- Skip to main content
- Skip to primary sidebar
- Skip to footer
- QuestionPro

- Solutions Industries Gaming Automotive Sports and events Education Government Travel & Hospitality Financial Services Healthcare Cannabis Technology Use Case AskWhy Communities Audience Contactless surveys Mobile LivePolls Member Experience GDPR Positive People Science 360 Feedback Surveys
- Resources Blog eBooks Survey Templates Case Studies Training Help center

Home Market Research
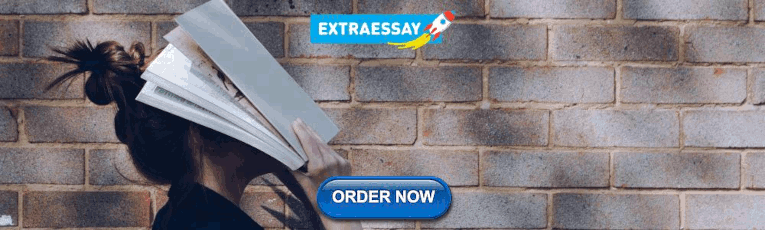
Qualitative Data Analysis: What is it, Methods + Examples
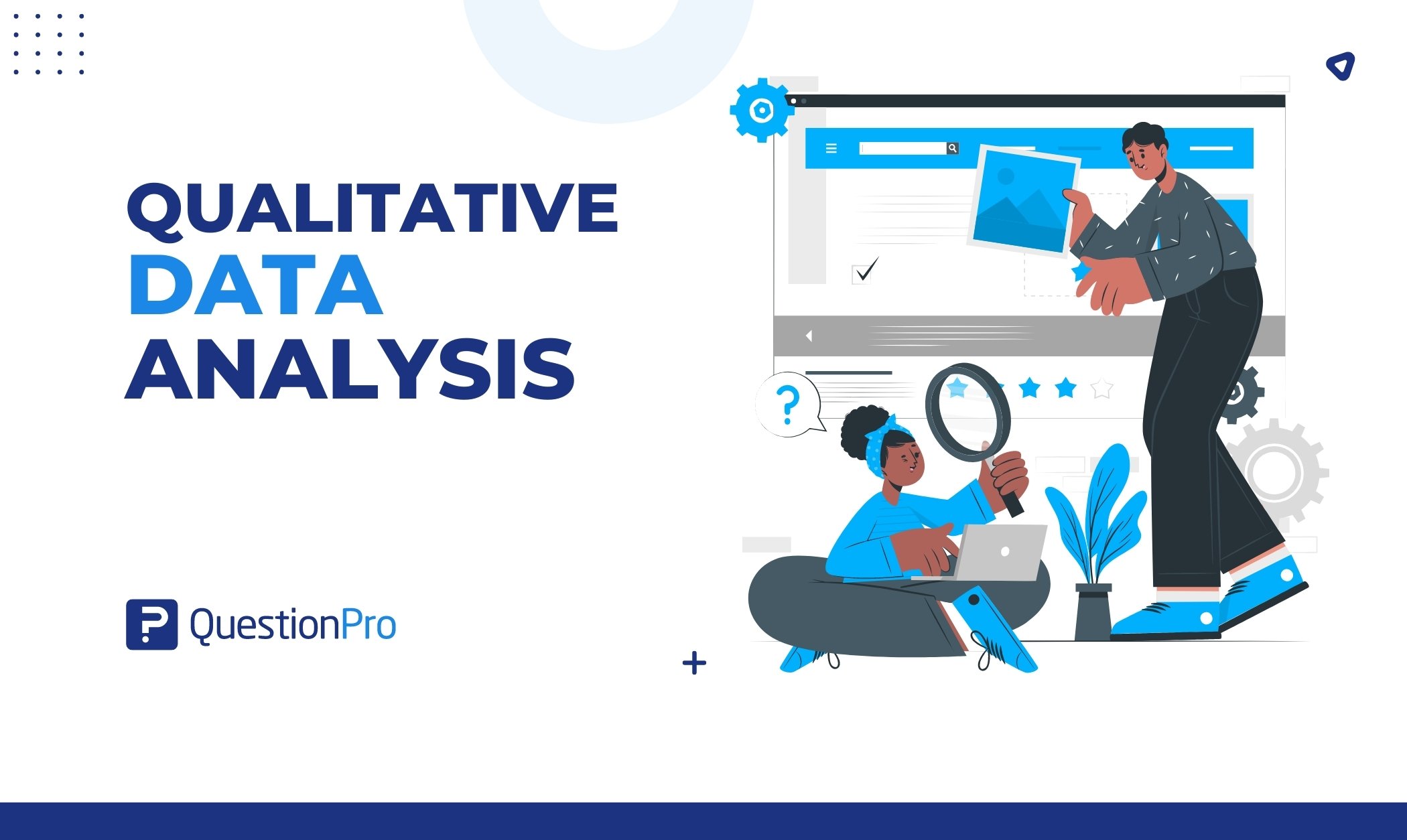
In a world rich with information and narrative, understanding the deeper layers of human experiences requires a unique vision that goes beyond numbers and figures. This is where the power of qualitative data analysis comes to light.
In this blog, we’ll learn about qualitative data analysis, explore its methods, and provide real-life examples showcasing its power in uncovering insights.
What is Qualitative Data Analysis?
Qualitative data analysis is a systematic process of examining non-numerical data to extract meaning, patterns, and insights.
In contrast to quantitative analysis, which focuses on numbers and statistical metrics, the qualitative study focuses on the qualitative aspects of data, such as text, images, audio, and videos. It seeks to understand every aspect of human experiences, perceptions, and behaviors by examining the data’s richness.
Companies frequently conduct this analysis on customer feedback. You can collect qualitative data from reviews, complaints, chat messages, interactions with support centers, customer interviews, case notes, or even social media comments. This kind of data holds the key to understanding customer sentiments and preferences in a way that goes beyond mere numbers.
Importance of Qualitative Data Analysis
Qualitative data analysis plays a crucial role in your research and decision-making process across various disciplines. Let’s explore some key reasons that underline the significance of this analysis:
In-Depth Understanding
It enables you to explore complex and nuanced aspects of a phenomenon, delving into the ‘how’ and ‘why’ questions. This method provides you with a deeper understanding of human behavior, experiences, and contexts that quantitative approaches might not capture fully.
Contextual Insight
You can use this analysis to give context to numerical data. It will help you understand the circumstances and conditions that influence participants’ thoughts, feelings, and actions. This contextual insight becomes essential for generating comprehensive explanations.
Theory Development
You can generate or refine hypotheses via qualitative data analysis. As you analyze the data attentively, you can form hypotheses, concepts, and frameworks that will drive your future research and contribute to theoretical advances.
Participant Perspectives
When performing qualitative research, you can highlight participant voices and opinions. This approach is especially useful for understanding marginalized or underrepresented people, as it allows them to communicate their experiences and points of view.
Exploratory Research
The analysis is frequently used at the exploratory stage of your project. It assists you in identifying important variables, developing research questions, and designing quantitative studies that will follow.
Types of Qualitative Data
When conducting qualitative research, you can use several qualitative data collection methods , and here you will come across many sorts of qualitative data that can provide you with unique insights into your study topic. These data kinds add new views and angles to your understanding and analysis.
Interviews and Focus Groups
Interviews and focus groups will be among your key methods for gathering qualitative data. Interviews are one-on-one talks in which participants can freely share their thoughts, experiences, and opinions.
Focus groups, on the other hand, are discussions in which members interact with one another, resulting in dynamic exchanges of ideas. Both methods provide rich qualitative data and direct access to participant perspectives.
Observations and Field Notes
Observations and field notes are another useful sort of qualitative data. You can immerse yourself in the research environment through direct observation, carefully documenting behaviors, interactions, and contextual factors.
These observations will be recorded in your field notes, providing a complete picture of the environment and the behaviors you’re researching. This data type is especially important for comprehending behavior in their natural setting.
Textual and Visual Data
Textual and visual data include a wide range of resources that can be qualitatively analyzed. Documents, written narratives, and transcripts from various sources, such as interviews or speeches, are examples of textual data.
Photographs, films, and even artwork provide a visual layer to your research. These forms of data allow you to investigate what is spoken and the underlying emotions, details, and symbols expressed by language or pictures.
When to Choose Qualitative Data Analysis over Quantitative Data Analysis
As you begin your research journey, understanding why the analysis of qualitative data is important will guide your approach to understanding complex events. If you analyze qualitative data, it will provide new insights that complement quantitative methodologies, which will give you a broader understanding of your study topic.
It is critical to know when to use qualitative analysis over quantitative procedures. You can prefer qualitative data analysis when:
- Complexity Reigns: When your research questions involve deep human experiences, motivations, or emotions, qualitative research excels at revealing these complexities.
- Exploration is Key: Qualitative analysis is ideal for exploratory research. It will assist you in understanding a new or poorly understood topic before formulating quantitative hypotheses.
- Context Matters: If you want to understand how context affects behaviors or results, qualitative data analysis provides the depth needed to grasp these relationships.
- Unanticipated Findings: When your study provides surprising new viewpoints or ideas, qualitative analysis helps you to delve deeply into these emerging themes.
- Subjective Interpretation is Vital: When it comes to understanding people’s subjective experiences and interpretations, qualitative data analysis is the way to go.
You can make informed decisions regarding the right approach for your research objectives if you understand the importance of qualitative analysis and recognize the situations where it shines.
Qualitative Data Analysis Methods and Examples
Exploring various qualitative data analysis methods will provide you with a wide collection for making sense of your research findings. Once the data has been collected, you can choose from several analysis methods based on your research objectives and the data type you’ve collected.
There are five main methods for analyzing qualitative data. Each method takes a distinct approach to identifying patterns, themes, and insights within your qualitative data. They are:
Method 1: Content Analysis
Content analysis is a methodical technique for analyzing textual or visual data in a structured manner. In this method, you will categorize qualitative data by splitting it into manageable pieces and assigning the manual coding process to these units.
As you go, you’ll notice ongoing codes and designs that will allow you to conclude the content. This method is very beneficial for detecting common ideas, concepts, or themes in your data without losing the context.
Steps to Do Content Analysis
Follow these steps when conducting content analysis:
- Collect and Immerse: Begin by collecting the necessary textual or visual data. Immerse yourself in this data to fully understand its content, context, and complexities.
- Assign Codes and Categories: Assign codes to relevant data sections that systematically represent major ideas or themes. Arrange comparable codes into groups that cover the major themes.
- Analyze and Interpret: Develop a structured framework from the categories and codes. Then, evaluate the data in the context of your research question, investigate relationships between categories, discover patterns, and draw meaning from these connections.
Benefits & Challenges
There are various advantages to using content analysis:
- Structured Approach: It offers a systematic approach to dealing with large data sets and ensures consistency throughout the research.
- Objective Insights: This method promotes objectivity, which helps to reduce potential biases in your study.
- Pattern Discovery: Content analysis can help uncover hidden trends, themes, and patterns that are not always obvious.
- Versatility: You can apply content analysis to various data formats, including text, internet content, images, etc.
However, keep in mind the challenges that arise:
- Subjectivity: Even with the best attempts, a certain bias may remain in coding and interpretation.
- Complexity: Analyzing huge data sets requires time and great attention to detail.
- Contextual Nuances: Content analysis may not capture all of the contextual richness that qualitative data analysis highlights.
Example of Content Analysis
Suppose you’re conducting market research and looking at customer feedback on a product. As you collect relevant data and analyze feedback, you’ll see repeating codes like “price,” “quality,” “customer service,” and “features.” These codes are organized into categories such as “positive reviews,” “negative reviews,” and “suggestions for improvement.”
According to your findings, themes such as “price” and “customer service” stand out and show that pricing and customer service greatly impact customer satisfaction. This example highlights the power of content analysis for obtaining significant insights from large textual data collections.
Method 2: Thematic Analysis
Thematic analysis is a well-structured procedure for identifying and analyzing recurring themes in your data. As you become more engaged in the data, you’ll generate codes or short labels representing key concepts. These codes are then organized into themes, providing a consistent framework for organizing and comprehending the substance of the data.
The analysis allows you to organize complex narratives and perspectives into meaningful categories, which will allow you to identify connections and patterns that may not be visible at first.
Steps to Do Thematic Analysis
Follow these steps when conducting a thematic analysis:
- Code and Group: Start by thoroughly examining the data and giving initial codes that identify the segments. To create initial themes, combine relevant codes.
- Code and Group: Begin by engaging yourself in the data, assigning first codes to notable segments. To construct basic themes, group comparable codes together.
- Analyze and Report: Analyze the data within each theme to derive relevant insights. Organize the topics into a consistent structure and explain your findings, along with data extracts that represent each theme.
Thematic analysis has various benefits:
- Structured Exploration: It is a method for identifying patterns and themes in complex qualitative data.
- Comprehensive knowledge: Thematic analysis promotes an in-depth understanding of the complications and meanings of the data.
- Application Flexibility: This method can be customized to various research situations and data kinds.
However, challenges may arise, such as:
- Interpretive Nature: Interpreting qualitative data in thematic analysis is vital, and it is critical to manage researcher bias.
- Time-consuming: The study can be time-consuming, especially with large data sets.
- Subjectivity: The selection of codes and topics might be subjective.
Example of Thematic Analysis
Assume you’re conducting a thematic analysis on job satisfaction interviews. Following your immersion in the data, you assign initial codes such as “work-life balance,” “career growth,” and “colleague relationships.” As you organize these codes, you’ll notice themes develop, such as “Factors Influencing Job Satisfaction” and “Impact on Work Engagement.”
Further investigation reveals the tales and experiences included within these themes and provides insights into how various elements influence job satisfaction. This example demonstrates how thematic analysis can reveal meaningful patterns and insights in qualitative data.
Method 3: Narrative Analysis
The narrative analysis involves the narratives that people share. You’ll investigate the histories in your data, looking at how stories are created and the meanings they express. This method is excellent for learning how people make sense of their experiences through narrative.
Steps to Do Narrative Analysis
The following steps are involved in narrative analysis:
- Gather and Analyze: Start by collecting narratives, such as first-person tales, interviews, or written accounts. Analyze the stories, focusing on the plot, feelings, and characters.
- Find Themes: Look for recurring themes or patterns in various narratives. Think about the similarities and differences between these topics and personal experiences.
- Interpret and Extract Insights: Contextualize the narratives within their larger context. Accept the subjective nature of each narrative and analyze the narrator’s voice and style. Extract insights from the tales by diving into the emotions, motivations, and implications communicated by the stories.
There are various advantages to narrative analysis:
- Deep Exploration: It lets you look deeply into people’s personal experiences and perspectives.
- Human-Centered: This method prioritizes the human perspective, allowing individuals to express themselves.
However, difficulties may arise, such as:
- Interpretive Complexity: Analyzing narratives requires dealing with the complexities of meaning and interpretation.
- Time-consuming: Because of the richness and complexities of tales, working with them can be time-consuming.
Example of Narrative Analysis
Assume you’re conducting narrative analysis on refugee interviews. As you read the stories, you’ll notice common themes of toughness, loss, and hope. The narratives provide insight into the obstacles that refugees face, their strengths, and the dreams that guide them.
The analysis can provide a deeper insight into the refugees’ experiences and the broader social context they navigate by examining the narratives’ emotional subtleties and underlying meanings. This example highlights how narrative analysis can reveal important insights into human stories.
Method 4: Grounded Theory Analysis
Grounded theory analysis is an iterative and systematic approach that allows you to create theories directly from data without being limited by pre-existing hypotheses. With an open mind, you collect data and generate early codes and labels that capture essential ideas or concepts within the data.
As you progress, you refine these codes and increasingly connect them, eventually developing a theory based on the data. Grounded theory analysis is a dynamic process for developing new insights and hypotheses based on details in your data.
Steps to Do Grounded Theory Analysis
Grounded theory analysis requires the following steps:
- Initial Coding: First, immerse yourself in the data, producing initial codes that represent major concepts or patterns.
- Categorize and Connect: Using axial coding, organize the initial codes, which establish relationships and connections between topics.
- Build the Theory: Focus on creating a core category that connects the codes and themes. Regularly refine the theory by comparing and integrating new data, ensuring that it evolves organically from the data.
Grounded theory analysis has various benefits:
- Theory Generation: It provides a one-of-a-kind opportunity to generate hypotheses straight from data and promotes new insights.
- In-depth Understanding: The analysis allows you to deeply analyze the data and reveal complex relationships and patterns.
- Flexible Process: This method is customizable and ongoing, which allows you to enhance your research as you collect additional data.
However, challenges might arise with:
- Time and Resources: Because grounded theory analysis is a continuous process, it requires a large commitment of time and resources.
- Theoretical Development: Creating a grounded theory involves a thorough understanding of qualitative data analysis software and theoretical concepts.
- Interpretation of Complexity: Interpreting and incorporating a newly developed theory into existing literature can be intellectually hard.
Example of Grounded Theory Analysis
Assume you’re performing a grounded theory analysis on workplace collaboration interviews. As you open code the data, you will discover notions such as “communication barriers,” “team dynamics,” and “leadership roles.” Axial coding demonstrates links between these notions, emphasizing the significance of efficient communication in developing collaboration.
You create the core “Integrated Communication Strategies” category through selective coding, which unifies new topics.
This theory-driven category serves as the framework for understanding how numerous aspects contribute to effective team collaboration. This example shows how grounded theory analysis allows you to generate a theory directly from the inherent nature of the data.
Method 5: Discourse Analysis
Discourse analysis focuses on language and communication. You’ll look at how language produces meaning and how it reflects power relations, identities, and cultural influences. This strategy examines what is said and how it is said; the words, phrasing, and larger context of communication.
The analysis is precious when investigating power dynamics, identities, and cultural influences encoded in language. By evaluating the language used in your data, you can identify underlying assumptions, cultural standards, and how individuals negotiate meaning through communication.
Steps to Do Discourse Analysis
Conducting discourse analysis entails the following steps:
- Select Discourse: For analysis, choose language-based data such as texts, speeches, or media content.
- Analyze Language: Immerse yourself in the conversation, examining language choices, metaphors, and underlying assumptions.
- Discover Patterns: Recognize the dialogue’s reoccurring themes, ideologies, and power dynamics. To fully understand the effects of these patterns, put them in their larger context.
There are various advantages of using discourse analysis:
- Understanding Language: It provides an extensive understanding of how language builds meaning and influences perceptions.
- Uncovering Power Dynamics: The analysis reveals how power dynamics appear via language.
- Cultural Insights: This method identifies cultural norms, beliefs, and ideologies stored in communication.
However, the following challenges may arise:
- Complexity of Interpretation: Language analysis involves navigating multiple levels of nuance and interpretation.
- Subjectivity: Interpretation can be subjective, so controlling researcher bias is important.
- Time-Intensive: Discourse analysis can take a lot of time because careful linguistic study is required in this analysis.
Example of Discourse Analysis
Consider doing discourse analysis on media coverage of a political event. You notice repeating linguistic patterns in news articles that depict the event as a conflict between opposing parties. Through deconstruction, you can expose how this framing supports particular ideologies and power relations.
You can illustrate how language choices influence public perceptions and contribute to building the narrative around the event by analyzing the speech within the broader political and social context. This example shows how discourse analysis can reveal hidden power dynamics and cultural influences on communication.
How to do Qualitative Data Analysis with the QuestionPro Research suite?
QuestionPro is a popular survey and research platform that offers tools for collecting and analyzing qualitative and quantitative data. Follow these general steps for conducting qualitative data analysis using the QuestionPro Research Suite:
- Collect Qualitative Data: Set up your survey to capture qualitative responses. It might involve open-ended questions, text boxes, or comment sections where participants can provide detailed responses.
- Export Qualitative Responses: Export the responses once you’ve collected qualitative data through your survey. QuestionPro typically allows you to export survey data in various formats, such as Excel or CSV.
- Prepare Data for Analysis: Review the exported data and clean it if necessary. Remove irrelevant or duplicate entries to ensure your data is ready for analysis.
- Code and Categorize Responses: Segment and label data, letting new patterns emerge naturally, then develop categories through axial coding to structure the analysis.
- Identify Themes: Analyze the coded responses to identify recurring themes, patterns, and insights. Look for similarities and differences in participants’ responses.
- Generate Reports and Visualizations: Utilize the reporting features of QuestionPro to create visualizations, charts, and graphs that help communicate the themes and findings from your qualitative research.
- Interpret and Draw Conclusions: Interpret the themes and patterns you’ve identified in the qualitative data. Consider how these findings answer your research questions or provide insights into your study topic.
- Integrate with Quantitative Data (if applicable): If you’re also conducting quantitative research using QuestionPro, consider integrating your qualitative findings with quantitative results to provide a more comprehensive understanding.
Qualitative data analysis is vital in uncovering various human experiences, views, and stories. If you’re ready to transform your research journey and apply the power of qualitative analysis, now is the moment to do it. Book a demo with QuestionPro today and begin your journey of exploration.
LEARN MORE FREE TRIAL
MORE LIKE THIS
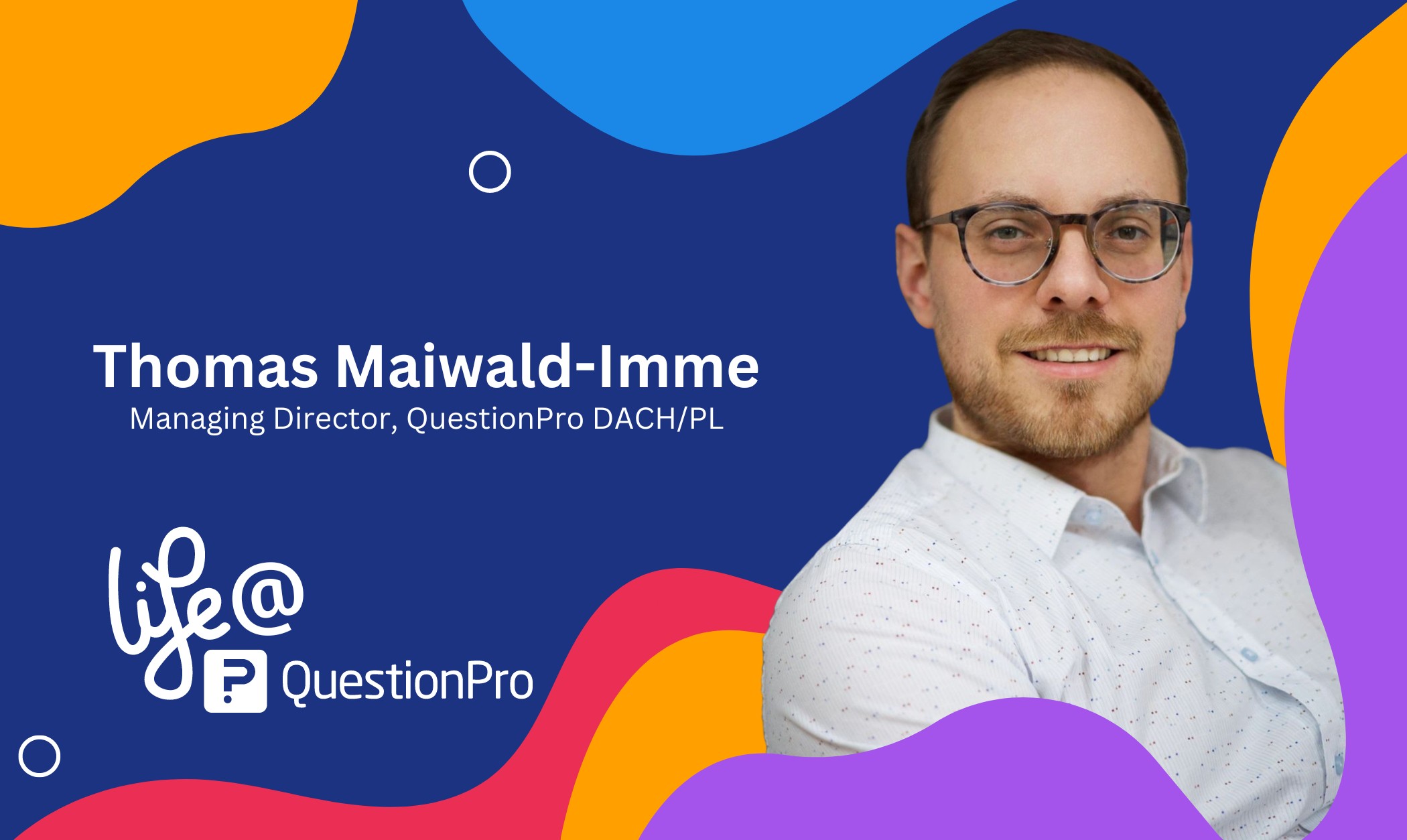
Life@QuestionPro: Thomas Maiwald-Immer’s Experience
Aug 9, 2024
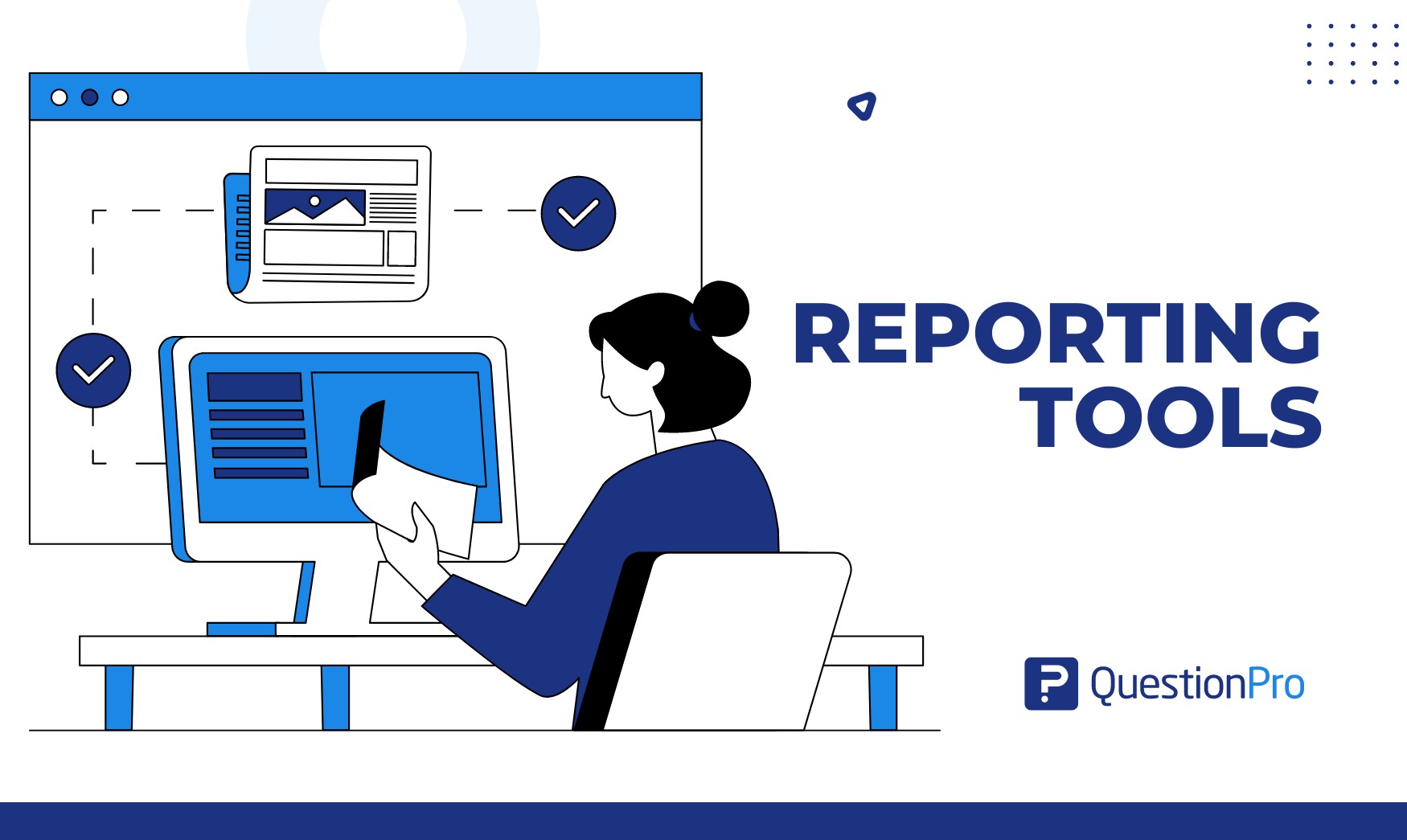
Top 13 Reporting Tools to Transform Your Data Insights & More
Aug 8, 2024
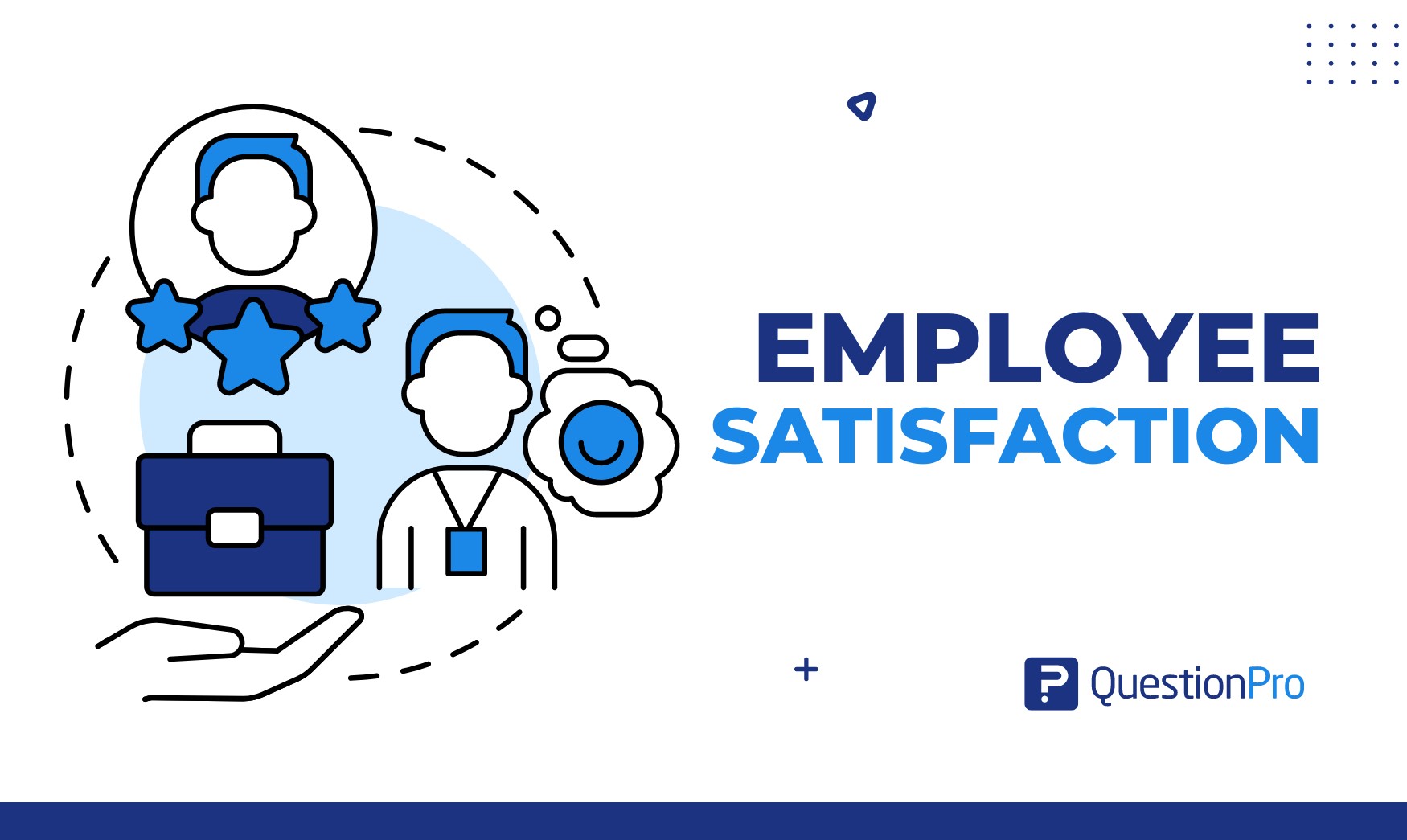
Employee Satisfaction: How to Boost Your Workplace Happiness?
Aug 7, 2024
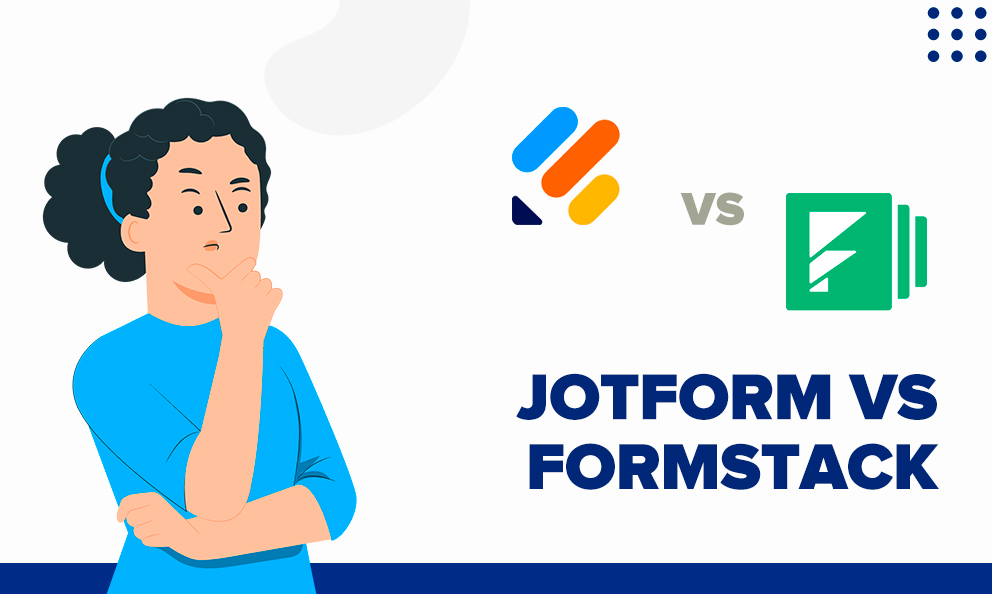
Jotform vs Formstack: Which Form Builder Should You Choose?
Other categories.
- Academic Research
- Artificial Intelligence
- Assessments
- Brand Awareness
- Case Studies
- Communities
- Consumer Insights
- Customer effort score
- Customer Engagement
- Customer Experience
- Customer Loyalty
- Customer Research
- Customer Satisfaction
- Employee Benefits
- Employee Engagement
- Employee Retention
- Friday Five
- General Data Protection Regulation
- Insights Hub
- Life@QuestionPro
- Market Research
- Mobile diaries
- Mobile Surveys
- New Features
- Online Communities
- Question Types
- Questionnaire
- QuestionPro Products
- Release Notes
- Research Tools and Apps
- Revenue at Risk
- Survey Templates
- Training Tips
- Tuesday CX Thoughts (TCXT)
- Uncategorized
- What’s Coming Up
- Workforce Intelligence
Have a language expert improve your writing
Run a free plagiarism check in 10 minutes, generate accurate citations for free.
- Knowledge Base
Methodology
- What Is Qualitative Research? | Methods & Examples
What Is Qualitative Research? | Methods & Examples
Published on June 19, 2020 by Pritha Bhandari . Revised on June 22, 2023.
Qualitative research involves collecting and analyzing non-numerical data (e.g., text, video, or audio) to understand concepts, opinions, or experiences. It can be used to gather in-depth insights into a problem or generate new ideas for research.
Qualitative research is the opposite of quantitative research , which involves collecting and analyzing numerical data for statistical analysis.
Qualitative research is commonly used in the humanities and social sciences, in subjects such as anthropology, sociology, education, health sciences, history, etc.
- How does social media shape body image in teenagers?
- How do children and adults interpret healthy eating in the UK?
- What factors influence employee retention in a large organization?
- How is anxiety experienced around the world?
- How can teachers integrate social issues into science curriculums?
Table of contents
Approaches to qualitative research, qualitative research methods, qualitative data analysis, advantages of qualitative research, disadvantages of qualitative research, other interesting articles, frequently asked questions about qualitative research.
Qualitative research is used to understand how people experience the world. While there are many approaches to qualitative research, they tend to be flexible and focus on retaining rich meaning when interpreting data.
Common approaches include grounded theory, ethnography , action research , phenomenological research, and narrative research. They share some similarities, but emphasize different aims and perspectives.
Approach | What does it involve? |
---|---|
Grounded theory | Researchers collect rich data on a topic of interest and develop theories . |
Researchers immerse themselves in groups or organizations to understand their cultures. | |
Action research | Researchers and participants collaboratively link theory to practice to drive social change. |
Phenomenological research | Researchers investigate a phenomenon or event by describing and interpreting participants’ lived experiences. |
Narrative research | Researchers examine how stories are told to understand how participants perceive and make sense of their experiences. |
Note that qualitative research is at risk for certain research biases including the Hawthorne effect , observer bias , recall bias , and social desirability bias . While not always totally avoidable, awareness of potential biases as you collect and analyze your data can prevent them from impacting your work too much.
Receive feedback on language, structure, and formatting
Professional editors proofread and edit your paper by focusing on:
- Academic style
- Vague sentences
- Style consistency
See an example
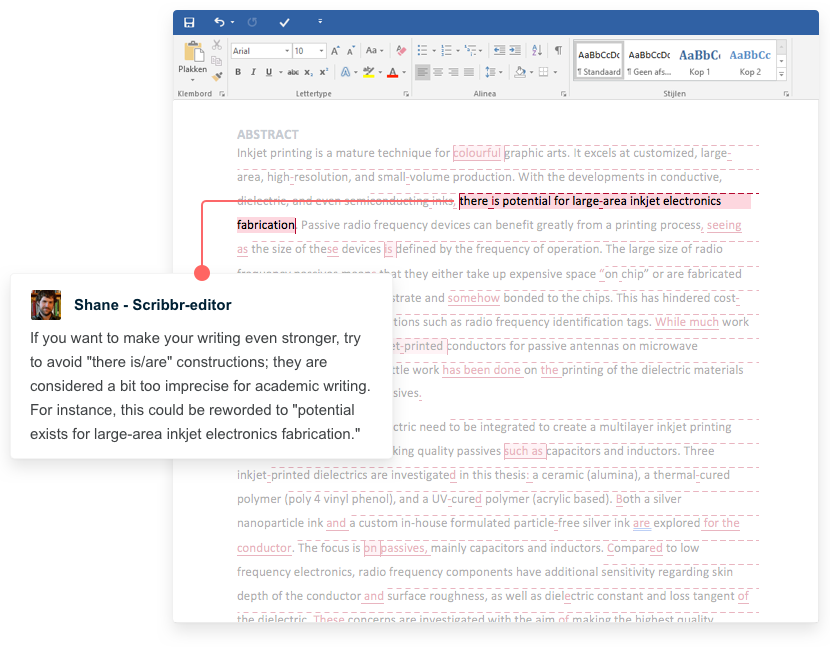
Each of the research approaches involve using one or more data collection methods . These are some of the most common qualitative methods:
- Observations: recording what you have seen, heard, or encountered in detailed field notes.
- Interviews: personally asking people questions in one-on-one conversations.
- Focus groups: asking questions and generating discussion among a group of people.
- Surveys : distributing questionnaires with open-ended questions.
- Secondary research: collecting existing data in the form of texts, images, audio or video recordings, etc.
- You take field notes with observations and reflect on your own experiences of the company culture.
- You distribute open-ended surveys to employees across all the company’s offices by email to find out if the culture varies across locations.
- You conduct in-depth interviews with employees in your office to learn about their experiences and perspectives in greater detail.
Qualitative researchers often consider themselves “instruments” in research because all observations, interpretations and analyses are filtered through their own personal lens.
For this reason, when writing up your methodology for qualitative research, it’s important to reflect on your approach and to thoroughly explain the choices you made in collecting and analyzing the data.
Qualitative data can take the form of texts, photos, videos and audio. For example, you might be working with interview transcripts, survey responses, fieldnotes, or recordings from natural settings.
Most types of qualitative data analysis share the same five steps:
- Prepare and organize your data. This may mean transcribing interviews or typing up fieldnotes.
- Review and explore your data. Examine the data for patterns or repeated ideas that emerge.
- Develop a data coding system. Based on your initial ideas, establish a set of codes that you can apply to categorize your data.
- Assign codes to the data. For example, in qualitative survey analysis, this may mean going through each participant’s responses and tagging them with codes in a spreadsheet. As you go through your data, you can create new codes to add to your system if necessary.
- Identify recurring themes. Link codes together into cohesive, overarching themes.
There are several specific approaches to analyzing qualitative data. Although these methods share similar processes, they emphasize different concepts.
Approach | When to use | Example |
---|---|---|
To describe and categorize common words, phrases, and ideas in qualitative data. | A market researcher could perform content analysis to find out what kind of language is used in descriptions of therapeutic apps. | |
To identify and interpret patterns and themes in qualitative data. | A psychologist could apply thematic analysis to travel blogs to explore how tourism shapes self-identity. | |
To examine the content, structure, and design of texts. | A media researcher could use textual analysis to understand how news coverage of celebrities has changed in the past decade. | |
To study communication and how language is used to achieve effects in specific contexts. | A political scientist could use discourse analysis to study how politicians generate trust in election campaigns. |
Qualitative research often tries to preserve the voice and perspective of participants and can be adjusted as new research questions arise. Qualitative research is good for:
- Flexibility
The data collection and analysis process can be adapted as new ideas or patterns emerge. They are not rigidly decided beforehand.
- Natural settings
Data collection occurs in real-world contexts or in naturalistic ways.
- Meaningful insights
Detailed descriptions of people’s experiences, feelings and perceptions can be used in designing, testing or improving systems or products.
- Generation of new ideas
Open-ended responses mean that researchers can uncover novel problems or opportunities that they wouldn’t have thought of otherwise.
Researchers must consider practical and theoretical limitations in analyzing and interpreting their data. Qualitative research suffers from:
- Unreliability
The real-world setting often makes qualitative research unreliable because of uncontrolled factors that affect the data.
- Subjectivity
Due to the researcher’s primary role in analyzing and interpreting data, qualitative research cannot be replicated . The researcher decides what is important and what is irrelevant in data analysis, so interpretations of the same data can vary greatly.
- Limited generalizability
Small samples are often used to gather detailed data about specific contexts. Despite rigorous analysis procedures, it is difficult to draw generalizable conclusions because the data may be biased and unrepresentative of the wider population .
- Labor-intensive
Although software can be used to manage and record large amounts of text, data analysis often has to be checked or performed manually.
If you want to know more about statistics , methodology , or research bias , make sure to check out some of our other articles with explanations and examples.
- Chi square goodness of fit test
- Degrees of freedom
- Null hypothesis
- Discourse analysis
- Control groups
- Mixed methods research
- Non-probability sampling
- Quantitative research
- Inclusion and exclusion criteria
Research bias
- Rosenthal effect
- Implicit bias
- Cognitive bias
- Selection bias
- Negativity bias
- Status quo bias
Quantitative research deals with numbers and statistics, while qualitative research deals with words and meanings.
Quantitative methods allow you to systematically measure variables and test hypotheses . Qualitative methods allow you to explore concepts and experiences in more detail.
There are five common approaches to qualitative research :
- Grounded theory involves collecting data in order to develop new theories.
- Ethnography involves immersing yourself in a group or organization to understand its culture.
- Narrative research involves interpreting stories to understand how people make sense of their experiences and perceptions.
- Phenomenological research involves investigating phenomena through people’s lived experiences.
- Action research links theory and practice in several cycles to drive innovative changes.
Data collection is the systematic process by which observations or measurements are gathered in research. It is used in many different contexts by academics, governments, businesses, and other organizations.
There are various approaches to qualitative data analysis , but they all share five steps in common:
- Prepare and organize your data.
- Review and explore your data.
- Develop a data coding system.
- Assign codes to the data.
- Identify recurring themes.
The specifics of each step depend on the focus of the analysis. Some common approaches include textual analysis , thematic analysis , and discourse analysis .
Cite this Scribbr article
If you want to cite this source, you can copy and paste the citation or click the “Cite this Scribbr article” button to automatically add the citation to our free Citation Generator.
Bhandari, P. (2023, June 22). What Is Qualitative Research? | Methods & Examples. Scribbr. Retrieved August 7, 2024, from https://www.scribbr.com/methodology/qualitative-research/
Is this article helpful?
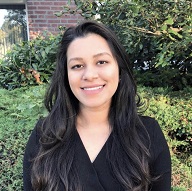
Pritha Bhandari
Other students also liked, qualitative vs. quantitative research | differences, examples & methods, how to do thematic analysis | step-by-step guide & examples, "i thought ai proofreading was useless but..".
I've been using Scribbr for years now and I know it's a service that won't disappoint. It does a good job spotting mistakes”
- AI & NLP
- Churn & Loyalty
- Customer Experience
- Customer Journeys
- Customer Metrics
- Feedback Analysis
- Product Experience
- Product Updates
- Sentiment Analysis
- Surveys & Feedback Collection
- Text Analytics
- Try Thematic
Welcome to the community
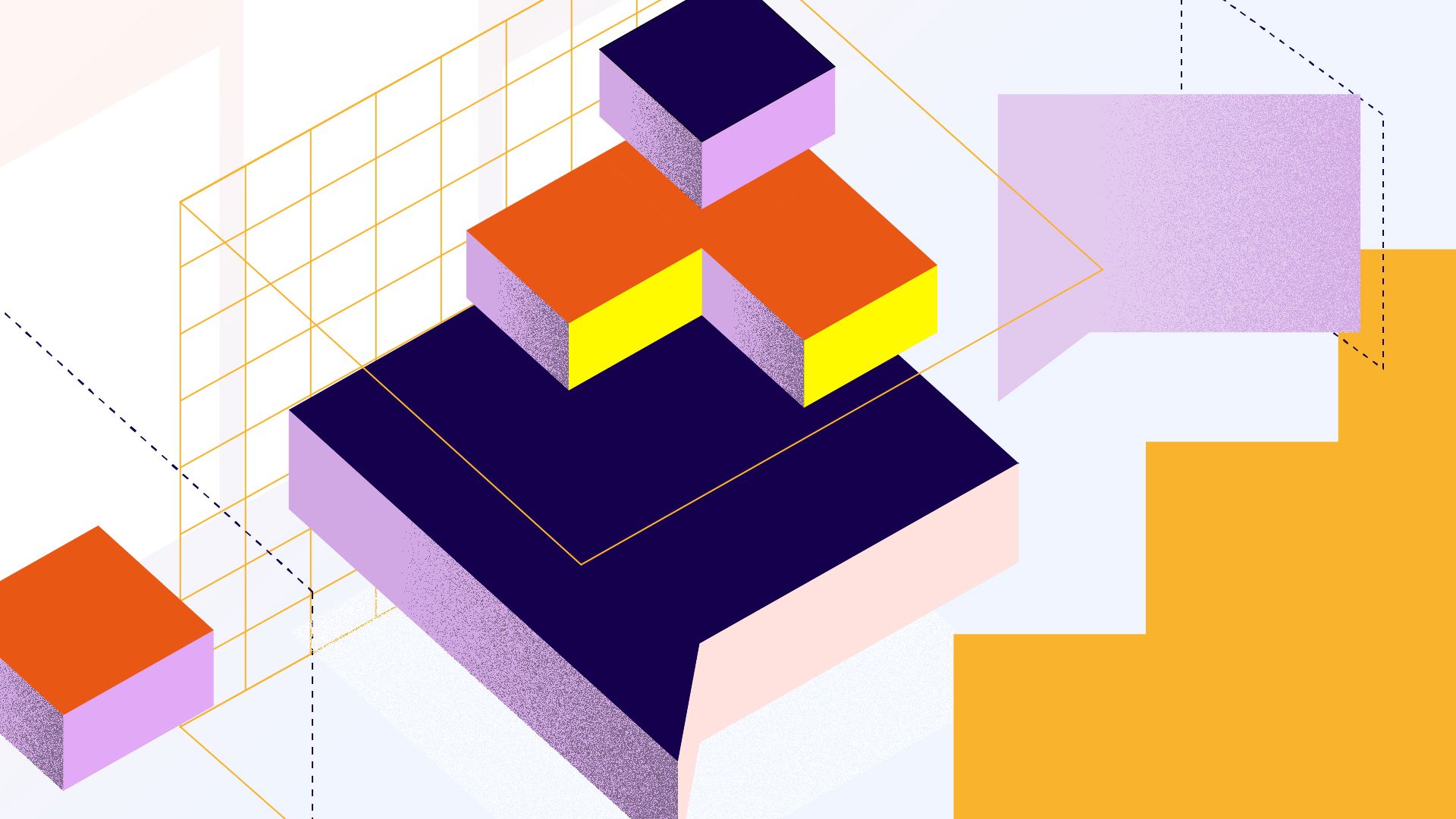
Qualitative Data Analysis: Step-by-Step Guide (Manual vs. Automatic)
When we conduct qualitative methods of research, need to explain changes in metrics or understand people's opinions, we always turn to qualitative data. Qualitative data is typically generated through:
- Interview transcripts
- Surveys with open-ended questions
- Contact center transcripts
- Texts and documents
- Audio and video recordings
- Observational notes
Compared to quantitative data, which captures structured information, qualitative data is unstructured and has more depth. It can answer our questions, can help formulate hypotheses and build understanding.
It's important to understand the differences between quantitative data & qualitative data . But unfortunately, analyzing qualitative data is difficult. While tools like Excel, Tableau and PowerBI crunch and visualize quantitative data with ease, there are a limited number of mainstream tools for analyzing qualitative data . The majority of qualitative data analysis still happens manually.
That said, there are two new trends that are changing this. First, there are advances in natural language processing (NLP) which is focused on understanding human language. Second, there is an explosion of user-friendly software designed for both researchers and businesses. Both help automate the qualitative data analysis process.
In this post we want to teach you how to conduct a successful qualitative data analysis. There are two primary qualitative data analysis methods; manual & automatic. We will teach you how to conduct the analysis manually, and also, automatically using software solutions powered by NLP. We’ll guide you through the steps to conduct a manual analysis, and look at what is involved and the role technology can play in automating this process.
More businesses are switching to fully-automated analysis of qualitative customer data because it is cheaper, faster, and just as accurate. Primarily, businesses purchase subscriptions to feedback analytics platforms so that they can understand customer pain points and sentiment.
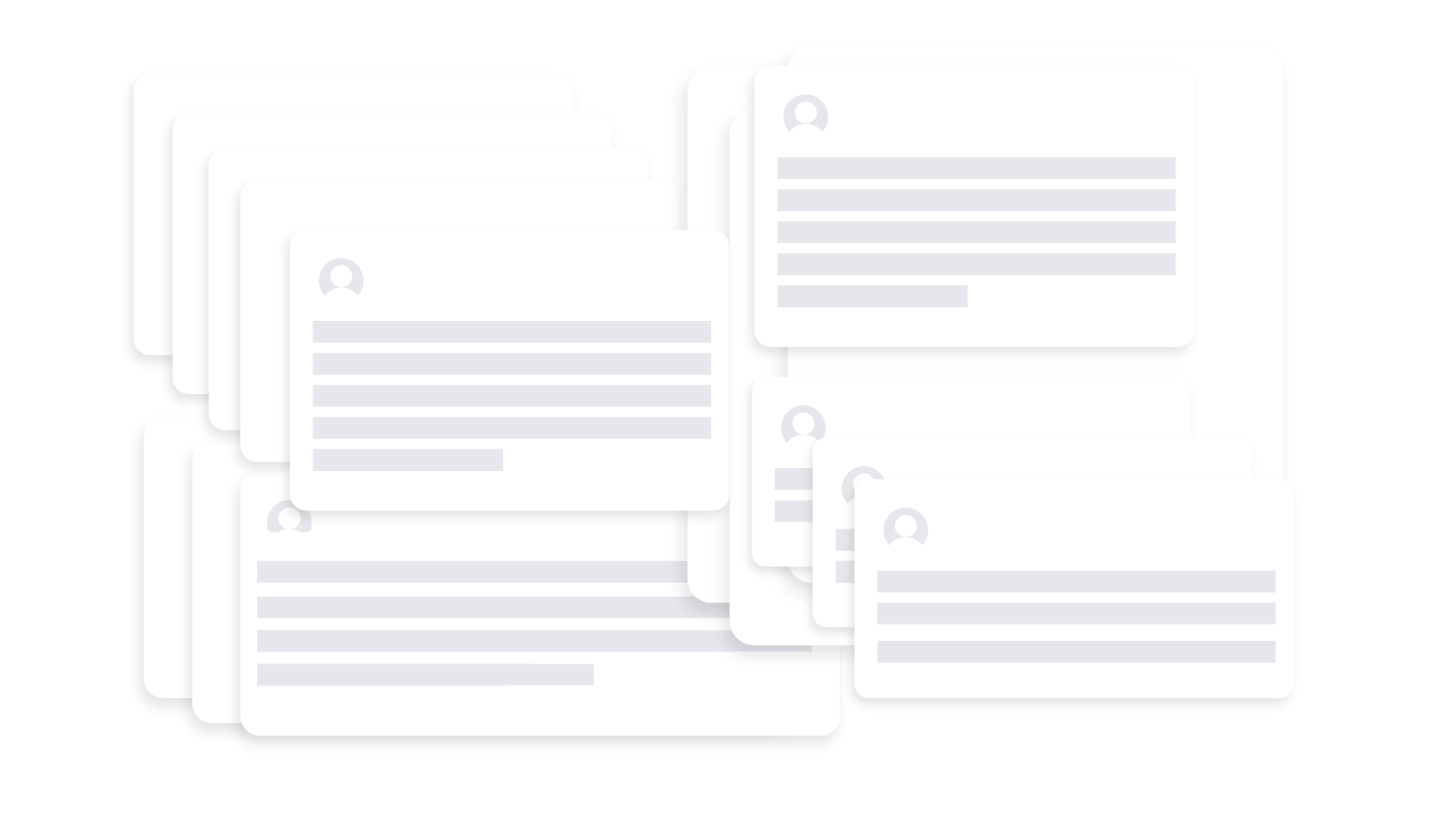
We’ll take you through 5 steps to conduct a successful qualitative data analysis. Within each step we will highlight the key difference between the manual, and automated approach of qualitative researchers. Here's an overview of the steps:
The 5 steps to doing qualitative data analysis
- Gathering and collecting your qualitative data
- Organizing and connecting into your qualitative data
- Coding your qualitative data
- Analyzing the qualitative data for insights
- Reporting on the insights derived from your analysis
What is Qualitative Data Analysis?
Qualitative data analysis is a process of gathering, structuring and interpreting qualitative data to understand what it represents.
Qualitative data is non-numerical and unstructured. Qualitative data generally refers to text, such as open-ended responses to survey questions or user interviews, but also includes audio, photos and video.
Businesses often perform qualitative data analysis on customer feedback. And within this context, qualitative data generally refers to verbatim text data collected from sources such as reviews, complaints, chat messages, support centre interactions, customer interviews, case notes or social media comments.
How is qualitative data analysis different from quantitative data analysis?
Understanding the differences between quantitative & qualitative data is important. When it comes to analyzing data, Qualitative Data Analysis serves a very different role to Quantitative Data Analysis. But what sets them apart?
Qualitative Data Analysis dives into the stories hidden in non-numerical data such as interviews, open-ended survey answers, or notes from observations. It uncovers the ‘whys’ and ‘hows’ giving a deep understanding of people’s experiences and emotions.
Quantitative Data Analysis on the other hand deals with numerical data, using statistics to measure differences, identify preferred options, and pinpoint root causes of issues. It steps back to address questions like "how many" or "what percentage" to offer broad insights we can apply to larger groups.
In short, Qualitative Data Analysis is like a microscope, helping us understand specific detail. Quantitative Data Analysis is like the telescope, giving us a broader perspective. Both are important, working together to decode data for different objectives.
Qualitative Data Analysis methods
Once all the data has been captured, there are a variety of analysis techniques available and the choice is determined by your specific research objectives and the kind of data you’ve gathered. Common qualitative data analysis methods include:
Content Analysis
This is a popular approach to qualitative data analysis. Other qualitative analysis techniques may fit within the broad scope of content analysis. Thematic analysis is a part of the content analysis. Content analysis is used to identify the patterns that emerge from text, by grouping content into words, concepts, and themes. Content analysis is useful to quantify the relationship between all of the grouped content. The Columbia School of Public Health has a detailed breakdown of content analysis .
Narrative Analysis
Narrative analysis focuses on the stories people tell and the language they use to make sense of them. It is particularly useful in qualitative research methods where customer stories are used to get a deep understanding of customers’ perspectives on a specific issue. A narrative analysis might enable us to summarize the outcomes of a focused case study.
Discourse Analysis
Discourse analysis is used to get a thorough understanding of the political, cultural and power dynamics that exist in specific situations. The focus of discourse analysis here is on the way people express themselves in different social contexts. Discourse analysis is commonly used by brand strategists who hope to understand why a group of people feel the way they do about a brand or product.
Thematic Analysis
Thematic analysis is used to deduce the meaning behind the words people use. This is accomplished by discovering repeating themes in text. These meaningful themes reveal key insights into data and can be quantified, particularly when paired with sentiment analysis . Often, the outcome of thematic analysis is a code frame that captures themes in terms of codes, also called categories. So the process of thematic analysis is also referred to as “coding”. A common use-case for thematic analysis in companies is analysis of customer feedback.
Grounded Theory
Grounded theory is a useful approach when little is known about a subject. Grounded theory starts by formulating a theory around a single data case. This means that the theory is “grounded”. Grounded theory analysis is based on actual data, and not entirely speculative. Then additional cases can be examined to see if they are relevant and can add to the original grounded theory.
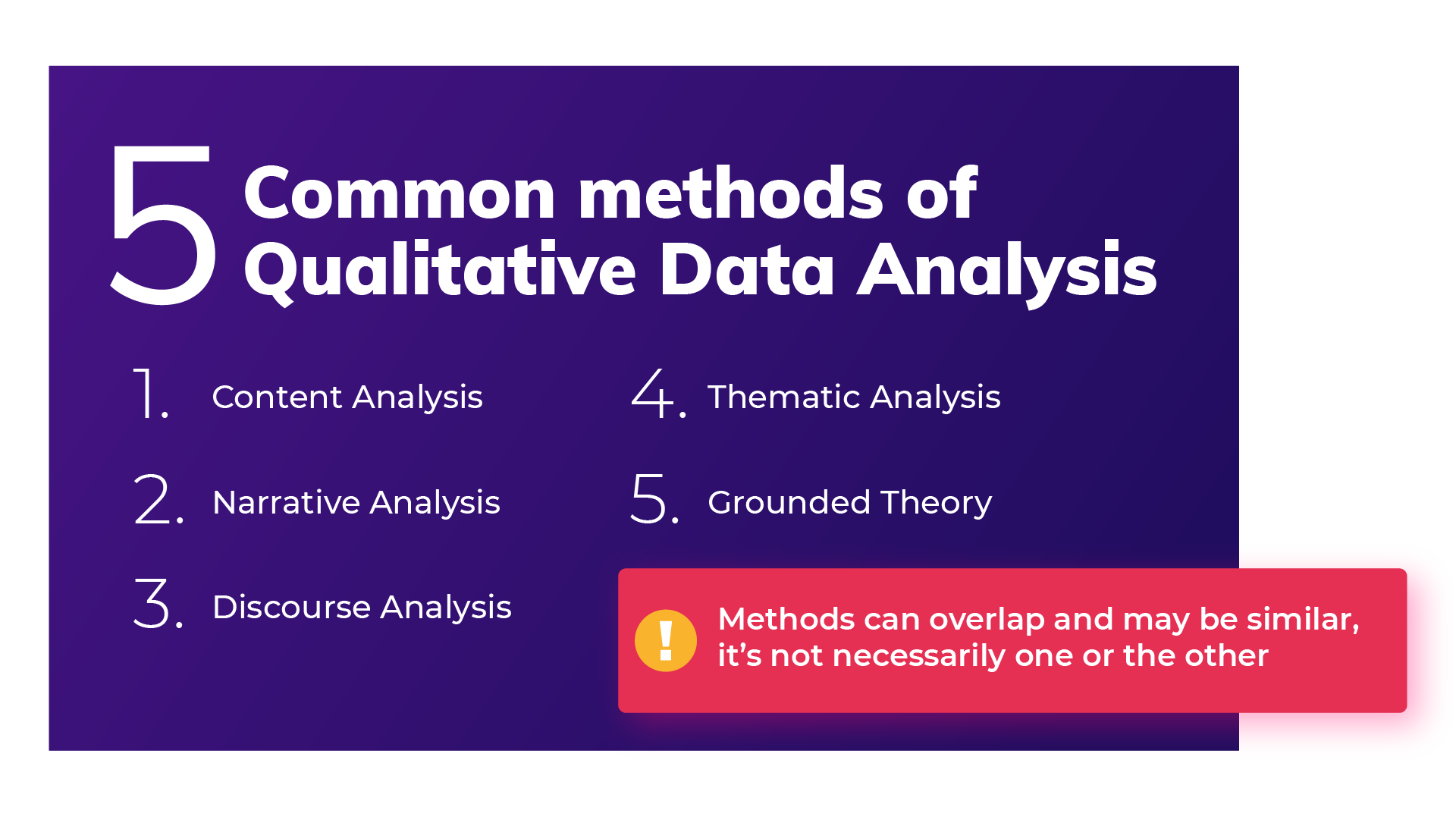
Challenges of Qualitative Data Analysis
While Qualitative Data Analysis offers rich insights, it comes with its challenges. Each unique QDA method has its unique hurdles. Let’s take a look at the challenges researchers and analysts might face, depending on the chosen method.
- Time and Effort (Narrative Analysis): Narrative analysis, which focuses on personal stories, demands patience. Sifting through lengthy narratives to find meaningful insights can be time-consuming, requires dedicated effort.
- Being Objective (Grounded Theory): Grounded theory, building theories from data, faces the challenges of personal biases. Staying objective while interpreting data is crucial, ensuring conclusions are rooted in the data itself.
- Complexity (Thematic Analysis): Thematic analysis involves identifying themes within data, a process that can be intricate. Categorizing and understanding themes can be complex, especially when each piece of data varies in context and structure. Thematic Analysis software can simplify this process.
- Generalizing Findings (Narrative Analysis): Narrative analysis, dealing with individual stories, makes drawing broad challenging. Extending findings from a single narrative to a broader context requires careful consideration.
- Managing Data (Thematic Analysis): Thematic analysis involves organizing and managing vast amounts of unstructured data, like interview transcripts. Managing this can be a hefty task, requiring effective data management strategies.
- Skill Level (Grounded Theory): Grounded theory demands specific skills to build theories from the ground up. Finding or training analysts with these skills poses a challenge, requiring investment in building expertise.
Benefits of qualitative data analysis
Qualitative Data Analysis (QDA) is like a versatile toolkit, offering a tailored approach to understanding your data. The benefits it offers are as diverse as the methods. Let’s explore why choosing the right method matters.
- Tailored Methods for Specific Needs: QDA isn't one-size-fits-all. Depending on your research objectives and the type of data at hand, different methods offer unique benefits. If you want emotive customer stories, narrative analysis paints a strong picture. When you want to explain a score, thematic analysis reveals insightful patterns
- Flexibility with Thematic Analysis: thematic analysis is like a chameleon in the toolkit of QDA. It adapts well to different types of data and research objectives, making it a top choice for any qualitative analysis.
- Deeper Understanding, Better Products: QDA helps you dive into people's thoughts and feelings. This deep understanding helps you build products and services that truly matches what people want, ensuring satisfied customers
- Finding the Unexpected: Qualitative data often reveals surprises that we miss in quantitative data. QDA offers us new ideas and perspectives, for insights we might otherwise miss.
- Building Effective Strategies: Insights from QDA are like strategic guides. They help businesses in crafting plans that match people’s desires.
- Creating Genuine Connections: Understanding people’s experiences lets businesses connect on a real level. This genuine connection helps build trust and loyalty, priceless for any business.
How to do Qualitative Data Analysis: 5 steps
Now we are going to show how you can do your own qualitative data analysis. We will guide you through this process step by step. As mentioned earlier, you will learn how to do qualitative data analysis manually , and also automatically using modern qualitative data and thematic analysis software.
To get best value from the analysis process and research process, it’s important to be super clear about the nature and scope of the question that’s being researched. This will help you select the research collection channels that are most likely to help you answer your question.
Depending on if you are a business looking to understand customer sentiment, or an academic surveying a school, your approach to qualitative data analysis will be unique.
Once you’re clear, there’s a sequence to follow. And, though there are differences in the manual and automatic approaches, the process steps are mostly the same.
The use case for our step-by-step guide is a company looking to collect data (customer feedback data), and analyze the customer feedback - in order to improve customer experience. By analyzing the customer feedback the company derives insights about their business and their customers. You can follow these same steps regardless of the nature of your research. Let’s get started.
Step 1: Gather your qualitative data and conduct research (Conduct qualitative research)
The first step of qualitative research is to do data collection. Put simply, data collection is gathering all of your data for analysis. A common situation is when qualitative data is spread across various sources.
Classic methods of gathering qualitative data
Most companies use traditional methods for gathering qualitative data: conducting interviews with research participants, running surveys, and running focus groups. This data is typically stored in documents, CRMs, databases and knowledge bases. It’s important to examine which data is available and needs to be included in your research project, based on its scope.
Using your existing qualitative feedback
As it becomes easier for customers to engage across a range of different channels, companies are gathering increasingly large amounts of both solicited and unsolicited qualitative feedback.
Most organizations have now invested in Voice of Customer programs , support ticketing systems, chatbot and support conversations, emails and even customer Slack chats.
These new channels provide companies with new ways of getting feedback, and also allow the collection of unstructured feedback data at scale.
The great thing about this data is that it contains a wealth of valubale insights and that it’s already there! When you have a new question about user behavior or your customers, you don’t need to create a new research study or set up a focus group. You can find most answers in the data you already have.
Typically, this data is stored in third-party solutions or a central database, but there are ways to export it or connect to a feedback analysis solution through integrations or an API.
Utilize untapped qualitative data channels
There are many online qualitative data sources you may not have considered. For example, you can find useful qualitative data in social media channels like Twitter or Facebook. Online forums, review sites, and online communities such as Discourse or Reddit also contain valuable data about your customers, or research questions.
If you are considering performing a qualitative benchmark analysis against competitors - the internet is your best friend, and review analysis is a great place to start. Gathering feedback in competitor reviews on sites like Trustpilot, G2, Capterra, Better Business Bureau or on app stores is a great way to perform a competitor benchmark analysis.
Customer feedback analysis software often has integrations into social media and review sites, or you could use a solution like DataMiner to scrape the reviews.
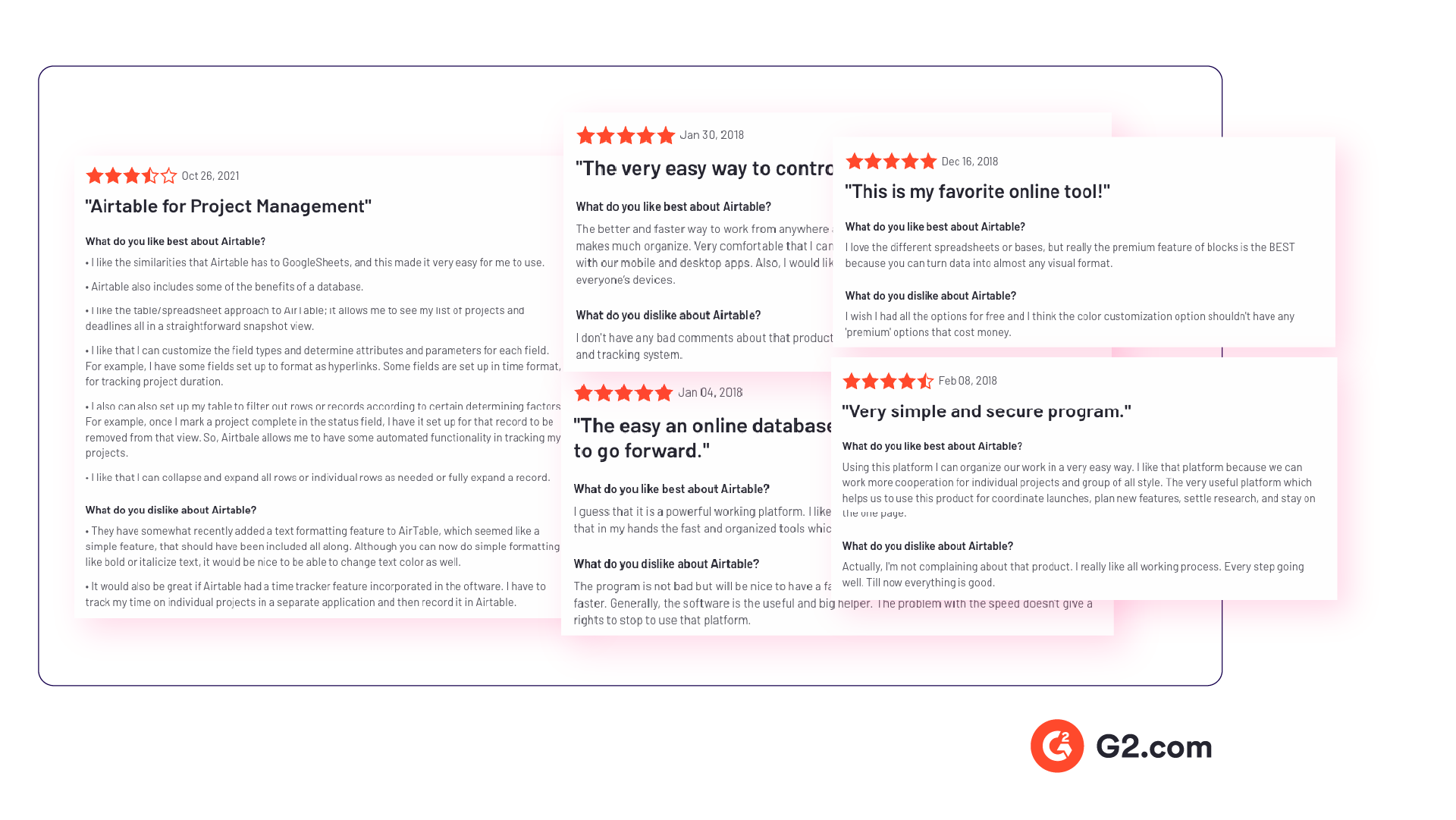
Step 2: Connect & organize all your qualitative data
Now you all have this qualitative data but there’s a problem, the data is unstructured. Before feedback can be analyzed and assigned any value, it needs to be organized in a single place. Why is this important? Consistency!
If all data is easily accessible in one place and analyzed in a consistent manner, you will have an easier time summarizing and making decisions based on this data.
The manual approach to organizing your data
The classic method of structuring qualitative data is to plot all the raw data you’ve gathered into a spreadsheet.
Typically, research and support teams would share large Excel sheets and different business units would make sense of the qualitative feedback data on their own. Each team collects and organizes the data in a way that best suits them, which means the feedback tends to be kept in separate silos.
An alternative and a more robust solution is to store feedback in a central database, like Snowflake or Amazon Redshift .
Keep in mind that when you organize your data in this way, you are often preparing it to be imported into another software. If you go the route of a database, you would need to use an API to push the feedback into a third-party software.
Computer-assisted qualitative data analysis software (CAQDAS)
Traditionally within the manual analysis approach (but not always), qualitative data is imported into CAQDAS software for coding.
In the early 2000s, CAQDAS software was popularised by developers such as ATLAS.ti, NVivo and MAXQDA and eagerly adopted by researchers to assist with the organizing and coding of data.
The benefits of using computer-assisted qualitative data analysis software:
- Assists in the organizing of your data
- Opens you up to exploring different interpretations of your data analysis
- Allows you to share your dataset easier and allows group collaboration (allows for secondary analysis)
However you still need to code the data, uncover the themes and do the analysis yourself. Therefore it is still a manual approach.
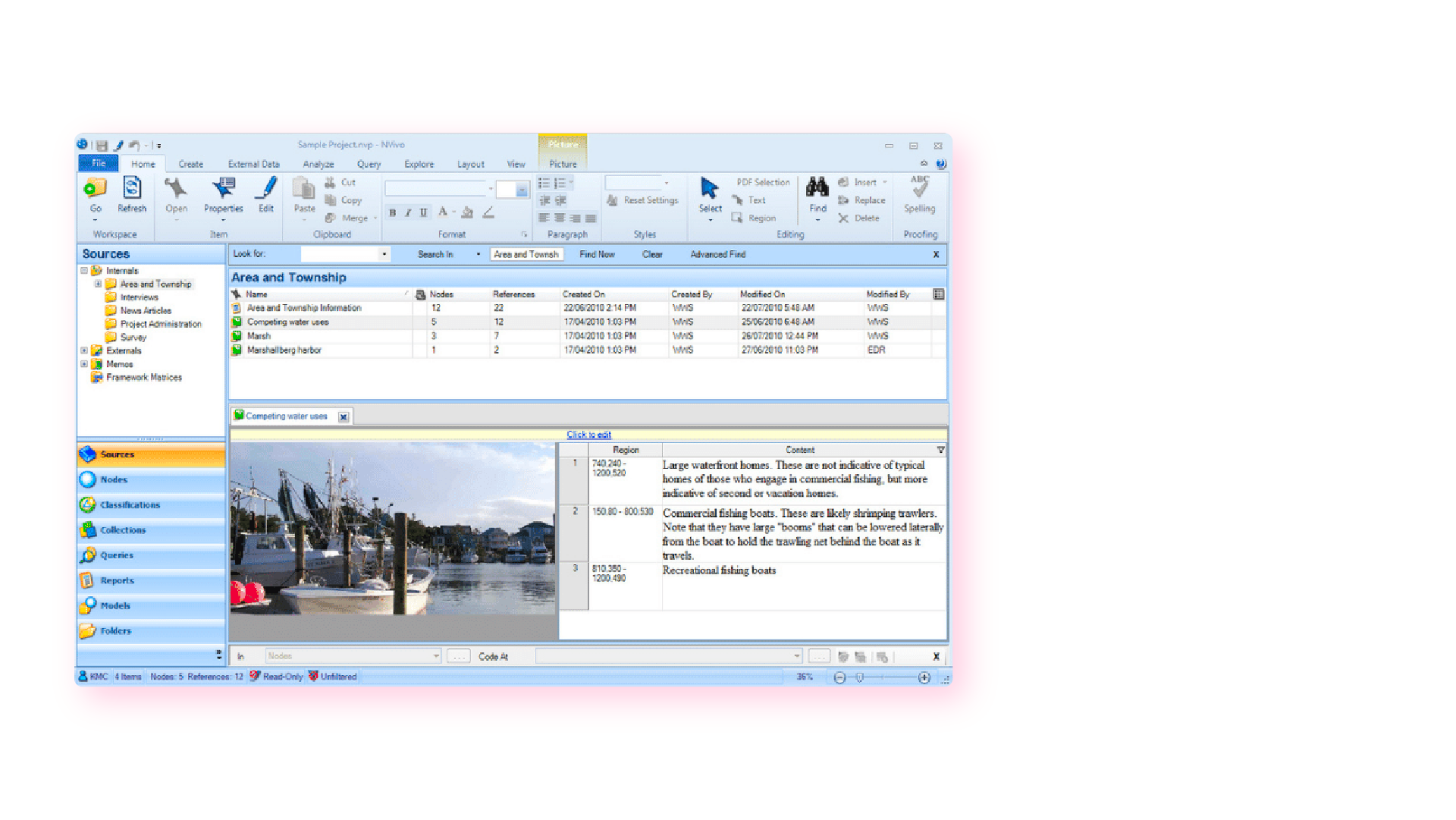
Organizing your qualitative data in a feedback repository
Another solution to organizing your qualitative data is to upload it into a feedback repository where it can be unified with your other data , and easily searchable and taggable. There are a number of software solutions that act as a central repository for your qualitative research data. Here are a couple solutions that you could investigate:
- Dovetail: Dovetail is a research repository with a focus on video and audio transcriptions. You can tag your transcriptions within the platform for theme analysis. You can also upload your other qualitative data such as research reports, survey responses, support conversations ( conversational analytics ), and customer interviews. Dovetail acts as a single, searchable repository. And makes it easier to collaborate with other people around your qualitative research.
- EnjoyHQ: EnjoyHQ is another research repository with similar functionality to Dovetail. It boasts a more sophisticated search engine, but it has a higher starting subscription cost.
Organizing your qualitative data in a feedback analytics platform
If you have a lot of qualitative customer or employee feedback, from the likes of customer surveys or employee surveys, you will benefit from a feedback analytics platform. A feedback analytics platform is a software that automates the process of both sentiment analysis and thematic analysis . Companies use the integrations offered by these platforms to directly tap into their qualitative data sources (review sites, social media, survey responses, etc.). The data collected is then organized and analyzed consistently within the platform.
If you have data prepared in a spreadsheet, it can also be imported into feedback analytics platforms.
Once all this rich data has been organized within the feedback analytics platform, it is ready to be coded and themed, within the same platform. Thematic is a feedback analytics platform that offers one of the largest libraries of integrations with qualitative data sources.
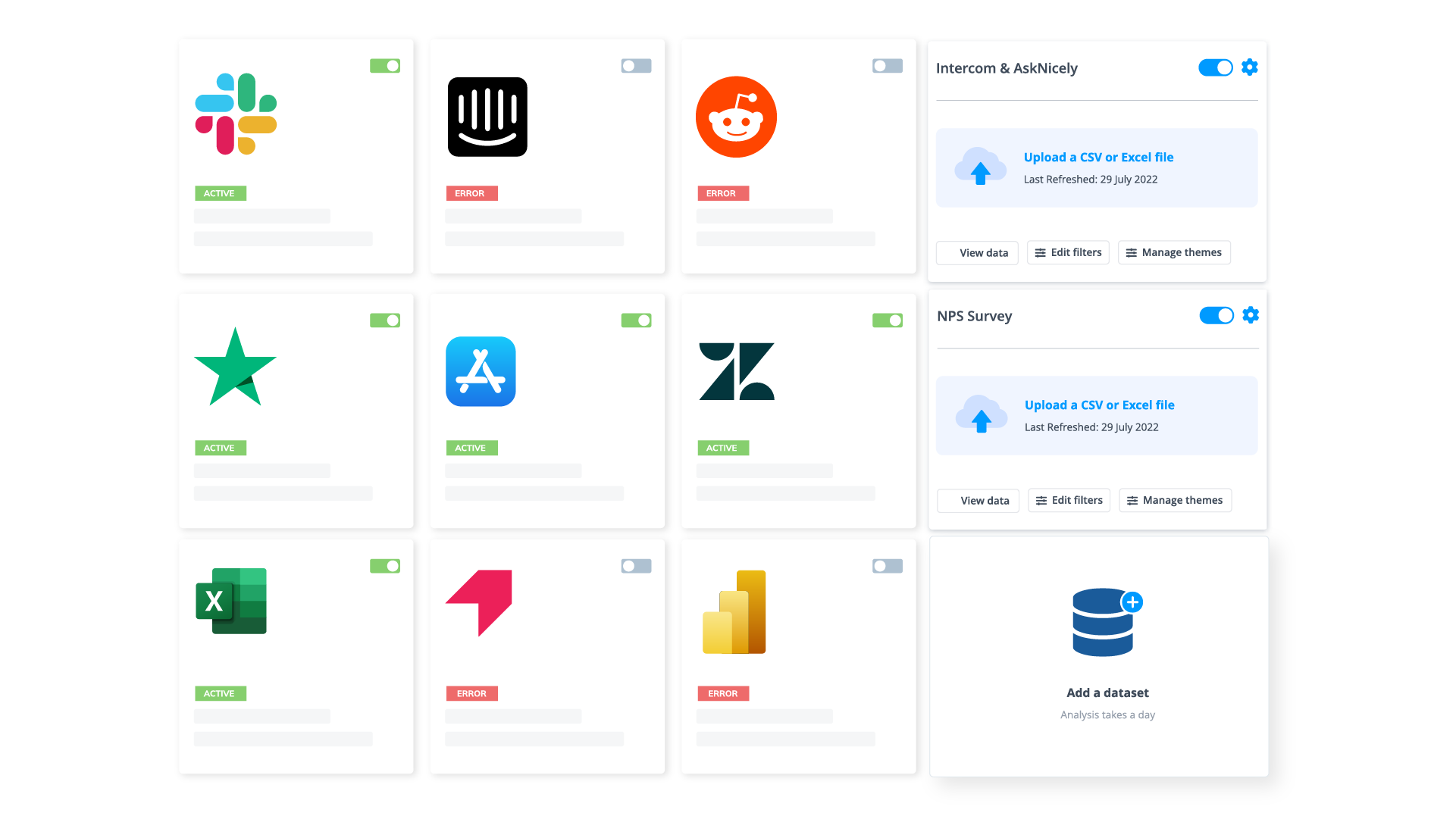
Step 3: Coding your qualitative data
Your feedback data is now organized in one place. Either within your spreadsheet, CAQDAS, feedback repository or within your feedback analytics platform. The next step is to code your feedback data so we can extract meaningful insights in the next step.
Coding is the process of labelling and organizing your data in such a way that you can then identify themes in the data, and the relationships between these themes.
To simplify the coding process, you will take small samples of your customer feedback data, come up with a set of codes, or categories capturing themes, and label each piece of feedback, systematically, for patterns and meaning. Then you will take a larger sample of data, revising and refining the codes for greater accuracy and consistency as you go.
If you choose to use a feedback analytics platform, much of this process will be automated and accomplished for you.
The terms to describe different categories of meaning (‘theme’, ‘code’, ‘tag’, ‘category’ etc) can be confusing as they are often used interchangeably. For clarity, this article will use the term ‘code’.
To code means to identify key words or phrases and assign them to a category of meaning. “I really hate the customer service of this computer software company” would be coded as “poor customer service”.
How to manually code your qualitative data
- Decide whether you will use deductive or inductive coding. Deductive coding is when you create a list of predefined codes, and then assign them to the qualitative data. Inductive coding is the opposite of this, you create codes based on the data itself. Codes arise directly from the data and you label them as you go. You need to weigh up the pros and cons of each coding method and select the most appropriate.
- Read through the feedback data to get a broad sense of what it reveals. Now it’s time to start assigning your first set of codes to statements and sections of text.
- Keep repeating step 2, adding new codes and revising the code description as often as necessary. Once it has all been coded, go through everything again, to be sure there are no inconsistencies and that nothing has been overlooked.
- Create a code frame to group your codes. The coding frame is the organizational structure of all your codes. And there are two commonly used types of coding frames, flat, or hierarchical. A hierarchical code frame will make it easier for you to derive insights from your analysis.
- Based on the number of times a particular code occurs, you can now see the common themes in your feedback data. This is insightful! If ‘bad customer service’ is a common code, it’s time to take action.
We have a detailed guide dedicated to manually coding your qualitative data .
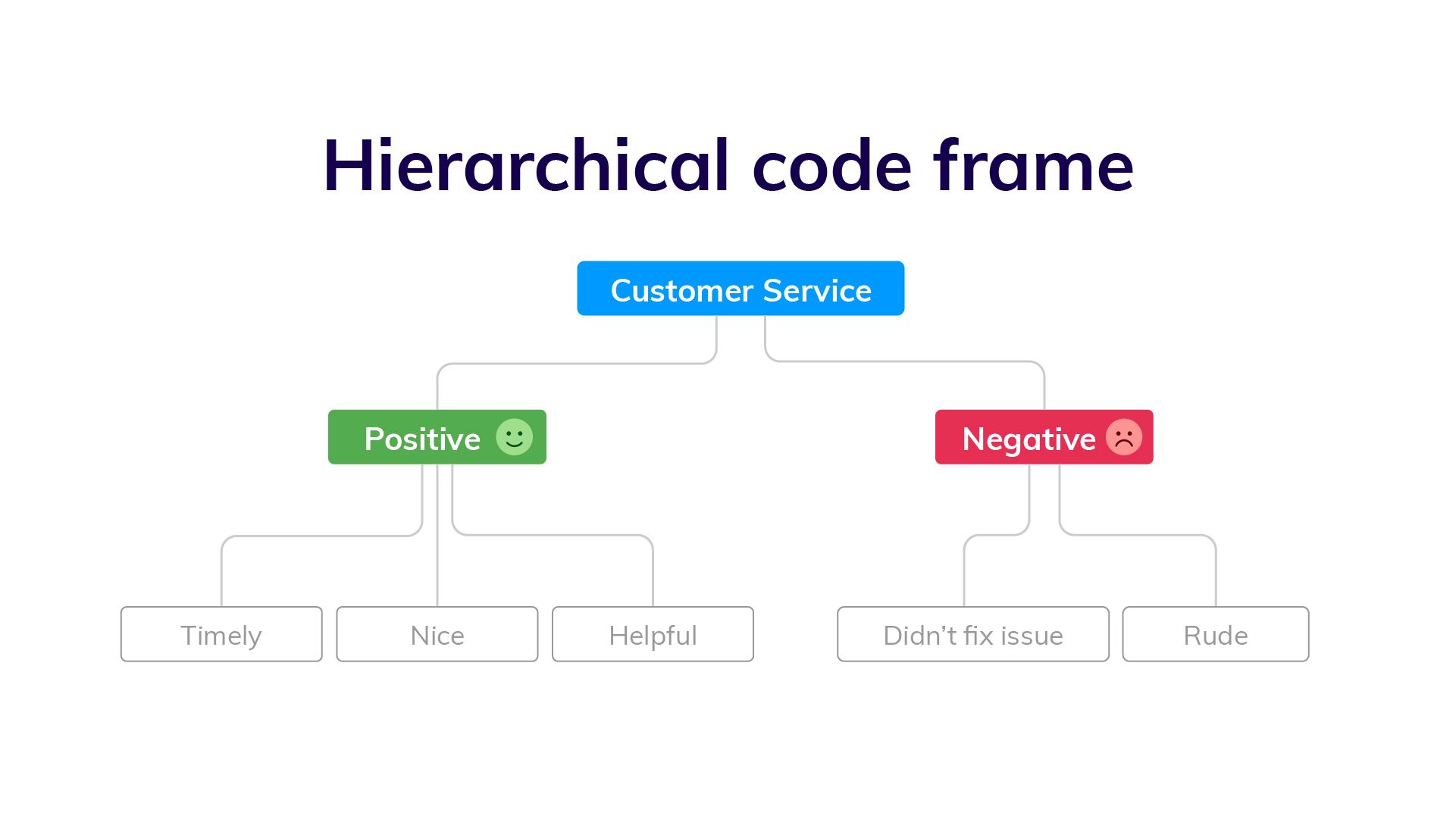
Using software to speed up manual coding of qualitative data
An Excel spreadsheet is still a popular method for coding. But various software solutions can help speed up this process. Here are some examples.
- CAQDAS / NVivo - CAQDAS software has built-in functionality that allows you to code text within their software. You may find the interface the software offers easier for managing codes than a spreadsheet.
- Dovetail/EnjoyHQ - You can tag transcripts and other textual data within these solutions. As they are also repositories you may find it simpler to keep the coding in one platform.
- IBM SPSS - SPSS is a statistical analysis software that may make coding easier than in a spreadsheet.
- Ascribe - Ascribe’s ‘Coder’ is a coding management system. Its user interface will make it easier for you to manage your codes.
Automating the qualitative coding process using thematic analysis software
In solutions which speed up the manual coding process, you still have to come up with valid codes and often apply codes manually to pieces of feedback. But there are also solutions that automate both the discovery and the application of codes.
Advances in machine learning have now made it possible to read, code and structure qualitative data automatically. This type of automated coding is offered by thematic analysis software .
Automation makes it far simpler and faster to code the feedback and group it into themes. By incorporating natural language processing (NLP) into the software, the AI looks across sentences and phrases to identify common themes meaningful statements. Some automated solutions detect repeating patterns and assign codes to them, others make you train the AI by providing examples. You could say that the AI learns the meaning of the feedback on its own.
Thematic automates the coding of qualitative feedback regardless of source. There’s no need to set up themes or categories in advance. Simply upload your data and wait a few minutes. You can also manually edit the codes to further refine their accuracy. Experiments conducted indicate that Thematic’s automated coding is just as accurate as manual coding .
Paired with sentiment analysis and advanced text analytics - these automated solutions become powerful for deriving quality business or research insights.
You could also build your own , if you have the resources!
The key benefits of using an automated coding solution
Automated analysis can often be set up fast and there’s the potential to uncover things that would never have been revealed if you had given the software a prescribed list of themes to look for.
Because the model applies a consistent rule to the data, it captures phrases or statements that a human eye might have missed.
Complete and consistent analysis of customer feedback enables more meaningful findings. Leading us into step 4.
Step 4: Analyze your data: Find meaningful insights
Now we are going to analyze our data to find insights. This is where we start to answer our research questions. Keep in mind that step 4 and step 5 (tell the story) have some overlap . This is because creating visualizations is both part of analysis process and reporting.
The task of uncovering insights is to scour through the codes that emerge from the data and draw meaningful correlations from them. It is also about making sure each insight is distinct and has enough data to support it.
Part of the analysis is to establish how much each code relates to different demographics and customer profiles, and identify whether there’s any relationship between these data points.
Manually create sub-codes to improve the quality of insights
If your code frame only has one level, you may find that your codes are too broad to be able to extract meaningful insights. This is where it is valuable to create sub-codes to your primary codes. This process is sometimes referred to as meta coding.
Note: If you take an inductive coding approach, you can create sub-codes as you are reading through your feedback data and coding it.
While time-consuming, this exercise will improve the quality of your analysis. Here is an example of what sub-codes could look like.
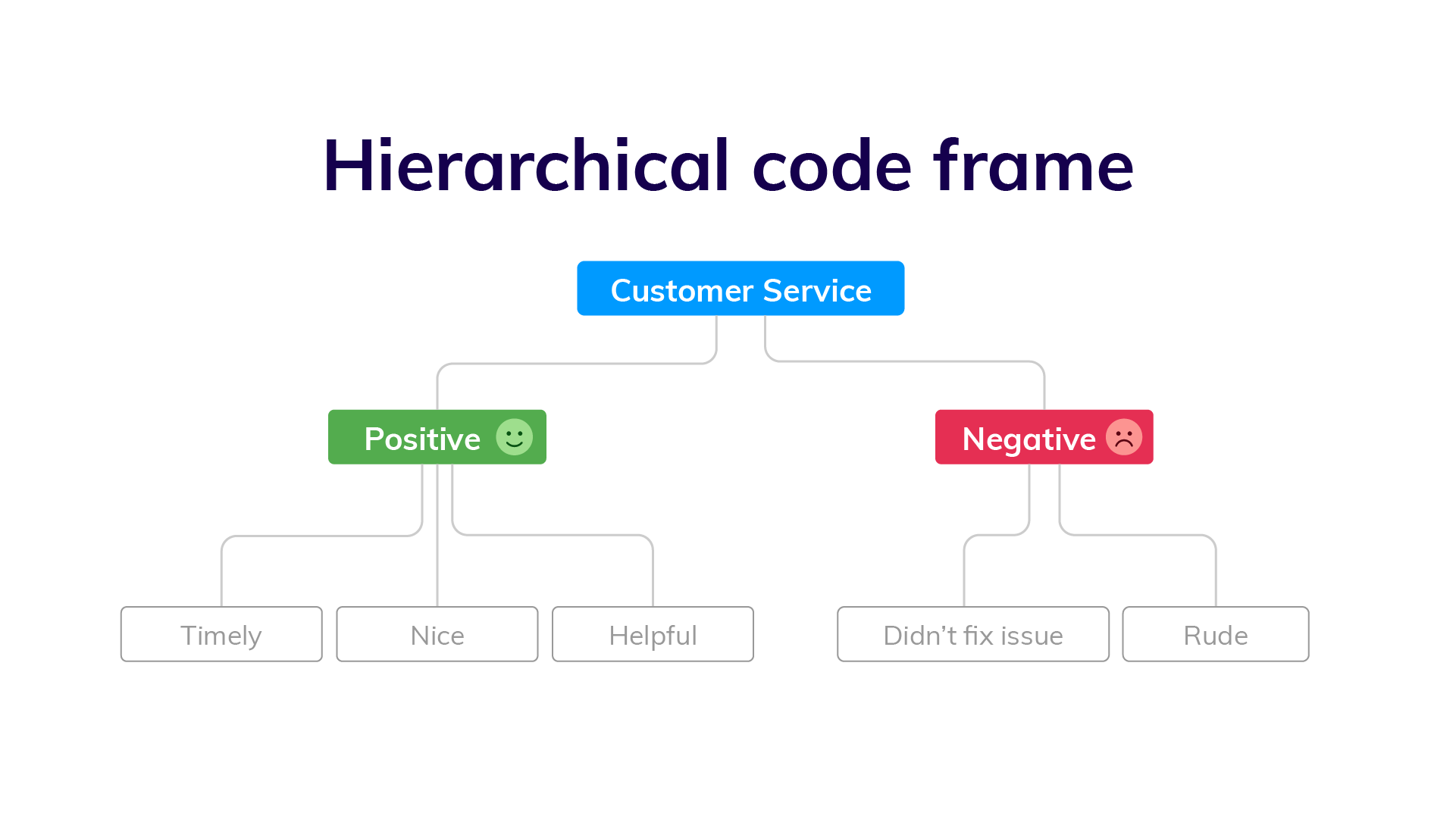
You need to carefully read your qualitative data to create quality sub-codes. But as you can see, the depth of analysis is greatly improved. By calculating the frequency of these sub-codes you can get insight into which customer service problems you can immediately address.
Correlate the frequency of codes to customer segments
Many businesses use customer segmentation . And you may have your own respondent segments that you can apply to your qualitative analysis. Segmentation is the practise of dividing customers or research respondents into subgroups.
Segments can be based on:
- Demographic
- And any other data type that you care to segment by
It is particularly useful to see the occurrence of codes within your segments. If one of your customer segments is considered unimportant to your business, but they are the cause of nearly all customer service complaints, it may be in your best interest to focus attention elsewhere. This is a useful insight!
Manually visualizing coded qualitative data
There are formulas you can use to visualize key insights in your data. The formulas we will suggest are imperative if you are measuring a score alongside your feedback.
If you are collecting a metric alongside your qualitative data this is a key visualization. Impact answers the question: “What’s the impact of a code on my overall score?”. Using Net Promoter Score (NPS) as an example, first you need to:
- Calculate overall NPS
- Calculate NPS in the subset of responses that do not contain that theme
- Subtract B from A
Then you can use this simple formula to calculate code impact on NPS .
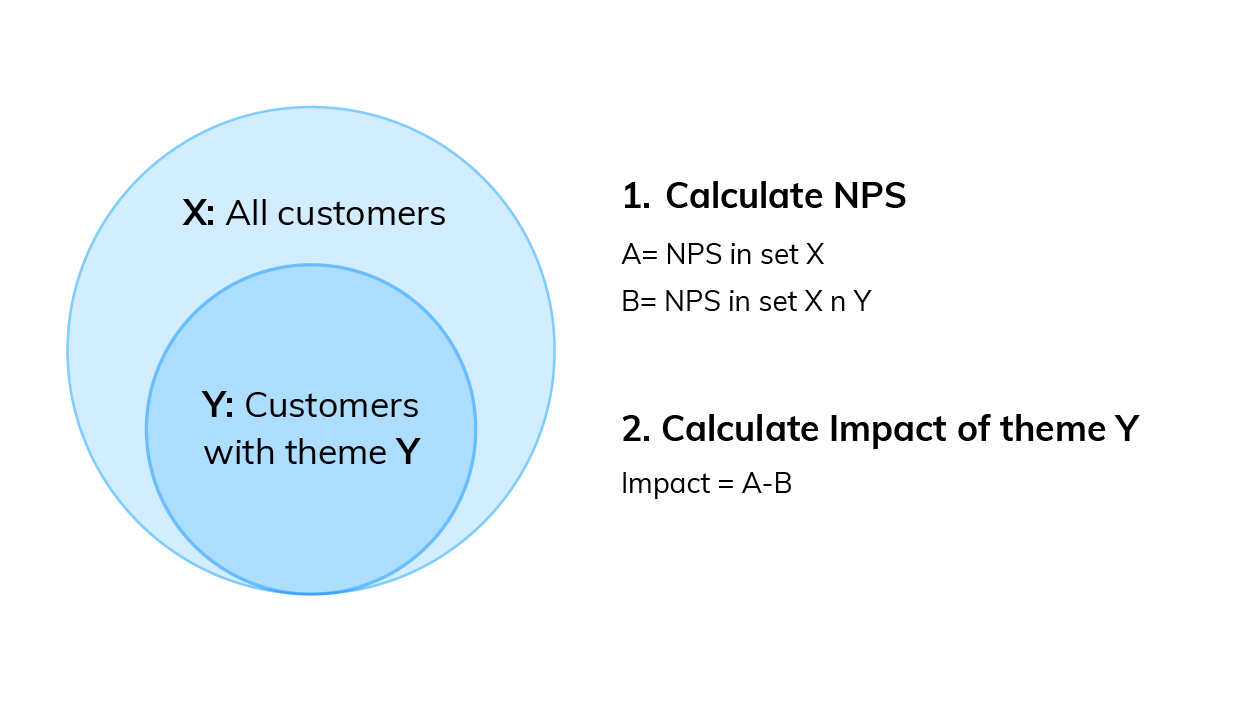
You can then visualize this data using a bar chart.
You can download our CX toolkit - it includes a template to recreate this.
Trends over time
This analysis can help you answer questions like: “Which codes are linked to decreases or increases in my score over time?”
We need to compare two sequences of numbers: NPS over time and code frequency over time . Using Excel, calculate the correlation between the two sequences, which can be either positive (the more codes the higher the NPS, see picture below), or negative (the more codes the lower the NPS).
Now you need to plot code frequency against the absolute value of code correlation with NPS. Here is the formula:
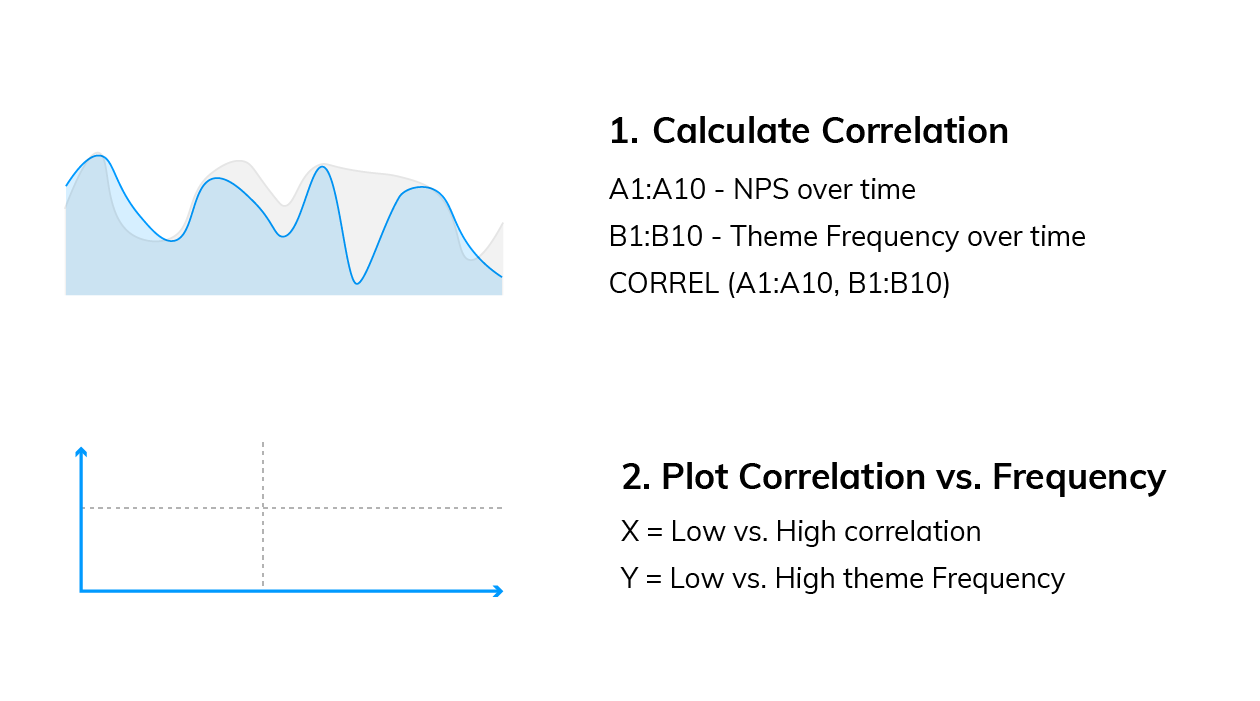
The visualization could look like this:
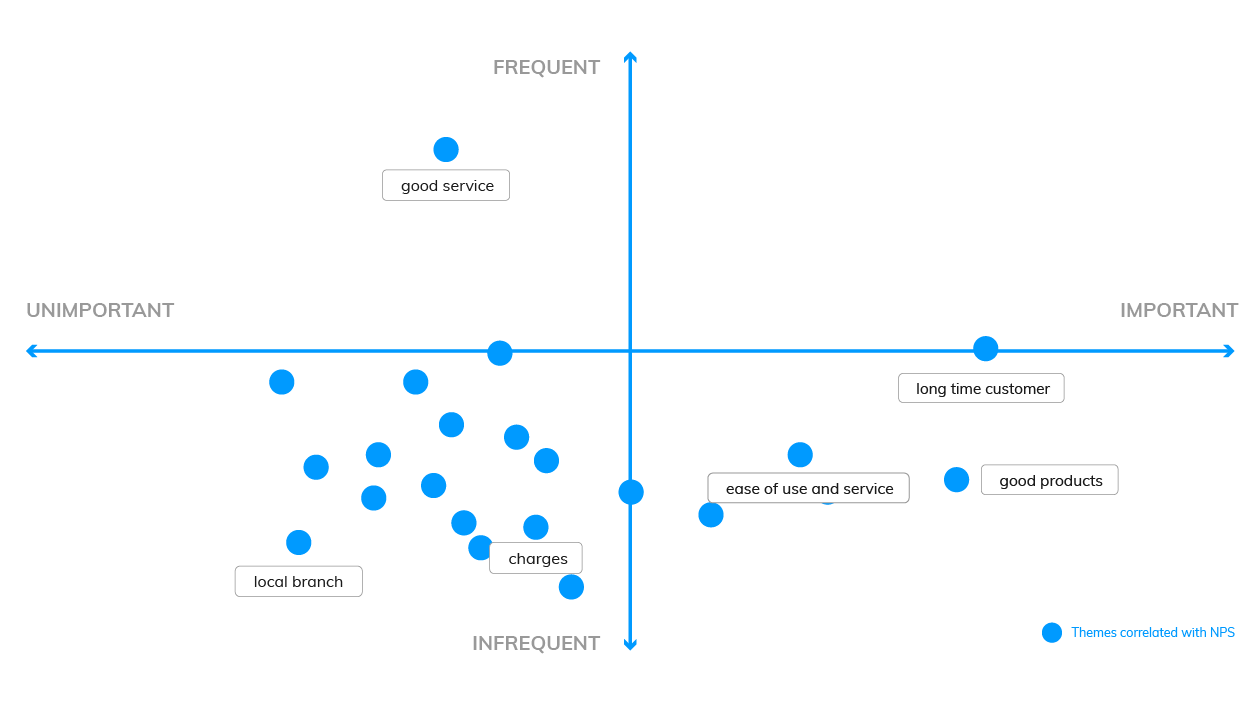
These are two examples, but there are more. For a third manual formula, and to learn why word clouds are not an insightful form of analysis, read our visualizations article .
Using a text analytics solution to automate analysis
Automated text analytics solutions enable codes and sub-codes to be pulled out of the data automatically. This makes it far faster and easier to identify what’s driving negative or positive results. And to pick up emerging trends and find all manner of rich insights in the data.
Another benefit of AI-driven text analytics software is its built-in capability for sentiment analysis, which provides the emotive context behind your feedback and other qualitative textual data therein.
Thematic provides text analytics that goes further by allowing users to apply their expertise on business context to edit or augment the AI-generated outputs.
Since the move away from manual research is generally about reducing the human element, adding human input to the technology might sound counter-intuitive. However, this is mostly to make sure important business nuances in the feedback aren’t missed during coding. The result is a higher accuracy of analysis. This is sometimes referred to as augmented intelligence .
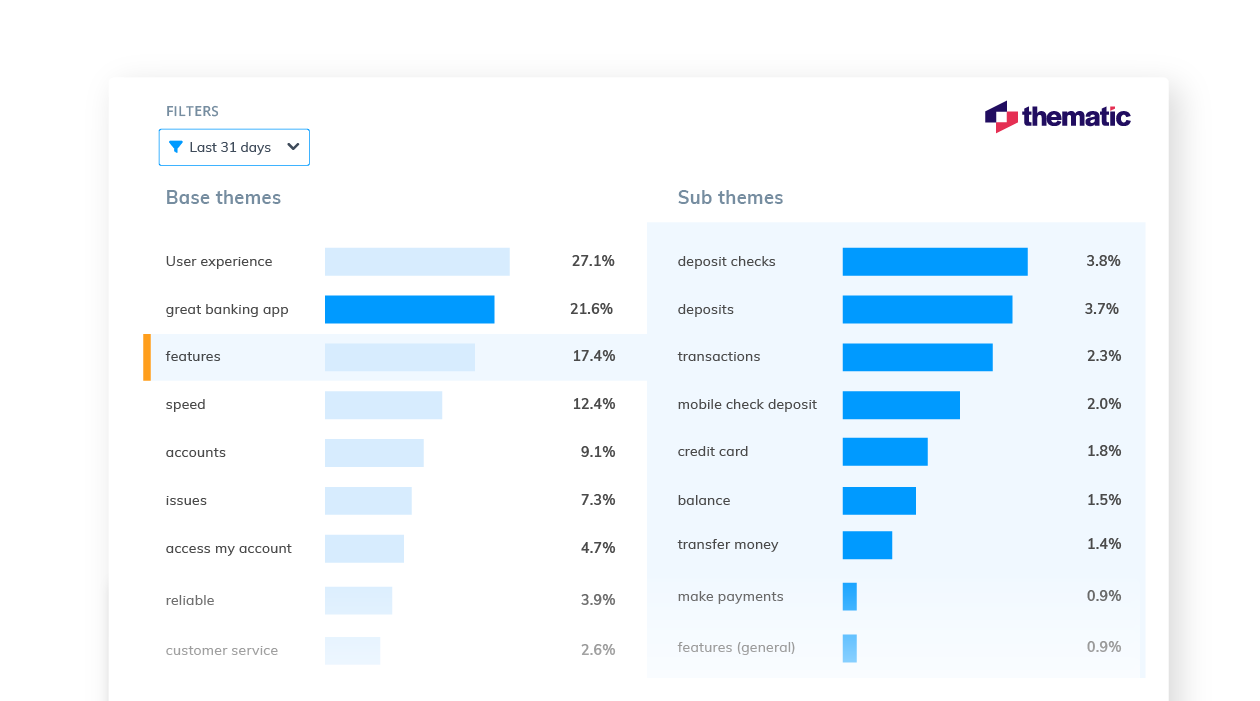
Step 5: Report on your data: Tell the story
The last step of analyzing your qualitative data is to report on it, to tell the story. At this point, the codes are fully developed and the focus is on communicating the narrative to the audience.
A coherent outline of the qualitative research, the findings and the insights is vital for stakeholders to discuss and debate before they can devise a meaningful course of action.
Creating graphs and reporting in Powerpoint
Typically, qualitative researchers take the tried and tested approach of distilling their report into a series of charts, tables and other visuals which are woven into a narrative for presentation in Powerpoint.
Using visualization software for reporting
With data transformation and APIs, the analyzed data can be shared with data visualisation software, such as Power BI or Tableau , Google Studio or Looker. Power BI and Tableau are among the most preferred options.
Visualizing your insights inside a feedback analytics platform
Feedback analytics platforms, like Thematic, incorporate visualisation tools that intuitively turn key data and insights into graphs. This removes the time consuming work of constructing charts to visually identify patterns and creates more time to focus on building a compelling narrative that highlights the insights, in bite-size chunks, for executive teams to review.
Using a feedback analytics platform with visualization tools means you don’t have to use a separate product for visualizations. You can export graphs into Powerpoints straight from the platforms.
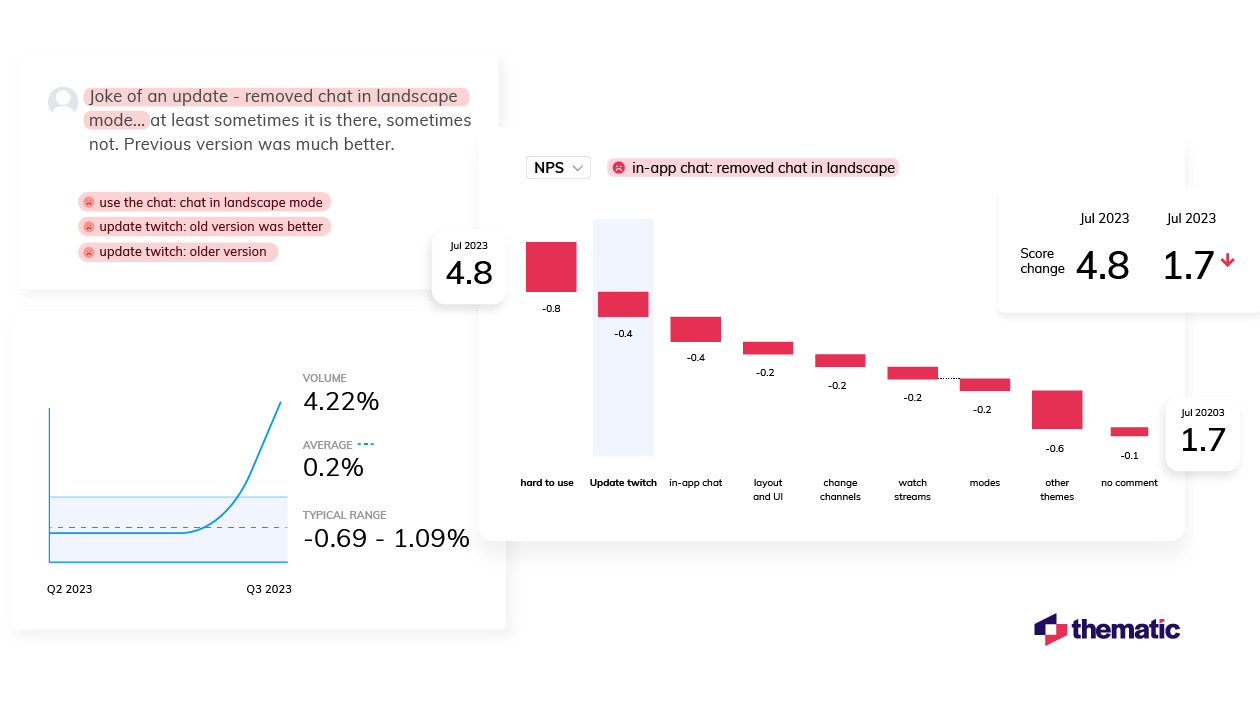
Conclusion - Manual or Automated?
There are those who remain deeply invested in the manual approach - because it’s familiar, because they’re reluctant to spend money and time learning new software, or because they’ve been burned by the overpromises of AI.
For projects that involve small datasets, manual analysis makes sense. For example, if the objective is simply to quantify a simple question like “Do customers prefer X concepts to Y?”. If the findings are being extracted from a small set of focus groups and interviews, sometimes it’s easier to just read them
However, as new generations come into the workplace, it’s technology-driven solutions that feel more comfortable and practical. And the merits are undeniable. Especially if the objective is to go deeper and understand the ‘why’ behind customers’ preference for X or Y. And even more especially if time and money are considerations.
The ability to collect a free flow of qualitative feedback data at the same time as the metric means AI can cost-effectively scan, crunch, score and analyze a ton of feedback from one system in one go. And time-intensive processes like focus groups, or coding, that used to take weeks, can now be completed in a matter of hours or days.
But aside from the ever-present business case to speed things up and keep costs down, there are also powerful research imperatives for automated analysis of qualitative data: namely, accuracy and consistency.
Finding insights hidden in feedback requires consistency, especially in coding. Not to mention catching all the ‘unknown unknowns’ that can skew research findings and steering clear of cognitive bias.
Some say without manual data analysis researchers won’t get an accurate “feel” for the insights. However, the larger data sets are, the harder it is to sort through the feedback and organize feedback that has been pulled from different places. And, the more difficult it is to stay on course, the greater the risk of drawing incorrect, or incomplete, conclusions grows.
Though the process steps for qualitative data analysis have remained pretty much unchanged since psychologist Paul Felix Lazarsfeld paved the path a hundred years ago, the impact digital technology has had on types of qualitative feedback data and the approach to the analysis are profound.
If you want to try an automated feedback analysis solution on your own qualitative data, you can get started with Thematic .
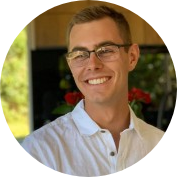
Community & Marketing
Tyler manages our community of CX, insights & analytics professionals. Tyler's goal is to help unite insights professionals around common challenges.
We make it easy to discover the customer and product issues that matter.
Unlock the value of feedback at scale, in one platform. Try it for free now!
- Questions to ask your Feedback Analytics vendor
- How to end customer churn for good
- Scalable analysis of NPS verbatims
- 5 Text analytics approaches
- How to calculate the ROI of CX
Our experts will show you how Thematic works, how to discover pain points and track the ROI of decisions. To access your free trial, book a personal demo today.
Recent posts
Become a qualitative theming pro! Creating a perfect code frame is hard, but thematic analysis software makes the process much easier.
Discover the power of thematic analysis to unlock insights from qualitative data. Learn about manual vs. AI-powered approaches, best practices, and how Thematic software can revolutionize your analysis workflow.
When two major storms wreaked havoc on Auckland and Watercare’s infrastructurem the utility went through a CX crisis. With a massive influx of calls to their support center, Thematic helped them get inisghts from this data to forge a new approach to restore services and satisfaction levels.
Qualitative Research : Definition
Qualitative research is the naturalistic study of social meanings and processes, using interviews, observations, and the analysis of texts and images. In contrast to quantitative researchers, whose statistical methods enable broad generalizations about populations (for example, comparisons of the percentages of U.S. demographic groups who vote in particular ways), qualitative researchers use in-depth studies of the social world to analyze how and why groups think and act in particular ways (for instance, case studies of the experiences that shape political views).
Events and Workshops
- Introduction to NVivo Have you just collected your data and wondered what to do next? Come join us for an introductory session on utilizing NVivo to support your analytical process. This session will only cover features of the software and how to import your records. Please feel free to attend any of the following sessions below: April 25th, 2024 12:30 pm - 1:45 pm Green Library - SVA Conference Room 125 May 9th, 2024 12:30 pm - 1:45 pm Green Library - SVA Conference Room 125
- Next: Choose an approach >>
- Choose an approach
- Find studies
- Learn methods
- Getting Started
- Get software
- Get data for secondary analysis
- Network with researchers

- Last Updated: May 23, 2024 1:27 PM
- URL: https://guides.library.stanford.edu/qualitative_research

Qualitative Data Analysis Methods 101:
The “big 6” methods + examples.
By: Kerryn Warren (PhD) | Reviewed By: Eunice Rautenbach (D.Tech) | May 2020 (Updated April 2023)
Qualitative data analysis methods. Wow, that’s a mouthful.
If you’re new to the world of research, qualitative data analysis can look rather intimidating. So much bulky terminology and so many abstract, fluffy concepts. It certainly can be a minefield!
Don’t worry – in this post, we’ll unpack the most popular analysis methods , one at a time, so that you can approach your analysis with confidence and competence – whether that’s for a dissertation, thesis or really any kind of research project.
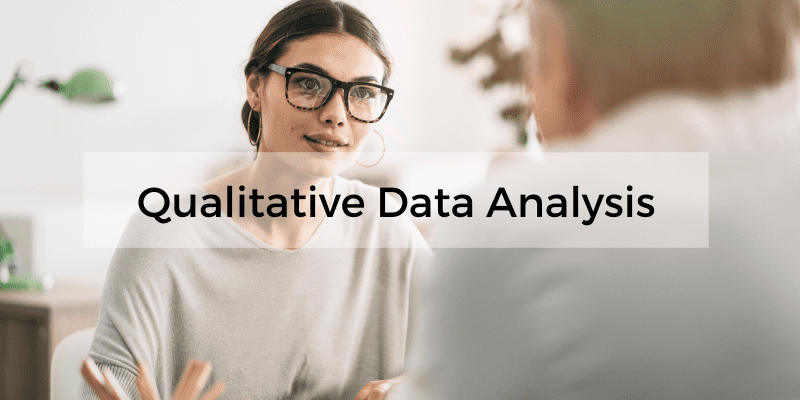
What (exactly) is qualitative data analysis?
To understand qualitative data analysis, we need to first understand qualitative data – so let’s step back and ask the question, “what exactly is qualitative data?”.
Qualitative data refers to pretty much any data that’s “not numbers” . In other words, it’s not the stuff you measure using a fixed scale or complex equipment, nor do you analyse it using complex statistics or mathematics.
So, if it’s not numbers, what is it?
Words, you guessed? Well… sometimes , yes. Qualitative data can, and often does, take the form of interview transcripts, documents and open-ended survey responses – but it can also involve the interpretation of images and videos. In other words, qualitative isn’t just limited to text-based data.
So, how’s that different from quantitative data, you ask?
Simply put, qualitative research focuses on words, descriptions, concepts or ideas – while quantitative research focuses on numbers and statistics . Qualitative research investigates the “softer side” of things to explore and describe , while quantitative research focuses on the “hard numbers”, to measure differences between variables and the relationships between them. If you’re keen to learn more about the differences between qual and quant, we’ve got a detailed post over here .
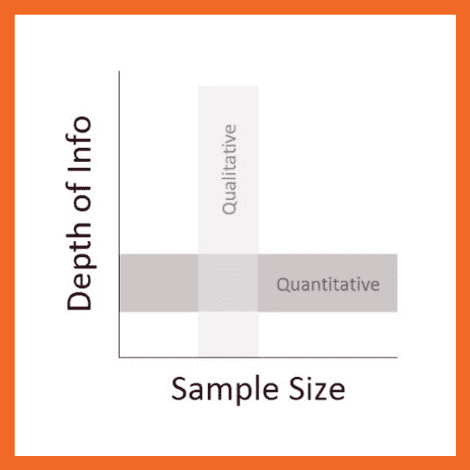
So, qualitative analysis is easier than quantitative, right?
Not quite. In many ways, qualitative data can be challenging and time-consuming to analyse and interpret. At the end of your data collection phase (which itself takes a lot of time), you’ll likely have many pages of text-based data or hours upon hours of audio to work through. You might also have subtle nuances of interactions or discussions that have danced around in your mind, or that you scribbled down in messy field notes. All of this needs to work its way into your analysis.
Making sense of all of this is no small task and you shouldn’t underestimate it. Long story short – qualitative analysis can be a lot of work! Of course, quantitative analysis is no piece of cake either, but it’s important to recognise that qualitative analysis still requires a significant investment in terms of time and effort.
Need a helping hand?
In this post, we’ll explore qualitative data analysis by looking at some of the most common analysis methods we encounter. We’re not going to cover every possible qualitative method and we’re not going to go into heavy detail – we’re just going to give you the big picture. That said, we will of course includes links to loads of extra resources so that you can learn more about whichever analysis method interests you.
Without further delay, let’s get into it.
The “Big 6” Qualitative Analysis Methods
There are many different types of qualitative data analysis, all of which serve different purposes and have unique strengths and weaknesses . We’ll start by outlining the analysis methods and then we’ll dive into the details for each.
The 6 most popular methods (or at least the ones we see at Grad Coach) are:
- Content analysis
- Narrative analysis
- Discourse analysis
- Thematic analysis
- Grounded theory (GT)
- Interpretive phenomenological analysis (IPA)
Let’s take a look at each of them…
QDA Method #1: Qualitative Content Analysis
Content analysis is possibly the most common and straightforward QDA method. At the simplest level, content analysis is used to evaluate patterns within a piece of content (for example, words, phrases or images) or across multiple pieces of content or sources of communication. For example, a collection of newspaper articles or political speeches.
With content analysis, you could, for instance, identify the frequency with which an idea is shared or spoken about – like the number of times a Kardashian is mentioned on Twitter. Or you could identify patterns of deeper underlying interpretations – for instance, by identifying phrases or words in tourist pamphlets that highlight India as an ancient country.
Because content analysis can be used in such a wide variety of ways, it’s important to go into your analysis with a very specific question and goal, or you’ll get lost in the fog. With content analysis, you’ll group large amounts of text into codes , summarise these into categories, and possibly even tabulate the data to calculate the frequency of certain concepts or variables. Because of this, content analysis provides a small splash of quantitative thinking within a qualitative method.
Naturally, while content analysis is widely useful, it’s not without its drawbacks . One of the main issues with content analysis is that it can be very time-consuming , as it requires lots of reading and re-reading of the texts. Also, because of its multidimensional focus on both qualitative and quantitative aspects, it is sometimes accused of losing important nuances in communication.
Content analysis also tends to concentrate on a very specific timeline and doesn’t take into account what happened before or after that timeline. This isn’t necessarily a bad thing though – just something to be aware of. So, keep these factors in mind if you’re considering content analysis. Every analysis method has its limitations , so don’t be put off by these – just be aware of them ! If you’re interested in learning more about content analysis, the video below provides a good starting point.
QDA Method #2: Narrative Analysis
As the name suggests, narrative analysis is all about listening to people telling stories and analysing what that means . Since stories serve a functional purpose of helping us make sense of the world, we can gain insights into the ways that people deal with and make sense of reality by analysing their stories and the ways they’re told.
You could, for example, use narrative analysis to explore whether how something is being said is important. For instance, the narrative of a prisoner trying to justify their crime could provide insight into their view of the world and the justice system. Similarly, analysing the ways entrepreneurs talk about the struggles in their careers or cancer patients telling stories of hope could provide powerful insights into their mindsets and perspectives . Simply put, narrative analysis is about paying attention to the stories that people tell – and more importantly, the way they tell them.
Of course, the narrative approach has its weaknesses , too. Sample sizes are generally quite small due to the time-consuming process of capturing narratives. Because of this, along with the multitude of social and lifestyle factors which can influence a subject, narrative analysis can be quite difficult to reproduce in subsequent research. This means that it’s difficult to test the findings of some of this research.
Similarly, researcher bias can have a strong influence on the results here, so you need to be particularly careful about the potential biases you can bring into your analysis when using this method. Nevertheless, narrative analysis is still a very useful qualitative analysis method – just keep these limitations in mind and be careful not to draw broad conclusions . If you’re keen to learn more about narrative analysis, the video below provides a great introduction to this qualitative analysis method.
QDA Method #3: Discourse Analysis
Discourse is simply a fancy word for written or spoken language or debate . So, discourse analysis is all about analysing language within its social context. In other words, analysing language – such as a conversation, a speech, etc – within the culture and society it takes place. For example, you could analyse how a janitor speaks to a CEO, or how politicians speak about terrorism.
To truly understand these conversations or speeches, the culture and history of those involved in the communication are important factors to consider. For example, a janitor might speak more casually with a CEO in a company that emphasises equality among workers. Similarly, a politician might speak more about terrorism if there was a recent terrorist incident in the country.
So, as you can see, by using discourse analysis, you can identify how culture , history or power dynamics (to name a few) have an effect on the way concepts are spoken about. So, if your research aims and objectives involve understanding culture or power dynamics, discourse analysis can be a powerful method.
Because there are many social influences in terms of how we speak to each other, the potential use of discourse analysis is vast . Of course, this also means it’s important to have a very specific research question (or questions) in mind when analysing your data and looking for patterns and themes, or you might land up going down a winding rabbit hole.
Discourse analysis can also be very time-consuming as you need to sample the data to the point of saturation – in other words, until no new information and insights emerge. But this is, of course, part of what makes discourse analysis such a powerful technique. So, keep these factors in mind when considering this QDA method. Again, if you’re keen to learn more, the video below presents a good starting point.
QDA Method #4: Thematic Analysis
Thematic analysis looks at patterns of meaning in a data set – for example, a set of interviews or focus group transcripts. But what exactly does that… mean? Well, a thematic analysis takes bodies of data (which are often quite large) and groups them according to similarities – in other words, themes . These themes help us make sense of the content and derive meaning from it.
Let’s take a look at an example.
With thematic analysis, you could analyse 100 online reviews of a popular sushi restaurant to find out what patrons think about the place. By reviewing the data, you would then identify the themes that crop up repeatedly within the data – for example, “fresh ingredients” or “friendly wait staff”.
So, as you can see, thematic analysis can be pretty useful for finding out about people’s experiences , views, and opinions . Therefore, if your research aims and objectives involve understanding people’s experience or view of something, thematic analysis can be a great choice.
Since thematic analysis is a bit of an exploratory process, it’s not unusual for your research questions to develop , or even change as you progress through the analysis. While this is somewhat natural in exploratory research, it can also be seen as a disadvantage as it means that data needs to be re-reviewed each time a research question is adjusted. In other words, thematic analysis can be quite time-consuming – but for a good reason. So, keep this in mind if you choose to use thematic analysis for your project and budget extra time for unexpected adjustments.
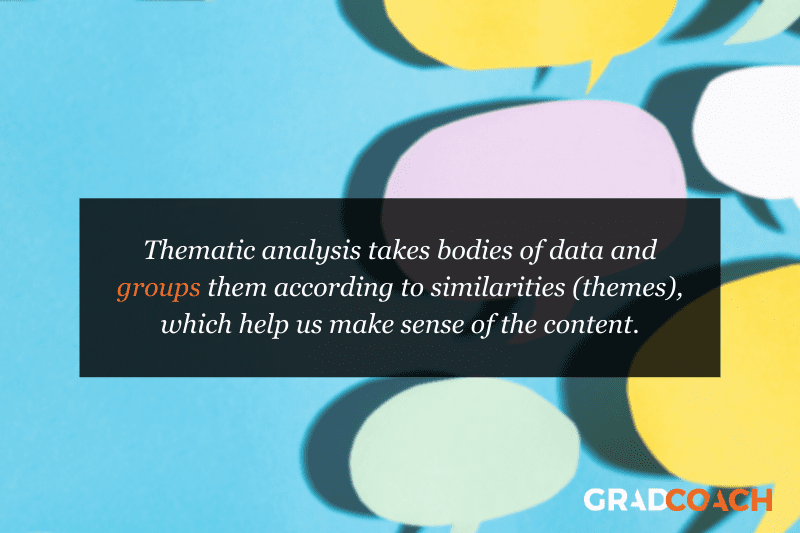
QDA Method #5: Grounded theory (GT)
Grounded theory is a powerful qualitative analysis method where the intention is to create a new theory (or theories) using the data at hand, through a series of “ tests ” and “ revisions ”. Strictly speaking, GT is more a research design type than an analysis method, but we’ve included it here as it’s often referred to as a method.
What’s most important with grounded theory is that you go into the analysis with an open mind and let the data speak for itself – rather than dragging existing hypotheses or theories into your analysis. In other words, your analysis must develop from the ground up (hence the name).
Let’s look at an example of GT in action.
Assume you’re interested in developing a theory about what factors influence students to watch a YouTube video about qualitative analysis. Using Grounded theory , you’d start with this general overarching question about the given population (i.e., graduate students). First, you’d approach a small sample – for example, five graduate students in a department at a university. Ideally, this sample would be reasonably representative of the broader population. You’d interview these students to identify what factors lead them to watch the video.
After analysing the interview data, a general pattern could emerge. For example, you might notice that graduate students are more likely to read a post about qualitative methods if they are just starting on their dissertation journey, or if they have an upcoming test about research methods.
From here, you’ll look for another small sample – for example, five more graduate students in a different department – and see whether this pattern holds true for them. If not, you’ll look for commonalities and adapt your theory accordingly. As this process continues, the theory would develop . As we mentioned earlier, what’s important with grounded theory is that the theory develops from the data – not from some preconceived idea.
So, what are the drawbacks of grounded theory? Well, some argue that there’s a tricky circularity to grounded theory. For it to work, in principle, you should know as little as possible regarding the research question and population, so that you reduce the bias in your interpretation. However, in many circumstances, it’s also thought to be unwise to approach a research question without knowledge of the current literature . In other words, it’s a bit of a “chicken or the egg” situation.
Regardless, grounded theory remains a popular (and powerful) option. Naturally, it’s a very useful method when you’re researching a topic that is completely new or has very little existing research about it, as it allows you to start from scratch and work your way from the ground up .
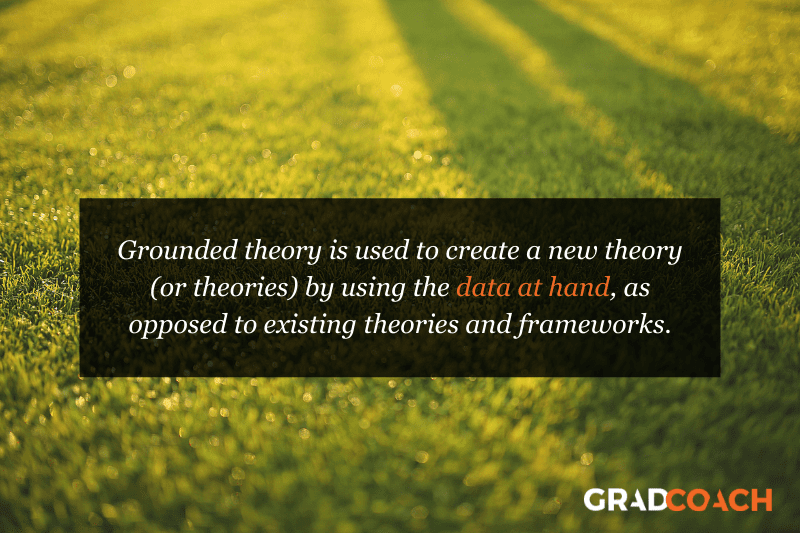
QDA Method #6: Interpretive Phenomenological Analysis (IPA)
Interpretive. Phenomenological. Analysis. IPA . Try saying that three times fast…
Let’s just stick with IPA, okay?
IPA is designed to help you understand the personal experiences of a subject (for example, a person or group of people) concerning a major life event, an experience or a situation . This event or experience is the “phenomenon” that makes up the “P” in IPA. Such phenomena may range from relatively common events – such as motherhood, or being involved in a car accident – to those which are extremely rare – for example, someone’s personal experience in a refugee camp. So, IPA is a great choice if your research involves analysing people’s personal experiences of something that happened to them.
It’s important to remember that IPA is subject – centred . In other words, it’s focused on the experiencer . This means that, while you’ll likely use a coding system to identify commonalities, it’s important not to lose the depth of experience or meaning by trying to reduce everything to codes. Also, keep in mind that since your sample size will generally be very small with IPA, you often won’t be able to draw broad conclusions about the generalisability of your findings. But that’s okay as long as it aligns with your research aims and objectives.
Another thing to be aware of with IPA is personal bias . While researcher bias can creep into all forms of research, self-awareness is critically important with IPA, as it can have a major impact on the results. For example, a researcher who was a victim of a crime himself could insert his own feelings of frustration and anger into the way he interprets the experience of someone who was kidnapped. So, if you’re going to undertake IPA, you need to be very self-aware or you could muddy the analysis.
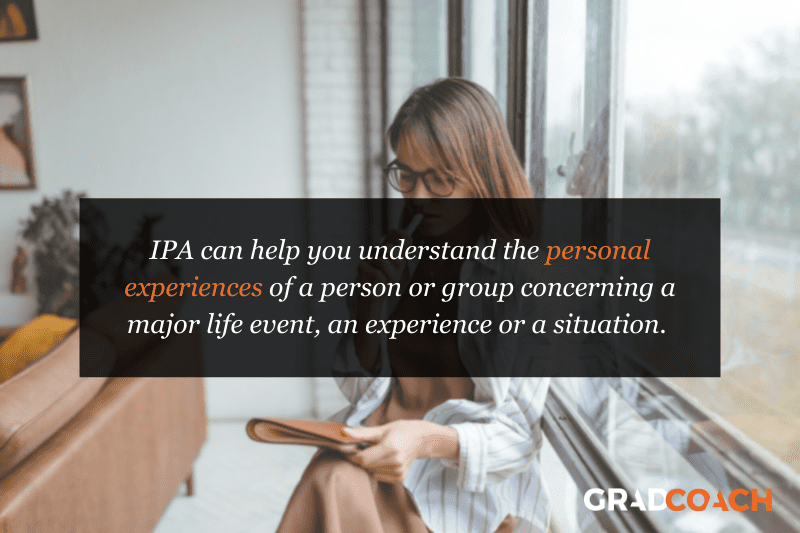
How to choose the right analysis method
In light of all of the qualitative analysis methods we’ve covered so far, you’re probably asking yourself the question, “ How do I choose the right one? ”
Much like all the other methodological decisions you’ll need to make, selecting the right qualitative analysis method largely depends on your research aims, objectives and questions . In other words, the best tool for the job depends on what you’re trying to build. For example:
- Perhaps your research aims to analyse the use of words and what they reveal about the intention of the storyteller and the cultural context of the time.
- Perhaps your research aims to develop an understanding of the unique personal experiences of people that have experienced a certain event, or
- Perhaps your research aims to develop insight regarding the influence of a certain culture on its members.
As you can probably see, each of these research aims are distinctly different , and therefore different analysis methods would be suitable for each one. For example, narrative analysis would likely be a good option for the first aim, while grounded theory wouldn’t be as relevant.
It’s also important to remember that each method has its own set of strengths, weaknesses and general limitations. No single analysis method is perfect . So, depending on the nature of your research, it may make sense to adopt more than one method (this is called triangulation ). Keep in mind though that this will of course be quite time-consuming.
As we’ve seen, all of the qualitative analysis methods we’ve discussed make use of coding and theme-generating techniques, but the intent and approach of each analysis method differ quite substantially. So, it’s very important to come into your research with a clear intention before you decide which analysis method (or methods) to use.
Start by reviewing your research aims , objectives and research questions to assess what exactly you’re trying to find out – then select a qualitative analysis method that fits. Never pick a method just because you like it or have experience using it – your analysis method (or methods) must align with your broader research aims and objectives.
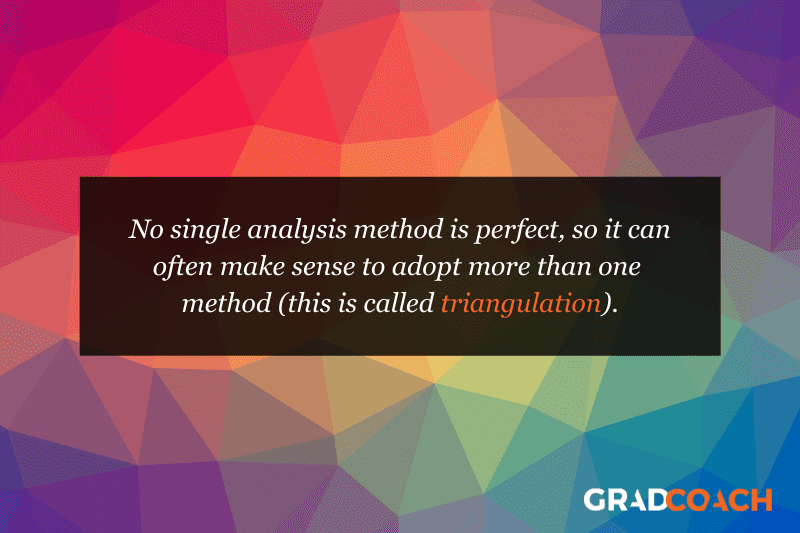
Let’s recap on QDA methods…
In this post, we looked at six popular qualitative data analysis methods:
- First, we looked at content analysis , a straightforward method that blends a little bit of quant into a primarily qualitative analysis.
- Then we looked at narrative analysis , which is about analysing how stories are told.
- Next up was discourse analysis – which is about analysing conversations and interactions.
- Then we moved on to thematic analysis – which is about identifying themes and patterns.
- From there, we went south with grounded theory – which is about starting from scratch with a specific question and using the data alone to build a theory in response to that question.
- And finally, we looked at IPA – which is about understanding people’s unique experiences of a phenomenon.
Of course, these aren’t the only options when it comes to qualitative data analysis, but they’re a great starting point if you’re dipping your toes into qualitative research for the first time.
If you’re still feeling a bit confused, consider our private coaching service , where we hold your hand through the research process to help you develop your best work.
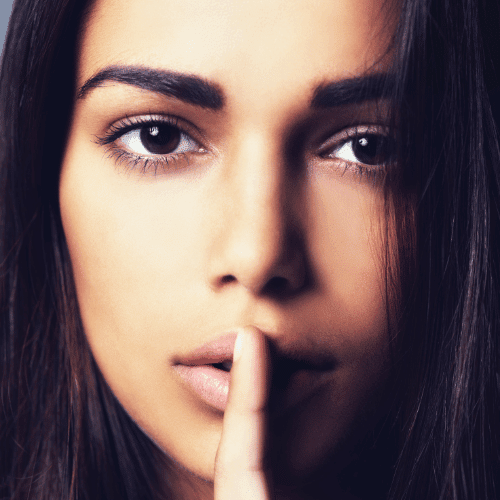
Psst... there’s more!
This post was based on one of our popular Research Bootcamps . If you're working on a research project, you'll definitely want to check this out ...
87 Comments
This has been very helpful. Thank you.
Thank you madam,
Thank you so much for this information
I wonder it so clear for understand and good for me. can I ask additional query?
Very insightful and useful
Good work done with clear explanations. Thank you.
Thanks so much for the write-up, it’s really good.
Thanks madam . It is very important .
thank you very good
Great presentation
This has been very well explained in simple language . It is useful even for a new researcher.
Great to hear that. Good luck with your qualitative data analysis, Pramod!
This is very useful information. And it was very a clear language structured presentation. Thanks a lot.
Thank you so much.
very informative sequential presentation
Precise explanation of method.
Hi, may we use 2 data analysis methods in our qualitative research?
Thanks for your comment. Most commonly, one would use one type of analysis method, but it depends on your research aims and objectives.
You explained it in very simple language, everyone can understand it. Thanks so much.
Thank you very much, this is very helpful. It has been explained in a very simple manner that even a layman understands
Thank nicely explained can I ask is Qualitative content analysis the same as thematic analysis?
Thanks for your comment. No, QCA and thematic are two different types of analysis. This article might help clarify – https://onlinelibrary.wiley.com/doi/10.1111/nhs.12048
This is my first time to come across a well explained data analysis. so helpful.
I have thoroughly enjoyed your explanation of the six qualitative analysis methods. This is very helpful. Thank you!
Thank you very much, this is well explained and useful
i need a citation of your book.
Thanks a lot , remarkable indeed, enlighting to the best
Hi Derek, What other theories/methods would you recommend when the data is a whole speech?
Keep writing useful artikel.
It is important concept about QDA and also the way to express is easily understandable, so thanks for all.
Thank you, this is well explained and very useful.
Very helpful .Thanks.
Hi there! Very well explained. Simple but very useful style of writing. Please provide the citation of the text. warm regards
The session was very helpful and insightful. Thank you
This was very helpful and insightful. Easy to read and understand
As a professional academic writer, this has been so informative and educative. Keep up the good work Grad Coach you are unmatched with quality content for sure.
Keep up the good work Grad Coach you are unmatched with quality content for sure.
Its Great and help me the most. A Million Thanks you Dr.
It is a very nice work
Very insightful. Please, which of this approach could be used for a research that one is trying to elicit students’ misconceptions in a particular concept ?
This is Amazing and well explained, thanks
great overview
What do we call a research data analysis method that one use to advise or determining the best accounting tool or techniques that should be adopted in a company.
Informative video, explained in a clear and simple way. Kudos
Waoo! I have chosen method wrong for my data analysis. But I can revise my work according to this guide. Thank you so much for this helpful lecture.
This has been very helpful. It gave me a good view of my research objectives and how to choose the best method. Thematic analysis it is.
Very helpful indeed. Thanku so much for the insight.
This was incredibly helpful.
Very helpful.
very educative
Nicely written especially for novice academic researchers like me! Thank you.
choosing a right method for a paper is always a hard job for a student, this is a useful information, but it would be more useful personally for me, if the author provide me with a little bit more information about the data analysis techniques in type of explanatory research. Can we use qualitative content analysis technique for explanatory research ? or what is the suitable data analysis method for explanatory research in social studies?
that was very helpful for me. because these details are so important to my research. thank you very much
I learnt a lot. Thank you
Relevant and Informative, thanks !
Well-planned and organized, thanks much! 🙂
I have reviewed qualitative data analysis in a simplest way possible. The content will highly be useful for developing my book on qualitative data analysis methods. Cheers!
Clear explanation on qualitative and how about Case study
This was helpful. Thank you
This was really of great assistance, it was just the right information needed. Explanation very clear and follow.
Wow, Thanks for making my life easy
This was helpful thanks .
Very helpful…. clear and written in an easily understandable manner. Thank you.
This was so helpful as it was easy to understand. I’m a new to research thank you so much.
so educative…. but Ijust want to know which method is coding of the qualitative or tallying done?
Thank you for the great content, I have learnt a lot. So helpful
precise and clear presentation with simple language and thank you for that.
very informative content, thank you.
You guys are amazing on YouTube on this platform. Your teachings are great, educative, and informative. kudos!
Brilliant Delivery. You made a complex subject seem so easy. Well done.
Beautifully explained.
Thanks a lot
Is there a video the captures the practical process of coding using automated applications?
Thanks for the comment. We don’t recommend using automated applications for coding, as they are not sufficiently accurate in our experience.
content analysis can be qualitative research?
THANK YOU VERY MUCH.
Thank you very much for such a wonderful content
do you have any material on Data collection
What a powerful explanation of the QDA methods. Thank you.
Great explanation both written and Video. i have been using of it on a day to day working of my thesis project in accounting and finance. Thank you very much for your support.
very helpful, thank you so much
The tutorial is useful. I benefited a lot.
This is an eye opener for me and very informative, I have used some of your guidance notes on my Thesis, I wonder if you can assist with your 1. name of your book, year of publication, topic etc., this is for citing in my Bibliography,
I certainly hope to hear from you
Submit a Comment Cancel reply
Your email address will not be published. Required fields are marked *
Save my name, email, and website in this browser for the next time I comment.
- Print Friendly
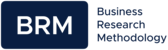
Qualitative Data Analysis
Qualitative data refers to non-numeric information such as interview transcripts, notes, video and audio recordings, images and text documents. Qualitative data analysis can be divided into the following five categories:
1. Content analysis . This refers to the process of categorizing verbal or behavioural data to classify, summarize and tabulate the data.
2. Narrative analysis . This method involves the reformulation of stories presented by respondents taking into account context of each case and different experiences of each respondent. In other words, narrative analysis is the revision of primary qualitative data by researcher.
3. Discourse analysis . A method of analysis of naturally occurring talk and all types of written text.
4. Framework analysis . This is more advanced method that consists of several stages such as familiarization, identifying a thematic framework, coding, charting, mapping and interpretation.
5. Grounded theory . This method of qualitative data analysis starts with an analysis of a single case to formulate a theory. Then, additional cases are examined to see if they contribute to the theory.
Qualitative data analysis can be conducted through the following three steps:
Step 1: Developing and Applying Codes . Coding can be explained as categorization of data. A ‘code’ can be a word or a short phrase that represents a theme or an idea. All codes need to be assigned meaningful titles. A wide range of non-quantifiable elements such as events, behaviours, activities, meanings etc. can be coded.
There are three types of coding:
- Open coding . The initial organization of raw data to try to make sense of it.
- Axial coding . Interconnecting and linking the categories of codes.
- Selective coding . Formulating the story through connecting the categories.
Coding can be done manually or using qualitative data analysis software such as
NVivo, Atlas ti 6.0, HyperRESEARCH 2.8, Max QDA and others.
When using manual coding you can use folders, filing cabinets, wallets etc. to gather together materials that are examples of similar themes or analytic ideas. Manual method of coding in qualitative data analysis is rightly considered as labour-intensive, time-consuming and outdated.
In computer-based coding, on the other hand, physical files and cabinets are replaced with computer based directories and files. When choosing software for qualitative data analysis you need to consider a wide range of factors such as the type and amount of data you need to analyse, time required to master the software and cost considerations.
Moreover, it is important to get confirmation from your dissertation supervisor prior to application of any specific qualitative data analysis software.
The following table contains examples of research titles, elements to be coded and identification of relevant codes:
Born or bred: revising The Great Man theory of leadership in the 21 century | Leadership practice | Born leaders Made leaders Leadership effectiveness |
A study into advantages and disadvantages of various entry strategies to Chinese market
|
Market entry strategies | Wholly-owned subsidiaries Joint-ventures Franchising Exporting Licensing |
Impacts of CSR programs and initiative on brand image: a case study of Coca-Cola Company UK. |
Activities, phenomenon | Philanthropy Supporting charitable courses Ethical behaviour Brand awareness Brand value |
An investigation into the ways of customer relationship management in mobile marketing environment |
Tactics | Viral messages Customer retention Popularity of social networking sites |
Qualitative data coding
Step 2: Identifying themes, patterns and relationships . Unlike quantitative methods , in qualitative data analysis there are no universally applicable techniques that can be applied to generate findings. Analytical and critical thinking skills of researcher plays significant role in data analysis in qualitative studies. Therefore, no qualitative study can be repeated to generate the same results.
Nevertheless, there is a set of techniques that you can use to identify common themes, patterns and relationships within responses of sample group members in relation to codes that have been specified in the previous stage.
Specifically, the most popular and effective methods of qualitative data interpretation include the following:
- Word and phrase repetitions – scanning primary data for words and phrases most commonly used by respondents, as well as, words and phrases used with unusual emotions;
- Primary and secondary data comparisons – comparing the findings of interview/focus group/observation/any other qualitative data collection method with the findings of literature review and discussing differences between them;
- Search for missing information – discussions about which aspects of the issue was not mentioned by respondents, although you expected them to be mentioned;
- Metaphors and analogues – comparing primary research findings to phenomena from a different area and discussing similarities and differences.
Step 3: Summarizing the data . At this last stage you need to link research findings to hypotheses or research aim and objectives. When writing data analysis chapter, you can use noteworthy quotations from the transcript in order to highlight major themes within findings and possible contradictions.
It is important to note that the process of qualitative data analysis described above is general and different types of qualitative studies may require slightly different methods of data analysis.
My e-book, The Ultimate Guide to Writing a Dissertation in Business Studies: a step by step approach contains a detailed, yet simple explanation of qualitative data analysis methods . The e-book explains all stages of the research process starting from the selection of the research area to writing personal reflection. Important elements of dissertations such as research philosophy, research approach, research design, methods of data collection and data analysis are explained in simple words. John Dudovskiy
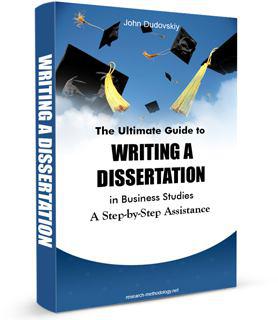
Qualitative vs Quantitative Research Methods & Data Analysis
Saul McLeod, PhD
Editor-in-Chief for Simply Psychology
BSc (Hons) Psychology, MRes, PhD, University of Manchester
Saul McLeod, PhD., is a qualified psychology teacher with over 18 years of experience in further and higher education. He has been published in peer-reviewed journals, including the Journal of Clinical Psychology.
Learn about our Editorial Process
Olivia Guy-Evans, MSc
Associate Editor for Simply Psychology
BSc (Hons) Psychology, MSc Psychology of Education
Olivia Guy-Evans is a writer and associate editor for Simply Psychology. She has previously worked in healthcare and educational sectors.
On This Page:
What is the difference between quantitative and qualitative?
The main difference between quantitative and qualitative research is the type of data they collect and analyze.
Quantitative research collects numerical data and analyzes it using statistical methods. The aim is to produce objective, empirical data that can be measured and expressed in numerical terms. Quantitative research is often used to test hypotheses, identify patterns, and make predictions.
Qualitative research , on the other hand, collects non-numerical data such as words, images, and sounds. The focus is on exploring subjective experiences, opinions, and attitudes, often through observation and interviews.
Qualitative research aims to produce rich and detailed descriptions of the phenomenon being studied, and to uncover new insights and meanings.
Quantitative data is information about quantities, and therefore numbers, and qualitative data is descriptive, and regards phenomenon which can be observed but not measured, such as language.
What Is Qualitative Research?
Qualitative research is the process of collecting, analyzing, and interpreting non-numerical data, such as language. Qualitative research can be used to understand how an individual subjectively perceives and gives meaning to their social reality.
Qualitative data is non-numerical data, such as text, video, photographs, or audio recordings. This type of data can be collected using diary accounts or in-depth interviews and analyzed using grounded theory or thematic analysis.
Qualitative research is multimethod in focus, involving an interpretive, naturalistic approach to its subject matter. This means that qualitative researchers study things in their natural settings, attempting to make sense of, or interpret, phenomena in terms of the meanings people bring to them. Denzin and Lincoln (1994, p. 2)
Interest in qualitative data came about as the result of the dissatisfaction of some psychologists (e.g., Carl Rogers) with the scientific study of psychologists such as behaviorists (e.g., Skinner ).
Since psychologists study people, the traditional approach to science is not seen as an appropriate way of carrying out research since it fails to capture the totality of human experience and the essence of being human. Exploring participants’ experiences is known as a phenomenological approach (re: Humanism ).
Qualitative research is primarily concerned with meaning, subjectivity, and lived experience. The goal is to understand the quality and texture of people’s experiences, how they make sense of them, and the implications for their lives.
Qualitative research aims to understand the social reality of individuals, groups, and cultures as nearly as possible as participants feel or live it. Thus, people and groups are studied in their natural setting.
Some examples of qualitative research questions are provided, such as what an experience feels like, how people talk about something, how they make sense of an experience, and how events unfold for people.
Research following a qualitative approach is exploratory and seeks to explain ‘how’ and ‘why’ a particular phenomenon, or behavior, operates as it does in a particular context. It can be used to generate hypotheses and theories from the data.
Qualitative Methods
There are different types of qualitative research methods, including diary accounts, in-depth interviews , documents, focus groups , case study research , and ethnography.
The results of qualitative methods provide a deep understanding of how people perceive their social realities and in consequence, how they act within the social world.
The researcher has several methods for collecting empirical materials, ranging from the interview to direct observation, to the analysis of artifacts, documents, and cultural records, to the use of visual materials or personal experience. Denzin and Lincoln (1994, p. 14)
Here are some examples of qualitative data:
Interview transcripts : Verbatim records of what participants said during an interview or focus group. They allow researchers to identify common themes and patterns, and draw conclusions based on the data. Interview transcripts can also be useful in providing direct quotes and examples to support research findings.
Observations : The researcher typically takes detailed notes on what they observe, including any contextual information, nonverbal cues, or other relevant details. The resulting observational data can be analyzed to gain insights into social phenomena, such as human behavior, social interactions, and cultural practices.
Unstructured interviews : generate qualitative data through the use of open questions. This allows the respondent to talk in some depth, choosing their own words. This helps the researcher develop a real sense of a person’s understanding of a situation.
Diaries or journals : Written accounts of personal experiences or reflections.
Notice that qualitative data could be much more than just words or text. Photographs, videos, sound recordings, and so on, can be considered qualitative data. Visual data can be used to understand behaviors, environments, and social interactions.
Qualitative Data Analysis
Qualitative research is endlessly creative and interpretive. The researcher does not just leave the field with mountains of empirical data and then easily write up his or her findings.
Qualitative interpretations are constructed, and various techniques can be used to make sense of the data, such as content analysis, grounded theory (Glaser & Strauss, 1967), thematic analysis (Braun & Clarke, 2006), or discourse analysis .
For example, thematic analysis is a qualitative approach that involves identifying implicit or explicit ideas within the data. Themes will often emerge once the data has been coded .
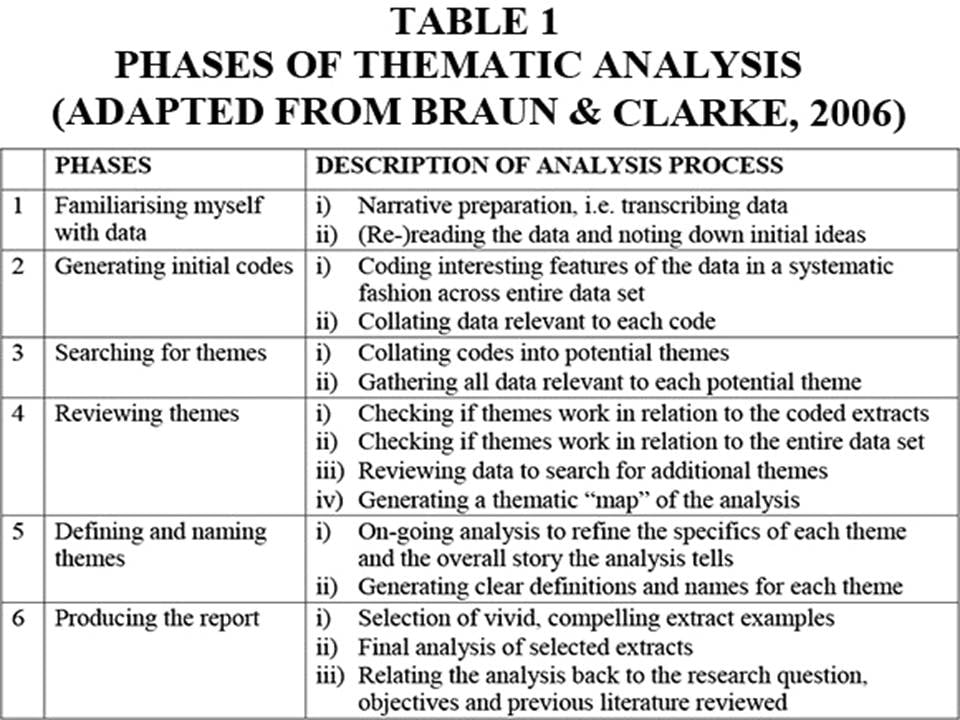
Key Features
- Events can be understood adequately only if they are seen in context. Therefore, a qualitative researcher immerses her/himself in the field, in natural surroundings. The contexts of inquiry are not contrived; they are natural. Nothing is predefined or taken for granted.
- Qualitative researchers want those who are studied to speak for themselves, to provide their perspectives in words and other actions. Therefore, qualitative research is an interactive process in which the persons studied teach the researcher about their lives.
- The qualitative researcher is an integral part of the data; without the active participation of the researcher, no data exists.
- The study’s design evolves during the research and can be adjusted or changed as it progresses. For the qualitative researcher, there is no single reality. It is subjective and exists only in reference to the observer.
- The theory is data-driven and emerges as part of the research process, evolving from the data as they are collected.
Limitations of Qualitative Research
- Because of the time and costs involved, qualitative designs do not generally draw samples from large-scale data sets.
- The problem of adequate validity or reliability is a major criticism. Because of the subjective nature of qualitative data and its origin in single contexts, it is difficult to apply conventional standards of reliability and validity. For example, because of the central role played by the researcher in the generation of data, it is not possible to replicate qualitative studies.
- Also, contexts, situations, events, conditions, and interactions cannot be replicated to any extent, nor can generalizations be made to a wider context than the one studied with confidence.
- The time required for data collection, analysis, and interpretation is lengthy. Analysis of qualitative data is difficult, and expert knowledge of an area is necessary to interpret qualitative data. Great care must be taken when doing so, for example, looking for mental illness symptoms.
Advantages of Qualitative Research
- Because of close researcher involvement, the researcher gains an insider’s view of the field. This allows the researcher to find issues that are often missed (such as subtleties and complexities) by the scientific, more positivistic inquiries.
- Qualitative descriptions can be important in suggesting possible relationships, causes, effects, and dynamic processes.
- Qualitative analysis allows for ambiguities/contradictions in the data, which reflect social reality (Denscombe, 2010).
- Qualitative research uses a descriptive, narrative style; this research might be of particular benefit to the practitioner as she or he could turn to qualitative reports to examine forms of knowledge that might otherwise be unavailable, thereby gaining new insight.
What Is Quantitative Research?
Quantitative research involves the process of objectively collecting and analyzing numerical data to describe, predict, or control variables of interest.
The goals of quantitative research are to test causal relationships between variables , make predictions, and generalize results to wider populations.
Quantitative researchers aim to establish general laws of behavior and phenomenon across different settings/contexts. Research is used to test a theory and ultimately support or reject it.
Quantitative Methods
Experiments typically yield quantitative data, as they are concerned with measuring things. However, other research methods, such as controlled observations and questionnaires , can produce both quantitative information.
For example, a rating scale or closed questions on a questionnaire would generate quantitative data as these produce either numerical data or data that can be put into categories (e.g., “yes,” “no” answers).
Experimental methods limit how research participants react to and express appropriate social behavior.
Findings are, therefore, likely to be context-bound and simply a reflection of the assumptions that the researcher brings to the investigation.
There are numerous examples of quantitative data in psychological research, including mental health. Here are a few examples:
Another example is the Experience in Close Relationships Scale (ECR), a self-report questionnaire widely used to assess adult attachment styles .
The ECR provides quantitative data that can be used to assess attachment styles and predict relationship outcomes.
Neuroimaging data : Neuroimaging techniques, such as MRI and fMRI, provide quantitative data on brain structure and function.
This data can be analyzed to identify brain regions involved in specific mental processes or disorders.
For example, the Beck Depression Inventory (BDI) is a clinician-administered questionnaire widely used to assess the severity of depressive symptoms in individuals.
The BDI consists of 21 questions, each scored on a scale of 0 to 3, with higher scores indicating more severe depressive symptoms.
Quantitative Data Analysis
Statistics help us turn quantitative data into useful information to help with decision-making. We can use statistics to summarize our data, describing patterns, relationships, and connections. Statistics can be descriptive or inferential.
Descriptive statistics help us to summarize our data. In contrast, inferential statistics are used to identify statistically significant differences between groups of data (such as intervention and control groups in a randomized control study).
- Quantitative researchers try to control extraneous variables by conducting their studies in the lab.
- The research aims for objectivity (i.e., without bias) and is separated from the data.
- The design of the study is determined before it begins.
- For the quantitative researcher, the reality is objective, exists separately from the researcher, and can be seen by anyone.
- Research is used to test a theory and ultimately support or reject it.
Limitations of Quantitative Research
- Context: Quantitative experiments do not take place in natural settings. In addition, they do not allow participants to explain their choices or the meaning of the questions they may have for those participants (Carr, 1994).
- Researcher expertise: Poor knowledge of the application of statistical analysis may negatively affect analysis and subsequent interpretation (Black, 1999).
- Variability of data quantity: Large sample sizes are needed for more accurate analysis. Small-scale quantitative studies may be less reliable because of the low quantity of data (Denscombe, 2010). This also affects the ability to generalize study findings to wider populations.
- Confirmation bias: The researcher might miss observing phenomena because of focus on theory or hypothesis testing rather than on the theory of hypothesis generation.
Advantages of Quantitative Research
- Scientific objectivity: Quantitative data can be interpreted with statistical analysis, and since statistics are based on the principles of mathematics, the quantitative approach is viewed as scientifically objective and rational (Carr, 1994; Denscombe, 2010).
- Useful for testing and validating already constructed theories.
- Rapid analysis: Sophisticated software removes much of the need for prolonged data analysis, especially with large volumes of data involved (Antonius, 2003).
- Replication: Quantitative data is based on measured values and can be checked by others because numerical data is less open to ambiguities of interpretation.
- Hypotheses can also be tested because of statistical analysis (Antonius, 2003).
Antonius, R. (2003). Interpreting quantitative data with SPSS . Sage.
Black, T. R. (1999). Doing quantitative research in the social sciences: An integrated approach to research design, measurement and statistics . Sage.
Braun, V. & Clarke, V. (2006). Using thematic analysis in psychology . Qualitative Research in Psychology , 3, 77–101.
Carr, L. T. (1994). The strengths and weaknesses of quantitative and qualitative research : what method for nursing? Journal of advanced nursing, 20(4) , 716-721.
Denscombe, M. (2010). The Good Research Guide: for small-scale social research. McGraw Hill.
Denzin, N., & Lincoln. Y. (1994). Handbook of Qualitative Research. Thousand Oaks, CA, US: Sage Publications Inc.
Glaser, B. G., Strauss, A. L., & Strutzel, E. (1968). The discovery of grounded theory; strategies for qualitative research. Nursing research, 17(4) , 364.
Minichiello, V. (1990). In-Depth Interviewing: Researching People. Longman Cheshire.
Punch, K. (1998). Introduction to Social Research: Quantitative and Qualitative Approaches. London: Sage
Further Information
- Mixed methods research
- Designing qualitative research
- Methods of data collection and analysis
- Introduction to quantitative and qualitative research
- Checklists for improving rigour in qualitative research: a case of the tail wagging the dog?
- Qualitative research in health care: Analysing qualitative data
- Qualitative data analysis: the framework approach
- Using the framework method for the analysis of
- Qualitative data in multi-disciplinary health research
- Content Analysis
- Grounded Theory
- Thematic Analysis

- Open access
- Published: 27 May 2020
How to use and assess qualitative research methods
- Loraine Busetto ORCID: orcid.org/0000-0002-9228-7875 1 ,
- Wolfgang Wick 1 , 2 &
- Christoph Gumbinger 1
Neurological Research and Practice volume 2 , Article number: 14 ( 2020 ) Cite this article
766k Accesses
345 Citations
90 Altmetric
Metrics details
This paper aims to provide an overview of the use and assessment of qualitative research methods in the health sciences. Qualitative research can be defined as the study of the nature of phenomena and is especially appropriate for answering questions of why something is (not) observed, assessing complex multi-component interventions, and focussing on intervention improvement. The most common methods of data collection are document study, (non-) participant observations, semi-structured interviews and focus groups. For data analysis, field-notes and audio-recordings are transcribed into protocols and transcripts, and coded using qualitative data management software. Criteria such as checklists, reflexivity, sampling strategies, piloting, co-coding, member-checking and stakeholder involvement can be used to enhance and assess the quality of the research conducted. Using qualitative in addition to quantitative designs will equip us with better tools to address a greater range of research problems, and to fill in blind spots in current neurological research and practice.
The aim of this paper is to provide an overview of qualitative research methods, including hands-on information on how they can be used, reported and assessed. This article is intended for beginning qualitative researchers in the health sciences as well as experienced quantitative researchers who wish to broaden their understanding of qualitative research.
What is qualitative research?
Qualitative research is defined as “the study of the nature of phenomena”, including “their quality, different manifestations, the context in which they appear or the perspectives from which they can be perceived” , but excluding “their range, frequency and place in an objectively determined chain of cause and effect” [ 1 ]. This formal definition can be complemented with a more pragmatic rule of thumb: qualitative research generally includes data in form of words rather than numbers [ 2 ].
Why conduct qualitative research?
Because some research questions cannot be answered using (only) quantitative methods. For example, one Australian study addressed the issue of why patients from Aboriginal communities often present late or not at all to specialist services offered by tertiary care hospitals. Using qualitative interviews with patients and staff, it found one of the most significant access barriers to be transportation problems, including some towns and communities simply not having a bus service to the hospital [ 3 ]. A quantitative study could have measured the number of patients over time or even looked at possible explanatory factors – but only those previously known or suspected to be of relevance. To discover reasons for observed patterns, especially the invisible or surprising ones, qualitative designs are needed.
While qualitative research is common in other fields, it is still relatively underrepresented in health services research. The latter field is more traditionally rooted in the evidence-based-medicine paradigm, as seen in " research that involves testing the effectiveness of various strategies to achieve changes in clinical practice, preferably applying randomised controlled trial study designs (...) " [ 4 ]. This focus on quantitative research and specifically randomised controlled trials (RCT) is visible in the idea of a hierarchy of research evidence which assumes that some research designs are objectively better than others, and that choosing a "lesser" design is only acceptable when the better ones are not practically or ethically feasible [ 5 , 6 ]. Others, however, argue that an objective hierarchy does not exist, and that, instead, the research design and methods should be chosen to fit the specific research question at hand – "questions before methods" [ 2 , 7 , 8 , 9 ]. This means that even when an RCT is possible, some research problems require a different design that is better suited to addressing them. Arguing in JAMA, Berwick uses the example of rapid response teams in hospitals, which he describes as " a complex, multicomponent intervention – essentially a process of social change" susceptible to a range of different context factors including leadership or organisation history. According to him, "[in] such complex terrain, the RCT is an impoverished way to learn. Critics who use it as a truth standard in this context are incorrect" [ 8 ] . Instead of limiting oneself to RCTs, Berwick recommends embracing a wider range of methods , including qualitative ones, which for "these specific applications, (...) are not compromises in learning how to improve; they are superior" [ 8 ].
Research problems that can be approached particularly well using qualitative methods include assessing complex multi-component interventions or systems (of change), addressing questions beyond “what works”, towards “what works for whom when, how and why”, and focussing on intervention improvement rather than accreditation [ 7 , 9 , 10 , 11 , 12 ]. Using qualitative methods can also help shed light on the “softer” side of medical treatment. For example, while quantitative trials can measure the costs and benefits of neuro-oncological treatment in terms of survival rates or adverse effects, qualitative research can help provide a better understanding of patient or caregiver stress, visibility of illness or out-of-pocket expenses.
How to conduct qualitative research?
Given that qualitative research is characterised by flexibility, openness and responsivity to context, the steps of data collection and analysis are not as separate and consecutive as they tend to be in quantitative research [ 13 , 14 ]. As Fossey puts it : “sampling, data collection, analysis and interpretation are related to each other in a cyclical (iterative) manner, rather than following one after another in a stepwise approach” [ 15 ]. The researcher can make educated decisions with regard to the choice of method, how they are implemented, and to which and how many units they are applied [ 13 ]. As shown in Fig. 1 , this can involve several back-and-forth steps between data collection and analysis where new insights and experiences can lead to adaption and expansion of the original plan. Some insights may also necessitate a revision of the research question and/or the research design as a whole. The process ends when saturation is achieved, i.e. when no relevant new information can be found (see also below: sampling and saturation). For reasons of transparency, it is essential for all decisions as well as the underlying reasoning to be well-documented.
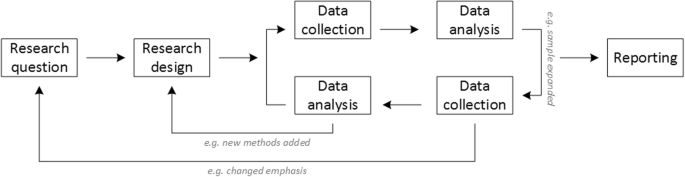
Iterative research process
While it is not always explicitly addressed, qualitative methods reflect a different underlying research paradigm than quantitative research (e.g. constructivism or interpretivism as opposed to positivism). The choice of methods can be based on the respective underlying substantive theory or theoretical framework used by the researcher [ 2 ].
Data collection
The methods of qualitative data collection most commonly used in health research are document study, observations, semi-structured interviews and focus groups [ 1 , 14 , 16 , 17 ].
Document study
Document study (also called document analysis) refers to the review by the researcher of written materials [ 14 ]. These can include personal and non-personal documents such as archives, annual reports, guidelines, policy documents, diaries or letters.
Observations
Observations are particularly useful to gain insights into a certain setting and actual behaviour – as opposed to reported behaviour or opinions [ 13 ]. Qualitative observations can be either participant or non-participant in nature. In participant observations, the observer is part of the observed setting, for example a nurse working in an intensive care unit [ 18 ]. In non-participant observations, the observer is “on the outside looking in”, i.e. present in but not part of the situation, trying not to influence the setting by their presence. Observations can be planned (e.g. for 3 h during the day or night shift) or ad hoc (e.g. as soon as a stroke patient arrives at the emergency room). During the observation, the observer takes notes on everything or certain pre-determined parts of what is happening around them, for example focusing on physician-patient interactions or communication between different professional groups. Written notes can be taken during or after the observations, depending on feasibility (which is usually lower during participant observations) and acceptability (e.g. when the observer is perceived to be judging the observed). Afterwards, these field notes are transcribed into observation protocols. If more than one observer was involved, field notes are taken independently, but notes can be consolidated into one protocol after discussions. Advantages of conducting observations include minimising the distance between the researcher and the researched, the potential discovery of topics that the researcher did not realise were relevant and gaining deeper insights into the real-world dimensions of the research problem at hand [ 18 ].
Semi-structured interviews
Hijmans & Kuyper describe qualitative interviews as “an exchange with an informal character, a conversation with a goal” [ 19 ]. Interviews are used to gain insights into a person’s subjective experiences, opinions and motivations – as opposed to facts or behaviours [ 13 ]. Interviews can be distinguished by the degree to which they are structured (i.e. a questionnaire), open (e.g. free conversation or autobiographical interviews) or semi-structured [ 2 , 13 ]. Semi-structured interviews are characterized by open-ended questions and the use of an interview guide (or topic guide/list) in which the broad areas of interest, sometimes including sub-questions, are defined [ 19 ]. The pre-defined topics in the interview guide can be derived from the literature, previous research or a preliminary method of data collection, e.g. document study or observations. The topic list is usually adapted and improved at the start of the data collection process as the interviewer learns more about the field [ 20 ]. Across interviews the focus on the different (blocks of) questions may differ and some questions may be skipped altogether (e.g. if the interviewee is not able or willing to answer the questions or for concerns about the total length of the interview) [ 20 ]. Qualitative interviews are usually not conducted in written format as it impedes on the interactive component of the method [ 20 ]. In comparison to written surveys, qualitative interviews have the advantage of being interactive and allowing for unexpected topics to emerge and to be taken up by the researcher. This can also help overcome a provider or researcher-centred bias often found in written surveys, which by nature, can only measure what is already known or expected to be of relevance to the researcher. Interviews can be audio- or video-taped; but sometimes it is only feasible or acceptable for the interviewer to take written notes [ 14 , 16 , 20 ].
Focus groups
Focus groups are group interviews to explore participants’ expertise and experiences, including explorations of how and why people behave in certain ways [ 1 ]. Focus groups usually consist of 6–8 people and are led by an experienced moderator following a topic guide or “script” [ 21 ]. They can involve an observer who takes note of the non-verbal aspects of the situation, possibly using an observation guide [ 21 ]. Depending on researchers’ and participants’ preferences, the discussions can be audio- or video-taped and transcribed afterwards [ 21 ]. Focus groups are useful for bringing together homogeneous (to a lesser extent heterogeneous) groups of participants with relevant expertise and experience on a given topic on which they can share detailed information [ 21 ]. Focus groups are a relatively easy, fast and inexpensive method to gain access to information on interactions in a given group, i.e. “the sharing and comparing” among participants [ 21 ]. Disadvantages include less control over the process and a lesser extent to which each individual may participate. Moreover, focus group moderators need experience, as do those tasked with the analysis of the resulting data. Focus groups can be less appropriate for discussing sensitive topics that participants might be reluctant to disclose in a group setting [ 13 ]. Moreover, attention must be paid to the emergence of “groupthink” as well as possible power dynamics within the group, e.g. when patients are awed or intimidated by health professionals.
Choosing the “right” method
As explained above, the school of thought underlying qualitative research assumes no objective hierarchy of evidence and methods. This means that each choice of single or combined methods has to be based on the research question that needs to be answered and a critical assessment with regard to whether or to what extent the chosen method can accomplish this – i.e. the “fit” between question and method [ 14 ]. It is necessary for these decisions to be documented when they are being made, and to be critically discussed when reporting methods and results.
Let us assume that our research aim is to examine the (clinical) processes around acute endovascular treatment (EVT), from the patient’s arrival at the emergency room to recanalization, with the aim to identify possible causes for delay and/or other causes for sub-optimal treatment outcome. As a first step, we could conduct a document study of the relevant standard operating procedures (SOPs) for this phase of care – are they up-to-date and in line with current guidelines? Do they contain any mistakes, irregularities or uncertainties that could cause delays or other problems? Regardless of the answers to these questions, the results have to be interpreted based on what they are: a written outline of what care processes in this hospital should look like. If we want to know what they actually look like in practice, we can conduct observations of the processes described in the SOPs. These results can (and should) be analysed in themselves, but also in comparison to the results of the document analysis, especially as regards relevant discrepancies. Do the SOPs outline specific tests for which no equipment can be observed or tasks to be performed by specialized nurses who are not present during the observation? It might also be possible that the written SOP is outdated, but the actual care provided is in line with current best practice. In order to find out why these discrepancies exist, it can be useful to conduct interviews. Are the physicians simply not aware of the SOPs (because their existence is limited to the hospital’s intranet) or do they actively disagree with them or does the infrastructure make it impossible to provide the care as described? Another rationale for adding interviews is that some situations (or all of their possible variations for different patient groups or the day, night or weekend shift) cannot practically or ethically be observed. In this case, it is possible to ask those involved to report on their actions – being aware that this is not the same as the actual observation. A senior physician’s or hospital manager’s description of certain situations might differ from a nurse’s or junior physician’s one, maybe because they intentionally misrepresent facts or maybe because different aspects of the process are visible or important to them. In some cases, it can also be relevant to consider to whom the interviewee is disclosing this information – someone they trust, someone they are otherwise not connected to, or someone they suspect or are aware of being in a potentially “dangerous” power relationship to them. Lastly, a focus group could be conducted with representatives of the relevant professional groups to explore how and why exactly they provide care around EVT. The discussion might reveal discrepancies (between SOPs and actual care or between different physicians) and motivations to the researchers as well as to the focus group members that they might not have been aware of themselves. For the focus group to deliver relevant information, attention has to be paid to its composition and conduct, for example, to make sure that all participants feel safe to disclose sensitive or potentially problematic information or that the discussion is not dominated by (senior) physicians only. The resulting combination of data collection methods is shown in Fig. 2 .

Possible combination of data collection methods
Attributions for icons: “Book” by Serhii Smirnov, “Interview” by Adrien Coquet, FR, “Magnifying Glass” by anggun, ID, “Business communication” by Vectors Market; all from the Noun Project
The combination of multiple data source as described for this example can be referred to as “triangulation”, in which multiple measurements are carried out from different angles to achieve a more comprehensive understanding of the phenomenon under study [ 22 , 23 ].
Data analysis
To analyse the data collected through observations, interviews and focus groups these need to be transcribed into protocols and transcripts (see Fig. 3 ). Interviews and focus groups can be transcribed verbatim , with or without annotations for behaviour (e.g. laughing, crying, pausing) and with or without phonetic transcription of dialects and filler words, depending on what is expected or known to be relevant for the analysis. In the next step, the protocols and transcripts are coded , that is, marked (or tagged, labelled) with one or more short descriptors of the content of a sentence or paragraph [ 2 , 15 , 23 ]. Jansen describes coding as “connecting the raw data with “theoretical” terms” [ 20 ]. In a more practical sense, coding makes raw data sortable. This makes it possible to extract and examine all segments describing, say, a tele-neurology consultation from multiple data sources (e.g. SOPs, emergency room observations, staff and patient interview). In a process of synthesis and abstraction, the codes are then grouped, summarised and/or categorised [ 15 , 20 ]. The end product of the coding or analysis process is a descriptive theory of the behavioural pattern under investigation [ 20 ]. The coding process is performed using qualitative data management software, the most common ones being InVivo, MaxQDA and Atlas.ti. It should be noted that these are data management tools which support the analysis performed by the researcher(s) [ 14 ].
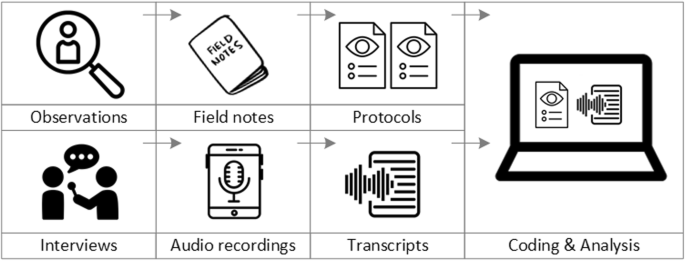
From data collection to data analysis
Attributions for icons: see Fig. 2 , also “Speech to text” by Trevor Dsouza, “Field Notes” by Mike O’Brien, US, “Voice Record” by ProSymbols, US, “Inspection” by Made, AU, and “Cloud” by Graphic Tigers; all from the Noun Project
How to report qualitative research?
Protocols of qualitative research can be published separately and in advance of the study results. However, the aim is not the same as in RCT protocols, i.e. to pre-define and set in stone the research questions and primary or secondary endpoints. Rather, it is a way to describe the research methods in detail, which might not be possible in the results paper given journals’ word limits. Qualitative research papers are usually longer than their quantitative counterparts to allow for deep understanding and so-called “thick description”. In the methods section, the focus is on transparency of the methods used, including why, how and by whom they were implemented in the specific study setting, so as to enable a discussion of whether and how this may have influenced data collection, analysis and interpretation. The results section usually starts with a paragraph outlining the main findings, followed by more detailed descriptions of, for example, the commonalities, discrepancies or exceptions per category [ 20 ]. Here it is important to support main findings by relevant quotations, which may add information, context, emphasis or real-life examples [ 20 , 23 ]. It is subject to debate in the field whether it is relevant to state the exact number or percentage of respondents supporting a certain statement (e.g. “Five interviewees expressed negative feelings towards XYZ”) [ 21 ].
How to combine qualitative with quantitative research?
Qualitative methods can be combined with other methods in multi- or mixed methods designs, which “[employ] two or more different methods [ …] within the same study or research program rather than confining the research to one single method” [ 24 ]. Reasons for combining methods can be diverse, including triangulation for corroboration of findings, complementarity for illustration and clarification of results, expansion to extend the breadth and range of the study, explanation of (unexpected) results generated with one method with the help of another, or offsetting the weakness of one method with the strength of another [ 1 , 17 , 24 , 25 , 26 ]. The resulting designs can be classified according to when, why and how the different quantitative and/or qualitative data strands are combined. The three most common types of mixed method designs are the convergent parallel design , the explanatory sequential design and the exploratory sequential design. The designs with examples are shown in Fig. 4 .

Three common mixed methods designs
In the convergent parallel design, a qualitative study is conducted in parallel to and independently of a quantitative study, and the results of both studies are compared and combined at the stage of interpretation of results. Using the above example of EVT provision, this could entail setting up a quantitative EVT registry to measure process times and patient outcomes in parallel to conducting the qualitative research outlined above, and then comparing results. Amongst other things, this would make it possible to assess whether interview respondents’ subjective impressions of patients receiving good care match modified Rankin Scores at follow-up, or whether observed delays in care provision are exceptions or the rule when compared to door-to-needle times as documented in the registry. In the explanatory sequential design, a quantitative study is carried out first, followed by a qualitative study to help explain the results from the quantitative study. This would be an appropriate design if the registry alone had revealed relevant delays in door-to-needle times and the qualitative study would be used to understand where and why these occurred, and how they could be improved. In the exploratory design, the qualitative study is carried out first and its results help informing and building the quantitative study in the next step [ 26 ]. If the qualitative study around EVT provision had shown a high level of dissatisfaction among the staff members involved, a quantitative questionnaire investigating staff satisfaction could be set up in the next step, informed by the qualitative study on which topics dissatisfaction had been expressed. Amongst other things, the questionnaire design would make it possible to widen the reach of the research to more respondents from different (types of) hospitals, regions, countries or settings, and to conduct sub-group analyses for different professional groups.
How to assess qualitative research?
A variety of assessment criteria and lists have been developed for qualitative research, ranging in their focus and comprehensiveness [ 14 , 17 , 27 ]. However, none of these has been elevated to the “gold standard” in the field. In the following, we therefore focus on a set of commonly used assessment criteria that, from a practical standpoint, a researcher can look for when assessing a qualitative research report or paper.
Assessors should check the authors’ use of and adherence to the relevant reporting checklists (e.g. Standards for Reporting Qualitative Research (SRQR)) to make sure all items that are relevant for this type of research are addressed [ 23 , 28 ]. Discussions of quantitative measures in addition to or instead of these qualitative measures can be a sign of lower quality of the research (paper). Providing and adhering to a checklist for qualitative research contributes to an important quality criterion for qualitative research, namely transparency [ 15 , 17 , 23 ].
Reflexivity
While methodological transparency and complete reporting is relevant for all types of research, some additional criteria must be taken into account for qualitative research. This includes what is called reflexivity, i.e. sensitivity to the relationship between the researcher and the researched, including how contact was established and maintained, or the background and experience of the researcher(s) involved in data collection and analysis. Depending on the research question and population to be researched this can be limited to professional experience, but it may also include gender, age or ethnicity [ 17 , 27 ]. These details are relevant because in qualitative research, as opposed to quantitative research, the researcher as a person cannot be isolated from the research process [ 23 ]. It may influence the conversation when an interviewed patient speaks to an interviewer who is a physician, or when an interviewee is asked to discuss a gynaecological procedure with a male interviewer, and therefore the reader must be made aware of these details [ 19 ].
Sampling and saturation
The aim of qualitative sampling is for all variants of the objects of observation that are deemed relevant for the study to be present in the sample “ to see the issue and its meanings from as many angles as possible” [ 1 , 16 , 19 , 20 , 27 ] , and to ensure “information-richness [ 15 ]. An iterative sampling approach is advised, in which data collection (e.g. five interviews) is followed by data analysis, followed by more data collection to find variants that are lacking in the current sample. This process continues until no new (relevant) information can be found and further sampling becomes redundant – which is called saturation [ 1 , 15 ] . In other words: qualitative data collection finds its end point not a priori , but when the research team determines that saturation has been reached [ 29 , 30 ].
This is also the reason why most qualitative studies use deliberate instead of random sampling strategies. This is generally referred to as “ purposive sampling” , in which researchers pre-define which types of participants or cases they need to include so as to cover all variations that are expected to be of relevance, based on the literature, previous experience or theory (i.e. theoretical sampling) [ 14 , 20 ]. Other types of purposive sampling include (but are not limited to) maximum variation sampling, critical case sampling or extreme or deviant case sampling [ 2 ]. In the above EVT example, a purposive sample could include all relevant professional groups and/or all relevant stakeholders (patients, relatives) and/or all relevant times of observation (day, night and weekend shift).
Assessors of qualitative research should check whether the considerations underlying the sampling strategy were sound and whether or how researchers tried to adapt and improve their strategies in stepwise or cyclical approaches between data collection and analysis to achieve saturation [ 14 ].
Good qualitative research is iterative in nature, i.e. it goes back and forth between data collection and analysis, revising and improving the approach where necessary. One example of this are pilot interviews, where different aspects of the interview (especially the interview guide, but also, for example, the site of the interview or whether the interview can be audio-recorded) are tested with a small number of respondents, evaluated and revised [ 19 ]. In doing so, the interviewer learns which wording or types of questions work best, or which is the best length of an interview with patients who have trouble concentrating for an extended time. Of course, the same reasoning applies to observations or focus groups which can also be piloted.
Ideally, coding should be performed by at least two researchers, especially at the beginning of the coding process when a common approach must be defined, including the establishment of a useful coding list (or tree), and when a common meaning of individual codes must be established [ 23 ]. An initial sub-set or all transcripts can be coded independently by the coders and then compared and consolidated after regular discussions in the research team. This is to make sure that codes are applied consistently to the research data.
Member checking
Member checking, also called respondent validation , refers to the practice of checking back with study respondents to see if the research is in line with their views [ 14 , 27 ]. This can happen after data collection or analysis or when first results are available [ 23 ]. For example, interviewees can be provided with (summaries of) their transcripts and asked whether they believe this to be a complete representation of their views or whether they would like to clarify or elaborate on their responses [ 17 ]. Respondents’ feedback on these issues then becomes part of the data collection and analysis [ 27 ].
Stakeholder involvement
In those niches where qualitative approaches have been able to evolve and grow, a new trend has seen the inclusion of patients and their representatives not only as study participants (i.e. “members”, see above) but as consultants to and active participants in the broader research process [ 31 , 32 , 33 ]. The underlying assumption is that patients and other stakeholders hold unique perspectives and experiences that add value beyond their own single story, making the research more relevant and beneficial to researchers, study participants and (future) patients alike [ 34 , 35 ]. Using the example of patients on or nearing dialysis, a recent scoping review found that 80% of clinical research did not address the top 10 research priorities identified by patients and caregivers [ 32 , 36 ]. In this sense, the involvement of the relevant stakeholders, especially patients and relatives, is increasingly being seen as a quality indicator in and of itself.
How not to assess qualitative research
The above overview does not include certain items that are routine in assessments of quantitative research. What follows is a non-exhaustive, non-representative, experience-based list of the quantitative criteria often applied to the assessment of qualitative research, as well as an explanation of the limited usefulness of these endeavours.
Protocol adherence
Given the openness and flexibility of qualitative research, it should not be assessed by how well it adheres to pre-determined and fixed strategies – in other words: its rigidity. Instead, the assessor should look for signs of adaptation and refinement based on lessons learned from earlier steps in the research process.
Sample size
For the reasons explained above, qualitative research does not require specific sample sizes, nor does it require that the sample size be determined a priori [ 1 , 14 , 27 , 37 , 38 , 39 ]. Sample size can only be a useful quality indicator when related to the research purpose, the chosen methodology and the composition of the sample, i.e. who was included and why.
Randomisation
While some authors argue that randomisation can be used in qualitative research, this is not commonly the case, as neither its feasibility nor its necessity or usefulness has been convincingly established for qualitative research [ 13 , 27 ]. Relevant disadvantages include the negative impact of a too large sample size as well as the possibility (or probability) of selecting “ quiet, uncooperative or inarticulate individuals ” [ 17 ]. Qualitative studies do not use control groups, either.
Interrater reliability, variability and other “objectivity checks”
The concept of “interrater reliability” is sometimes used in qualitative research to assess to which extent the coding approach overlaps between the two co-coders. However, it is not clear what this measure tells us about the quality of the analysis [ 23 ]. This means that these scores can be included in qualitative research reports, preferably with some additional information on what the score means for the analysis, but it is not a requirement. Relatedly, it is not relevant for the quality or “objectivity” of qualitative research to separate those who recruited the study participants and collected and analysed the data. Experiences even show that it might be better to have the same person or team perform all of these tasks [ 20 ]. First, when researchers introduce themselves during recruitment this can enhance trust when the interview takes place days or weeks later with the same researcher. Second, when the audio-recording is transcribed for analysis, the researcher conducting the interviews will usually remember the interviewee and the specific interview situation during data analysis. This might be helpful in providing additional context information for interpretation of data, e.g. on whether something might have been meant as a joke [ 18 ].
Not being quantitative research
Being qualitative research instead of quantitative research should not be used as an assessment criterion if it is used irrespectively of the research problem at hand. Similarly, qualitative research should not be required to be combined with quantitative research per se – unless mixed methods research is judged as inherently better than single-method research. In this case, the same criterion should be applied for quantitative studies without a qualitative component.
The main take-away points of this paper are summarised in Table 1 . We aimed to show that, if conducted well, qualitative research can answer specific research questions that cannot to be adequately answered using (only) quantitative designs. Seeing qualitative and quantitative methods as equal will help us become more aware and critical of the “fit” between the research problem and our chosen methods: I can conduct an RCT to determine the reasons for transportation delays of acute stroke patients – but should I? It also provides us with a greater range of tools to tackle a greater range of research problems more appropriately and successfully, filling in the blind spots on one half of the methodological spectrum to better address the whole complexity of neurological research and practice.
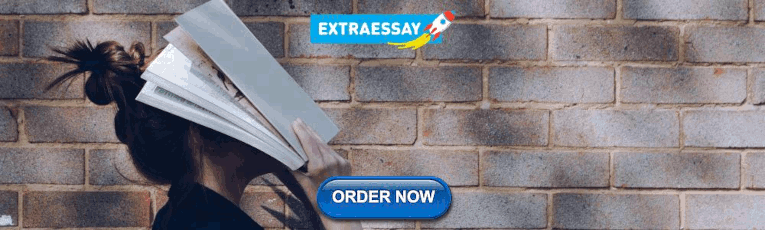
Availability of data and materials
Not applicable.
Abbreviations
Endovascular treatment
Randomised Controlled Trial
Standard Operating Procedure
Standards for Reporting Qualitative Research
Philipsen, H., & Vernooij-Dassen, M. (2007). Kwalitatief onderzoek: nuttig, onmisbaar en uitdagend. In L. PLBJ & H. TCo (Eds.), Kwalitatief onderzoek: Praktische methoden voor de medische praktijk . [Qualitative research: useful, indispensable and challenging. In: Qualitative research: Practical methods for medical practice (pp. 5–12). Houten: Bohn Stafleu van Loghum.
Chapter Google Scholar
Punch, K. F. (2013). Introduction to social research: Quantitative and qualitative approaches . London: Sage.
Kelly, J., Dwyer, J., Willis, E., & Pekarsky, B. (2014). Travelling to the city for hospital care: Access factors in country aboriginal patient journeys. Australian Journal of Rural Health, 22 (3), 109–113.
Article Google Scholar
Nilsen, P., Ståhl, C., Roback, K., & Cairney, P. (2013). Never the twain shall meet? - a comparison of implementation science and policy implementation research. Implementation Science, 8 (1), 1–12.
Howick J, Chalmers I, Glasziou, P., Greenhalgh, T., Heneghan, C., Liberati, A., Moschetti, I., Phillips, B., & Thornton, H. (2011). The 2011 Oxford CEBM evidence levels of evidence (introductory document) . Oxford Center for Evidence Based Medicine. https://www.cebm.net/2011/06/2011-oxford-cebm-levels-evidence-introductory-document/ .
Eakin, J. M. (2016). Educating critical qualitative health researchers in the land of the randomized controlled trial. Qualitative Inquiry, 22 (2), 107–118.
May, A., & Mathijssen, J. (2015). Alternatieven voor RCT bij de evaluatie van effectiviteit van interventies!? Eindrapportage. In Alternatives for RCTs in the evaluation of effectiveness of interventions!? Final report .
Google Scholar
Berwick, D. M. (2008). The science of improvement. Journal of the American Medical Association, 299 (10), 1182–1184.
Article CAS Google Scholar
Christ, T. W. (2014). Scientific-based research and randomized controlled trials, the “gold” standard? Alternative paradigms and mixed methodologies. Qualitative Inquiry, 20 (1), 72–80.
Lamont, T., Barber, N., Jd, P., Fulop, N., Garfield-Birkbeck, S., Lilford, R., Mear, L., Raine, R., & Fitzpatrick, R. (2016). New approaches to evaluating complex health and care systems. BMJ, 352:i154.
Drabble, S. J., & O’Cathain, A. (2015). Moving from Randomized Controlled Trials to Mixed Methods Intervention Evaluation. In S. Hesse-Biber & R. B. Johnson (Eds.), The Oxford Handbook of Multimethod and Mixed Methods Research Inquiry (pp. 406–425). London: Oxford University Press.
Chambers, D. A., Glasgow, R. E., & Stange, K. C. (2013). The dynamic sustainability framework: Addressing the paradox of sustainment amid ongoing change. Implementation Science : IS, 8 , 117.
Hak, T. (2007). Waarnemingsmethoden in kwalitatief onderzoek. In L. PLBJ & H. TCo (Eds.), Kwalitatief onderzoek: Praktische methoden voor de medische praktijk . [Observation methods in qualitative research] (pp. 13–25). Houten: Bohn Stafleu van Loghum.
Russell, C. K., & Gregory, D. M. (2003). Evaluation of qualitative research studies. Evidence Based Nursing, 6 (2), 36–40.
Fossey, E., Harvey, C., McDermott, F., & Davidson, L. (2002). Understanding and evaluating qualitative research. Australian and New Zealand Journal of Psychiatry, 36 , 717–732.
Yanow, D. (2000). Conducting interpretive policy analysis (Vol. 47). Thousand Oaks: Sage University Papers Series on Qualitative Research Methods.
Shenton, A. K. (2004). Strategies for ensuring trustworthiness in qualitative research projects. Education for Information, 22 , 63–75.
van der Geest, S. (2006). Participeren in ziekte en zorg: meer over kwalitatief onderzoek. Huisarts en Wetenschap, 49 (4), 283–287.
Hijmans, E., & Kuyper, M. (2007). Het halfopen interview als onderzoeksmethode. In L. PLBJ & H. TCo (Eds.), Kwalitatief onderzoek: Praktische methoden voor de medische praktijk . [The half-open interview as research method (pp. 43–51). Houten: Bohn Stafleu van Loghum.
Jansen, H. (2007). Systematiek en toepassing van de kwalitatieve survey. In L. PLBJ & H. TCo (Eds.), Kwalitatief onderzoek: Praktische methoden voor de medische praktijk . [Systematics and implementation of the qualitative survey (pp. 27–41). Houten: Bohn Stafleu van Loghum.
Pv, R., & Peremans, L. (2007). Exploreren met focusgroepgesprekken: de ‘stem’ van de groep onder de loep. In L. PLBJ & H. TCo (Eds.), Kwalitatief onderzoek: Praktische methoden voor de medische praktijk . [Exploring with focus group conversations: the “voice” of the group under the magnifying glass (pp. 53–64). Houten: Bohn Stafleu van Loghum.
Carter, N., Bryant-Lukosius, D., DiCenso, A., Blythe, J., & Neville, A. J. (2014). The use of triangulation in qualitative research. Oncology Nursing Forum, 41 (5), 545–547.
Boeije H: Analyseren in kwalitatief onderzoek: Denken en doen, [Analysis in qualitative research: Thinking and doing] vol. Den Haag Boom Lemma uitgevers; 2012.
Hunter, A., & Brewer, J. (2015). Designing Multimethod Research. In S. Hesse-Biber & R. B. Johnson (Eds.), The Oxford Handbook of Multimethod and Mixed Methods Research Inquiry (pp. 185–205). London: Oxford University Press.
Archibald, M. M., Radil, A. I., Zhang, X., & Hanson, W. E. (2015). Current mixed methods practices in qualitative research: A content analysis of leading journals. International Journal of Qualitative Methods, 14 (2), 5–33.
Creswell, J. W., & Plano Clark, V. L. (2011). Choosing a Mixed Methods Design. In Designing and Conducting Mixed Methods Research . Thousand Oaks: SAGE Publications.
Mays, N., & Pope, C. (2000). Assessing quality in qualitative research. BMJ, 320 (7226), 50–52.
O'Brien, B. C., Harris, I. B., Beckman, T. J., Reed, D. A., & Cook, D. A. (2014). Standards for reporting qualitative research: A synthesis of recommendations. Academic Medicine : Journal of the Association of American Medical Colleges, 89 (9), 1245–1251.
Saunders, B., Sim, J., Kingstone, T., Baker, S., Waterfield, J., Bartlam, B., Burroughs, H., & Jinks, C. (2018). Saturation in qualitative research: Exploring its conceptualization and operationalization. Quality and Quantity, 52 (4), 1893–1907.
Moser, A., & Korstjens, I. (2018). Series: Practical guidance to qualitative research. Part 3: Sampling, data collection and analysis. European Journal of General Practice, 24 (1), 9–18.
Marlett, N., Shklarov, S., Marshall, D., Santana, M. J., & Wasylak, T. (2015). Building new roles and relationships in research: A model of patient engagement research. Quality of Life Research : an international journal of quality of life aspects of treatment, care and rehabilitation, 24 (5), 1057–1067.
Demian, M. N., Lam, N. N., Mac-Way, F., Sapir-Pichhadze, R., & Fernandez, N. (2017). Opportunities for engaging patients in kidney research. Canadian Journal of Kidney Health and Disease, 4 , 2054358117703070–2054358117703070.
Noyes, J., McLaughlin, L., Morgan, K., Roberts, A., Stephens, M., Bourne, J., Houlston, M., Houlston, J., Thomas, S., Rhys, R. G., et al. (2019). Designing a co-productive study to overcome known methodological challenges in organ donation research with bereaved family members. Health Expectations . 22(4):824–35.
Piil, K., Jarden, M., & Pii, K. H. (2019). Research agenda for life-threatening cancer. European Journal Cancer Care (Engl), 28 (1), e12935.
Hofmann, D., Ibrahim, F., Rose, D., Scott, D. L., Cope, A., Wykes, T., & Lempp, H. (2015). Expectations of new treatment in rheumatoid arthritis: Developing a patient-generated questionnaire. Health Expectations : an international journal of public participation in health care and health policy, 18 (5), 995–1008.
Jun, M., Manns, B., Laupacis, A., Manns, L., Rehal, B., Crowe, S., & Hemmelgarn, B. R. (2015). Assessing the extent to which current clinical research is consistent with patient priorities: A scoping review using a case study in patients on or nearing dialysis. Canadian Journal of Kidney Health and Disease, 2 , 35.
Elsie Baker, S., & Edwards, R. (2012). How many qualitative interviews is enough? In National Centre for Research Methods Review Paper . National Centre for Research Methods. http://eprints.ncrm.ac.uk/2273/4/how_many_interviews.pdf .
Sandelowski, M. (1995). Sample size in qualitative research. Research in Nursing & Health, 18 (2), 179–183.
Sim, J., Saunders, B., Waterfield, J., & Kingstone, T. (2018). Can sample size in qualitative research be determined a priori? International Journal of Social Research Methodology, 21 (5), 619–634.
Download references
Acknowledgements
no external funding.
Author information
Authors and affiliations.
Department of Neurology, Heidelberg University Hospital, Im Neuenheimer Feld 400, 69120, Heidelberg, Germany
Loraine Busetto, Wolfgang Wick & Christoph Gumbinger
Clinical Cooperation Unit Neuro-Oncology, German Cancer Research Center, Heidelberg, Germany
Wolfgang Wick
You can also search for this author in PubMed Google Scholar
Contributions
LB drafted the manuscript; WW and CG revised the manuscript; all authors approved the final versions.
Corresponding author
Correspondence to Loraine Busetto .
Ethics declarations
Ethics approval and consent to participate, consent for publication, competing interests.
The authors declare no competing interests.
Additional information
Publisher’s note.
Springer Nature remains neutral with regard to jurisdictional claims in published maps and institutional affiliations.
Rights and permissions
Open Access This article is licensed under a Creative Commons Attribution 4.0 International License, which permits use, sharing, adaptation, distribution and reproduction in any medium or format, as long as you give appropriate credit to the original author(s) and the source, provide a link to the Creative Commons licence, and indicate if changes were made. The images or other third party material in this article are included in the article's Creative Commons licence, unless indicated otherwise in a credit line to the material. If material is not included in the article's Creative Commons licence and your intended use is not permitted by statutory regulation or exceeds the permitted use, you will need to obtain permission directly from the copyright holder. To view a copy of this licence, visit http://creativecommons.org/licenses/by/4.0/ .
Reprints and permissions
About this article
Cite this article.
Busetto, L., Wick, W. & Gumbinger, C. How to use and assess qualitative research methods. Neurol. Res. Pract. 2 , 14 (2020). https://doi.org/10.1186/s42466-020-00059-z
Download citation
Received : 30 January 2020
Accepted : 22 April 2020
Published : 27 May 2020
DOI : https://doi.org/10.1186/s42466-020-00059-z
Share this article
Anyone you share the following link with will be able to read this content:
Sorry, a shareable link is not currently available for this article.
Provided by the Springer Nature SharedIt content-sharing initiative
- Qualitative research
- Mixed methods
- Quality assessment
Neurological Research and Practice
ISSN: 2524-3489
- Submission enquiries: Access here and click Contact Us
- General enquiries: [email protected]
Qualitative data analysis: how market researchers can crack the code
What is qualitative data, what are the ingredients of a good qualitative data analysis, how to conduct an enlightening qualitative data analysis, the pros and cons of qualitative data analysis, get great results from qualitative data analysis.
When numbers fall short and you need the full story, qualitative data analysis comes to the rescue. Instead of following assumptions based on numerical data, qualitative data analysis methods let you dig deeper. Qualitative data analysis examines non-numerical data – words, images, and observations, to uncover themes, patterns, and meanings.
And in this article, we’ll tell you exactly how to do it yourself, in-house.
Qualitative data analysis uncovers the stories and feelings behind numbers. Qualitative methods gain information from conversations, interviews, and observations, capturing what people think and why they act a certain way. Unlike hard numbers, qualitative data helps us see the color and texture of people’s opinions, experiences, and emotions.
Examples of the textual data that often makes up qualitative data pieces are a user’s detailed feedback on a mobile app’s usability, a shopper’s narrative about choosing eco-friendly products, or observational notes on customer behavior in a retail setting.
This type of qualitative data collection helps us understand real feelings and thoughts, and goes beyond numbers and assumptions.
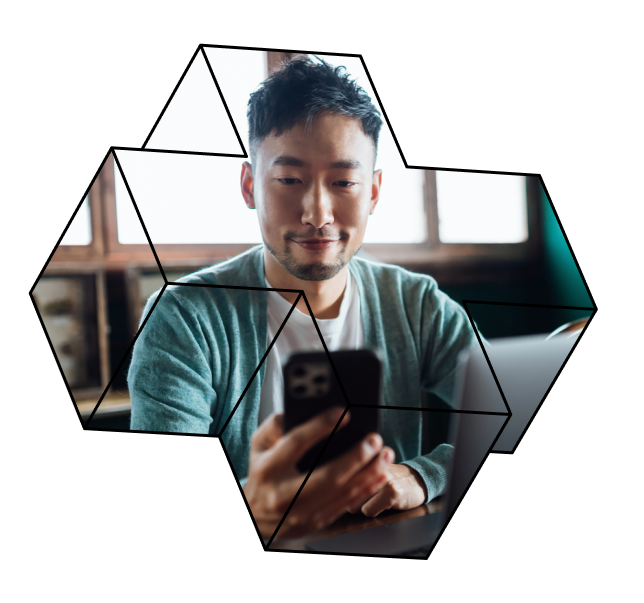
Get qual research with Video Responses
Unlock the voice of the consumer with qualitative insights. Get fast, reliable Video Responses straight from your target customers.
There’s a big difference between knowing that 50% of customers prefer your new product and understanding the nuanced reasons behind that preference.
It’s easy to get blinded by shiny numbers. In this case, a preference signals that you’re doing something great. But not knowing what, means you can’t replicate it, or double down on it to crank up that 50% even more.
So what you’ll need to do is dig into the ‘why’ behind the ‘what’. And we mean really dig. A strong qualitative data analysis process really aims at not putting words inside your customers mouths but letting them speak for themselves.
Another example is when a company finds out through a quick quantitative data survey that customers rate their service 4 out of 5. Which isn’t bad. But how can they improve it – or even work to maintain it? Guesswork is lethal here, yet it’s what so many companies resort to.
Which leads to obvious follow-up actions that are usually not customer-centric. Let’s say that this company assumes people are mostly happy because of their quick response times. So, they implement chatbots to take care of the first part of conversations, to speed things up even more. What could be wrong with that?
But what if through in-depth interviews, they could have discovered that the personal touch from the staff right from the get-go is what customers really value?
In consumer research, these nuances are gold. They allow your team to make finely tuned adjustments that resonate deeply with your audience. It’s what helps you move beyond the one-size-fits-all approach suggested by quantitative data.
So if you want to start making experiences and products that feel personal and relevant to each customer, here are some ways to approach qualitative data research.
Content analysis: unveiling customer sentiments
What it is: Content analysis involves examining texts, reviews, and comments to identify frequently occurring words and sentiments, providing a quantitative measure of qualitative feedback.
Good to know:
- Focus on reviews, comments, and social media posts.
- Look for repeating words and sentiments to identify trends.
- Helps prioritize actions based on frequently mentioned topics by customers.
Chances are, you already have a lot of content that can be analyzed for qualitative data research. In that case, content analysis is your go-to approach to getting started. Content analysis means zooming in on recurring words, phrases, and sentiments scattered across reviews and comments.
Dig into reviews, comments, and emails and start flagging words and phrases that keep coming back. These can help you identify areas for improvement, but also show you what really is working.
This way, content analysis offers a quantitative measure of qualitative feedback, enabling you to prioritize actions based on what’s most mentioned by your customers, when they’re not prompted or asked anything specifically.
By systematically categorizing and quantifying this feedback, you’ll be able to make informed decisions on product features, marketing messages, and even future design innovations.
Narrative analysis: connecting through stories
What it is: Narrative analysis delves into customers’ stories to understand their experiences, decisions, and emotions throughout their journey with your brand.
- Analyze customer stories from initial contact to purchase.
- Focus on customers’ thoughts and feelings at each stage.
- Useful for identifying communication and support opportunities.
A lot of times brands are mostly interested in the beginning and end of a customer journey: how do I get in front of customers, and how do I get in their shopping basket?
But the story of what happens between those two moments is just as, if not more important. And with narrative analysis, you can help connect the dots.
You won’t just be looking at the touchpoints there were, but also what customers were thinking and feeling at each stage. By interpreting qualitative data, you can create a full story from start to finish on how customers think and feel and make decisions in your market.
And that is so much more than just a nice story. Narrative analysis shows you where you can swoop in, where you should change your communications or where you should offer more support — for a happy ever after.
Discourse analysis: shaping perceptions through conversation
What it is: Discourse analysis examines language and communication on platforms like social media to understand how they influence public perception and consumer behavior.
- Explore broader conversations around topics relevant to your brand.
- Understand cultural, social, and environmental contexts.
- Align your messaging with audience values and lead discussions.
Discourse analysis looks at the broader conversation around topics relevant to your brand. This qualitative data analysis method looks at how language and communication on platforms like social media shape public perception and influence consumer behavior.
Discourse analysis not just about what’s being said about your brand and products; it’s about understanding the cultural, social, and environmental currents that drive these conversations.
For example, when customers discuss “sustainability,” they’re not just talking about your specific packaging; they’re engaging in a larger dialogue about corporate responsibility, environmental impact, and ethical consumption.
Discourse analysis helps you grasp the nuances of these discussions, revealing how your brand can authentically contribute to and lead within these conversations.
This strategic insight allows you to align your messaging with your audience’s values, build credibility, and position your brand as a leader in meaningful sustainability efforts.
By engaging with and influencing the discourse, you can adapt to current consumer expectations but you can even take it a step further, and shape future trends and behaviors in alignment with your brand’s values and goals.
Thematic analysis: finding overlapping themes in chaos
What it is: Thematic analysis seeks to find common themes within qualitative data, moving beyond individual opinions to uncover broader patterns.
- Organize feedback into distinct themes.
- Requires systematic data collection and coding.
- Offers clear, actionable insights for different business areas.
Plenty of brands are already sitting on qualitative data from thousands of customer interactions, which might seem like a jumble of individual opinions and experiences.
You might look at them and think ‘ ha, humans really all want or value different things ’. But there will be overlap, and that is where the real value lies.
Thematic analysis aims at finding common themes in this qualitative data. You move beyond surface-level chaos by categorizing all pieces of feedback into distinct themes.
These themes could range from specific product features, such as “battery life” in electronics, to broader experiential factors, like “customer service excellence” or “ease of use.” By identifying these recurring patterns, you gain a clearer, more organized understanding of your customers’ priorities and pain points.
One of the benefits of thematic analysis is that it helps you organize a wide range of feedback into clear, actionable insights for each team in your business. You may uncover themes about the product, about communication, or other parts of your business that customers get exposed to. In other words: every business could benefit from some thematic analysis.
Grounded theory: building strategies from real feedback
What it is: Grounded theory uses early feedback from users to develop theories and strategies that meet their needs, focusing on continuous improvement.
- Start with feedback from early users or testers.
- Engage deeply with feedback to guide product development.
- Ideal for new services or products, ensuring they align with customer expectations.
For those launching a new service, grounded theory takes feedback from early users and starts building from there. It uses real, raw customer thoughts to shape a strategy that better meets their needs.
This approach isn’t just about collecting data; it’s about letting qualitative data direct your next moves, ensuring your innovations are not just shots in the dark but informed, strategic decisions aimed at fulfilling genuine customer needs.
When you adopt grounded theory, you commit to a process of continuous improvement and adaptation. As feedback starts rolling in from those first users or beta testers, you’re given a unique opportunity to see your product through the eyes of those it’s meant to serve.
This early-stage feedback is gold—unfiltered, direct, and incredibly insightful. It tells you what’s resonating with your audience, what’s missing the mark, and, crucially, how to adjust your offering for better alignment with customer expectations.
Bear in mind that when done right, grounded theory goes beyond merely reacting to feedback. It’s about proactively seeking it out and engaging with it. This means not just reading comments or reviews, but diving deeper through follow-up questions, interviews, or focus groups to really understand the why behind the feedback.
Diving into qualitative data analysis can feel like a big task for many brands. There’s often worry about how much time it’ll take. Or how much money. And then there’s the question of whether all that detail might lead you off track instead of to clear answers.
After all, businesses move fast these days, and spending a lot of time on a research project doesn’t always fit the schedule.
But those worries don’t have to stop you. With the right plan and the best tools, you can dodge those issues. Start by creating a roadmap, so you know what the next few days, weeks or months will look like. See? It’s less daunting already.
Below, we’ll break the whole process down into simple steps. We’re going to walk through how to tackle qualitative data analysis without getting bogged down.
1. Transcribing interviews and collecting qualitative survey data
When it comes to qualitative research, if something’s said, it’s crucial. And that means you gotta write it down. Or at least have a tool to do it for you.
‘ ’I don’t wanna miss a thing’ ’ is your theme song for this step.
Every chuckle, pause, or sigh can give you insights into what your customers really think and feel. Now, I know what you’re thinking: “Transcribing interviews sounds like a lot of work. Let alone conducting all of them!”
But here’s the good news—using Attest makes this step a pleasant breeze on a hot summer night. With Attest, you can send out surveys that dive deep into all the qualitative questions you’ve been itching to ask. Our platform is designed to capture rich, detailed responses in a way that is easy to search and analyze.
This means you don’t have to worry about spending hours transcribing interviews. The responses are already there in writing, ready for you to analyze. This doesn’t just save time; it ensures accuracy. You’re getting the unfiltered voice of your customer, directly and conveniently. No more playing detective with hours of audio recordings.
2. Organize data and identify common patterns
Next, sift through your transcribed interviews, survey responses, and notes. Your goal here is to spot patterns or themes that crop up repeatedly.
This could be similar sentiments about a product feature or shared experiences with your service. Organizing data helps you identify themes that move from scattered bits of feedback to clear, common threads that tell a bigger story.
3. Using tools to make the process easier
There are plenty of software tools out there designed to help with qualitative data analysis. These tools can help you code your qualitative data, which means tagging parts of the text with keywords or themes, making it easier to organize and analyze textual data. They can save you a heap of time and help you stay accurate and consistent in your analysis.
That’s where Attest’s innovative Video Responses come into play, offering a seamless and impactful way to gather and analyze qualitative data directly from your target audience – all in the same platform as your quantitative data.
Here’s how we transform qualitative research:
- Easy to use : Attest’s platform lets you quickly add video questions to surveys, making it straightforward to collect in-depth feedback.
- Fast insights : With automated transcriptions, you can swiftly analyze video responses, identifying key themes without the wait.
- Reliable data : Attest ensures feedback comes from a diverse, representative audience, giving you confidence in the insights you gather.
- Rich context : Video responses capture the full spectrum of customer emotions and nuances, providing a deeper understanding than text alone.
- Seamless integration : Mix qualitative and quantitative data effortlessly, for a comprehensive view of your customer base.
As consumer behaviors and preferences continue to evolve at lightning speed, it’s products like Video Responses that will help brands win more based on decisions made with a deeper understanding of their customers. Jeremy King, CEO and Founder of Attest
4. Highlight context alongside data where relevant
Understanding the context in which feedback is provided is crucial in qualitative analysis. It’s not just about what your customers are saying; it’s also about why they’re saying it at that particular moment. This deeper layer of insight can significantly impact how you interpret and act on the data you collect.
Why context matters:
- Timing : Feedback given right after a new product launch can contain initial impressions that might evolve over time. Similarly, responses collected during a major sale or promotion might be influenced by the excitement or urgency of the moment.
- External factors : Consider the broader environment. For example, feedback during a major social event, a public holiday, or even a global crisis can be colored by the emotions and experiences of that time. This can shift priorities or change the way people interact with your brand.
- Customer journey stage : The stage of the customer journey at which feedback is given can also provide important context. Early-stage feedback might focus on first impressions and expectations, while later-stage feedback could offer deeper insights into user experience and satisfaction.
How to account for context in your qualitative analysis:
- Document the circumstances : When collecting data, make a note of the timing and any relevant external factors.
- Consider the source : Different platforms can also provide context. For instance, feedback from a public social media post might differ from what’s shared in a private survey due to the public nature of the medium.
- Use context to guide action : Let the context inform how you prioritize and respond to feedback. Initial excitement might warrant a quick thank-you message, while deeper, contextual insights might lead to product or service improvements.
5. Seek participant validation
Once you’ve got some preliminary findings, it’s a good idea to circle back to your participants. This could mean confirming your interpretations with them or diving deeper into certain areas.
This will help you be sure your analysis aligns with your respondents’ intended meanings and experiences. Plus, it shows respect for their contributions and can uncover even richer insights.
6. Compile a final report with a mix of data and visualization techniques
Finally, bring your analysis to life in a report that mixes clear, concise writing with visual elements like charts, graphs, and quotes.
Visualization helps make complex insights more accessible, engaging, and persuasive. Your report should not only present what you’ve found but also tell the story of how these research findings can influence decisions and strategies.
7. Put insights into action
The real value of qualitative data analysis lies in its application. Use the insights to inform decisions, refine strategies, and better meet your customers’ needs. This is where your analytical journey makes a tangible impact on your business.
Previously when we’ve had to do qualitative research, it’s taken months and months. Attest gets the information that we need quickly. By the very next day we’re able to implement some of the changes and then go back for round two. Simon Gray, Head of Marketing, Zzoomm
Qualitative data analysis looks at the human side of data. It offers insights that numbers alone can’t provide. But like all research methods, even qualitative data analysis methods have their strengths and weaknesses, especially when it comes to shaping a marketing plan that hits the mark.
Advantages of qualitative data analysis
Bringing qualitative data into your strategy brings about transformative advantages that can significantly transform how your business connects with your audience and adapts to the market. Without further ado, let’s look at the benefits it brings.
Qualitative data gives you truly rich insights
Want to go beyond meeting the explicit needs of your customers, and also address their unspoken desires and creating experiences that truly matter to them? Qualitative analysis offers an unparalleled depth of understanding by capturing the subtleties and complexities of customer behavior and sentiment.
By engaging directly with your audience through interviews, focus groups, or social media interactions, you gain nuanced perspectives that quantitative data alone cannot provide. These rich insights enable you to craft marketing strategies and product innovations that resonate on a deeper level with your audience.
Qualitative data is a lot more flexible than numbers
Numbers can be quite limiting. The benefit of qualitative analysis is that you’re not confined to a predetermined set of questions or outcomes.
Instead, you have the freedom to explore new directions, probe interesting findings further, and let the data guide your research process. This flexibility means your research process can evolve in real-time, responding to unexpected insights or shifting market dynamics.
Qualitative data is great for strategic decision-making
The insights gained from qualitative analysis can significantly inform strategic decision-making. By understanding the nuances of customer feedback, you can make informed and detailed choices about where to allocate resources, which product features to prioritize, and how to position your business in the market.
You can go beyond generic moves in the right direction and make sure you hit the nail on the head on the first try, instead of slowly creeping towards it.
Qualitative research data fuels innovation and differentiation
Businesses are always looking for ways to innovate, but where to look? It’s often less obvious and loud than you think. And innovation doesn’t always have to be massively disrupting or a big pivot. Sometimes small changes made by listening to your customers’ unmet needs and emerging desires will tell you everything you need to know for your next product launch.
Innovation that brings information in from customers is often much more to-the-point than innovation that comes from inside the business, where people tend to be focused on the product and possibilities around it a lot. But try a different approach every once in a while. Listen to the people that use your product, not just the ones who create it.
Qualitative research data will fuel a customer-centric culture
Qualitative data puts your customer’s voice front and center. It highlights their stories, opinions, and feelings, making your marketing strategy more empathetic and customer-focused. This will allow you to build stronger connections with your audience.
Not by any marketing gimmicks, creating online communities or carefully curated UGC campaigns, but by speaking directly to customers’ experiences and emotions. Using qualitative data across your organization brings transformative effects, deeply embedding a culture of attentiveness, adaptability, and unwavering focus on the customer at every level of your business.
This approach does more than just inform product development or marketing strategies—it reshapes the very foundation of how your business operates and interacts with the people it was created for.
Disadvantages of qualitative data analysis
We’re not going to pretend that qualitative data analysis is something you can do on autopilot. But while qualitative data analysis brings its set of challenges, understanding these can help you navigate through them more effectively.
Moreover, with the right tools and strategies, the benefits you gain far outweigh any of the potential drawbacks we’ve listed below. Here’s a closer look at these challenges and how to turn them into opportunities:
Qualitative data analysis can time-consuming
Yes, qualitative analysis often* demands time and resources. The depth it requires—from collecting detailed narratives to transcribing and interpreting vast amounts of text—can seem daunting. However, this investment in time is what uncovers the nuanced insights that quantitative methods might miss.
*… but not always. With Attest’s Video Responses, you get reliable qual insights fast, alongside your quantitative data!
Qualitative data analysis is pretty subjective
Of course, the interpretive nature of qualitative data analysis does introduce the risk of subjectivity and bias. But ignoring all opinions and thoughts around your product or brand is arguably worse. What this challenge underscores all the more is the importance of a structured, systematic approach to analysis.
By implementing standardized procedures for coding and analyzing data, and employing tools that facilitate consistency across the process, you can mitigate the risks of subjective bias.
And if you involve a diverse team in the analysis process and make sure you pick a representative set of respondents, qualitative research can enable a deeper, more empathetic understanding of ALL your customers; experiences and perspectives.
Qualitative data analysis methods come with scaling issues
Qualitative data collection can indeed be tricky to scale and generalize across a broader market. But who said you can only do qualitative research with in-person interviews? With the right survey tool, like Attest, you can ask quantitative questions at scale, to an audience that is large and diverse.
Our participant audience consists of 125 million people spread across 59 countries, and once you send out a survey, results can come back in mere minutes or hours. So if scalability is holding you back, online surveys with video responses are the answer.
Unlock the full potential of qualitative data analysis with Attest. Gain actionable insights, bridge the gap between raw data and emotional intelligence, and make informed decisions. Discover how Attest can support your journey to deeper consumer understanding at Attest for insights professionals and learn about our commitment to data quality .
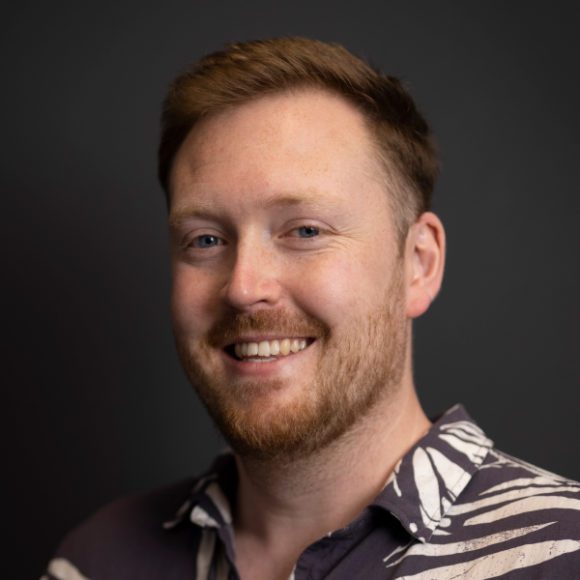
Customer Research Manager
Related articles
How to find gaps in the market – 8 important steps, market analysis, consumer research guide: best process and examples, hybrid and flexible working at attest, subscribe to our newsletter.
Fill in your email and we’ll drop fresh insights and events info into your inbox each week.
* I agree to receive communications from Attest. Privacy Policy .
You're now subscribed to our mailing list to receive exciting news, reports, and other updates!
Qualitative vs. Quantitative Data: 7 Key Differences
Qualitative data is information you can describe with words rather than numbers.
Quantitative data is information represented in a measurable way using numbers.
One type of data isn’t better than the other.
To conduct thorough research, you need both. But knowing the difference between them is important if you want to harness the full power of both qualitative and quantitative data.
In this post, we’ll explore seven key differences between these two types of data.
#1. The Type of Data
The single biggest difference between quantitative and qualitative data is that one deals with numbers, and the other deals with concepts and ideas.
The words “qualitative” and “quantitative” are really similar, which can make it hard to keep track of which one is which. I like to think of them this way:
- Quantitative = quantity = numbers-related data
- Qualitative = quality = descriptive data
Qualitative data—the descriptive one—usually involves written or spoken words, images, or even objects. It’s collected in all sorts of ways: video recordings, interviews, open-ended survey responses, and field notes, for example.
I like how researcher James W. Crick defines qualitative research in a 2021 issue of the Journal of Strategic Marketing : “Qualitative research is designed to generate in-depth and subjective findings to build theory.”
In other words, qualitative research helps you learn more about a topic—usually from a primary, or firsthand, source—so you can form ideas about what it means. This type of data is often rich in detail, and its interpretation can vary depending on who’s analyzing it.
Here’s what I mean: if you ask five different people to observe how 60 kittens behave when presented with a hamster wheel, you’ll get five different versions of the same event.
Quantitative data, on the other hand, is all about numbers and statistics. There’s no wiggle room when it comes to interpretation. In our kitten scenario, quantitative data might show us that of the 60 kittens presented with a hamster wheel, 40 pawed at it, 5 jumped inside and started spinning, and 15 ignored it completely.
There’s no ifs, ands, or buts about the numbers. They just are.
#2. When to Use Each Type of Data
You should use both quantitative and quantitative data to make decisions for your business.
Quantitative data helps you get to the what . Qualitative data unearths the why .
Quantitative data collects surface information, like numbers. Qualitative data dives deep beneath these same numbers and fleshes out the nuances there.
Research projects can often benefit from both types of data, which is why you’ll see the term “mixed-method” research in peer-reviewed journals. The term “mixed-method” refers to using both quantitative and qualitative methods in a study.
So, maybe you’re diving into original research. Or maybe you’re looking at other peoples’ studies to make an important business decision. In either case, you can use both quantitative and qualitative data to guide you.
Imagine you want to start a company that makes hamster wheels for cats. You run that kitten experiment, only to learn that most kittens aren’t all that interested in the hamster wheel. That’s what your quantitative data seems to say. Of the 60 kittens who participated in the study, only 5 hopped into the wheel.
But 40 of the kittens pawed at the wheel. According to your quantitative data, these 40 kittens touched the wheel but did not get inside.
This is where your qualitative data comes into play. Why did these 40 kittens touch the wheel but stop exploring it? You turn to the researchers’ observations. Since there were five different researchers, you have five sets of detailed notes to study.
From these observations, you learn that many of the kittens seemed frightened when the wheel moved after they pawed it. They grew suspicious of the structure, meowing and circling it, agitated.
One researcher noted that the kittens seemed desperate to enjoy the wheel, but they didn’t seem to feel it was safe.
So your idea isn’t a flop, exactly.
It just needs tweaking.
According to your quantitative data, 75% of the kittens studied either touched or actively participated in the hamster wheel. Your qualitative data suggests more kittens would have jumped into the wheel if it hadn’t moved so easily when they pawed at it.
You decide to make your kitten wheel sturdier and try the whole test again with a new set of kittens. Hopefully, this time a higher percentage of your feline participants will hop in and enjoy the fun.
This is a very simplistic and fictional example of how a mixed-method approach can help you make important choices for your business.
#3. Data You Have Access To
When you can swing it, you should look at both qualitative and quantitative data before you make any big decisions.
But this is where we come to another big difference between quantitative vs. qualitative data: it’s a lot easier to source qualitative data than quantitative data.
Why? Because it’s easy to run a survey, host a focus group, or conduct a round of interviews. All you have to do is hop on SurveyMonkey or Zoom and you’re on your way to gathering original qualitative data.
And yes, you can get some quantitative data here. If you run a survey and 45 customers respond, you can collect demographic data and yes/no answers for that pool of 45 respondents.
But this is a relatively small sample size. (More on why this matters in a moment.)
To tell you anything meaningful, quantitative data must achieve statistical significance.
If it’s been a while since your college statistics class, here’s a refresh: statistical significance is a measuring stick. It tells you whether the results you get are due to a specific cause or if they can be attributed to random chance.
To achieve statistical significance in a study, you have to be really careful to set the study up the right way and with a meaningful sample size.
This doesn’t mean it’s impossible to get quantitative data. But unless you have someone on your team who knows all about null hypotheses and p-values and statistical analysis, you might need to outsource quantitative research.
Plenty of businesses do this, but it’s pricey.
When you’re just starting out or you’re strapped for cash, qualitative data can get you valuable information—quickly and without gouging your wallet.
#4. Big vs. Small Sample Size
Another reason qualitative data is more accessible? It requires a smaller sample size to achieve meaningful results.
Even one person’s perspective brings value to a research project—ever heard of a case study?
The sweet spot depends on the purpose of the study, but for qualitative market research, somewhere between 10-40 respondents is a good number.
Any more than that and you risk reaching saturation. That’s when you keep getting results that echo each other and add nothing new to the research.
Quantitative data needs enough respondents to reach statistical significance without veering into saturation territory.
The ideal sample size number is usually higher than it is for qualitative data. But as with qualitative data, there’s no single, magic number. It all depends on statistical values like confidence level, population size, and margin of error.
Because it often requires a larger sample size, quantitative research can be more difficult for the average person to do on their own.
#5. Methods of Analysis
Running a study is just the first part of conducting qualitative and quantitative research.
After you’ve collected data, you have to study it. Find themes, patterns, consistencies, inconsistencies. Interpret and organize the numbers or survey responses or interview recordings. Tidy it all up into something you can draw conclusions from and apply to various situations.
This is called data analysis, and it’s done in completely different ways for qualitative vs. quantitative data.
For qualitative data, analysis includes:
- Data prep: Make all your qualitative data easy to access and read. This could mean organizing survey results by date, or transcribing interviews, or putting photographs into a slideshow format.
- Coding: No, not that kind. Think color coding, like you did for your notes in school. Assign colors or codes to specific attributes that make sense for your study—green for positive emotions, for instance, and red for angry emotions. Then code each of your responses.
- Thematic analysis: Organize your codes into themes and sub-themes, looking for the meaning—and relationships—within each one.
- Content analysis: Quantify the number of times certain words or concepts appear in your data. If this sounds suspiciously like quantitative research to you, it is. Sort of. It’s looking at qualitative data with a quantitative eye to identify any recurring themes or patterns.
- Narrative analysis: Look for similar stories and experiences and group them together. Study them and draw inferences from what they say.
- Interpret and document: As you organize and analyze your qualitative data, decide what the findings mean for you and your project.
You can often do qualitative data analysis manually or with tools like NVivo and ATLAS.ti. These tools help you organize, code, and analyze your subjective qualitative data.
Quantitative data analysis is a lot less subjective. Here’s how it generally goes:
- Data cleaning: Remove all inconsistencies and inaccuracies from your data. Check for duplicates, incorrect formatting (mistakenly writing a 1.00 value as 10.1, for example), and incomplete numbers.
- Summarize data with descriptive statistics: Use mean, median, mode, range, and standard deviation to summarize your data.
- Interpret the data with inferential statistics: This is where it gets more complicated. Instead of simply summarizing stats, you’ll now use complicated mathematical and statistical formulas and tests—t-tests, chi-square tests, analysis of variance (ANOVA), and correlation, for starters—to assign meaning to your data.
Researchers generally use sophisticated data analysis tools like RapidMiner and Tableau to help them do this work.
#6. Flexibility
Quantitative research tends to be less flexible than qualitative research. It relies on structured data collection methods, which researchers must set up well before the study begins.
This rigid structure is part of what makes quantitative data so reliable. But the downside here is that once you start the study, it’s hard to change anything without negatively affecting the results. If something unexpected comes up—or if new questions arise—researchers can’t easily change the scope of the study.
Qualitative research is a lot more flexible. This is why qualitative data can go deeper than quantitative data. If you’re interviewing someone and an interesting, unexpected topic comes up, you can immediately explore it.
Other qualitative research methods offer flexibility, too. Most big survey software brands allow you to build flexible surveys using branching and skip logic. These features let you customize which questions respondents see based on the answers they give.
This flexibility is unheard of in quantitative research. But even though it’s as flexible as an Olympic gymnast, qualitative data can be less reliable—and harder to validate.
#7. Reliability and Validity
Quantitative data is more reliable than qualitative data. Numbers can’t be massaged to fit a certain bias. If you replicate the study—in other words, run the exact same quantitative study two or more times—you should get nearly identical results each time. The same goes if another set of researchers runs the same study using the same methods.
This is what gives quantitative data that reliability factor.
There are a few key benefits here. First, reliable data means you can confidently make generalizations that apply to a larger population. It also means the data is valid and accurately measures whatever it is you’re trying to measure.
And finally, reliable data is trustworthy. Big industries like healthcare, marketing, and education frequently use quantitative data to make life-or-death decisions. The more reliable and trustworthy the data, the more confident these decision-makers can be when it’s time to make critical choices.
Unlike quantitative data, qualitative data isn’t overtly reliable. It’s not easy to replicate. If you send out the same qualitative survey on two separate occasions, you’ll get a new mix of responses. Your interpretations of the data might look different, too.
There’s still incredible value in qualitative data, of course—and there are ways to make sure the data is valid. These include:
- Member checking: Circling back with survey, interview, or focus group respondents to make sure you accurately summarized and interpreted their feedback.
- Triangulation: Using multiple data sources, methods, or researchers to cross-check and corroborate findings.
- Peer debriefing: Showing the data to peers—other researchers—so they can review the research process and its findings and provide feedback on both.
Whether you’re dealing with qualitative or quantitative data, transparency, accuracy, and validity are crucial. Focus on sourcing (or conducting) quantitative research that’s easy to replicate and qualitative research that’s been peer-reviewed.
With rock-solid data like this, you can make critical business decisions with confidence.
Make your website better. Instantly.
Keep reading about user experience.
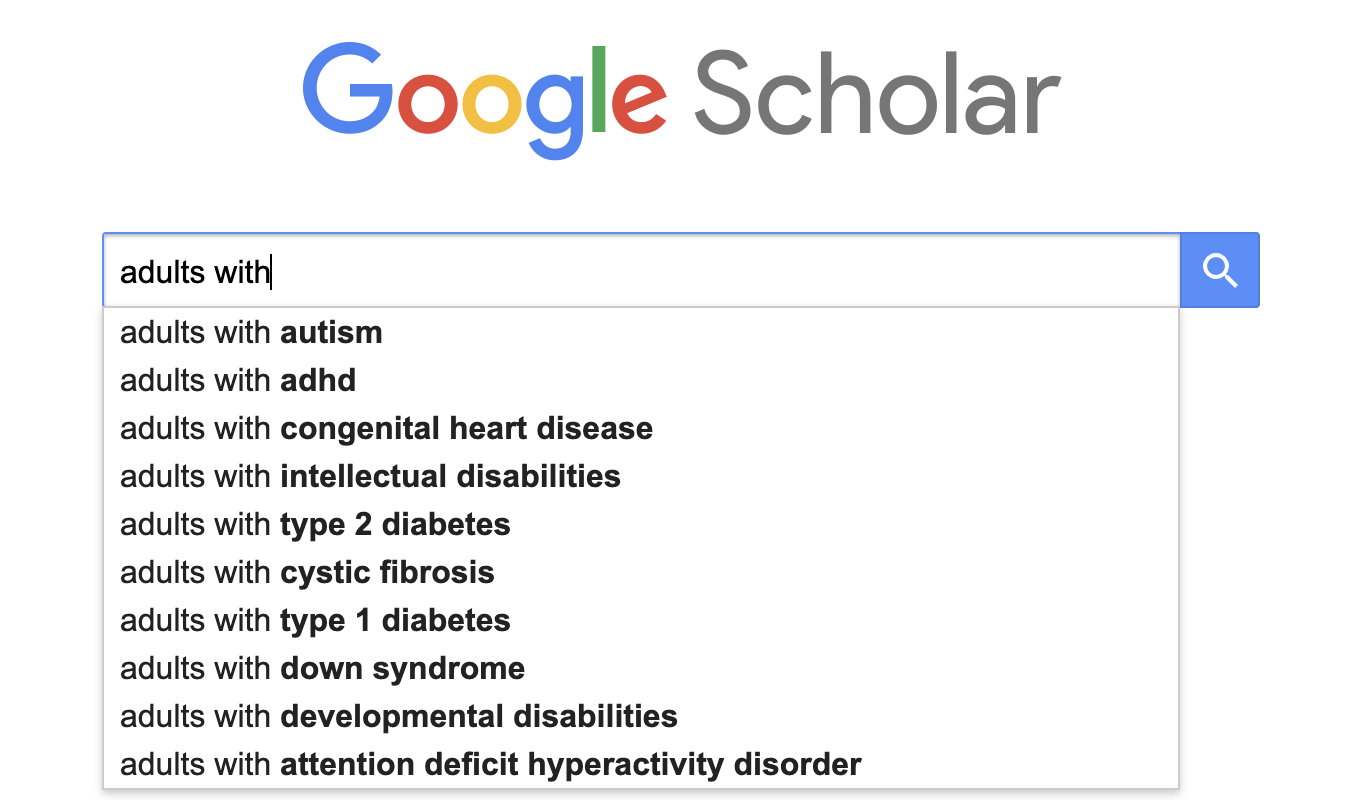
7 Qualitative Data Examples and Why They Work
Qualitative data presents information using descriptive language, images, and videos instead of numbers. To help make sense of this type of data—as opposed to quantitative…
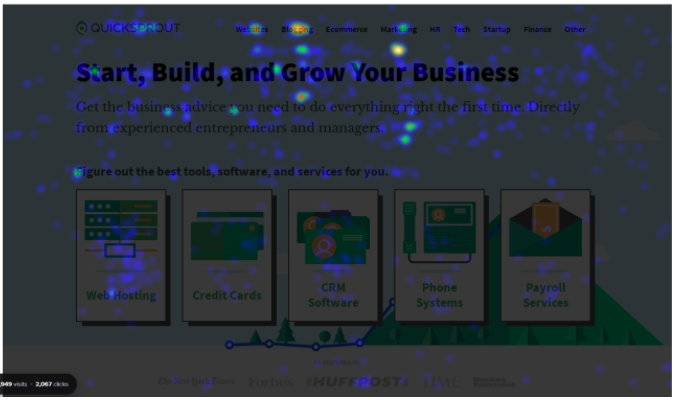
The 5 Best Usability Testing Tools Compared
Usability testing helps designers, product managers, and other teams figure out how easily users can use a website, app, or product. With these tools, user…
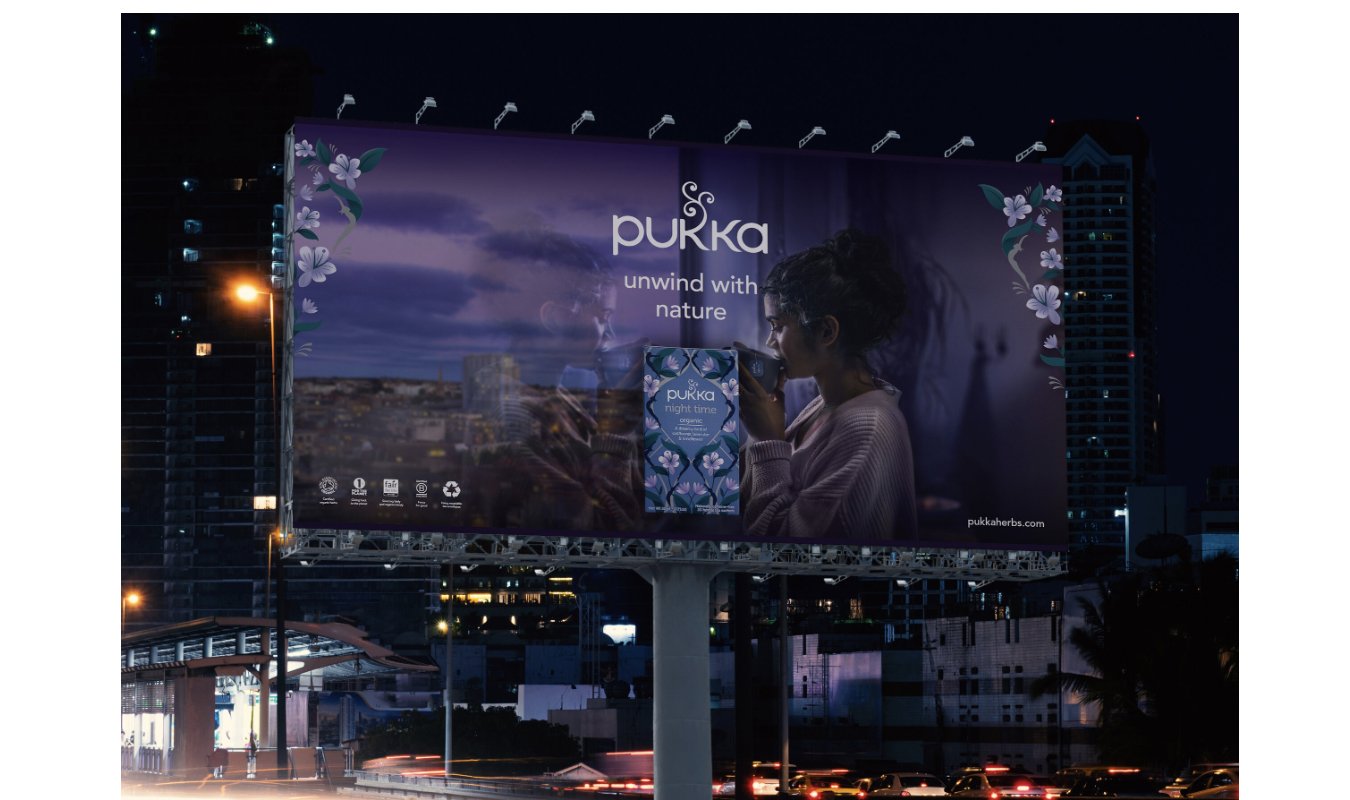
5 Qualitative Data Analysis Methods + When To Use Each
Qualitative data analysis is the work of organizing and interpreting descriptive data. Interview recordings, open-ended survey responses, and focus group observations all yield descriptive—qualitative—information. This…
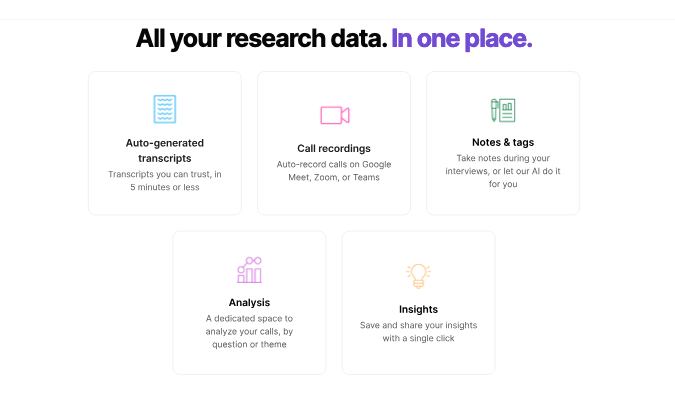
The 5 Best UX Research Tools Compared
UX research tools help designers, product managers, and other teams understand users and how they interact with a company’s products and services. The tools provide…
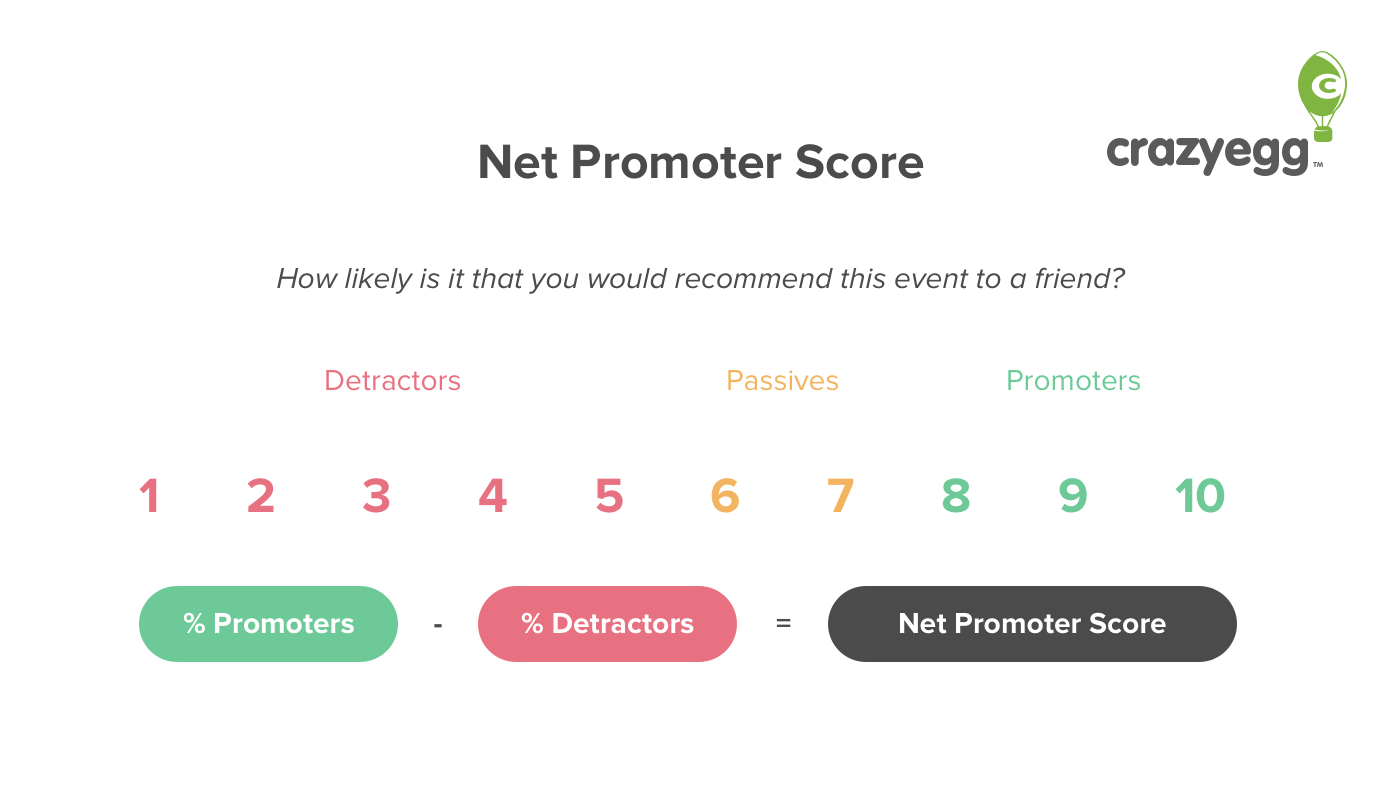
Qualitative data is information you can describe with words rather than numbers. Quantitative data is information represented in a measurable way using numbers. One type…
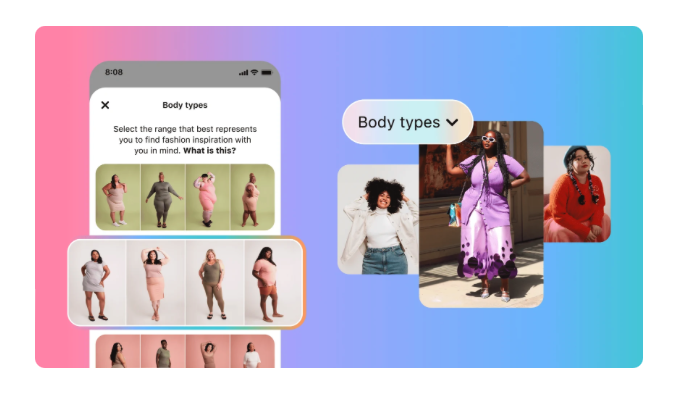
6 Real Ways AI Has Improved the User Experience
It seems like every other company is bragging about their AI-enhanced user experiences. Consumers and the UX professionals responsible for designing great user experiences are…
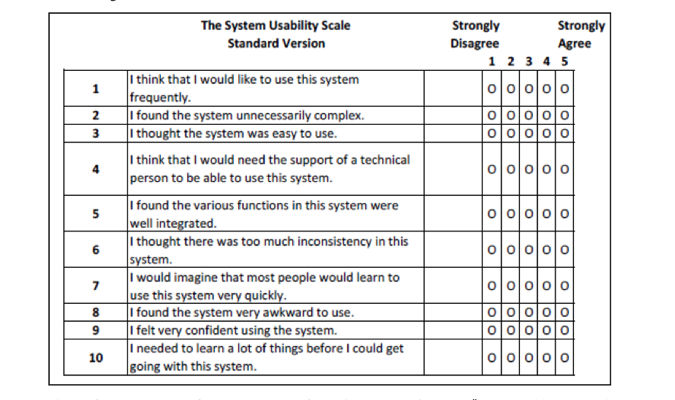
12 Key UX Metrics: What They Mean + How To Calculate Each
UX metrics help identify where users struggle when using an app or website and where they are successful. The data collected helps designers, developers, and…
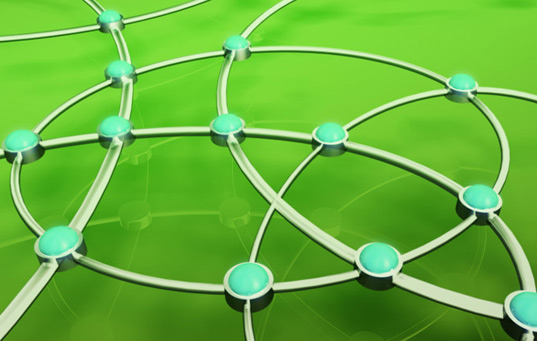
5 Key Principles Of Good Website Usability
Ease of use is a common expectation for a site to be considered well designed. Over the past few years, we have been used to…
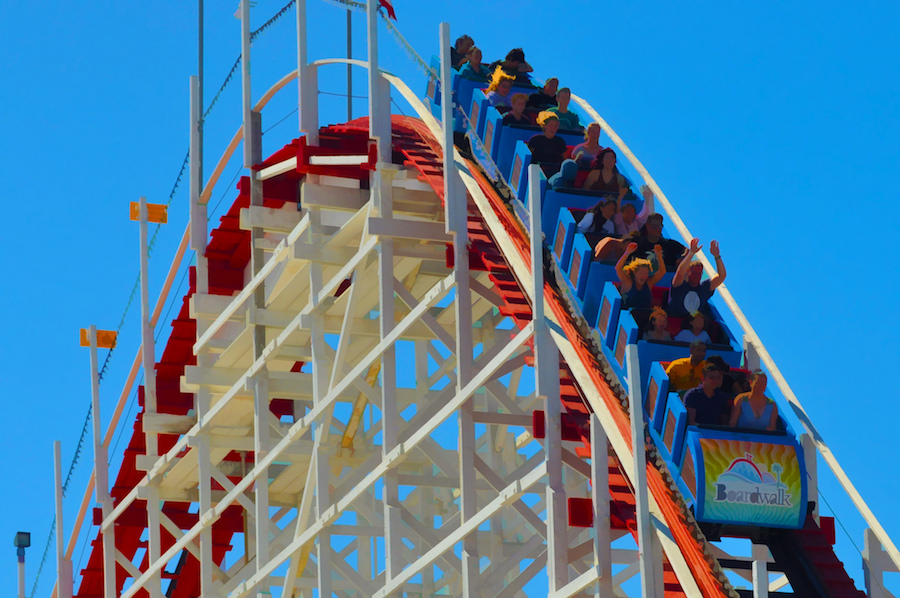
20 Ways to Speed Up Your Website and Improve Conversion in 2024
Think that speeding up your website isn’t important? Big mistake. A one-second delay in page load time yields: Your site taking a few extra seconds to…
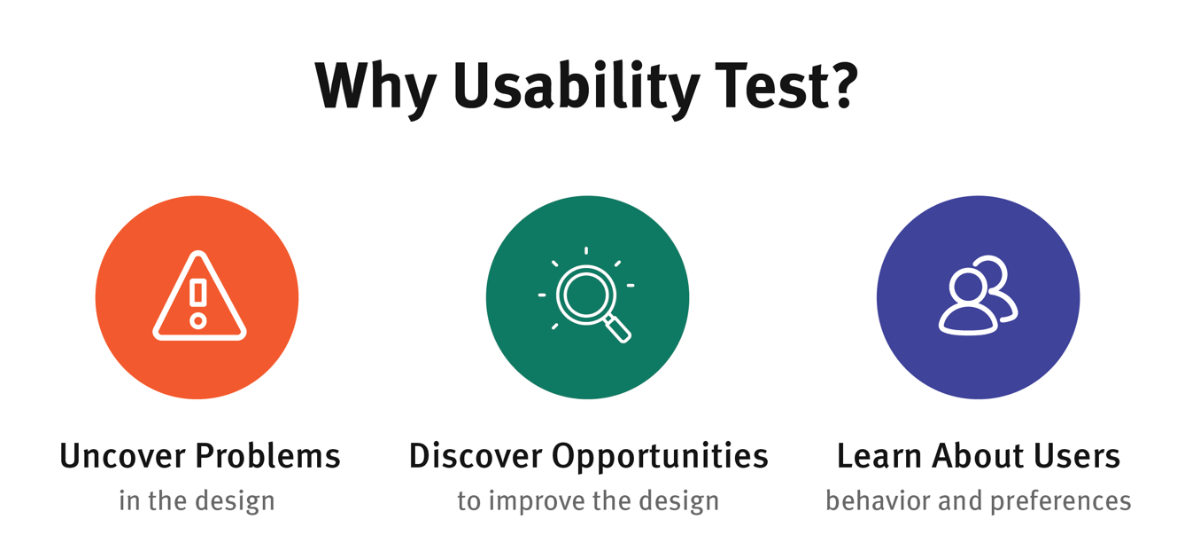
How to Do Usability Testing Right
User experience is one of the most important aspects of having a successful website, app, piece of software, or any other product that you’ve built. …
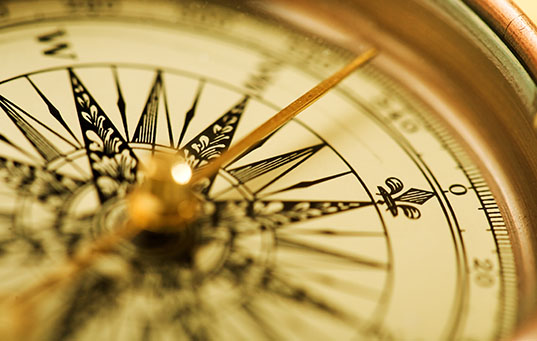
Website Navigation: Tips, Examples and Best Practices
Your website’s navigation structure has a huge impact on conversions, sales, and bounce rates. If visitors can’t figure out where to find what they want,…
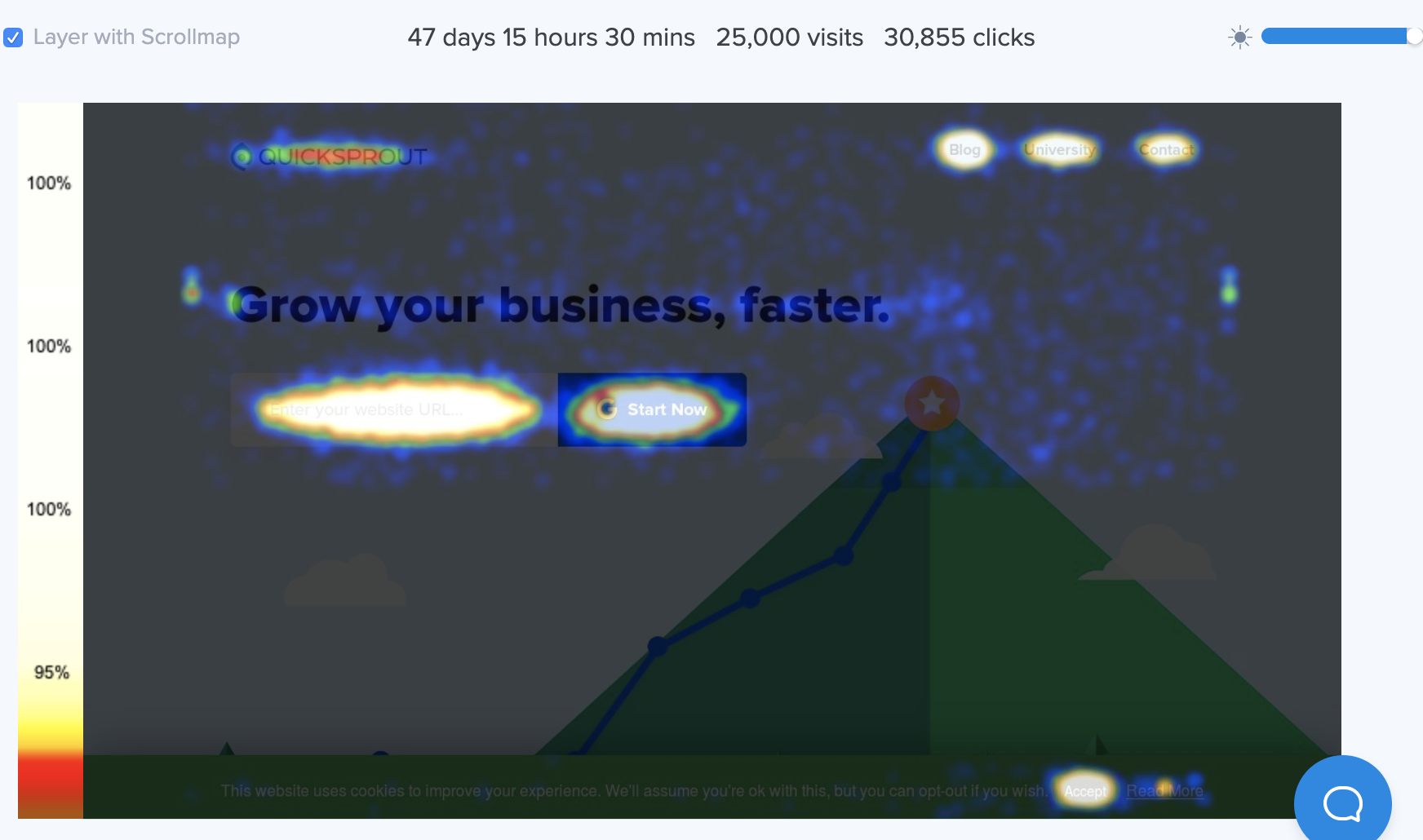
How to Create a Heatmap Online for Free in Less Than 15 Minutes
A heatmap is an extremely valuable tool for anyone with a website. Heatmaps are a visual representation of crucial website data. With just a simple…
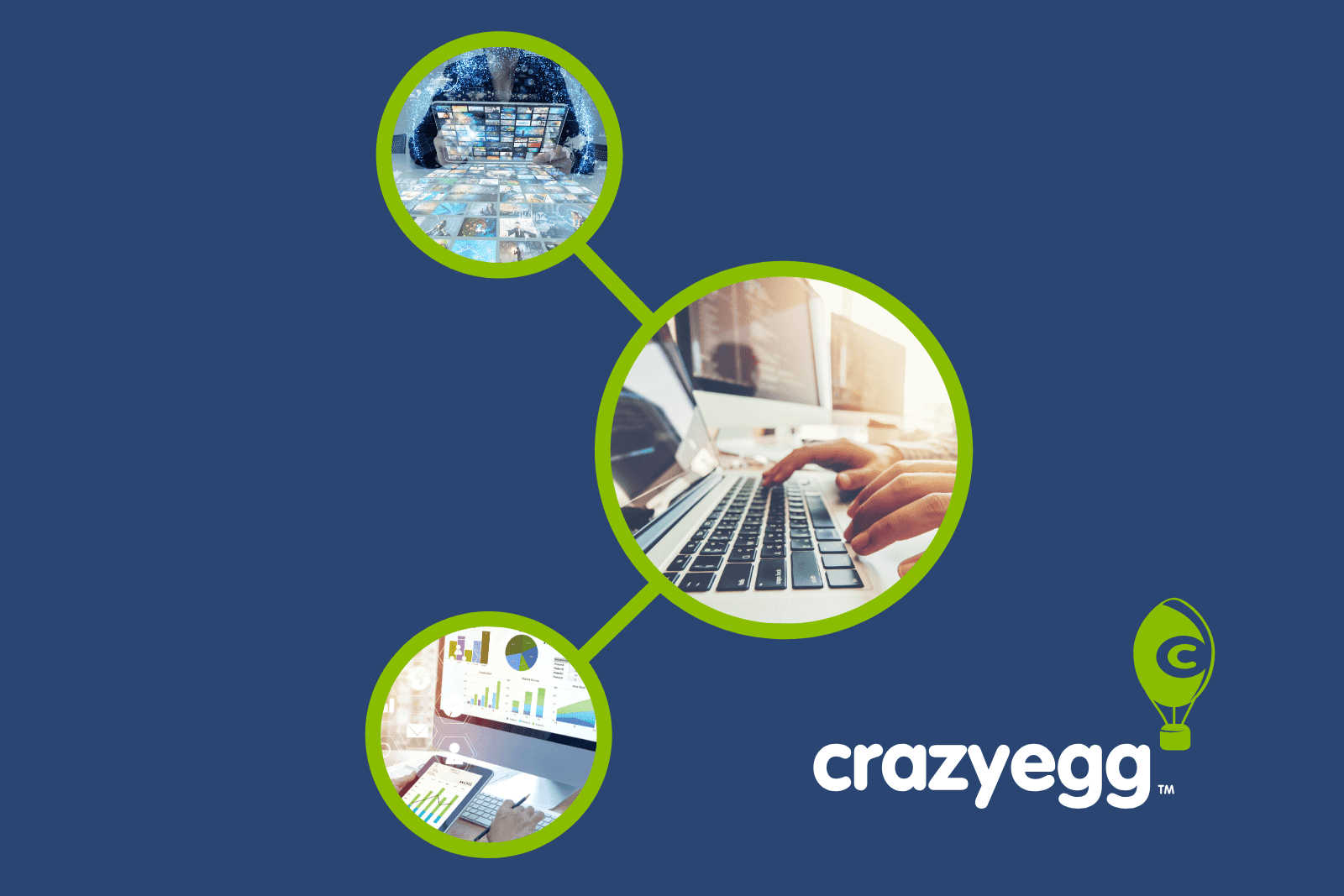
Website Analysis: Our 4-Step Process Plus the Tools and Examples You Need to Get Started
The problem with a lot of the content that covers website analysis is that the term “website analysis” can refer to a lot of different things—and…
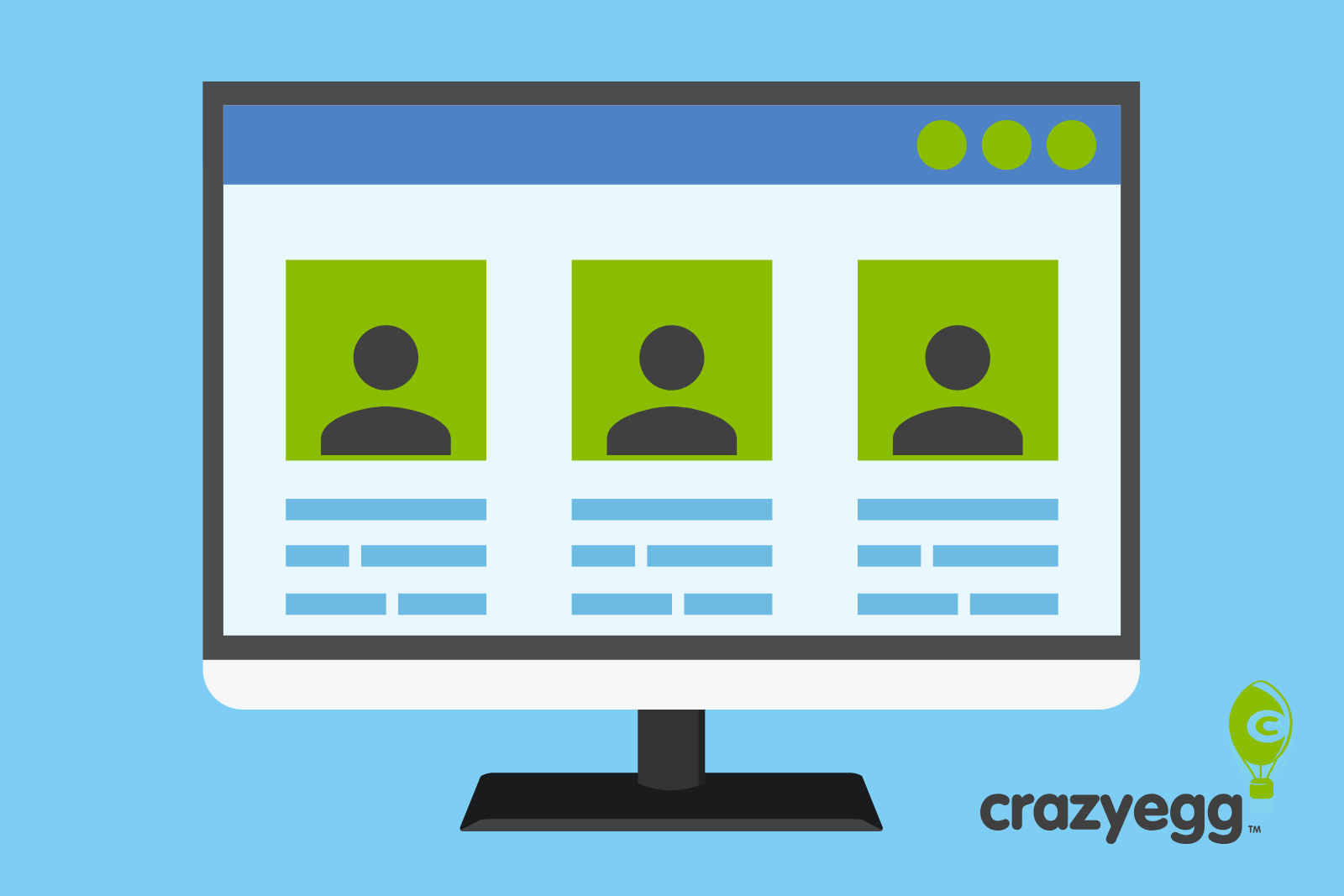
How to Improve My Website: Grow Engagement and Conversions by Fixing 3 Common Website Problems
Here, we show you how to use Google Analytics together with Crazy Egg’s heatmap reports to easily identify and fix 3 common website problems.
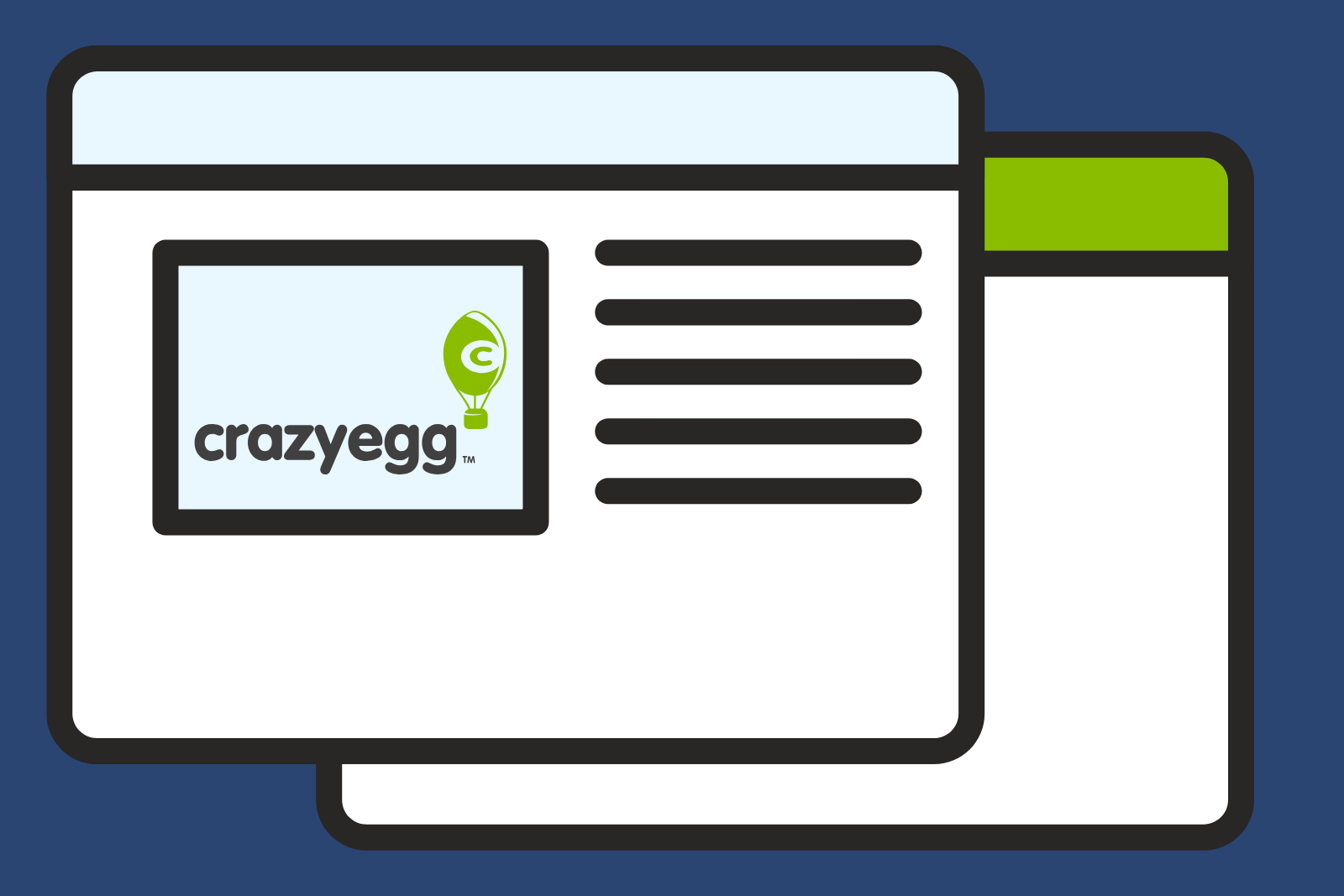
Comprehensive Guide to Website Usability Testing (With Tools and Software to Help)
We share the 3-step process for the website usability testing we recommend to our customers, plus the tools to pull actionable insights out of the process.
Over 300,000 websites use Crazy Egg to improve what's working, fix what isn't and test new ideas.
Last Updated on September 14, 2021
- Privacy Policy

Home » Content Analysis – Methods, Types and Examples
Content Analysis – Methods, Types and Examples
Table of Contents

Content Analysis
Definition:
Content analysis is a research method used to analyze and interpret the characteristics of various forms of communication, such as text, images, or audio. It involves systematically analyzing the content of these materials, identifying patterns, themes, and other relevant features, and drawing inferences or conclusions based on the findings.
Content analysis can be used to study a wide range of topics, including media coverage of social issues, political speeches, advertising messages, and online discussions, among others. It is often used in qualitative research and can be combined with other methods to provide a more comprehensive understanding of a particular phenomenon.
Types of Content Analysis
There are generally two types of content analysis:
Quantitative Content Analysis
This type of content analysis involves the systematic and objective counting and categorization of the content of a particular form of communication, such as text or video. The data obtained is then subjected to statistical analysis to identify patterns, trends, and relationships between different variables. Quantitative content analysis is often used to study media content, advertising, and political speeches.
Qualitative Content Analysis
This type of content analysis is concerned with the interpretation and understanding of the meaning and context of the content. It involves the systematic analysis of the content to identify themes, patterns, and other relevant features, and to interpret the underlying meanings and implications of these features. Qualitative content analysis is often used to study interviews, focus groups, and other forms of qualitative data, where the researcher is interested in understanding the subjective experiences and perceptions of the participants.
Methods of Content Analysis
There are several methods of content analysis, including:
Conceptual Analysis
This method involves analyzing the meanings of key concepts used in the content being analyzed. The researcher identifies key concepts and analyzes how they are used, defining them and categorizing them into broader themes.
Content Analysis by Frequency
This method involves counting and categorizing the frequency of specific words, phrases, or themes that appear in the content being analyzed. The researcher identifies relevant keywords or phrases and systematically counts their frequency.
Comparative Analysis
This method involves comparing the content of two or more sources to identify similarities, differences, and patterns. The researcher selects relevant sources, identifies key themes or concepts, and compares how they are represented in each source.
Discourse Analysis
This method involves analyzing the structure and language of the content being analyzed to identify how the content constructs and represents social reality. The researcher analyzes the language used and the underlying assumptions, beliefs, and values reflected in the content.
Narrative Analysis
This method involves analyzing the content as a narrative, identifying the plot, characters, and themes, and analyzing how they relate to the broader social context. The researcher identifies the underlying messages conveyed by the narrative and their implications for the broader social context.
Content Analysis Conducting Guide
Here is a basic guide to conducting a content analysis:
- Define your research question or objective: Before starting your content analysis, you need to define your research question or objective clearly. This will help you to identify the content you need to analyze and the type of analysis you need to conduct.
- Select your sample: Select a representative sample of the content you want to analyze. This may involve selecting a random sample, a purposive sample, or a convenience sample, depending on the research question and the availability of the content.
- Develop a coding scheme: Develop a coding scheme or a set of categories to use for coding the content. The coding scheme should be based on your research question or objective and should be reliable, valid, and comprehensive.
- Train coders: Train coders to use the coding scheme and ensure that they have a clear understanding of the coding categories and procedures. You may also need to establish inter-coder reliability to ensure that different coders are coding the content consistently.
- Code the content: Code the content using the coding scheme. This may involve manually coding the content, using software, or a combination of both.
- Analyze the data: Once the content is coded, analyze the data using appropriate statistical or qualitative methods, depending on the research question and the type of data.
- Interpret the results: Interpret the results of the analysis in the context of your research question or objective. Draw conclusions based on the findings and relate them to the broader literature on the topic.
- Report your findings: Report your findings in a clear and concise manner, including the research question, methodology, results, and conclusions. Provide details about the coding scheme, inter-coder reliability, and any limitations of the study.
Applications of Content Analysis
Content analysis has numerous applications across different fields, including:
- Media Research: Content analysis is commonly used in media research to examine the representation of different groups, such as race, gender, and sexual orientation, in media content. It can also be used to study media framing, media bias, and media effects.
- Political Communication : Content analysis can be used to study political communication, including political speeches, debates, and news coverage of political events. It can also be used to study political advertising and the impact of political communication on public opinion and voting behavior.
- Marketing Research: Content analysis can be used to study advertising messages, consumer reviews, and social media posts related to products or services. It can provide insights into consumer preferences, attitudes, and behaviors.
- Health Communication: Content analysis can be used to study health communication, including the representation of health issues in the media, the effectiveness of health campaigns, and the impact of health messages on behavior.
- Education Research : Content analysis can be used to study educational materials, including textbooks, curricula, and instructional materials. It can provide insights into the representation of different topics, perspectives, and values.
- Social Science Research: Content analysis can be used in a wide range of social science research, including studies of social media, online communities, and other forms of digital communication. It can also be used to study interviews, focus groups, and other qualitative data sources.
Examples of Content Analysis
Here are some examples of content analysis:
- Media Representation of Race and Gender: A content analysis could be conducted to examine the representation of different races and genders in popular media, such as movies, TV shows, and news coverage.
- Political Campaign Ads : A content analysis could be conducted to study political campaign ads and the themes and messages used by candidates.
- Social Media Posts: A content analysis could be conducted to study social media posts related to a particular topic, such as the COVID-19 pandemic, to examine the attitudes and beliefs of social media users.
- Instructional Materials: A content analysis could be conducted to study the representation of different topics and perspectives in educational materials, such as textbooks and curricula.
- Product Reviews: A content analysis could be conducted to study product reviews on e-commerce websites, such as Amazon, to identify common themes and issues mentioned by consumers.
- News Coverage of Health Issues: A content analysis could be conducted to study news coverage of health issues, such as vaccine hesitancy, to identify common themes and perspectives.
- Online Communities: A content analysis could be conducted to study online communities, such as discussion forums or social media groups, to understand the language, attitudes, and beliefs of the community members.
Purpose of Content Analysis
The purpose of content analysis is to systematically analyze and interpret the content of various forms of communication, such as written, oral, or visual, to identify patterns, themes, and meanings. Content analysis is used to study communication in a wide range of fields, including media studies, political science, psychology, education, sociology, and marketing research. The primary goals of content analysis include:
- Describing and summarizing communication: Content analysis can be used to describe and summarize the content of communication, such as the themes, topics, and messages conveyed in media content, political speeches, or social media posts.
- Identifying patterns and trends: Content analysis can be used to identify patterns and trends in communication, such as changes over time, differences between groups, or common themes or motifs.
- Exploring meanings and interpretations: Content analysis can be used to explore the meanings and interpretations of communication, such as the underlying values, beliefs, and assumptions that shape the content.
- Testing hypotheses and theories : Content analysis can be used to test hypotheses and theories about communication, such as the effects of media on attitudes and behaviors or the framing of political issues in the media.
When to use Content Analysis
Content analysis is a useful method when you want to analyze and interpret the content of various forms of communication, such as written, oral, or visual. Here are some specific situations where content analysis might be appropriate:
- When you want to study media content: Content analysis is commonly used in media studies to analyze the content of TV shows, movies, news coverage, and other forms of media.
- When you want to study political communication : Content analysis can be used to study political speeches, debates, news coverage, and advertising.
- When you want to study consumer attitudes and behaviors: Content analysis can be used to analyze product reviews, social media posts, and other forms of consumer feedback.
- When you want to study educational materials : Content analysis can be used to analyze textbooks, instructional materials, and curricula.
- When you want to study online communities: Content analysis can be used to analyze discussion forums, social media groups, and other forms of online communication.
- When you want to test hypotheses and theories : Content analysis can be used to test hypotheses and theories about communication, such as the framing of political issues in the media or the effects of media on attitudes and behaviors.
Characteristics of Content Analysis
Content analysis has several key characteristics that make it a useful research method. These include:
- Objectivity : Content analysis aims to be an objective method of research, meaning that the researcher does not introduce their own biases or interpretations into the analysis. This is achieved by using standardized and systematic coding procedures.
- Systematic: Content analysis involves the use of a systematic approach to analyze and interpret the content of communication. This involves defining the research question, selecting the sample of content to analyze, developing a coding scheme, and analyzing the data.
- Quantitative : Content analysis often involves counting and measuring the occurrence of specific themes or topics in the content, making it a quantitative research method. This allows for statistical analysis and generalization of findings.
- Contextual : Content analysis considers the context in which the communication takes place, such as the time period, the audience, and the purpose of the communication.
- Iterative : Content analysis is an iterative process, meaning that the researcher may refine the coding scheme and analysis as they analyze the data, to ensure that the findings are valid and reliable.
- Reliability and validity : Content analysis aims to be a reliable and valid method of research, meaning that the findings are consistent and accurate. This is achieved through inter-coder reliability tests and other measures to ensure the quality of the data and analysis.
Advantages of Content Analysis
There are several advantages to using content analysis as a research method, including:
- Objective and systematic : Content analysis aims to be an objective and systematic method of research, which reduces the likelihood of bias and subjectivity in the analysis.
- Large sample size: Content analysis allows for the analysis of a large sample of data, which increases the statistical power of the analysis and the generalizability of the findings.
- Non-intrusive: Content analysis does not require the researcher to interact with the participants or disrupt their natural behavior, making it a non-intrusive research method.
- Accessible data: Content analysis can be used to analyze a wide range of data types, including written, oral, and visual communication, making it accessible to researchers across different fields.
- Versatile : Content analysis can be used to study communication in a wide range of contexts and fields, including media studies, political science, psychology, education, sociology, and marketing research.
- Cost-effective: Content analysis is a cost-effective research method, as it does not require expensive equipment or participant incentives.
Limitations of Content Analysis
While content analysis has many advantages, there are also some limitations to consider, including:
- Limited contextual information: Content analysis is focused on the content of communication, which means that contextual information may be limited. This can make it difficult to fully understand the meaning behind the communication.
- Limited ability to capture nonverbal communication : Content analysis is limited to analyzing the content of communication that can be captured in written or recorded form. It may miss out on nonverbal communication, such as body language or tone of voice.
- Subjectivity in coding: While content analysis aims to be objective, there may be subjectivity in the coding process. Different coders may interpret the content differently, which can lead to inconsistent results.
- Limited ability to establish causality: Content analysis is a correlational research method, meaning that it cannot establish causality between variables. It can only identify associations between variables.
- Limited generalizability: Content analysis is limited to the data that is analyzed, which means that the findings may not be generalizable to other contexts or populations.
- Time-consuming: Content analysis can be a time-consuming research method, especially when analyzing a large sample of data. This can be a disadvantage for researchers who need to complete their research in a short amount of time.
About the author
Muhammad Hassan
Researcher, Academic Writer, Web developer
You may also like

Grounded Theory – Methods, Examples and Guide

Graphical Methods – Types, Examples and Guide

Regression Analysis – Methods, Types and Examples

Phenomenology – Methods, Examples and Guide

Histogram – Types, Examples and Making Guide

Multidimensional Scaling – Types, Formulas and...
- DOI: 10.1002/cae.22749
- Corpus ID: 269998844
Beyond analytics: Using computer‐aided methods in educational research to extend qualitative data analysis
- Camilo Vieira , J. Ortega-Alvarez , +1 author Mireille Boutin
- Published in Computer Applications in… 23 May 2024
- Computer Science, Education
24 References
Visual learning analytics of educational data: a systematic literature review and research agenda, student explanations in the context of computational science and engineering education, students' experimentation strategies in design: is process data enough, applying machine learning in science assessment: a systematic review, teaching thematic analysis: overcoming challenges and developing strategies for effective learning, demystifying content analysis, writing in-code comments to self-explain in computational science and engineering education, add‐on preferential groups (apg): analyzing student preferences of teaching methods, beliefs, practices, and reflection: exploring a science teacher’s classroom assessment through the assessment triangle model, the evolution of big data and learning analytics in american higher education, related papers.
Showing 1 through 3 of 0 Related Papers
- +1 (800) 826-0777
- VIRTUAL TOUR
- Mass Notification
- Threat Intelligence
- Employee Safety Monitoring
- Travel Risk Management
- Emergency Preparedness
- Remote Workforce
- Location and Asset Protection
- Critical Communication
- Business Continuity
- Why AlertMedia
- Who We Serve
- Customer Spotlights
- Resource Library
- Downloads & Guides
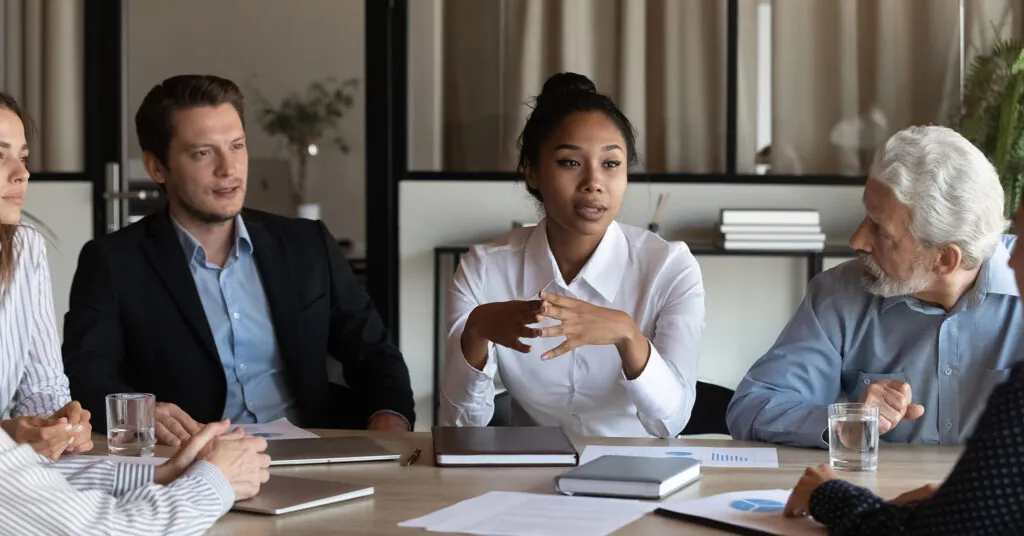
Qualitative Risk Analysis & Other Types of Risk Assessment
Choosing the right risk assessment methodology, whether qualitative, quantitative, or both, is crucial for effectively managing threats.
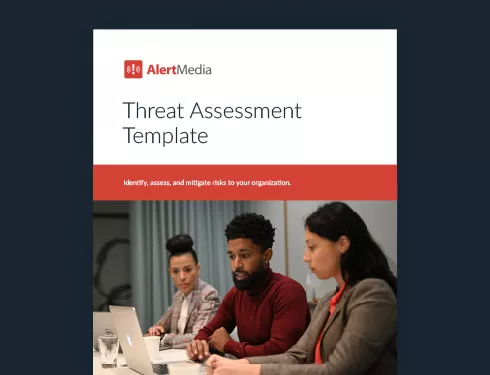
- Risk Identification and Responses
Qualitative Risk Analysis
Quantitative risk evaluation, semi-qualitative risk assessment tools.
“Research the threat and apply data from sources like public records, social media, dark net—so that you can learn as much as possible.” Lukas Quanstrom Co-Founder and CEO, Ontic
When is a threatening letter to a business more than an empty threat? How do you determine when it becomes a genuine risk? Lukas Quanstrom , co-founder and CEO at Ontic, regularly confronts these challenges. In a recent interview on The Employee Safety Podcast , Quanstrom shared how understanding the severity of such threats requires more than surface-level analysis. It requires a detailed, strategic, and proactive approach.
“Once a potential threat has been identified, the next step is to research the threat and apply data from sources like public records, social media, dark net—so that you can learn as much as possible,” Quanstrom said. Research and assessment are critical to understanding how to manage business threats, and there are a few different risk assessment methodologies, each with its strengths and formats.
These strategies range from a qualitative risk analysis that relies on expert judgment and scenario analysis to quantitative methods that use statistical data and mathematical models. Some methodologies even sit squarely in the middle, using descriptions and data to establish a risk management program. Picking the correct method will help you manage threats against your business by providing a structure to understand the likelihood and impact of those threats.
Download Our Threat Assessment Template
Risk identification and risk responses.
When assessing risks, it’s essential to recognize that various types of risks can affect a business. This article will focus on four key categories of risk: strategic, compliance, financial, and operational risk . But it’s important to remember that these are just a few examples of the broad range of risks businesses may encounter.
Risks arising from adverse business decisions, poor implementation, or a lack of responsiveness to industry changes. (e.g., a new competitor enters the market) | Risks related to legal requirements, regulations, or internal policies—which can lead to legal penalties, financial forfeiture, and reputational damage. (e.g., a new privacy law is introduced) | Risks involving potential financial losses due to market fluctuations, credit risk, liquidity issues, and investments. (e.g., interest rates rise on a business loan) | Risks from failures in processes, people, systems, or . (e.g., a cybersecurity incident takes out company networks and infrastructure) |
A practical risk assessment aims to better understand how to manage those risks. These are the four primary risk management responses:
- Avoidance: Eliminating the risk
- Mitigation: Reducing the impact or likelihood of the risk event
- Transfer: Shifting the risk to another party, such as through insurance
- Acceptance: Acknowledging the risk and choosing to deal with its potential consequences
Your chosen risk assessment methodologies will help you understand the threats your business faces and choose from one of the above options for handling them. With thorough analysis, you can make informed decisions that align with your organization’s risk tolerance and strategic goals.
3 Risk Assessment Methodologies
The steps of a risk assessment are relatively straightforward: identify threats, assess those threats, develop controls, and evaluate your response.
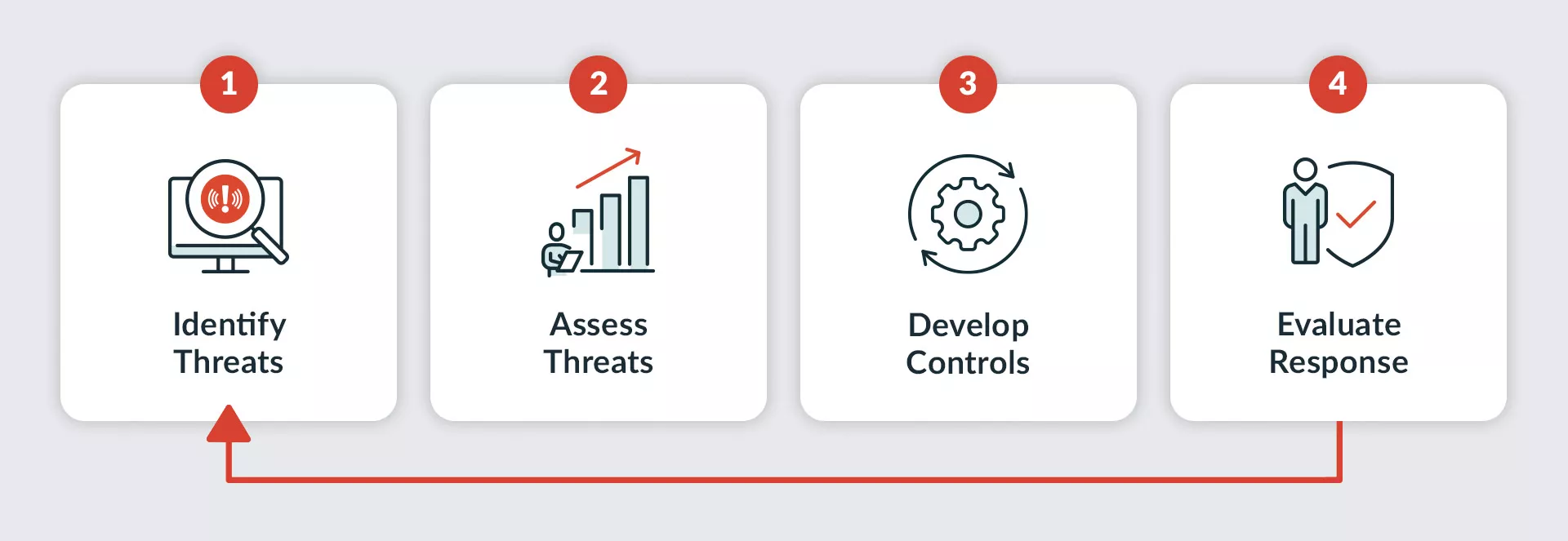
However, these four steps leave much room for interpretation—and error. A risk assessment methodology is a systematic approach underlying all four stages by qualifying or quantifying the threat. That is why most risk assessments use one of these three methodologies:
- Qualitative: Determining risks based on subjective judgment and descriptive measures rather than numerical data or statistics.
- Quantitative: Evaluating risks by estimating specific threats’ probability and potential impact using numerical data and statistical methods.
- Semi-Qualitative: Combining aspects of both qualitative and quantitative analysis.
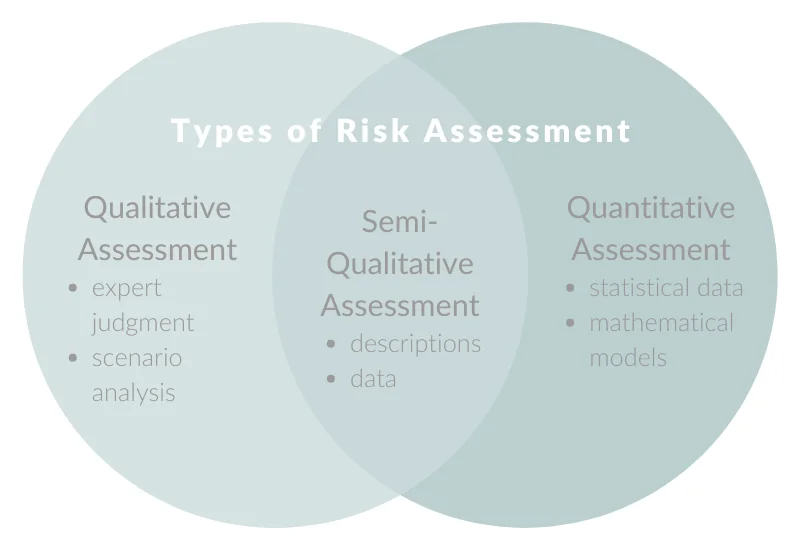
Qualitative vs. quantitative risk analysis, with a blended assessment option
Qualitative risk assessments are a type of risk evaluation that relies on subjective judgment and expert opinions rather than numerical data. This methodology is beneficial when data is unavailable, incomplete, or difficult to quantify. Qualitative risk assessments often involve identifying potential risks through brainstorming sessions, expert interviews, and workshops. These assessments rely on scenarios and descriptive analysis to evaluate the likelihood of occurrence and impact of risks.
One key benefit of qualitative risk assessments is their flexibility. They can be tailored to fit a business’s needs and context, making them highly adaptable to various industries and situations. Unlike quantitative assessment methods, which require detailed data and statistical models, you can perform a qualitative risk analysis with limited information and still consider a broad range of risks.
Another benefit is their ability to incorporate the insights and expertise of individuals who may deeply understand potential risks but lack access to comprehensive data. This subjective approach can provide valuable context and nuance that quantitative methods might overlook.
Numerous customized qualitative risk assessment models are available, with several well-established methodologies, such as those from the International Organization for Standardization (ISO), gaining widespread recognition for their effectiveness. These methodologies offer structured approaches to identifying and analyzing risks based on expert judgment and descriptive analysis.
ISO 27001 is a qualitative strategy typically associated with cybersecurity, but its influence can extend into other domains. It is tailored to establish, implement, maintain, and continuously improve information security management systems (ISMS) to safeguard sensitive information.
This widely recognized qualitative risk assessment methodology is primarily associated with cybersecurity, though you can apply its principles across various domains. This standard provides a structured approach for establishing, implementing, maintaining, and continuously improving an Information Security Management System (ISMS) to protect sensitive information.
An ISO 27001 assessment focuses on three critical areas—people, processes, and technology.
- People: This involves assessing risks related to employees and other individuals with sensitive information access. It includes evaluating the effectiveness of training programs, identifying potential insider threats, and ensuring that roles and responsibilities for information security are clearly defined and communicated.
- Processes: This area focuses on evaluating and improving the procedures and policies to manage information security. It includes reviewing existing security policies, conducting risk assessments, and implementing controls to address identified risks. Regular audits and reviews are part of this process to ensure that procedures remain effective and are updated as needed.
- Technology: This involves assessing the technical controls and systems that protect sensitive data. It includes evaluating the security of IT infrastructure, software, and hardware and then implementing measures such as encryption, access controls, and monitoring systems to prevent and respond to security incidents.
By addressing these three critical areas, ISO 27001 helps organizations systematically identify, mitigate, and manage risks to information security.
Decision trees
A decision tree is a dynamic risk assessment tool that uses a diagrammatic approach to map out possible outcomes and their associated risks for decision-making. Seeing a visual representation of the flow can often help stakeholders understand the potential consequences of each decision branch.
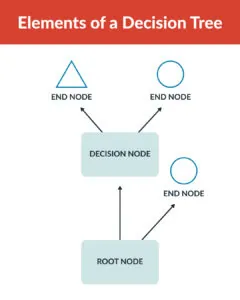
As the diagram above shows, decision trees have three components: the root, the decisions, and the endpoints.
- Root: The root of a decision tree represents the starting point or initial decision that needs to be made.
- Decisions: Decision nodes are points within the decision tree where choices or alternatives are considered. Each decision node branches out into one or more possible outcomes or actions that can be taken based on the decision made at that point.
- Endpoints: These are the outcomes or results of following a specific path through the decision tree. They represent the consequences or outcomes of the decisions made at each preceding node.
You can apply many qualitative methodologies to improve risk assessments, but they’re not always ideal because they lack precision and quantifiable metrics. While qualitative approaches provide valuable insights into subjective factors such as organizational culture and human behavior, they may struggle to deliver measurable and comparable data points for rigorous analysis and decision-making. This is where semi-qualitative risk assessment methodologies become invaluable.
Quantitative risk assessments involve assigning numerical values to potential threats, allowing organizations to evaluate the likelihood of risks, predict their impacts, and estimate potential losses. This data-driven approach provides a precise measurement of risk, which can be invaluable for businesses looking to make informed decisions based on empirical evidence.
A business chooses this risk assessment approach for a few different reasons. Some of the significant benefits of a quantitative risk assessment are:
- Increased accuracy: Quantitative risk assessments provide precise numerical data, which helps businesses measure risk more accurately than qualitative methods that rely on subjective judgment.
- Enhanced objectivity: Quantitative assessments minimize personal biases with statistical data and mathematical models, offering a more objective risk evaluation than qualitative approaches.
- Improved comparability: Quantitative data allows for direct comparison between different risks, which is often more challenging with qualitative assessments that may use descriptive or subjective criteria.
- Better forecasting: Quantitative methods use historical data to predict future risk scenarios, offering more reliable forecasting capabilities than qualitative methods that may not use historical data as effectively.
- Clear communication: Quantitative results provide concrete numbers that can be easier to communicate and justify to stakeholders, as opposed to qualitative descriptions that might be more open to interpretation.
- Benchmarking and performance measurement: Quantitative data enables benchmarking against industry standards and measuring performance over time, which qualitative methods may not directly support.
One standard quantitative risk methodology involves using historical weather data and statistical models to predict the probability of a hurricane occurring in a specific area. Meteorologists might analyze past hurricane patterns, sea surface temperatures, and atmospheric conditions to calculate the likelihood of a hurricane’s landfall in a given region over the next year. They pass this information on to businesses that use quantitative data to manage the risk.
Businesses in vulnerable areas can prioritize risks and management efforts by assigning numerical probabilities to different levels of hurricane risk. For example, a company operating in a region with a high probability of hurricanes might invest in enhanced building infrastructure and emergency response plans. At the same time, a business in a lower-risk area might allocate fewer resources to hurricane preparedness. This data-driven approach allows for targeted risk mitigation strategies based on quantified risk probabilities.
Another example of quantitative risk assessment involves using financial models to predict the effects of changes in interest rates on a company’s bottom line. A company with significant debt might use historical interest rate data and financial modeling techniques to estimate how fluctuations in interest rates could impact its interest expenses and overall profitability.
The company can project potential financial outcomes under different interest rate scenarios by applying quantitative methods, such as sensitivity analysis or scenario modeling. For example, if interest rates are predicted to rise by 1%, the company can estimate the increase in interest payments and its effect on net income. These insights allow the business to make informed decisions about financial strategies, such as refinancing debt or adjusting investment plans, based on numerical projections of potential impacts.
Semi-qualitative risk assessment methodologies combine the precision of quantitative data with the depth of qualitative analysis, offering a thorough and rigorous approach to understanding risks. This method leverages numerical data to provide concrete risk probability and impact estimates while incorporating qualitative insights to capture nuances that numbers alone may not reveal.
Semi-qualitative assessments enable organizations to examine risks from multiple angles by integrating data-driven and judgment-based perspectives. This comprehensive approach ensures that potential threats are evaluated in terms of statistical likelihood while considering contextual implications and expert opinions.
This combined approach results in a more complete risk profile, facilitating better-informed decision-making. Organizations benefit from a detailed understanding of risks, allowing them to develop strategies that address both the measurable and less quantifiable aspects of potential threats.
Risk matrix
A risk matrix is the most straightforward semi-qualitative approach. This tool categorizes direct risks based on their likelihood and potential impact, as depicted below.
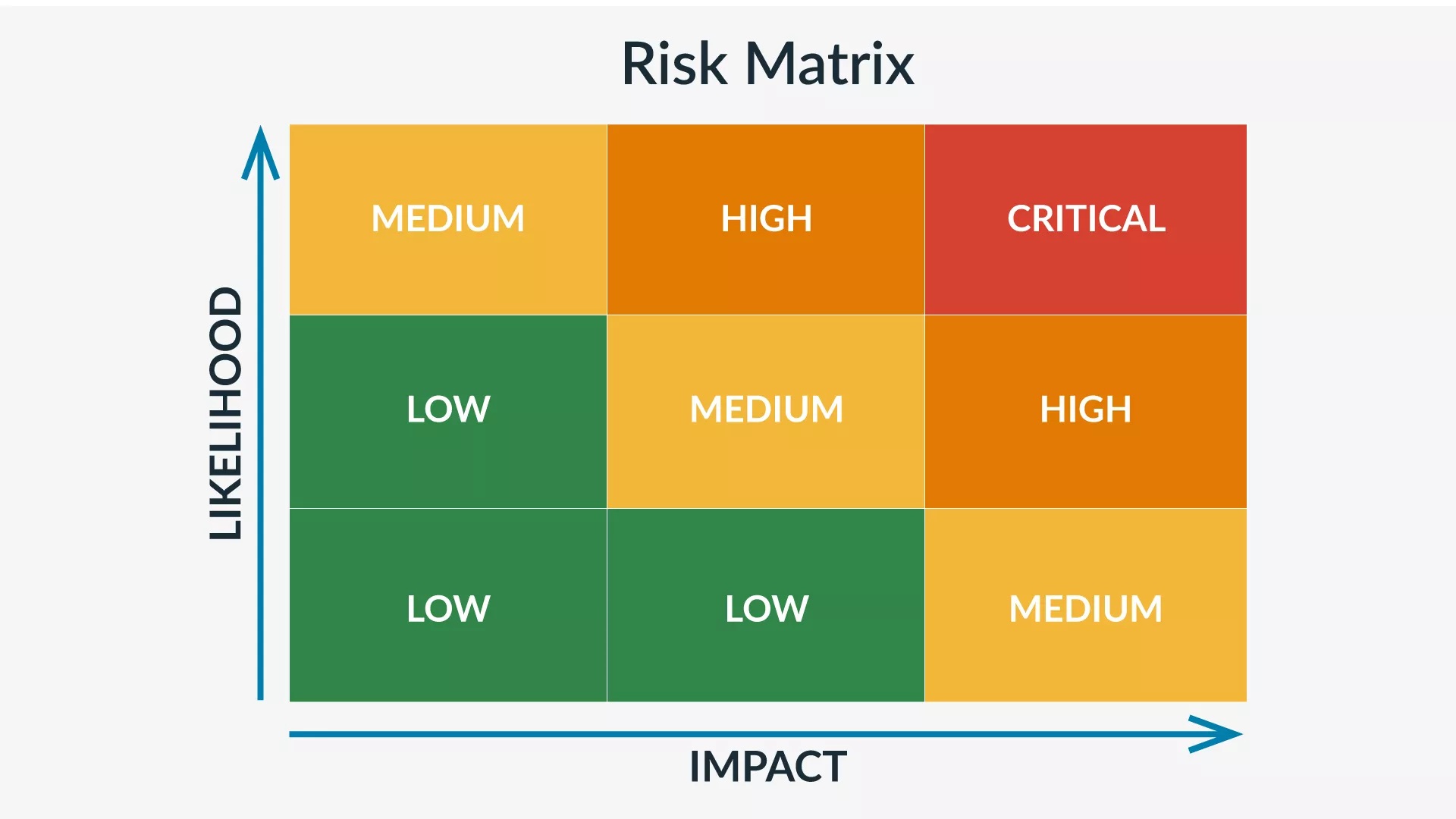
Using a risk matrix enables efficient risk management through hazard identification and categorization. The tool weighs risks based on their likelihood and impact, visually presenting the severity of each risk scenario. Using a risk matrix—especially along with a risk register —organizations can prioritize resources and efforts toward mitigating significant, high risks while effectively communicating the rationale behind risk management decisions to key stakeholders.
Suppose the risk matrix is a straightforward example of a semi-qualitative methodology. In that case, an integrated threat intelligence system represents the opposite end of the spectrum with more detailed analysis.
Integrated threat intelligence system
Threat intelligence systems gather and analyze comprehensive information about potential hazards, aiding organizations in proactive risk mitigation and response strategies. They can also be critical tools for assessing and understanding your specific risks. In his interview, Lukas Quanstrom also highlighted the evolving role of threat intelligence in proactive corporate security risk assessment. Quanstrom emphasized that traditional reactive threat management is giving way to a more proactive approach enabled by what he terms “protective intelligence.”
Protective intelligence integrates investigative (qualitative) techniques with advanced analytics (quantitative) to detect and assess potential threats early on. These indicators, such as threatening communications or unusual patterns in employee behaviors, serve as early warning signals that enable proactive risk mitigation strategies . Using data to define a threat’s likelihood and impact allows organizations to prioritize strategies.
Quanstrom explained, “By adopting a proactive, always-on security approach, you can continually collect and connect pre-incident physical threat indicators, providing the critical knowledge needed to prevent bad things from happening.” This proactive stance safeguards assets and personnel, supports business continuity, and enhances stakeholder confidence in the organization’s resilience to emerging threats.
Combining Risk Analysis Processes
Qualitative, quantitative, and semi-qualitative risk assessment methodologies have strengths and limitations. Integrating all three of these approaches offers you a more comprehensive risk assessment so you better understand your potential threats and vulnerabilities. With that situational awareness, you can make informed decisions and prioritize resources where they are most needed.
By combining qualitative insights with quantitative data and semi-qualitative assessments, businesses can establish a dynamic risk management plan that adapts to identified risks and enhances overall resilience.
More Articles You May Be Interested In
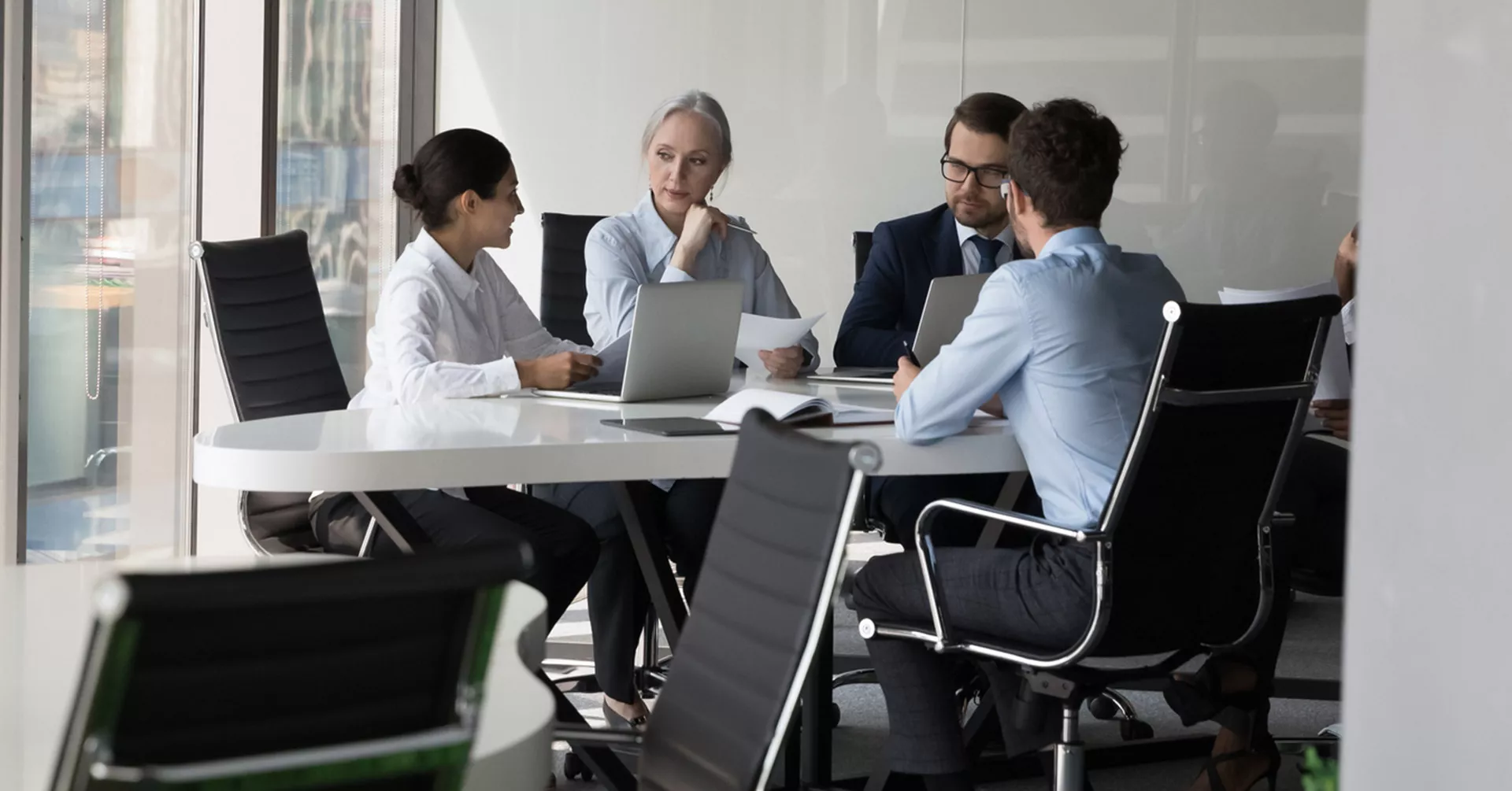
Threat Assessment Template
Please complete the form below to receive this resource.
Check Your Inbox!
The document you requested has been sent to your provided email address.
Cookies are required to play this video.
Click the blue shield icon on the bottom left of your screen to edit your cookie preferences.
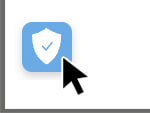

An official website of the United States government
The .gov means it’s official. Federal government websites often end in .gov or .mil. Before sharing sensitive information, make sure you’re on a federal government site.
The site is secure. The https:// ensures that you are connecting to the official website and that any information you provide is encrypted and transmitted securely.
- Publications
- Account settings
Preview improvements coming to the PMC website in October 2024. Learn More or Try it out now .
- Advanced Search
- Journal List
- Malays Fam Physician
- v.3(1); 2008
Data Analysis in Qualitative Research: A Brief Guide to Using Nvivo
MSc, PhD, Faculty of Medicine, University of Malaya, Kuala Lumpur, Malaysia
Qualitative data is often subjective, rich, and consists of in-depth information normally presented in the form of words. Analysing qualitative data entails reading a large amount of transcripts looking for similarities or differences, and subsequently finding themes and developing categories. Traditionally, researchers ‘cut and paste’ and use coloured pens to categorise data. Recently, the use of software specifically designed for qualitative data management greatly reduces technical sophistication and eases the laborious task, thus making the process relatively easier. A number of computer software packages has been developed to mechanise this ‘coding’ process as well as to search and retrieve data. This paper illustrates the ways in which NVivo can be used in the qualitative data analysis process. The basic features and primary tools of NVivo which assist qualitative researchers in managing and analysing their data are described.
QUALITATIVE RESEARCH IN MEDICINE
Qualitative research has seen an increased popularity in the last two decades and is becoming widely accepted across a wide range of medical and health disciplines, including health services research, health technology assessment, nursing, and allied health. 1 There has also been a corresponding rise in the reporting of qualitative research studies in medical and health related journals. 2
The increasing popularity of qualitative methods is a result of failure of quantitative methods to provide insight into in-depth information about the attitudes, beliefs, motives, or behaviours of people, for example in understanding the emotions, perceptions and actions of people who suffer from a medical condition. Qualitative methods explore the perspective and meaning of experiences, seek insight and identify the social structures or processes that explain people”s behavioural meaning. 1 , 3 Most importantly, qualitative research relies on extensive interaction with the people being studied, and often allows researchers to uncover unexpected or unanticipated information, which is not possible in the quantitative methods. In medical research, it is particularly useful, for example, in a health behaviour study whereby health or education policies can be effectively developed if reasons for behaviours are clearly understood when observed or investigated using qualitative methods. 4
ANALYSING QUALITATIVE DATA
Qualitative research yields mainly unstructured text-based data. These textual data could be interview transcripts, observation notes, diary entries, or medical and nursing records. In some cases, qualitative data can also include pictorial display, audio or video clips (e.g. audio and visual recordings of patients, radiology film, and surgery videos), or other multimedia materials. Data analysis is the part of qualitative research that most distinctively differentiates from quantitative research methods. It is not a technical exercise as in quantitative methods, but more of a dynamic, intuitive and creative process of inductive reasoning, thinking and theorising. 5 In contrast to quantitative research, which uses statistical methods, qualitative research focuses on the exploration of values, meanings, beliefs, thoughts, experiences, and feelings characteristic of the phenomenon under investigation. 6
Data analysis in qualitative research is defined as the process of systematically searching and arranging the interview transcripts, observation notes, or other non-textual materials that the researcher accumulates to increase the understanding of the phenomenon. 7 The process of analysing qualitative data predominantly involves coding or categorising the data. Basically it involves making sense of huge amounts of data by reducing the volume of raw information, followed by identifying significant patterns, and finally drawing meaning from data and subsequently building a logical chain of evidence. 8
Coding or categorising the data is the most important stage in the qualitative data analysis process. Coding and data analysis are not synonymous, though coding is a crucial aspect of the qualitative data analysis process. Coding merely involves subdividing the huge amount of raw information or data, and subsequently assigning them into categories. 9 In simple terms, codes are tags or labels for allocating identified themes or topics from the data compiled in the study. Traditionally, coding was done manually, with the use of coloured pens to categorise data, and subsequently cutting and sorting the data. Given the advancement of software technology, electronic methods of coding data are increasingly used by qualitative researchers.
Nevertheless, the computer does not do the analysis for the researchers. Users still have to create the categories, code, decide what to collate, identify the patterns and draw meaning from the data. The use of computer software in qualitative data analysis is limited due to the nature of qualitative research itself in terms of the complexity of its unstructured data, the richness of the data and the way in which findings and theories emerge from the data. 10 The programme merely takes over the marking, cutting, and sorting tasks that qualitative researchers used to do with a pair of scissors, paper and note cards. It helps to maximise efficiency and speed up the process of grouping data according to categories and retrieving coded themes. Ultimately, the researcher still has to synthesise the data and interpret the meanings that were extracted from the data. Therefore, the use of computers in qualitative analysis merely made organisation, reduction and storage of data more efficient and manageable. The qualitative data analysis process is illustrated in Figure 1 .

Qualitative data analysis flowchart
USING NVIVO IN QUALITATIVE DATA ANALYSIS
NVivo is one of the computer-assisted qualitative data analysis softwares (CAQDAS) developed by QSR International (Melbourne, Australia), the world’s largest qualitative research software developer. This software allows for qualitative inquiry beyond coding, sorting and retrieval of data. It was also designed to integrate coding with qualitative linking, shaping and modelling. The following sections discuss the fundamentals of the NVivo software (version 2.0) and illustrates the primary tools in NVivo which assist qualitative researchers in managing their data.
Key features of NVivo
To work with NVivo, first and foremost, the researcher has to create a Project to hold the data or study information. Once a project is created, the Project pad appears ( Figure 2 ). The project pad of NVivo has two main menus: Document browser and Node browser . In any project in NVivo, the researcher can create and explore documents and nodes, when the data is browsed, linked and coded. Both document and node browsers have an Attribute feature, which helps researchers to refer the characteristics of the data such as age, gender, marital status, ethnicity, etc.

Project pad with documents tab selected
The document browser is the main work space for coding documents ( Figure 3 ). Documents in NVivo can be created inside the NVivo project or imported from MS Word or WordPad in a rich text (.rtf) format into the project. It can also be imported as a plain text file (.txt) from any word processor. Transcripts of interview data and observation notes are examples of documents that can be saved as individual documents in NVivo. In the document browser all the documents can be viewed in a database with short descriptions of each document.

Document browser with coder and coding stripe activated
NVivo is also designed to allow the researcher to place a Hyperlink to other files (for example audio, video and image files, web pages, etc.) in the documents to capture conceptual links which are observed during the analysis. The readers can click on it and be taken to another part of the same document, or a separate file. A hyperlink is very much like a footnote.
The second menu is Node explorer ( Figure 4 ), which represents categories throughout the data. The codes are saved within the NVivo database as nodes. Nodes created in NVivo are equivalent to sticky notes that the researcher places on the document to indicate that a particular passage belongs to a certain theme or topic. Unlike sticky notes, the nodes in NVivo are retrievable, easily organised, and give flexibility to the researcher to either create, delete, alter or merge at any stage. There are two most common types of node: tree nodes (codes that are organised in a hierarchical structure) and free nodes (free standing and not associated with a structured framework of themes or concepts). Once the coding process is complete, the researcher can browse the nodes. To view all the quotes on a particular Node, select the particular node on the Node Explorer and click the Browse button ( Figure 5 ).

Node explorer with a tree node highlighted

Browsing a node
Coding in NVivo using Coder
Coding is done in the document browser. Coding involves the desegregation of textual data into segments, examining the data similarities and differences, and grouping together conceptually similar data in the respective nodes. 11 The organised list of nodes will appear with a click on the Coder button at the bottom of document browser window.
To code a segment of the text in a project document under a particular node, highlight the particular segment and drag the highlighted text to the desired node in the coder window ( Figure 3 ). The segments that have been coded to a particular node are highlighted in colours and nodes that have attached to a document turns bold. Multiple codes can be assigned to the same segment of text using the same process. Coding Stripes can be activated to view the quotes that are associated with the particular nodes. With the guide of highlighted text and coding stripes, the researcher can return to the data to do further coding or refine the coding.
Coding can be done with pre-constructed coding schemes where the nodes are first created using the Node explorer followed by coding using the coder. Alternatively, a bottom-up approach can be used where the researcher reads the documents and creates nodes when themes arise from the data as he or she codes.
Making and using memos
In analysing qualitative data, pieces of reflective thinking, ideas, theories, and concepts often emerge as the researcher reads through the data. NVivo allows the user the flexibility to record ideas about the research as they emerge in the Memos . Memos can be seen as add-on documents, treated as full status data and coded like any other documents. 12 Memos can be placed in a document or at a node. A memo itself can have memos (e.g. documents or nodes) linked to it, using DocLinks and NodeLinks .
Creating attributes
Attributes are characteristics (e.g. age, marital status, ethnicity, educational level, etc.) that the researcher associates with a document or node. Attributes have different values (for example, the values of the attribute for ethnicity are ‘Malay’, ‘Chinese’ and ‘Indian’). NVivo makes it possible to assign attributes to either document or node. Items in attributes can be added, removed or rearranged to help the researcher in making comparisons. Attributes are also integrated with the searching process; for example, linking the attributes to documents will enable the researcher to conduct searches pertaining to documents with specified characteristics ( Figure 6 ).

Document attribute explorer
Search operation
The three most useful types of searches in NVivo are Single item (text, node, or attribute value), Boolean and Proximity searches. Single item search is particularly important, for example, if researchers want to ensure that every mention of the word ‘cure’ has been coded under the ‘Curability of cervical cancer’ tree node. Every paragraph in which this word is used can be viewed. The results of the search can also be compiled into a single document in the node browser and by viewing the coding stripe. The researcher can check whether each of the resulting passages has been coded under a particular node. This is particularly useful for the researcher to further determine whether conducting further coding is necessary.
Boolean searches combine codes using the logical terms like ‘and’, ‘or’ and ‘not’. Common Boolean searches are ‘or’ (also referred to as ‘combination’ or ‘union’) and ‘and’ (also called ‘intersection’). For example, the researcher may wish to search for a node and an attributed value, such as ‘ever screened for cervical cancer’ and ‘primary educated’. Search results can be displayed in matrix form and it is possible for the researcher to perform quantitative interpretations or simple counts to provide useful summaries of some aspects of the analysis. 13 Proximity searches are used to find places where two items (e.g. text patterns, attribute values, nodes) appear near each other in the text.
Using models to show relationships
Models or visualisations are an essential way to describe and explore relationships in qualitative research. NVivo provides a Modeler designated for visual exploration and explanation of relationships between various nodes and documents. In Model Explorer, the researcher can create, label and connect ideas or concepts. NVivo allows the user to create a model over time and have any number of layers to track the progress of theory development to enable the researcher to examine the stages in the model-building over time ( Figure 7 ). Any documents, nodes or attributes can be placed in a model and clicking on the item will enable the researcher to inspect its properties.

Model explorer showing the perceived risk factors of cervical cancer
NVivo has clear advantages and can greatly enhance research quality as outlined above. It can ease the laborious task of data analysis which would otherwise be performed manually. The software certainly removes the tremendous amount of manual tasks and allows more time for the researcher to explore trends, identify themes, and make conclusions. Ultimately, analysis of qualitative data is now more systematic and much easier. In addition, NVivo is ideal for researchers working in a team as the software has a Merge tool that enables researchers that work in separate teams to bring their work together into one project.
The NVivo software has been revolutionised and enhanced recently. The newly released NVivo 7 (released March 2006) and NVivo 8 (released March 2008) are even more sophisticated, flexible, and enable more fluid analysis. These new softwares come with a more user-friendly interface that resembles the Microsoft Windows XP applications. Furthermore, they have new data handling capacities such as to enable tables or images embedded in rich text files to be imported and coded as well. In addition, the user can also import and work on rich text files in character based languages such as Chinese or Arabic.
To sum up, qualitative research undoubtedly has been advanced greatly by the development of CAQDAS. The use of qualitative methods in medical and health care research is postulated to grow exponentially in years to come with the further development of CAQDAS.
More information about the NVivo software
Detailed information about NVivo’s functionality is available at http://www.qsrinternational.com . The website also carries information about the latest versions of NVivo. Free demonstrations and tutorials are available for download.
ACKNOWLEDGEMENT
The examples in this paper were adapted from the data of the study funded by the Ministry of Science, Technology and Environment, Malaysia under the Intensification of Research in Priority Areas (IRPA) 06-02-1032 PR0024/09-06.
TERMINOLOGY
Attributes : An attribute is a property of a node, case or document. It is equivalent to a variable in quantitative analysis. An attribute (e.g. ethnicity) may have several values (e.g. Malay, Chinese, Indian, etc.). Any particular node, case or document may be assigned one value for each attribute. Similarities within or differences between groups can be identified using attributes. Attribute Explorer displays a table of all attributes assigned to a document, node or set.
CAQDAS : Computer Aided Qualitative Data Analysis. The CAQDAS programme assists data management and supports coding processes. The software does not really analyse data, but rather supports the qualitative analysis process. NVivo is one of the CAQDAS programmes; others include NUDIST, ATLAS-ti, AQUAD, ETHNOGRAPH and MAXQDA.
Code : A term that represents an idea, theme, theory, dimension, characteristic, etc., of the data.
Coder : A tool used to code a passage of text in a document under a particular node. The coder can be accessed from the Document or Node Browser .
Coding : The action of identifying a passage of text in a document that exemplifies ideas or concepts and connecting it to a node that represents that idea or concept. Multiple codes can be assigned to the same segment of text in a document.
Coding stripes : Coloured vertical lines displayed at the right-hand pane of a Document ; each is named with title of the node at which the text is coded.
DataLinks : A tool for linking the information in a document or node to the information outside the project, or between project documents. DocLinks , NodeLinks and DataBite Links are all forms of DataLink .
Document : A document in an NVivo project is an editable rich text or plain text file. It may be a transcription of project data or it may be a summary of such data or memos, notes or passages written by the researcher. The text in a document can be coded, may be given values of document attributes and may be linked (via DataLinks ) to other related documents, annotations, or external computer files. The Document Explorer shows the list of all project documents.
Memo : A document containing the researcher”s commentary flagged (linked) on any text in a Document or Node. Any files (text, audio or video, or picture data) can be linked via MemoLink .
Model : NVivo models are made up of symbols, usually representing items in the project, which are joined by lines or arrows, designed to represent the relationship between key elements in a field of study. Models are constructed in the Modeller .
Node : Relevant passages in the project”s documents are coded at nodes. A Node represents a code, theme, or idea about the data in a project. Nodes can be kept as Free Nodes (without organisation) or may be organised hierarchically in Trees (of categories and subcategories). Free nodes are free-standing and are not associated to themes or concepts. Early on in the project, tentative ideas may be stored in the Free Nodes area. Free nodes can be kept in a simple list and can be moved to a logical place in the Tree Node when higher levels of categories are discovered. Nodes can be given values of attributes according to the features of what they represent, and can be grouped in sets. Nodes can be organised (created, edited) in Node Explorer (a window listing all the project nodes and node sets). The Node Browser displays the node”s coding and allow the researcher to change the coding.
Project : Collection of all the files, documents, codes, nodes, attributes, etc. associated with a research project. The Project pad is a window in NVivo when a project is open which gives access to all the main functions of the programme.
Sets : Sets in NVivo hold shortcuts to any nodes or documents, as a way of holding those items together without actually combining them. Sets are used primarily as a way of indicating items that in some way are related conceptually or theoretically. It provides different ways of sorting and managing data.
Tree Node : Nodes organised hierarchically into trees to catalogue categories and subcategories.
This paper is in the following e-collection/theme issue:
Published on 7.8.2024 in Vol 26 (2024)
Ethical, Legal, and Practical Concerns Surrounding the Implemention of New Forms of Consent for Health Data Research: Qualitative Interview Study
Authors of this article:

Original Paper
- Svenja Wiertz * , DPhil ;
- Joachim Boldt * , PhD, PD
Department of Medical Ethics and the History of Medicine, University of Freiburg, Freiburg, Germany
*all authors contributed equally
Corresponding Author:
Svenja Wiertz, DPhil
Department of Medical Ethics and the History of Medicine
University of Freiburg
Stefan-Meier-Str. 26
Freiburg, 79104
Phone: 49 761 203 5044
Fax:49 761 203 5039
Email: [email protected]
Background: In Europe, within the scope of the General Data Protection Regulation, more and more digital infrastructures are created to allow for large-scale access to patients’ health data and their use for research. When the research is performed on the basis of patient consent, traditional study-specific consent appears too cumbersome for many researchers. Alternative models of consent are currently being discussed and introduced in different contexts.
Objective: This study explores stakeholder perspectives on ethical, legal, and practical concerns regarding models of consent for health data research at German university medical centers.
Methods: Semistructured focus group interviews were conducted with medical researchers at German university medical centers, health IT specialists, data protection officers, and patient representatives. The interviews were analyzed using a software-supported structuring qualitative content analysis.
Results: Stakeholders regarded broad consent to be only marginally less laborious to implement and manage than tiered consent. Patient representatives favored specific consent, with tiered consent as a possible alternative. All stakeholders lamented that information material was difficult to understand. Oral information and videos were mentioned as a means of improvement. Patient representatives doubted that researchers had a sufficient degree of data security expertise to act as sole information providers. They were afraid of undue pressure if obtaining health data research consent were part of medical appointments. IT specialists and other stakeholders regarded the withdrawal of consent to be a major challenge and called for digital consent management solutions. On the one hand, the transfer of health data to non-European countries and for-profit organizations is seen as a necessity for research. On the other hand, there are data security concerns with regard to these actors. Research without consent is legally possible under certain conditions but deemed problematic by all stakeholder groups, albeit for differing reasons and to different degrees.
Conclusions: More efforts should be made to determine which options of choice should be included in health data research consent. Digital tools could improve patient information and facilitate consent management. A unified and strict regulation for research without consent is required at the national and European Union level. Obtaining consent for health data research should be independent of medical appointments, and additional personnel should be trained in data security to provide information on health data research.
Introduction
In the European Union (EU), the outlines of a European health data space are currently being developed and discussed. It aims to facilitate accessing health data for research purposes, among other features [ 1 ]. In some countries, such as Estonia and Finland, fully digitalized health data infrastructures that also allow for secondary use of health data for research have already been implemented. In other countries, among them Germany, developments are ongoing [ 2 - 7 ]. In Germany, a central example of such developments is the government’s medical informatics initiative (MII; Medizininformatik-Initiative ) [ 8 ]. Its purpose is to create data integration centers at German university medical centers and to establish centralized access to the data.
In regard to these infrastructures, it is an important question from ethical, legal, and social perspectives whether and how patients are informed about the use of their data and how they consent to this use [ 9 - 11 ]. Informed consent consists of 2 elements, as the term indicates: information ensures that patients know what their health data are used for. From an ethical point of view, information can be regarded as a demand for the principle of transparency. By contrast, consent safeguards the ability to control what one’s data are used for and serves to retain privacy. Together, transparency and control allow autonomy rights to be exercised meaningfully.
Various models of informed consent could be used for health data research. Specific consent , the standard in research involving humans, demands that trial participants are informed about and consent to the specific aims and methods of the trial that they take part in. Applied to health data research, this would mean that patients are informed about and consent to each health data research project that accesses and uses the patient’s data. Since this would place major obstacles in the way of research, among other drawbacks (compare in the Previous Research section), a number of alternatives are discussed, such as broad consent, tiered consent, meta-consent, and dynamic consent.
In broad consent patients consent to the use of their health data for research purposes in unspecified future research. The model of tiered consent enables patients to tailor individual consent profiles through the selection of broad and narrow options for data use ( tiers ) and thus allows them to adjust their preferences regarding the kinds of future research to which they are willing to consent. The dynamic consent framework facilitates study-specific consent by implementing consent requests and information on a web-based platform. Similarly, meta-consent implements tiered consent on a web-based platform, including options to ask for study-specific consent or to opt for broad consent [ 12 - 15 ].
The General Data Protection Regulation (GDPR), which was introduced in the EU in 2018, constitutes an important part of existing governance frameworks. Although it contributes to harmonizing legal approaches to data sharing in member states, it does not prescribe a specific model of consent when consent is used as a basis to legally justify the use of health data for research. In fact, under certain conditions, the GDPR permits accessing health data for research without consent [ 16 , 17 ]. Article 9, paragraph 2, of the GDPR allows EU member states to issue such exemptions for scientific research. The prerequisite is that the processing of personal health data is necessary for the purpose of the research and that safeguards are in place to protect the rights of the “data subjects” in accordance with Article 89, paragraph 1. In addition, the processing must be proportionate to the objective pursued. In Germany, section 27 of the German Federal Data Protection Act constitutes such a national regulation.
The World Medical Association’s Declaration of Taipei introduces consent as a necessary condition for using health data in research involving humans with few exemptions and lists information that must be included in informed consent for data sharing and biobanking [ 18 ]. These stipulations allow for but do not require specific consent for data stored in repositories that are designed for “multiple and indefinite uses.” They are compatible with other models of consent as well.
The German MII continues to consider consent as the appropriate legal basis for authorizing the secondary use of health data for research purposes, as is standard in many other EU countries [ 3 , 8 ]. More specifically, the initiative opts for a standardized model of broad consent. All participating medical centers are asked to use the same information and consent form, to which optional modules (eg, concerning biobanking) can be added according to local needs. These developments raise the question of how informed consent should be implemented in health data research infrastructure and which model of consent is to be favored. Moreover, the first experiences of stakeholders in this implementation process can provide valuable insight and information on the challenges connected to operationalizing informed consent in the context of health data research.
Previous Research
Informed consent safeguards transparency and enables patients to exert control over the use of their personal health data and retain privacy. In the ethical debate on health data research, informed consent thus plays an important role. In addition, in the GDPR, obtaining consent is the standard way to legally justify the use of personal health data for research, although consent can be waived if member states fulfill certain conditions (compare in the Background section).
In the current scholarly ethical debate, there is wide agreement that specific informed consent, an ethical cornerstone of clinical research, has significant drawbacks when applied to biobank and health data research [ 12 ]. For a number of reasons, staying with specific consent in the case of health data research appears problematic. Since valuable data research will often involve data from a large number of patients, obtaining consent will be cumbersome and delay or prevent studies [ 12 ]. This may impede studies and diminish the positive impact that these studies could have had on health and society. Implementing specific consent on a digital platform may mitigate these problems, but it will still constitute an obstacle to large-scale data research. In addition, patients themselves might be irritated by being asked to consent to data research for single studies if approached repeatedly for a large number of studies [ 19 , 20 ]. As some surveys show, many patients and citizens generally deem the use of their health data for research acceptable. These surveys also show that while patients and citizens might prefer traditional specific consent, they deem other forms of consent acceptable [ 21 - 24 ]. Finally, although there are serious risks connected to the use of health data, such as loss of data and misuse, these risks are different and arguably less severe than the risks of clinical research [ 12 ].
Accordingly, in the conceptual ethical debate about alternative consent models for data- or biospecimen-based research, broad consent to unspecified future research is suggested as an alternative to specific consent [ 12 , 15 ]. The main issues highlighted as problematic in this debate so far regard the problem of consenting to research that is as of yet unknown. The information conveyed at the time of obtaining consent is necessarily restricted to general information on risks and opportunities of accessing and storing health data, pseudonymization, and anonymization procedures, data security principles, and features of typical health data research projects. By contrast, no information can be supplied concerning specific research projects, their goals and methods, and their risks. Since the validity of consent is based on the knowledge of what one consents to and since broad consent information is necessarily scarce, the validity of this consent is called into question [ 14 , 25 , 26 ]. Indeed, the provocative question of whether broad consent can be counted as informed consent at all has been raised [ 27 ].
Various other proposals have been made as to how the goal of relieving researchers and research participants of the need for frequent and time-consuming information and consent processes can be achieved while at the same time maintaining a high standard of informational self-determination [ 13 ]. Making use of digital infrastructure for collecting and managing consent has been a prominent suggestion in this debate. The dynamic consent framework has been discussed as a possible way forward that could allow for study-specific consent through a web-based platform, which would facilitate the process of obtaining consent. The suggested framework of meta-consent combines this idea with the principle of tiered consent, in which patients are enabled to tailor their individual consent profiles through the selection of broad and narrow options for data use (tiers), and allowed to adjust their preferences flexibly from home through a web-based platform [ 28 - 32 ] (for our interviews, we have separated the issues of consent models from their digital or analog implementation. We are thus not discussing meta-consent and dynamic consent by name but are referring to the same concepts when we discuss options of a digital implementation of specific or tiered consent).
With a focus on Europe, it has been discussed how GDPR-based rights can be safeguarded and implemented as part of biobank consent management procedures. These rights include the right to be informed about the collection and use of personal data, the right to rectification of wrong personal data, and the right to erasure of personal data [ 33 ]. Since research on specimens always also involves the use of health data, the GDPR is a relevant legal framework for this kind of research as well as for research solely on health data. The findings of this debate thus are generally relevant for health data research as well. Nevertheless, the GDPR biobank debate does not touch upon possible alternative forms of consent. In biobank research today, broad consent is the standard and widely accepted model of consent [ 15 ].
Attitudes toward data sharing for health research have been studied in the United States as well as in Europe, and some meta-studies have attempted to summarize the results [ 22 , 34 - 37 ]. They not only report widespread support for data sharing for research purposes under certain conditions (eg, data security, trust, control, and privacy) but also highlight ethnocultural differences in attitude [ 38 ]. Concerns identified relate to sharing of data (or specimens) with for-profit organizations [ 22 ] as well as transferring data across borders, especially from EU countries to non-EU countries [ 37 ].
For Germany in particular, acceptance of broad consent has been studied among outpatients of a university hospital in northern Germany [ 23 ] as well as among participants of a cancer registry in the state of Baden-Württemberg [ 24 ]. Both studies observe differing levels of acceptance of broad consent (58.9% vs 86.9%, respectively). In a qualitative study from Germany on the perception of risks and opportunities of health data research in general, stakeholder groups from health research, health care, medical informatics, patient advocacy groups, and politics were interviewed. Regarding informed consent, the study concludes that some stakeholders are afraid that patients may be unduly influenced to provide consent if they are, as patients, under distress or wrongly assume that health data research will directly benefit them [ 39 ]. The study does not differentiate between models of consent. Overall, although, on a conceptual level, the pros and cons of models of consent regarding health data research have been debated, there are no studies empirically examining the acceptance and perceptions of advantages, disadvantages, and implementation challenges of different models of consent from stakeholder perspectives.
In our study, we build on and complement the aforementioned studies by focusing on different models of consent and consent procedures. Different models of consent for health data research have been developed on the basis of conceptual and normative arguments. So far, empirical studies and surveys have not taken up these models and compared their acceptance and perception of drawbacks and opportunities from the perspective of stakeholder groups. We aimed to explore the perception of drawbacks and opportunities of different models of consent among stakeholders from patient associations, data protection, medical informatics, and medical research.
The study is explorative. We assume that the perspectives of stakeholders who are dealing with health data research and informed consent in different roles and with different tasks can help to uncover blind spots in the debate and challenges in practical application that have not received adequate attention in the conceptual, ethical debate on consent models for health data research so far.
We interviewed stakeholders who are and will be affected by the implementation of informed consent regarding health data research and can be assumed to have a perspective on the drawbacks and opportunities of consent models. Medical researchers will use the relevant infrastructures and data and will have to abide by consent provisions. Patients are the ones whose individual rights and interests are meant to be protected by consent. Data protection officers safeguard that data handling procedures, including consent procedures, conform to the law. Health IT specialists implement and run the IT infrastructure for storing and managing health data and research requests, as well as consent specifications. We included 3 to 4 participants from each stakeholder group, 13 participants in total.
For the profession-based groups, we focused on stakeholders with ties to German university medical centers. These centers are currently implementing broad consent as devised by the MII. Their employees can thus be assumed to have a level of experiential and expert knowledge that enables them to take a stance on models of consent and their implementation.
Representatives of these groups were identified via a web-based search and contacted by publicly available email addresses. The selection criteria were to include participants who are familiar with the topic of informed consent (documented statements, publications, participation in relevant conferences or workshops, etc) and to ensure diversity of individuals and perspectives within the groups (geographical location, specific area of expertise, and gender). Our group of medical researchers comprised 2 physician researchers and 1 epidemiologist.
To recruit patient representatives familiar with the topics of consent and research, as well as patients’ attitudes beyond their own, we checked and contacted several established German patient associations and invited individuals, on the basis of either publicly available records of experience or recommendations by board members of the associations.
Ethical Considerations
The study was approved by the ethics commission of the University of Freiburg (approval number 21-1701). It was conducted in accordance with applicable German and EU data protection regulations. Participation was voluntary and not compensated. All interviewees were informed about the aims, methods, benefits, and risks of participating in the study and gave documented informed consent. Any information allowing personal identification was removed from the interview transcripts before analysis. All data in the publication, as well as any data shared on request, were anonymized.
We conducted qualitative, semistructured interviews with the respective stakeholders. We opted for group interviews in order to profit from the effect of homogeneous focus groups to illuminate the topic in breadth and depth [ 40 ].
Of all the persons interviewed (N=13), the patient representatives (n=3, 23%), the medical researchers (n=3, 23%), and the health IT specialists (n=4, 31%) were interviewed in groups as planned. For the data protection officers (n=3, 23%), 3 individual interviews had to be conducted due to organizational reasons.
The interviews took place between May and December 2022 in a videoconference format. All interviews were jointly conducted by both authors. The interview guide consisted of a series of general questions and some group-specific questions. One interview session lasted between 60 and 150 minutes. The interviews were recorded and subsequently transcribed by an external service provider.
The interview guide was prefaced by a short explanation of broad consent, tiered consent, specific consent, and digital consent platforms ( Multimedia Appendix 1 ). The guide included 8 questions that were addressed to all groups equally, plus 4 to 8 group-specific questions. The explanatory text and the questions were sent to the participants by email several days before the interview.
Data Analysis
The transcriptions were analyzed in an iterative process using qualitative content analysis. We applied the structuring qualitative content analysis as developed by Mayring [ 41 ] and Kuckartz [ 42 ]. The software MAXQDA (version 22.61; VERBI Software GmbH, 2022) was used for coding. Given the small number of participants in each group, no quantitative analysis was carried out. No interrater reliability or percent level of agreement were calculated.
The coding categories of the material were initially systematized according to action steps in the process of devising and carrying out a data research project: from planning the research to obtaining consent, adapting and withdrawing consent, to handling of consent data and treatment data (in research contexts), to handling of research results, and to addressing data loss and misuse. The category scheme according to which the material was sorted was developed before the analysis and applied deductively. Where necessary, it was later adapted inductively based on the content of the material. The identification of topics, problem areas, and proposed solutions was carried out inductively using the sorted material.
All items were annotated with a 1-sentence summary. On the basis of these summaries, a table was created to grant an overview of the topics of the interview ( Multimedia Appendices 2 and 3 ). For this purpose, suggestions for solutions as well as objections in regard to either the problem itself or a suggested solution were grouped with the problems they addressed. In some cases, solutions were offered to implied problems without the problems themselves being named. The implied problems were added to the table where needed. This sorting was conducted by SW, and JB reviewed all resulting categories. Any disagreements regarding either the placement of a particular statement in a category or the usefulness or exact borders of the categories were discussed between the 2 researchers until a consensus was reached.
Our interviewees brought up a large number of problems, concerns, and possible solutions regarding models of consent and their implementation. A complete overview of the results can be found in Table S1 in Multimedia Appendix 2 and Table S1 in Multimedia Appendix 3 .
The following representation of results highlights topics that either are addressed repeatedly by different stakeholder groups or have not received much attention in the existing ethical debate on models of consent and can thus be considered new and potentially worthy of further consideration.
Attitudes on Specific and Broad Consent
When discussing models of consent for health data research, our interviewees first focused on broad consent and specific consent. The expressed points of view coincide in many respects with the current state of the scholarly debate on this topic. For example, patient representatives doubted that broad consent conveyed all relevant information and prima facie favored specific consent. Data protection officers agreed that specific consent was better suited than broad consent to inform patients adequately, while they deemed broad consent more advantageous for researchers. Researchers pointed to the amount of work needed to design and obtain specific consent and to obtain project-specific ethics approval. They claimed that in order to be workable, biobanks and large health data registries presupposed broad consent solutions for research:
Also natürlich, vielmehr auch spezifisch eigentlich spricht mich prinzipiell am meisten an, weil ich dann am ehesten die Klarheit habe, wofür genau, für welches Ziel und wer und in welchem Rahmen und so. [Patient representative 1]
[So of course, it’s specific that appeals to me the most in principle, because that’s when I have the most clarity about what exactly, for what goal and who and in what context and so on.] [Patient representative 1, translation by SW]
Umgekehrt haben wir natürlich bei den projektspezifischen Dingen allein so viel Reibungs- oder Zeitverluste, dass sie durch die Ethik durchmüssen, das dreimal überarbeiten müssen und darüber verliert man auch viel. Und deswegen ist die spezifische Aufklärung manchmal schon durchaus auch ein Problem... [Medical researcher 3]
[Conversely, of course, with project-specific things alone we have so much friction and loss of time; they have to pass through ethics, they have to be revised 3 times and thereby there is a lot of loss as well. And therefore specific consent is indeed sometimes also a problem...] [Medical researcher 3, translation by SW]
As a less obvious point, both data protection officers and IT specialists remarked that designing and implementing broad consent could be very complex and time-consuming as well. Data protection officers mentioned the standard broad consent form of the MII as an example, as it contains a number of modules that can but need not be included in local applications. They pointed out that MII broad consent came in regularly updated versions that needed to be tracked and offered some yes or no options for patients (MII yes or no options include transferring data to countries without EU adequacy decision, access to data from outpatient care, transferring data to and from other medical research facilities, and being contacted in case of incidental findings). They also referred to requests to data use and access committees, pseudonymization, and related data management issues that remain laborious when using broad consent. IT specialists stressed the costs of implementing broad consent and the labor needed to establish consent procedures that were not project specific. They also stressed that once established, the efficiency of digital consent implementation of either broad consent or other consent models could be high:
Gerade, wenn wir jetzt Medizininformatik noch mal berücksichtigen, die verschiedenen Einwilligungsversionen, also das macht es extrem komplex. Ich habe einen Patienten, der hat meinetwegen die 1.6D-Variante unterschrieben, hat aber nicht mehr die 1.72 unterschrieben. Das muss ich alles irgendwo ab- oder möchte man abbilden, muss man aus meiner Sicht auch abbilden. [Data protection officer 1]
[In particular, if we look at the medical informatics initiative once again, the different versions, so that makes it extremely complex. I have a patient, who has, let’s assume, signed version 1.6D, but hasn’t signed 1.72 anymore. One has to—or one wants to, has to in my opinion, map that.] [Data protection officer 1, translation by SW]
Aber ich meine, die meisten Lösungen, die ich kenne, die versuchen jetzt alles, also sowohl die klinischen als auch die studienspezifischen als auch den Broad Consent über so eine selbe Plattformlösung abzubilden, und dann hat man natürlich vereinheitlichte Prozesse. Dann kann man da sehr effektiv mit umgehen. [Health IT specialist 3]
[But I think, most of the solutions I know, they attempt to do it all, so the clinical, as well as the study specific, as well as the broad consent—to map it all over the same platform solution. And then one has standardized processes. Then one can work very effectively.] [Health IT specialist 3, translation by SW]
Tiered Consent
Tiered consent is the least well-known model among our participants. Nevertheless, it was regarded by some as the best option to allow for something broader than specific consent while still providing patients with a good degree of control over their data:
ist aus meiner Sicht das gestufte Modell natürlich, also wenn man schon, sagen wir mal so, in die Forschung grundsätzlich einwilligt als Patient, dann ist das gestufte Modell natürlich das mit der besten Flexibilität, gegebenenfalls mit der höchsten Transparenz. [Patient representative 2]
[From my point of view, of course, if one, let’s say, generally consents to research as a patient, then the tiered model is of course the one with the best flexibility, possibly with the highest transparency.] [Patient representative 2, translation by SW]
It was identified as somewhat less favorable for data research assuming the resulting average permission for the use of data would be narrower than for broad consent. Concerns regarding the efforts needed to manage individual consent profiles were raised. Broad consent, as suggested by the MII in Germany, is seen as not far removed from a model of tiered consent, as it offers options for patients to express preferences (compare in the Attitudes on Specific and Broad Consent section).
Comprehensibility
Lack of comprehensibility of information material was named by all groups as an important obstacle to obtaining adequate consent, regardless of which consent model is chosen. IT representatives and researchers generally ascribed a lack of comprehensibility to legal requirements, including GDPR requirements:
Also die Vorgaben...die...für Einwilligungserklärungen vorgegeben werden, die sind nicht mehr leicht verständlich. Die sind sehr komplex. Da sind sehr viele Rechtstexte drin. Also das braucht mir niemand erzählen, dass es Teilnehmer gibt, die sich das bis zum Ende wirklich exakt durchlesen und dann auch noch verstanden haben, das glaube ich nicht. [Health IT specialist 2]
[As in, those guidelines...for consent forms, they are no longer easy to understand. They are highly complex. There is a lot of legal text in them. Like, no one has to tell me that there are participants who actually read this in detail, through to the end, and after that have really understood it. I don’t believe it.] [Health IT Specialist 2, translation by SW]
One interviewed data protection officer pointed out that while data protection information may often, by habit, make use of complicated legal terminology, the GDPR explicitly asks for comprehensible information. Solutions offered include oral information by specifically trained personnel alone or together with researchers (for more details concerning this point, see the Expertise and Professional Background of Persons Obtaining Consent section).
Videos on specific data protection issues (such as pseudonymization and anonymization) were regarded by patient representatives and researchers as valuable supplements to in-person information, especially because these materials provide the opportunity for patients to become acquainted with relevant issues at home or in other places distinct from the clinical and therapeutic context.
Finally, IT specialists and data protection officers welcomed the option of web-based patient information sites on health data research as a tool to ameliorate information transfer. Data protection officers argued that such a platform could be used to recruit patients for health data research as well, which could lead to more active involvement of patients:
Und dann kann man das noch größer denken. Dann kann man eine solche Plattform, Transparenz, kann man so gestalten, dass die Bürger sich erkundigen können: Was gibt es für Forschungsprojekte? Dann können wiederum Forscher in dieser Plattform ihre Forschungsprojekte präsentieren. Und die Bürger können sich aktiv bewerben darum und sagen: Kann ich da nicht mitmachen bei dem Forschungsprojekt? [Data protection officer 2]
[And then one can think that on a bigger scale. One could create such a platform, transparency, fashion it in a way that the citizens can inform themselves: what research projects are there? And then the researchers can use the platform to present their projects. And citizens can actively apply for them and say: Can I join in this research project?] [Data protection officer 2, translation by SW]
Expertise and Professional Background of Persons Obtaining Consent
Patient representatives were reluctant to accept researcher physicians as the sole contact persons for obtaining in-person health data research information. This is due to suspected partiality and lack of relevant data protection expertise. As a consequence, patient representatives in our interviews preferred researcher-independent information providers with specific data expertise in addition to researchers providing information:
Also ich stelle mir schon vor, dass die Aufklärung sehr gut ist und differenziert ist und verständlich ist, also dass mir ein Datenschutzmensch und ein Computermensch erklären, worin die Chancen und Risiken bestehen, dass mir ein medizinisch geschulter Mensch erklärt, was der Sinn dieser ganzen Sache ist und auch mir den Ablauf erklären kann…Aber für mich ist immer wichtig, dass es auch aus kritischer Seite beleuchtet wird. Wenn da nur Befürworter sozusagen Propaganda mir liefern [lacht], dann fühle ich mich nicht wirklich aufgeklärt. [Patient representative 1]
[So, I imagine it like this, that the disclosure of information is very good and differentiated and intelligible, as in that a data protection person and an IT person explain where the risks and opportunities lie, and that a medically informed person tells me what the purpose of the whole thing is and also explains the process to me.... But for me, it is always important that the critical aspects are also highlighted. If there are advocates delivering propaganda [laughs] than I do not really feel well informed.] [Patient representative 1, translation by SW]
Researchers also pointed out that compared to physicians, specifically trained personnel or reception desk personnel are better options for providing information in the informed consent procedure. The main reason invoked is the amount of time needed to communicate in person: scarce time for researcher physicians that ought to be used for what they deem to be more important tasks. Researchers assume that the amount of time needed to provide information in person will be huge, not only with regard to specific consent but also regarding broad consent. In addition to calling on other professions, researchers also mentioned web-based information and consent management platforms as possible sources of information that may help to reduce the amount of time that they must spend on data information and consent.
Undue Pressure to Consent
Patient representatives referred to undue time pressure when data consent is tied to a clinical appointment. One representative referred to the mental overload of being asked to consent to health data research in a situation when patients seek treatment. Patient representatives generally agreed on the advantages of a data information and consent procedure scheduled independently of clinical appointments: at the clinic after treatment, at home before an appointment, or at home at a point of time completely at one’s disposal:
Und das ist letztlich auch eine Überforderung in dieser Situation, dass man wirklich noch gut abwägen könnte. Das heißt, man muss erst in einem Zustand sein der Ruhe oder der guten Überlegung, wo man wieder sein Hirn normal zur Verfügung hat, um dann zu entscheiden. [Patient representative 1]
[And that is in the end also an overburdening, in that situation, that you could really weigh your options. I mean, one needs to be in a state of calm, or careful consideration, where you can access your brain normally, to decide.] [Patient representative 1, translation by SW]
Withdrawal of Consent
A topic that was discussed broadly in our interviews that has so far seen little attention in the ethical literature on consent concerns the implementation of withdrawal of consent. Researchers and data protection officers not only highlighted how important they consider this option to be but also pointed out that hospitals have not yet established smooth procedures to deal with withdrawal requests. Up until now, it has been difficult and time-consuming for them to handle such withdrawals. IT specialists were very much aware of this topic as well and highlighted that all digital solutions that are currently in development also aim to make changes to consent and withdrawal of consent transparent and easy to handle. From the perspective of patients, this topic was touched upon when they discussed whether and how their legal rights can be assured. Patient representatives were concerned that patients have no means to verify that their data have been erased (or samples have actually been destroyed) in response to their request:
Es muss ein Management geben, das die Einwilligungserklärungen widerrufbar macht. Wo geht der Widerruf ein von dem Patienten? Was wird mit dem Widerruf gemacht? Wie wird der umgesetzt? Wer ist dafür zuständig? In welcher Frist wird das gemacht? Was ist, wenn der Patient Auskunft haben möchte? Genau dasselbe Spiel. Eine Einwilligungslösung, eine Einwilligung bedeutet nicht ein Stück Papier, was im Schrank steht, abgeheftet wird und niemand hat damit mehr was zu tun, sondern all das müsste dann auch sichergestellt sein. [Data protection officer 2]
[There needs to be a management, that allows for withdrawal of consent. Where is the withdrawal of a patient registered? What happens with the withdrawal? How is it implemented? Who is responsible for that? In what timeframe? What if the patient is asking for disclosure? The same thing again. A solution for consent, a consent, isn’t just a piece of paper that is put into the cabinet, filed away, and no one will ever interact with it anymore, but all of this needs to be ensured.] [Data protection officer 2, translation by SW]
Consent Management
IT specialists and the data protection officers agreed that not only for a model of tiered consent but also for the wide introduction of broad consent in German university medical centers, a digital system for consent management is required. Having to deal with a vast number of paper-based consent forms does not appear to be viable in any way. They pointed out that even in the case of broad consent, a given consent does not equal another given consent since, first, the MII broad consent offers options for patients to specify consent, and, second, the standardized MII consent sample text is regularly updated and thus comes in a number of versions that need to be tracked (as referred in the Attitudes on Specific and Broad Consent section). If any given consent is to be respected in regard to what it does or does not cover, a system that allows researchers or data trustees to access and manage consent forms is a necessity. Many of our interview partners opined that without this management, broad consent, or any other model of consent, cannot be handled efficiently. It was pointed out that the development of a software application for consent management would likely prove to be too expensive for smaller research projects since there are no such systems readily available on the market yet:
Nur ich sage mal, also das Medium an sich ist jetzt wahrscheinlich dann gar nicht so relevant, ob das auf Papier oder digital ist. Wichtig ist, glaube ich, nur, dass man größere Mengen dann einfach nicht mehr auf Papier händeln kann, was dann aber eher eine Rolle spielt in Richtung Datenherausgabe/Probenherausgabe und solche Sachen, dass man da einfach schon gezwungen ist, digital diese Consente abzubilden in irgendeiner Form. [Health IT specialist 2]
[Well, I’d say, like, the medium itself probably isn’t all that relevant, whether it is paper or digital. What is important, I think, is that with larger numbers you cannot handle it on paper anymore, which in that case is more important in regard to handing out data or specimens and such things, that you are forced in that case, to map the consent digitally in some form.] [Health IT specialist 2, translation by SW]
Transfer of Health Data
Statements regarding the transfer of health data to for-profit organizations or non-EU countries revealed a dilemma. Concerns were voiced not only predominantly by patient representatives but also by the other groups that actors in the private sector have to be considered as less trustworthy. Industry partners cannot always be expected to act in the best interest of the public; data security standards might be lower in countries beyond the EU, and, therefore, patients should have the possibility to exclude the transfer of their data to industry partners or third countries:
Und ganz schwierig finde ich es, sage ich mal, wenn solche Daten halt, wie gesagt, an die Industrie weitergeleitet werden. Dann sind sie vollkommen außerhalb des Einflussbereiches der Uniklinika und so weiter und so fort. Und da kann man mir noch so viel andere Sachen halt erzählen, es geht in der Privatindustrie immer darum, Geld zu verdienen, immer möglichst viel Geld zu verdienen. Altruistische Motive sehe ich da in der Regel nicht am Werke. [Data protection officer 3]
[And I think it is really problematic, let’s say, if such data, as we said, is handed over to industry. Then they are completely outside the area of influence of university medical centers and so on and so forth. And you can tell me different as much as you like, in the private industry, it is always about money. Making as much money as possible. I generally do not see any altruistic motivations at work there.] [Data protection officer 3, translation by SW]
Regarding the contemporary research landscape, the necessity of exactly these kinds of cooperations was highlighted. The lack of permission to share data with non-European countries (or countries without an EU adequacy decision) was named as a showstopper for biobanks. As an example, the problematic legal status of the sensible wish of US-based companies to extract data from their robotic surgery systems used in German clinics in order to further develop their technologies was mentioned. It was pointed out that even publishing research results in US-based journals might be legally challenging, given the current regulation, if this requires uploading nonanonymized health data to US servers. With regard to broad consent in particular, a consent type that excludes these types of cooperation is regarded to significantly hinder valuable research:
Es gab, ich glaube, die Version 1.6D der Einwilligung...die aber die Einschränkung oder die wesentliche Einschränkung hatte, dass der Drittlandstransfer ausgeschlossen war, und dass eine Kooperation oder Zusammenarbeit mit Industrie ausgeschlossen war. ..., da kam für uns auch das Feedback: Ja Entschuldigung, damit, mit diesem Informed Consent können wir eigentlich im Grunde nichts anfangen oder sind extrem beschnitten. [Data protection officer 1]
[There was, I believe, that version 1.6D of the consent...but that had the essential limitation that transfer of data to third countries was excluded, and that also a cooperation with industry was excluded....so we got the feedback: Well, excuse me, but ultimately, we can’t make use of this consent or we are extremely limited.] [Data protection officer 1, translation by SW]
Research Without Consent
One topic that was discussed controversially in regard to the legal framework regulating consent for health data research concerns the need for consent in general. Researchers voiced the opinion that collecting consent, no matter in which form, is a time-consuming process. They claimed that more research could be accomplished if research was possible without consent. While some researchers considered this as a desirable scenario, others highlighted the need to obtain consent and involve patients to ensure their continuous support. Beyond personal attitudes, all the researchers were pessimistic about research without consent becoming a viable option in Germany in the near future, notwithstanding the fact that the GDPR does allow for research without consent under certain conditions (compare with the information in the Background section):
Fände ich super. Das funktioniert nicht mit der Organspende und das funktioniert nicht mit dem Broad Consent für Forschung, weil da gibt es viel zu viele, die da... Nein, never ever. Aber finde ich gut. [Medical researcher 1]
[That would be great. But that doesn’t work for organ donation and that doesn’t work for broad consent to research. Because, there are way too many.... No, never ever. But I think it would be good.] [Medical researcher 1, translation by SW]
Referring to the GDPR, data protection officers did mention research options without consent. On the basis of specific state laws, local regulations exist which enable research without consent, most typically in cases of retrospective studies that only make use of health data stored at the hospital at which the research takes place (so-called Eigenforschung ). However, the differences between federal state regulations were mentioned as a hindrance to multicenter cross-state research. A national regulation for health data sharing was suggested as a helpful means to achieve legal and governance clarity on this issue:
wenn Sie das mit einer Einwilligung versuchen, wird es immer Personen geben, die aus den verschiedensten Gründen sagen: Möchte ich nicht. Und wenn wir dann aber über ein gesundheitspolitisches oder gesellschaftspolitisches Gesundheitsmanagement sprechen, dann wäre es natürlich schon hilfreich, wenn unter klar definierten Rahmenbedingungen Patientendaten, also die digital vorhandenen Daten, für definierte Forschungsvorhaben verwendet werden dürften. [Data protection officer 1]
[If you try that with consent, there will always be someone saying no, for different reasons. I don’t want to. And if we are talking about health policy, or sociopolitical management of public health, then it would of course be helpful if under clearly defined conditions patient data, data available in digital form, could be used for specific research purposes.] [Data protection officer 1, translation by SW]
IT specialists were less concerned with this topic, but some voiced the opinion that any regulation that does not leave at least the possibility to opt out would stand in stark opposition to informational self-determination. This assessment was shared by some of the data protection officers.
This topic did not receive much explicit attention from patient representatives. However, when it was mentioned, it was not seen favorable at all. Options of control for patients in regard to the use of their data were rated as highly important, as expressed through a preference for models of specific or tiered consent, while even broad consent was rejected as not giving sufficient degrees of control:
wir reden ja zum Glück nicht um einwilligungsfreie Forschung, das ist ja nicht das Thema [Patient representative 2]
[Luckily we are not talking about research without consent. It is not our topic here.] [Patient representative 2, translation by SW]
oder dass man das [die Einwilligung] dann teilweise gar nicht braucht, gerade wenn man noch nach Europa schaut. Da werden ganz andere Sachen vorgeschlagen, inklusive Genom-Daten, auch der selbstverständlichen Freigabe ohne Rückholmöglichkeit. Also wenn wir jetzt schon in einem Ethikdiskurs sind, finde ich das irgendwie empörend. [Patient representative 2]
[So that partially, it [consent] is not needed, in particular if we look at Europe. There are completely different things being suggested, including genomic data, as well as the releasing of data without an option to retract, as a matter of course. If we are already in an ethical discourse here, I find that somewhat outrageous.] [Patient representative 2, translation by SW]
Importance of Findings
In light of the current EU efforts to establish a European health data space and initiatives in other countries, including Germany, to develop digital infrastructures that allow large-scale access to health data for research, determining ethically, legally, and socially acceptable consent procedures is a task of utmost importance [ 1 , 3 - 8 ].
The attitudes expressed by our sample of German stakeholders provide some insight into how workable and acceptable consent may look like in Germany. As developments with regard to consent within the European health data space and in other countries are still in flux, these findings can prove valuable for other national contexts and the EU as well.
Limitations
Our study has an explorative character, and the significance of the results is necessarily limited due to the small size of the study.
The stakeholders interviewed were limited to the 4 groups of medical researchers at university medical centers, health IT specialists, data protection officers, and patient representatives, all from Germany. With the European health data space in the making, interviews with stakeholders from other European countries on consent and models of consent would be of immense value. Perspectives from other stakeholder groups, such as for-profit health research companies, clinic administrative employees, and members of hospital executive boards, could provide further valuable insights.
Regarding patient attitudes, qualitative and quantitative studies on a larger scale would be needed to provide a clearer picture of interests and concerns.
Options of Choice Within Consent
Our interviews do not reveal unequivocal endorsement of 1 specific model of consent across stakeholder groups. While researchers favored broad consent, patient representatives stressed that only specific consent opens up the possibility of receiving detailed information on planned research and the use of data within this research, with tiered consent being regarded as a possibly acceptable alternative. IT specialists and data protection officers were more neutral regarding this controversy. However, IT specialists pointed out that broad consent (at least as devised by the German MII) has limited advantages over tiered consent in regard to management effort as it comes in versions, contains optional modules for research locations, and provides some options for patients to tailor their consent.
In practice, with regard to implementation and management efforts, broad consent thus might often come close to tiered consent. If this holds true and if one wants to harness the advantage of tiered consent to be able to mirror patient preferences, more efforts should be made to determine which options of choice should actually be included. As it appears now, options are dictated mainly by legal requirements.
This issue appears important as patient representatives in our sample also raised concerns about for-profit organizations accessing their data. Currently, although the MII consent includes some options of choice for patients such as data being transferred to non-EU countries, data transfer to for-profit organizations is not among them. It is obligatorily included in the scope of the MII consent.
Digital Tools and Platforms
There is no doubt that efficient data sharing across borders and between different institutions within the EU presupposes a well-functioning digital infrastructure for health data as well as consent data.
In addition, a variety of issues point toward including digital formats and tools as part of the information and consenting process as well. Researchers are reluctant to spend much time on informing patients and obtaining consent. Conflating clinical appointments with providing consent to health data research might result in mental overload and undue pressure. Patient information is often incomprehensible and does not enable patients to become well-informed. Digital tools could diminish researcher workload and, at the same time, provide patients with better opportunities to absorb relevant information. All these issues highlight the importance of adequate place, time, and format for providing information. Whether or not researchers should still be involved in this process in person remains a disputed issue.
Ensuring that withdrawal of consent can be handled smoothly and effectively is not as trivial as it may sound. Appropriate workflows and processes should still be implemented in many German hospitals. Digital consent management solutions will have to be able to deal with this challenge. Patient representatives in our sample doubted that it would be possible for patients to obtain information on which consent they have granted to an institution and which personal information is stored and used. They also expressed doubt whether a request for data erasure, a key GDPR right of persons whose data are used, would be fulfilled. These concerns and challenges could be mitigated by digital interfaces and platforms that are especially geared toward handling consent and patient requests concerning erasure, rectification, and withdrawal and are directly linked to the systems storing health data and processing research requests, as already in place in, for example, Estonia [ 43 ].
The GDPR leaves room for the use of health data for research without consent (compare with the Background section). In specific cases and under specific conditions, German hospitals make use of this option. Due to the local character of these agreements and the diverse landscape of the federal state regulations in Germany, it is not transparent where and under which conditions such research takes place. In addition, the lack of unified regulation renders multicenter collaborations with centers in different states difficult. As a consequence, national regulations on health data research without consent appear desirable.
The stakeholder groups were divided on whether research on health data without consent could become more of a standard approach, compared with the few cases today. While data protection officers pointed out that the GDPR leaves room for this kind of research if national regulation is in place, medical researchers were pessimistic with regard to societal acceptance. Patient representatives in our sample were highly critical of this option.
Opt-out solutions, potentially tiered, in combination with easily accessible information about health data research and the possibility of checking for patients which studies make use of their data may be met with more acceptance since such a framework would be transparent and allow for a certain degree of control.
Implementation of Consent and Consent Management
Implementation of the current procedures to obtain consent is criticized for various reasons, most importantly for lack of comprehensibility of information and implicit pressure to consent due to the integration of the data information and consent process in regular medical appointments. In addition, the impartiality of researchers and their competence regarding data protection are called into question. For researchers, providing information on data research is time-consuming and goes at the expense of other duties.
Detaching consent procedures from the clinical setting; providing additional schooled personnel for informing and obtaining consent; and providing alternative forms of information material, such as videos and well-structured web pages, seem promising avenues to mitigate the concerns and drawbacks of current consent implementation.
Conclusions
Politically, in the EU and countries such as Germany, broad consent and, of lately, opt-out solutions are discussed and pushed forward as part of initiatives to build large health data research infrastructures. Our results indicate that these solutions as such may not do justice to the concerns and demands of patients. From the perspectives of participants in our study, both broad consent and opt-out solutions should include tiered options of consenting and opting out, respectively. These tiered options should mirror major patient concerns.
Regarding the implementation of consent procedures, including broad consent, digital management appears to be indispensable in enabling the handling of requests for information disclosure, erasure, rectification, and withdrawal of information. Participants in our study assumed that digital implementation holds great potential for improving workflows and diminishing researcher workload.
Participants in our study pointed out that having access to regularly updated information on ongoing studies is important. This issue should not be underestimated. It is relevant for both broad consent and opt-out solutions. Again, digital data management may allow the implementation of patient information modules that provide easily accessible, timely, and adequate information about ongoing research.
Finally, participants in our study stressed that it would be desirable to remove health data consent procedures from admission to the hospital and the clinical context in order to allow for a well-considered decision. Although digital consent management could allow for such a separation, implementation of consent in the German MII, for example, still connects hospital admission and consent.
Acknowledgments
Our research was supported by the research project DaDuHealth—Data Access and Data Use in Medical Institutional and Consumer Health Settings. An Ethical, Legal, and Social Analysis, funded by the German Ministry of Education and Research (grant 01GP1902).
Conflicts of Interest
None declared.
Interview guide (German).
Overview of interview results (English version).
Overview of interview results (German version).
- European health data space. European Commission. URL: https://health.ec.europa.eu/ehealth-digital-health-and-care/european-health-data-space_en [accessed 2024-04-29]
- Truyers C, Goderis G, Dewitte H, Akker MV, Buntinx F. The Intego database: background, methods and basic results of a Flemish general practice-based continuous morbidity registration project. BMC Med Inform Decis Mak. Jun 06, 2014;14:48. [ FREE Full text ] [ CrossRef ] [ Medline ]
- Abboud L, Cosgrove S, Kesisoglou I. Country factsheets: mapping health data management systems through country visits: development, needs and expectations of the EHDS. The European Union. 2023. URL: https://tehdas.eu/app/uploads/2023/04/tehdas-mapping-health-data-management-systems-through-country-visits.pdf [accessed 2024-04-29]
- The guidance function closes report. Én Indgang til sundhedsdata. URL: https://www.vejledningsfunktionen.dk/en/homepage/ [accessed 2024-04-29]
- InnoHealth datalake project. InnoHealth. URL: https://innohealth.eu/en/datalake/project/ [accessed 2024-04-29]
- The PHIRI project. The Population Health Information Research Infrastructure. URL: https://www.phiri.eu/project [accessed 2024-04-29]
- ECRIN overview. European Clinical Research Infrastructure Network. URL: https://ecrin.org/ecrin-overview [accessed 2024-04-29]
- Über die initiative. Medizin Informatik Initiative. URL: https://www.medizininformatik-initiative.de/de/ueber-die-initiative [accessed 2024-04-29]
- Big Data und Gesundheit: Datensouveränität als Informationelle Freiheitsgestaltung. Berlin, Germany. Deutscher Ethikrat; 2018.
- Mittelstadt BD, Floridi L. The ethics of big data: current and foreseeable issues in biomedical contexts. Sci Eng Ethics. Apr 2016;22(2):303-341. [ CrossRef ] [ Medline ]
- Ploug T, Holm S. The 'expiry Problem' of broad consent for biobank research - and why a meta consent model solves it. J Med Ethics. Sep 2020;46(9):629-631. [ CrossRef ] [ Medline ]
- Mikkelsen RB, Gjerris M, Waldemar G, Sandøe P. Broad consent for biobanks is best - provided it is also deep. BMC Med Ethics. Oct 15, 2019;20(1):71. [ FREE Full text ] [ CrossRef ] [ Medline ]
- Wiertz S, Boldt J. Evaluating models of consent in changing health research environments. Med Health Care Philos. Jun 2022;25(2):269-280. [ FREE Full text ] [ CrossRef ] [ Medline ]
- Caulfield T, Murdoch B. Genes, cells, and biobanks: yes, there's still a consent problem. PLoS Biol. Jul 2017;15(7):e2002654. [ FREE Full text ] [ CrossRef ] [ Medline ]
- Rothstein MA, Harrell HL, Saulnier KM, Dove ES, Fan CT, Hung TH, et al. Broad consent for future research: international perspectives. IRB. Nov 01, 2018;40(6):7-12. [ CrossRef ]
- Donnelly M, McDonagh M. Health research, consent and the GDPR exemption. Eur J Health Law. Apr 24, 2019;26(2):97-119. [ FREE Full text ] [ CrossRef ] [ Medline ]
- Dove ES, Chen J. Should consent for data processing be privileged in health research? A comparative legal analysis. Int Data Priv Law. 2020;10(2):117-131. [ CrossRef ]
- WMA declaration of Taipei on ethical considerations regarding health databases and biobanks: adopted by the 53rd WMA general assembly, Washington, DC, USA, October 2002 and revised by the 67th WMA general assembly, Taipei, Taiwan, October 2016. World Medical Association. 2016. URL: https://www.wma.net/policies-post/wma-declaration-of-taipei-on-ethical-considerations-regarding-health-databases-and-biobanks/ [accessed 2024-04-29]
- Ploug T, Holm S. Informed consent and routinisation. J Med Ethics. Apr 2013;39(4):214-218. [ CrossRef ] [ Medline ]
- Cambon-Thomsen A. The social and ethical issues of post-genomic human biobanks. Nat Rev Genet. Nov 2004;5(11):866-873. [ CrossRef ] [ Medline ]
- Morain SR, Largent EA. Public attitudes toward consent when research is integrated into care-any "ought" from all the "is"? Hastings Cent Rep. Mar 2021;51(2):22-32. [ FREE Full text ] [ CrossRef ] [ Medline ]
- Warner TD, Weil CJ, Andry C, Degenholtz HB, Parker L, Carithers LJ, et al. Broad consent for research on biospecimens: the views of actual donors at four U.S. medical centers. J Empir Res Hum Res Ethics. Apr 2018;13(2):115-124. [ FREE Full text ] [ CrossRef ] [ Medline ]
- Richter G, Krawczak M, Lieb W, Wolff L, Schreiber S, Buyx A. Broad consent for health care-embedded biobanking: understanding and reasons to donate in a large patient sample. Genet Med. Jan 2018;20(1):76-82. [ FREE Full text ] [ CrossRef ] [ Medline ]
- Köngeter A, Schickhardt C, Jungkunz M, Bergbold S, Mehlis K, Winkler EC. Patients' willingness to provide their clinical data for research purposes and acceptance of different consent models: findings from a representative survey of patients with cancer. J Med Internet Res. Aug 25, 2022;24(8):e37665. [ FREE Full text ] [ CrossRef ] [ Medline ]
- Wiertz S. Die zeitliche dimension des broad consent. Ethik Med. Aug 06, 2022;34(4):645-667. [ CrossRef ]
- Master Z, Nelson E, Murdoch B, Caulfield T. Biobanks, consent and claims of consensus. Nat Methods. Sep 2012;9(9):885-888. [ CrossRef ] [ Medline ]
- Hofmann B. Broadening consent--and diluting ethics? J Med Ethics. Mar 2009;35(2):125-129. [ CrossRef ] [ Medline ]
- Budin-Ljøsne I, Teare HJ, Kaye J, Beck S, Bentzen HB, Caenazzo L, et al. Dynamic consent: a potential solution to some of the challenges of modern biomedical research. BMC Med Ethics. Jan 25, 2017;18(1):4. [ FREE Full text ] [ CrossRef ] [ Medline ]
- Kaye J, Whitley EA, Lund D, Morrison M, Teare H, Melham K. Dynamic consent: a patient interface for twenty-first century research networks. Eur J Hum Genet. Feb 2015;23(2):141-146. [ FREE Full text ] [ CrossRef ] [ Medline ]
- Ploug T, Holm S. Meta consent: a flexible and autonomous way of obtaining informed consent for secondary research. BMJ. May 07, 2015;350:h2146. [ CrossRef ] [ Medline ]
- Prictor M, Teare HJ, Kaye J. Equitable participation in biobanks: the risks and benefits of a "dynamic consent" approach. Front Public Health. 2018;6:253. [ FREE Full text ] [ CrossRef ] [ Medline ]
- Nembaware V, Johnston K, Diallo AA, Kotze MJ, Matimba A, Moodley K, et al. A framework for tiered informed consent for health genomic research in Africa. Nat Genet. Nov 2019;51(11):1566-1571. [ FREE Full text ] [ CrossRef ] [ Medline ]
- Slokenberga S, Tzortzatou O, Reichel J. GDPR and Biobanking: Individual Rights, Public Interest and Research Regulation across Europe. Cham, Switzerland. Springer Nature; 2021.
- Kalkman S, van Delden J, Banerjee A, Tyl B, Mostert M, van Thiel G. Patients' and public views and attitudes towards the sharing of health data for research: a narrative review of the empirical evidence. J Med Ethics. Nov 12, 2019;48(1):3-13. [ FREE Full text ] [ CrossRef ] [ Medline ]
- Aitken M, de St Jorre J, Pagliari C, Jepson R, Cunningham-Burley S. Public responses to the sharing and linkage of health data for research purposes: a systematic review and thematic synthesis of qualitative studies. BMC Med Ethics. Nov 10, 2016;17(1):73. [ FREE Full text ] [ CrossRef ] [ Medline ]
- Shah N, Coathup V, Teare H, Forgie I, Giordano GN, Hansen TH, et al. Motivations for data sharing-views of research participants from four European countries: a DIRECT study. Eur J Hum Genet. May 2019;27(5):721-729. [ CrossRef ] [ Medline ]
- Richter G, Borzikowsky C, Hoyer BF, Laudes M, Krawczak M. Secondary research use of personal medical data: patient attitudes towards data donation. BMC Med Ethics. Dec 15, 2021;22(1):164. [ FREE Full text ] [ CrossRef ] [ Medline ]
- Brown KM, Drake BF, Gehlert S, Wolf LE, DuBois J, Seo J, et al. Differences in preferences for models of consent for biobanks between black and white women. J Community Genet. Jan 2016;7(1):41-49. [ FREE Full text ] [ CrossRef ] [ Medline ]
- Köngeter A, Jungkunz M, Winkler EC, Schickhardt C, Mehlis K. Sekundärnutzung klinischer Daten aus der Patientenversorgung für Forschungszwecke – eine qualitative Interviewstudie zu Nutzen- und Risikopotenzialen aus Sicht von Expertinnen und Experten für den deutschen Forschungskontext. In: Richter G, Loh W, Buyx A, von Kielmansegg SG, editors. Datenreiche Medizin und das Problem der Einwilligung: Ethische, rechtliche und sozialwissenschaftliche Persp. Cham, Switzerland. Springer; 2022:185-210.
- Kitzinger J. Qualitative research. Introducing focus groups. BMJ. Jul 29, 1995;311(7000):299-302. [ FREE Full text ] [ Medline ]
- Mayring P. Qualitative inhaltsanalyse. In: Boehm A, Mengel A, Muhr T, editors. Texte Verstehen: Konzepte, Methoden, Werkzeuge. Konstank, Germany. Universitätsverlag Konstanz; 1994:159-175.
- Kuckartz U. Qualitative Inhaltsanalyse. Methoden, Praxis, Computerunterstützung. Weinheim, Basel. Beltz Juventa; 2018.
- Metsallik J, Ross P, Draheim D, Piho G. Ten years of the e-health system in Estonia. CEUR Workshop Proceedings. 2018. URL: https://ceur-ws.org/Vol-2336/MMHS2018_invited.pdf [accessed 2024-07-17]
Abbreviations
European Union |
General Data Protection Regulation |
medical informatics initiative |
Edited by S Ma; submitted 29.08.23; peer-reviewed by G Richter, R Hendricks-Sturrup; comments to author 28.11.23; revised version received 15.01.24; accepted 31.05.24; published 07.08.24.
©Svenja Wiertz, Joachim Boldt. Originally published in the Journal of Medical Internet Research (https://www.jmir.org), 07.08.2024.
This is an open-access article distributed under the terms of the Creative Commons Attribution License (https://creativecommons.org/licenses/by/4.0/), which permits unrestricted use, distribution, and reproduction in any medium, provided the original work, first published in the Journal of Medical Internet Research (ISSN 1438-8871), is properly cited. The complete bibliographic information, a link to the original publication on https://www.jmir.org/, as well as this copyright and license information must be included.
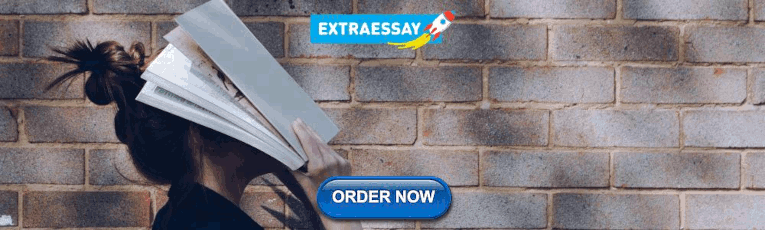
IMAGES
VIDEO
COMMENTS
Qualitative data analysis is a systematic process of examining non-numerical data to extract meaning, patterns, and insights. In contrast to quantitative analysis, which focuses on numbers and statistical metrics, the qualitative study focuses on the qualitative aspects of data, such as text, images, audio, and videos.
Qualitative research involves collecting and analyzing non-numerical data (e.g., text, video, or audio) to understand concepts, opinions, or experiences. It can be used to gather in-depth insights into a problem or generate new ideas for research. Qualitative research is the opposite of quantitative research, which involves collecting and ...
Qualitative Research. Qualitative research is a type of research methodology that focuses on exploring and understanding people's beliefs, attitudes, behaviors, and experiences through the collection and analysis of non-numerical data. It seeks to answer research questions through the examination of subjective data, such as interviews, focus ...
Qualitative Data Analysis methods. Once all the data has been captured, there are a variety of analysis techniques available and the choice is determined by your specific research objectives and the kind of data you've gathered. Common qualitative data analysis methods include: Content Analysis. This is a popular approach to qualitative data ...
How to conduct qualitative research? Given that qualitative research is characterised by flexibility, openness and responsivity to context, the steps of data collection and analysis are not as separate and consecutive as they tend to be in quantitative research [13, 14].As Fossey puts it: "sampling, data collection, analysis and interpretation are related to each other in a cyclical ...
The SAGE Handbook of. tive Data AnalysisUwe FlickMapping the FieldData analys. s is the central step in qualitative research. Whatever the data are, it is their analysis that, in a de. isive way, forms the outcomes of the research. Sometimes, data collection is limited to recording and docu-menting naturally occurring ph.
Qualitative research is the naturalistic study of social meanings and processes, using interviews, observations, and the analysis of texts and images. In contrast to quantitative researchers, whose statistical methods enable broad generalizations about populations (for example, comparisons of the percentages of U.S. demographic groups who vote in particular ways), qualitative researchers use ...
Although qualitative research does not appear to be defined in terms of a specific method, it is certainly common that fieldwork, i.e., research that entails that the researcher spends considerable time in the field that is studied and use the knowledge gained as data, is seen as emblematic of or even identical to qualitative research.
INTRODUCTION. Qualitative research methods refer to techniques of investigation that rely on nonstatistical and nonnumerical methods of data collection, analysis, and evidence production. Qualitative research techniques provide a lens for learning about nonquantifiable phenomena such as people's experiences, languages, histories, and cultures.
The wide range of approaches to data analysis in qualitative research can seem daunting even for experienced researchers. This handbook is the first to provide a state-of-the art overview of the whole field of QDA; from general analytic strategies used in qualitative research, to approaches specific to particular types of qualitative data, including talk, text, sounds, images and virtual data.
QDA Method #3: Discourse Analysis. Discourse is simply a fancy word for written or spoken language or debate. So, discourse analysis is all about analysing language within its social context. In other words, analysing language - such as a conversation, a speech, etc - within the culture and society it takes place.
5. Grounded theory. This method of qualitative data analysis starts with an analysis of a single case to formulate a theory. Then, additional cases are examined to see if they contribute to the theory. Qualitative data analysis can be conducted through the following three steps: Step 1: Developing and Applying Codes.
grams for qualitative data analysis; you will see that these increasingly popular programs are blurring the distinctions between quantitative and qualitative approaches to textual analysis. 22 Features of Qualitative Data Analysis The distinctive features of qualitative data collection methods that you studied in Chapter 9 are also reflected
Quantitative research, with its emphasis on numerical data and statistical analysis, is a cornerstone of scientific inquiry in many fields, particularly the biomedical sciences. However, qualitative research offers a complementary approach, allowing researchers to explore the depth and complexity of human phenomena in greater detail, delving ...
Analyze data: Analyze the data using appropriate qualitative data analysis methods, such as thematic analysis or content analysis. Interpret findings: Interpret the findings of the data analysis in the context of the research question and the relevant literature. This may involve developing new theories or frameworks, or validating existing ...
Qualitative data is non-numerical data, such as text, video, photographs, or audio recordings. This type of data can be collected using diary accounts or in-depth interviews and analyzed using grounded theory or thematic analysis. Qualitative research is multimethod in focus, involving an interpretive, naturalistic approach to its subject matter.
Qualitative data analysis is. concerned with transforming raw data by searching, evaluating, recogni sing, cod ing, mapping, exploring and describing patterns, trends, themes an d categories in ...
Data collection. The methods of qualitative data collection most commonly used in health research are document study, observations, semi-structured interviews and focus groups [1, 14, 16, 17]. Document study. Document study (also called document analysis) refers to the review by the researcher of written materials . These can include personal ...
Doing qualitative research is not easy and may require a complete rethink of how research is conducted, particularly for researchers who are more familiar with quantitative approaches. There are many ways of conducting qualitative research, and this paper has covered some of the practical issues regarding data collection, analysis, and management.
Qualitative research is a type of research that aims to gather and analyse non-numerical (descriptive) data in order to gain an understanding of individuals' social reality, including understanding their attitudes, beliefs, and motivation. This type of research typically involves in-depth interviews, focus groups, or observations in order to collect data that is rich in detail and context.
The pros and cons of qualitative data analysis. Qualitative data analysis looks at the human side of data. It offers insights that numbers alone can't provide. But like all research methods, even qualitative data analysis methods have their strengths and weaknesses, especially when it comes to shaping a marketing plan that hits the mark.
I argue, drawing on both the philosophical and qualitative research literature, that causation is a legitimate concept for qualitative research, and that qualitative methods are essential for ...
Qualitative data dives deep beneath these same numbers and fleshes out the nuances there. Research projects can often benefit from both types of data, which is why you'll see the term "mixed-method" research in peer-reviewed journals. The term "mixed-method" refers to using both quantitative and qualitative methods in a study.
→ To learn more, check out The Practical Guide to Grounded Theory.. Qualitative Content Analysis Overview. Qualitative content analysis is a research method used to systematically categorize and interpret textual data. It organizes large amounts of data to identify patterns, concepts, keywords, categories, and themes.
Qualitative research is a type of research that explores and provides deeper insights into real-world problems.[1] Instead of collecting numerical data points or intervening or introducing treatments just like in quantitative research, qualitative research helps generate hypothenar to further investigate and understand quantitative data. Qualitative research gathers participants' experiences ...
Qualitative content analysis is often used to study interviews, focus groups, and other forms of qualitative data, where the researcher is interested in understanding the subjective experiences and perceptions of the participants. Methods of Content Analysis. There are several methods of content analysis, including: Conceptual Analysis
A principled approach to using machine learning in qualitative education research based on the three interrelated elements of the assessment triangle is proposed based on the three interrelated elements of the assessment triangle: cognition, observation, and interpretation. This study proposes and demonstrates how computer‐aided methods can be used to extend qualitative data analysis by ...
Qualitative Risk Analysis. Qualitative risk assessments are a type of risk evaluation that relies on subjective judgment and expert opinions rather than numerical data. This methodology is beneficial when data is unavailable, incomplete, or difficult to quantify.
In some cases, qualitative data can also include pictorial display, audio or video clips (e.g. audio and visual recordings of patients, radiology film, and surgery videos), or other multimedia materials. Data analysis is the part of qualitative research that most distinctively differentiates from quantitative research methods.
Background: In Europe, within the scope of the General Data Protection Regulation, more and more digital infrastructures are created to allow for large-scale access to patients' health data and their use for research. When the research is performed on the basis of patient consent, traditional study-specific consent appears too cumbersome for many researchers.