Techniques or tools used for gathering research data include:
Qualitative Techniques or Tools | Quantitative Techniques or Tools |
: these can be structured, semi-structured or unstructured in-depth sessions with the researcher and a participant. | Surveys or questionnaires: which ask the same questions to large numbers of participants or use Likert scales which measure opinions as numerical data. |
: with several participants discussing a particular topic or a set of questions. Researchers can be facilitators or observers. | Observation: which can either involve counting the number of times a specific phenomenon occurs, or the coding of observational data in order to translate it into numbers. |
: On-site, in-context or role-play options. | Document screening: sourcing numerical data from financial reports or counting word occurrences. |
: Interrogation of correspondence (letters, diaries, emails etc) or reports. | Experiments: testing hypotheses in laboratories, testing cause and effect relationships, through field experiments, or via quasi- or natural experiments. |
: Remembrances or memories of experiences told to the researcher. | |
SAGE research methods
- SAGE research methods online This link opens in a new window Research methods tool to help researchers gather full-text resources, design research projects, understand a particular method and write up their research. Includes access to collections of video, business cases and eBooks,
Help and Information
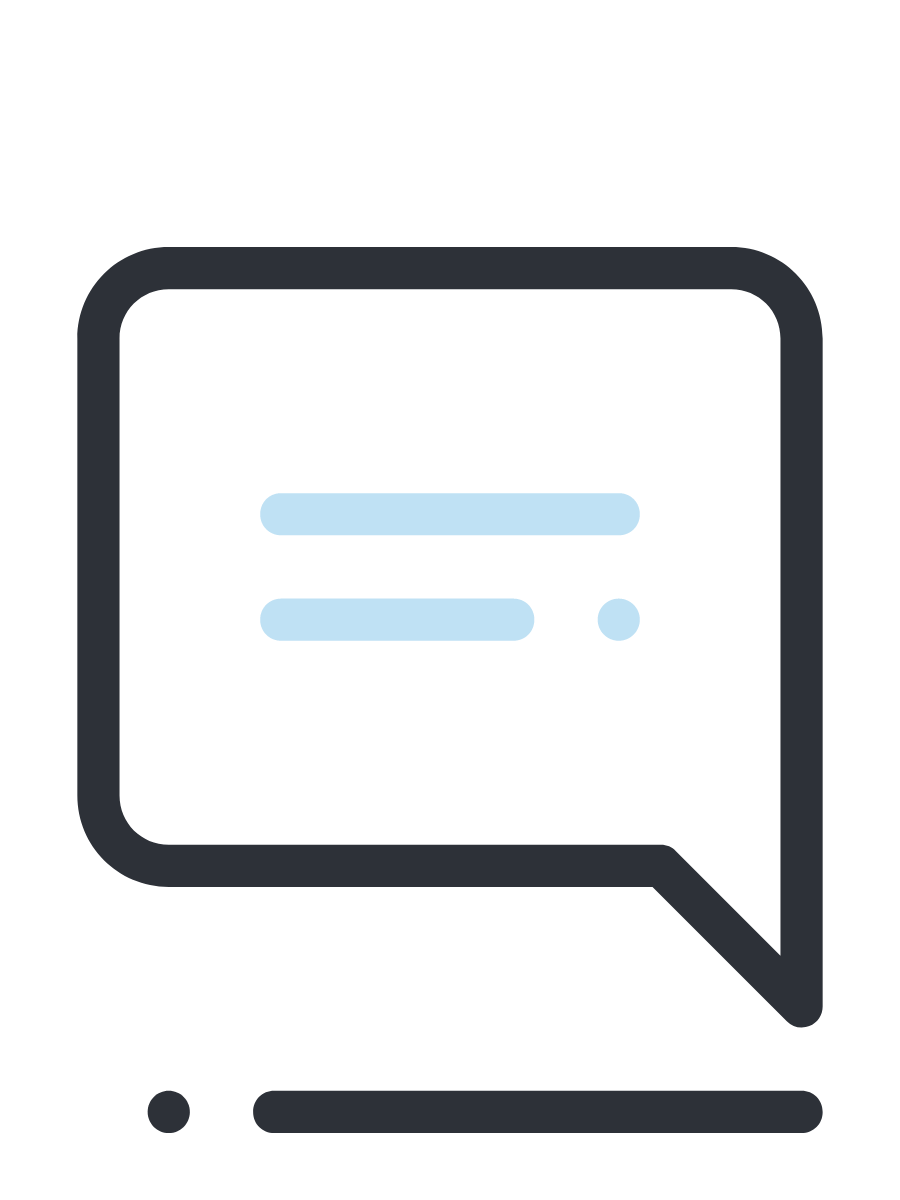
- Next: Finding qualitative research >>
- Last Updated: Aug 19, 2024 3:39 PM
- URL: https://libguides.newcastle.edu.au/researchmethods

- The Chicago School
- The Chicago School Library
- Research Guides
Quantitative Research Methods
What is quantitative research, about this guide, introduction, quantitative research methodologies.
- Key Resources
- Quantitative Software
- Finding Qualitative Studies
The purpose of this guide is to provide a starting point for learning about quantitative research. In this guide, you'll find:
- Resources on diverse types of quantitative research.
- An overview of resources for data, methods & analysis
- Popular quantitative software options
- Information on how to find quantitative studies
Research involving the collection of data in numerical form for quantitative analysis. The numerical data can be durations, scores, counts of incidents, ratings, or scales. Quantitative data can be collected in either controlled or naturalistic environments, in laboratories or field studies, from special populations or from samples of the general population. The defining factor is that numbers result from the process, whether the initial data collection produced numerical values, or whether non-numerical values were subsequently converted to numbers as part of the analysis process, as in content analysis.
Citation: Garwood, J. (2006). Quantitative research. In V. Jupp (Ed.), The SAGE dictionary of social research methods. (pp. 251-252). London, England: SAGE Publications. doi:10.4135/9780857020116
Watch the following video to learn more about Quantitative Research:
(Video best viewed in Edge and Chrome browsers, or click here to view in the Sage Research Methods Database)
Correlational
Researchers will compare two sets of numbers to try and identify a relationship (if any) between two things.
Descriptive
Researchers will attempt to quantify a variety of factors at play as they study a particular type of phenomenon or action. For example, researchers might use a descriptive methodology to understand the effects of climate change on the life cycle of a plant or animal.
Experimental
To understand the effects of a variable, researchers will design an experiment where they can control as many factors as possible. This can involve creating control and experimental groups. The experimental group will be exposed to the variable to study its effects. The control group provides data about what happens when the variable is absent. For example, in a study about online teaching, the control group might receive traditional face-to-face instruction while the experimental group would receive their instruction virtually.
Quasi-Experimental/Quasi-Comparative
Researchers will attempt to determine what (if any) effect a variable can have. These studies may have multiple independent variables (causes) and multiple dependent variables (effects), but this can complicate researchers' efforts to find out if A can cause B or if X, Y, and Z are also playing a role.
Surveys can be considered a quantitative methodology if the researchers require their respondents to choose from pre-determined responses.
- Next: Key Resources >>
- Last Updated: Aug 20, 2024 5:29 PM
- URL: https://library.thechicagoschool.edu/quantitative
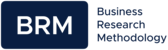
Regression Analysis
Regression analysis is a quantitative research method which is used when the study involves modelling and analysing several variables, where the relationship includes a dependent variable and one or more independent variables. In simple terms, regression analysis is a quantitative method used to test the nature of relationships between a dependent variable and one or more independent variables.
The basic form of regression models includes unknown parameters (β), independent variables (X), and the dependent variable (Y).
Regression model, basically, specifies the relation of dependent variable (Y) to a function combination of independent variables (X) and unknown parameters (β)
Y ≈ f (X, β)
Regression equation can be used to predict the values of ‘y’, if the value of ‘x’ is given, and both ‘y’ and ‘x’ are the two sets of measures of a sample size of ‘n’. The formulae for regression equation would be
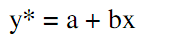
Do not be intimidated by visual complexity of correlation and regression formulae above. You don’t have to apply the formula manually, and correlation and regression analyses can be run with the application of popular analytical software such as Microsoft Excel, Microsoft Access, SPSS and others.
Linear regression analysis is based on the following set of assumptions:
1. Assumption of linearity . There is a linear relationship between dependent and independent variables.
2. Assumption of homoscedasticity . Data values for dependent and independent variables have equal variances.
3. Assumption of absence of collinearity or multicollinearity . There is no correlation between two or more independent variables.
4. Assumption of normal distribution . The data for the independent variables and dependent variable are normally distributed
My e-book, The Ultimate Guide to Writing a Dissertation in Business Studies: a step by step assistance offers practical assistance to complete a dissertation with minimum or no stress. The e-book covers all stages of writing a dissertation starting from the selection to the research area to submitting the completed version of the work within the deadline. John Dudovskiy
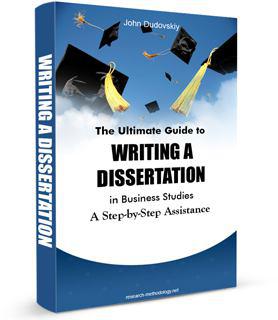
Have a language expert improve your writing
Run a free plagiarism check in 10 minutes, generate accurate citations for free.
Methodology
- Content Analysis | Guide, Methods & Examples
Content Analysis | Guide, Methods & Examples
Published on July 18, 2019 by Amy Luo . Revised on June 22, 2023.
Content analysis is a research method used to identify patterns in recorded communication. To conduct content analysis, you systematically collect data from a set of texts, which can be written, oral, or visual:
- Books, newspapers and magazines
- Speeches and interviews
- Web content and social media posts
- Photographs and films
Content analysis can be both quantitative (focused on counting and measuring) and qualitative (focused on interpreting and understanding). In both types, you categorize or “code” words, themes, and concepts within the texts and then analyze the results.
Table of contents
What is content analysis used for, advantages of content analysis, disadvantages of content analysis, how to conduct content analysis, other interesting articles.
Researchers use content analysis to find out about the purposes, messages, and effects of communication content. They can also make inferences about the producers and audience of the texts they analyze.
Content analysis can be used to quantify the occurrence of certain words, phrases, subjects or concepts in a set of historical or contemporary texts.
Quantitative content analysis example
To research the importance of employment issues in political campaigns, you could analyze campaign speeches for the frequency of terms such as unemployment , jobs , and work and use statistical analysis to find differences over time or between candidates.
In addition, content analysis can be used to make qualitative inferences by analyzing the meaning and semantic relationship of words and concepts.
Qualitative content analysis example
To gain a more qualitative understanding of employment issues in political campaigns, you could locate the word unemployment in speeches, identify what other words or phrases appear next to it (such as economy, inequality or laziness ), and analyze the meanings of these relationships to better understand the intentions and targets of different campaigns.
Because content analysis can be applied to a broad range of texts, it is used in a variety of fields, including marketing, media studies, anthropology, cognitive science, psychology, and many social science disciplines. It has various possible goals:
- Finding correlations and patterns in how concepts are communicated
- Understanding the intentions of an individual, group or institution
- Identifying propaganda and bias in communication
- Revealing differences in communication in different contexts
- Analyzing the consequences of communication content, such as the flow of information or audience responses
Prevent plagiarism. Run a free check.
- Unobtrusive data collection
You can analyze communication and social interaction without the direct involvement of participants, so your presence as a researcher doesn’t influence the results.
- Transparent and replicable
When done well, content analysis follows a systematic procedure that can easily be replicated by other researchers, yielding results with high reliability .
You can conduct content analysis at any time, in any location, and at low cost – all you need is access to the appropriate sources.
Focusing on words or phrases in isolation can sometimes be overly reductive, disregarding context, nuance, and ambiguous meanings.
Content analysis almost always involves some level of subjective interpretation, which can affect the reliability and validity of the results and conclusions, leading to various types of research bias and cognitive bias .
Manually coding large volumes of text is extremely time-consuming, and it can be difficult to automate effectively.
If you want to use content analysis in your research, you need to start with a clear, direct research question .
Example research question for content analysis
Is there a difference in how the US media represents younger politicians compared to older ones in terms of trustworthiness?
Next, you follow these five steps.
1. Select the content you will analyze
Based on your research question, choose the texts that you will analyze. You need to decide:
- The medium (e.g. newspapers, speeches or websites) and genre (e.g. opinion pieces, political campaign speeches, or marketing copy)
- The inclusion and exclusion criteria (e.g. newspaper articles that mention a particular event, speeches by a certain politician, or websites selling a specific type of product)
- The parameters in terms of date range, location, etc.
If there are only a small amount of texts that meet your criteria, you might analyze all of them. If there is a large volume of texts, you can select a sample .
2. Define the units and categories of analysis
Next, you need to determine the level at which you will analyze your chosen texts. This means defining:
- The unit(s) of meaning that will be coded. For example, are you going to record the frequency of individual words and phrases, the characteristics of people who produced or appear in the texts, the presence and positioning of images, or the treatment of themes and concepts?
- The set of categories that you will use for coding. Categories can be objective characteristics (e.g. aged 30-40 , lawyer , parent ) or more conceptual (e.g. trustworthy , corrupt , conservative , family oriented ).
Your units of analysis are the politicians who appear in each article and the words and phrases that are used to describe them. Based on your research question, you have to categorize based on age and the concept of trustworthiness. To get more detailed data, you also code for other categories such as their political party and the marital status of each politician mentioned.
3. Develop a set of rules for coding
Coding involves organizing the units of meaning into the previously defined categories. Especially with more conceptual categories, it’s important to clearly define the rules for what will and won’t be included to ensure that all texts are coded consistently.
Coding rules are especially important if multiple researchers are involved, but even if you’re coding all of the text by yourself, recording the rules makes your method more transparent and reliable.
In considering the category “younger politician,” you decide which titles will be coded with this category ( senator, governor, counselor, mayor ). With “trustworthy”, you decide which specific words or phrases related to trustworthiness (e.g. honest and reliable ) will be coded in this category.
4. Code the text according to the rules
You go through each text and record all relevant data in the appropriate categories. This can be done manually or aided with computer programs, such as QSR NVivo , Atlas.ti and Diction , which can help speed up the process of counting and categorizing words and phrases.
Following your coding rules, you examine each newspaper article in your sample. You record the characteristics of each politician mentioned, along with all words and phrases related to trustworthiness that are used to describe them.
5. Analyze the results and draw conclusions
Once coding is complete, the collected data is examined to find patterns and draw conclusions in response to your research question. You might use statistical analysis to find correlations or trends, discuss your interpretations of what the results mean, and make inferences about the creators, context and audience of the texts.
Let’s say the results reveal that words and phrases related to trustworthiness appeared in the same sentence as an older politician more frequently than they did in the same sentence as a younger politician. From these results, you conclude that national newspapers present older politicians as more trustworthy than younger politicians, and infer that this might have an effect on readers’ perceptions of younger people in politics.
If you want to know more about statistics , methodology , or research bias , make sure to check out some of our other articles with explanations and examples.
- Normal distribution
- Measures of central tendency
- Chi square tests
- Confidence interval
- Quartiles & Quantiles
- Cluster sampling
- Stratified sampling
- Thematic analysis
- Cohort study
- Peer review
- Ethnography
Research bias
- Implicit bias
- Cognitive bias
- Conformity bias
- Hawthorne effect
- Availability heuristic
- Attrition bias
- Social desirability bias
Cite this Scribbr article
If you want to cite this source, you can copy and paste the citation or click the “Cite this Scribbr article” button to automatically add the citation to our free Citation Generator.
Luo, A. (2023, June 22). Content Analysis | Guide, Methods & Examples. Scribbr. Retrieved August 22, 2024, from https://www.scribbr.com/methodology/content-analysis/
Is this article helpful?

Other students also liked
Qualitative vs. quantitative research | differences, examples & methods, descriptive research | definition, types, methods & examples, reliability vs. validity in research | difference, types and examples, what is your plagiarism score.
The 7 Most Useful Data Analysis Methods and Techniques
Data analytics is the process of analyzing raw data to draw out meaningful insights. These insights are then used to determine the best course of action.
When is the best time to roll out that marketing campaign? Is the current team structure as effective as it could be? Which customer segments are most likely to purchase your new product?
Ultimately, data analytics is a crucial driver of any successful business strategy. But how do data analysts actually turn raw data into something useful? There are a range of methods and techniques that data analysts use depending on the type of data in question and the kinds of insights they want to uncover.
You can get a hands-on introduction to data analytics in this free short course .
In this post, we’ll explore some of the most useful data analysis techniques. By the end, you’ll have a much clearer idea of how you can transform meaningless data into business intelligence. We’ll cover:
- What is data analysis and why is it important?
- What is the difference between qualitative and quantitative data?
- Regression analysis
- Monte Carlo simulation
- Factor analysis
- Cohort analysis
- Cluster analysis
- Time series analysis
- Sentiment analysis
- The data analysis process
- The best tools for data analysis
- Key takeaways
The first six methods listed are used for quantitative data , while the last technique applies to qualitative data. We briefly explain the difference between quantitative and qualitative data in section two, but if you want to skip straight to a particular analysis technique, just use the clickable menu.
1. What is data analysis and why is it important?
Data analysis is, put simply, the process of discovering useful information by evaluating data. This is done through a process of inspecting, cleaning, transforming, and modeling data using analytical and statistical tools, which we will explore in detail further along in this article.
Why is data analysis important? Analyzing data effectively helps organizations make business decisions. Nowadays, data is collected by businesses constantly: through surveys, online tracking, online marketing analytics, collected subscription and registration data (think newsletters), social media monitoring, among other methods.
These data will appear as different structures, including—but not limited to—the following:
The concept of big data —data that is so large, fast, or complex, that it is difficult or impossible to process using traditional methods—gained momentum in the early 2000s. Then, Doug Laney, an industry analyst, articulated what is now known as the mainstream definition of big data as the three Vs: volume, velocity, and variety.
- Volume: As mentioned earlier, organizations are collecting data constantly. In the not-too-distant past it would have been a real issue to store, but nowadays storage is cheap and takes up little space.
- Velocity: Received data needs to be handled in a timely manner. With the growth of the Internet of Things, this can mean these data are coming in constantly, and at an unprecedented speed.
- Variety: The data being collected and stored by organizations comes in many forms, ranging from structured data—that is, more traditional, numerical data—to unstructured data—think emails, videos, audio, and so on. We’ll cover structured and unstructured data a little further on.
This is a form of data that provides information about other data, such as an image. In everyday life you’ll find this by, for example, right-clicking on a file in a folder and selecting “Get Info”, which will show you information such as file size and kind, date of creation, and so on.
Real-time data
This is data that is presented as soon as it is acquired. A good example of this is a stock market ticket, which provides information on the most-active stocks in real time.
Machine data
This is data that is produced wholly by machines, without human instruction. An example of this could be call logs automatically generated by your smartphone.
Quantitative and qualitative data
Quantitative data—otherwise known as structured data— may appear as a “traditional” database—that is, with rows and columns. Qualitative data—otherwise known as unstructured data—are the other types of data that don’t fit into rows and columns, which can include text, images, videos and more. We’ll discuss this further in the next section.
2. What is the difference between quantitative and qualitative data?
How you analyze your data depends on the type of data you’re dealing with— quantitative or qualitative . So what’s the difference?
Quantitative data is anything measurable , comprising specific quantities and numbers. Some examples of quantitative data include sales figures, email click-through rates, number of website visitors, and percentage revenue increase. Quantitative data analysis techniques focus on the statistical, mathematical, or numerical analysis of (usually large) datasets. This includes the manipulation of statistical data using computational techniques and algorithms. Quantitative analysis techniques are often used to explain certain phenomena or to make predictions.
Qualitative data cannot be measured objectively , and is therefore open to more subjective interpretation. Some examples of qualitative data include comments left in response to a survey question, things people have said during interviews, tweets and other social media posts, and the text included in product reviews. With qualitative data analysis, the focus is on making sense of unstructured data (such as written text, or transcripts of spoken conversations). Often, qualitative analysis will organize the data into themes—a process which, fortunately, can be automated.
Data analysts work with both quantitative and qualitative data , so it’s important to be familiar with a variety of analysis methods. Let’s take a look at some of the most useful techniques now.
3. Data analysis techniques
Now we’re familiar with some of the different types of data, let’s focus on the topic at hand: different methods for analyzing data.
a. Regression analysis
Regression analysis is used to estimate the relationship between a set of variables. When conducting any type of regression analysis , you’re looking to see if there’s a correlation between a dependent variable (that’s the variable or outcome you want to measure or predict) and any number of independent variables (factors which may have an impact on the dependent variable). The aim of regression analysis is to estimate how one or more variables might impact the dependent variable, in order to identify trends and patterns. This is especially useful for making predictions and forecasting future trends.
Let’s imagine you work for an ecommerce company and you want to examine the relationship between: (a) how much money is spent on social media marketing, and (b) sales revenue. In this case, sales revenue is your dependent variable—it’s the factor you’re most interested in predicting and boosting. Social media spend is your independent variable; you want to determine whether or not it has an impact on sales and, ultimately, whether it’s worth increasing, decreasing, or keeping the same. Using regression analysis, you’d be able to see if there’s a relationship between the two variables. A positive correlation would imply that the more you spend on social media marketing, the more sales revenue you make. No correlation at all might suggest that social media marketing has no bearing on your sales. Understanding the relationship between these two variables would help you to make informed decisions about the social media budget going forward. However: It’s important to note that, on their own, regressions can only be used to determine whether or not there is a relationship between a set of variables—they don’t tell you anything about cause and effect. So, while a positive correlation between social media spend and sales revenue may suggest that one impacts the other, it’s impossible to draw definitive conclusions based on this analysis alone.
There are many different types of regression analysis, and the model you use depends on the type of data you have for the dependent variable. For example, your dependent variable might be continuous (i.e. something that can be measured on a continuous scale, such as sales revenue in USD), in which case you’d use a different type of regression analysis than if your dependent variable was categorical in nature (i.e. comprising values that can be categorised into a number of distinct groups based on a certain characteristic, such as customer location by continent). You can learn more about different types of dependent variables and how to choose the right regression analysis in this guide .
Regression analysis in action: Investigating the relationship between clothing brand Benetton’s advertising expenditure and sales
b. Monte Carlo simulation
When making decisions or taking certain actions, there are a range of different possible outcomes. If you take the bus, you might get stuck in traffic. If you walk, you might get caught in the rain or bump into your chatty neighbor, potentially delaying your journey. In everyday life, we tend to briefly weigh up the pros and cons before deciding which action to take; however, when the stakes are high, it’s essential to calculate, as thoroughly and accurately as possible, all the potential risks and rewards.
Monte Carlo simulation, otherwise known as the Monte Carlo method, is a computerized technique used to generate models of possible outcomes and their probability distributions. It essentially considers a range of possible outcomes and then calculates how likely it is that each particular outcome will be realized. The Monte Carlo method is used by data analysts to conduct advanced risk analysis, allowing them to better forecast what might happen in the future and make decisions accordingly.
So how does Monte Carlo simulation work, and what can it tell us? To run a Monte Carlo simulation, you’ll start with a mathematical model of your data—such as a spreadsheet. Within your spreadsheet, you’ll have one or several outputs that you’re interested in; profit, for example, or number of sales. You’ll also have a number of inputs; these are variables that may impact your output variable. If you’re looking at profit, relevant inputs might include the number of sales, total marketing spend, and employee salaries. If you knew the exact, definitive values of all your input variables, you’d quite easily be able to calculate what profit you’d be left with at the end. However, when these values are uncertain, a Monte Carlo simulation enables you to calculate all the possible options and their probabilities. What will your profit be if you make 100,000 sales and hire five new employees on a salary of $50,000 each? What is the likelihood of this outcome? What will your profit be if you only make 12,000 sales and hire five new employees? And so on. It does this by replacing all uncertain values with functions which generate random samples from distributions determined by you, and then running a series of calculations and recalculations to produce models of all the possible outcomes and their probability distributions. The Monte Carlo method is one of the most popular techniques for calculating the effect of unpredictable variables on a specific output variable, making it ideal for risk analysis.
Monte Carlo simulation in action: A case study using Monte Carlo simulation for risk analysis
c. Factor analysis
Factor analysis is a technique used to reduce a large number of variables to a smaller number of factors. It works on the basis that multiple separate, observable variables correlate with each other because they are all associated with an underlying construct. This is useful not only because it condenses large datasets into smaller, more manageable samples, but also because it helps to uncover hidden patterns. This allows you to explore concepts that cannot be easily measured or observed—such as wealth, happiness, fitness, or, for a more business-relevant example, customer loyalty and satisfaction.
Let’s imagine you want to get to know your customers better, so you send out a rather long survey comprising one hundred questions. Some of the questions relate to how they feel about your company and product; for example, “Would you recommend us to a friend?” and “How would you rate the overall customer experience?” Other questions ask things like “What is your yearly household income?” and “How much are you willing to spend on skincare each month?”
Once your survey has been sent out and completed by lots of customers, you end up with a large dataset that essentially tells you one hundred different things about each customer (assuming each customer gives one hundred responses). Instead of looking at each of these responses (or variables) individually, you can use factor analysis to group them into factors that belong together—in other words, to relate them to a single underlying construct. In this example, factor analysis works by finding survey items that are strongly correlated. This is known as covariance . So, if there’s a strong positive correlation between household income and how much they’re willing to spend on skincare each month (i.e. as one increases, so does the other), these items may be grouped together. Together with other variables (survey responses), you may find that they can be reduced to a single factor such as “consumer purchasing power”. Likewise, if a customer experience rating of 10/10 correlates strongly with “yes” responses regarding how likely they are to recommend your product to a friend, these items may be reduced to a single factor such as “customer satisfaction”.
In the end, you have a smaller number of factors rather than hundreds of individual variables. These factors are then taken forward for further analysis, allowing you to learn more about your customers (or any other area you’re interested in exploring).
Factor analysis in action: Using factor analysis to explore customer behavior patterns in Tehran
d. Cohort analysis
Cohort analysis is a data analytics technique that groups users based on a shared characteristic , such as the date they signed up for a service or the product they purchased. Once users are grouped into cohorts, analysts can track their behavior over time to identify trends and patterns.
So what does this mean and why is it useful? Let’s break down the above definition further. A cohort is a group of people who share a common characteristic (or action) during a given time period. Students who enrolled at university in 2020 may be referred to as the 2020 cohort. Customers who purchased something from your online store via the app in the month of December may also be considered a cohort.
With cohort analysis, you’re dividing your customers or users into groups and looking at how these groups behave over time. So, rather than looking at a single, isolated snapshot of all your customers at a given moment in time (with each customer at a different point in their journey), you’re examining your customers’ behavior in the context of the customer lifecycle. As a result, you can start to identify patterns of behavior at various points in the customer journey—say, from their first ever visit to your website, through to email newsletter sign-up, to their first purchase, and so on. As such, cohort analysis is dynamic, allowing you to uncover valuable insights about the customer lifecycle.
This is useful because it allows companies to tailor their service to specific customer segments (or cohorts). Let’s imagine you run a 50% discount campaign in order to attract potential new customers to your website. Once you’ve attracted a group of new customers (a cohort), you’ll want to track whether they actually buy anything and, if they do, whether or not (and how frequently) they make a repeat purchase. With these insights, you’ll start to gain a much better understanding of when this particular cohort might benefit from another discount offer or retargeting ads on social media, for example. Ultimately, cohort analysis allows companies to optimize their service offerings (and marketing) to provide a more targeted, personalized experience. You can learn more about how to run cohort analysis using Google Analytics .
Cohort analysis in action: How Ticketmaster used cohort analysis to boost revenue
e. Cluster analysis
Cluster analysis is an exploratory technique that seeks to identify structures within a dataset. The goal of cluster analysis is to sort different data points into groups (or clusters) that are internally homogeneous and externally heterogeneous. This means that data points within a cluster are similar to each other, and dissimilar to data points in another cluster. Clustering is used to gain insight into how data is distributed in a given dataset, or as a preprocessing step for other algorithms.
There are many real-world applications of cluster analysis. In marketing, cluster analysis is commonly used to group a large customer base into distinct segments, allowing for a more targeted approach to advertising and communication. Insurance firms might use cluster analysis to investigate why certain locations are associated with a high number of insurance claims. Another common application is in geology, where experts will use cluster analysis to evaluate which cities are at greatest risk of earthquakes (and thus try to mitigate the risk with protective measures).
It’s important to note that, while cluster analysis may reveal structures within your data, it won’t explain why those structures exist. With that in mind, cluster analysis is a useful starting point for understanding your data and informing further analysis. Clustering algorithms are also used in machine learning—you can learn more about clustering in machine learning in our guide .
Cluster analysis in action: Using cluster analysis for customer segmentation—a telecoms case study example
f. Time series analysis
Time series analysis is a statistical technique used to identify trends and cycles over time. Time series data is a sequence of data points which measure the same variable at different points in time (for example, weekly sales figures or monthly email sign-ups). By looking at time-related trends, analysts are able to forecast how the variable of interest may fluctuate in the future.
When conducting time series analysis, the main patterns you’ll be looking out for in your data are:
- Trends: Stable, linear increases or decreases over an extended time period.
- Seasonality: Predictable fluctuations in the data due to seasonal factors over a short period of time. For example, you might see a peak in swimwear sales in summer around the same time every year.
- Cyclic patterns: Unpredictable cycles where the data fluctuates. Cyclical trends are not due to seasonality, but rather, may occur as a result of economic or industry-related conditions.
As you can imagine, the ability to make informed predictions about the future has immense value for business. Time series analysis and forecasting is used across a variety of industries, most commonly for stock market analysis, economic forecasting, and sales forecasting. There are different types of time series models depending on the data you’re using and the outcomes you want to predict. These models are typically classified into three broad types: the autoregressive (AR) models, the integrated (I) models, and the moving average (MA) models. For an in-depth look at time series analysis, refer to our guide .
Time series analysis in action: Developing a time series model to predict jute yarn demand in Bangladesh
g. Sentiment analysis
When you think of data, your mind probably automatically goes to numbers and spreadsheets.
Many companies overlook the value of qualitative data, but in reality, there are untold insights to be gained from what people (especially customers) write and say about you. So how do you go about analyzing textual data?
One highly useful qualitative technique is sentiment analysis , a technique which belongs to the broader category of text analysis —the (usually automated) process of sorting and understanding textual data.
With sentiment analysis, the goal is to interpret and classify the emotions conveyed within textual data. From a business perspective, this allows you to ascertain how your customers feel about various aspects of your brand, product, or service.
There are several different types of sentiment analysis models, each with a slightly different focus. The three main types include:
Fine-grained sentiment analysis
If you want to focus on opinion polarity (i.e. positive, neutral, or negative) in depth, fine-grained sentiment analysis will allow you to do so.
For example, if you wanted to interpret star ratings given by customers, you might use fine-grained sentiment analysis to categorize the various ratings along a scale ranging from very positive to very negative.
Emotion detection
This model often uses complex machine learning algorithms to pick out various emotions from your textual data.
You might use an emotion detection model to identify words associated with happiness, anger, frustration, and excitement, giving you insight into how your customers feel when writing about you or your product on, say, a product review site.
Aspect-based sentiment analysis
This type of analysis allows you to identify what specific aspects the emotions or opinions relate to, such as a certain product feature or a new ad campaign.
If a customer writes that they “find the new Instagram advert so annoying”, your model should detect not only a negative sentiment, but also the object towards which it’s directed.
In a nutshell, sentiment analysis uses various Natural Language Processing (NLP) algorithms and systems which are trained to associate certain inputs (for example, certain words) with certain outputs.
For example, the input “annoying” would be recognized and tagged as “negative”. Sentiment analysis is crucial to understanding how your customers feel about you and your products, for identifying areas for improvement, and even for averting PR disasters in real-time!
Sentiment analysis in action: 5 Real-world sentiment analysis case studies
4. The data analysis process
In order to gain meaningful insights from data, data analysts will perform a rigorous step-by-step process. We go over this in detail in our step by step guide to the data analysis process —but, to briefly summarize, the data analysis process generally consists of the following phases:
Defining the question
The first step for any data analyst will be to define the objective of the analysis, sometimes called a ‘problem statement’. Essentially, you’re asking a question with regards to a business problem you’re trying to solve. Once you’ve defined this, you’ll then need to determine which data sources will help you answer this question.
Collecting the data
Now that you’ve defined your objective, the next step will be to set up a strategy for collecting and aggregating the appropriate data. Will you be using quantitative (numeric) or qualitative (descriptive) data? Do these data fit into first-party, second-party, or third-party data?
Learn more: Quantitative vs. Qualitative Data: What’s the Difference?
Cleaning the data
Unfortunately, your collected data isn’t automatically ready for analysis—you’ll have to clean it first. As a data analyst, this phase of the process will take up the most time. During the data cleaning process, you will likely be:
- Removing major errors, duplicates, and outliers
- Removing unwanted data points
- Structuring the data—that is, fixing typos, layout issues, etc.
- Filling in major gaps in data
Analyzing the data
Now that we’ve finished cleaning the data, it’s time to analyze it! Many analysis methods have already been described in this article, and it’s up to you to decide which one will best suit the assigned objective. It may fall under one of the following categories:
- Descriptive analysis , which identifies what has already happened
- Diagnostic analysis , which focuses on understanding why something has happened
- Predictive analysis , which identifies future trends based on historical data
- Prescriptive analysis , which allows you to make recommendations for the future
Visualizing and sharing your findings
We’re almost at the end of the road! Analyses have been made, insights have been gleaned—all that remains to be done is to share this information with others. This is usually done with a data visualization tool, such as Google Charts, or Tableau.
Learn more: 13 of the Most Common Types of Data Visualization
To sum up the process, Will’s explained it all excellently in the following video:
5. The best tools for data analysis
As you can imagine, every phase of the data analysis process requires the data analyst to have a variety of tools under their belt that assist in gaining valuable insights from data. We cover these tools in greater detail in this article , but, in summary, here’s our best-of-the-best list, with links to each product:
The top 9 tools for data analysts
- Microsoft Excel
- Jupyter Notebook
- Apache Spark
- Microsoft Power BI
6. Key takeaways and further reading
As you can see, there are many different data analysis techniques at your disposal. In order to turn your raw data into actionable insights, it’s important to consider what kind of data you have (is it qualitative or quantitative?) as well as the kinds of insights that will be useful within the given context. In this post, we’ve introduced seven of the most useful data analysis techniques—but there are many more out there to be discovered!
So what now? If you haven’t already, we recommend reading the case studies for each analysis technique discussed in this post (you’ll find a link at the end of each section). For a more hands-on introduction to the kinds of methods and techniques that data analysts use, try out this free introductory data analytics short course. In the meantime, you might also want to read the following:
- The Best Online Data Analytics Courses for 2024
- What Is Time Series Data and How Is It Analyzed?
- What is Spatial Analysis?
- MS in the Learning Sciences
- Tuition & Financial Aid

Qualitative vs. quantitative data analysis: How do they differ?
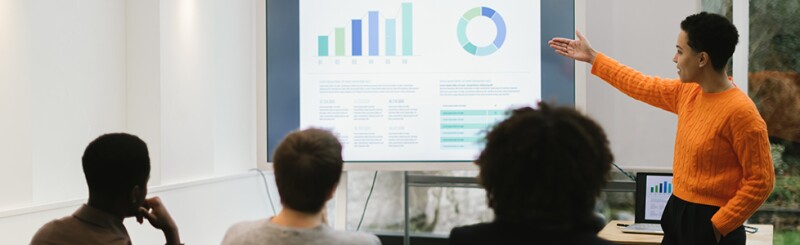
Learning analytics have become the cornerstone for personalizing student experiences and enhancing learning outcomes. In this data-informed approach to education there are two distinct methodologies: qualitative and quantitative analytics. These methods, which are typical to data analytics in general, are crucial to the interpretation of learning behaviors and outcomes. This blog will explore the nuances that distinguish qualitative and quantitative research, while uncovering their shared roles in learning analytics, program design and instruction.
What is qualitative data?
Qualitative data is descriptive and includes information that is non numerical. Qualitative research is used to gather in-depth insights that can't be easily measured on a scale like opinions, anecdotes and emotions. In learning analytics qualitative data could include in depth interviews, text responses to a prompt, or a video of a class period. 1
What is quantitative data?
Quantitative data is information that has a numerical value. Quantitative research is conducted to gather measurable data used in statistical analysis. Researchers can use quantitative studies to identify patterns and trends. In learning analytics quantitative data could include test scores, student demographics, or amount of time spent in a lesson. 2
Key difference between qualitative and quantitative data
It's important to understand the differences between qualitative and quantitative data to both determine the appropriate research methods for studies and to gain insights that you can be confident in sharing.
Data Types and Nature
Examples of qualitative data types in learning analytics:
- Observational data of human behavior from classroom settings such as student engagement, teacher-student interactions, and classroom dynamics
- Textual data from open-ended survey responses, reflective journals, and written assignments
- Feedback and discussions from focus groups or interviews
- Content analysis from various media
Examples of quantitative data types:
- Standardized test, assessment, and quiz scores
- Grades and grade point averages
- Attendance records
- Time spent on learning tasks
- Data gathered from learning management systems (LMS), including login frequency, online participation, and completion rates of assignments
Methods of Collection
Qualitative and quantitative research methods for data collection can occasionally seem similar so it's important to note the differences to make sure you're creating a consistent data set and will be able to reliably draw conclusions from your data.
Qualitative research methods
Because of the nature of qualitative data (complex, detailed information), the research methods used to collect it are more involved. Qualitative researchers might do the following to collect data:
- Conduct interviews to learn about subjective experiences
- Host focus groups to gather feedback and personal accounts
- Observe in-person or use audio or video recordings to record nuances of human behavior in a natural setting
- Distribute surveys with open-ended questions
Quantitative research methods
Quantitative data collection methods are more diverse and more likely to be automated because of the objective nature of the data. A quantitative researcher could employ methods such as:
- Surveys with close-ended questions that gather numerical data like birthdates or preferences
- Observational research and record measurable information like the number of students in a classroom
- Automated numerical data collection like information collected on the backend of a computer system like button clicks and page views
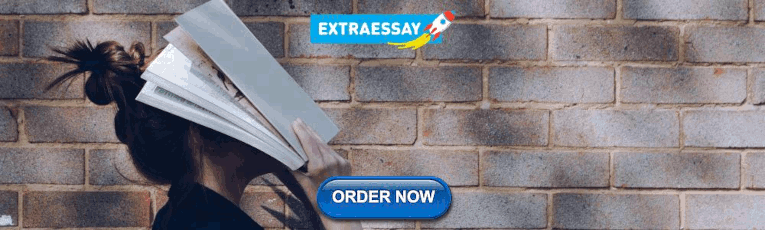
Analysis techniques
Qualitative and quantitative data can both be very informative. However, research studies require critical thinking for productive analysis.
Qualitative data analysis methods
Analyzing qualitative data takes a number of steps. When you first get all your data in one place you can do a review and take notes of trends you think you're seeing or your initial reactions. Next, you'll want to organize all the qualitative data you've collected by assigning it categories. Your central research question will guide your data categorization whether it's by date, location, type of collection method (interview vs focus group, etc), the specific question asked or something else. Next, you'll code your data. Whereas categorizing data is focused on the method of collection, coding is the process of identifying and labeling themes within the data collected to get closer to answering your research questions. Finally comes data interpretation. To interpret the data you'll take a look at the information gathered including your coding labels and see what results are occurring frequently or what other conclusions you can make. 3
Quantitative analysis techniques
The process to analyze quantitative data can be time-consuming due to the large volume of data possible to collect. When approaching a quantitative data set, start by focusing in on the purpose of your evaluation. Without making a conclusion, determine how you will use the information gained from analysis; for example: The answers of this survey about study habits will help determine what type of exam review session will be most useful to a class. 4
Next, you need to decide who is analyzing the data and set parameters for analysis. For example, if two different researchers are evaluating survey responses that rank preferences on a scale from 1 to 5, they need to be operating with the same understanding of the rankings. You wouldn't want one researcher to classify the value of 3 to be a positive preference while the other considers it a negative preference. It's also ideal to have some type of data management system to store and organize your data, such as a spreadsheet or database. Within the database, or via an export to data analysis software, the collected data needs to be cleaned of things like responses left blank, duplicate answers from respondents, and questions that are no longer considered relevant. Finally, you can use statistical software to analyze data (or complete a manual analysis) to find patterns and summarize your findings. 4
Qualitative and quantitative research tools
From the nuanced, thematic exploration enabled by tools like NVivo and ATLAS.ti, to the statistical precision of SPSS and R for quantitative analysis, each suite of data analysis tools offers tailored functionalities that cater to the distinct natures of different data types.
Qualitative research software:
NVivo: NVivo is qualitative data analysis software that can do everything from transcribe recordings to create word clouds and evaluate uploads for different sentiments and themes. NVivo is just one tool from the company Lumivero, which offers whole suites of data processing software. 5
ATLAS.ti: Similar to NVivo, ATLAS.ti allows researchers to upload and import data from a variety of sources to be tagged and refined using machine learning and presented with visualizations and ready for insert into reports. 6
SPSS: SPSS is a statistical analysis tool for quantitative research, appreciated for its user-friendly interface and comprehensive statistical tests, which makes it ideal for educators and researchers. With SPSS researchers can manage and analyze large quantitative data sets, use advanced statistical procedures and modeling techniques, predict customer behaviors, forecast market trends and more. 7
R: R is a versatile and dynamic open-source tool for quantitative analysis. With a vast repository of packages tailored to specific statistical methods, researchers can perform anything from basic descriptive statistics to complex predictive modeling. R is especially useful for its ability to handle large datasets, making it ideal for educational institutions that generate substantial amounts of data. The programming language offers flexibility in customizing analysis and creating publication-quality visualizations to effectively communicate results. 8
Applications in Educational Research
Both quantitative and qualitative data can be employed in learning analytics to drive informed decision-making and pedagogical enhancements. In the classroom, quantitative data like standardized test scores and online course analytics create a foundation for assessing and benchmarking student performance and engagement. Qualitative insights gathered from surveys, focus group discussions, and reflective student journals offer a more nuanced understanding of learners' experiences and contextual factors influencing their education. Additionally feedback and practical engagement metrics blend these data types, providing a holistic view that informs curriculum development, instructional strategies, and personalized learning pathways. Through these varied data sets and uses, educators can piece together a more complete narrative of student success and the impacts of educational interventions.
Master Data Analysis with an M.S. in Learning Sciences From SMU
Whether it is the detailed narratives unearthed through qualitative data or the informative patterns derived from quantitative analysis, both qualitative and quantitative data can provide crucial information for educators and researchers to better understand and improve learning. Dive deeper into the art and science of learning analytics with SMU's online Master of Science in the Learning Sciences program . At SMU, innovation and inquiry converge to empower the next generation of educators and researchers. Choose the Learning Analytics Specialization to learn how to harness the power of data science to illuminate learning trends, devise impactful strategies, and drive educational innovation. You could also find out how advanced technologies like augmented reality (AR), virtual reality (VR), and artificial intelligence (AI) can revolutionize education, and develop the insight to apply embodied cognition principles to enhance learning experiences in the Learning and Technology Design Specialization , or choose your own electives to build a specialization unique to your interests and career goals.
For more information on our curriculum and to become part of a community where data drives discovery, visit SMU's MSLS program website or schedule a call with our admissions outreach advisors for any queries or further discussion. Take the first step towards transforming education with data today.
- Retrieved on August 8, 2024, from nnlm.gov/guides/data-glossary/qualitative-data
- Retrieved on August 8, 2024, from nnlm.gov/guides/data-glossary/quantitative-data
- Retrieved on August 8, 2024, from cdc.gov/healthyyouth/evaluation/pdf/brief19.pdf
- Retrieved on August 8, 2024, from cdc.gov/healthyyouth/evaluation/pdf/brief20.pdf
- Retrieved on August 8, 2024, from lumivero.com/solutions/
- Retrieved on August 8, 2024, from atlasti.com/
- Retrieved on August 8, 2024, from ibm.com/products/spss-statistics
- Retrieved on August 8, 2024, from cran.r-project.org/doc/manuals/r-release/R-intro.html#Introduction-and-preliminaries
Return to SMU Online Learning Sciences Blog
Southern Methodist University has engaged Everspring , a leading provider of education and technology services, to support select aspects of program delivery.
This will only take a moment

An official website of the United States government
The .gov means it’s official. Federal government websites often end in .gov or .mil. Before sharing sensitive information, make sure you’re on a federal government site.
The site is secure. The https:// ensures that you are connecting to the official website and that any information you provide is encrypted and transmitted securely.
- Publications
- Account settings
Preview improvements coming to the PMC website in October 2024. Learn More or Try it out now .
- Advanced Search
- Journal List
- Indian J Anaesth
- v.60(9); 2016 Sep
Basic statistical tools in research and data analysis
Zulfiqar ali.
Department of Anaesthesiology, Division of Neuroanaesthesiology, Sheri Kashmir Institute of Medical Sciences, Soura, Srinagar, Jammu and Kashmir, India
S Bala Bhaskar
1 Department of Anaesthesiology and Critical Care, Vijayanagar Institute of Medical Sciences, Bellary, Karnataka, India
Statistical methods involved in carrying out a study include planning, designing, collecting data, analysing, drawing meaningful interpretation and reporting of the research findings. The statistical analysis gives meaning to the meaningless numbers, thereby breathing life into a lifeless data. The results and inferences are precise only if proper statistical tests are used. This article will try to acquaint the reader with the basic research tools that are utilised while conducting various studies. The article covers a brief outline of the variables, an understanding of quantitative and qualitative variables and the measures of central tendency. An idea of the sample size estimation, power analysis and the statistical errors is given. Finally, there is a summary of parametric and non-parametric tests used for data analysis.
INTRODUCTION
Statistics is a branch of science that deals with the collection, organisation, analysis of data and drawing of inferences from the samples to the whole population.[ 1 ] This requires a proper design of the study, an appropriate selection of the study sample and choice of a suitable statistical test. An adequate knowledge of statistics is necessary for proper designing of an epidemiological study or a clinical trial. Improper statistical methods may result in erroneous conclusions which may lead to unethical practice.[ 2 ]
Variable is a characteristic that varies from one individual member of population to another individual.[ 3 ] Variables such as height and weight are measured by some type of scale, convey quantitative information and are called as quantitative variables. Sex and eye colour give qualitative information and are called as qualitative variables[ 3 ] [ Figure 1 ].

Classification of variables
Quantitative variables
Quantitative or numerical data are subdivided into discrete and continuous measurements. Discrete numerical data are recorded as a whole number such as 0, 1, 2, 3,… (integer), whereas continuous data can assume any value. Observations that can be counted constitute the discrete data and observations that can be measured constitute the continuous data. Examples of discrete data are number of episodes of respiratory arrests or the number of re-intubations in an intensive care unit. Similarly, examples of continuous data are the serial serum glucose levels, partial pressure of oxygen in arterial blood and the oesophageal temperature.
A hierarchical scale of increasing precision can be used for observing and recording the data which is based on categorical, ordinal, interval and ratio scales [ Figure 1 ].
Categorical or nominal variables are unordered. The data are merely classified into categories and cannot be arranged in any particular order. If only two categories exist (as in gender male and female), it is called as a dichotomous (or binary) data. The various causes of re-intubation in an intensive care unit due to upper airway obstruction, impaired clearance of secretions, hypoxemia, hypercapnia, pulmonary oedema and neurological impairment are examples of categorical variables.
Ordinal variables have a clear ordering between the variables. However, the ordered data may not have equal intervals. Examples are the American Society of Anesthesiologists status or Richmond agitation-sedation scale.
Interval variables are similar to an ordinal variable, except that the intervals between the values of the interval variable are equally spaced. A good example of an interval scale is the Fahrenheit degree scale used to measure temperature. With the Fahrenheit scale, the difference between 70° and 75° is equal to the difference between 80° and 85°: The units of measurement are equal throughout the full range of the scale.
Ratio scales are similar to interval scales, in that equal differences between scale values have equal quantitative meaning. However, ratio scales also have a true zero point, which gives them an additional property. For example, the system of centimetres is an example of a ratio scale. There is a true zero point and the value of 0 cm means a complete absence of length. The thyromental distance of 6 cm in an adult may be twice that of a child in whom it may be 3 cm.
STATISTICS: DESCRIPTIVE AND INFERENTIAL STATISTICS
Descriptive statistics[ 4 ] try to describe the relationship between variables in a sample or population. Descriptive statistics provide a summary of data in the form of mean, median and mode. Inferential statistics[ 4 ] use a random sample of data taken from a population to describe and make inferences about the whole population. It is valuable when it is not possible to examine each member of an entire population. The examples if descriptive and inferential statistics are illustrated in Table 1 .
Example of descriptive and inferential statistics

Descriptive statistics
The extent to which the observations cluster around a central location is described by the central tendency and the spread towards the extremes is described by the degree of dispersion.
Measures of central tendency
The measures of central tendency are mean, median and mode.[ 6 ] Mean (or the arithmetic average) is the sum of all the scores divided by the number of scores. Mean may be influenced profoundly by the extreme variables. For example, the average stay of organophosphorus poisoning patients in ICU may be influenced by a single patient who stays in ICU for around 5 months because of septicaemia. The extreme values are called outliers. The formula for the mean is

where x = each observation and n = number of observations. Median[ 6 ] is defined as the middle of a distribution in a ranked data (with half of the variables in the sample above and half below the median value) while mode is the most frequently occurring variable in a distribution. Range defines the spread, or variability, of a sample.[ 7 ] It is described by the minimum and maximum values of the variables. If we rank the data and after ranking, group the observations into percentiles, we can get better information of the pattern of spread of the variables. In percentiles, we rank the observations into 100 equal parts. We can then describe 25%, 50%, 75% or any other percentile amount. The median is the 50 th percentile. The interquartile range will be the observations in the middle 50% of the observations about the median (25 th -75 th percentile). Variance[ 7 ] is a measure of how spread out is the distribution. It gives an indication of how close an individual observation clusters about the mean value. The variance of a population is defined by the following formula:

where σ 2 is the population variance, X is the population mean, X i is the i th element from the population and N is the number of elements in the population. The variance of a sample is defined by slightly different formula:

where s 2 is the sample variance, x is the sample mean, x i is the i th element from the sample and n is the number of elements in the sample. The formula for the variance of a population has the value ‘ n ’ as the denominator. The expression ‘ n −1’ is known as the degrees of freedom and is one less than the number of parameters. Each observation is free to vary, except the last one which must be a defined value. The variance is measured in squared units. To make the interpretation of the data simple and to retain the basic unit of observation, the square root of variance is used. The square root of the variance is the standard deviation (SD).[ 8 ] The SD of a population is defined by the following formula:

where σ is the population SD, X is the population mean, X i is the i th element from the population and N is the number of elements in the population. The SD of a sample is defined by slightly different formula:

where s is the sample SD, x is the sample mean, x i is the i th element from the sample and n is the number of elements in the sample. An example for calculation of variation and SD is illustrated in Table 2 .
Example of mean, variance, standard deviation

Normal distribution or Gaussian distribution
Most of the biological variables usually cluster around a central value, with symmetrical positive and negative deviations about this point.[ 1 ] The standard normal distribution curve is a symmetrical bell-shaped. In a normal distribution curve, about 68% of the scores are within 1 SD of the mean. Around 95% of the scores are within 2 SDs of the mean and 99% within 3 SDs of the mean [ Figure 2 ].

Normal distribution curve
Skewed distribution
It is a distribution with an asymmetry of the variables about its mean. In a negatively skewed distribution [ Figure 3 ], the mass of the distribution is concentrated on the right of Figure 1 . In a positively skewed distribution [ Figure 3 ], the mass of the distribution is concentrated on the left of the figure leading to a longer right tail.

Curves showing negatively skewed and positively skewed distribution
Inferential statistics
In inferential statistics, data are analysed from a sample to make inferences in the larger collection of the population. The purpose is to answer or test the hypotheses. A hypothesis (plural hypotheses) is a proposed explanation for a phenomenon. Hypothesis tests are thus procedures for making rational decisions about the reality of observed effects.
Probability is the measure of the likelihood that an event will occur. Probability is quantified as a number between 0 and 1 (where 0 indicates impossibility and 1 indicates certainty).
In inferential statistics, the term ‘null hypothesis’ ( H 0 ‘ H-naught ,’ ‘ H-null ’) denotes that there is no relationship (difference) between the population variables in question.[ 9 ]
Alternative hypothesis ( H 1 and H a ) denotes that a statement between the variables is expected to be true.[ 9 ]
The P value (or the calculated probability) is the probability of the event occurring by chance if the null hypothesis is true. The P value is a numerical between 0 and 1 and is interpreted by researchers in deciding whether to reject or retain the null hypothesis [ Table 3 ].
P values with interpretation

If P value is less than the arbitrarily chosen value (known as α or the significance level), the null hypothesis (H0) is rejected [ Table 4 ]. However, if null hypotheses (H0) is incorrectly rejected, this is known as a Type I error.[ 11 ] Further details regarding alpha error, beta error and sample size calculation and factors influencing them are dealt with in another section of this issue by Das S et al .[ 12 ]
Illustration for null hypothesis

PARAMETRIC AND NON-PARAMETRIC TESTS
Numerical data (quantitative variables) that are normally distributed are analysed with parametric tests.[ 13 ]
Two most basic prerequisites for parametric statistical analysis are:
- The assumption of normality which specifies that the means of the sample group are normally distributed
- The assumption of equal variance which specifies that the variances of the samples and of their corresponding population are equal.
However, if the distribution of the sample is skewed towards one side or the distribution is unknown due to the small sample size, non-parametric[ 14 ] statistical techniques are used. Non-parametric tests are used to analyse ordinal and categorical data.
Parametric tests
The parametric tests assume that the data are on a quantitative (numerical) scale, with a normal distribution of the underlying population. The samples have the same variance (homogeneity of variances). The samples are randomly drawn from the population, and the observations within a group are independent of each other. The commonly used parametric tests are the Student's t -test, analysis of variance (ANOVA) and repeated measures ANOVA.
Student's t -test
Student's t -test is used to test the null hypothesis that there is no difference between the means of the two groups. It is used in three circumstances:

where X = sample mean, u = population mean and SE = standard error of mean

where X 1 − X 2 is the difference between the means of the two groups and SE denotes the standard error of the difference.
- To test if the population means estimated by two dependent samples differ significantly (the paired t -test). A usual setting for paired t -test is when measurements are made on the same subjects before and after a treatment.
The formula for paired t -test is:

where d is the mean difference and SE denotes the standard error of this difference.
The group variances can be compared using the F -test. The F -test is the ratio of variances (var l/var 2). If F differs significantly from 1.0, then it is concluded that the group variances differ significantly.
Analysis of variance
The Student's t -test cannot be used for comparison of three or more groups. The purpose of ANOVA is to test if there is any significant difference between the means of two or more groups.
In ANOVA, we study two variances – (a) between-group variability and (b) within-group variability. The within-group variability (error variance) is the variation that cannot be accounted for in the study design. It is based on random differences present in our samples.
However, the between-group (or effect variance) is the result of our treatment. These two estimates of variances are compared using the F-test.
A simplified formula for the F statistic is:

where MS b is the mean squares between the groups and MS w is the mean squares within groups.
Repeated measures analysis of variance
As with ANOVA, repeated measures ANOVA analyses the equality of means of three or more groups. However, a repeated measure ANOVA is used when all variables of a sample are measured under different conditions or at different points in time.
As the variables are measured from a sample at different points of time, the measurement of the dependent variable is repeated. Using a standard ANOVA in this case is not appropriate because it fails to model the correlation between the repeated measures: The data violate the ANOVA assumption of independence. Hence, in the measurement of repeated dependent variables, repeated measures ANOVA should be used.
Non-parametric tests
When the assumptions of normality are not met, and the sample means are not normally, distributed parametric tests can lead to erroneous results. Non-parametric tests (distribution-free test) are used in such situation as they do not require the normality assumption.[ 15 ] Non-parametric tests may fail to detect a significant difference when compared with a parametric test. That is, they usually have less power.
As is done for the parametric tests, the test statistic is compared with known values for the sampling distribution of that statistic and the null hypothesis is accepted or rejected. The types of non-parametric analysis techniques and the corresponding parametric analysis techniques are delineated in Table 5 .
Analogue of parametric and non-parametric tests

Median test for one sample: The sign test and Wilcoxon's signed rank test
The sign test and Wilcoxon's signed rank test are used for median tests of one sample. These tests examine whether one instance of sample data is greater or smaller than the median reference value.
This test examines the hypothesis about the median θ0 of a population. It tests the null hypothesis H0 = θ0. When the observed value (Xi) is greater than the reference value (θ0), it is marked as+. If the observed value is smaller than the reference value, it is marked as − sign. If the observed value is equal to the reference value (θ0), it is eliminated from the sample.
If the null hypothesis is true, there will be an equal number of + signs and − signs.
The sign test ignores the actual values of the data and only uses + or − signs. Therefore, it is useful when it is difficult to measure the values.
Wilcoxon's signed rank test
There is a major limitation of sign test as we lose the quantitative information of the given data and merely use the + or – signs. Wilcoxon's signed rank test not only examines the observed values in comparison with θ0 but also takes into consideration the relative sizes, adding more statistical power to the test. As in the sign test, if there is an observed value that is equal to the reference value θ0, this observed value is eliminated from the sample.
Wilcoxon's rank sum test ranks all data points in order, calculates the rank sum of each sample and compares the difference in the rank sums.
Mann-Whitney test
It is used to test the null hypothesis that two samples have the same median or, alternatively, whether observations in one sample tend to be larger than observations in the other.
Mann–Whitney test compares all data (xi) belonging to the X group and all data (yi) belonging to the Y group and calculates the probability of xi being greater than yi: P (xi > yi). The null hypothesis states that P (xi > yi) = P (xi < yi) =1/2 while the alternative hypothesis states that P (xi > yi) ≠1/2.
Kolmogorov-Smirnov test
The two-sample Kolmogorov-Smirnov (KS) test was designed as a generic method to test whether two random samples are drawn from the same distribution. The null hypothesis of the KS test is that both distributions are identical. The statistic of the KS test is a distance between the two empirical distributions, computed as the maximum absolute difference between their cumulative curves.
Kruskal-Wallis test
The Kruskal–Wallis test is a non-parametric test to analyse the variance.[ 14 ] It analyses if there is any difference in the median values of three or more independent samples. The data values are ranked in an increasing order, and the rank sums calculated followed by calculation of the test statistic.
Jonckheere test
In contrast to Kruskal–Wallis test, in Jonckheere test, there is an a priori ordering that gives it a more statistical power than the Kruskal–Wallis test.[ 14 ]
Friedman test
The Friedman test is a non-parametric test for testing the difference between several related samples. The Friedman test is an alternative for repeated measures ANOVAs which is used when the same parameter has been measured under different conditions on the same subjects.[ 13 ]
Tests to analyse the categorical data
Chi-square test, Fischer's exact test and McNemar's test are used to analyse the categorical or nominal variables. The Chi-square test compares the frequencies and tests whether the observed data differ significantly from that of the expected data if there were no differences between groups (i.e., the null hypothesis). It is calculated by the sum of the squared difference between observed ( O ) and the expected ( E ) data (or the deviation, d ) divided by the expected data by the following formula:

A Yates correction factor is used when the sample size is small. Fischer's exact test is used to determine if there are non-random associations between two categorical variables. It does not assume random sampling, and instead of referring a calculated statistic to a sampling distribution, it calculates an exact probability. McNemar's test is used for paired nominal data. It is applied to 2 × 2 table with paired-dependent samples. It is used to determine whether the row and column frequencies are equal (that is, whether there is ‘marginal homogeneity’). The null hypothesis is that the paired proportions are equal. The Mantel-Haenszel Chi-square test is a multivariate test as it analyses multiple grouping variables. It stratifies according to the nominated confounding variables and identifies any that affects the primary outcome variable. If the outcome variable is dichotomous, then logistic regression is used.
SOFTWARES AVAILABLE FOR STATISTICS, SAMPLE SIZE CALCULATION AND POWER ANALYSIS
Numerous statistical software systems are available currently. The commonly used software systems are Statistical Package for the Social Sciences (SPSS – manufactured by IBM corporation), Statistical Analysis System ((SAS – developed by SAS Institute North Carolina, United States of America), R (designed by Ross Ihaka and Robert Gentleman from R core team), Minitab (developed by Minitab Inc), Stata (developed by StataCorp) and the MS Excel (developed by Microsoft).
There are a number of web resources which are related to statistical power analyses. A few are:
- StatPages.net – provides links to a number of online power calculators
- G-Power – provides a downloadable power analysis program that runs under DOS
- Power analysis for ANOVA designs an interactive site that calculates power or sample size needed to attain a given power for one effect in a factorial ANOVA design
- SPSS makes a program called SamplePower. It gives an output of a complete report on the computer screen which can be cut and paste into another document.
It is important that a researcher knows the concepts of the basic statistical methods used for conduct of a research study. This will help to conduct an appropriately well-designed study leading to valid and reliable results. Inappropriate use of statistical techniques may lead to faulty conclusions, inducing errors and undermining the significance of the article. Bad statistics may lead to bad research, and bad research may lead to unethical practice. Hence, an adequate knowledge of statistics and the appropriate use of statistical tests are important. An appropriate knowledge about the basic statistical methods will go a long way in improving the research designs and producing quality medical research which can be utilised for formulating the evidence-based guidelines.
Financial support and sponsorship
Conflicts of interest.
There are no conflicts of interest.
Integrations
What's new?
In-Product Prompts
Participant Management
Interview Studies
Prototype Testing
Card Sorting
Tree Testing
Live Website Testing
Automated Reports
Templates Gallery
Choose from our library of pre-built mazes to copy, customize, and share with your own users
Browse all templates
Financial Services
Tech & Software
Product Designers
Product Managers
User Researchers
By use case
Concept & Idea Validation
Wireframe & Usability Test
Content & Copy Testing
Feedback & Satisfaction
Content Hub
Educational resources for product, research and design teams
Explore all resources
Question Bank
Maze Research Success Hub
Guides & Reports
Help Center
Future of User Research Report
The Optimal Path Podcast
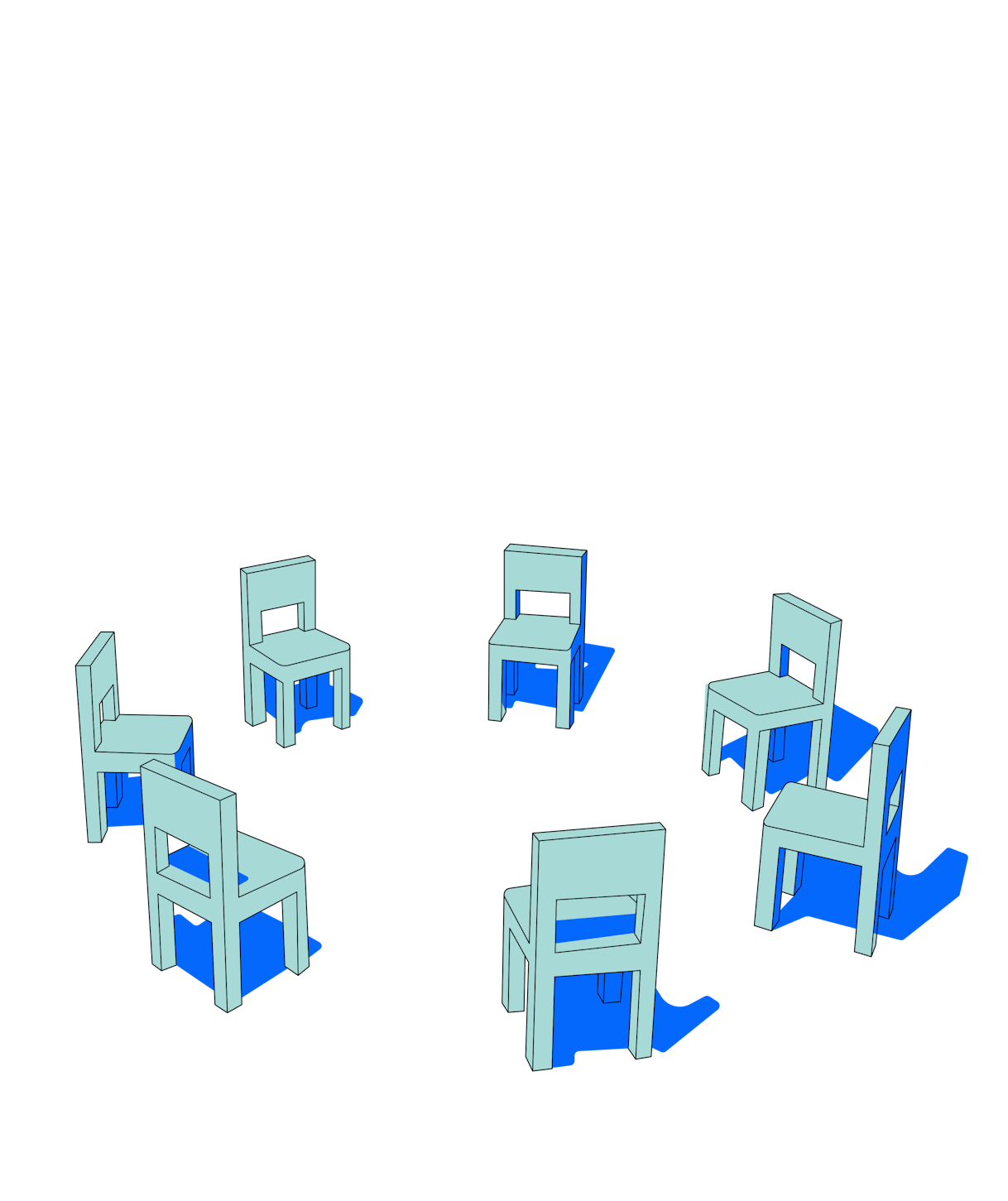
User Research
Aug 19, 2024 • 17 minutes read
Qualitative research examples: How to unlock, rich, descriptive insights
Qualitative research uncovers in-depth user insights, but what does it look like? Here are seven methods and examples to help you get the data you need.
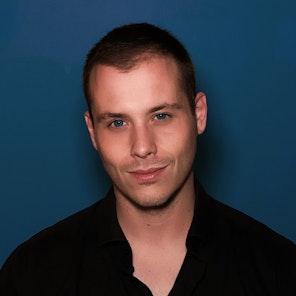
Armin Tanovic
Behind every what, there’s a why . Qualitative research is how you uncover that why. It enables you to connect with users and understand their thoughts, feelings, wants, needs, and pain points.
There’s many methods for conducting qualitative research, and many objectives it can help you pursue—you might want to explore ways to improve NPS scores, combat reduced customer retention, or understand (and recreate) the success behind a well-received product. The common thread? All these metrics impact your business, and qualitative research can help investigate and improve that impact.
In this article, we’ll take you through seven methods and examples of qualitative research, including when and how to use them.
Qualitative UX research made easy
Conduct qualitative research with Maze, analyze data instantly, and get rich, descriptive insights that drive decision-making.
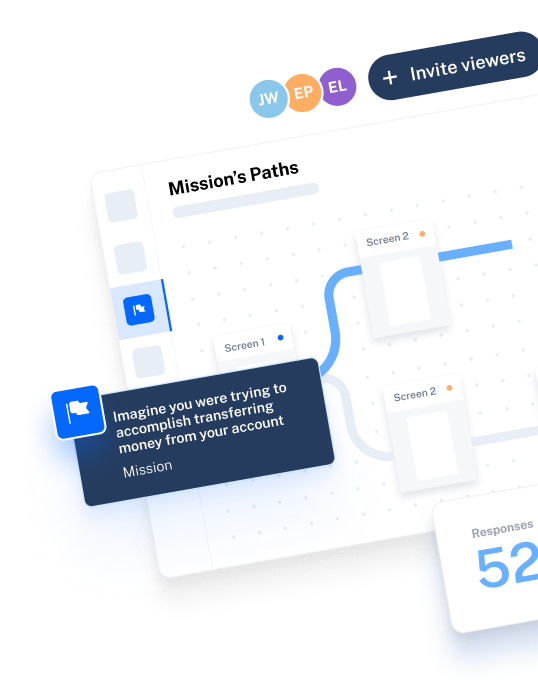
7 Qualitative research methods: An overview
There are various qualitative UX research methods that can help you get in-depth, descriptive insights. Some are suited to specific phases of the design and development process, while others are more task-oriented.
Here’s our overview of the most common qualitative research methods. Keep reading for their use cases, and detailed examples of how to conduct them.
Method | | | |
---|
User interviews | | | |
---|
Focus groups | | | |
---|
Ethnographic research | | | |
---|
Qualitative observation | | | |
---|
Case study research | | | |
---|
Secondary research | | | |
---|
Open-ended surveys | | | to extract descriptive insights. |
---|
1. User interviews
A user interview is a one-on-one conversation between a UX researcher, designer or Product Manager and a target user to understand their thoughts, perspectives, and feelings on a product or service. User interviews are a great way to get non-numerical data on individual experiences with your product, to gain a deeper understanding of user perspectives.
Interviews can be structured, semi-structured, or unstructured . Structured interviews follow a strict interview script and can help you get answers to your planned questions, while semi and unstructured interviews are less rigid in their approach and typically lead to more spontaneous, user-centered insights.
When to use user interviews
Interviews are ideal when you want to gain an in-depth understanding of your users’ perspectives on your product or service, and why they feel a certain way.
Interviews can be used at any stage in the product design and development process, being particularly helpful during:
- The discovery phase: To better understand user needs, problems, and the context in which they use your product—revealing the best potential solutions
- The design phase: To get contextual feedback on mockups, wireframes, and prototypes, helping you pinpoint issues and the reasons behind them
- Post-launch: To assess if your product continues to meet users’ shifting expectations and understand why or why not
How to conduct user interviews: The basics
- Draft questions based on your research objectives
- Recruit relevant research participants and schedule interviews
- Conduct the interview and transcribe responses
- Analyze the interview responses to extract insights
- Use your findings to inform design, product, and business decisions
💡 A specialized user interview tool makes interviewing easier. With Maze Interview Studies , you can recruit, host, and analyze interviews all on one platform.
User interviews: A qualitative research example
Let’s say you’ve designed a recruitment platform, called Tech2Talent , that connects employers with tech talent. Before starting the design process, you want to clearly understand the pain points employers experience with existing recruitment tools'.
You draft a list of ten questions for a semi-structured interview for 15 different one-on-one interviews. As it’s semi-structured, you don’t expect to ask all the questions—the script serves as more of a guide.
One key question in your script is: “Have tech recruitment platforms helped you find the talent you need in the past?”
Most respondents answer with a resounding and passionate ‘no’ with one of them expanding:
“For our company, it’s been pretty hit or miss honestly. They let just about anyone make a profile and call themselves tech talent. It’s so hard sifting through serious candidates. I can’t see any of their achievements until I invest time setting up an interview.”
You begin to notice a pattern in your responses: recruitment tools often lack easily accessible details on talent profiles.
You’ve gained contextual feedback on why other recruitment platforms fail to solve user needs.
2. Focus groups
A focus group is a research method that involves gathering a small group of people—around five to ten users—to discuss a specific topic, such as their’ experience with your new product feature. Unlike user interviews, focus groups aim to capture the collective opinion of a wider market segment and encourage discussion among the group.
When to use focus groups
You should use focus groups when you need a deeper understanding of your users’ collective opinions. The dynamic discussion among participants can spark in-depth insights that might not emerge from regular interviews.
Focus groups can be used before, during, and after a product launch. They’re ideal:
- Throughout the problem discovery phase: To understand your user segment’s pain points and expectations, and generate product ideas
- Post-launch: To evaluate and understand the collective opinion of your product’s user experience
- When conducting market research: To grasp usage patterns, consumer perceptions, and market opportunities for your product
How to conduct focus group studies: The basics
- Draft prompts to spark conversation, or a series of questions based on your UX research objectives
- Find a group of five to ten users who are representative of your target audience (or a specific user segment) and schedule your focus group session
- Conduct the focus group by talking and listening to users, then transcribe responses
- Analyze focus group responses and extract insights
- Use your findings to inform design decisions
The number of participants can make it difficult to take notes or do manual transcriptions. We recommend using a transcription or a specialized UX research tool , such as Maze, that can automatically create ready-to-share reports and highlight key user insights.
Focus groups: A qualitative research example
You’re a UX researcher at FitMe , a fitness app that creates customized daily workouts for gym-goers. Unlike many other apps, FitMe takes into account the previous day’s workout and aims to create one that allows users to effectively rest different muscles.
However, FitMe has an issue. Users are generating workouts but not completing them. They’re accessing the app, taking the necessary steps to get a workout for the day, but quitting at the last hurdle.
Time to talk to users.
You organize a focus group to get to the root of the drop-off issue. You invite five existing users, all of whom have dropped off at the exact point you’re investigating, and ask them questions to uncover why.
A dialog develops:
Participant 1: “Sometimes I’ll get a workout that I just don’t want to do. Sure, it’s a good workout—but I just don’t want to physically do it. I just do my own thing when that happens.”
Participant 2: “Same here, some of them are so boring. I go to the gym because I love it. It’s an escape.”
Participant 3: “Right?! I get that the app generates the best one for me on that specific day, but I wish I could get a couple of options.”
Participant 4: “I’m the same, there are some exercises I just refuse to do. I’m not coming to the gym to do things I dislike.”
Conducting the focus groups and reviewing the transcripts, you realize that users want options. A workout that works for one gym-goer doesn’t necessarily work for the next.
A possible solution? Adding the option to generate a new workout (that still considers previous workouts)and the ability to blacklist certain exercises, like burpees.
3. Ethnographic research
Ethnographic research is a research method that involves observing and interacting with users in a real-life environment. By studying users in their natural habitat, you can understand how your product fits into their daily lives.
Ethnographic research can be active or passive. Active ethnographic research entails engaging with users in their natural environment and then following up with methods like interviews. Passive ethnographic research involves letting the user interact with the product while you note your observations.
When to use ethnographic research
Ethnographic research is best suited when you want rich insights into the context and environment in which users interact with your product. Keep in mind that you can conduct ethnographic research throughout the entire product design and development process —from problem discovery to post-launch. However, it’s mostly done early in the process:
- Early concept development: To gain an understanding of your user's day-to-day environment. Observe how they complete tasks and the pain points they encounter. The unique demands of their everyday lives will inform how to design your product.
- Initial design phase: Even if you have a firm grasp of the user’s environment, you still need to put your solution to the test. Conducting ethnographic research with your users interacting with your prototype puts theory into practice.
How to conduct ethnographic research:
- Recruit users who are reflective of your audience
- Meet with them in their natural environment, and tell them to behave as they usually would
- Take down field notes as they interact with your product
- Engage with your users, ask questions, or host an in-depth interview if you’re doing an active ethnographic study
- Collect all your data and analyze it for insights
While ethnographic studies provide a comprehensive view of what potential users actually do, they are resource-intensive and logistically difficult. A common alternative is diary studies. Like ethnographic research, diary studies examine how users interact with your product in their day-to-day, but the data is self-reported by participants.
⚙️ Recruiting participants proving tough and time-consuming? Maze Panel makes it easy, with 400+ filters to find your ideal participants from a pool of 3 million participants.
Ethnographic research: A qualitative research example
You're a UX researcher for a project management platform called ProFlow , and you’re conducting an ethnographic study of the project creation process with key users, including a startup’s COO.
The first thing you notice is that the COO is rushing while navigating the platform. You also take note of the 46 tabs and Zoom calls opened on their monitor. Their attention is divided, and they let out an exasperated sigh as they repeatedly hit “refresh” on your website’s onboarding interface.
You conclude the session with an interview and ask, “How easy or difficult did you find using ProFlow to coordinate a project?”
The COO answers: “Look, the whole reason we turn to project platforms is because we need to be quick on our feet. I’m doing a million things so I need the process to be fast and simple. The actual project management is good, but creating projects and setting up tables is way too complicated.”
You realize that ProFlow ’s project creation process takes way too much time for professionals working in fast-paced, dynamic environments. To solve the issue, propose a quick-create option that enables them to move ahead with the basics instead of requiring in-depth project details.
4. Qualitative observation
Qualitative observation is a similar method to ethnographic research, though not as deep. It involves observing your users in a natural or controlled environment and taking notes as they interact with a product. However, be sure not to interrupt them, as this compromises the integrity of the study and turns it into active ethnographic research.
When to qualitative observation
Qualitative observation is best when you want to record how users interact with your product without anyone interfering. Much like ethnographic research, observation is best done during:
- Early concept development: To help you understand your users' daily lives, how they complete tasks, and the problems they deal with. The observations you collect in these instances will help you define a concept for your product.
- Initial design phase: Observing how users deal with your prototype helps you test if they can easily interact with it in their daily environments
How to conduct qualitative observation:
- Recruit users who regularly use your product
- Meet with users in either their natural environment, such as their office, or within a controlled environment, such as a lab
- Observe them and take down field notes based on what you notice
Qualitative observation: An qualitative research example
You’re conducting UX research for Stackbuilder , an app that connects businesses with tools ideal for their needs and budgets. To determine if your app is easy to use for industry professionals, you decide to conduct an observation study.
Sitting in with the participant, you notice they breeze past the onboarding process, quickly creating an account for their company. Yet, after specifying their company’s budget, they suddenly slow down. They open links to each tool’s individual page, confusingly switching from one tab to another. They let out a sigh as they read through each website.
Conducting your observation study, you realize that users find it difficult to extract information from each tool’s website. Based on your field notes, you suggest including a bullet-point summary of each tool directly on your platform.
5. Case study research
Case studies are a UX research method that provides comprehensive and contextual insights into a real-world case over a long period of time. They typically include a range of other qualitative research methods, like interviews, observations, and ethnographic research. A case study allows you to form an in-depth analysis of how people use your product, helping you uncover nuanced differences between your users.
When to use case studies
Case studies are best when your product involves complex interactions that need to be tracked over a longer period or through in-depth analysis. You can also use case studies when your product is innovative, and there’s little existing data on how users interact with it.
As for specific phases in the product design and development process:
- Initial design phase: Case studies can help you rigorously test for product issues and the reasons behind them, giving you in-depth feedback on everything between user motivations, friction points, and usability issues
- Post-launch phase: Continuing with case studies after launch can give you ongoing feedback on how users interact with the product in their day-to-day lives. These insights ensure you can meet shifting user expectations with product updates and future iterations
How to conduct case studies:
- Outline an objective for your case study such as examining specific user tasks or the overall user journey
- Select qualitative research methods such as interviews, ethnographic studies, or observations
- Collect and analyze your data for comprehensive insights
- Include your findings in a report with proposed solutions
Case study research: A qualitative research example
Your team has recently launched Pulse , a platform that analyzes social media posts to identify rising digital marketing trends. Pulse has been on the market for a year, and you want to better understand how it helps small businesses create successful campaigns.
To conduct your case study, you begin with a series of interviews to understand user expectations, ethnographic research sessions, and focus groups. After sorting responses and observations into common themes you notice a main recurring pattern. Users have trouble interpreting the data from their dashboards, making it difficult to identify which trends to follow.
With your synthesized insights, you create a report with detailed narratives of individual user experiences, common themes and issues, and recommendations for addressing user friction points.
Some of your proposed solutions include creating intuitive graphs and summaries for each trend study. This makes it easier for users to understand trends and implement strategic changes in their campaigns.
6. Secondary research
Secondary research is a research method that involves collecting and analyzing documents, records, and reviews that provide you with contextual data on your topic. You’re not connecting with participants directly, but rather accessing pre-existing available data. For example, you can pull out insights from your UX research repository to reexamine how they apply to your new UX research objective.
Strictly speaking, it can be both qualitative and quantitative—but today we focus on its qualitative application.
When to use secondary research
Record keeping is particularly useful when you need supplemental insights to complement, validate, or compare current research findings. It helps you analyze shifting trends amongst your users across a specific period. Some other scenarios where you need record keeping include:
- Initial discovery or exploration phase: Secondary research can help you quickly gather background information and data to understand the broader context of a market
- Design and development phase: See what solutions are working in other contexts for an idea of how to build yours
Secondary research is especially valuable when your team faces budget constraints, tight deadlines, or limited resources. Through review mining and collecting older findings, you can uncover useful insights that drive decision-making throughout the product design and development process.
How to conduct secondary research:
- Outline your UX research objective
- Identify potential data sources for information on your product, market, or target audience. Some of these sources can include: a. Review websites like Capterra and G2 b. Social media channels c. Customer service logs and disputes d. Website reviews e. Reports and insights from previous research studies f. Industry trends g. Information on competitors
- Analyze your data by identifying recurring patterns and themes for insights
Secondary research: A qualitative research example
SafeSurf is a cybersecurity platform that offers threat detection, security audits, and real-time reports. After conducting multiple rounds of testing, you need a quick and easy way to identify remaining usability issues. Instead of conducting another resource-intensive method, you opt for social listening and data mining for your secondary research.
Browsing through your company’s X, you identify a recurring theme: many users without a background in tech find SafeSurf ’s reports too technical and difficult to read. Users struggle with understanding what to do if their networks are breached.
After checking your other social media channels and review sites, the issue pops up again.
With your gathered insights, your team settles on introducing a simplified version of reports, including clear summaries, takeaways, and step-by-step protocols for ensuring security.
By conducting secondary research, you’ve uncovered a major usability issue—all without spending large amounts of time and resources to connect with your users.
7. Open-ended surveys
Open-ended surveys are a type of unmoderated UX research method that involves asking users to answer a list of qualitative research questions designed to uncover their attitudes, expectations, and needs regarding your service or product. Open-ended surveys allow users to give in-depth, nuanced, and contextual responses.
When to use open-ended surveys
User surveys are an effective qualitative research method for reaching a large number of users. You can use them at any stage of the design and product development process, but they’re particularly useful:
- When you’re conducting generative research : Open-ended surveys allow you to reach a wide range of users, making them especially useful during initial research phases when you need broad insights into user experiences
- When you need to understand customer satisfaction: Open-ended customer satisfaction surveys help you uncover why your users might be dissatisfied with your product, helping you find the root cause of their negative experiences
- In combination with close-ended surveys: Get a combination of numerical, statistical insights and rich descriptive feedback. You ’ll know what a specific percentage of your users think and why they think it.
How to conduct open-ended surveys:
- Design your survey and draft out a list of survey questions
- Distribute your surveys to respondents
- Analyze survey participant responses for key themes and patterns
- Use your findings to inform your design process
Open-ended surveys: A qualitative research example
You're a UX researcher for RouteReader , a comprehensive logistics platform that allows users to conduct shipment tracking and route planning. Recently, you’ve launched a new predictive analytics feature that allows users to quickly identify and prepare for supply chain disruptions.
To better understand if users find the new feature helpful, you create an open-ended, in-app survey.
The questions you ask your users:
- “What has been your experience with our new predictive analytics feature?"
- “Do you find it easy or difficult to rework your routes based on our predictive suggestions?”
- “Does the predictive analytics feature make planning routes easier? Why or why not?”
Most of the responses are positive. Users report using the predictive analytics feature to make last-minute adjustments to their route plans, and some even rely on it regularly. However, a few users find the feature hard to notice, making it difficult to adjust their routes on time.
To ensure users have supply chain insights on time, you integrate the new feature into each interface so users can easily spot important information and adjust their routes accordingly.
💡 Surveys are a lot easier with a quality survey tool. Maze’s Feedback Surveys solution has all you need to ensure your surveys get the insights you need—including AI-powered follow-up and automated reports.
Qualitative research vs. quantitative research: What’s the difference?
Alongside qualitative research approaches, UX teams also use quantitative research methods. Despite the similar names, the two are very different.
Here are some of the key differences between qualitative research and quantitative research .
Research type | | | | |
---|
Qualitative research | | | . | |
---|
Quantitative research | | | | |
---|
Before selecting either qualitative or quantitative methods, first identify what you want to achieve with your UX research project. As a general rule of thumb, think qualitative data collection for in-depth understanding and quantitative studies for measurement and validation.
Conduct qualitative research with Maze
You’ll often find that knowing the what is pointless without understanding the accompanying why . Qualitative research helps you uncover your why.
So, what about how —how do you identify your 'what' and your 'why'?
The answer is with a user research tool like Maze.
Maze is the leading user research platform that lets you organize, conduct, and analyze both qualitative and quantitative research studies—all from one place. Its wide variety of UX research methods and advanced AI capabilities help you get the insights you need to build the right products and experiences faster.
Frequently asked questions about qualitative research examples
What is qualitative research?
Qualitative research is a research method that aims to provide contextual, descriptive, and non-numerical insights on a specific issue. Qualitative research methods like interviews, case studies, and ethnographic studies allow you to uncover the reasoning behind your user’s attitudes and opinions.
Can a study be both qualitative and quantitative?
Absolutely! You can use mixed methods in your research design, which combines qualitative and quantitative approaches to gain both descriptive and statistical insights.
For example, user surveys can have both close-ended and open-ended questions, providing comprehensive data like percentages of user views and descriptive reasoning behind their answers.
Is qualitative or quantitative research better?
The choice between qualitative and quantitative research depends upon your research goals and objectives.
Qualitative research methods are better suited when you want to understand the complexities of your user’s problems and uncover the underlying motives beneath their thoughts, feelings, and behaviors. Quantitative research excels in giving you numerical data, helping you gain a statistical view of your user's attitudes, identifying trends, and making predictions.
What are some approaches to qualitative research?
There are many approaches to qualitative studies. An approach is the underlying theory behind a method, and a method is a way of implementing the approach. Here are some approaches to qualitative research:
- Grounded theory: Researchers study a topic and develop theories inductively
- Phenomenological research: Researchers study a phenomenon through the lived experiences of those involved
- Ethnography: Researchers immerse themselves in organizations to understand how they operate
In order to utilize all of the features of this web site, JavaScript must be enabled in your browser.
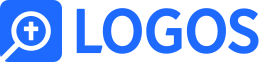
Interpretative Phenomenological Analysis: Theory, Method and Research
Digital Logos Edition
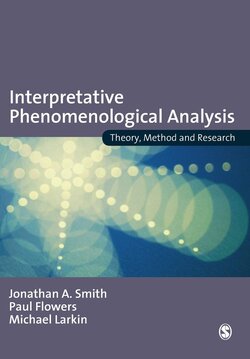
In production
This book presents a comprehensive guide to interpretative phenomenological analysis (IPA) which is an increasingly popular approach to qualitative inquiry taught to undergraduate and postgraduate students today. The first chapter outlines the theoretical foundations for IPA. It discusses phenomenology, hermeneutics, and idiography and how they have been taken up by IPA. The next four chapters provide detailed, step by step guidelines to conducting IPA research: study design, data collection and interviewing, data analysis, and writing up. In the next section, the authors give extended worked examples from their own studies in health, sexuality, psychological distress, and identity to illustrate the breadth and depth of IPA research. The final section of the book considers how IPA connects with other contemporary qualitative approaches like discourse and narrative analysis and how it addresses issues to do with validity.
Key Features
- Presents a comprehensive guide to interpretative phenomenological analysis.
- Outlines the theoretical foundations for IPA.
- Provides detailed, step by step guidelines to conducting IPA research.
Product Details
- Title : Interpretative Phenomenological Analysis: Theory, Method and Research
- Authors : Jonathan A. Smith , Paul Flowers , Michael Larkin
- Edition: 2nd Edition
- Publisher : SAGE
- Print Publication Date: 2022
- Logos Release Date: 2024
- Era: era:contemporary
- Language : English
- Resources: 1
- Format : Digital › Logos Research Edition
- Subjects : Phenomenological psychology; Psychology › Research
- ISBNs : 9781529753806 , 9781529753790 , 1529753805 , 1529753791
- Resource ID: LLS:NTRPRTTVPHRSRCH
- Resource Type: Monograph
- Metadata Last Updated: 2024-08-16T22:26:51Z
Sign in with your Logos account
Information
Initiatives
You are accessing a machine-readable page. In order to be human-readable, please install an RSS reader.
All articles published by MDPI are made immediately available worldwide under an open access license. No special permission is required to reuse all or part of the article published by MDPI, including figures and tables. For articles published under an open access Creative Common CC BY license, any part of the article may be reused without permission provided that the original article is clearly cited. For more information, please refer to https://www.mdpi.com/openaccess .
Feature papers represent the most advanced research with significant potential for high impact in the field. A Feature Paper should be a substantial original Article that involves several techniques or approaches, provides an outlook for future research directions and describes possible research applications.
Feature papers are submitted upon individual invitation or recommendation by the scientific editors and must receive positive feedback from the reviewers.
Editor’s Choice articles are based on recommendations by the scientific editors of MDPI journals from around the world. Editors select a small number of articles recently published in the journal that they believe will be particularly interesting to readers, or important in the respective research area. The aim is to provide a snapshot of some of the most exciting work published in the various research areas of the journal.
Original Submission Date Received: .
- Active Journals
- Find a Journal
- Proceedings Series
- For Authors
- For Reviewers
- For Editors
- For Librarians
- For Publishers
- For Societies
- For Conference Organizers
- Open Access Policy
- Institutional Open Access Program
- Special Issues Guidelines
- Editorial Process
- Research and Publication Ethics
- Article Processing Charges
- Testimonials
- Preprints.org
- SciProfiles
- Encyclopedia
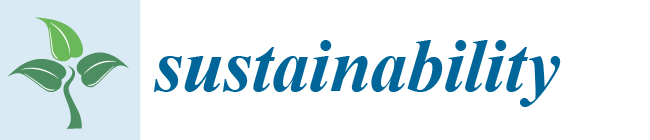
Article Menu

- Subscribe SciFeed
- Recommended Articles
- Google Scholar
- on Google Scholar
- Table of Contents
Find support for a specific problem in the support section of our website.
Please let us know what you think of our products and services.
Visit our dedicated information section to learn more about MDPI.
JSmol Viewer
Investigating the effectiveness of endogenous and exogenous drivers of the sustainability (re)orientation of family smes in slovenia: qualitative content analysis approach.
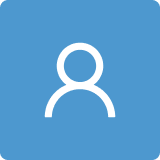
1. Introduction
2. literature review, 2.1. legal framework on sustainable corporate governance (with a focus on smes), 2.1.1. corporate sustainability reporting directive, 2.1.2. corporate sustainability due diligence directive, 2.1.3. scope of the csddd for smes, 2.2. drivers of the family businesses’ (re)orientation towards sustainability, 2.3. endogenous drivers, 2.3.1. the protection of sew, 2.3.2. ownership and management composition, 2.3.3. values, beliefs and attitudes of family owner-managers, 2.3.4. transgenerational continuity and long-term orientation, 2.3.5. knowledge of sustainability, 2.4. exogenous drivers, 2.4.1. stakeholders pressure, 2.4.2. the impact of institutional environment and local communities, 3. empirical research, 3.1. institutional context of slovenia, 3.2. research method, 3.3. sampling and data collection, 3.4. data analysis, 4.1. results of the final coding of the family businesses’ sustainability (re)orientation, 4.2. references to responsibility, preserving (natural) environment and sustainability/sustainable development in the analysed statements, 4.3. family businesses with a higher level of sustainability awareness and orientation, 5. discussion, 5.1. sustainability awareness and readiness of investigated family smes to comply with the new eu legal framework, 5.2. the effectiveness of endogenous and exogenous drivers of family businesses’ sustainability (re)orientation, 6. conclusions, author contributions, institutional review board statement, informed consent statement, data availability statement, conflicts of interest.
- Communication from the Commission to the European Parliament, the Council, the European Economic and Social Committee and the Committee of The Regions, An SME Strategy for a sustainable and digital Europe, COM/2020/103 final. Available online: https://eur-lex.europa.eu/legal-content/EN/TXT/?uri=COM%3A2020%3A103%3AFIN (accessed on 25 June 2024).
- Al Malki, M.A. Review of Sustainable Growth challenges faced by Small and Medium Enterprises. Int. J. Glob. Acad. Sci. Res. 2023 , 2 , 35–43. [ Google Scholar ] [ CrossRef ]
- European Parliament and the Council. Directive (EU) 2022/2464 of the European Parliament and of the Council of 14 December 2022 amending Regulation (EU) No 537/2014, Directive 2004/109/EC, Directive 2006/43/EC, and Directive 2013/34/EU. OJ L 322, 16.12.2022, 15–80. Available online: https://eur-lex.europa.eu/eli/dir/2022/2464 (accessed on 21 August 2024).
- Fogarty, V.; Flucker, S. Sustainability. In Data Centre Essentials: Design, Construction, and Operation of Data Centres for the Non-expert ; Wiley Online Library: Hoboken, NJ, USA, 2023. [ Google Scholar ] [ CrossRef ]
- Primec, A.; Belak, J. Sustainable CSR: Legal and Managerial Demands of the New EU Legislation (CSRD) for the Future Corporate Governance Practices. Sustainability 2022 , 14 , 16648. [ Google Scholar ] [ CrossRef ]
- Umantsiv, H. Reporting on sustainable development in the context of corporate social responsibility. Cientia Ructuosa 2023 , 149 , 59–71. [ Google Scholar ] [ CrossRef ]
- European Parliament and the Council. Directive of the European Parliament and of the Council on Corporate Sustainability Due Diligence and amending Directive (EU) 2019/1937 and Regulation (EU) 2023/2859. Available online: https://eur-lex.europa.eu/legal-content/EN/TXT/PDF/?uri=CONSIL:PE_9_2024_REV_1 (accessed on 25 June 2024).
- Proposal for a Directive on Corporate Sustainability Due Diligence and amending Directive (EU) 2019/1937. COM/2022/71 final. Available online: https://eur-lex.europa.eu/legal-content/EN/TXT/?uri=CELEX%3A52022PC0071 (accessed on 29 June 2024).
- Sørensen, K.E. Corporate Sustainability Due Diligence in Groups of Companies. Eur. Co. Law 2022 , 19 , 119–130. [ Google Scholar ] [ CrossRef ]
- Arena, C.; Michelon, G. A matter of control or identity? Family firms’ environmental reporting decisions along the corporate life cycle. Bus. Strategy Environ. 2018 , 27 , 1596–1608. [ Google Scholar ] [ CrossRef ]
- Dekker, J.; Hasso, T. Environmental Performance Focus in Private Family Firms: The Role of Social Embeddedness. J. Bus. Ethics 2016 , 136 , 293–309. [ Google Scholar ] [ CrossRef ]
- Sharma, P.; Sharma, S. The Role of Family Firms in Corporate Sustainability. In The Oxford Handbook of Management Ideas ; Sturdy, A., Hueskinveld, S., Reay, T., Strang, D., Eds.; Oxford Handbooks Online: Oxford, UK, 2019; pp. 1–18. Available online: https://www.academia.edu/44598484/The_Role_of_Family_Firms_in_Corporate_Sustainability (accessed on 10 October 2023).
- European Family Business Barometer. EFB and KPMG Enterprise. Available online: http://www.europeanfamilybusinesses.eu/uploads/Modules/Publications/european-family-business-barometer7ed.pdf (accessed on 25 January 2023).
- Report on family businesses in Europe. Brussel: Committee on Industry, Research and Energy. Available online: https://www.europarl.europa.eu/doceo/document/TA-8-2015-0290_EN.pdf (accessed on 1 June 2024).
- Berrone, P.; Cruz, C.; Gomez-Mejia, L.R.; Larraza-Kintana, M. Socioemotional Wealth and Corporate Responses to Institutional Pressures: Do Family-Controlled Firms Pollute Less? Adm. Sci. Q. 2010 , 55 , 82–113. [ Google Scholar ] [ CrossRef ]
- Canavati, S. Corporate social performance in family firms: A meta-analysis. J. Fam. Bus. Manag. 2018 , 8 , 235–273. [ Google Scholar ] [ CrossRef ]
- Dyer, W.G.; Whetten, D.A. Family Firms and Social Responsibility: Preliminary Evidence from the S&P 500. Entrep. Theory Pract. 2006 , 30 , 785–802. [ Google Scholar ] [ CrossRef ]
- Cruz, C.; Larraza-Kintana, M.; Garcés-Galdeano, L.; Berrone, P. Are Family Firms Really More Socially Responsible? Entrep. Theory Pract. 2014 , 38 , 1295–1316. [ Google Scholar ] [ CrossRef ]
- Le Breton-Miller, I.; Miller, D. Family firms and practices of sustainability: A contingency view. J. Fam. Bus. Strategy 2016 , 7 , 26–33. [ Google Scholar ] [ CrossRef ]
- Samara, G.; Jamalia, D.; Sierrac, V.; Paradab, M.J. Who are the best performers? The environmental social performance of family firms. J. Fam. Bus. Strategy 2018 , 9 , 33–43. [ Google Scholar ] [ CrossRef ]
- Dal Maso, L.; Basco, R.; Bassetti, T.; Lattanzi, N. Family ownership and environmental performance: The mediation effect of human resource practices. Bus. Strategy Environ. 2020 , 29 , 1548–1562. [ Google Scholar ] [ CrossRef ]
- Dou, J.; Su, E.; Wang, S. When Does Family Ownership Promote Proactive Environmental Strategy? The Role of the Firm’s Long-Term Orientation. J. Bus. Ethics 2019 , 158 , 81–95. [ Google Scholar ] [ CrossRef ]
- Graafland, J. Family business ownership and cleaner production: Moderation by company size and family management. J. Clean. Prod. 2020 , 255 , 1–10. [ Google Scholar ] [ CrossRef ]
- Miroshnychenko, I.; De Massis, A.; Barontini, R.; Testa, F. Family Firms and Environmental Performance: A Meta-Analytic Review. Fam. Bus. Rev. 2022 , 35 , 68–90. [ Google Scholar ] [ CrossRef ]
- Van Gils, A.; Dibrell, C.; Neubaum, D.O.; Craig, J.B. Social Issues in the Family Enterprise. Fam. Bus. Rev. 2014 , 27 , 193–205. [ Google Scholar ] [ CrossRef ]
- Kim, J.; Fairclough, S.; Dibrell, C. Attention, Action, and Greenwash in Family-Influenced Firms? Evidence From Polluting Industries. Organ. Environ. 2017 , 30 , 304–323. [ Google Scholar ] [ CrossRef ]
- Sharma, P.; Sharma, S. Drivers of Proactive Environmental Strategy in Family Firms. Bus. Ethics Q. 2011 , 21 , 309–334. [ Google Scholar ] [ CrossRef ]
- Roxas, B.; Coetzer, A. Institutional Environment, Managerial Attitudes and Environmental Sustainability Orientation of Small Firms. J. Bus. Ethics 2012 , 111 , 461–476. [ Google Scholar ] [ CrossRef ]
- Vourvachis, P.; Woodward, T. Content analysis in social and environmental reporting research: Trends and challenges. J. Appl. Account. Res. 2015 , 16 , 166–195. [ Google Scholar ] [ CrossRef ]
- Eriksson, P.; Kovalainen, A. Qualitative Methods in Business Research , 2nd ed.; SAGE: Thousand Oak, CA, USA, 2016. [ Google Scholar ]
- Schreier, M. Qualitative Content Analysis in Practice ; SAGE: London, UK, 2012. [ Google Scholar ]
- Antončič, B.; Auer Antončič, J.; Juričič, D. Družinsko Podjetništvo: Značilnosti v Sloveniji (Family Entrepreneurship: Characteristics in Slovenia) ; EY: Ljubljana, Slovenia, 2015. [ Google Scholar ]
- Berrone, P.; Cruz, C.; Gomez-Mejia, L.R. Socioemotional Wealth in Family Firms: Theoretical Dimensions, Assessment Approaches, and Agenda for Future Research. Fam. Bus. Rev. 2012 , 25 , 258–279. [ Google Scholar ] [ CrossRef ]
- Gómez-Mejía, L.R.; Takács Haynes, K.; Núñez-Nickel, M.; Jacobson, K.J.L.; Moyano-Fuentes, J. Socioemotional Wealth and Business Risks in Family-controlled Firms: Evidence from Spanish Olive Oil Mills. Adm. Sci. Q. 2007 , 52 , 106–137. [ Google Scholar ] [ CrossRef ]
- Darnall, N.; Henriques, I.; Sadorsky, P. Adopting Proactive Environmental Strategy: The Influence of Stakeholders and Firm Size. J. Manag. Stud. 2010 , 47 , 1072–1094. [ Google Scholar ] [ CrossRef ]
- Gómez-Mejía, L.R.; Cruz, C.; Berrone, P.; De Castro, J. The Bind that Ties: Socioemotional Wealth Preservation in Family Firms. Acad. Manag. Ann. 2011 , 5 , 653–707. [ Google Scholar ] [ CrossRef ]
- Studdert, J.; Govender, V.; Spies, J.; Jarque Branguli, M.; Nievas, S.; Roca Silva, M.F.; Zhu, W.; Diebschlag, P.; Sassine, R.; Cilliers, W.; et al. United Nations Global Compact. Available online: https://unglobalcompact.org/what-is-gc/mission/principles (accessed on 17 March 2024).
- Primec, A.; Tičar, B. A new challenge in corporate governance—the new legal regulation of nonfinancial reporting. In Recent Challenges in Corporate Governance ; Bohinc, R., Ed.; Fakulteta za družbene vede, Založba FDV: Ljubljana, Slovenia, 2023; pp. 91–112. [ Google Scholar ] [ CrossRef ]
- Primec, A. Nefinančno poročanje kot pravni institut za vzpostavljanje korporativne družbene odgovornosti. Podjet. Delo 2023 , 6–7 , 1231–1240. Available online: https://www.dlib.si/details/URN:NBN:SI:doc-MF5DYSLE (accessed on 21 August 2024).
- Pacces, A.M. Civil Liability in the EU Corporate Sustainability Due Diligence Directive Proposal: A Law & Economics Analysis. European Corporate Governance Institute - Law Working Paper No. 691/2023, Amsterdam Law School Research Paper No. 2023-14, Amsterdam Center for Law & Economics Working Paper No. 2023-02. Forthcoming in Ondernemingsrecht. [ CrossRef ]
- Delmas, M.A.; Gergaud, O. Sustainable Certification for Future Generations: The Case of Family Business. Fam. Bus. Rev. 2014 , 27 , 228–243. [ Google Scholar ] [ CrossRef ]
- Bingham, J.B.; Dyer, W.G., Jr.; Smith, I.; Adams, G.L. A Stakeholder Identity Orientation Approach to Corporate Social Performance in Family Firms. J. Bus. Ethics 2010 , 99 , 565–585. [ Google Scholar ] [ CrossRef ]
- Cennamo, C.; Berrone, P.; Cruz, C.; Gomez-Mejia, L.R. Socioemotional Wealth and Proactive Stakeholder Engagement: Why Family-Controlled Firms Care More About Their Stakeholders. Entrep. Theory Pract. 2012 , 36 , 1153–1173. [ Google Scholar ] [ CrossRef ]
- Duh, M.; Tominc, P. Pomen, značilnosti in prihodnost družinskih podjetij (Importance, characteristics and future of family businesses). In Slovenski Podjetniški Observatorij 2004 , 2nd ed.; Rebernik, M., Tominc, P., Duh, M., Krošlin, T., Radonjič, G., Eds.; Inštitut za podjetništvo in management malih podjetij, Ekonomsko-poslovna fakulteta, Univerza v Mariboru: Maribor, Slovenia, 2005; pp. 19–31. [ Google Scholar ]
- Mandl, I. Overview of Family Business Relevant Issues. Final Report, Austrian Institute for SME Research: Vienna. Available online: https://ec.europa.eu/docsroom/documents/10389/attachments/1/translations/en/renditions/native (accessed on 1 February 2023).
- Sachs, J.D.; Kroll, C.; Lafortune, G.; Fuller, G.; Woelm, F. Sustainable Development Report 2021: Includes the SDG Index and Dashboards ; The Decade of Action for the Sustainable Development Goal; SDSN, Bertelsmann Stiftung, Cambridge University Press: Cambridge, UK, 2021. [ Google Scholar ] [ CrossRef ]
- Sachs, J.D.; Lafortune, G.; Fuller, G. The SDGs and the UN Summit of the Future. Sustainable Development Report 2024 ; SDSN: Paris, France; Dublin University Press: Dublin, Ireland, 2024. [ Google Scholar ] [ CrossRef ]
- UMAR. Poročilo o Razvoju 2023 (Report on Development 2023). Ljubljana: Urad RS za Makroekonomske Analize in Razvoj. Available online: https://www.umar.gov.si/fileadmin/user_upload/razvoj_slovenije/2023/slovenski/POR2023-splet.pdf (accessed on 10 April 2024).
- Creswell, J.W. Qualitative Inquiry and Research Design: Choosing among Five Approaches ; SAGE: Thousand Oaks, CA, USA, 2013. [ Google Scholar ]
- Myers, M.D. Qualitative Research in Business & Management ; SAGE: Thousand Oaks, CA, USA, 2013. [ Google Scholar ]
- De Massis, A.; Kotlar, A. The case study method in family business research: Guidelines for qualitative scholarship. J. Fam. Bus. Strategy 2014 , 5 , 15–29. [ Google Scholar ] [ CrossRef ]
- Campopiano, G.; De Massis, A. Corporate Social Responsibility Reporting: A Content Analysis in Family and Nonfamily. J. Bus. Ethics 2015 , 129 , 511–534. [ Google Scholar ] [ CrossRef ]
- Craig, J.; Dibrell, C. The Natural Environment, Innovation, and Firm Performance: A Comparative Study. Fam. Bus. Rev. 2006 , 19 , 275–288. [ Google Scholar ] [ CrossRef ]
- Sharma, P.; Chrisman, J.J.; Chua, J.H. Predictors of satisfaction with the succession process in family firms. J. Bus. Ventur. 2003 , 18 , 667–687. [ Google Scholar ] [ CrossRef ]
- Whittingham, K.L.; Earle, A.G.; Leyva-de la Hiz, D.I.; Argiolas, A. The impact of the United Nations Sustainable Development Goals on corporate sustainability reporting. Bus. Res. Q. 2023 , 26 , 45–61. [ Google Scholar ] [ CrossRef ]
- Cabrera-Suárez, K. Leadership transfer and the successor’s development in the family firm. Leadership Quarterly 2005 , 16 , 71–96. [ Google Scholar ] [ CrossRef ]
- Rus, D.; Močnik, D.; Crnogaj, K. Podjetniška Demografija in Značilnosti Startup in Scaleup Podjetij: Slovenski Podjetniški Observatorij 2022 (Business Demographics and Characteristics of Startup and Scaleup Companies: Slovenian Entrepreneurship Observatory 2022) ; niverza v Mariboru, Univerzitetna založba: Maribor, Slovenia, 2023. [ Google Scholar ]
- Data base of Slovenian exporters. Available online: https://www.sloexport.si/ (accessed on 30 August 2023).
- Bizi. Data base of financial and contact data of Slovenian companies. Available online: https://www.bizi.si/ (accessed on 30 August 2023).
- Family Business Slovenia 2019. Ljubljana: EY. Available online: https://www.ey.com/en_si/family-enterprise/familiy-business-book-slovenia-2019 (accessed on 30 November 2023).
- Family Business Slovenia 2020. Ljubljana: EY. Available online: https://www.ey.com/en_si/family-enterprise/family-business-book-slovenia-2021 (accessed on 30 November 2023).
- Family Business Slovenia 2021. Ljubljana: EY. Available online: https://www.ey.com/en_si/family-enterprise/family-business-book-slovenia-2020 (accessed on 30 November 2023).
- Payne, G.T.; Brigham, K.H.; Broberg, J.C.; Moss, T.W.; Short, J.C. Organizational Virtue Orientation and Family Firms. Bus. Ethics Q. 2011 , 21 , 257–285. [ Google Scholar ] [ CrossRef ]
- Schreier, M. Qualitative Content Analysis. In The SAGE Handbook of Qualitative Data Analysis ; Flick, U., Ed.; SAGE: Thousand Oaks, CA, USA, 2014; pp. 170–183. [ Google Scholar ]
- Müller-Stewens, G.; Lechner, C. Strategisches Management, 5. Auflage ; Schäffer-Poeschel Verlag: Stuttgart, Germany, 2016. [ Google Scholar ]
- Wheelen, T.L.; Hunger, J.D. Strategic Management and Business Policy. Toward Global Sustainability , 13th ed.; Prentice Hall, Pearson: New Jersey, NJ, USA, 2012. [ Google Scholar ]
- WCED. Our Common Future. Report of the World Commission on Environment and Development. Available online: https://sustainabledevelopment.un.org/content/documents/5987our-common-future.pdf (accessed on 1 October 2021).
- Sjåfjell, B. Reforming EU company law to secure the future of European business. Eur. Co. Financ. Law Rev. 2021 , 18 , 190–217. [ Google Scholar ] [ CrossRef ]
No. of Category | Category Name and Its Definition | No. of Subcat. | Subcategory |
---|
C1 | Vision Describe what a firm would like to become. | C1.1 | Reference to sustainability/sustainable development |
| | C1.2 | Reference to preserving (natural) environment |
| | C1.3 | Reference to a position in market(s) and/or industry |
| | C1.4 | Reference to the characteristics of products |
| | C1.5 | Miscellaneous |
C2 | Mission Defines the purpose and reason why a firm exists. | C2.1 | Reference to sustainability/sustainable development |
| | C2.2 | Reference to preserving (natural) environment |
| | C2.3 | Reference to the characteristics of products |
| | C2.4 | Reference to the customers’ needs |
C3 | Goals The result of planned activities, can be quantified or open-ended statement with no quantification. | C3.1 | Reference to sustainability/sustainable development |
| | C3.2 | Reference to a position in market(s) and/or industry |
| | C3.3 | Miscellaneous |
C4 | Values Consider what should be and what is desirable. | C4.1 | Reference to sustainability/sustainable development |
| | C4.2 | Reference to preserving (natural) environment |
| | C4.3 | Reference to responsibility |
| | C4.4 | Miscellaneous |
C5 | Strategies or strategic directions State how a company is going to achieve its vision, mission and goals. | C5.1 | Reference to sustainability/sustainable development |
| | C5.2 | Reference to preserving (natural) environment |
| | C5.3 | References to (expansion to) new markets |
C6 | Specific of functioning Activities, processes, behaviour. | C6.1 | Reference to sustainability/sustainable development |
| | C6.2 | Reference to preserving (natural) environment |
| | C6.3 | Reference to the characteristics of products |
| | C6.4 | Reference to competitive strengths |
| | C6.5 | Miscellaneous |
Unit of Analysis (A Family Business) | C1 Vision | C2 Mission | C3 Goals | C4 Values | C5 Strategies or Strategic Directions | C6 Specifics of Functioning |
---|
U1 | C1.1 | C2.1 | C3.2 | | C5.1 | |
U2 | | | | | C5.3 | C6.4 |
U3 | | | | | | C6.2 |
U4 | | C2.4 | C3.2 | | | |
U5 | C1.3 | | C3.2 | | C5.2 | |
U6 | C1.3 | C2.4 | | | | |
U7 | | | C3.2 | | | C6.3 |
U8 | C1.1 | | | C4.3 | | C6.1 |
U9 | C1.3 | C2.2 | | | C5.3 | C6.2 |
U10 | C1.4 | | | | | |
U11 | | | C3.2 | | | |
U12 | | | C3.2 | C4.2 | | C6.2 |
U13 | | | | C4.1 | | C6.2 |
U14 | C1.2 | C2.3 | | | | C6.4 |
U15 | C1.4 | C2.3 | | | | |
U16 | C1.1 | | | | | C6.1 |
U17 | | | | | | C6.4 |
U18 | C1.5 | | | C4.2 | | |
U19 | C1.2 | | C3.3 | | | C6.2 |
U20 | | | | | | C6.3 |
U21 | C1.3 | C2.4 | | C4.2 | | |
U22 | C1.3 | | | C4.2 | | C6.2 |
U23 | C1.1 | | | C4.4 | C5.1 | C6.1 |
U24 | C1.3 | | | C4.3 | | C6.4 |
U25 | C1.1 | C2.2 | C3.1 | | C5.1 | C6.2 |
U26 | | | | | | C6.4 |
Family businesses with published statement (number) | 16 | 8 | 8 | 8 | 6 | 17 |
Family businesses with reference to sustainability and protection of natural environment, responsibility (number) | 7 | 3 | 1 | 7 | 4 | 10 |
| U1 | U8 | U23 | U25 |
---|
Family name in in the name of a company | no | no | no | no |
Ownership (generation, number of family owners, % of family ownership) | first and second generation (father, two sons), 100% | first generation (founder), 100% | first generation (husband and wife), 100% | first generation (founder), 100% |
Management (generation, number of family managers) | second generation (two sons) | first generation (founder’s wife) | first and second generation (husband, wife, and both children) | first and second generation (founder—father, daughter) |
Size | small | medium-sized | medium-sized | medium-sized |
Main activity and markets | wholesale and retail trade; market: Slovenia | manufacturing; markets: Slovenia, other countries | manufacturing; markets: Slovenia, other countries | manufacturing; markets: Slovenia, other countries |
The year of establishment | 1990 | 1989 | 1995 | 1992 |
| Family Name in the Name of a Company | Ownership (Generation, % of Family Ownership) | Management (Generation) | Size | Main Activity | The Year of Establishment |
---|
U2 | no | first and second, 100% | second | small | manufacturing | 1993 |
U4 | yes | third, 100% | third | small | manufacturing | 1992 |
U6 | no | second, 100% | second | small | manufacturing | 1995 |
U7 | yes | first, 100% | first | small | wholesale and retail trade | 1993 |
U10 | no | first, 100% | first | micro | service activities | 2009 |
U11 | no | third, 100% | third | small | wholesale and retail trade | 1960 |
U15 | no | first and second, 100% | first and second | small | agriculture | 1991 |
U17 | no | first, 100% | first and second | micro | agriculture | 2007 |
U20 | yes | first, 100% | first and second | small | manufacturing | 1982 |
U26 | yes | Second, 100% | second | medium-sized | wholesale and retail trade | 1988 |
| The statements, opinions and data contained in all publications are solely those of the individual author(s) and contributor(s) and not of MDPI and/or the editor(s). MDPI and/or the editor(s) disclaim responsibility for any injury to people or property resulting from any ideas, methods, instructions or products referred to in the content. |
Share and Cite
Duh, M.; Primec, A. Investigating the Effectiveness of Endogenous and Exogenous Drivers of the Sustainability (Re)Orientation of Family SMEs in Slovenia: Qualitative Content Analysis Approach. Sustainability 2024 , 16 , 7285. https://doi.org/10.3390/su16177285
Duh M, Primec A. Investigating the Effectiveness of Endogenous and Exogenous Drivers of the Sustainability (Re)Orientation of Family SMEs in Slovenia: Qualitative Content Analysis Approach. Sustainability . 2024; 16(17):7285. https://doi.org/10.3390/su16177285
Duh, Mojca, and Andreja Primec. 2024. "Investigating the Effectiveness of Endogenous and Exogenous Drivers of the Sustainability (Re)Orientation of Family SMEs in Slovenia: Qualitative Content Analysis Approach" Sustainability 16, no. 17: 7285. https://doi.org/10.3390/su16177285
Article Metrics
Article access statistics, further information, mdpi initiatives, follow mdpi.
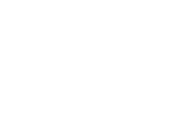
Subscribe to receive issue release notifications and newsletters from MDPI journals
Thank you for visiting nature.com. You are using a browser version with limited support for CSS. To obtain the best experience, we recommend you use a more up to date browser (or turn off compatibility mode in Internet Explorer). In the meantime, to ensure continued support, we are displaying the site without styles and JavaScript.
- View all journals
- Explore content
- About the journal
- Publish with us
- Sign up for alerts
- Open access
- Published: 23 August 2024
Tissue-resident memory T cells in epicardial adipose tissue comprise transcriptionally distinct subsets that are modulated in atrial fibrillation
- Vishal Vyas 1 , 2 ,
- Balraj Sandhar ORCID: orcid.org/0000-0001-7569-0163 1 ,
- Jack M. Keane ORCID: orcid.org/0009-0002-8248-7563 1 ,
- Elizabeth G. Wood 1 ,
- Hazel Blythe ORCID: orcid.org/0000-0002-7824-4019 1 ,
- Aled Jones ORCID: orcid.org/0000-0002-6718-0646 1 ,
- Eriomina Shahaj ORCID: orcid.org/0000-0001-5428-0405 1 ,
- Silvia Fanti ORCID: orcid.org/0000-0003-2505-7102 1 ,
- Jack Williams 1 ,
- Nasrine Metic ORCID: orcid.org/0009-0007-1723-5390 3 ,
- Mirjana Efremova ORCID: orcid.org/0000-0002-8107-9974 3 ,
- Han Leng Ng ORCID: orcid.org/0000-0001-7316-2842 4 ,
- Gayathri Nageswaran 5 ,
- Suzanne Byrne 5 ,
- Niklas Feldhahn 4 ,
- Federica Marelli-Berg ORCID: orcid.org/0000-0001-8747-5823 1 ,
- Benny Chain 5 ,
- Andrew Tinker ORCID: orcid.org/0000-0001-7703-4151 1 ,
- Malcolm C. Finlay 1 , 2 &
- M. Paula Longhi ORCID: orcid.org/0000-0003-1854-4594 1
Nature Cardiovascular Research ( 2024 ) Cite this article
131 Accesses
12 Altmetric
Metrics details
- Atrial fibrillation
- Inflammation
- Lymphocyte activation
Atrial fibrillation (AF) is the most common sustained arrhythmia and carries an increased risk of stroke and heart failure. Here we investigated how the immune infiltrate of human epicardial adipose tissue (EAT), which directly overlies the myocardium, contributes to AF. Flow cytometry analysis revealed an enrichment of tissue-resident memory T (T RM ) cells in patients with AF. Cellular indexing of transcriptomes and epitopes by sequencing (CITE-seq) and single-cell T cell receptor (TCR) sequencing identified two transcriptionally distinct CD8 + T RM cells that are modulated in AF. Spatial transcriptomic analysis of EAT and atrial tissue identified the border region between the tissues to be a region of intense inflammatory and fibrotic activity, and the addition of T RM populations to atrial cardiomyocytes demonstrated their ability to differentially alter calcium flux as well as activate inflammatory and apoptotic signaling pathways. This study identified EAT as a reservoir of T RM cells that can directly modulate vulnerability to cardiac arrhythmia.
Similar content being viewed by others
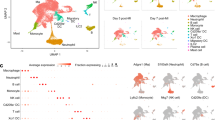
Spatiotemporal dynamics of macrophage heterogeneity and a potential function of Trem2 hi macrophages in infarcted hearts
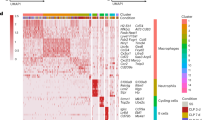
TREM2 hi resident macrophages protect the septic heart by maintaining cardiomyocyte homeostasis
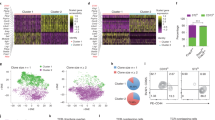
Insulin signaling establishes a developmental trajectory of adipose regulatory T cells
Atrial fibrillation (AF) is the most common sustained arrhythmia worldwide, defined by rapid, uncoordinated atrial activity with consequent deterioration of atrial mechanical function 1 . Patients suffering from AF have poorer outcomes in heart failure, an increased risk of cognitive decline and vascular dementia as well as a five-fold increased risk of stroke 2 , 3 . The financial costs are commensurate with this health burden, with over £2 billion spent annually in healthcare costs within England alone 4 .
The exact etiology of AF remains incompletely understood, requiring complex interactions between triggers and the underlying atrial substrate to sustain AF. Inflammation is known to play a key role in the formation of AF substrate, promoting electrical and structural remodeling of the atrium and increasing vulnerability to AF 5 . Several studies described an association between AF and serum inflammatory biomarkers, such as C-reactive protein (CRP) and IL-6 (refs. 6 , 7 ). However, only a limited number of studies have looked at the immune infiltrate in the atrial tissue itself. Abnormal atrial histology, characterized by inflammatory infiltrates, fibrosis and expression of pro-inflammatory cytokines, has been identified in tissue biopsies of patients with AF 8 , 9 , 10 , 11 . However, the patient numbers and range of tissue analyses in these studies remain limited.
Numerous lines of evidence suggest a role of epicardial adipose tissue (EAT) in the development of AF. EAT is the visceral fat depot of the heart that shares direct anatomic contact with the myocardium without fascial interruption. EAT has been demonstrated to be a significant source of inflammatory mediators that can exert paracrine and vasocrine effects on the myocardium 12 . Several observational studies have demonstrated that EAT volume is consistently associated with the presence, severity and recurrence of AF 13 , 14 , 15 . Furthermore, increased EAT inflammation, as measured by 18 F-fluorodeoxyglucose (FDG) uptake, was observed in patients with AF compared to those in sinus rhythm (SR) 16 , 17 . A range of pathophysiological mechanisms could contribute to the association between EAT and AF, including adipocyte infiltration, oxidative stress and the paracrine effect of pro-fibrotic and pro-inflammatory cytokines. However, the exact immune structure and cellular characterization of EAT in AF remains elusive.
In the present study, we investigated the pathophysiological significance of the immune infiltrate in the EAT of patients with AF. Flow cytometry analysis identified an enrichment of tissue-resident memory T (T RM ) cells in patients with AF compared to SR controls. T RM cells are a specialized subset of memory T cells that persist long term in peripheral tissues with minimal recirculation. To further characterize these cells, we applied cellular indexing of transcriptomes and epitopes by sequencing (CITE-seq) combined with single-cell T cell receptor (TCR) sequencing, which identified two transcriptionally distinct CD8 + T RM cells that are modulated in AF. Furthermore, spatial transcriptomic analysis of the border zone between the EAT and the atrial, together with functional analysis, suggests that EAT is a reservoir of T RM cells that can serve as mediators of inflammation in the myocardium.
T RM cells are increased in the EAT of patients with AF
A total of 153 participants undergoing open heart surgery were recruited to this study. The mean age was 66.1 years, with a mean body mass index (BMI) of 28.2 kg m −2 , and 75% of participants were male, with 50% undergoing a coronary artery bypass surgery (Supplementary Table 1 ). To evaluate the immune profile of the EAT in AF, participants were classified into two groups based on their 12-lead electrocardiogram (ECG)-confirmed rhythm, recorded preoperatively: AF or those in normal rhythm (SR). Thirty-one participants developed postoperative AF and were, therefore, excluded from the study. Patients with AF were older and more likely to undergo valve surgery (Supplementary Table 2 ). As expected, patients with AF had a more dilated left atrium, as previously described, as a result of tissue remodeling 18 . Thus, to account for key variables, we performed propensity score matching with age, gender, BMI, diabetes, hypertension and procedure type as covariates (Table 1 ). Key immune cells were identified by flow cytometry in the EAT, in subcutaneous adipose tissue (SAT) as a control adipose tissue and in blood as a marker of the systemic inflammatory state. No differences were observed in cell number between the groups and across the tissues (Extended Data Fig. 1a–d ). The frequencies of myeloid and lymphoid cells were largely unchanged with the exception of a decrease in CD14 + CD206 + macrophages and an increase in total CD3 + T cells in patients with AF (Extended Data Fig. 1e ). We previously showed that T cells are a predominant immune cell constituent in human EAT 19 . Analysis of T cell subsets identified an increase in T RM cells, as defined by high expression of CD69 and the inhibitory molecule programmed cell death 1 (PD1) receptor, in the EAT of patients with AF compared to SR (Fig. 1a and Extended Data Fig. 2a ), which was accompanied by a decrease in CD69 − memory T cells (Extended Data Fig. 1e ). The increase in T RM cells was readily evident in unmatched participants and independent of risk factors, such as age, hypertension and body weight (Extended Data Fig. 2b–e ). T RM cells are transcriptionally programmed for strong effector function to confer swift on-site immune protection 20 . We then evaluated T cell cytokine production by intracellular staining. The gating strategy is shown in Extended Data Fig. 2f,g . A clear correlation between IFN-γ and IL-17 production and the presence of CD4 + T RM cells was observed (Fig. 1b ). No such correlation was detected for IFN-γ and CD8 + T RM cells, which could be attributed to the high cytotoxic activity of these cells (Extended Data Fig. 2h,i ). Overall, our data suggest that T RM cells could play a pathological role in the development and/or persistence of AF.
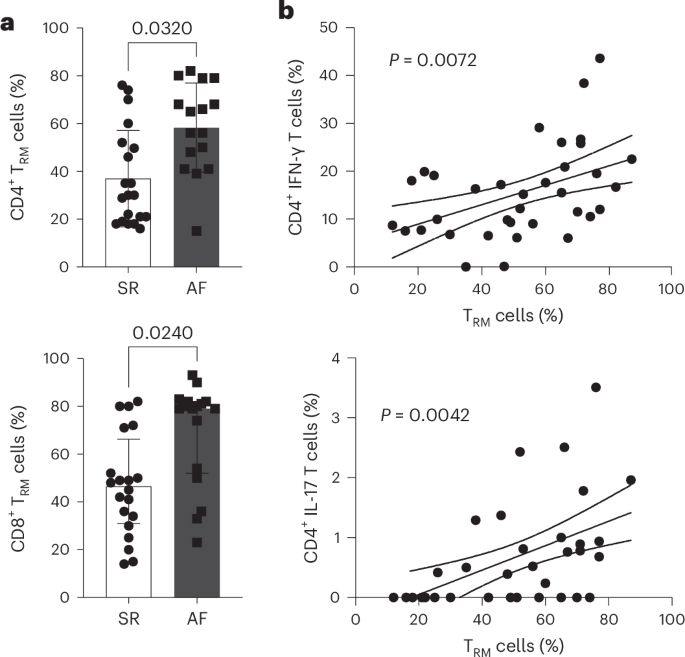
EAT immune infiltrate from patients with AF or patients in SR was characterized by flow cytometry. a , Bar graphs indicate the frequency of CD4 + and CD8 + T RM cells over total CD4 + or CD8 + CD45RO + T cells, respectively, in the EAT ( n = 26 SR and n = 18 AF biological replicates). Statistical significance was determined using unpaired two-tailed t -test for the parametrically distributed groups. Data are represented as mean ± s.d. b , Cytokine production was evaluated by intracellular staining by flow cytometry. Correlation analysis of IFN-γ and IL-17 production with the frequency of CD4 + T RM cells measured by linear regression. Graphs show 95% confidence bands ( r = 0.299) and two-tailed P value analysis. All data show individual patients ( n = 44).
EAT serves as a reservoir of T RM cells
T RM cells are transcriptionally, phenotypically and functionally distinct from other memory T cell populations. T RM cells can be identified in tissue by their expression of CD69 and a core gene signature shared between CD4 + and CD8 + T RM cells in multiple lymphoid and mucosal sites 20 . However, to establish long-term residency in different tissues, T RM cells are required to display tissue-specific transcriptional features to accommodate unique local environmental cues 21 . To confirm the identity of CD69 + PD1 + cells identified by flow cytometry, we performed CITE-seq profiling of immune cells within the EAT from two patients with AF. CITE-seq allows optimal annotation of cell populations and identification of protein isoforms, such as the canonical memory marker CD45RO, that cannot be identified by RNA sequencing (RNA-seq) alone 22 . In addition, to explore the interrelationship between T cells in the EAT and underlying myocardium, given the anatomical intimacy between these two tissues, we transcriptionally profiled paired EAT and atrial appendage (AA) samples. To improve sensitivity, given that immune cells comprise a relatively small proportion of cells in the EAT and AA, CITE-seq was performed on sorted CD45 + cells. Unsupervised clustering and uniform manifold approximation and projection (UMAP) dimensionality reduction from 28,242 cells across the two paired EAT and RAA samples yielded 19 clusters that were annotated based on the expression of data-driven marker genes (Fig. 2a ). Similar clusters could be observed in the EAT and paired AA, with a clear enrichment of adaptive immune cells, consistent with our previous work (Extended Data Fig. 3a ) 19 .
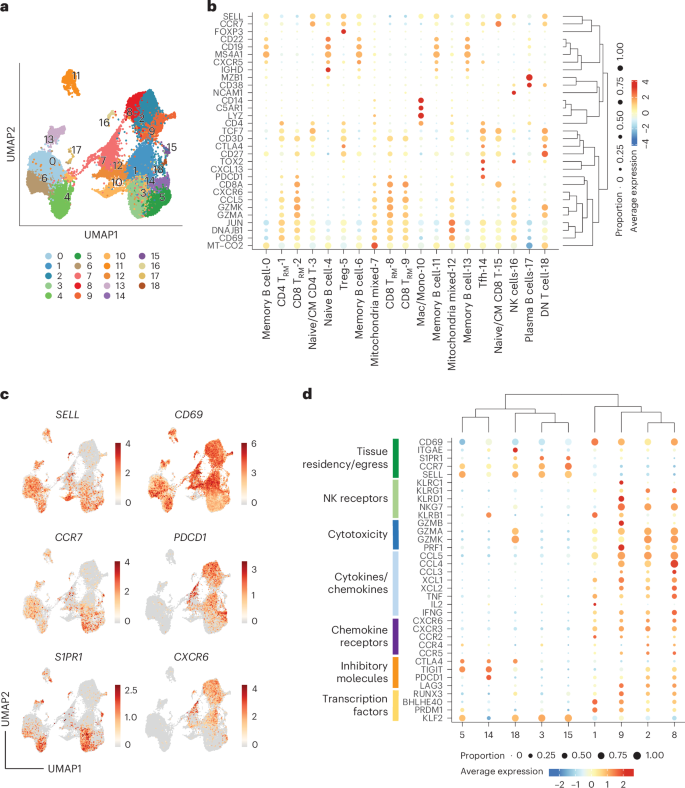
a , UMAP plots of merged EAT and AA samples identified 19 cell clusters. b , Bubble plot shows expression levels of representative markers within each cluster. c , Canonical markers used to identify T RM cells are represented in the UMAP plot. Data are colored according to average expression levels. Expression values are normalized for quantitative comparison within each dataset. d , Bubble plot showing the expression distribution of effector molecules, receptors and transcription factors among T cell populations. DN, double-negative.
Differential gene expression from the CITE-seq data resolved T cell subsets into nine clusters (Fig. 2a,b and Supplementary Table 3 ). CD4 + T cells comprised four clusters: a distinct T RM cluster expressing the canonical markers CD69 , PDCD1 and CXCR6 and low CCR7 and SELL expression (cluster 1); naive or T central memory (T CM ) cells expressing CCR7 , SELL and TCF7 (cluster 3); a cluster representing regulatory T cells (Tregs) expressing FOXP3 and CTLA4 (cluster 5); and a T follicular helper cell (Tfh) cluster characterized by the expression of CXCL13 , TOX2 , CXCR5 and PDCD1 (cluster 14). CD8 + T cells comprised four clusters that included the following: three T RM clusters expressing CCL5 , the T RM markers CD69 , PDCD1 and CXCR6 and the cytotoxic-associated genes GZMK and GZMA (clusters 2, 8 and 9); and naive or T CM cells expressing CCR7 , SELL and TCF7 (cluster 15). A small double-negative T cell cluster was identified by the expression of CD3D and absence of CD4 and CD8A expression (cluster 18). In addition, we identified five B cell clusters based on the expression of CD19 , CD22 and MS4A1 , with memory B cells expressing CD27 and naive B cells expressing IGHD ; high expression of CD38 and MZB1 defined a cluster of plasma B cells. A single cluster of monocytes/macrophages was identified based on the expression of C5AR1 , LYZ and CD14 , and a natural killer (NK) cell cluster was defined by the expression of NCAM1 . Two clusters were found to be enriched in mitochondrial and heat shock protein genes indicative of a stress-like state and were integrated by a mixed population of monocytes and T cells. Expression of surface markers detected by TotalSeq antibodies (oligonucleotide-tagged antibodies) confirmed the expression of CD45RO on memory T cells as well as PD1 and CD69 expression on T RM cells, which were also low on CCR7 (Extended Data Fig. 3b,c ).
Consistent with our flow cytometry data, T RM cells made up a sizeable proportion of the T cell repertoire, in particular for CD8 + T cell populations. As expected, they differentially express genes associated with tissue retention/egress, but they lack expression of CD103, which is normally expressed on CD8 + T RM cells at the epithelial barrier 23 (Fig. 2c,d ). T RM cells exhibit constitutively high expression of deployment-ready mRNAs encoding effector molecules, such as granzymes, cytokines and chemokines, enabling rapid immune responses (Fig. 2d ). Thus, to control their undue activation, they express the inhibitory molecules LAG3 and PDCD1 but lack the expression of CTLA4 (ref. 20 ). Thus, CITE-seq provided the necessary single-cell resolution to demonstrate a gene signature consistent with that observed in other organs, such as the lung, gut and skin 24 , and demonstrated that the elevated CD69 + PD1 + T cell population observed in patients with AF is consistent with a T RM cell phenotype with high effector cytotoxic function.
T RM cells are recruited into the atrial myocardium
The EAT is now considered an immune site harboring an array of innate and adaptive immune cells and is thought to act as a reservoir for memory T cells 25 . Due to the absence of fascial boundaries and close functional and anatomic relationship, T cells present in the EAT could migrate and exert a detrimental effect on the myocardium. To characterize the T RM cell populations between tissues, we identified differentially expressed genes (DEGs) for all T RM cell clusters (Supplementary Table 4 ). Supporting the strong immune crosstalk between tissues, T RM cells in EAT and AA showed a similar core phenotype. However, DEG analysis revealed an upregulation of activated genes—for example, JUNB , FOS , ZFP36 and IFNG —in the T RM cells present in the AA (Fig. 3a ) 26 , which could be confirmed by increased degranulation of CD8 + T RM cells (Fig. 3b ). This activated phenotype was not restricted to T RM cells but observed across immune cell clusters (Supplementary Table 4 ).
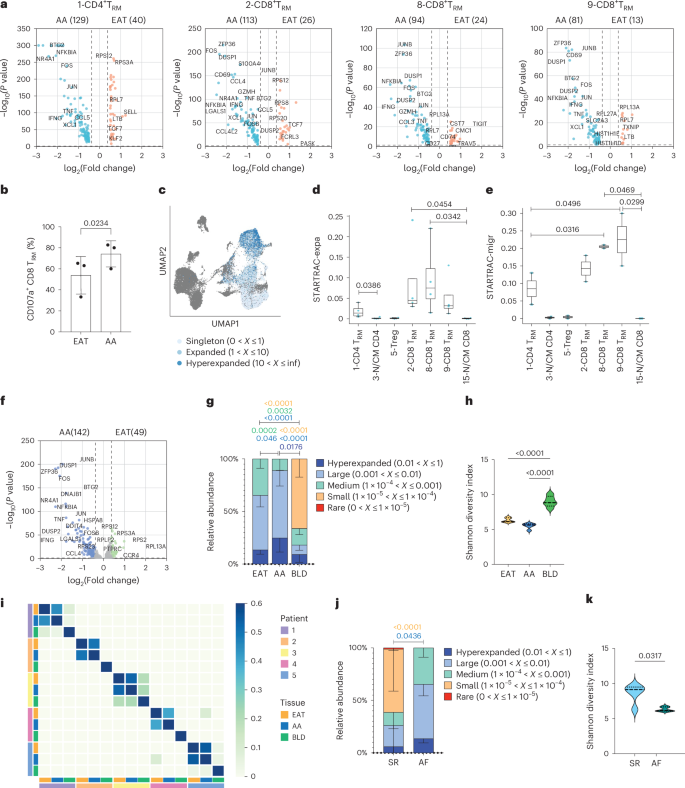
a , Volcano plots showing the average log fold changes and average Benjamini–Hochberg-corrected P values for pairwise differential expression between EAT and AA tissues for all T RM cluster populations based on the non-parametric Wilcoxon rank-sum test. b , Expression of surface CD107a was analyzed on activated CD8 + T RM cells by flow cytometry. Bar graph indicates the percentage of CD107a + CD8 + T RM cells in paired EAT and AA samples. Data are presented as mean ± s.d. Statistical significance was determined using paired two-tailed t -test ( n = 3 biological replicates). c , UMAP visualization of clonotype expansion levels among clusters. Data are colored according to clonal expansion levels. d , Clonal expansion levels of T cell clusters quantified by STARTRAC-expa indices for each sample. Statistical significance was determined using the Kruskal–Wallis test with Dunn’s multiple comparisons test ( n = 4 biological replicates) e , Migration potential of T cell clusters quantified by STARTRAC-migr indices for each patient. Statistical significance was determined using one-way ANOVA with Tukey’s multiple comparisons test ( n = 2 biological replicates). Box plots in d and e show data points from individual tissues with means and minimum/maximum values. f , Volcano plots showing the average log fold changes and average Benjamini–Hochberg-corrected P values for pairwise differential expression between hyperexpanded TCR clones in the EAT and AA tissues. g , Bar graph indicates the relative abundance of TCRα clonotypes in paired tissues EAT, AA and blood (BLD) ( n = 5 biological replicates). The relative abundance of TCRα clonotypes was calculated using the Immunarch package in R (version 1.0.0) and grouped accordingly as rare, small, medium, large and hyperexpanded. Data are presented as mean ± s.d. Statistical significance was evaluated using two-way ANOVA with Sidak’s multiple comparisons test. h , TCRα diversity between paired tissues ( n = 5 biological replicates). Statistical significance was evaluated with one-way ANOVA followed by the Tukey’s multiple comparison test. i , Heatmap illustrating the compositional TCRα similarity between paired samples assessed using the Morisita–Horn index. j , Bar graph indicates the relative abundance of TCRα clonotypes between patients with AF and patients in SR ( n = 5 biological replicates). Data are presented as mean values ± s.d. Statistical significance was evaluated using two-way ANOVA with Sidak’s multiple comparisons test. k , TCRα diversity between patients with AF and patients in SR ( n = 5 biological replicates). Statistical significance was evaluated using the two-tailed Mann–Whitney U -test for non-parametric data and represented as mean ± s.d. Panels h – k show medians, and light dotted lines show 1st and 3rd quartiles. inf, infinity.
Source data
To further evaluate the dynamic relationship of T cells among EAT and AA tissues, CITE-seq was combined with TCR α-chain and β-chain sequencing. Distinct clonotypes were assigned according to the presence of unique nucleotide sequences for both α and β chains. A distinct pattern of T cell clonal expansion could be observed, with T RM cells showing the highest degree of clonal expansion, in particular CD8 + T RM cells (Fig. 3c,d and Supplementary Table 5 ). STARTRAC-migr analysis revealed that T RM cells are associated with the highest mobility with a high degree of TCR sharing between EAT and AA (Fig. 3e ) 27 . TCR similarity was confirmed with the Morisita index (Extended Data Fig. 3d ). To understand the trajectory of these cells, we performed DEG analysis on shared expanded clones between EAT and AA. Shared expanded TCR clones in the AA upregulate expression of JUNB , FOS , IFNG , TNF and ZFP36 compared to EAT, which is consistent with T cell activation (Fig. 3f and Supplementary Table 6 ).
To confirm these findings, bulk TCRα-β sequencing was performed on matched blood, EAT and AA samples from six participants. Greater clonal expansion and lower clonotype diversity were detected in EAT and AA samples compared to blood (Fig. 3g,h and Extended Data Fig. 3e,f ). In addition, a high degree of TCR similarity could be detected between EAT and AA paired samples, whereas a relative low proportion of shared TCR clonotypes was observed between tissues and blood (Fig. 3i ), which is consistent with the tissue residency properties of T RM cells. We then looked at T cell expansion in the EAT between patients with AF and patients in SR. Supporting the observed T RM cell enrichment in patients with AF, clonal expansion was greater in the EAT of patients with AF compared to patients in SR, and diversity was reduced (Fig. 3j,k and Extended Data Fig. 3h,i ). Together, these data support the notion that EAT acts as a reservoir of T RM cells, which, upon activation, can migrate to the underlying myocardium to exert their function.
CD8 +/− T RM cells are transcriptionally diverse
Unsupervised clustering of CITE-seq data revealed a heterogeneous CD8 + T RM cell population. To further characterize the CD8 + T RM cells, DEGs between the three clusters were analyzed. Most of the DEGs were detected in cluster 9 compared to clusters 2 and 8, with clusters 2 and 8 differing only in the level of expression of effector molecules, such as CCL4 , CCL3 and IFNG (Fig. 4a,b and Supplementary Tables 7 and 8 ). Based on this, we concluded that clusters 2 and 8 are phenotypically similar populations in a different activation state. In contrary, cluster 9 appears to comprise a distinct CD8 + T RM cell population with differential expression of NK receptors and cytotoxic molecules (Fig. 4a and Supplementary Table 7 ). Two similar populations were described in human intestinal tissue, which could be differentiated by the expression of KLRG1 (refs. 28 , 29 ). With clusters 2 and 8 exhibiting a more cytotoxic/activated phenotype, we then investigated if these CD8 + T RM subsets were modulated in AF. We selected KLRG1 expression to assess CD8 + T RM heterogeneity, as this marker is more highly expressed in clusters 2 and 8 (Fig. 2d ); shows little overlap with genes expressed in cluster 9 (Fig. 4d ); and can easily distinguish two populations by flow cytometry (Fig. 4e ). We found that KLRG1 + CD8 + T RM cells were elevated in the EAT of patients with AF compared to patients in SR (Fig. 4f ). Although a causal relationship cannot be established, these results suggest that an increase in KLRG1 + CD8 + T RM cells could signal local atrial inflammatory activation in patients with AF.
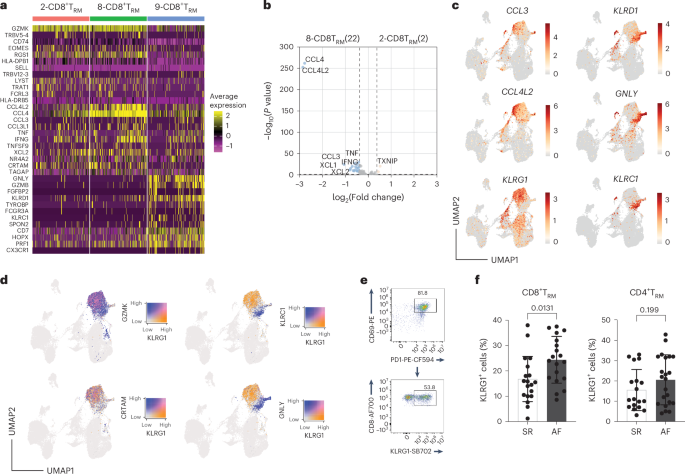
a , Heatmap shows average gene expression by curated CD8 + T RM cell populations that had a fold change greater than 2 and P < 0.05 by the binomial test for at least one of the clusters. b , Volcano plots showing the average log fold changes and average Benjamini–Hochberg-corrected P values for pairwise differential expression between CD8 + T RM cell clusters 8 and 2 based on the non-parametric Wilcoxon rank-sum test. c , UMAP of representative selected genes differentially expressed between two main CD8 + T RM cell clusters. Color bars indicate average expression. Expression values are normalized for quantitative comparison within each dataset. d , UMAP showing co-expression of selective genes differentially expressed between two main CD8 + T RM cell clusters. Color bars indicate level of overlap expression. e , Representative dot plot showing KLRG1 expression. f , Bar graphs indicate the frequency of KLRG1 + CD4 + and CD8 + T RM cells in the EAT. Each point represents an individual patient ( n = 22 SR and n = 18 AF). Statistical significance was determined using two-tailed unpaired t -test for the parametrically distributed groups. Data are represented as mean and s.d.
Regional tissue remodeling in the EAT
AF is characterized by structural remodeling of the atrial myocardium, which generally involves fibrotic changes in the atria 30 . EAT has been proposed as an important factor involved in structural and electrical remodeling, with recent work showing morphological changes in the EAT/atrial border zone 31 , 32 . Clusters of inflammatory cells were identified in the transition zone between adipocytes and fibrosis in the human atrium, with T cells being the dominant cell type 33 . The presence of tertiary lymphoid structures was not evident in our cohort (Extended Data Fig. 4a,b ). To examine biological changes and regionality dictating structural remodeling in AF, we performed NanoString GeoMx Digital Spatial Profiling (DSP) of tissue biopsies from two patients with AF and two patients in SR. The list of genes tested is shown in Supplementary Table 9 . For this regional transcriptional analysis, samples from deep in the tissue and at the EAT/AA border zone were used as shown in Extended Data Fig. 4c,d . To identify regional differences between the EAT and atrial tissue, we performed DEG analysis between regions from the same tissue. As expected, t-distributed stochastic neighbor embedding (t-SNE) and principal component analysis (PCA) showed a distinct transcriptomic profile between the tissues (Extended Data Fig. 4e ). In total, 780 genes were found to be differentially expressed between deep in the EAT compared to the border zone, with 813 genes found to be differentially expressed within regions in the atrium (Supplementary Tables 10 and 11 ). Volcano plots showing all the DEGs are shown in Fig. 5a,b . Genes associated with inflammation, including IFNG and IL17A , were upregulated in the border zone of the EAT (Fig. 5c ), whereas CD3G was found to be upregulated in the atrium border zone as well as CXCL13 and several Toll-like receptors (Fig. 5d ). Pathway analysis identified upregulation of the epithelial–mesenchymal transition, angiogenesis and inflammation pathways in both EAT and cardiomyocyte border zone (Fig. 5e,f ). A decrease in oxidative phosphorylation (OXPHOS) was detected in the EAT and AA border zone. A decline in mitochondrial OXPHOS activity was previously reported in chronic heart failure 34 .
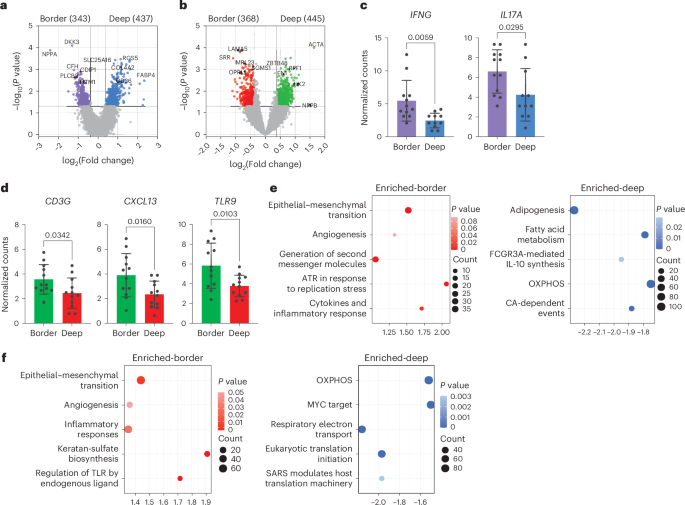
a , Volcano plot showing the average log fold changes in gene expression between border zone regions and deep in the tissue in the EAT. b , Volcano plot showing the average log fold changes in gene expression between border zone regions and deep in the tissue in the AA. a , b , Differential expression was performed using the linear mixed-effect model showing the average log fold changes and P values. c , d , Bar graph showing normalized counts of selective genes in the EAT ( c ) and AA ( d ), respectively. Statistical significance was determined using two-tailed paired t -test for parametric data, represented as mean and s.d. For normalized counts, Q3 normalization uses the top 25% of expressers to normalize across ROIs/segments e , GSEA pathway enrichment analysis of upregulated and downregulated DEGs in the EAT border zone compared to deep in the tissue. f , As in e but upregulated and dowregulated DEGs in the AA border zone compared to deep in the tissue. Pathway statistical significance was assessed using one-sided Fisher’s exact test. a – e , Assays were performed in three biological replicates in technical triplicates.
To investigate tissue remodeling, we performed a cellular deconvolution analysis. Cellular composition differs among tissues, with myeloid, lymphoid and mesothelial cells and fibroblasts being enriched in the EAT. As expected, adipocytes and atrial cardiomyocytes were exclusively present in the EAT and AA, respectively (Fig. 6a,b ). Similarly, mesothelial cells were elevated in the EAT, probably due to the mesothelial lining of the heart. The EAT hosts the cardiac autonomic nerve fibers, the ganglionated plexi and a considerable amount of endothelial progenitor cells, explaining the elevated proportion of endothelial and neuronal cells (Fig. 6a,b ). Consistent with the recognition of the adipose tissue as an immunological organ 35 , a proportion of monocytes and lymphocytes was elevated in the EAT. This was confirmed by flow cytometry (Extended Data Fig. 4f ). When investigating regional intra-tissue differences, fibroblasts were more abundant in the EAT border zone, whereas adipocytes were increased deep in the tissue, which supports previous reports on decreased adipogenesis in the border zone 31 (Fig. 6c ). No differences were observed within the AA (Extended Data Fig. 4g ).
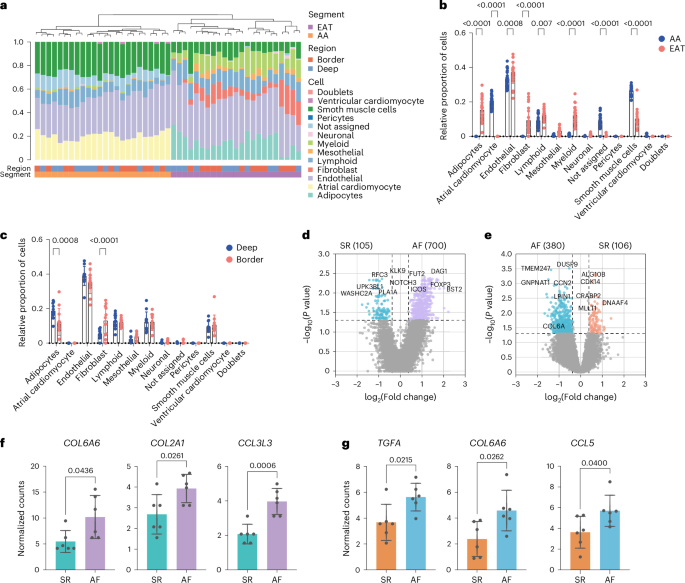
a , Proportion of cell types in the EAT and AA identified by cellular deconvolution. Each bar represents an individual ROI. b , Bar graph comparing the proportion of cell types over total cells between the EAT and AA. c , Bar graph comparing the proportion of cell types over total cells between the EAT border zone and deep in the tissue. b , c , Statistical significance was evaluated by two-way ANOVA with Sidak’s multiple comparison test. Bars represent mean ± s.d. d , Volcano plots showing the average log fold changes in gene expression in the EAT border zone between patients with AF and patients in SR. e , As in d but showing expression differences in the AA border zone between patients with AF and patients in SR. d , e , Differential expression was performed using the linear mixed-effect model showing the average log fold changes and P values. f , Bar graph showing normalized counts of selective genes in the EAT border zone between patients with AF and patients in SR. g , As in f but in the AA border zone between patients with AF and patients in SR. f , g , Statistical significance was determined using two-tailed paired t -test for parametric data, represented as mean and s.d. For normalized counts, Q3 normalization uses the top 25% of expressers to normalize across ROIs/segments. a – g , Assays were performed in three biological replicates in technical triplicates.
We then investigated differences between the border zone of patients with AF and SR controls. Cellular deconvolution analysis showed a trend toward an increase in smooth muscle cells in the EAT of patients with AF, albeit not significant ( P = 0.06) (Extended Data Fig. 3f,g ). However, DEG analysis identified 700 and 380 genes being upregulated in the EAT and AA of patients with AF, respectively (Supplementary Tables 12 and 13 ). In addition, both EAT and AA showed upregulated fibrosis-related genes in patients with AF compared to SR controls (Fig. 6d,e ). Similarly, inflammatory markers were upregulated in patients with AF (Fig. 6f,g ). CCL5, which is highly expressed by T RM cells, was upregulated in the AA border zone in AF (Fig. 6g and Extended Data Fig. 3h ). Together, these data indicate that the inflammatory response in the intersection within tissues is accompanied by secretion of pro-fibrotic factors and cellular remodeling at least in the EAT, which is more evident in patients with AF.
T RM cells can directly modulate cardiomyocyte function
To evaluate the hypothesis that T RM cells can significantly alter the electrical properties of coupled cardiomyocytes compared to non-T RM cells, we performed co-culture studies with induced pluripotent stem cell–derived cardiomyocytes (iPSC-CMs) with an atrial phenotype. Atrial cardiomyocytes were differentiated as described by Cyganek et al. 36 . Contracting cardiomyocytes could be visualized around day 8 after differentiation, with spontaneous and consistent beating cell sheets evident after further maturation (Extended Data Fig. 5a–c ). The cardiomyocyte phenotype was confirmed by transcriptomic, proteomic and electrophysiological analysis (Extended Data Fig. 5 ). Calcium is a fundamental link between the electrical activity in the heart and contractility of the cardiomyocytes. Changes in the calcium transient occur dynamically throughout the course of cardiomyocyte contraction, whereas perturbations in calcium flux are associated with arrhythmia vulnerability 37 . Co-culture of iPSC-CMs with CD4 + T RM cells isolated from EAT significantly altered calcium transient parameters at 50% (CaT 50 ) and 90% (CaT 90 ) decay in cellular calcium handling compared to co-cultures with non-T RM memory CD4 + T cells (Fig. 7a,b ). The low frequency of non-T RM memory CD8 + T cells precluded collection of sufficient cells to assay their effects on cardiomyocyte calcium handling. However, similar changes in calcium flux were observed with total CD8 + T cells (Extended Data Fig. 5d ).
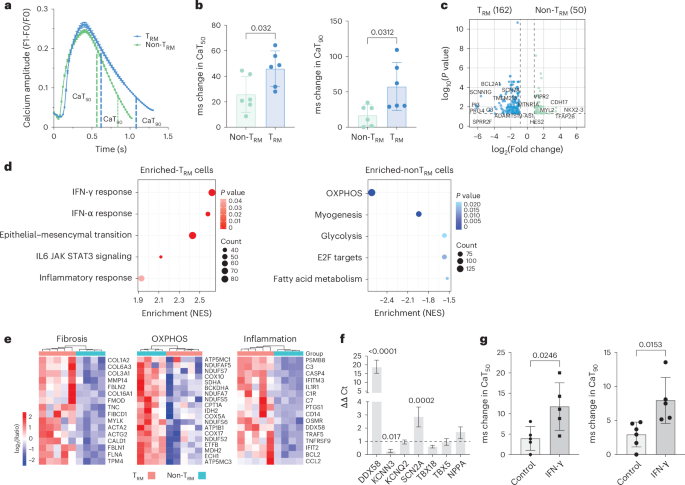
a , Graph demonstrating a typical calcium transient after addition of T cells with the key parameters CaT 50 and CaT 90 depicted ( n = 6 biological replicates). Each point represents mean ± s.e.m. b , Bar graphs demonstrating percentage change in CaT 50 and CaT 90 in CD4 + T RM and non-T RM cells ( n = 6 biological replicates). Statistical significance was assessed using two-tailed t -test for parametric data, represented as mean and s.d. c , Volcano plot demonstrating differential gene expression between the T RM and non-T RM samples. Significance threshold of P < 0.05 log 10 adjusted and log 2 fold change > 1. d , GSEA pathway enrichment analysis of upregulated and downregulated DEGs in the iPSC-CMs cultured with CD4 + T RM cells compared to non-T RM cell cultures. Pathway statistical significance was assessed using one-sided Fisher’s exact test. e , Heatmap showing expression of genes associated with fibrosis, OXPHOS and inflammation. c , e , The Wald test from the DESeq2 package was used to test significance using false discovery rate-adjusted P values. f , iPSC-CMs were cultured with recombinant 50 ng ml −1 IFN-γ for 8 h. Relative expression levels of selective genes in iPSC-CMs ( n = 6 technical replicates from three independent experiments) were analysed by RT–PCR. Expression levels were normalized to GAPDH expression. Bars represent expression in treated cells compared to untreated, which was set at 1 and indicated with dotted lines. Bars represent the mean ratio and upper and lower limits. Statistical significance was determined using unpaired two-tailed t -test. g , Bar graphs demonstrating percentage change in CaT 50 and CaT 90 in iPSC-CMs treated with IFN-γ ( n = 5 technical replicates). Statistical significance was determined using unpaired two-tailed t -test for parametric data, represented as mean and s.d. NES, normalized enrichment score.
To determine whether transcriptomic alterations accompanied changes observed in calcium handling, we performed RNA-seq analysis of iPSC-CMs isolated after co-cultures. DEG analysis revealed an upregulation of gene-encoding ion channels—for example, SCNN1 , SCN7A , SCN2A , KCNQ2 and KCNN3 —in iPSC-CMs co-cultured with CD4 + T RM cells compared to non-T RM cells, with KCNN3 and SCN2A previously associated with AF 38 , 39 (Fig. 7c and Supplementary Table 14 ). Interestingly, several extracellular matrix and collagen genes were upregulated in T RM cell co-cultures, including HAS1 , PAPLN , FMOD , COL3A1 , COL6A3 and COL1A2 . Similar collagen expression was reported in iPSC-CMs under fibrotic stiffness 40 . Genes associated with apoptosis, such as CASP4 and BCL2 , and complement activation, for example, C3 , C7 and C1R , were also upregulatef on iPSC-CM-T RM co-cultures. Gene set enrichment analysis (GSEA) identified an enrichment of genes associated with epithelial–mesenchymal transition and angiogenesis in T RM cell co-cultures, and OXPHOS, adipogenesis and fatty acid metabolism were upregulated in iPSC-CMs co-cultured with non-T RM memory CD4 + T cells, which is consistent with regional differences detected with spatial transcriptomic analysis (Fig. 7d,e and Supplementary Table 14 ). Inflammatory pathways, such IFNs and inflammatory responses, were upregulated in iPSC-CM-T RM co-cultures. This is likely a response to the enhanced production of pro-inflammatory cytokines and cytotoxic factors by T RM cells (Fig. 7d ). To test if cytokines alone could modulate iPSC-CMs, we analyzed selective gene expression changes in co-cultures performed in the presence of a 0.4-μm transwell. Expression of genes, such as NPPA and KCNN3 , was modulated by T RM in both conditions, albeit at a higher level with direct cell-to-cell contact, whereas KCNQ2 expression was upregulated only by direct contact (Extended Data Fig. 5e ). IFNG expression is upregulated in the atria of patients with AF, and plasma IFN-γ has been described as an independent risk factor for all-cause mortality in AF 41 , 42 . IFN-γ is highly produced by T RM cells, and its signaling pathway was upregulated in cardiomyocyte co-cultures. Thus, we then tested if IFN-γ alone could modulate iPSC-CM function. IFN-γ upregulates DDX58 expression, consistent with the upregulation of the IFN-γ pathway, and was sufficient to modulate ion channel expression, such as KCNN3 and SCN2A , and cellular calcium handling compared to untreated cells (Fig. 7f,g ). IFN-γ production by T cells has been shown to modulate cardiomyocyte and cardiac fibroblast gene expression 43 . Indeed, IFN-γ stimulation of cardiac fibroblasts upregulated the expression of DDR2 and collagen genes (Extended Data Fig. 5f ). Overall, our data suggest that T RM cells can promote AF by several mechanisms.
EAT has emerged as a risk factor and independent predictor of AF incidence and recurrence after ablation 44 . Inflammation has been described as a possible mechanism driving this increased risk. However, in-depth investigations of EAT immune profiling remain sparse. We and others have shown that EAT is highly enriched in adaptive immune cells, in particular T cells 19 , 45 . In the present study, we found that T RM cells, which comprise a distinct subset of memory T cells in tissue, were significantly elevated in the EAT of patients with AF. These cells showed a high degree of expansion and migration toward the atrial tissue and can directly impair cardiomyocyte calcium handling. Furthermore, a highly heterogenous spatial organization is present within atrial border zones, implying a mechanism by which EAT may cause the non-uniform conduction disturbances observed in human AF.
T RM cells have superior effector functions, including rapid chemokine/cytokine production and cytotoxicity 20 , providing local protective immune responses against pathogens. More recently, however, it was demonstrated that T RM cells can mediate autoimmunity, for example in inflammatory skin conditions, arthritis and Crohn’s disease 29 , 46 , 47 . The role of T RM cells in cardiovascular diseases, in particular AF, has not been explored. Here we demonstrated that the presence of T RM cells positively correlates with production of IL-17 and IFN-γ, both known to be implicated in AF risk 48 . Furthermore, CITE-seq analysis identified heterogeneity within the CD8 + T RM cell pool. KLRG1 expression was used to define two main CD8 + T RM populations with distinct effector functions. Interestingly, these two subsets closely resemble the ones described in the context of intestinal inflammation 28 , 49 , with KLRG1 + T RM cells identified by gene expression of GZMK , GZMH , CCL4 , CRTAM and KLRG1 , and a second population expressing CD103 was positive for CD7 , KLRB1 and CAPG expression, among others 49 . Of note, CD103 expression is limited to CD8 + T RM cells at mucosal sites and, therefore, was not expressed in T RM cells in the EAT and AA 24 . Although CD103 + T RM cell frequency has been noted in healthy intestines, KLRG1 + T RM cells showing enhanced cytotoxic and proliferative potential were elevated in Crohn’s disease 29 . These findings were later confirmed in a mouse model of intestinal infection 50 . In this model, CD103 fate mapping identified CD103 − intestinal T RM cells as the first responders to secondary infection. These cells were more frequently in contact with CD11c + dendritic cells in tissue and exhibited in situ proliferation, enhanced reactivation and effector function potential 50 . The association of KLRG1 expression with T RM cell pro-inflammatory phenotype is particularly relevant given that the proportion of KLRG1 + CD8 + T RM cells was higher in patients with AF. Additional work is required to establish a direct link between the presence of KLRG1 + CD8 + T RM cells in the tissue and AF pathogenesis, but KLRG1 + T RM cells have the potential to serve as a predictive biomarker of disease persistence and/or recurrence.
It is worth noting the constitutive high expression of PD1 on T RM cells 51 , 52 . PD1 is an inhibitory receptor, with its expression traditionally associated with T cell exhaustion. However, recent findings point toward an involvement of PD1 in restraining T RM cell activation and immunopathology. Indeed, chronic pancreatitis is associated with reduced PD1 expression, and inhibition of the PD1/PDL1 axis resulted in enhanced T RM cell-mediated functional responses 51 . Consistently, the presence of PD1 + T RM cells in tumors is associated with good prognosis and increased T cell effector capacity after anti-PD1/PDL1 immune checkpoint inhibitor (ICI) treatment 53 , 54 . However, PD1 blockade can also lead to cardiac arrhythmias in patients with cancer 49 , 55 . Moreover, the frequency of active episodes in patients with AF correlates with lower surface expression of PD1/PDL1 on peripheral CD4 + T and dendritic cells 56 . Further investigation is required to understand the risk factors and to establish a link between T RM cell activation and ICI-associated arrhythmias in these individuals.
EAT acts as a rich local depot of vasoactive molecules, cytokines and growth factors that can act on the heart or be secreted via the vaso vasorum into the coronary vessels (which supply both the EAT and myocardium) to exert its effects 12 . The EAT secretome varies considerably in physiological and pathophysiological states, with numerous lines of evidence implicating EAT inflammation as a key player in AF pathogenesis 12 , 16 . The EAT secretome applied to ex vivo atrial explants was found to induce fibrosis, a hallmark of AF, with transformation of fibroblasts into myofibroblasts and the production of a large amount of extracellular matrix components 57 . In vitro cultures with cardiomyocytes resulted in electrophysiological changes and electrical remodeling of cardiomyocytes 58 . Less is known about the direct role of immune cells and immune cell migration between tissues. Adipose tissue is increasingly recognized as an accessory immune organ contributing to immune responses. Fat-associated lymphoid clusters (FALCs) have been identified in several adipose tissue depots in mice and humans, which are not encapsulated organs, akin to tertiary lymph nodes supporting B cell responses 59 . In mice, adipose tissue has been shown to represent a memory T cell reservoir that provides rapid effector memory (EM) responses. These T cells were predominantly T RM cells that expanded in situ and were redeployed to adjacent tissues to confer protection against secondary infections 25 . Our flow cytometry and spatial transcriptomic data support this idea that EAT is an immune reservoir where myeloid and lymphoid cells are present at higher numbers than in the heart tissue. This numeric advantage can be the result of a bioenergetic rich environment provided by the EAT and/or protective mechanism to restrain the accumulation of adaptive immune cells in the heart.
EAT/AA has emerged as a hotspot of fibrosis and cellular infiltration with large amounts of collagen deposition 31 , 32 . Due to the anatomical contiguity between EAT and AA, EAT may be a key driver of the fibrotic milieu, as evidenced by the accumulation of fibroblasts and upregulation of inflammatory and extracellular matrix components in the EAT border zone, which was exacerbated in patients with AF. The absence of cellular differences within the AA was surprising, in particular the low proportion of fibroblasts compared to EAT. Notably, many key highly expressed genes are shared between the collagen-producing myofibroblast population and smooth muscle cells, such as MYH11 and ACTA2 , suggesting that these cells may have not been adequately distinguished by the deconvolution algorithm. In addition, the deconvolution analysis did not allow for the identification of lymphoid cell subsets. The regional differences could be explained, at least in part, by inter-tissue and intra-tissue T RM cell migration, supported by the dynamic relationship observed among T RM TCR clones. T cell expansion was predominately detected among T RM cells showing a high degree of shared clonality, highlighting the local immune crosstalk between the overlying adipose tissue (EAT) and the myocardium (AA). The finding that T RM cells in general, and TCR-expanded clones in particular, have a more activated phenotype in the AA compared to EAT suggests a migration process from the reservoir in the EAT toward the effector site in the AA. Similar findings have recently been described in the context of heart failure 60 . High shared clonality could be detected between the EAT and the heart, with T cells having a more activated phenotype in these patients. This is no surprise given that AF and heart failure have common pathophysiological mechanisms 61 , with EAT dysfunction and inflammation thought to play an instrumental role in both disease processes.
Relative to patients in SR, the number of T cells has been shown to be elevated in the atrial tissue of patients with AF 62 , with an early study highlighting an accumulation of CD8 + T cells at the EAT/AA tissue border zone 33 . However, how T cells can directly impair atrial conduction and function is not established. T cells are key mediators of tissue inflammation, which can alter atrial electrophysiology. Inflammatory cytokines, such as TNFα, IL-1β and IL-17, can markedly enhance the risk of arrhythmic events by directly promoting electrical and structural cardiac remodeling 48 . In addition, IFN-γ has been shown to exert a sustained inhibitory effect on cardiac L-type calcium channels 63 and to induce a metabolic shift in cardiomyocytes with downregulation of OXPHOS, which is consistent with the failing heart 43 . We showed that CD4 + T RM cells were able to uniquely alter the calcium handling properties of atrial cardiomyocytes by inducing electrical and structural changes as evidenced by gene expression changes in ion channels, calcium signaling, tracellular matrix and collagen. This is consistent with the high production of pro-inflammatory cytokines by T RM cells—for example, TNF-α and IFN-γ—although a direct cell-to-cell contact, for example by NK receptor binding, cannot be ruled out. T RM cells also express a cluster of chemokines and chemokine receptors, with CCL5 emerging as a possible mediator of tissue inflammation. CCL5 mediates trafficking and homing of T cells and innate cells to sites of inflammation. CCL5 is mainly expressed by T cells and monocytes, although CITE-seq analysis identified T RM cells as the main producers of CCL5 in the EAT and AA, with CCL5 + cells in the border zone showing a clear lymphocyte morphology. Blocking of CCL5 significantly reduced infarct size in mouse models of heart failure and was identified as a key inflammatory mediator in the EAT of patients with heart failure 60 , 64 . Furthermore, production of CCL5 by T RM cells is thought to be responsible for arthritis flares by promoting the recruitment of T EM cells to the joint 47 .
An important limitation of this study is that, due to the nature of the tissue analyzed, samples were obtained from patients with heart conditions that may themselves alter the physiology of the EAT and AA. Although the presented data provide evidence of a strong association between T RM cell-induced inflammation and increased susceptibility to AF, a causative relationship was only partially confirmed by in vitro iPSC cultures. A limitation of the iPSC-CM system is that T cell exposure to self-antigens that may be present in patients with AF is limited. In addition, it is likely that the effect of T RM cells in cardiomyocytes was underestimated, as the outcome of a positive feedback loop effect—for example, recruitment of innate and adaptive immune cells to the site of inflammation—can be assessed only in the presence of a full immune system.
Study population and sample collection
All patients provided written informed consent for their participation in the study as per local research procedures and Good Clinical Practice guidance (Research Ethics Committee reference: 14/EE/0007). Adult (≥18 years) patients undergoing on-pump open chest coronary artery bypass grafting (CABG) surgery and/or valve reconstruction (VR) surgery were recruited from Barts Heart Centre, St. Bartholomew’s Hospital, in London, United Kingdom (UK), via the Barts BioResource. Exclusion criteria included congenital heart disease; underlying cardiomyopathies or ion channelopathies; primarily undergoing other cardiac surgical procedures (for example, aortic surgery); off-pump CABG surgery; patients with active endocarditis, myocarditis or pericarditis; patients with pre-existing inflammatory diseases (for example, rheumatoid arthritis); active malignancy; patients on immunomodulatory or biologic drugs (for example, tacrolimus and anti-TNF-α agents); perioperative rhythm control therapies (for example, use of amiodarone); postoperative hemodynamic shock; uncorrected potassium derangement (K < 3.3 or K > 5.8); and uncorrected magnesium derangement (Mg < 0.5 or Mg > 1.5) detected on laboratory blood sample analysis. Fasting venous blood samples were collected preoperatively in the anesthetic room. Approximately 0.8–1 g of adipose tissue samples was collected in ice-cold PBS with 2% FBS. SAT was collected immediately after the median sternotomy incision, and EAT was obtained after opening up of the pericardial sac. AA tissue was obtained after insertion of the right atrial cannula as part of transitioning patients on to cardio-pulmonary bypass, and, typically, 0.1–0.5 g of AA tissue was harvested.
Sample processing
Adipose tissue samples were processed as previously described 65 . AA samples were enzymatically digested with 675 U collagenase I (Sigma-Aldrich), 187.5 U collagenase XI (Sigma-Aldrich) and 10 U DNase (Sigma-Aldrich) in 1 ml of HBSS modified with 10 mM HEPES but without phenol red (STEMCELL Technologies) per gram of tissue. The cell–enzyme suspension was incubated at 37 °C with 225-r.p.m. agitation for 45 min. Then, 5 ml of fasting venous blood samples was collected in EDTA tubes (BD Biosciences) preoperatively in the anesthetic room. Peripheral blood mononuclear cells (PBMCs) were isolated using Ficoll-Paque PLUS (Cytiva) as per the manufacturer’s instructions. Single-cell suspensions were obtained after centrifugation and red cell lysing before antibody staining.
Flow cytometry
Immune cells from blood, adipose tissue and AA tissue were isolated as described previously. Immune cells were stained with fixable Aqua Live/Dead cell stain (Invitrogen) diluted 1:1,000 and fluorochrome-conjugated antibodies specific for CD197-FITC, CD19-PerCP-Cy5.5, CD45RO-BV421, CD335-BV605, CD45-BV785, CD127-APC, CD8-AF700, CD3-APC/Cy7, CD69-PE, CD4-PE/Cy7, PD1-PE-CF594, CD303-FITC, CD123-PerCP/Cy5.5, CD206-BV421, CD3-BV605, CD19-BV605, CD14-APC, CD16-AF700, CD1c-APC/Cy7, Clec9A-PE, CD1a-PE-CF594 and CD141-PE/Cy7 from BioLegend and KLRG1-SB702 from eBioscience. The samples were stained at 4 °C for 18 min and then washed twice with fluorescence-activated cell sorting (FACS) buffer where the plate was centrifuged at 400 g for 3 min and the supernatant discarded. The samples were then fixed in stabilizing fixative buffer (BD Biosciences) containing 3% paraformaldehyde at 4 °C for 30 min.
For intracellular cytokine staining, samples were resuspended in 500 μl of Aim V medium with the addition of 1 μl of Cell Activation Cocktail (BioLegend). After 4-h incubation, samples were washed and stained for surface markers as detailed above, followed by permeabilization and fixation in the permeabilization/fixation buffer (BD Biosciences) at 4 °C for 12 min. Intracellular cytokine production was evaluated by incubation for 15 min at 4 °C with fluorochrome-conjugated antibodies specific for IFN-γ-APC, IL-17-APC/Cy7 and IL-22-PE (BioLegend). Data were acquired on a CytoFLEX (Beckman Coulter) and analyzed using FlowJo version 10 software.
CITE-seq and single-cell TCR sequencing
Paired AA and EAT samples were collected from two patients with AF (1× VR and 1× CABG) and digested as outlined above. The CITE-seq samples were prepared following the steps outlined in the 10x Genomics Cell Surface Protein Labeling for Single Cell RNA Sequencing protocols with the Feature Barcode technology protocol preparation guide (document CG000149). In brief, samples were resuspended in 50 μl of PBS + 1% BSA and 5 μl of human TruStain FcX and incubated for 10 min at 4 °C. Fixable Aqua Live/Dead cell stain (Invitrogen) and CD45 PE antibodies (BioLegend) and TotalSeq antibodies were resuspended in PBS and added to the sample suspension to a create a total sample volume of 155 μl. The following TotalSeq antibodies (BioLegend) were employed: C0138 anti-human CD5, C0358 anti-human CD163, C0160 anti-human CD1c, C0049 anti-human CD3, C0072 anti-human CD4, C0080 anti-human CD8a, C0087 anti-human CD45RO, C0148 anti-human CD197, C0146 anti-human CD69, C0088 anti-human CD279 and C1046 anti-human CD88. Samples were then incubated at 4 °C for 30 min in the dark. After washing, samples were incubated for 15 min with MojoSort human anti-PE Nanobeads followed by magnetic purification as per the manufacturer’s instructions (BioLegend) for CD45 + enrichement. Live CD45 + cells were further purified by FACS with an LSRFortessa analyzer (BD Biosciences).
The 10x Genomics CITE-seq
Chromium Next GEM Single Cell 5′ v2 (dual index) with Feature Barcode technology for Cell Surface Protein & Antigen Specificity User Guide (CG000330) was employed at the UCL Single-Cell Sequencing facility. In brief, the cell suspension was partitioned into a nanoliter-scale droplet emulsion using the 10x Genomics Chromium Single Cell Controller with RNA-seq libraires created using the Chromium Next GEM Single Cell 5′ Reagent Kits and a Gel Bead Kit v2. Gel beads in emulsion (GEM) were generated by combining the barcoded Single Cell VDL 5′ gel beads with a master mix containing the cell surface protein-labeled cells and partitioning oil onto a Chromium Next GEM chip. The gel beads were dissolved, and the cells were lysed. After reverse transcription, barcoded full-length cDNA was generated from the poly-adenylated mRNA. Silane magnetic beads (Dynabeads MyOne SILANE) were used to purify the 10x barcoded cDNA. Libraries were then sequenced in-house at the UCL Single-Cell Sequencing facility on an Illumina NextSeq 500/550 sequencing platform.
CITE-seq data processing
CITE-seq data processing was performed at the UCL City of London Centre Single-Cell Sequencing core. In brief, output from the Chromium Single Cell 5′ v2 sequencing was processed using Cell Ranger (version 6.0.1) analysis pipelines. FASTQ files were generated using Cell Ranger mkfastq (version 6.0.1). Gene expression reads were aligned to the human reference genome GRCh38 and counted using Cell Ranger count (version 6.01.) VDJ reads were aligned to the GRCh38 VDJ reference dataset using Cell Ranger vdj (version 6.01). 10x feature barcoding was performed using the antibodies outlined above, the reads for which were counted using Cell Ranger count.
Expression matrices were analyzed using the Seurat package (version 4.0.03) in R. Cells with mitochondrial reads making up more than 10% of the total read count, or with fewer than 400 genes detected, were removed. A multiplet filtering step using DoubletFinder (version 2.0.3) was performed using the author-recommended settings. Normalization was performed on the dataset using SCTransform (version 0.3.2) using centered log-ratio normalization and the top 3,000 variable genes. This was followed by Seurat (version 4.0.3) integration to remove batch effects, and the top 3,000 genes minus TCR genes were used as integration features. PCA and UMAP dimensionality reduction (dims 1:30) was performed using RunPCA and RunUMAP from the single-cell RNA-seq data only. Clustering was performed using the FindClusters function in the Seurat package using a resolution of 0.8. All differential gene expression analysis was carried out on log-normalized gene expression values using the Seurat NormalizeData function with default parameters through the MAST algorithm within FindMarkers. Feature barcoding reads were normalized using a centered log-ratio transformation. VDJ data were integrated using strict clone calling—matching VDJC gene and TRA/TRB nucleotide sequences. Analysis was performed using scRepertoire (version 1.3.5) and STARTRAC (version 0.1.0).
Bulk TCR sequencing
Bulk TCR α and β sequencing was performed from paired blood AA and EAT samples from five patients with AF. In addition, TCR sequencing was performed using additional EAT from five patients in SR. cDNA was extracted from tissues and whole blood. A quantitative experimental and computational TCR sequencing pipeline was employed as previously described 66 . The pipeline introduces unique molecular identifiers (UMIs) attached to individual cDNA TCR molecules, allowing correction for PCR and sequencing errors. TCR identification, error correction and CDR3 extraction were performed following a suite of tools available at https://github.com/innate2adaptive/Decombinator , as detailed in Peacock et al. 66 . TCR frequency and similarity were analyzed using the Immunarch package in R (version 1.0.0). The level of similarity between the different TCR repertoires was measured using the Morisita–Horn index, ranging from 0 (no similarity) to 1 (identical), which takes into account the shared sequences and clonal frequency between samples. Antigen matching analysis was performed via the McPAC-TCR database.
Spatial transcriptomics
Spatial profiling was carried out by NanoString Technologies using a GeoMx DSP. AA tissue with surrounding EAT samples was selected from four patients, of which two were from patients with AF and two were from patients in SR. The technology is based on the principle of in situ hybridization. After dewaxing of the formalin-fixed, paraffin-embedded (FFPE) slides, Tris-EDTA buffer was added to expose the RNA targets, and a proteinase K digestion was performed to remove any protein bound to RNA. The tissue was incubated overnight at 37 °C with GeoMx RNA detection probes. Labeled antibodies, FABP4 for adipocytes and troponin for cardiac tissue were added to image the tissue. The GeoMx DSP uses an automated microscope to image the tissue sections and cleave/collect the photocleavable indexing oligonucleotides. Specific regions of interest (ROIs) were then pre-selected on either side of the border zones of the AA/EAT and deep in the AA and deep in the EAT in triplicates. ROIs were quantified using RNA-seq technology on the Illumina platform to generate RNA expression data within a spatial context.
Spatial transcriptomics data processing
Data were analyzed with the GeoMx DSP Analysis Suite. The first quality control step looks at the ‘raw read threshold’ flagging segments with fewer than 1,000 raw reads. A second step assesses the percentage of aligned reads. The sequenced barcodes should match the known GeoMx library of barcodes; hence, a high percentage alignment should be expected (an alignment threshold value less than 80% is typically used to flag segments). Sequencing saturation was assessed as 1 − (aligned reads/de-duplicated reads). A value of less than 50% sequencing saturation was used to flag segments. A background quality control step was additionally performed based on negative probes. A no-template control was included in the first well of each collection plate with the negative control in PCR reading very low counts. The Grubbs outlier test is performed to exclude a probe from all segments if the probe is higher or lower in more than 20% of segments. A limit of quantitation value is also defined where a target is considered to be detected with high confidence, the default setting here being 2 standard deviations above the geometric mean of the negative probes. Filtering was performed to further refine the dataset, followed by Q3 normalization.
For spatial deconvolution, the SpatialDecon plugin in R version 1.2 (NanoString Technologies) was employed. The human heart benchmarking cell matrix used for the alignment of the GeoMx spatial gene expression data was extracted from the single cell data of the human heart (heartcellatlas.org). The deconvolution script with the adjusted cell matrix was run on the GeoMx DSP Analysis Suite applied to the normalized spatial gene expression data.
iPSC atrial cardiomyocyte and cardiac fibroblast differentiation
iPSC lines were acquired from the Human Induced Pluripotent Stem Cell Initiative (HipSci) deposited by the Wellcome Trust Sanger Institute into the Culture Collections archive (UK Health Security Agency). Each cell line was resuscitated as per the HipSci guidance ( https://www.culturecollections.org.uk/media/109442/general-guidelines-for-handling-hipsci-ipscs.pdf ) in TeSR-E8 media (STEMCELL Technologies). Plates were coated with a vitronectin coating (Thermo Fisher Scientific) to provide an appropiate adhesive surface for culturing iPSCs. Rho kinase (ROCK) inhibitor RevitaCell (Thermo Fisher Scientific) was added to TeSR-E8 medium to achieve a final concentration of 10 μM (1:1,000 dilution from the stock solution). Cells were plated in 2 ml of TeSR-E8 RevitaCell media per well in a six-well plate. iPSC media were replaced daily with fresh TeSR-E8. Cells were typically split at a ratio of 1:4 every 6–7 d, following HipSci guidance. iPSC-CM derivation was carried out as per Cygnaek et al. 36 . The base cardiomyocyte differentiation medium (CDM) used was RPMI 1640 with HEPES and GlutaMAX (Thermo Fisher Scientific) supplemented with 0.2 mg ml −1 ascorbic acid and 0.5 mg ml −1 albumin. Cells were sequentially treated with 4 μM CHIR99021 (Sigma-Aldrich) for 48 h, followed by 5 μM IWP2 (Sigma-Aldrich) for 48 h to induce a cardiac cell lineage. To drive differentiation toward an atrial cell phenotype, the cells were administered 1 μM retinoic acid at days 3–6. At day 6, simple CDM was added. Monolayers of beating iPSC-CMs were typically observed at days 7–8 onwards. The cells were maintained in RPMI 1640 + HEPES + GlutaMAX with the addition of 2% B27 supplement (Thermo Fisher Scientific).
For differentiation of cardiac fibroblasts, iPSCs were cultured in CDM with CHIR99021 for 1 d to induce a cardiac cell lineage as described above. After day 1, the medium was changed to CDM and cultured for an additional 24 h. After day 2, the medium was changed to a cardiac fibroblast-based medium (CFBM) comprising DMEM, 500 μg ml −1 albumin, 0.6 μM linoleic acid, 0.6 μg ml −1 lecithin, 50 μg ml −1 ascorbic acid, GlutaMAX, 1 μg ml −1 hydrocortisone hemisuccinate and 5 μg ml −1 insulin. CFBM was supplemented with 75 ng ml −1 bFGF, and media were replaced every other day until day 20 of differentiation.
iPSC atrial cardiomyocyte calcium imaging assays
The atrial cardiomyocytes were differentiated on a 48-well plate setup (Corning). Only wells where there was spontaneous and consistent beating of the cardiomyocytes across the well were imaged. The rate of spontaneous beating was not controlled, as this would require pacing the monolayer of the sheets. However, the percentage change in the decay time was calculated for each well before and after co-culture with T RM and non-T RM cells. Given that this was a percentage change for each well, taking into account the same cardiomyocyte well density and beating characteristics, different wells could be compared by determining the percentage change in decay time for each well.
For T RM cell purification, cells were initially stained with fixable Aqua Live/Dead cell stain, CD45 PE, CD45RO, CD8-AF700, CD3-APC/Cy7, CD69-PE, CD4-PE/Cy7 and PD1-PE-CF594 as previously discussed. Samples were then incubated at 4 °C for 20 min in the dark, after which samples were incubated for 15 min with MojoSort human anti-PE Nanobeads, followed by magnetic purification as per the manufacturer’s instructions (BioLegend) for CD45 + enrichement. Live T RM cells were purified by FACS (LSRFortessa analyzer) and CD45RO + CD69 + PD1 + cells. Non-T RM cells were purified as CD45RO + CD69 − PD1 − cells. Cells were added to cardiomyocytes at 2 × 10 3 per well.
Calcium imaging was performed using the calcium indicator dye Fluo-4 AM (Invitrogen). Monolayers were illuminated with a single green light from a high-power LED with a center at wavelengths of 505 nm filtered with a bandpass excitation filter (490–510 nm). The LED power supply was custom built by Cairn Research. A Hamamatsu ORCA Flash 4.0 V2 camera was connected to a Nikon Eclipse TE200 inverted microscope, and a camera magnification objective of ×10 (numerical aperture 0.3) was used to provide a broad field of view. A bandpass emission filter (520–550 nm) was used to ensure that fluorescence emitted only after calcium indicator excitation was detected. HCImageLive (Hamamatsu) imaging software was used to acquire the data. The recording was then analyzed using custom in-house software (Queen Mary University of London). A total recording time of 20 s was used with a frame rate of 50 frames per second (image acquisition every 20 ms) to produce an image stack of 1,000 frames. Images were cropped to an area of 600 × 600 pixels that contained the projected image, and pixel binning was performed to improve signal-to-noise ratio. The recording was analyzed to drive a signal average calcium waveform from across the entire field of view. Relevant waveform statistics, including time to peak, time to 50% recovery to baseline (T 50 ) and time to 90% recovery to baseline (T 90 ), were determined. The data were exported as a CSV file for analysis in GraphPad Prism. Comparisons between different experimental conditions were, therefore, possible in a consistent and reproducible fashion, enabling assessment of how cardiomyocyte calcium flux changed before and after T RM co-cultures.
iPSC co-culture RNA expression analysis
Cells were washed with PBS, and RNA was extracted with an RNeasy Micro Kit (Qiagen) following the manufacturer’s instructions. Illumina sequencing was carried out at Novogene Bioinformatics Technology Co., Ltd. Raw FASTQ files were first trimmed using Trim Galore (version 0.6.7) and inspected for quality control using fastqc (version 0.11.9) and multiqc (version 1.12). Transcript and genome files were downloaded from GENCODE, release 43 (GRCh38.p13), to generate decoy index files with Salmon (version 1.10.1). Next, Salmon was used to perform transcript quantification with the additional parameter –gcbias. Salmon transcript quantifications were imported into R (version 4.1.0) to aggregate transcripts to genes using the package tximport (version 1.22.0). Genes with a read count of less than 5 were excluded from further analysis: rowSums(counts()) ≥ 5. Differential gene analysis was performed using DESeq2 (version 1.34.0). GSEA (version 4.3.2) was performed using normalized gene counts generated by DESeq2.
For RT–PCR, reverse transcription to cDNA was performed using High-Capacity RNA-to-cDNA kits (Applied Biosystems, Thermo Fisher Scientific). The relevant primer sequences can be found in Supplementary Table 15 (Thermo Fisher Scientific). Gene expression was performed using SYBR Green Supermix (Bio-Rad), as per the manufacturer’s instructions, and analyzed using the Light Cycler System (Roche). Relative gene expression values were determined using the ΔΔCT method and normalized to a stable reference housekeeping gene control (GAPDH). The control values were set at 1. Given that the ΔΔCT method is not normally distributed, the geometric mean was used for the representation of the data.
Statistical analysis
Statistical significance was determined for continuous variables where two groups were assessed using two-tailed Student’s t -test where the data were parametric and Mann–Whitney U -test for non-parametric data. The χ 2 test or Fisher’s exact test was used for categorical data. Data were analyzed using GraphPad Prism version 8. Normality was assessed using the Kolmogorov–Smirnov and Shapiro–Wilk tests. Where parametric data are represented, the mean and standard deviation values are reported, and, for non-parametric data, median and interquartile ranges are reported. A P value of less than 0.05 was considered statistically significant.
Reporting summary
Further information on research design is available in the Nature Portfolio Reporting Summary linked to this article.
Data availability
CITE-seq and RNA-seq raw and processed data are deposited in the Gene Expression Omnibus (GEO) under accession number GSE263154 . Cell Ranger version 6.0.1 was used with default parameters to map all the data from the samples to the human reference genome (GRCh38; https://www.ncbi.nlm.nih.gov/datasets/genome/GCF_000001405.26/ ). Bulk TCR sequencing data are available at Zenodo ( https://doi.org/10.5281/zenodo.13318819 ) (ref. 67 ). The suite of tools for TCR sequencing analysis can be accessed at https://github.com/innate2adaptive/Decombinator . Spatial transcriptomic raw sequencing data have been deposited in the GEO under accession number GSE261363 . Spatial profiling was carried out using the NanoString Technologies GeoMx Digital Spatial Profiler. iPSC-CM RNA-seq datasets have been deposited in the GEO under accession number GSE256520 . Additional data generated in this study are provided in the Supplementary Information and Source Data sections.
Wijesurendra, R. S. & Casadei, B. Mechanisms of atrial fibrillation. Heart 105 , 1860–1867 (2019).
Article CAS PubMed Google Scholar
Wang, T. J. et al. Temporal relations of atrial fibrillation and congestive heart failure and their joint influence on mortality: the Framingham Heart Study. Circulation 107 , 2920–2925 (2003).
Article PubMed Google Scholar
Wolf, P. A., Abbott, R. D. & Kannel, W. B. Atrial fibrillation as an independent risk factor for stroke: the Framingham Study. Stroke 22 , 983–988 (1991).
Burdett, P. & Lip, G. Y. H. Atrial fibrillation in the UK: predicting costs of an emerging epidemic recognizing and forecasting the cost drivers of atrial fibrillation-related costs. Eur. Heart J. Qual. Care. Clin. Outcomes 8 , 187–194 (2022).
Zhou, X. & Dudley, S. C. Evidence for inflammation as a driver of atrial fibrillation. Front. Cardiovasc. Med. 7 , 62 (2020).
Article CAS PubMed PubMed Central Google Scholar
Anderson, J. L. et al. Frequency of elevation of C-reactive protein in atrial fibrillation. Am. J. Cardiol. 94 , 1255–1259 (2004).
Marcus, G. M. et al. Interleukin-6 and atrial fibrillation in patients with coronary artery disease: data from the Heart and Soul Study. Am. Heart J. 155 , 303–309 (2008).
Chen, M. C. et al. Increased inflammatory cell infiltration in the atrial myocardium of patients with atrial fibrillation. Am. J. Cardiol. 102 , 861–865 (2008).
Yamashita, T. et al. Recruitment of immune cells across atrial endocardium in human atrial fibrillation. Circ. J. 74 , 262–270 (2010).
Qu, Y. C. et al. Activated nuclear factor-κB and increased tumor necrosis factor-α in atrial tissue of atrial fibrillation. Scand. Cardiovasc. J. 43 , 292–297 (2009).
Ihara, K. & Sasano, T. Role of inflammation in the pathogenesis of atrial fibrillation. Front. Physiol. 13 , 862164 (2022).
Article PubMed PubMed Central Google Scholar
Mazurek, T. et al. Human epicardial adipose tissue is a source of inflammatory mediators. Circulation 108 , 2460–2466 (2003).
Vyas, V., Hunter, R. J., Longhi, M. P. & Finlay, M. C. Inflammation and adiposity: new frontiers in atrial fibrillation. Europace 22 , 1609–1618 (2020).
Nakamori, S., Nezafat, M., Ngo, L. H., Manning, W. J. & Nezafat, R. Left atrial epicardial fat volume is associated with atrial fibrillation: a prospective cardiovascular magnetic resonance 3D Dixon study. J. Am. Heart Assoc. 7 , e008232 (2018).
Yorgun, H. et al. Association of epicardial and peri-atrial adiposity with the presence and severity of non-valvular atrial fibrillation. Int. J. Cardiovasc. Imaging 31 , 649–657 (2015).
Mazurek, T. et al. Relation of proinflammatory activity of epicardial adipose tissue to the occurrence of atrial fibrillation. Am. J. Cardiol. 113 , 1505–1508 (2014).
Xie, B., Chen, B. X., Wu, J. Y., Liu, X. & Yang, M. F. Factors relevant to atrial 18 F-fluorodeoxyglucose uptake in atrial fibrillation. J. Nucl. Cardiol. 27 , 1501–1512 (2020).
Sanfilippo, A. J. et al. Atrial enlargement as a consequence of atrial fibrillation. A prospective echocardiographic study. Circulation 82 , 792–797 (1990).
Vyas, V. et al. Obesity and diabetes rather than coronary disease per se are major risk factors for epicardial adipose tissue inflammation. JCI Insight 6 , e145495 (2021).
Kumar, B. V. et al. Human tissue-resident memory T cells are defined by core transcriptional and functional signatures in lymphoid and mucosal sites. Cell Rep. 20 , 2921–2934 (2017).
Liu, Y., Ma, C. & Zhang, N. Tissue-specific control of tissue-resident memory T cells. Crit. Rev. Immunol. 38 , 79–103 (2018).
Stoeckius, M. et al. Simultaneous epitope and transcriptome measurement in single cells. Nat. Methods 14 , 865–868 (2017).
Barros, L., Ferreira, C. & Veldhoen, M. The fellowship of regulatory and tissue-resident memory cells. Mucosal Immunol. 15 , 64–73 (2022).
Szabo, P. A., Miron, M. & Farber, D. L. Location, location, location: tissue resident memory T cells in mice and humans. Sci. Immunol. 4 , eaas9673 (2019).
Han, S.-J. et al. White adipose tissue is a reservoir for memory T cells and promotes protective memory responses to infection. Immunity 47 , 1154–1168 (2017).
Jurgens, A. P., Popović, B. & Wolkers, M. C. T cells at work: how post-transcriptional mechanisms control T cell homeostasis and activation. Eur. J. Immunol. 51 , 2178–2187 (2021).
Zhang, L. et al. Lineage tracking reveals dynamic relationships of T cells in colorectal cancer. Nature 564 , 268–272 (2018).
FitzPatrick, M. E. B. et al. Human intestinal tissue-resident memory T cells comprise transcriptionally and functionally distinct subsets. Cell Rep. 34 , 108661 (2021).
Bottois, H. et al. KLRG1 and CD103 expressions define distinct intestinal tissue-resident memory CD8 T cell subsets modulated in Crohn’s disease. Front. Immunol. 11 , 896 (2020).
Corradi, D., Callegari, S., Maestri, R., Benussi, S. & Alfieri, O. Structural remodeling in atrial fibrillation. Nat. Clin. Pract. Cardiovasc. Med. 5 , 782–796 (2008).
Ishii, Y. et al. Detection of fibrotic remodeling of epicardial adipose tissue in patients with atrial fibrillation: imaging approach based on histological observation. Heart Rhythm O2 2 , 311–323 (2021).
van den Berg, N. W. E. et al. Epicardial and endothelial cell activation concurs with extracellular matrix remodeling in atrial fibrillation. Clin. Transl. Med. 11 , e558 (2021).
Haemers, P. et al. Atrial fibrillation is associated with the fibrotic remodelling of adipose tissue in the subepicardium of human and sheep atria. Eur. Heart J. 38 , 53–61 (2017).
Zhou, B. & Tian, R. Mitochondrial dysfunction in pathophysiology of heart failure. J. Clin. Invest. 128 , 3716–3726 (2018).
Grant, R. W. & Dixit, V. D. Adipose tissue as an immunological organ. Obesity 23 , 512–518 (2015).
Cyganek, L. et al. Deep phenotyping of human induced pluripotent stem cell-derived atrial and ventricular cardiomyocytes. JCI Insight 3 , e99941 (2018).
Sobie, E. A., Song, L. S. & Lederer, W. J. Restitution of Ca 2+ release and vulnerability to arrhythmias. J. Cardiovasc. Electrophysiol. 17 , S64–S70 (2006).
Kim, J. A., Chelu, M. G. & Li, N. Genetics of atrial fibrillation. Curr. Opin. Cardiol. 36 , 281–287 (2021).
Tzialla, C. et al. SCN2A and arrhythmia: a potential correlation? A case report and literature review. Eur. J. Med. Genet. 65 , 104639 (2022).
Heras-Bautista, C. O. et al. Cardiomyocytes facing fibrotic conditions re-express extracellular matrix transcripts. Acta Biomater. 89 , 180–192 (2019).
Zeemering, S. et al. Atrial fibrillation in the presence and absence of heart failure enhances expression of genes involved in cardiomyocyte structure, conduction properties, fibrosis, inflammation, and endothelial dysfunction. Heart Rhythm 19 , 2115–2124 (2022).
Huang, J. et al. Plasma level of interferon-γ predicts the prognosis in patients with new-onset atrial fibrillation. Heart Lung Circ. 29 , e168–e176 (2020).
Ashour, D. et al. An interferon gamma response signature links myocardial aging and immunosenescence. Cardiovasc. Res. 119 , 2458–2468 (2023).
Iacobellis, G. Epicardial adipose tissue in contemporary cardiology. Nat. Rev. Cardiol. 19 , 593–606 (2022).
Lenz, M., Arts, I. C. W., Peeters, R. L. M., de Kok, T. M. & Ertaylan, G. Adipose tissue in health and disease through the lens of its building blocks. Sci. Rep. 10 , 10433 (2020).
Ryan, G. E., Harris, J. E., Richmond, J. M. & Resident Memory, T. Cells in autoimmune skin diseases. Front. Immunol. 12 , 652191 (2021).
Chang, M. H. et al. Arthritis flares mediated by tissue-resident memory T cells in the joint. Cell Rep. 37 , 109902 (2021).
Lazzerini, P. E., Abbate, A., Boutjdir, M. & Capecchi, P. L. Fir(e)ing the rhythm: inflammatory cytokines and cardiac arrhythmias. JACC Basic Transl. Sci. 8 , 728–750 (2023).
Wang, F., Wei, Q. & Wu, X. Cardiac arrhythmias associated with immune checkpoint inhibitors: a comprehensive disproportionality analysis of the FDA adverse event reporting system. Front. Pharmacol. 13 , 986357 (2022).
Fung, H. Y., Teryek, M., Lemenze, A. D. & Bergsbaken, T. CD103 fate mapping reveals that intestinal CD103 − tissue-resident memory T cells are the primary responders to secondary infection. Sci. Immunol. 7 , eabl9925 (2022).
Weisberg, S. P. et al. Tissue-resident memory T cells mediate immune homeostasis in the human pancreas through the PD-1/PD-L1 pathway. Cell Rep. 29 , 3916–3932 (2019).
Jaiswal, A. et al. An activation to memory differentiation trajectory of tumor-infiltrating lymphocytes informs metastatic melanoma outcomes. Cancer Cell 40 , 524–544 (2022).
Mami-Chouaib, F. et al. Resident memory T cells, critical components in tumor immunology. J. Immunother. Cancer 6 , 87 (2018).
Sasson, S. C. et al. Interferon-gamma–producing CD8 + tissue resident memory T cells are a targetable hallmark of immune checkpoint inhibitor–colitis. Gastroenterology 161 , 1229–1244 (2021).
Joseph, L. et al. Incidence of cancer treatment induced arrhythmia associated with immune checkpoint inhibitors. J. Atr. Fibrillation 13 , 2461 (2021).
Liu, L. et al. PD-1/PD-L1 expression on CD 4+ T cells and myeloid DCs correlates with the immune pathogenesis of atrial fibrillation. J. Cell. Mol. Med. 19 , 1223–1233 (2015).
Venteclef, N. et al. Human epicardial adipose tissue induces fibrosis of the atrial myocardium through the secretion of adipo-fibrokines. Eur. Heart J. 36 , 795–805 (2015).
Ernault, A. C. et al. Secretome of atrial epicardial adipose tissue facilitates reentrant arrhythmias by myocardial remodeling. Heart Rhythm 19 , 1461–1470 (2022).
Bénézech, C. et al. Inflammation-induced formation of fat-associated lymphoid clusters. Nat. Immunol. 16 , 819–828 (2015).
Zhang, X. Z. et al. T lymphocyte characteristics and immune repertoires in the epicardial adipose tissue of heart failure patients. Front. Immunol. 14 , 1126997 (2023).
Santema, B. T. et al. Pathophysiological pathways in patients with heart failure and atrial fibrillation. Cardiovasc. Res. 118 , 2478–2487 (2021).
Article PubMed Central Google Scholar
Yao, Y., Yang, M., Liu, D. & Zhao, Q. Immune remodeling and atrial fibrillation. Front. Physiol. 13 , 927221 (2022).
Mitrokhin, V. et al. L-type Ca 2+ channels’ involvement in IFN-γ-induced signaling in rat ventricular cardiomyocytes. J. Physiol. Biochem. 75 , 109–115 (2019).
Dusi, V., Ghidoni, A., Ravera, A., De Ferrari, G. M. & Calvillo, L. Chemokines and heart disease: a network connecting cardiovascular biology to immune and autonomic nervous systems. Mediators Inflamm. 2016 , 5902947 (2016).
Hearnden, R., Sandhar, B., Vyas, V. & Longhi, M. P. Isolation of stromal vascular fraction cell suspensions from mouse and human adipose tissues for downstream applications. STAR Protoc. 2 , 100422 (2021).
Peacock, T., Heather, J. M., Ronel, T. & Chain, B. Decombinator V4: an improved AIRR compliant-software package for T-cell receptor sequence annotation? Bioinformatics 37 , 876–878 (2021).
Longhi, P. & Vyas, V. Bulk TCR-seq from paired EAT, atrial appendage and blood samples. Zenodo https://doi.org/10.5281/zenodo.13318819 (2024).
Download references
Acknowledgements
We are indebted to all the individuals who kindly agreed to participate in this study. We thank all members of the Barts Heart Centre, the Barts Bioresource staff, S. Petersen and M. Burton who assisted with participant consent and access to medical records. This work was supported by Barts Charity MGU0413 (V.V. and M.P.L.); Abbott (V.V. and M.C.F.); Medical Research Council MR/T008059/1 (V.V.); British Heart Foundation FS/19/62/34901 (B.B.); British Heart Foundation FS/13/49/30421 (M.P.L. and H.B.) and PG/16/79/32419 (E.G.W.); the NIHR Barts Biomedical Research Centre (M.C.F.); British Heart Foundation FS/4yPhD/F/22/34174B (J.M.K.); British Heart Foundation Accelerator Award AA/18/5/34222 (F.M.-B. and for purchasing of the CytoFLEX flow cytometer); and the Rosetrees Trust and the UCLH Biomedical Research Centre (B.C.).
Author information
Authors and affiliations.
William Harvey Research Institute, Barts and The London School of Medicine and Dentistry, Queen Mary University of London, London, UK
Vishal Vyas, Balraj Sandhar, Jack M. Keane, Elizabeth G. Wood, Hazel Blythe, Aled Jones, Eriomina Shahaj, Silvia Fanti, Jack Williams, Federica Marelli-Berg, Andrew Tinker, Malcolm C. Finlay & M. Paula Longhi
Department of Cardiology, Barts Heart Centre, St. Bartholomew’s Hospital, London, UK
Vishal Vyas & Malcolm C. Finlay
Cancer Research UK, Barts Centre, Queen Mary University of London, London, UK
Nasrine Metic & Mirjana Efremova
Department of Immunology and Inflammation, Centre for Haematology, Faculty of Medicine, Imperial College London, London, UK
Han Leng Ng & Niklas Feldhahn
UCL Division of Infection and Immunity, University College London, London, UK
Gayathri Nageswaran, Suzanne Byrne & Benny Chain
You can also search for this author in PubMed Google Scholar
Contributions
M.P.L. conceived the study. V.V. and M.P.L. designed experiments. V.V., H.B., E.G.W. and B.S. collected processed tissue samples. V.V. performed and analyzed experiments. J.M.K. performed co-culture experiments. S.F. and F.M.-B. provided support for tissue imaging. E.S., J.W., A.J. and A.T. helped with iPSC-CM culture, characterization and electrophysiology studies. M.E. and N.M. supported deconvolution analysis. H.L.N. and N.F. performed RNA sequencing analysis. G.N., S.B. and B.C. performed bulk TCR sequencing analysis. M.P.L. wrote the paper.
Corresponding author
Correspondence to M. Paula Longhi .
Ethics declarations
Competing interests.
The authors declare no competing interests.
Peer review
Peer review information.
Nature Cardiovascular Research thanks Stephane Hatem, Klaus Ley, Na Li and the other, anonymous, reviewer(s) for their contribution to the peer review of this work.
Additional information
Publisher’s note Springer Nature remains neutral with regard to jurisdictional claims in published maps and institutional affiliations.
Extended data
Extended data fig. 1 immune profiling of eat..
a . Gating strategy for the identification of lymphoid and myeloid cells in tissue. b-d . Absolute number of immune cells in patients with AF and on SR across EAT ( b ), SAT ( c ) and blood ( d ). e . Percentages of myeloid and lymphoid cells in the EAT. Statistical significance was determined by the unpaired two-tailed Mann-Whitney test, and data are represented as Mean ± SD. b-e . Data shows individual patients (n=26 SN and 18 AF biological replicates).
Extended Data Fig. 2. Frequency of T RM cells in AF.
a . Gating strategy for the identification of T RM cells. Immune cells were gated on live CD3 + CD4 + or CD8 + T cells as shown in Extended Data Fig. 1a . b . Frequency of CD4 + and CD8 + T RM cells in unmatched patients with AF compared to SR controls. Patients with post-operative AF were excluded. c . Frequency of CD4 + and CD8 + T RM cells in normotensive (N) compared to hypertensive (HTN) patients. d . Frequency of CD4 + and CD8 + T RM cells between patients undergoing VR or CABG surgery. b-d . Significance was obtained using two-tailed Mann-Whitney test. Data is represented as Mean ± SD e-f . Correlation analysis of CD4 + and CD8 + T RM cells with age ( d ) and BMI ( e) by lineal regression and two-tailed p-value analysis . g-h . Gating strategy for the identification of cytokine production by CD4 + ( f ) or CD8 + ( g ) T cells T RM cells. i . Correlation analysis of IL22 production with the frequency of CD4 + T RM cells measured by lineal regression and two-tailed p-value analysis. j . Correlation analysis of IFNγ production with the frequency of CD8 + T RM cells measured by lineal regression and two-tailed p-value analysis. b-i . Data shows individual patients (n=122).
Extended Data Fig. 3 Distinct protein profile across immune cells in EAT and AA.
a . UMAP plots of EAT and AA samples identifying similar 19 cell clusters. b . Bubbleplotshowing surface expression of antibody-derived tag protein expression value for selective markers. c . UMAP plot representation of antibody-derived tag protein expression value for selective markers for the identification of memory T cells, B and myeloid cells. Colour bar key represents average expression levels. Expression values are normalized for quantitative comparison within each dataset. d . TCR clonal overlap detected by the Morisita index. e . Bar graph indicates the relative abundance of TCRβ clonotypes in paired tissues EAT, AA and Blood (BLD). The relative abundance of TCRβ clonotypes. f . TCRβ diversity between paired tissues. Statistical significance was evaluated with 2-way Anova followed Benjamini-Hochberg-correction. g . Heatmap illustrating the compositional TCRβ similarity between paired samples assessed using the Morisita-Horn index. Colour bar key represents the Morisita index. h . Bar graph indicates the relative abundance of TCRβ clonotypes between patients with AF and on SR. Data are presented as mean values ± SD. e-h . Statistical significance was evaluated using 2-way ANOVA with Sidak’s multiple comparisons test. i . TCRβ diversity between AF and SR patients. Statistical significance was evaluated using the two-tailed Mann-Whitney U test for nonparametric data and represented as mean± SD. e-i . Data are from 5 biological replicates.
Extended Data Fig. 4 Spatial transcriptomics.
a . Representative Masson’s Trichrome stains on tissue atrial specimens from 3 patients. b . Representative H&E stains on EAT specimens from 3 patients. c . Representative Masson’s Trichrome stain on tissue specimen at 2.0x magnification with the blue colour depicting the collagen deposition in the EAT/RAA interface and red-brown the atrial tissue from 3 patients. d . Representative immunofluorescent staining used for the identification of regions of interest (ROI) for spatial transcriptomic analysis. Cardiomyocytes were identified by troponin staining (blue), adipocytes by FABP4 expression (green) and immune cells by CD45 (red). e . Dimensionality reduction plots demonstrating sample clustering by type of tissue. f . Absolute number of immune cells in paired EAT and AA tissue samples. Statistical significance was determined by paired two-tailed T test, and data are represented as mean± SD (n=18 biological replicates). g . Bar graph comparing the proportion of cell types over total populations between the AA borderzone and deep in the tissue. h-i . Bar graph comparing the proportion of cell types over total populations between the EAT ( h ) or AA ( i ) borderzoneand deep in the tissue between patients with AF and on SR. g-h . Statistical significance was evaluated by two-way ANOVA with Sidak’s multiple comparison test. Bars represent mean±SD. g-i . Assays were performed in 3 biological replicates in technical triplicates. j . Representative immunohistochemistry staining of CCL5.
Extended Data Fig. 5 Characterisation of iPSC-derived atrial cardiomyocytes.
a . Representative immunofluorescence images depicting DAPI (nuclear stain), troponin (TNNT2) and smooth muscle actinin (ACTN2), and the merged images (DAPI) in blue, TNNT2 and ACTN2 in red). Scale bar 50 μM (3 independent experiments). b . Heat map demonstrating the relative expression of key atrial cardiomyocyte genes in independently differentiated cardiomyocyte plates (n=3). c . Atrial cardiomyocyte action potential waveform recorded using the voltage-sensitive dye, di-4-ANEPPS. d . Bar graphs demonstrating percentage change in CaT 50 and CaT 90 in CD8 + T cells compared to untreated cells. Biological replicates n=6. Statistical significance was assessed using two-tailed t-test for parametric data, represented as mean and SD e . iPSC-cardiomyocytes were cultured directly with T RM cells or in the presence of a 0.4 μM transwell were analysed by RT-PCR. Relative expression levels of selective genes in iPSC-cardiomyocytes (n = 6 biological replicates in triplicates) were analysed by RT-PCR. f . As in e , but bars represent expression of IFNγ−treated iPSC-cardiac fibroblast compared to untreated cells from three independently experiments (n = 9). f-e . Bars represent the mean ratio and upper and lower limits. Statistical significance was determined using unpaired two-tailed t -test.
Supplementary information
Reporting summary, supplementary table 1.
All patientsʼ clinical characteristics
Supplementary Table 2
Grouped patientsʼ clinical characteristics
Supplementary Table 3
Genes differentially expressed within each cluster
Supplementary Table 4
DEG between EAT versus SAT for each cluster
Supplementary Table 5
List of TCR clones
Supplementary Table 6
DEG of hyperexpanded clones in EAT versus AA
Supplementary Table 7
DEG between CD8 + T RM clusters: cluster 2 (T RM -1), cluster 8 (T RM -2) and cluster 9 (T RM -3)
Supplementary Table 8
DEG between CD8 + T RM clusters 2 versus 8
Supplementary Table 9
List of genes in the GeoMX platform
Supplementary Table 10
DEG deep in the tissue and border zone in the EAT
Supplementary Table 11
DEG deep in the tissue and border zone in the AA
Supplementary Table 12
DEG in the EAT border zone in AF versus SR patients
Supplementary Table 13
DEG in the AA border zone in AF versus SR patients
Supplementary Table 14
DEG of iPSC-CMs cultured with T EM versus CD4 + T RM
Supplementary Table 15
List of primers for RT–PCR
Source Data Fig. 3
Patients’ clinical characteristics for Fig. 3g–k.
Source Data Fig. 7
Statistical source data for Fig. 7b,f.
Source Data Extended Data Fig. 5
Statistical source data for Extended Data Fig. 5e,f.
Rights and permissions
Open Access This article is licensed under a Creative Commons Attribution 4.0 International License, which permits use, sharing, adaptation, distribution and reproduction in any medium or format, as long as you give appropriate credit to the original author(s) and the source, provide a link to the Creative Commons licence, and indicate if changes were made. The images or other third party material in this article are included in the article’s Creative Commons licence, unless indicated otherwise in a credit line to the material. If material is not included in the article’s Creative Commons licence and your intended use is not permitted by statutory regulation or exceeds the permitted use, you will need to obtain permission directly from the copyright holder. To view a copy of this licence, visit http://creativecommons.org/licenses/by/4.0/ .
Reprints and permissions
About this article
Cite this article.
Vyas, V., Sandhar, B., Keane, J.M. et al. Tissue-resident memory T cells in epicardial adipose tissue comprise transcriptionally distinct subsets that are modulated in atrial fibrillation. Nat Cardiovasc Res (2024). https://doi.org/10.1038/s44161-024-00532-x
Download citation
Received : 20 September 2023
Accepted : 29 July 2024
Published : 23 August 2024
DOI : https://doi.org/10.1038/s44161-024-00532-x
Share this article
Anyone you share the following link with will be able to read this content:
Sorry, a shareable link is not currently available for this article.
Provided by the Springer Nature SharedIt content-sharing initiative
Quick links
- Explore articles by subject
- Guide to authors
- Editorial policies
Sign up for the Nature Briefing newsletter — what matters in science, free to your inbox daily.


Home » Qualitative Research – Methods, Analysis Types and Guide
Qualitative Research – Methods, Analysis Types and Guide
Table of Contents

Qualitative Research
Qualitative research is a type of research methodology that focuses on exploring and understanding people’s beliefs, attitudes, behaviors, and experiences through the collection and analysis of non-numerical data. It seeks to answer research questions through the examination of subjective data, such as interviews, focus groups, observations, and textual analysis.
Qualitative research aims to uncover the meaning and significance of social phenomena, and it typically involves a more flexible and iterative approach to data collection and analysis compared to quantitative research. Qualitative research is often used in fields such as sociology, anthropology, psychology, and education.
Qualitative Research Methods

Qualitative Research Methods are as follows:
One-to-One Interview
This method involves conducting an interview with a single participant to gain a detailed understanding of their experiences, attitudes, and beliefs. One-to-one interviews can be conducted in-person, over the phone, or through video conferencing. The interviewer typically uses open-ended questions to encourage the participant to share their thoughts and feelings. One-to-one interviews are useful for gaining detailed insights into individual experiences.
Focus Groups
This method involves bringing together a group of people to discuss a specific topic in a structured setting. The focus group is led by a moderator who guides the discussion and encourages participants to share their thoughts and opinions. Focus groups are useful for generating ideas and insights, exploring social norms and attitudes, and understanding group dynamics.
Ethnographic Studies
This method involves immersing oneself in a culture or community to gain a deep understanding of its norms, beliefs, and practices. Ethnographic studies typically involve long-term fieldwork and observation, as well as interviews and document analysis. Ethnographic studies are useful for understanding the cultural context of social phenomena and for gaining a holistic understanding of complex social processes.
Text Analysis
This method involves analyzing written or spoken language to identify patterns and themes. Text analysis can be quantitative or qualitative. Qualitative text analysis involves close reading and interpretation of texts to identify recurring themes, concepts, and patterns. Text analysis is useful for understanding media messages, public discourse, and cultural trends.
This method involves an in-depth examination of a single person, group, or event to gain an understanding of complex phenomena. Case studies typically involve a combination of data collection methods, such as interviews, observations, and document analysis, to provide a comprehensive understanding of the case. Case studies are useful for exploring unique or rare cases, and for generating hypotheses for further research.
Process of Observation
This method involves systematically observing and recording behaviors and interactions in natural settings. The observer may take notes, use audio or video recordings, or use other methods to document what they see. Process of observation is useful for understanding social interactions, cultural practices, and the context in which behaviors occur.
Record Keeping
This method involves keeping detailed records of observations, interviews, and other data collected during the research process. Record keeping is essential for ensuring the accuracy and reliability of the data, and for providing a basis for analysis and interpretation.
This method involves collecting data from a large sample of participants through a structured questionnaire. Surveys can be conducted in person, over the phone, through mail, or online. Surveys are useful for collecting data on attitudes, beliefs, and behaviors, and for identifying patterns and trends in a population.
Qualitative data analysis is a process of turning unstructured data into meaningful insights. It involves extracting and organizing information from sources like interviews, focus groups, and surveys. The goal is to understand people’s attitudes, behaviors, and motivations
Qualitative Research Analysis Methods
Qualitative Research analysis methods involve a systematic approach to interpreting and making sense of the data collected in qualitative research. Here are some common qualitative data analysis methods:
Thematic Analysis
This method involves identifying patterns or themes in the data that are relevant to the research question. The researcher reviews the data, identifies keywords or phrases, and groups them into categories or themes. Thematic analysis is useful for identifying patterns across multiple data sources and for generating new insights into the research topic.
Content Analysis
This method involves analyzing the content of written or spoken language to identify key themes or concepts. Content analysis can be quantitative or qualitative. Qualitative content analysis involves close reading and interpretation of texts to identify recurring themes, concepts, and patterns. Content analysis is useful for identifying patterns in media messages, public discourse, and cultural trends.
Discourse Analysis
This method involves analyzing language to understand how it constructs meaning and shapes social interactions. Discourse analysis can involve a variety of methods, such as conversation analysis, critical discourse analysis, and narrative analysis. Discourse analysis is useful for understanding how language shapes social interactions, cultural norms, and power relationships.
Grounded Theory Analysis
This method involves developing a theory or explanation based on the data collected. Grounded theory analysis starts with the data and uses an iterative process of coding and analysis to identify patterns and themes in the data. The theory or explanation that emerges is grounded in the data, rather than preconceived hypotheses. Grounded theory analysis is useful for understanding complex social phenomena and for generating new theoretical insights.
Narrative Analysis
This method involves analyzing the stories or narratives that participants share to gain insights into their experiences, attitudes, and beliefs. Narrative analysis can involve a variety of methods, such as structural analysis, thematic analysis, and discourse analysis. Narrative analysis is useful for understanding how individuals construct their identities, make sense of their experiences, and communicate their values and beliefs.
Phenomenological Analysis
This method involves analyzing how individuals make sense of their experiences and the meanings they attach to them. Phenomenological analysis typically involves in-depth interviews with participants to explore their experiences in detail. Phenomenological analysis is useful for understanding subjective experiences and for developing a rich understanding of human consciousness.
Comparative Analysis
This method involves comparing and contrasting data across different cases or groups to identify similarities and differences. Comparative analysis can be used to identify patterns or themes that are common across multiple cases, as well as to identify unique or distinctive features of individual cases. Comparative analysis is useful for understanding how social phenomena vary across different contexts and groups.
Applications of Qualitative Research
Qualitative research has many applications across different fields and industries. Here are some examples of how qualitative research is used:
- Market Research: Qualitative research is often used in market research to understand consumer attitudes, behaviors, and preferences. Researchers conduct focus groups and one-on-one interviews with consumers to gather insights into their experiences and perceptions of products and services.
- Health Care: Qualitative research is used in health care to explore patient experiences and perspectives on health and illness. Researchers conduct in-depth interviews with patients and their families to gather information on their experiences with different health care providers and treatments.
- Education: Qualitative research is used in education to understand student experiences and to develop effective teaching strategies. Researchers conduct classroom observations and interviews with students and teachers to gather insights into classroom dynamics and instructional practices.
- Social Work : Qualitative research is used in social work to explore social problems and to develop interventions to address them. Researchers conduct in-depth interviews with individuals and families to understand their experiences with poverty, discrimination, and other social problems.
- Anthropology : Qualitative research is used in anthropology to understand different cultures and societies. Researchers conduct ethnographic studies and observe and interview members of different cultural groups to gain insights into their beliefs, practices, and social structures.
- Psychology : Qualitative research is used in psychology to understand human behavior and mental processes. Researchers conduct in-depth interviews with individuals to explore their thoughts, feelings, and experiences.
- Public Policy : Qualitative research is used in public policy to explore public attitudes and to inform policy decisions. Researchers conduct focus groups and one-on-one interviews with members of the public to gather insights into their perspectives on different policy issues.
How to Conduct Qualitative Research
Here are some general steps for conducting qualitative research:
- Identify your research question: Qualitative research starts with a research question or set of questions that you want to explore. This question should be focused and specific, but also broad enough to allow for exploration and discovery.
- Select your research design: There are different types of qualitative research designs, including ethnography, case study, grounded theory, and phenomenology. You should select a design that aligns with your research question and that will allow you to gather the data you need to answer your research question.
- Recruit participants: Once you have your research question and design, you need to recruit participants. The number of participants you need will depend on your research design and the scope of your research. You can recruit participants through advertisements, social media, or through personal networks.
- Collect data: There are different methods for collecting qualitative data, including interviews, focus groups, observation, and document analysis. You should select the method or methods that align with your research design and that will allow you to gather the data you need to answer your research question.
- Analyze data: Once you have collected your data, you need to analyze it. This involves reviewing your data, identifying patterns and themes, and developing codes to organize your data. You can use different software programs to help you analyze your data, or you can do it manually.
- Interpret data: Once you have analyzed your data, you need to interpret it. This involves making sense of the patterns and themes you have identified, and developing insights and conclusions that answer your research question. You should be guided by your research question and use your data to support your conclusions.
- Communicate results: Once you have interpreted your data, you need to communicate your results. This can be done through academic papers, presentations, or reports. You should be clear and concise in your communication, and use examples and quotes from your data to support your findings.
Examples of Qualitative Research
Here are some real-time examples of qualitative research:
- Customer Feedback: A company may conduct qualitative research to understand the feedback and experiences of its customers. This may involve conducting focus groups or one-on-one interviews with customers to gather insights into their attitudes, behaviors, and preferences.
- Healthcare : A healthcare provider may conduct qualitative research to explore patient experiences and perspectives on health and illness. This may involve conducting in-depth interviews with patients and their families to gather information on their experiences with different health care providers and treatments.
- Education : An educational institution may conduct qualitative research to understand student experiences and to develop effective teaching strategies. This may involve conducting classroom observations and interviews with students and teachers to gather insights into classroom dynamics and instructional practices.
- Social Work: A social worker may conduct qualitative research to explore social problems and to develop interventions to address them. This may involve conducting in-depth interviews with individuals and families to understand their experiences with poverty, discrimination, and other social problems.
- Anthropology : An anthropologist may conduct qualitative research to understand different cultures and societies. This may involve conducting ethnographic studies and observing and interviewing members of different cultural groups to gain insights into their beliefs, practices, and social structures.
- Psychology : A psychologist may conduct qualitative research to understand human behavior and mental processes. This may involve conducting in-depth interviews with individuals to explore their thoughts, feelings, and experiences.
- Public Policy: A government agency or non-profit organization may conduct qualitative research to explore public attitudes and to inform policy decisions. This may involve conducting focus groups and one-on-one interviews with members of the public to gather insights into their perspectives on different policy issues.
Purpose of Qualitative Research
The purpose of qualitative research is to explore and understand the subjective experiences, behaviors, and perspectives of individuals or groups in a particular context. Unlike quantitative research, which focuses on numerical data and statistical analysis, qualitative research aims to provide in-depth, descriptive information that can help researchers develop insights and theories about complex social phenomena.
Qualitative research can serve multiple purposes, including:
- Exploring new or emerging phenomena : Qualitative research can be useful for exploring new or emerging phenomena, such as new technologies or social trends. This type of research can help researchers develop a deeper understanding of these phenomena and identify potential areas for further study.
- Understanding complex social phenomena : Qualitative research can be useful for exploring complex social phenomena, such as cultural beliefs, social norms, or political processes. This type of research can help researchers develop a more nuanced understanding of these phenomena and identify factors that may influence them.
- Generating new theories or hypotheses: Qualitative research can be useful for generating new theories or hypotheses about social phenomena. By gathering rich, detailed data about individuals’ experiences and perspectives, researchers can develop insights that may challenge existing theories or lead to new lines of inquiry.
- Providing context for quantitative data: Qualitative research can be useful for providing context for quantitative data. By gathering qualitative data alongside quantitative data, researchers can develop a more complete understanding of complex social phenomena and identify potential explanations for quantitative findings.
When to use Qualitative Research
Here are some situations where qualitative research may be appropriate:
- Exploring a new area: If little is known about a particular topic, qualitative research can help to identify key issues, generate hypotheses, and develop new theories.
- Understanding complex phenomena: Qualitative research can be used to investigate complex social, cultural, or organizational phenomena that are difficult to measure quantitatively.
- Investigating subjective experiences: Qualitative research is particularly useful for investigating the subjective experiences of individuals or groups, such as their attitudes, beliefs, values, or emotions.
- Conducting formative research: Qualitative research can be used in the early stages of a research project to develop research questions, identify potential research participants, and refine research methods.
- Evaluating interventions or programs: Qualitative research can be used to evaluate the effectiveness of interventions or programs by collecting data on participants’ experiences, attitudes, and behaviors.
Characteristics of Qualitative Research
Qualitative research is characterized by several key features, including:
- Focus on subjective experience: Qualitative research is concerned with understanding the subjective experiences, beliefs, and perspectives of individuals or groups in a particular context. Researchers aim to explore the meanings that people attach to their experiences and to understand the social and cultural factors that shape these meanings.
- Use of open-ended questions: Qualitative research relies on open-ended questions that allow participants to provide detailed, in-depth responses. Researchers seek to elicit rich, descriptive data that can provide insights into participants’ experiences and perspectives.
- Sampling-based on purpose and diversity: Qualitative research often involves purposive sampling, in which participants are selected based on specific criteria related to the research question. Researchers may also seek to include participants with diverse experiences and perspectives to capture a range of viewpoints.
- Data collection through multiple methods: Qualitative research typically involves the use of multiple data collection methods, such as in-depth interviews, focus groups, and observation. This allows researchers to gather rich, detailed data from multiple sources, which can provide a more complete picture of participants’ experiences and perspectives.
- Inductive data analysis: Qualitative research relies on inductive data analysis, in which researchers develop theories and insights based on the data rather than testing pre-existing hypotheses. Researchers use coding and thematic analysis to identify patterns and themes in the data and to develop theories and explanations based on these patterns.
- Emphasis on researcher reflexivity: Qualitative research recognizes the importance of the researcher’s role in shaping the research process and outcomes. Researchers are encouraged to reflect on their own biases and assumptions and to be transparent about their role in the research process.
Advantages of Qualitative Research
Qualitative research offers several advantages over other research methods, including:
- Depth and detail: Qualitative research allows researchers to gather rich, detailed data that provides a deeper understanding of complex social phenomena. Through in-depth interviews, focus groups, and observation, researchers can gather detailed information about participants’ experiences and perspectives that may be missed by other research methods.
- Flexibility : Qualitative research is a flexible approach that allows researchers to adapt their methods to the research question and context. Researchers can adjust their research methods in real-time to gather more information or explore unexpected findings.
- Contextual understanding: Qualitative research is well-suited to exploring the social and cultural context in which individuals or groups are situated. Researchers can gather information about cultural norms, social structures, and historical events that may influence participants’ experiences and perspectives.
- Participant perspective : Qualitative research prioritizes the perspective of participants, allowing researchers to explore subjective experiences and understand the meanings that participants attach to their experiences.
- Theory development: Qualitative research can contribute to the development of new theories and insights about complex social phenomena. By gathering rich, detailed data and using inductive data analysis, researchers can develop new theories and explanations that may challenge existing understandings.
- Validity : Qualitative research can offer high validity by using multiple data collection methods, purposive and diverse sampling, and researcher reflexivity. This can help ensure that findings are credible and trustworthy.
Limitations of Qualitative Research
Qualitative research also has some limitations, including:
- Subjectivity : Qualitative research relies on the subjective interpretation of researchers, which can introduce bias into the research process. The researcher’s perspective, beliefs, and experiences can influence the way data is collected, analyzed, and interpreted.
- Limited generalizability: Qualitative research typically involves small, purposive samples that may not be representative of larger populations. This limits the generalizability of findings to other contexts or populations.
- Time-consuming: Qualitative research can be a time-consuming process, requiring significant resources for data collection, analysis, and interpretation.
- Resource-intensive: Qualitative research may require more resources than other research methods, including specialized training for researchers, specialized software for data analysis, and transcription services.
- Limited reliability: Qualitative research may be less reliable than quantitative research, as it relies on the subjective interpretation of researchers. This can make it difficult to replicate findings or compare results across different studies.
- Ethics and confidentiality: Qualitative research involves collecting sensitive information from participants, which raises ethical concerns about confidentiality and informed consent. Researchers must take care to protect the privacy and confidentiality of participants and obtain informed consent.
Also see Research Methods
About the author

Muhammad Hassan
Researcher, Academic Writer, Web developer
You may also like

Ethnographic Research -Types, Methods and Guide

Explanatory Research – Types, Methods, Guide

Experimental Design – Types, Methods, Guide

Questionnaire – Definition, Types, and Examples

Observational Research – Methods and Guide

Descriptive Research Design – Types, Methods and...
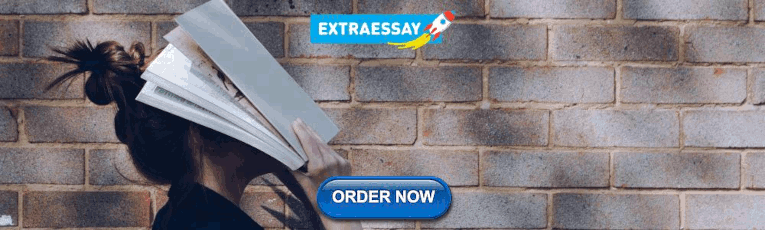
IMAGES
COMMENTS
Qualitative analysis tends to be quite flexible and relies on the researcher's judgement, so you have to reflect carefully on your choices and assumptions and be careful to avoid research bias. Quantitative analysis methods. Quantitative analysis uses numbers and statistics to understand frequencies, averages and correlations (in descriptive ...
Definition of research in data analysis: According to LeCompte and Schensul, research data analysis is a process used by researchers to reduce data to a story and interpret it to derive insights. The data analysis process helps reduce a large chunk of data into smaller fragments, which makes sense. Three essential things occur during the data ...
Statistical analysis is the most common quantitative research analysis method. It involves using statistical tools and techniques to analyze the numerical data collected during the research process. Statistical analysis can be used to identify patterns, trends, and relationships between variables, and to test hypotheses and theories. Regression ...
Quantitative research methods are used to collect and analyze numerical data. This type of research is useful when the objective is to test a hypothesis, determine cause-and-effect relationships, and measure the prevalence of certain phenomena. Quantitative research methods include surveys, experiments, and secondary data analysis.
Exploratory analysis is often used in the early stages of research or data analysis to generate hypotheses and identify areas for further investigation. Data Analysis Methods. Data Analysis Methods are as follows: Statistical Analysis. This method involves the use of mathematical models and statistical tools to analyze and interpret data.
About Research Methods. This guide provides an overview of research methods, how to choose and use them, and supports and resources at UC Berkeley. As Patten and Newhart note in the book Understanding Research Methods, "Research methods are the building blocks of the scientific enterprise. They are the "how" for building systematic knowledge.
Statistical analysis means investigating trends, patterns, and relationships using quantitative data. It is an important research tool used by scientists, governments, businesses, and other organizations. ... Your choice of statistical test depends on your research questions, research design, sampling method, and data characteristics ...
Introduction. Statistical analysis is necessary for any research project seeking to make quantitative conclusions. The following is a primer for research-based statistical analysis. It is intended to be a high-level overview of appropriate statistical testing, while not diving too deep into any specific methodology.
To analyse data collected in a statistically valid manner (e.g. from experiments, surveys, and observations). Meta-analysis. Quantitative. To statistically analyse the results of a large collection of studies. Can only be applied to studies that collected data in a statistically valid manner. Thematic analysis.
The SAGE Handbook of. tive Data AnalysisUwe FlickMapping the FieldData analys. s is the central step in qualitative research. Whatever the data are, it is their analysis that, in a de. isive way, forms the outcomes of the research. Sometimes, data collection is limited to recording and docu-menting naturally occurring ph.
Jessica Nina Lester is an associate professor of Counseling and Educational Psychology at Indiana University. She received her PhD from the University of Tennessee, Knoxville. Her research strand focuses on the study and development of qualitative research methodologies and methods at a theoretical, conceptual, and technical level.
In this tutorial paper, we will use the term methodological study to refer to any study that reports on the design, conduct, analysis or reporting of primary or secondary research-related reports (such as trial registry entries and conference abstracts). In the past 10 years, there has been an increase in the use of terms related to ...
Data Analysis and Presentation Techniques that Apply to both Survey and Interview Research. Create a documentation of the data and the process of data collection. Analyze the data rather than just describing it - use it to tell a story that focuses on answering the research question. Use charts or tables to help the reader understand the data ...
QDA Method #3: Discourse Analysis. Discourse is simply a fancy word for written or spoken language or debate. So, discourse analysis is all about analysing language within its social context. In other words, analysing language - such as a conversation, a speech, etc - within the culture and society it takes place.
INTRODUCTION. Scientific research is usually initiated by posing evidenced-based research questions which are then explicitly restated as hypotheses.1,2 The hypotheses provide directions to guide the study, solutions, explanations, and expected results.3,4 Both research questions and hypotheses are essentially formulated based on conventional theories and real-world processes, which allow the ...
Data analysis techniques in research are categorized into qualitative and quantitative methods, each with its specific approaches and tools. These techniques are instrumental in extracting meaningful insights, patterns, and relationships from data to support informed decision-making, validate hypotheses, and derive actionable recommendations.
What are research methods. Research methods are the strategies, processes or techniques utilized in the collection of data or evidence for analysis in order to uncover new information or create better understanding of a topic. There are different types of research methods which use different tools for data collection.
Research involving the collection of data in numerical form for quantitative analysis. The numerical data can be durations, scores, counts of incidents, ratings, or scales. Quantitative data can be collected in either controlled or naturalistic environments, in laboratories or field studies, from special populations or from samples of the ...
Regression analysis is a quantitative research method which is used when the study involves modelling and analysing several variables, where the relationship includes a dependent variable and one or more independent variables. In simple terms, regression analysis is a quantitative method used to test the nature of relationships between a dependent variable and one or more independent variables.
Content analysis is a research method used to identify patterns in recorded communication. To conduct content analysis, you systematically collect data from a set of texts, which can be written, oral, or visual: Books, newspapers and magazines. Speeches and interviews. Web content and social media posts. Photographs and films.
Cluster analysis. Time series analysis. Sentiment analysis. The data analysis process. The best tools for data analysis. Key takeaways. The first six methods listed are used for quantitative data, while the last technique applies to qualitative data.
7 data analysis methods. Implementing data analysis methods is important for you to help your organization get the most out of its data. Depending on your industry and goals, you might use certain types of analysis more than others. As you start learning different types of methods, seven different analysis types you can start with include the ...
Qualitative research methods. Because of the nature of qualitative data (complex, detailed information), the research methods used to collect it are more involved. ... SPSS: SPSS is a statistical analysis tool for quantitative research, appreciated for its user-friendly interface and comprehensive statistical tests, ...
Statistical methods involved in carrying out a study include planning, designing, collecting data, analysing, drawing meaningful interpretation and reporting of the research findings. The statistical analysis gives meaning to the meaningless numbers, thereby breathing life into a lifeless data. The results and inferences are precise only if ...
Qualitative research is a research method that aims to provide contextual, descriptive, and non-numerical insights on a specific issue. Qualitative research methods like interviews, case studies, and ethnographic studies allow you to uncover the reasoning behind your user's attitudes and opinions.
Qualitative Research Methodology. This is a research methodology that involves the collection and analysis of non-numerical data such as words, images, and observations. This type of research is often used to explore complex phenomena, to gain an in-depth understanding of a particular topic, and to generate hypotheses.
This book presents a comprehensive guide to interpretative phenomenological analysis (IPA) which is an increasingly popular approach to qualitative inquiry taught to undergraduate and postgraduate students today. The first chapter outlines the theoretical foundations for IPA. It discusses phenomenology, hermeneutics, and idiography and how they have been taken up by IPA. The next four chapters ...
As a method of data analysis, we applied content analysis, which is recognized as one of the most appropriate methods in environmental and sustainability reporting and disclosure research [10,26,29]. The method is also applied in diverse areas of family business research [ 63 ].
Fig. 2: CITE-seq analysis of immune cells in the EAT and AA. Fig. 3: Characterization of T RM cells across EAT and AA tissues. Fig. 4: CITE-seq identifies two CD8 + T RM cell populations with a ...
Qualitative Research. Qualitative research is a type of research methodology that focuses on exploring and understanding people's beliefs, attitudes, behaviors, and experiences through the collection and analysis of non-numerical data. It seeks to answer research questions through the examination of subjective data, such as interviews, focus ...