and Ergonomics. In terms of journals, “PSYCHOLOGY, EDUCATION, HEALTH” represents a leading field, with key publications including Computers in Human Behavior , Journal of Medical Internet Research , and International Journal of Human-Computer Interaction . These journals possess significant academic authority and extensive influence in the field. Research on technology acceptance among older adults is particularly active in developed countries, with China and USA publishing significantly more than other nations. The Netherlands leads in high average citation rates, indicating the depth and impact of its research. Meanwhile, the UK stands out in terms of international collaboration. At the institutional level, City University of Hong Kong and The University of Hong Kong in China are in leading positions. Tilburg University in the Netherlands demonstrates exceptional research quality through its high average citation count. At the author level, Chen from China has the highest number of publications, while Peek from the Netherlands has the highest average citation count. Co-citation analysis of references indicates that the knowledge base in this field is divided into three main categories: theoretical model deepening, emerging technology applications, and research methods and evaluation. Seminal literature focuses on four areas: specific technology use by older adults, expansion of theoretical models of technology acceptance, information technology adoption behavior, and research perspectives. Research themes have evolved from initial theoretical deepening and analysis of influencing factors to empirical studies on individual factors and emerging technologies. Keyword analysis indicates that TAM and UTAUT are the most frequently occurring terms, while “assistive technology” and “virtual reality” are focal points with high frequency and centrality. Keyword clustering analysis reveals that research hotspots are concentrated on the influencing factors of technology adoption, human-robot interaction experiences, mobile health management, and technology for aging in place. Time-zone evolution mapping and burst keyword analysis have revealed the research evolution from preliminary exploration of influencing factors, to enhancements in quality of life and health management, and onto advanced technology applications and deepening of theoretical models. Furthermore, analysis of research quality distribution indicates that Usage Experience and Assisted Living Technology have become core topics, while Smart Devices, Theoretical Models, and Mobile Health Applications point towards future research directions. Through this study, we have systematically reviewed the dynamics, core issues, and evolutionary trends in the field of older adults’ technology acceptance, constructing a comprehensive Knowledge Mapping of the domain and presenting a clear framework of existing research. This not only lays the foundation for subsequent theoretical discussions and innovative applications in the field but also provides an important reference for relevant scholars. LimitationsTo our knowledge, this is the first bibliometric analysis concerning technology acceptance among older adults, and we adhered strictly to bibliometric standards throughout our research. However, this study relies on the Web of Science Core Collection, and while its authority and breadth are widely recognized, this choice may have missed relevant literature published in other significant databases such as PubMed, Scopus, and Google Scholar, potentially overlooking some critical academic contributions. Moreover, given that our analysis was confined to literature in English, it may not reflect studies published in other languages, somewhat limiting the global representativeness of our data sample. It is noteworthy that with the rapid development of AI technology, its increasingly widespread application in elderly care services is significantly transforming traditional care models. AI is profoundly altering the lifestyles of the elderly, from health monitoring and smart diagnostics to intelligent home systems and personalized care, significantly enhancing their quality of life and health care standards. The potential for AI technology within the elderly population is immense, and research in this area is rapidly expanding. However, due to the restrictive nature of the search terms used in this study, it did not fully cover research in this critical area, particularly in addressing key issues such as trust, privacy, and ethics. Consequently, future research should not only expand data sources, incorporating multilingual and multidatabase literature, but also particularly focus on exploring older adults’ acceptance of AI technology and its applications, in order to construct a more comprehensive academic landscape of older adults’ technology acceptance, thereby enriching and extending the knowledge system and academic trends in this field. Data availabilityThe datasets analyzed during the current study are available in the Dataverse repository: https://doi.org/10.7910/DVN/6K0GJH . Abdi S, de Witte L, Hawley M (2020) Emerging technologies with potential care and support applications for older people: review of gray literature. JMIR Aging 3(2):e17286. https://doi.org/10.2196/17286 Article PubMed PubMed Central Google Scholar Achuthan K, Nair VK, Kowalski R, Ramanathan S, Raman R (2023) Cyberbullying research—Alignment to sustainable development and impact of COVID-19: Bibliometrics and science mapping analysis. Comput Human Behav 140:107566. https://doi.org/10.1016/j.chb.2022.107566 Article Google Scholar Ahmad A, Mozelius P (2022) Human-Computer Interaction for Older Adults: a Literature Review on Technology Acceptance of eHealth Systems. J Eng Res Sci 1(4):119–126. https://doi.org/10.55708/js0104014 Ale Ebrahim N, Salehi H, Embi MA, Habibi F, Gholizadeh H, Motahar SM (2014) Visibility and citation impact. Int Educ Stud 7(4):120–125. https://doi.org/10.5539/ies.v7n4p120 Amin MS, Johnson VL, Prybutok V, Koh CE (2024) An investigation into factors affecting the willingness to disclose personal health information when using AI-enabled caregiver robots. Ind Manag Data Syst 124(4):1677–1699. https://doi.org/10.1108/IMDS-09-2023-0608 Baer NR, Vietzke J, Schenk L (2022) Middle-aged and older adults’ acceptance of mobile nutrition and fitness apps: a systematic mixed studies review. PLoS One 17(12):e0278879. https://doi.org/10.1371/journal.pone.0278879 Barnard Y, Bradley MD, Hodgson F, Lloyd AD (2013) Learning to use new technologies by older adults: Perceived difficulties, experimentation behaviour and usability. Comput Human Behav 29(4):1715–1724. https://doi.org/10.1016/j.chb.2013.02.006 Berkowsky RW, Sharit J, Czaja SJ (2017) Factors predicting decisions about technology adoption among older adults. Innov Aging 3(1):igy002. https://doi.org/10.1093/geroni/igy002 Braun MT (2013) Obstacles to social networking website use among older adults. Comput Human Behav 29(3):673–680. https://doi.org/10.1016/j.chb.2012.12.004 Article MathSciNet Google Scholar Campo-Prieto P, Rodríguez-Fuentes G, Cancela-Carral JM (2021) Immersive virtual reality exergame promotes the practice of physical activity in older people: An opportunity during COVID-19. Multimodal Technol Interact 5(9):52. https://doi.org/10.3390/mti5090052 Chen C (2006) CiteSpace II: Detecting and visualizing emerging trends and transient patterns in scientific literature. J Am Soc Inf Sci Technol 57(3):359–377. https://doi.org/10.1002/asi.20317 Chen C, Dubin R, Kim MC (2014) Emerging trends and new developments in regenerative medicine: a scientometric update (2000–2014). Expert Opin Biol Ther 14(9):1295–1317. https://doi.org/10.1517/14712598.2014.920813 Article PubMed Google Scholar Chen C, Leydesdorff L (2014) Patterns of connections and movements in dual‐map overlays: A new method of publication portfolio analysis. J Assoc Inf Sci Technol 65(2):334–351. https://doi.org/10.1002/asi.22968 Chen J, Wang C, Tang Y (2022) Knowledge mapping of volunteer motivation: A bibliometric analysis and cross-cultural comparative study. Front Psychol 13:883150. https://doi.org/10.3389/fpsyg.2022.883150 Chen JY, Liu YD, Dai J, Wang CL (2023) Development and status of moral education research: Visual analysis based on knowledge graph. Front Psychol 13:1079955. https://doi.org/10.3389/fpsyg.2022.1079955 Chen K, Chan AH (2011) A review of technology acceptance by older adults. Gerontechnology 10(1):1–12. https://doi.org/10.4017/gt.2011.10.01.006.00 Chen K, Chan AH (2014) Gerontechnology acceptance by elderly Hong Kong Chinese: a senior technology acceptance model (STAM). Ergonomics 57(5):635–652. https://doi.org/10.1080/00140139.2014.895855 Chen K, Zhang Y, Fu X (2019) International research collaboration: An emerging domain of innovation studies? Res Policy 48(1):149–168. https://doi.org/10.1016/j.respol.2018.08.005 Chen X, Hu Z, Wang C (2024) Empowering education development through AIGC: A systematic literature review. Educ Inf Technol 1–53. https://doi.org/10.1007/s10639-024-12549-7 Chen Y, Chen CM, Liu ZY, Hu ZG, Wang XW (2015) The methodology function of CiteSpace mapping knowledge domains. Stud Sci Sci 33(2):242–253. https://doi.org/10.16192/j.cnki.1003-2053.2015.02.009 Codfrey GS, Baharum A, Zain NHM, Omar M, Deris FD (2022) User Experience in Product Design and Development: Perspectives and Strategies. Math Stat Eng Appl 71(2):257–262. https://doi.org/10.17762/msea.v71i2.83 Dai J, Zhang X, Wang CL (2024) A meta-analysis of learners’ continuance intention toward online education platforms. Educ Inf Technol 1–36. https://doi.org/10.1007/s10639-024-12654-7 Davis FD (1989) Perceived usefulness, perceived ease of use, and user acceptance of information technology. MIS Q 13(3):319–340. https://doi.org/10.2307/249008 Delmastro F, Dolciotti C, Palumbo F, Magrini M, Di Martino F, La Rosa D, Barcaro U (2018) Long-term care: how to improve the quality of life with mobile and e-health services. In 2018 14th International Conference on Wireless and Mobile Computing, Networking and Communications (WiMob), pp. 12–19. IEEE. https://doi.org/10.1109/WiMOB.2018.8589157 Dupuis K, Tsotsos LE (2018) Technology for remote health monitoring in an older population: a role for mobile devices. Multimodal Technol Interact 2(3):43. https://doi.org/10.3390/mti2030043 Ferguson C, Hickman LD, Turkmani S, Breen P, Gargiulo G, Inglis SC (2021) Wearables only work on patients that wear them”: Barriers and facilitators to the adoption of wearable cardiac monitoring technologies. Cardiovasc Digit Health J 2(2):137–147. https://doi.org/10.1016/j.cvdhj.2021.02.001 Fisk AD, Czaja SJ, Rogers WA, Charness N, Sharit J (2020) Designing for older adults: Principles and creative human factors approaches. CRC Press. https://doi.org/10.1201/9781420080681 Friesen S, Brémault-Phillips S, Rudrum L, Rogers LG (2016) Environmental design that supports healthy aging: Evaluating a new supportive living facility. J Hous Elderly 30(1):18–34. https://doi.org/10.1080/02763893.2015.1129380 Garcia Reyes EP, Kelly R, Buchanan G, Waycott J (2023) Understanding Older Adults’ Experiences With Technologies for Health Self-management: Interview Study. JMIR Aging 6:e43197. https://doi.org/10.2196/43197 Geng Z, Wang J, Liu J, Miao J (2024) Bibliometric analysis of the development, current status, and trends in adult degenerative scoliosis research: A systematic review from 1998 to 2023. J Pain Res 17:153–169. https://doi.org/10.2147/JPR.S437575 González A, Ramírez MP, Viadel V (2012) Attitudes of the elderly toward information and communications technologies. Educ Gerontol 38(9):585–594. https://doi.org/10.1080/03601277.2011.595314 Guner H, Acarturk C (2020) The use and acceptance of ICT by senior citizens: a comparison of technology acceptance model (TAM) for elderly and young adults. Univ Access Inf Soc 19(2):311–330. https://doi.org/10.1007/s10209-018-0642-4 Halim I, Saptari A, Perumal PA, Abdullah Z, Abdullah S, Muhammad MN (2022) A Review on Usability and User Experience of Assistive Social Robots for Older Persons. Int J Integr Eng 14(6):102–124. https://penerbit.uthm.edu.my/ojs/index.php/ijie/article/view/8566 He Y, He Q, Liu Q (2022) Technology acceptance in socially assistive robots: Scoping review of models, measurement, and influencing factors. J Healthc Eng 2022(1):6334732. https://doi.org/10.1155/2022/6334732 Heerink M, Kröse B, Evers V, Wielinga B (2010) Assessing acceptance of assistive social agent technology by older adults: the almere model. Int J Soc Robot 2:361–375. https://doi.org/10.1007/s12369-010-0068-5 Ho A (2020) Are we ready for artificial intelligence health monitoring in elder care? BMC Geriatr 20(1):358. https://doi.org/10.1186/s12877-020-01764-9 Hoque R, Sorwar G (2017) Understanding factors influencing the adoption of mHealth by the elderly: An extension of the UTAUT model. Int J Med Inform 101:75–84. https://doi.org/10.1016/j.ijmedinf.2017.02.002 Hota PK, Subramanian B, Narayanamurthy G (2020) Mapping the intellectual structure of social entrepreneurship research: A citation/co-citation analysis. J Bus Ethics 166(1):89–114. https://doi.org/10.1007/s10551-019-04129-4 Huang R, Yan P, Yang X (2021) Knowledge map visualization of technology hotspots and development trends in China’s textile manufacturing industry. IET Collab Intell Manuf 3(3):243–251. https://doi.org/10.1049/cim2.12024 Article ADS Google Scholar Jing Y, Wang C, Chen Y, Wang H, Yu T, Shadiev R (2023) Bibliometric mapping techniques in educational technology research: A systematic literature review. Educ Inf Technol 1–29. https://doi.org/10.1007/s10639-023-12178-6 Jing YH, Wang CL, Chen ZY, Shen SS, Shadiev R (2024a) A Bibliometric Analysis of Studies on Technology-Supported Learning Environments: Hotopics and Frontier Evolution. J Comput Assist Learn 1–16. https://doi.org/10.1111/jcal.12934 Jing YH, Wang HM, Chen XJ, Wang CL (2024b) What factors will affect the effectiveness of using ChatGPT to solve programming problems? A quasi-experimental study. Humanit Soc Sci Commun 11:319. https://doi.org/10.1057/s41599-024-02751-w Kamrani P, Dorsch I, Stock WG (2021) Do researchers know what the h-index is? And how do they estimate its importance? Scientometrics 126(7):5489–5508. https://doi.org/10.1007/s11192-021-03968-1 Kim HS, Lee KH, Kim H, Kim JH (2014) Using mobile phones in healthcare management for the elderly. Maturitas 79(4):381–388. https://doi.org/10.1016/j.maturitas.2014.08.013 Article MathSciNet PubMed Google Scholar Kleinberg J (2002) Bursty and hierarchical structure in streams. In Proceedings of the eighth ACM SIGKDD international conference on Knowledge discovery and data mining, pp. 91–101. https://doi.org/10.1145/775047.775061 Kruse C, Fohn J, Wilson N, Patlan EN, Zipp S, Mileski M (2020) Utilization barriers and medical outcomes commensurate with the use of telehealth among older adults: systematic review. JMIR Med Inform 8(8):e20359. https://doi.org/10.2196/20359 Kumar S, Lim WM, Pandey N, Christopher Westland J (2021) 20 years of electronic commerce research. Electron Commer Res 21:1–40. https://doi.org/10.1007/s10660-021-09464-1 Kwiek M (2021) What large-scale publication and citation data tell us about international research collaboration in Europe: Changing national patterns in global contexts. Stud High Educ 46(12):2629–2649. https://doi.org/10.1080/03075079.2020.1749254 Lee C, Coughlin JF (2015) PERSPECTIVE: Older adults’ adoption of technology: an integrated approach to identifying determinants and barriers. J Prod Innov Manag 32(5):747–759. https://doi.org/10.1111/jpim.12176 Lee CH, Wang C, Fan X, Li F, Chen CH (2023) Artificial intelligence-enabled digital transformation in elderly healthcare field: scoping review. Adv Eng Inform 55:101874. https://doi.org/10.1016/j.aei.2023.101874 Leydesdorff L, Rafols I (2012) Interactive overlays: A new method for generating global journal maps from Web-of-Science data. J Informetr 6(2):318–332. https://doi.org/10.1016/j.joi.2011.11.003 Li J, Ma Q, Chan AH, Man S (2019) Health monitoring through wearable technologies for older adults: Smart wearables acceptance model. Appl Ergon 75:162–169. https://doi.org/10.1016/j.apergo.2018.10.006 Article ADS PubMed Google Scholar Li X, Zhou D (2020) Product design requirement information visualization approach for intelligent manufacturing services. China Mech Eng 31(07):871, http://www.cmemo.org.cn/EN/Y2020/V31/I07/871 Google Scholar Lin Y, Yu Z (2024a) An integrated bibliometric analysis and systematic review modelling students’ technostress in higher education. Behav Inf Technol 1–25. https://doi.org/10.1080/0144929X.2024.2332458 Lin Y, Yu Z (2024b) A bibliometric analysis of artificial intelligence chatbots in educational contexts. Interact Technol Smart Educ 21(2):189–213. https://doi.org/10.1108/ITSE-12-2022-0165 Liu L, Duffy VG (2023) Exploring the future development of Artificial Intelligence (AI) applications in chatbots: a bibliometric analysis. Int J Soc Robot 15(5):703–716. https://doi.org/10.1007/s12369-022-00956-0 Liu R, Li X, Chu J (2022) Evolution of applied variables in the research on technology acceptance of the elderly. In: International Conference on Human-Computer Interaction, Cham: Springer International Publishing, pp 500–520. https://doi.org/10.1007/978-3-031-05581-23_5 Luijkx K, Peek S, Wouters E (2015) “Grandma, you should do it—It’s cool” Older Adults and the Role of Family Members in Their Acceptance of Technology. Int J Environ Res Public Health 12(12):15470–15485. https://doi.org/10.3390/ijerph121214999 Lussier M, Lavoie M, Giroux S, Consel C, Guay M, Macoir J, Bier N (2018) Early detection of mild cognitive impairment with in-home monitoring sensor technologies using functional measures: a systematic review. IEEE J Biomed Health Inform 23(2):838–847. https://doi.org/10.1109/JBHI.2018.2834317 López-Robles JR, Otegi-Olaso JR, Porto Gomez I, Gamboa-Rosales NK, Gamboa-Rosales H, Robles-Berumen H (2018) Bibliometric network analysis to identify the intellectual structure and evolution of the big data research field. In: International Conference on Intelligent Data Engineering and Automated Learning, Cham: Springer International Publishing, pp 113–120. https://doi.org/10.1007/978-3-030-03496-2_13 Ma Q, Chan AH, Chen K (2016) Personal and other factors affecting acceptance of smartphone technology by older Chinese adults. Appl Ergon 54:62–71. https://doi.org/10.1016/j.apergo.2015.11.015 Ma Q, Chan AHS, Teh PL (2021) Insights into Older Adults’ Technology Acceptance through Meta-Analysis. Int J Hum-Comput Interact 37(11):1049–1062. https://doi.org/10.1080/10447318.2020.1865005 Macedo IM (2017) Predicting the acceptance and use of information and communication technology by older adults: An empirical examination of the revised UTAUT2. Comput Human Behav 75:935–948. https://doi.org/10.1016/j.chb.2017.06.013 Maidhof C, Offermann J, Ziefle M (2023) Eyes on privacy: acceptance of video-based AAL impacted by activities being filmed. Front Public Health 11:1186944. https://doi.org/10.3389/fpubh.2023.1186944 Majumder S, Aghayi E, Noferesti M, Memarzadeh-Tehran H, Mondal T, Pang Z, Deen MJ (2017) Smart homes for elderly healthcare—Recent advances and research challenges. Sensors 17(11):2496. https://doi.org/10.3390/s17112496 Article ADS PubMed PubMed Central Google Scholar Mhlanga D (2023) Artificial Intelligence in elderly care: Navigating ethical and responsible AI adoption for seniors. Available at SSRN 4675564. 4675564 min) Identifying citation patterns of scientific breakthroughs: A perspective of dynamic citation process. Inf Process Manag 58(1):102428. https://doi.org/10.1016/j.ipm.2020.102428 Mitzner TL, Boron JB, Fausset CB, Adams AE, Charness N, Czaja SJ, Sharit J (2010) Older adults talk technology: Technology usage and attitudes. Comput Human Behav 26(6):1710–1721. https://doi.org/10.1016/j.chb.2010.06.020 Mitzner TL, Savla J, Boot WR, Sharit J, Charness N, Czaja SJ, Rogers WA (2019) Technology adoption by older adults: Findings from the PRISM trial. Gerontologist 59(1):34–44. https://doi.org/10.1093/geront/gny113 Mongeon P, Paul-Hus A (2016) The journal coverage of Web of Science and Scopus: a comparative analysis. Scientometrics 106:213–228. https://doi.org/10.1007/s11192-015-1765-5 Mostaghel R (2016) Innovation and technology for the elderly: Systematic literature review. J Bus Res 69(11):4896–4900. https://doi.org/10.1016/j.jbusres.2016.04.049 Mujirishvili T, Maidhof C, Florez-Revuelta F, Ziefle M, Richart-Martinez M, Cabrero-García J (2023) Acceptance and privacy perceptions toward video-based active and assisted living technologies: Scoping review. J Med Internet Res 25:e45297. https://doi.org/10.2196/45297 Naseri RNN, Azis SN, Abas N (2023) A Review of Technology Acceptance and Adoption Models in Consumer Study. FIRM J Manage Stud 8(2):188–199. https://doi.org/10.33021/firm.v8i2.4536 Nguyen UP, Hallinger P (2020) Assessing the distinctive contributions of Simulation & Gaming to the literature, 1970–2019: A bibliometric review. Simul Gaming 51(6):744–769. https://doi.org/10.1177/1046878120941569 Olmedo-Aguirre JO, Reyes-Campos J, Alor-Hernández G, Machorro-Cano I, Rodríguez-Mazahua L, Sánchez-Cervantes JL (2022) Remote healthcare for elderly people using wearables: A review. Biosensors 12(2):73. https://doi.org/10.3390/bios12020073 Pan S, Jordan-Marsh M (2010) Internet use intention and adoption among Chinese older adults: From the expanded technology acceptance model perspective. Comput Human Behav 26(5):1111–1119. https://doi.org/10.1016/j.chb.2010.03.015 Pan X, Yan E, Cui M, Hua W (2018) Examining the usage, citation, and diffusion patterns of bibliometric map software: A comparative study of three tools. J Informetr 12(2):481–493. https://doi.org/10.1016/j.joi.2018.03.005 Park JS, Kim NR, Han EJ (2018) Analysis of trends in science and technology using keyword network analysis. J Korea Ind Inf Syst Res 23(2):63–73. https://doi.org/10.9723/jksiis.2018.23.2.063 Peek ST, Luijkx KG, Rijnaard MD, Nieboer ME, Van Der Voort CS, Aarts S, Wouters EJ (2016) Older adults’ reasons for using technology while aging in place. Gerontology 62(2):226–237. https://doi.org/10.1159/000430949 Peek ST, Luijkx KG, Vrijhoef HJ, Nieboer ME, Aarts S, van der Voort CS, Wouters EJ (2017) Origins and consequences of technology acquirement by independent-living seniors: Towards an integrative model. BMC Geriatr 17:1–18. https://doi.org/10.1186/s12877-017-0582-5 Peek ST, Wouters EJ, Van Hoof J, Luijkx KG, Boeije HR, Vrijhoef HJ (2014) Factors influencing acceptance of technology for aging in place: a systematic review. Int J Med Inform 83(4):235–248. https://doi.org/10.1016/j.ijmedinf.2014.01.004 Peek STM, Luijkx KG, Vrijhoef HJM, Nieboer ME, Aarts S, Van Der Voort CS, Wouters EJM (2019) Understanding changes and stability in the long-term use of technologies by seniors who are aging in place: a dynamical framework. BMC Geriatr 19:1–13. https://doi.org/10.1186/s12877-019-1241-9 Perez AJ, Siddiqui F, Zeadally S, Lane D (2023) A review of IoT systems to enable independence for the elderly and disabled individuals. Internet Things 21:100653. https://doi.org/10.1016/j.iot.2022.100653 Piau A, Wild K, Mattek N, Kaye J (2019) Current state of digital biomarker technologies for real-life, home-based monitoring of cognitive function for mild cognitive impairment to mild Alzheimer disease and implications for clinical care: systematic review. J Med Internet Res 21(8):e12785. https://doi.org/10.2196/12785 Pirzada P, Wilde A, Doherty GH, Harris-Birtill D (2022) Ethics and acceptance of smart homes for older adults. Inform Health Soc Care 47(1):10–37. https://doi.org/10.1080/17538157.2021.1923500 Pranckutė R (2021) Web of Science (WoS) and Scopus: The titans of bibliographic information in today’s academic world. Publications 9(1):12. https://doi.org/10.3390/publications9010012 Qian K, Zhang Z, Yamamoto Y, Schuller BW (2021) Artificial intelligence internet of things for the elderly: From assisted living to health-care monitoring. IEEE Signal Process Mag 38(4):78–88. https://doi.org/10.1109/MSP.2021.3057298 Redner S (1998) How popular is your paper? An empirical study of the citation distribution. Eur Phys J B-Condens Matter Complex Syst 4(2):131–134. https://doi.org/10.1007/s100510050359 Sayago S (ed.) (2019) Perspectives on human-computer interaction research with older people. Switzerland: Springer International Publishing. https://doi.org/10.1007/978-3-030-06076-3 Schomakers EM, Ziefle M (2023) Privacy vs. security: trade-offs in the acceptance of smart technologies for aging-in-place. Int J Hum Comput Interact 39(5):1043–1058. https://doi.org/10.1080/10447318.2022.2078463 Schroeder T, Dodds L, Georgiou A, Gewald H, Siette J (2023) Older adults and new technology: Mapping review of the factors associated with older adults’ intention to adopt digital technologies. JMIR Aging 6(1):e44564. https://doi.org/10.2196/44564 Seibert K, Domhoff D, Bruch D, Schulte-Althoff M, Fürstenau D, Biessmann F, Wolf-Ostermann K (2021) Application scenarios for artificial intelligence in nursing care: rapid review. J Med Internet Res 23(11):e26522. https://doi.org/10.2196/26522 Seuwou P, Banissi E, Ubakanma G (2016) User acceptance of information technology: A critical review of technology acceptance models and the decision to invest in Information Security. In: Global Security, Safety and Sustainability-The Security Challenges of the Connected World: 11th International Conference, ICGS3 2017, London, UK, January 18-20, 2017, Proceedings 11:230-251. Springer International Publishing. https://doi.org/10.1007/978-3-319-51064-4_19 Shiau WL, Wang X, Zheng F (2023) What are the trend and core knowledge of information security? A citation and co-citation analysis. Inf Manag 60(3):103774. https://doi.org/10.1016/j.im.2023.103774 Sinha S, Verma A, Tiwari P (2021) Technology: Saving and enriching life during COVID-19. Front Psychol 12:647681. https://doi.org/10.3389/fpsyg.2021.647681 Soar J (2010) The potential of information and communication technologies to support ageing and independent living. Ann Telecommun 65:479–483. https://doi.org/10.1007/s12243-010-0167-1 Strotmann A, Zhao D (2012) Author name disambiguation: What difference does it make in author‐based citation analysis? J Am Soc Inf Sci Technol 63(9):1820–1833. https://doi.org/10.1002/asi.22695 Talukder MS, Sorwar G, Bao Y, Ahmed JU, Palash MAS (2020) Predicting antecedents of wearable healthcare technology acceptance by elderly: A combined SEM-Neural Network approach. Technol Forecast Soc Change 150:119793. https://doi.org/10.1016/j.techfore.2019.119793 Taskin Z, Al U (2019) Natural language processing applications in library and information science. Online Inf Rev 43(4):676–690. https://doi.org/10.1108/oir-07-2018-0217 Touqeer H, Zaman S, Amin R, Hussain M, Al-Turjman F, Bilal M (2021) Smart home security: challenges, issues and solutions at different IoT layers. J Supercomput 77(12):14053–14089. https://doi.org/10.1007/s11227-021-03825-1 United Nations Department of Economic and Social Affairs (2023) World population ageing 2023: Highlights. https://www.un.org/zh/193220 Valk CAL, Lu Y, Randriambelonoro M, Jessen J (2018) Designing for technology acceptance of wearable and mobile technologies for senior citizen users. In: 21st DMI: Academic Design Management Conference (ADMC 2018), Design Management Institute, pp 1361–1373. https://www.dmi.org/page/ADMC2018 Van Eck N, Waltman L (2010) Software survey: VOSviewer, a computer program for bibliometric mapping. Scientometrics 84(2):523–538. https://doi.org/10.1007/s11192-009-0146-3 Vancea M, Solé-Casals J (2016) Population aging in the European Information Societies: towards a comprehensive research agenda in eHealth innovations for elderly. Aging Dis 7(4):526. https://doi.org/10.14336/AD.2015.1214 Venkatesh V, Morris MG, Davis GB, Davis FD (2003) User acceptance of information technology: Toward a unified view. MIS Q 27(3):425–478. https://doi.org/10.2307/30036540 Wagner N, Hassanein K, Head M (2010) Computer use by older adults: A multi-disciplinary review. Comput Human Behav 26(5):870–882. https://doi.org/10.1016/j.chb.2010.03.029 Wahlroos N, Narsakka N, Stolt M, Suhonen R (2023) Physical environment maintaining independence and self-management of older people in long-term care settings—An integrative literature review. J Aging Environ 37(3):295–313. https://doi.org/10.1080/26892618.2022.2092927 Wang CL, Chen XJ, Yu T, Liu YD, Jing YH (2024a) Education reform and change driven by digital technology: a bibliometric study from a global perspective. Humanit Soc Sci Commun 11(1):1–17. https://doi.org/10.1057/s41599-024-02717-y Wang CL, Dai J, Zhu KK, Yu T, Gu XQ (2023a) Understanding the Continuance Intention of College Students Toward New E-learning Spaces Based on an Integrated Model of the TAM and TTF. Int J Hum-comput Int 1–14. https://doi.org/10.1080/10447318.2023.2291609 Wang CL, Wang HM, Li YY, Dai J, Gu XQ, Yu T (2024b) Factors Influencing University Students’ Behavioral Intention to Use Generative Artificial Intelligence: Integrating the Theory of Planned Behavior and AI Literacy. Int J Hum-comput Int 1–23. https://doi.org/10.1080/10447318.2024.2383033 Wang J, Zhao W, Zhang Z, Liu X, Xie T, Wang L, Zhang Y (2024c) A journey of challenges and victories: a bibliometric worldview of nanomedicine since the 21st century. Adv Mater 36(15):2308915. https://doi.org/10.1002/adma.202308915 Wang J, Chen Y, Huo S, Mai L, Jia F (2023b) Research hotspots and trends of social robot interaction design: A bibliometric analysis. Sensors 23(23):9369. https://doi.org/10.3390/s23239369 Wang KH, Chen G, Chen HG (2017) A model of technology adoption by older adults. Soc Behav Personal 45(4):563–572. https://doi.org/10.2224/sbp.5778 Wang S, Bolling K, Mao W, Reichstadt J, Jeste D, Kim HC, Nebeker C (2019) Technology to Support Aging in Place: Older Adults’ Perspectives. Healthcare 7(2):60. https://doi.org/10.3390/healthcare7020060 Wang Z, Liu D, Sun Y, Pang X, Sun P, Lin F, Ren K (2022) A survey on IoT-enabled home automation systems: Attacks and defenses. IEEE Commun Surv Tutor 24(4):2292–2328. https://doi.org/10.1109/COMST.2022.3201557 Wilkowska W, Offermann J, Spinsante S, Poli A, Ziefle M (2022) Analyzing technology acceptance and perception of privacy in ambient assisted living for using sensor-based technologies. PloS One 17(7):e0269642. https://doi.org/10.1371/journal.pone.0269642 Wilson J, Heinsch M, Betts D, Booth D, Kay-Lambkin F (2021) Barriers and facilitators to the use of e-health by older adults: a scoping review. BMC Public Health 21:1–12. https://doi.org/10.1186/s12889-021-11623-w Xia YQ, Deng YL, Tao XY, Zhang SN, Wang CL (2024) Digital art exhibitions and psychological well-being in Chinese Generation Z: An analysis based on the S-O-R framework. Humanit Soc Sci Commun 11:266. https://doi.org/10.1057/s41599-024-02718-x Xie H, Zhang Y, Duan K (2020) Evolutionary overview of urban expansion based on bibliometric analysis in Web of Science from 1990 to 2019. Habitat Int 95:102100. https://doi.org/10.1016/j.habitatint.2019.10210 Xu Z, Ge Z, Wang X, Skare M (2021) Bibliometric analysis of technology adoption literature published from 1997 to 2020. Technol Forecast Soc Change 170:120896. https://doi.org/10.1016/j.techfore.2021.120896 Yap YY, Tan SH, Choon SW (2022) Elderly’s intention to use technologies: a systematic literature review. Heliyon 8(1). https://doi.org/10.1016/j.heliyon.2022.e08765 Yu T, Dai J, Wang CL (2023) Adoption of blended learning: Chinese university students’ perspectives. Humanit Soc Sci Commun 10:390. https://doi.org/10.1057/s41599-023-01904-7 Yusif S, Soar J, Hafeez-Baig A (2016) Older people, assistive technologies, and the barriers to adoption: A systematic review. Int J Med Inform 94:112–116. https://doi.org/10.1016/j.ijmedinf.2016.07.004 Zhang J, Zhu L (2022) Citation recommendation using semantic representation of cited papers’ relations and content. Expert Syst Appl 187:115826. https://doi.org/10.1016/j.eswa.2021.115826 Zhao Y, Li J (2024) Opportunities and challenges of integrating artificial intelligence in China’s elderly care services. Sci Rep 14(1):9254. https://doi.org/10.1038/s41598-024-60067-w Article ADS MathSciNet PubMed PubMed Central Google Scholar Download references AcknowledgementsThis research was supported by the Social Science Foundation of Shaanxi Province in China (Grant No. 2023J014). Author informationAuthors and affiliations. School of Art and Design, Shaanxi University of Science and Technology, Xi’an, China Xianru Shang, Zijian Liu, Chen Gong, Zhigang Hu & Yuexuan Wu Department of Education Information Technology, Faculty of Education, East China Normal University, Shanghai, China Chengliang Wang You can also search for this author in PubMed Google Scholar ContributionsConceptualization, XS, YW, CW; methodology, XS, ZL, CG, CW; software, XS, CG, YW; writing-original draft preparation, XS, CW; writing-review and editing, XS, CG, ZH, CW; supervision, ZL, ZH, CW; project administration, ZL, ZH, CW; funding acquisition, XS, CG. All authors read and approved the final manuscript. All authors have read and approved the re-submission of the manuscript. Corresponding authorCorrespondence to Chengliang Wang . Ethics declarationsCompeting interests. The authors declare no competing interests. Ethical approvalEthical approval was not required as the study did not involve human participants. Informed consentInformed consent was not required as the study did not involve human participants. Additional informationPublisher’s note Springer Nature remains neutral with regard to jurisdictional claims in published maps and institutional affiliations. Rights and permissionsOpen Access This article is licensed under a Creative Commons Attribution-NonCommercial-NoDerivatives 4.0 International License, which permits any non-commercial use, sharing, distribution and reproduction in any medium or format, as long as you give appropriate credit to the original author(s) and the source, provide a link to the Creative Commons licence, and indicate if you modified the licensed material. You do not have permission under this licence to share adapted material derived from this article or parts of it. The images or other third party material in this article are included in the article’s Creative Commons licence, unless indicated otherwise in a credit line to the material. If material is not included in the article’s Creative Commons licence and your intended use is not permitted by statutory regulation or exceeds the permitted use, you will need to obtain permission directly from the copyright holder. To view a copy of this licence, visit http://creativecommons.org/licenses/by-nc-nd/4.0/ . Reprints and permissions About this articleCite this article. Shang, X., Liu, Z., Gong, C. et al. Knowledge mapping and evolution of research on older adults’ technology acceptance: a bibliometric study from 2013 to 2023. Humanit Soc Sci Commun 11 , 1115 (2024). https://doi.org/10.1057/s41599-024-03658-2 Download citation Received : 20 June 2024 Accepted : 21 August 2024 Published : 31 August 2024 DOI : https://doi.org/10.1057/s41599-024-03658-2 Share this articleAnyone you share the following link with will be able to read this content: Sorry, a shareable link is not currently available for this article. Provided by the Springer Nature SharedIt content-sharing initiative Quick links
![]() ![]()
| This article contains that is inaccurate for the current version. It was last updated for . Unreflected changes in the discussed game mechanics are detailed here: • • • • • | |

The Research Tree for the BASE GAME Automation Innovation Pack
Research is an Errand done at a Research Station , Super Computer , Telescope , Virtual Planetarium as well as Geysers . Research speed can be improved by higher duplicant Science and a lit workspace.
Viewing the Research Tree requires the colony have either a Research Station, Super Computer or Virtual Planetarium.
Base Game [ | ]
The Research Station is available at the start of the game. It provides Novice Research points which are required to research every tech in the game. In order to perform Novice Research, you must have power and Dirt delivered to your Research Station . As you will not have access to the higher tier power generators when you start, you will need a Manual Generator . Each Novice Research point costs 50 kg of dirt. A total of 143 tons of dirt will be needed to complete all research.
The Super Computer is available after researching the Advanced Research tech. Requiring a Duplicant with the Advanced Research skill , it provides Advanced Research points which are needed for every tech in the third tier of the research tree and beyond. It requires power and 50 kg of Water per research point. A total of 137 tons of water will be needed to complete all research.
The Telescope is available after researching the Celestial Detection tech. It requires a duplicant with the Field Research skill , and is required to view the Starmap and allows for the research of interstellar bodies.
The Virtual Planetarium is available after researching the Computing tech. It needs to be operated by a duplicant with the Astronomy skill and provides Interstellar Research points which are needed for tier 8 research and higher. Every Interstellar Research point costs power and 1 Data Bank . A total of 2,800 data banks will be needed to complete all research. Data Banks can only be acquired via space missions with Research Modules installed.
- The only ways to improve the Learning skill are tied to mastering a Research job, either to conduct research or to analyze objects, and there's a limited amount of both available. It's advisable to adjust priorities so that only dupes that currently run the Research jobs are conducting the errand.
- In Spaced Out!, the entire research tree requires 3,415 Novice Research points, 3,280 Advanced Research points, 2,715 Applied Sciences Research points, and 1,730 Data Analysis Research points.
- Analyzing Geysers takes a long time, and many geysers are very dangerous. Either wait until the geyser is dormant, or seal the geyser chamber beforehand and make sure that researchers wears an Atmo Suit .
See also [ | ]
- Category:Research – for a full list of research.
- 2 Steam Turbine
UW-Madison Libraries
Available 9 a.m.–5 p.m.
Additional Options
- smartphone Call / Text
- voice_chat Consultation Appointment
- place Visit
- email Email
Chat with a Specific library
- Business Library Business Library Chat is Offline
- College Library (Undergraduate) College Library Chat is Offline
- Ebling Library (Health Sciences) Ebling Library Chat is Offline
- Gender and Women's Studies Librarian GWS Library Chat is Offline
- Information School Library (Information Studies) iSchool Library Chat is Offline
- Law Library (Law) Law Library Chat is Offline
- Memorial Library (Humanities & Social Sciences) Memorial Library Chat is Offline
- MERIT Library (Education) MERIT Library Chat is Offline
- Steenbock Library (Agricultural & Life Sciences, Engineering) Steenbock Library Chat is Offline
- Ask a Librarian Hours & Policy
- Library Research Tutorials
Search the for Website expand_more Articles Find articles in journals, magazines, newspapers, and more Catalog Explore books, music, movies, and more Databases Locate databases by title and description Journals Find journal titles UWDC Discover digital collections, images, sound recordings, and more Website Find information on spaces, staff, services, and more
Language website search.
Find information on spaces, staff, and services.
- ASK a Librarian
- Library by Appointment
- Locations & Hours
- Resources by Subject
book Catalog Search
Search the physical and online collections at UW-Madison, UW System libraries, and the Wisconsin Historical Society.
- Available Online
- Print/Physical Items
- Limit to UW-Madison
- Advanced Search
- Browse by...
collections_bookmark Database Search
Find databases subscribed to by UW-Madison Libraries, searchable by title and description.
- Browse by Subject/Type
- Introductory Databases
- Top 10 Databases
article Journal Search
Find journal titles available online and in print.
- Browse by Subject / Title
- Citation Search
description Article Search
Find articles in journals, magazines, newspapers, and more.
- Scholarly (peer-reviewed)
- Open Access
- Library Databases
collections UW Digital Collections Search
Discover digital objects and collections curated by the UW-Digital Collections Center .
- Browse Collections
- Browse UWDC Items
- University of Wisconsin–Madison
- Email/Calendar
- Google Apps
- Loans & Requests
- Poster Printing
- Account Details
- Archives and Special Collections Requests
- Library Room Reservations
Learn how to use Python and/or R programming languages for data analysis (via Zoom)
Learn programming skills for computational research during the R workshop series and the Python workshop series. Attend any or all of the sessions. Brought to you as a part of the UW Libraries Graduate Support workshop series. Open to all UW-Madison students, faculty, and staff.
Location: Instruction online via Zoom. Connection information will be sent in advance.
The R Series
*Registration required. Registration is by workshop, not for the entire series. See links below to register for individual workshops. Sessions are filling up fast!
To find out more about this series, see: https://researchguides.library.wisc.edu/R
Friday, September 20, 10am-12pm R Programming: R Basics Register: https://go.wisc.edu/mwzmu0
This workshop is for the absolute beginner wanting to slowly walk through the process of getting started with R, a programming language commonly used for data analysis. The session will introduce you to the RStudio interface for coding in R. We will work through setting up a project directory, cover key concepts and terminology, and load and inspect a dataset. This workshop is geared toward programming novices, so no previous experience is required.
Friday, September 27, 10am-12pm R Programming: R Basics (repeat) Register: https://go.wisc.edu/ios5k1
This workshop is an exact repeat of the September 20th “R Programming: R Basics” workshop (see above).
Friday, October 4, 10am-12pm R Programming: Data Wrangling Register: https://go.wisc.edu/zx7b11
Data is rarely perfect out of the box. This workshop will cover how to manipulate datasets using an R package called dplyr. After this session, you will be able to select rows and columns, add new columns, remove missing data and create summary tables of your data. A basic working knowledge of R and RStudio (e.g., functions, operators, data types) would be helpful for you to get the most out of this session.
Friday, October 11, 10am-12pm R Programming: Data Visualization Register: https://go.wisc.edu/3480bq
So you’re familiar with R, but want to do more with your plots than the base graphics package. This workshop will show you how to use the ggplot2 package in R. After this session, you will be able to create a variety of plot types, alter their aesthetics, and create custom themes. A working knowledge of R, RStudio, and dplyr would be helpful for you to get the most out of this session.
Friday, October 18, 10am-12pm R Programming: Reports Register: https://go.wisc.edu/s52531
One way to automate your reports is to create files with human readable text and machine readable code. This workshop will cover creating reproducible reports of this type in RStudio using Quarto. After this session, you will be able to create Quarto documents, add formatted text and executable code blocks, and render the document into a final report. A working knowledge of R and RStudio would be helpful for you to get the most out of this session.
Friday, October 25, 10am-12:30pm R Programming: README Files in RStudio Register: https://go.wisc.edu/23sblv
Documenting your analysis in a way that is understandable to a colleague (or yourself 3 months later) can be challenging. README files are text documents that record your computational environment, methodologies, and more. After this session, you will be able to use Quarto in RStudio to create a README file template. A working knowledge of R and RStudio, and some experience with a markdown language would be helpful for you to get the most out of this session.
The Python Series
*Registration required. Registration is by workshop, not for the entire series. See links below to register for individual workshops. Sessions are filling up fast!
To find out more about this series, see: https://researchguides.library.wisc.edu/python
Tuesday, September 17, 10am-12pm Python Programming: Introduction Register: https://go.wisc.edu/wwsod8
This workshop is for the absolute beginner wanting to slowly walk through the process of getting started with Python, a programming language commonly used for data analysis. We’ll work through installation and setup of some helpful software and introduce basic concepts and terminology used in Python. Finally, we’ll work together to create your first simple but useful program! This workshop is geared toward programming novices, so no previous experience is required.
Tuesday, September 24, 10am-12pm Python Programming: Introduction (repeat) Register: https://go.wisc.edu/ah44bj
This workshop is an exact repeat of the September 17th “Python: Introduction” workshop (see above).
Tuesday, October 1, 10am-12pm Python Programming: Loops, Lists, and Functions Register: https://go.wisc.edu/ay0m55
This workshop will take a deeper dive into Python, covering essential topics such as automating tasks using loops, lists, and functions. Prerequisite: Understanding of basic Python concepts (e.g., variables, data types) is helpful.
Tuesday, October 8, 10am-12pm Python Programming: Spreadsheets and Data Manipulation Register: https://go.wisc.edu/x0q10i
Real-world data can be messy. This workshop will cover a range of topics related to organizing and manipulating spreadsheet data for more effective analysis. We’ll use pandas, a popular and free data analysis library written for Python. Prerequisite: Understanding of basic Python concepts (e.g., functions, operators, data types) is helpful.
Tuesday, October 15, 10am-12pm Python Programming: Data Visualization with Seaborn Register: https://go.wisc.edu/uto86g
In this workshop, we will explore different methods and tools for visualizing data. We’ll use seaborn, a popular and free data visualization library written for Python. Prerequisite: Understanding of basic Python concepts (e.g., functions, operators, data types) is helpful.
Work shop Organizers
Heather shimon.
Heather Shimon is a Science and Engineering Librarian specializing research data management.
Questions? [email protected]
Trisha Adamus
Trisha Adamus is a Health Sciences Librarian at Ebling Library specializing data services.
Questions? [email protected]
Dave Bloom is a Science and Engineering Librarian specializing in research data management.
Questions? [email protected]
Lisa Abler is a Science and Engineering Librarian specializing in research data management.
Questions? [email protected]
Instructors:
Imraan Alas, Researcher
Chris Endemann, Data Science Facilitator, Data Science Hub
Erwin Lares, Data Science Platform Lead, Research Cyberinfrastructure, Division of Information Technology (DoIT)
Casey Schacher, Research Storage Lead, Research Cyberinfrastructure, Division of Information Technology (DoIT)
John Shadle, Health Equity Survey Analyst, University Health Services
Sarah Stevens, Director, Data Science Hub
Katie Dunn, Electronic Resources Librarian, University of Wisconsin Law Library
Sarah Graves, Scientist, Forest & Wildlife Ecology
Corey Halpin, Software Engineer, Internet Scout
Todd Hayes-Birchler, Database Administrator, School of Medicine and Public Health
Annika Pratt, Research Assistant, Plant Pathology
Caitlin Roa, Academic Program Specialist, Department of Psychology
Angel Tang, Science & Engineering Librarian, UW-Madison Libraries
Kimberlie Vera, Research Assistant, Forest & Wildlife Ecology
Sarah Whitcomb, Research Scientist, USDA
Maria Widmer, Instructional Design & Engagement Specialist, L&S Instructional Design Collaborative
This website uses cookies to ensure you get the best experience. Learn more about DOAJ’s privacy policy.
Hide this message
You are using an outdated browser. Please upgrade your browser to improve your experience and security.
The Directory of Open Access Journals
Quick search.
Ianna Journal of Interdisciplinary Studies (Aug 2024)
Can the Digital Software Method Outperform the Manual Method in Qualitative Data Analysis? Findings from a Quasi-experimental Research
- Ugochukwu Simeon Asogwa,
- Hannah Ifedapo Maiyekogbon,
- Margaret Offoboche Agada-Mba,
- Oluwaseyi John Jemisenia
Affiliations
Read online
Background: In the dynamic field of qualitative research, a contentious issue persists: Is digital software a more effective tool for research analysis than the manual method? To shed light on this debate, we undertook quasi-experimental research, focusing on our study's unique contribution to exploring the capabilities of both methods in analysing health datasets. Objective: Our study aims to compare the effectiveness of qualitative analysis between researchers who are proficient in digital software and those skilled in the manual method. We seek to understand which method is more effective in data analysis. Methodology: We employed a quasi-experimental design and a purposive sampling approach to select our study participants. These participants (n=150) were then divided into two groups: those proficient in digital software and those skilled in the manual method. We then conducted an intervention in which participants analysed a qualitative dataset using their preferred method. The data collected was then analysed using quantitative measures, such as percentages, central tendency measures, and independent samples t-tests. Results: The t-test result showed that statistically significant differences exist between the two groups across all indicators (all Ps<.0001). Specific observation of the mean scores revealed that for perceived efficiency (M=3.50 [SD=0.55]), productivity (M=3.40 [SD=0.60]), collaboration (M=3.55 [SD=0.50]), identification of complex themes (M=3.60 [0.45]), and visualisation techniques(M=3.60 [SD=0.45]), participants who used digital software scored higher than those who used manual method of data analysis. However, for perceived depth of analysis (M=3.50 [SD=0.55]), coding flexibility(M=3.45 [SD=0.50]), reflective quality(M=3.60 [SD=0.50]) and integration of contextual knowledge(M=3.55 [SD=0.45]), participants in the manual method group scored higher compared to those in the digital software group Contribution: This study adds to burgeoning and existing knowledge on the need for a complementary approach to adopting and using digital tools and manual methods in conducting qualitative data analysis. Although using both methods can offer many benefits, it is crucial to use the advantages of one method to address the drawbacks of the other where possible. While these benefits should be observed when combining both methods, the challenges of both methods must be acknowledged. Conclusion: This study emphasises the complementary advantages of digital and manual qualitative data analysis methods. Recommendation: A well-rounded strategy that uses the benefits of both approaches is advised to provide thorough and complex qualitative research results.
- Qualitative data analysis
- Digital software
- Manual methods
- Quasi-experimental research
WeChat QR code
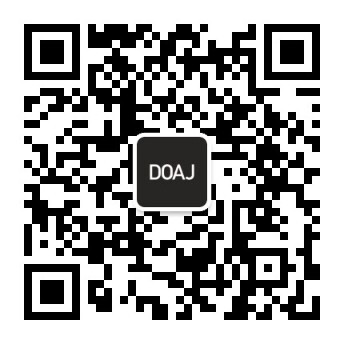
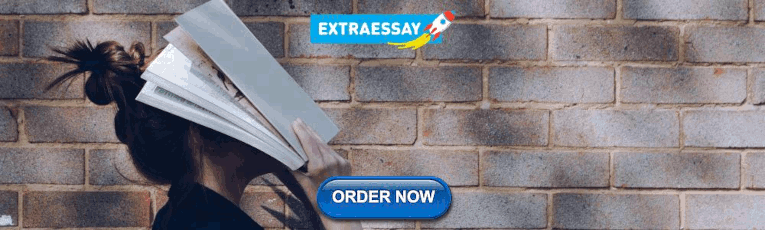
COMMENTS
Orbital Research is a tertiary tier of Research points. It is required for all tier 6 and higher research categories. Orbital Research Points are obtainable by a Duplicant with Orbital Researcher Skill to research at Orbital Microlab on open space, which consumes 5kg of Plastic per Research Point. Due to Rocketry changes in Spaced Out!, its much easier to send a Rocket into a space in early ...
Data Analysis Research is a tertiary tier of Research points. It is required for all tier 6 and higher research categories. Data Analysis Research Points are obtainable by a Duplicant by processing Data Banks at the Virtual Planetarium. Data Banks can be generated in a number of ways, including using the Orbital Data Collection Lab, studying Geysers, analyzing Artifacts, and searching Crashed ...
An overview of how to complete the Data Analysis Research so you can tech up all the way! How to get data banks and use them.Research & Scanning Playlist! ht...
Data Banks are a special resource that are used to research advanced rocketry technologies. Data banks are acquired on Space Missions by rockets with one or more Research Modules equipped. Every space mission destination has five areas of research which award 50 Data Banks each upon completion. One research module can study one area per mission. Once a destination is fully studied each ...
Orbital Data Collection Lab in Spaced Out generates Data Banks needed for Data Analysis Research. The lab requires Duplicant operation, as well as 5 kg of Plastic per Data Bank unit. This has a base speed of 33 s, accelerated by the duplicant's Machinery attribute. With a sustainable source of Plastic, the lab can supply a colony with research points indefinitely. To complete the entire ...
There is a bunch of useful stuff in the research tree that is locked behind the third and fourth kinds of research. (The first two kinds are covered earlier in this guide.) For the third kind of research you will need Data Banks, which in turn means you will have to send a dupe (and some plastic) into orbit. (Don't worry - you get the dupe back.)
TO ALL NEW PLAYERS (Data banks) Discussion. I've only just notice it and I've got 140 hours on the game, when you start your data analysis research you can "inspect" the printing pod for an extra data bank, so there is an extra one there for you alongside double check all gravitas buildings and naturally formed structures for an inspect button ...
Uses a standard spacefarer module with a data collection lab and standard telescope. Can sustain two dupes in space for long periods of research. In place of the standard telescope a second data collection lab can be installed to double the research output. Large Scope. Same as the regular research rocket, except it uses the large (enclosed ...
In this first release I focused on data extraction and relations, to provide the "Exploration" part, where is possible, for example, start from Oxygen, see all the possible way to produce oxygen and how you can use it and navigate from a relation to another.
and remember too, that adding this has allowed for, yes, a lengthier research process, but also an easier way to research, as you can get 1-4 basic data analysis-required researches before space. in the end, giving us a way to stack up research points for late game, and get earlier research for early game.
6. Sarkazeoh. • 1 yr. ago. It'd be kinda cool if there was a set of final research techs that boost dupe stats. Lightweight suit servos athletics +1 while suited. Hi power servos +1 strength while suited. etc. Maybe some that work without suits, these are just the first ideas to pop into my head. 6. sunderthebolt.
First thing to research is the pixel packs. That will get you enough morale to not worry about stress, and you can stop rebuilding the manual generator each day if you were doing that. Second thing to research is the small gas pump. It will be in place well before CO2 takes over the cabin. Duplicant +1 Barracks +1 Mess hall +3 Four shift break +3
Find relations between elements, information and attributes. Explore all the Oni element, building, plant, seed, critter, egg and food. Raw Data that can be processed into Data Analysis Research points. How to obtain Data Bank and how to use Data Bank ONI Db. Oxygen Not Included Database. Version 4.0.0 FF - Changelog. Elements Buildings ...
DESCRIPTION: Warm (red) and cold (blue) periods based on a threshold of +/- 0.5 o C for the Oceanic Niño Index (ONI) [3 month running mean of ERSST.v5 SST anomalies in the Niño 3.4 region (5 o N-5 o S, 120 o -170 o W)], based on centered 30-year base periods updated every 5 years.
At the simplest level, Saunders' (2007)research onion describes the different decisions you'll need to make when developing a research methodology - whether that's for your dissertation, thesis or any other formal research project. As you work from the outside of the onion inwards, you'll face a range of choices that progress from ...
ONI is here to support you along your super-resolution journey. Answer a few simple questions to discover key resources that can help you make the most of single-molecule imaging technology across a wide range of applications. I'm new to super-resolution microscopy I'm planning my experiment I'm ready to image I want to analyze my data.
This article is a practical guide to conducting data analysis in general literature reviews. The general literature review is a synthesis and analysis of published research on a relevant clinical issue, and is a common format for academic theses at the bachelor's and master's levels in nursing, physiotherapy, occupational therapy, public health and other related fields.
Research Module is a rocket module used to conduct research to further progress on the tech tree and survey space mission destinations. It weighs 200 kg. Note: This page references parts for the base game of Oxygen Not Included. For information about rocketry in Spaced Out, see Rocketry (Spaced Out). Each mission destination has five areas of research that can be surveyed once for 50 data ...
Yet another research rocket design. Build. Edit: Included more overlays, and minor adjustments. Contains a great hall (+6 morale), a washroom (+2), and a barracks (+1). Lit workspace for both orbital data collection lab. The maximum sustained radiation is 83 rads for the top data collection lab, but lower (~77 rads) once oxygen is pumped in.
38 Data Analysis Intern jobs available in Remote ; on Indeed.com. Apply to Research Intern, Intern, Marketing Intern and more!
By participating in the challenge, you will: leverage data types and formats from the GDC to develop your tool. help provide the cancer research community with new data analysis tools within the GDC.; use the GDC Analysis Tool Software Development Kit to integrate an analysis tool with the GDC data portal using data from the GDC.
Serum and Plasma Analysis at Biopsy. ... Diet Research Data:Effects of Quick Fat Diet on Female C57BL/6J Mice. Diet Research Data:Effects of Quick Fat Diet on Male C57BL/6J Mice. アーカイブ / Archives. 2024年09月 (2) 2024年08月 (18) 2024年07月 (10) 2024年06月 (12)
An analysis of 20 years of health funding data shows that federal transfers have mostly outpaced increases to provincial health budgets. ... research suggests. 1 year ago. Duration 2:56.
The shoebox-sized BurstCube satellite has observed its first gamma-ray burst, the most powerful kind of explosion in the universe, according to a recent analysis of observations collected over the last several months. "We're excited to collect science data," said Sean Semper, BurstCube's lead engineer at NASA's Goddard Space Flight Center in Greenbelt, Maryland. "It's an ...
The overarching objective of this paper is to survey, identify and qualitatively analyse all the published studies in the area of artificial intelligence in economics and business-related research with the application of the leading nonparametric DEA (Data Envelopment Analysis) methodology thus far, as well as to reveal their findings, map some ...
Research method. In recent years, bibliometrics has become one of the crucial methods for analyzing literature reviews and is widely used in disciplinary and industrial intelligence analysis (Jing ...
Research is an Errand done at a Research Station, Super Computer, Telescope, Virtual Planetarium as well as Geysers. Research speed can be improved by higher duplicant Science and a lit workspace. Viewing the Research Tree requires the colony have either a Research Station, Super Computer or Virtual Planetarium. The Research Station is available at the start of the game. It provides Novice ...
This is a mid-game sustainable orbital research rocket with a great hall, washroom and barracks, plus food storage. This requires a gas + liquid cargo module and a battery module, so can only be used once you have petroleum engines. P. Water is recycled and chilled to 25 degrees, providing cooling for the rocket interior.
Real-world data can be messy. This workshop will cover a range of topics related to organizing and manipulating spreadsheet data for more effective analysis. We'll use pandas, a popular and free data analysis library written for Python. Prerequisite: Understanding of basic Python concepts (e.g., functions, operators, data types) is helpful.
We seek to understand which method is more effective in data analysis. Methodology: We employed a quasi-experimental design and a purposive sampling approach to select our study participants. These participants (n=150) were then divided into two groups: those proficient in digital software and those skilled in the manual method.