Information
- Author Services
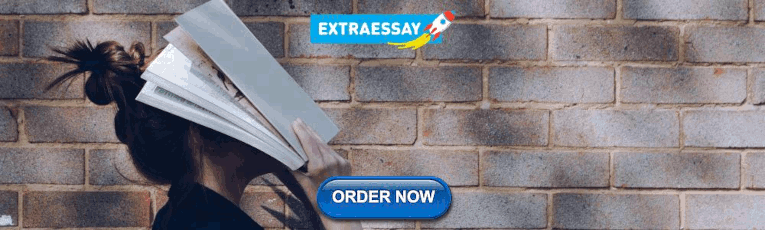
Initiatives
You are accessing a machine-readable page. In order to be human-readable, please install an RSS reader.
All articles published by MDPI are made immediately available worldwide under an open access license. No special permission is required to reuse all or part of the article published by MDPI, including figures and tables. For articles published under an open access Creative Common CC BY license, any part of the article may be reused without permission provided that the original article is clearly cited. For more information, please refer to https://www.mdpi.com/openaccess .
Feature papers represent the most advanced research with significant potential for high impact in the field. A Feature Paper should be a substantial original Article that involves several techniques or approaches, provides an outlook for future research directions and describes possible research applications.
Feature papers are submitted upon individual invitation or recommendation by the scientific editors and must receive positive feedback from the reviewers.
Editor’s Choice articles are based on recommendations by the scientific editors of MDPI journals from around the world. Editors select a small number of articles recently published in the journal that they believe will be particularly interesting to readers, or important in the respective research area. The aim is to provide a snapshot of some of the most exciting work published in the various research areas of the journal.
Original Submission Date Received: .
- Active Journals
- Find a Journal
- Proceedings Series
- For Authors
- For Reviewers
- For Editors
- For Librarians
- For Publishers
- For Societies
- For Conference Organizers
- Open Access Policy
- Institutional Open Access Program
- Special Issues Guidelines
- Editorial Process
- Research and Publication Ethics
- Article Processing Charges
- Testimonials
- Preprints.org
- SciProfiles
- Encyclopedia
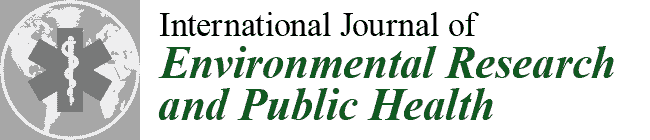
Article Menu

- Subscribe SciFeed
- Recommended Articles
- PubMed/Medline
- Google Scholar
- on Google Scholar
- Table of Contents
Find support for a specific problem in the support section of our website.
Please let us know what you think of our products and services.
Visit our dedicated information section to learn more about MDPI.
JSmol Viewer
Demands for community services and associated factors among residents in smart communities: a case study of xuzhou city.
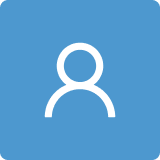
1. Introduction
2. literature review and hypotheses, 2.1. different types of community services in smart communities, 2.2. factors influencing residents’ demands for community services in smart communities, 2.3. hypotheses of this study, 3.1. variables and measures.
- A succinct explanation of community services in smart communities and the intention of this survey;
- Respondents’ basic information;
- The measurement of respondents’ demands for community services in smart communities. The question “Are you in need of this type of community service?” was used in the measurement and residents’ responses were measured as a dichotomous variable, with 1 representing a need for this type of community service and 0 otherwise. In light of the literature review, seven categories of community services in smart communities mentioned above were chosen as outcome variables and measured through residents’ responses to the question;
- Factors influencing respondents’ demands for community services in smart communities, including respondents’ sociodemographic characteristics, living characteristics, economic characteristics, and individual attitude characteristics.
3.2. Sampling and Data Collection
3.3. statistical model and analysis, 4.1. descriptive statistics of the respondents, 4.2. residents’ demands for community services in smart communities in xuzhou, 4.3. results of the binary logistic regression test, 4.3.1. assessment of model fit, 4.3.2. validation of predicted probabilities, 4.3.3. explanation of coefficients in the binary logistic regression, 5. discussion, 6. conclusions, supplementary materials, author contributions, institutional review board statement, informed consent statement, data availability statement, acknowledgments, conflicts of interest, abbreviations.
SS | Self-service Supermarket |
SES | Smart E-commerce System |
SFM | Smart Farmers Market |
PDS | Package Delivery System |
SCS | Smart Childcare System |
SPHC | Service Platform for House Cleaning |
SMSWE | Smart Management System of Water and Electricity |
SISPA | Smart Illumination System in Public Area |
SPS1 | Smart Parking System |
SSSPM | Smart Security System of Property Management |
SPS2 | Smart Payment System |
SWB | Smart Waste Bin |
MMSPM | Maintenance Management System of Property Management |
CSEGS | Smart Environmental Greening System |
ESND | Emergency System of Natural Disaster |
ESA | Emergency System of Accident |
ESSSE | Emergency System of Social Security Event |
ESPHE | Emergency System of Public Health Event |
SHSC | Smart Healthcare Service Center |
SMRS | Smart Medical Record System |
TS | Telemedicine System |
SRS | Smart Referral System |
SECF | Smart Elderly Care Facilities |
SEHE | Smart Elderly Health Examination |
OLEC | Online Lectures about Elderly Care |
HRMSE | Health Record Management System of the Elderly |
ASRFMCE | Appointment System of Regular and Free Medical Consultations for the Elderly |
FSE | First-aid Service for the Elderly |
SF | Smart Forum |
SAC | Smart Activity Center |
PC | Psychological Counseling |
DEP | Demand Expression Platform |
GSS | Government Service System |
GMS | Grid Management System |
EP | Employment Platform |
LS | Legal Service |
SBB | Smart Bulletin Board |
PA | Poverty Assistance |
VSS | Volunteer Service System |
- The Smart Community Construction and Operation Guide (2021) Has Been Officially Released. Available online: http://scdrc.sic.gov.cn/News/339/11118.htm (accessed on 8 May 2022). (In Chinese)
- Enayet, A.; Razzaque, M.A.; Hassan, M.M.; Alamri, A.; Fortino, G. A Mobility-Aware Optimal Resource Allocation Architecture for Big Data Task Execution on Mobile Cloud in Smart Cities. IEEE Commun. Mag. 2018 , 56 , 110–117. [ Google Scholar ] [ CrossRef ]
- Yigitcanlar, T.; Kamruzzaman, M.; Buys, L.; Ioppolo, G.; Sabatini-Marques, J.; Da Costa, E.M.; Yun, J.J. Understanding ‘Smart Cities’: Intertwining Development Drivers with Desired Outcomes in a Multidimensional Framework. Cities 2018 , 81 , 145–160. [ Google Scholar ] [ CrossRef ]
- Li, Q.; Yang, J.; Zhan, X. Assessment of social stability risk in the construction of lntelligent community: Based on bow-tie model and bayesian network. J. Shanghai Adm. Inst. 2019 , 20 , 89–99. (In Chinese) [ Google Scholar ]
- Wu, X.; He, R. Actors, interest interaction and integration strategy in the construction of smart community: An exploratory study based on grounded theory. J. Gansu Adm. Inst. 2019 , 28 , 80–94. (In Chinese) [ Google Scholar ]
- Yang, X.; Feng, D. Research hotspot and development trend of smart community informatization construction. Inf. Sci. 2021 , 39 , 187–193. (In Chinese) [ Google Scholar ]
- Planning Was Divorced from Reality and Construction Stalled. How Can Village Planning Avoid Detours. Available online: https://www.163.com/dy/article/EKSIOC8H05149JLH.html (accessed on 13 August 2022). (In Chinese).
- Farahani, B.; Firouzi, F.; Chang, V.; Badaroglu, M.; Constant, N.; Mankodiya, K. Towards Fog-Driven Iot Ehealth: Promises and Challenges of Lot in Medicine and Healthcare. Future Gener. Comput. Syst. 2018 , 78 , 659–676. [ Google Scholar ] [ CrossRef ] [ Green Version ]
- Ni, J.; Zhang, K.; Lin, X.; Shen, X.S. Securing Fog Computing for Internet of Things Applications: Challenges and Solutions. IEEE Commun. Surv. Tutor. 2018 , 20 , 601–628. [ Google Scholar ] [ CrossRef ]
- Pan, J.; McElhannon, J. Future Edge Cloud and Edge Computing for Internet of Things Applications. IEEE Internet Things J. 2018 , 5 , 439–449. [ Google Scholar ] [ CrossRef ]
- Rahmani, A.M.; Gia, T.N.; Negash, B.; Anzanpour, A.; Azimi, I.; Jiang, M.; Liljeberg, P. Exploiting Smart E-Health Gateways at the Edge of Healthcare Internet-of-Things: A Fog Computing Approach. Future Gener. Comput. Syst. 2018 , 78 , 641–658. [ Google Scholar ] [ CrossRef ]
- Yahaya, A.S.; Javaid, N.; Javed, M.U.; Almogren, A.; Radwan, A. Blockchain Based Secure Energy Trading with Mutual Verifiable Fairness in a Smart Community. IEEE Trans. Ind. Inform. 2022 , 18 , 1. [ Google Scholar ] [ CrossRef ]
- Yousefpour, A.; Fung, C.; Tam, N.; Kadiyala, K.; Jalali, F.; Niakanlahiji, A.; Kong, J.; Jue, J.P. All One Needs to Know About Fog Computing and Related Edge Computing Paradigms: A Complete Survey. J. Syst. Archit. 2019 , 98 , 289–330. [ Google Scholar ] [ CrossRef ]
- Yu, W.; Liang, F.; He, X.; Hatcher, W.G.; Lu, C.; Lin, J.; Yang, X. A Survey on the Edge Computing for the Internet of Things. IEEE Access 2018 , 6 , 6900–6919. [ Google Scholar ] [ CrossRef ]
- Zhang, J.; Hu, X.; Ning, Z.; Ngai, E.C.H.; Zhou, L.; Wei, J.; Cheng, J.; Hu, B. Energy-Latency Tradeoff for Energy-Aware Offloading in Mobile Edge Computing Networks. IEEE Internet Things J. 2018 , 5 , 2633–2645. [ Google Scholar ] [ CrossRef ]
- Gao, F. The design of rule of law need coordinate with the governance of smart communities. Frontiers 2020 , 9 , 100–103. (In Chinese) [ Google Scholar ]
- Liu, Q.; Qian, Z.; Huang, D.; Zheng, T. The medicine effect and smart community for triggering unknown innovation. Urban Dev. Stud. 2020 , 27 , 100–108. (In Chinese) [ Google Scholar ]
- Wang, D. The future trend of smart community development: From design-based to life-based. Fujian Trib. 2020 , 40 , 92–102. (In Chinese) [ Google Scholar ]
- Zhang, Y.; Zhao, H.; Yao, G. Research on the driving factors of service brand value of smart communities based on WSR system methodology. Manag. Rev. 2021 , 33 , 174–183. (In Chinese) [ Google Scholar ]
- Song, X.; Wang, Q. Smart community: New relations between subjects and new forms of governance. E-Government 2020 , 17 , 121–128. (In Chinese) [ Google Scholar ]
- Zhu, Y.; Han, Y. The construction and optimization of smart community in China. Leadersh. Sci. 2020 , 36 , 122–124. (In Chinese) [ Google Scholar ]
- Bai, H.; Liu, J.; Ran, X. Research on environmental evaluation of intelligent community based on multi-source big data. J. China Acad. Electron. Inf. Technol. 2021 , 16 , 270–276. (In Chinese) [ Google Scholar ]
- Su, Y.; Zhang, Q. Research on collaborative governance mode of information system of ethnic minority smart community based on the hierarchy of needs theory. Libr. Inf. 2020 , 41 , 86–92. (In Chinese) [ Google Scholar ]
- Van Cutsem, O.; Dac, D.H.; Boudou, P.; Kayal, M. Cooperative Energy Management of a Community of Smart-Buildings: A Blockchain Approach. Int. J. Electr. Power Energy Syst. 2020 , 117 , 105643. [ Google Scholar ] [ CrossRef ]
- Zhou, S.; Zou, F.; Wu, Z.; Gu, W.; Hong, Q.; Booth, C. A Smart Community Energy Management Scheme Considering User Dominated Demand Side Response and P2P Trading. Int. J. Electr. Power Energy Syst. 2020 , 114 , 105378. [ Google Scholar ] [ CrossRef ]
- Gu, T.; Li, D.; Li, L. The Elderly’s Demand for Community-Based Care Services and its Determinants: A Comparison of the Elderly in the Affordable Housing Community and Commercial Housing Community of China. J. Healthc. Eng. 2020 , 2020 , 1840543. [ Google Scholar ] [ CrossRef ] [ PubMed ]
- Liu, X. Construction of healthy elderly care service system from the perspective of smart community elderly care. Chin. J. Gerontol. 2018 , 38 , 1743–1745. (In Chinese) [ Google Scholar ]
- Escarce, J.J.; Wozniak, G.D.; Tsipas, S.; Pane, J.D.; Brotherton, S.E.; Yu, H. Effects of the Affordable Care Act Medicaid Expansion On the Distribution of New General Internists Across States. Med. Care 2021 , 59 , 653–660. [ Google Scholar ] [ CrossRef ] [ PubMed ]
- Shao, H.; Jin, C.; Xu, J.; Zhong, Y.; Xu, B. Supply-Demand Matching of Medical Services at a City Level Under the Background of Hierarchical Diagnosis and Treatment-Based On Didi Chuxing Data in Haikou, China. BMC Health Serv. Res. 2022 , 22 , 3541. [ Google Scholar ] [ CrossRef ]
- Zhang, Y.; Zhang, T.; Zeng, Y.; Yu, C.; Zheng, S. The Rising and Heterogeneous Demand for Urban Green Space by Chinese Urban Residents: Evidence from Beijing. J. Clean Prod. 2021 , 313 , 127781. [ Google Scholar ] [ CrossRef ]
- Xing, L.; Liu, Y.; Liu, X. Measuring Spatial Disparity in Accessibility with a Multi-Mode Method Based On Park Green Spaces Classification in Wuhan, China. Appl. Geogr. 2018 , 94 , 251–261. [ Google Scholar ] [ CrossRef ]
- Wang, X.; Zhang, Z. Model Construction of Urban Public Sports Service System under the Background of Information Technology. Mob. Inf. Syst. 2022 , 2022 , 5207857. [ Google Scholar ] [ CrossRef ]
- Bai, Y.; Song, J.; Wu, S.; Wang, W.; Lo, J.T.Y.; Lo, S.M. Comparing the Impacts of Location Attributes On Residents’ Preferences and Residential Values in Compact Cities: A Case Study of Hong Kong. Sustainability 2020 , 12 , 4867. [ Google Scholar ] [ CrossRef ]
- Cui, L.; Wang, Y.; Chen, W.; Wen, W.; Han, M.S. Predicting Determinants of Consumers’ Purchase Motivation for Electric Vehicles: An Application of Maslow’s Hierarchy of Needs Model. Energy Policy 2021 , 151 , 112167. [ Google Scholar ] [ CrossRef ]
- Shih, C.; Huang, C.; Huang, M.; Chen, C.; Lin, C.; Tang, F. The Association of Sociodemographic Factors and Needs of Haemodialysis Patients According to Maslow’s Hierarchy of Needs. J. Clin. Nurs. 2019 , 28 , 270–278. [ Google Scholar ] [ CrossRef ] [ PubMed ]
- Cheung, C.; Takashima, M.; Choi, H.H.; Yang, H.; Tung, V. The Impact of COVID-19 Pandemic on the Psychological Needs of Tourists: Implications for the Travel and Tourism Industry. J. Travel Tour. Mark. 2021 , 38 , 155–166. [ Google Scholar ] [ CrossRef ]
- Li, H.; Yuan, B.; Wang, D.; Meng, Q. Motivating Factors On Performance of Primary Care Workers in China: A Systematic Review and Meta-Analysis. BMJ Open 2019 , 9 , e2861911. [ Google Scholar ] [ CrossRef ] [ PubMed ] [ Green Version ]
- Qi, L.; Guo, J. Development of Smart City Community Service Integrated Management Platform. Int. J. Distrib. Sens. Netw. 2019 , 15 , 370582068. [ Google Scholar ] [ CrossRef ]
- Li, X.; Lu, R.; Liang, X.; Shen, X.S.; Chen, J.; Lin, X. Smart Community: An Internet of Things Application. IEEE Commun. Mag. 2011 , 49 , 68–75. [ Google Scholar ] [ CrossRef ]
- Mital, M.; Pani, A.K.; Damodaran, S.; Ramesh, R. Cloud Based Management and Control System for Smart Communities: A Practical Case Study. Comput. Ind. 2015 , 74 , 162–172. [ Google Scholar ] [ CrossRef ]
- Mo, L.; Li, C. Passive Uhf-Rfid Localization Based On the Similarity Measurement of Virtual Reference Tags. IEEE Trans. Instrum. Meas. 2019 , 68 , 2926–2933. [ Google Scholar ] [ CrossRef ]
- Sattlegger, L. Making Food Manageable—Packaging as a Code of Practice for Work Practices at the Supermarket. J. Contemp. Ethnogr. 2021 , 50 , 341–367. [ Google Scholar ] [ CrossRef ]
- Nuss, H.; Skizim, M.; Afaneh, H.; Miele, L.; Sothern, M. Farmers’ Market Utilization among Supplemental Nutrition Assistance Program Recipients in New Orleans, Louisiana: Preliminary Findings. Ethn. Dis. 2017 , 271 , 295–302. [ Google Scholar ] [ CrossRef ] [ PubMed ] [ Green Version ]
- Li, R.; Huang, Q.; Chen, X.; Zheng, B.; Liu, H. Factors Affecting Smart Community Service Adoption Intention: Affective Community Commitment and Motivation Theory. Behav. Inf. Technol. 2019 , 38 , 1324–1336. [ Google Scholar ] [ CrossRef ]
- Wang, F.; Zhang, J.; Zhang, P. Influencing Factors of Smart Community Service Quality: Evidence from China. Teh. Vjesn. 2021 , 28 , 1187–1196. [ Google Scholar ]
- Jiang, H. Design and Implementation of Smart Community Big Data Dynamic Analysis Model Based On Logistic Regression Model. Comput. Intell. Neurosci. 2022 , 2022 , 4038084. [ Google Scholar ] [ CrossRef ]
- Pardini, K.; Rodrigues, J.J.P.C.; Diallo, O.; Das, A.K.; de Albuquerque, V.H.C.; Kozlov, S.A. A Smart Waste Management Solution Geared Towards Citizens. Sensors 2020 , 20 , 2380. [ Google Scholar ] [ CrossRef ] [ Green Version ]
- Zhang, Q.; Li, H.; Wan, X.; Skitmore, M.; Sun, H. An Intelligent Waste Removal System for Smarter Communities. Sustainability 2020 , 12 , 6829. [ Google Scholar ] [ CrossRef ]
- Corral-De-Witt, D.; Carrera, E.V.; Munoz-Romero, S.; Luis Rojo-Alvarez, J. Statistical, Spatial and Temporal Mapping of 911 Emergencies in Ecuador. Appl. Sci. 2018 , 8 , 199. [ Google Scholar ] [ CrossRef ] [ Green Version ]
- Ansari, S.; Yoon, S.; Albert, L.A. An Approximate Hypercube Model for Public Service Systems with Co-Located Servers and Multiple Response. Transp. Res. Part E-Logist. Transp. Rev. 2017 , 103 , 143–157. [ Google Scholar ] [ CrossRef ]
- Buitron De La Vega, P.; Losi, S.; Martinez, L.S.; Bovell-Ammon, A.; Garg, A.; James, T.; Ewen, A.M.; Stack, M.; DeCarvalho, H.; Sandel, M.; et al. Implementing an Ehr-Based Screening and Referral System to Address Social Determinants of Health in Primary Care. Med. Care 2019 , 572 , S133–S139. [ Google Scholar ] [ CrossRef ]
- Doshmangir, L.; Bazyar, M.; Majdzadeh, R.; Takian, A. So Near, so Far: Four Decades of Health Policy Reforms in Iran, Achievements and Challenges. Arch. Iran. Med. 2019 , 22 , 592–605. [ Google Scholar ]
- Alabdulatif, A.; Khalil, I.; Forkan, A.R.M.; Atiquzzaman, M. Real-Time Secure Health Surveillance for Smarter Health Communities. IEEE Commun. Mag. 2019 , 57 , 122–129. [ Google Scholar ] [ CrossRef ]
- Sahi, P.K.; Mishra, D.; Singh, T. Medical Education Amid the COVID-19 Pandemic. Indian Pediatr. 2020 , 57 , 652–657. [ Google Scholar ] [ CrossRef ]
- Camargo, C.P.; Tempski, P.Z.; Busnardo, F.F.; Martins, M.D.A.; Gemperli, R. Online Learning and COVID-19: A Meta-Synthesis Analysis. Clinics 2020 , 75 , e2286. [ Google Scholar ] [ CrossRef ] [ PubMed ]
- Iyal, H.A.; Shuaib, F.; Dauda, M.; Suleiman, A.; Braka, F.; Tegegne, S.G.; Nsubuga, P.; Nomhwange, T.; Yehualashet, Y.G.; Ishaku, S.; et al. Assessment of Unmet Needs to Address Noncompliant Households during Polio Supplemental Immunization Activities in Kaduna State, 2014-2016. BMC Public Health 2018 , 184 , 1309. [ Google Scholar ] [ CrossRef ] [ PubMed ] [ Green Version ]
- Higuchi, M.; Endo, M.; Yoshino, A. Factors Associated with Access to Health Care among Foreign Residents Living in Aichi Prefecture, Japan: Secondary Data Analysis. Int. J. Equity Health 2021 , 20 , 1351. [ Google Scholar ] [ CrossRef ] [ PubMed ]
- Zhang, Y.; Zhao, H.; Hu, Y.; Yao, G. Smart Community Service Brand Functional Value and Sustainable Brand Relationship-the Mediating Role of Customer Emotional Cognition. Sustainability 2021 , 13 , 1833. [ Google Scholar ] [ CrossRef ]
- Guo, J.; Ling, W. Impact of Smart City Planning and Construction on Community Governance under Dynamic Game. Complexity 2021 , 2021 , 6690648. [ Google Scholar ] [ CrossRef ]
- Mittelstaedt, J.C. The Grid Management System in Contemporary China: Grass-Roots Governance in Social Surveillance and Service Provision. China Inf. 2022 , 36 , 3–22. [ Google Scholar ] [ CrossRef ]
- Wheeler, L.; Garlick, R.; Johnson, E.; Shaw, P.; Gargano, M. Linkedin(to) Job Opportunities: Experimental Evidence From Job Readiness Training. Am. Econ. J.-Appl. Econ. 2022 , 14 , 101–125. [ Google Scholar ] [ CrossRef ]
- Alarie, B.; Niblett, A.; Yoon, A.H. How Artificial Intelligence Will Affect the Practice of Law. Univ. Tor. Law J. 2018 , 681 , 106–124. [ Google Scholar ] [ CrossRef ]
- Lim, S.H.; Kim, D.J. The Effect of Unmindfulness on Impulse Purchasing Behaviours in the Context of Online Shopping From a Classical Attitude Theory Perspective. Behav. Inf. Technol. 2021 , 16 , 3432–3449. [ Google Scholar ] [ CrossRef ]
- Olya, H.G.T.; Alipour, H.; Peyravi, B.; Dalir, S. Tourism Climate Insurance: Implications and Prospects. Asia Pac. J. Tour. Res. 2019 , 24 , 269–280. [ Google Scholar ] [ CrossRef ]
- Yoo, S.; Ko, Y.J.; Hur, Y.; Bang, H.; Kim, T. Exploring Volunteer Engagement in a Mega Sport Events: The Role of Functional Attitudes. Eur. Sport Manag. Q. 2022 , 1–20. [ Google Scholar ] [ CrossRef ]
- Gu, T.; Yuan, J.; Li, L.; Shao, Q.; Zheng, C. Demand for Community-Based Care Services and its Influencing Factors Among the Elderly in Affordable Housing Communities: A Case Study in Nanjing City. BMC Health Serv. Res. 2020 , 20 , 2411. [ Google Scholar ] [ CrossRef ]
- Liu, T.; Xiao, W. The ‘Intention to Stay’ of the Floating Migrant Population: A Spatiotemporal Meta-Analysis in Chinese Cities. Popul. Space Place 2022 , 28 , e2574. [ Google Scholar ] [ CrossRef ]
- Shang, X.; Huang, Y.; Li, B.; Yang, Q.; Zhao, Y.; Wang, W.; Liu, Y.; Lin, J.; Hu, C.; Qiu, Y. Residents’ Awareness of Family Doctor Contract Services, Status of Contract with a Family Doctor, and Contract Service Needs in Zhejiang Province, China: A Cross-Sectional Study. Int. J. Environ. Res. Public Health 2019 , 16 , 3312. [ Google Scholar ] [ CrossRef ] [ Green Version ]
- Yang, S.; Wang, D.; Li, C.; Wang, C.; Wang, M. Medical Treatment Behaviour of the Elderly Population in Shanghai: Group Features and Influencing Factor Analysis. Int. J. Environ. Res. Public Health 2021 , 18 , 4108. [ Google Scholar ] [ CrossRef ]
- Yu, Z.; Wang, L.; Ariyo, T. Supply and Demand-Related Decisive Factors in the Utilization of Non-Medical Community Healthcare Services Among Elderly Chinese. Int. J. Environ. Res. Public Health 2021 , 18 , 228. [ Google Scholar ] [ CrossRef ]
- Cui, N.; Gu, H.; Shen, T.; Feng, C. The Impact of Micro-Level Influencing Factors on Home Value: A Housing Price-Rent Comparison. Sustainability 2018 , 10 , 4343. [ Google Scholar ] [ CrossRef ] [ Green Version ]
- Zhang, J.; Wang, Z.; Lin, L.; Huang, J.; Dong, Y.; Li, H. Determinants of Preference for Future Living Arrangement in Disabled Elders: A Cross-Sectional Study of Elderly Residents in Kunshan, China. Psychogeriatrics 2022 , 22 , 324–331. [ Google Scholar ] [ CrossRef ]
- Zhang, L.; Tsung, L. Learning Chinese as a Second Language in China: Positive Emotions and Enjoyment. System 2021 , 96 , 102410. [ Google Scholar ] [ CrossRef ]
- Seidler, C.; Rau, T.; Allroggen, M. The Relationship between a Sense of Security and Psychological Problems in Adolescents Living in Boarding Schools and Youth-Welfare Institutions. Z. Kinder Jugendpsychiatr. Psychother. 2018 , 46 , 230–237. [ Google Scholar ] [ CrossRef ] [ PubMed ]
- De Clercq, D.; Kaciak, E.; Thongpapanl, N. Happy at Home, Successful in Competition: The Beneficial Role of Happiness and Entrepreneurial Orientation for Women Entrepreneurs. Int. J. Entrep. Behav. Res. 2022 , 28 , 1463–1488. [ Google Scholar ] [ CrossRef ]
- Ravi, K.E.; Robinson, S.R.; Schrag, R.V. Facilitators of Formal Help-Seeking for Adult Survivors of Ipv in the United States: A Systematic Review. Trauma Violence Abus. 2021 , 23 , 1420–1436. [ Google Scholar ] [ CrossRef ]
- Approval of the Ministry of Civil Affairs on the Recognition of Beijing Shijingshan District and Other Units as the National Community Governance and Service Innovation Experimental Zones. Available online: http://www.mca.gov.cn/article/gk/wj/201904/20190400016136.shtml (accessed on 6 May 2022). (In Chinese)
- “Smart Community 2.0 Platform” Was Officially Launched in Gulou District, Xuzhou City. Available online: http://mzt.jiangsu.gov.cn/art/2020/11/20/art_54981_9581601.html (accessed on 5 May 2022). (In Chinese)
- Notice of the Ministry of Civil Affairs on Confirming the Evaluation Results of the National Community Governance and Service Innovation Experimental Zones. Available online: http://www.mca.gov.cn/article/wh/whbq/jsmlsq/cssqzl/202112/20211200038387.shtml (accessed on 6 May 2022). (In Chinese)
- The “Gulou Path” of Smart Community Governance and Service Innovation in Xuzhou. Available online: http://mz.xz.gov.cn/001/001004/20220127/849d3ed2-b53f-4cfe-aa1f-fba5720498bf.html (accessed on 11 October 2022). (In Chinese)
- Jacquerioz, F.; Baggio, S.; Gayet-Ageron, A.; Chappuis, F.; Getaz, L.; Guessous, I.; Kaiser, L.; Vernaz, N.; Yerly, S.; Salamun, J.; et al. Development and Validation of the Outcov Score to Predict the Risk of Hospitalisation Among Patients with SARS-CoV-2 Infection in Ambulatory Settings: A Prospective Cohort Study. BMJ Open 2021 , 11 , e44242. [ Google Scholar ] [ CrossRef ]
- Courvoisier, D.S.; Combescure, C.; Agoritsas, T.; Gayet-Ageron, A.; Perneger, T.V. Performance of Logistic Regression Modeling: Beyond the Number of Events per Variable, the Role of Data Structure. J. Clin. Epidemiol. 2011 , 64 , 993–1000. [ Google Scholar ] [ CrossRef ]
- Vittinghoff, E.; McCulloch, C.E. Relaxing the Rule of Ten Events Per Variable in Logistic and Cox Regression. Am. J. Epidemiol. 2007 , 165 , 710–718. [ Google Scholar ] [ CrossRef ] [ Green Version ]
- Jeon, K.J.; Lee, C.; Choi, Y.J.; Han, S. Analysis of Three-Dimensional Imaging Findings and Clinical Symptoms in Patients with Temporomandibular Joint Disorders. Quant. Imaging Med. Surg. 2021 , 11 , 1921–1931. [ Google Scholar ] [ CrossRef ]
- Saha, A.; Beasley, M.; Hatton, N.; Dickinson, P.; Franks, K.; Clarke, K.; Jain, P.; Teo, M.; Murray, P.; Lilley, J. Clinical and Dosimetric Predictors of Radiation Pneumonitis in Early-Stage Lung Cancer Treated with Stereotactic Ablative Radiotherapy (Sabr)—An Analysis of Uk’s Largest Cohort of Lung Sabr Patients. Radiother. Oncol. 2021 , 156 , 153–159. [ Google Scholar ] [ CrossRef ]
- Gao, Y.; Li, T.; Han, M.; Li, X.; Wu, D.; Xu, Y.; Zhu, Y.; Liu, Y.; Wang, X.; Wang, L. Diagnostic Utility of Clinical Laboratory Data Determinations for Patients with the Severe COVID-19. J. Med. Virol. 2020 , 92 , 791–796. [ Google Scholar ] [ CrossRef ]
- Haenssle, H.A.; Fink, C.; Schneiderbauer, R.; Toberer, F.; Buhl, T.; Blum, A.; Kalloo, A.; Hassens, A.B.H.; Thomas, L.; Enk, A.; et al. Man Against Machine: Diagnostic Performance of a Deep Learning Convolutional Neural Network for Dermoscopic Melanoma Recognition in Comparison to 58 Dermatologists. Ann. Oncol. 2018 , 29 , 1836–1842. [ Google Scholar ] [ CrossRef ]
- Xu, J.; Wu, L.; Jiang, W.; Fan, G. Effect of Nursing Intervention Based On Maslow’s Hierarchy of Needs in Patients with Coronary Heart Disease Interventional Surgery. World J. Clin. Cases 2021 , 9 , 10189–10197. [ Google Scholar ] [ CrossRef ]
- Wang, T.; Chen, W.; Kang, Y.; Lin, C.; Cheng, C.; Suk, F.; Chen, H.; Hsu, C.; Fong, T.; Huang, W. Why Do Pre-Clinical Medical Students Learn Ultrasound? Exploring Learning Motivation through Erg Theory. BMC Med. Educ. 2021 , 21 , 4381. [ Google Scholar ] [ CrossRef ]
- Troisi, R.; De Simone, S.; Vargas, M.; Franco, M. The Other Side of the Crisis: Organizational Flexibility in Balancing COVID-19 and Non-COVID-19 Health-Care Services. BMC Health Serv. Res. 2022 , 22 , 1096. [ Google Scholar ] [ CrossRef ]
- Wake, A.D. The Willingness to Receive COVID-19 Vaccine and its Associated Factors: "Vaccination Refusal Could Prolong the War of this Pandemic" a Systematic Review. Risk Manag. Healthc. Policy 2021 , 14 , 2609–2623. [ Google Scholar ] [ CrossRef ]
- Liu, M.; Hu, L.; Guo, R.; Wang, H.; Cao, M.; Chen, X.; Liu, Y. The Influence of Patient and Hospital Characteristics on Inpatient Satisfaction at Beijing District-Level Hospitals. Patient Prefer. Adherence 2021 , 15 , 1451–1460. [ Google Scholar ] [ CrossRef ] [ PubMed ]
- Deng, Q.; Zhang, S.; Deng, Y.; Liu, F.; Shi, W.; Xie, H.; Xiao, Y.; Huang, Y.; Li, J. Willingness-to-Pay and Benefit-Cost Analysis of Ipl for Rosacea Treatment: A Cross-Sectional Study in China. Patient Prefer. Adherence 2020 , 14 , 1843–1852. [ Google Scholar ] [ CrossRef ] [ PubMed ]
- Hounsome, N.; Kassahun, M.M.; Ngari, M.; Berkley, J.A.; Kivaya, E.; Njuguna, P.; Fegan, G.; Tamiru, A.; Kelemework, A.; Amberbir, T.; et al. Cost-Effectiveness and Social Outcomes of a Community-Based Treatment for Podoconiosis Lymphoedema in the East Gojjam Zone, Ethiopia. PLoS Negl. Trop. Dis. 2019 , 13 , e778010. [ Google Scholar ] [ CrossRef ] [ PubMed ] [ Green Version ]
- Yang, T.; Lan, C.E. Impacts of Skin Disorders Associated with Facial Discoloration On Quality of Life: Novel Insights Explaining Discordance Between Life Quality Scores and Willingness to Pay. J. Cosmet. Dermatol. 2022 , 21 , 3053–3058. [ Google Scholar ] [ CrossRef ] [ PubMed ]
- Goeb, J.; Dillon, A.; Lupi, F.; Tschirley, D. Pesticides: What You Don’t Know Can Hurt You. J. Assoc. Environ. Resour. Econ. 2020 , 7 , 801–836. [ Google Scholar ] [ CrossRef ]
- Zheng, Z.; Henneberry, S.R.; Sun, C.; Nayga, R.M., Jr. Consumer Demand for Genetically Modified Rice in Urban China. J. Agric. Econ. 2018 , 69 , 705–725. [ Google Scholar ] [ CrossRef ]
- Ryan-Pettes, S.R.; Lange, L.L.; Magnuson, K.I. Mobile Phone Access and Preference for Technology-Assisted Aftercare among Low-Income Caregivers of Teens Enrolled in Outpatient Substance Use Treatment: Questionnaire Study. Jmir Mhealth Uhealth 2019 , 7 , e124079. [ Google Scholar ] [ CrossRef ] [ PubMed ]
- Jang, J.; Ko, J. Factors Associated with Commuter Satisfaction Across Travel Time Ranges. Transp. Res. Part F-Traffic Psychol. Behav. 2019 , 66 , 393–405. [ Google Scholar ] [ CrossRef ]
- Mion, S.; Duval, B.; Besnard, T.; Darne, B.; Mouton, C.; Jecker, O.; Labrousse, L.; Remy, A.; Zaouter, C.; Ouattara, A. U-Shaped Relationship Between Pre-Operative Plasma Fibrinogen Levels and Severe Peri-Operative Bleeding in Cardiac Surgery. Eur. J. Anaesth. 2020 , 37 , 889–897. [ Google Scholar ] [ CrossRef ] [ PubMed ]
Click here to enlarge figure
Category | Type | Researchers |
---|---|---|
Smart business service | 1. Self-service Supermarket (SS) | [ , ] |
2. Smart E-commerce System (SES) | [ ] | |
3. Smart Farmers Market (SFM) | [ ] | |
4. Package Delivery System (PDS) | [ ] | |
5. Smart Childcare System (SCS) | [ ] | |
6. Service Platform for House Cleaning (SPHC) | [ , ] | |
Smart property service | 1. Smart Management System of Water and Electricity (SMSWE) | [ , ] |
2. Smart Illumination System in Public Area (SISPA) | [ ] | |
3. Smart Parking System (SPS1) | [ , ] | |
4. Smart Security System of Property Management (SSSPM) | [ , , , ] | |
5. Smart Payment System (SPS2) | [ , ] | |
6. Smart Waste Bin (SWB) | [ , ] | |
7. Maintenance Management System of Property Management (CMMSPM) | [ ] | |
8. Smart Environmental Greening System (SEGS) | [ , , ] | |
Smart emergency service | 1. Emergency System of Natural Disaster (ESND) | [ , , , ] |
2. Emergency System of Accident (ESA) | [ , , ] | |
3. Emergency System of Social Security Event (ESSSE) | [ , ] | |
4. Emergency System of Public Health Event (ESPHE) | [ ] | |
Smart medical care service | 1. Smart Healthcare Service Center (SHSC) | [ ] |
2. Smart Medical Record System (SMRS) | [ ] | |
3. Telemedicine System (TS) | [ ] | |
4. Smart Referral System (SRS) | [ , ] | |
Smart elderly care service | 1. Smart Elderly Care Facilities (SECF) | [ , ] |
2. Smart Elderly Health Examination (SEHE) | [ ] | |
3. Online Lectures about Elderly Care (OLEC) | [ , ] | |
4. Health Record Management System of the Elderly (HRMSE) | [ ] | |
5. Appointment System of Regular and Free Medical Consultations for the Elderly (ASRFMCE) | [ , ] | |
6. First-aid Service for the Elderly (FSE) | [ ] | |
Smart communication service | 1. Smart Forum (SF) | [ , , ] |
2. Smart Activity Center (SAC) | [ , , ] | |
3. Psychological Counseling (PC) | [ ] | |
Smart government service | 1. Demand Expression Platform (DEP) | [ , ] |
2. Government Service System (GSS) | [ ] | |
3. Grid Management System (GMS) | [ ] | |
4. Employment Platform (EP) | [ ] | |
5. Legal Service (LS) | [ ] | |
6. Smart Bulletin Board (SBB) | [ , ] | |
7. Poverty Assistance (PA) | [ ] | |
8. Volunteer Service System (VSS) | [ ] |
Category | Type | Researchers |
---|---|---|
Sociodemographic characteristics | 1. Gender | [ , , ] |
2. Age | [ , , ] | |
3. Career | [ ] | |
4. Educational level | [ , , , , ] | |
5. Marital status | [ , ] | |
6. Health status | [ , , , ] | |
Living characteristics | 1. Living duration | [ ] |
2. Living status | [ , ] | |
3. Housing choice | [ ] | |
Economic characteristics | 1. Monthly income | [ , , , , ] |
2. Whether paying social insurance | [ , ] | |
Individual attitude characteristics | 1. Sense of gain | [ ] |
2. Sense of safety | [ ] | |
3. Sense of happiness | [ ] | |
4. Perception of community services | [ ] | |
5. Desire for smart community services | [ ] |
Category | Type | Option | Frequency | Percentage (N = 221) |
---|---|---|---|---|
Sociodemographic characteristics | Gender | Male | 109 | 49.32% |
Female | 112 | 50.68% | ||
Age | 17 years old and below | 13 | 5.88% | |
18–35 years old | 109 | 49.32% | ||
36–45 years old | 41 | 18.55% | ||
46–69 years old | 58 | 26.24% | ||
70 years old and above | 0 | 0.00% | ||
Career | Civil servant | 14 | 6.33% | |
Staff of state-owned enterprises and institutions | 62 | 28.05% | ||
Staff of private and foreign enterprises and institutions | 56 | 25.34% | ||
Individual industrial and commercial household | 15 | 6.79% | ||
Freelancer | 21 | 9.50% | ||
Student | 41 | 18.55% | ||
Other | 12 | 5.43% | ||
Educational level | Primary school or below | 8 | 3.62% | |
Middle school | 12 | 5.43% | ||
High school and technical secondary school | 34 | 15.38% | ||
Junior college | 39 | 17.65% | ||
Bachelor’s degree | 99 | 44.80% | ||
Master’s degree or above | 29 | 13.12% | ||
Marital status | Married | 157 | 71.04% | |
Unmarried | 64 | 28.96% | ||
Health status | Good | 195 | 88.24% | |
General | 25 | 11.31% | ||
Bad | 1 | 0.45% | ||
Living characteristics | Living duration | Less than 1 year | 23 | 10.41% |
1 to 3 years | 44 | 19.91% | ||
More than 3 years | 154 | 69.68% | ||
Living status | Living alone | 14 | 6.33% | |
Not living alone | 207 | 93.67% | ||
Housing choice | Renter | 28 | 12.67% | |
House owner | 171 | 77.38% | ||
Other | 22 | 9.95% | ||
Economic characteristics | Monthly income | Within 1000 RMB (about 146.69 USD) | 25 | 11.31% |
1000–3000 RMB (about 146.69–440.09 USD) | 39 | 17.65% | ||
3000–5000 RMB (about 440.09–733.54 USD) | 43 | 19.46% | ||
5000–7000 RMB (about 733.54–1026.92 USD) | 48 | 21.72% | ||
Above 7000 RMB (about 1026.92 USD) | 66 | 29.86% | ||
Whether paying social insurance | All | 147 | 66.52% | |
Partly (e.g., only medical insurance) | 50 | 22.62% | ||
Not at all | 24 | 10.86% | ||
Individual attitude characteristics | Sense of gain | Mean score of sense of gain | 3.95 | |
Sense of safety | Mean score of sense of safety | 4.09 | ||
Sense of happiness | Mean score of sense of happiness | 3.97 | ||
Perception of community services | Mean score of perception of community services | 3.37 | ||
Desire for smart community services | Mean score of desire for smart community services | 4.31 |
The statements, opinions and data contained in all publications are solely those of the individual author(s) and contributor(s) and not of MDPI and/or the editor(s). MDPI and/or the editor(s) disclaim responsibility for any injury to people or property resulting from any ideas, methods, instructions or products referred to in the content. |
Share and Cite
Chen, J.; Wang, L.; Gu, T.; Wang, C.; Hao, E. Demands for Community Services and Associated Factors among Residents in Smart Communities: A Case Study of Xuzhou City. Int. J. Environ. Res. Public Health 2023 , 20 , 3750. https://doi.org/10.3390/ijerph20043750
Chen J, Wang L, Gu T, Wang C, Hao E. Demands for Community Services and Associated Factors among Residents in Smart Communities: A Case Study of Xuzhou City. International Journal of Environmental Research and Public Health . 2023; 20(4):3750. https://doi.org/10.3390/ijerph20043750
Chen, Jiongxun, Linxiu Wang, Tiantian Gu, Chenyang Wang, and Enyang Hao. 2023. "Demands for Community Services and Associated Factors among Residents in Smart Communities: A Case Study of Xuzhou City" International Journal of Environmental Research and Public Health 20, no. 4: 3750. https://doi.org/10.3390/ijerph20043750
Article Metrics
Article access statistics, supplementary material.
ZIP-Document (ZIP, 781 KiB)
Further Information
Mdpi initiatives, follow mdpi.
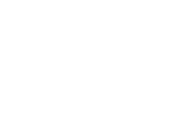
Subscribe to receive issue release notifications and newsletters from MDPI journals

An official website of the United States government
The .gov means it’s official. Federal government websites often end in .gov or .mil. Before sharing sensitive information, make sure you’re on a federal government site.
The site is secure. The https:// ensures that you are connecting to the official website and that any information you provide is encrypted and transmitted securely.
- Publications
- Account settings
- My Bibliography
- Collections
- Citation manager
Save citation to file
Email citation, add to collections.
- Create a new collection
- Add to an existing collection
Add to My Bibliography
Your saved search, create a file for external citation management software, your rss feed.
- Search in PubMed
- Search in NLM Catalog
- Add to Search
Demands for Community Services and Associated Factors among Residents in Smart Communities: A Case Study of Xuzhou City
Affiliation.
- 1 School of Mechanics and Civil Engineering, China University of Mining and Technology, Xuzhou 221116, China.
- PMID: 36834442
- PMCID: PMC9964050
- DOI: 10.3390/ijerph20043750
Smart community enables a sustainable and livable community future, in which residents' demands play an important role in its success. Though great efforts have been made to encourage residents' participation in the implementation of smart communities, inefficient service supply still exists. Thus, this study aimed to classify residents' demands for community services in smart communities and to explore relevant influencing factors based on the developed conceptual framework. Data from 221 respondents in Xuzhou city of China were analyzed by using binary logistic regression. The results indicated that more than 70% of respondents had demands for all community services in smart communities. Moreover, the demands were influenced by distinct factors, including sociodemographic characteristics, living characteristics, economic characteristics, and individual attitude characteristics. The types of community services in smart communities are clarified and fresh insights are provided into associated factors related to residents' demands for these services in this study, through which enhanced provision of community services and effective implementation of smart communities can be achieved.
Keywords: binary logistic regression; influencing factors; residents’ demands; smart community.
PubMed Disclaimer
Conflict of interest statement
The authors declare no conflict of interest.
The conceptual framework for this…
The conceptual framework for this research.
Respondents’ expressed demands for 39…
Respondents’ expressed demands for 39 types of community services in smart communities.
A combination of the ROC…
A combination of the ROC curves for initial demands and prediction performance. Note:…
Similar articles
- Community Services and Social Involvement in COVID-19 Governance: Evidence from China. Ding J, Xu J, Weise T, Wang H. Ding J, et al. Int J Environ Res Public Health. 2022 Nov 18;19(22):15279. doi: 10.3390/ijerph192215279. Int J Environ Res Public Health. 2022. PMID: 36429997 Free PMC article.
- Demand for community-based care services and its influencing factors among the elderly in affordable housing communities: a case study in Nanjing City. Gu T, Yuan J, Li L, Shao Q, Zheng C. Gu T, et al. BMC Health Serv Res. 2020 Mar 23;20(1):241. doi: 10.1186/s12913-020-5067-0. BMC Health Serv Res. 2020. PMID: 32293427 Free PMC article.
- Residents' Awareness of Family Doctor Contract Services, Status of Contract with a Family Doctor, and Contract Service Needs in Zhejiang Province, China: A Cross-Sectional Study. Shang X, Huang Y, Li B, Yang Q, Zhao Y, Wang W, Liu Y, Lin J, Hu C, Qiu Y. Shang X, et al. Int J Environ Res Public Health. 2019 Sep 9;16(18):3312. doi: 10.3390/ijerph16183312. Int J Environ Res Public Health. 2019. PMID: 31505783 Free PMC article.
- Defining the concepts of a smart nursing home and its potential technology utilities that integrate medical services and are acceptable to stakeholders: a scoping review. Zhao Y, Rokhani FZ, Sazlina SG, Devaraj NK, Su J, Chew BH. Zhao Y, et al. BMC Geriatr. 2022 Oct 7;22(1):787. doi: 10.1186/s12877-022-03424-6. BMC Geriatr. 2022. PMID: 36207705 Free PMC article. Review.
- Applying community resilience theory to engagement with residents facing cumulative environmental exposure risks: lessons from Louisiana's industrial corridor. Reams MA, Irving JK. Reams MA, et al. Rev Environ Health. 2019 Sep 25;34(3):235-244. doi: 10.1515/reveh-2019-0022. Rev Environ Health. 2019. PMID: 31473690 Review.
- The Smart Community Construction and Operation Guide (2021) Has Been Officially Released. [(accessed on 8 May 2022)]; Available online: http://scdrc.sic.gov.cn/News/339/11118.htm . (In Chinese)
- Enayet A., Razzaque M.A., Hassan M.M., Alamri A., Fortino G. A Mobility-Aware Optimal Resource Allocation Architecture for Big Data Task Execution on Mobile Cloud in Smart Cities. IEEE Commun. Mag. 2018;56:110–117. doi: 10.1109/MCOM.2018.1700293. - DOI
- Yigitcanlar T., Kamruzzaman M., Buys L., Ioppolo G., Sabatini-Marques J., Da Costa E.M., Yun J.J. Understanding ‘Smart Cities’: Intertwining Development Drivers with Desired Outcomes in a Multidimensional Framework. Cities. 2018;81:145–160. doi: 10.1016/j.cities.2018.04.003. - DOI
- Li Q., Yang J., Zhan X. Assessment of social stability risk in the construction of lntelligent community: Based on bow-tie model and bayesian network. J. Shanghai Adm. Inst. 2019;20:89–99. (In Chinese)
- Wu X., He R. Actors, interest interaction and integration strategy in the construction of smart community: An exploratory study based on grounded theory. J. Gansu Adm. Inst. 2019;28:80–94. (In Chinese)
Publication types
- Search in MeSH
Grants and funding
Linkout - more resources, full text sources.
- Europe PubMed Central
- PubMed Central
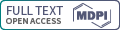
- Citation Manager
NCBI Literature Resources
MeSH PMC Bookshelf Disclaimer
The PubMed wordmark and PubMed logo are registered trademarks of the U.S. Department of Health and Human Services (HHS). Unauthorized use of these marks is strictly prohibited.

An official website of the United States government
The .gov means it’s official. Federal government websites often end in .gov or .mil. Before sharing sensitive information, make sure you’re on a federal government site.
The site is secure. The https:// ensures that you are connecting to the official website and that any information you provide is encrypted and transmitted securely.
- Publications
- Account settings
Preview improvements coming to the PMC website in October 2024. Learn More or Try it out now .
- Advanced Search
- Journal List
- HHS Author Manuscripts

Community-Based Supports and Services for Older Adults: A Primer for Clinicians
Eugenia l. siegler.
1 Division of Geriatrics and Palliative Medicine, Weill Cornell Medical College, New York, NY 10065, USA
Sonam D. Lama
Michael g. knight.
2 Department of Medicine, New York Presbyterian Hospital, Weill Cornell Campus, New York, NY 10065, USA
Evelyn Laureano
3 Neighborhood Self Help by Older Persons Project (SHOPP), Bronx, NY 10459, USA
M. Carrington Reid
Although 20% of adults 60 years and older receive community-based supports and services (CBSS), clinicians may have little more than a vague awareness of what is available and which services may benefit their patients. As health care shifts toward more creative and holistic models of care, there are opportunities for CBSS staff and primary care clinicians to collaborate toward the goal of maintaining patients’ health and enabling them to remain safely in the community. This primer reviews the half-century history of these organizations in the United States, describes the most commonly used services, and explains how to access them.
1. Introduction
Community-based supports and services (CBSS) are designed to help community-dwelling older adults remain safely in their homes and delay or prevent institutionalization. CBSS provide (and act as a link to) specific resources for older adults and their caregivers that include wellness programs, nutritional support, educational programs about health and aging, and counseling services for caregivers, as well as general assistance with housing, finances, and home safety. CBSS also provide opportunities for community and civic engagement through various volunteer programs [ 1 ] and can enhance individuals’ skills and attitudes “to live in and gain more control over local aspects of their communities” [ 2 ].
More than 20% of older adults (i.e., those aged 60 and above) currently receive CBSS [ 3 ]. Older adults who use these services need them: over 90% of service users have multiple chronic conditions [ 4 ] and corresponding activity of daily living (ADL) deficits [ 5 ]. With the rapid aging of our population, even as overall health improves the number of older adults who could benefit from CBSS is expected to increase significantly in the coming years [ 6 ].
A recent nationwide survey of community-dwelling older adults found that a substantial majority were very interested in receiving information about CBSS [ 7 ]. However, respondents often did not know the range of services provided or where (or how) to access them. Survey respondents viewed health care providers as one of their major sources for information about CBSS and were less likely to contact community-based agencies directly [ 7 ]. Many older adults and caregivers feel most comfortable discussing health and social issues with their health care provider. As such, health care providers are ideally positioned to educate older patients and their caregivers about CBSS and to refer them for services and supports when appropriate.
There is little information in the literature about health care providers’ knowledge of and referral patterns to agencies providing CBSS. One Canadian study published almost 25 years ago found that physicians lacked basic information about these services; almost half (47%) acknowledged that lack of information contributed to their failure to refer patients for CBSS [ 8 ]. Although we did not identify any recent studies on this topic, we suspect that most health care providers still lack basic knowledge about the types of services provided by these agencies, which types of patients are eligible to receive them, and how to refer older patients (and/or caregivers) for services when appropriate.
This paper seeks to address this gap by (1) briefly describing the history of and funding sources for agencies providing CBSS; (2) defining the specific types of CBSS available and describing several types of agencies that provide them; (3) defining who is eligible to receive these services; and finally (4) providing practical tips about how to access CBSS. For the purposes of this paper, we define an agency providing CBSS as one that delivers services (e.g., home delivered meals) or programs (e.g., chronic disease management classes at senior centers) in a community-based setting. We exclude certified home health services (such as visiting nurse or home physical therapy) and state Medicaid waiver programs to focus the discussion on individual organizations less familiar to clinicians.
2. A Brief History of Agencies Providing Community-Based Supports and Services and Their Funding Sources
Although clinicians ultimately must look locally to find out what their patients need and which CBSS are available to help address that need, the origins of nationally supported CBSS begin in Washington. Locally run agencies providing CBSS owe their growth to a federal infrastructure that has enabled and supported them through funding for administration, services, and demonstration projects.
Federal funds were first allocated for social service programs targeting older adults in 1952 [ 9 ]. More than a decade later, the passage of the Older Americans Act (OAA) in 1965—the same year Medicare and Medicaid were established—created the formal framework for large-scale federal support of agencies providing CBSS. The OAA established the Administration on Aging (AoA) and mandated creation of state Agencies on Aging to promote delivery of social services to older Americans [ 9 ].
Currently, the AoA is one of the units of the Administration for Community Living in the Department of Health and Human Services. The AoA is divided into five offices: (1) Supportive and Caregiver Services; (2) Nutritional and Health Promotion Programs; (3) Elder Rights; (4) American Indian, Alaskan Native and Native Hawaiian Programs; and (5) Long-Term Care Ombudsman Programs [ 10 ]. The national aging services network through which the AoA promotes home and community-based services consists of 56 state (and territorial) units on aging, 629 area agencies on aging (AAA), 256 Native American and Native Hawaiian organizations, together with the tens of thousands of direct service providers and volunteers [ 3 ].
The OAA funds services under several different titles. Title III, which accounts for nearly three-quarters of the AoA’s budget (1.2 billion dollars in 2012) [ 11 ], funds the State Units on Aging and AAAs. The target population consists of individuals aged 60 and over. Although there is no means testing, 30% of those receiving Title III services in fiscal year 2010 had an income below the federal poverty line [ 11 ]. People who participate in OAA programs also often need and receive non-Title III services, as well. For example 29% of those who receive home delivered meals also receive Medicaid; 22% of those who receive homemaker services also receive energy assistance [ 12 ].
Federal dollars only partially fund AAAs and the organizations they support, and a community organization’s funding is often quite precarious. Only 57% of senior centers received OAA funding in fiscal year 2012 [ 3 ]. New York City’s AAA, the NYC Department for the Aging, is the largest in the country with a proposed budget of over $262,000,000 for 2014–2015. Approximately 29% of this amount is expected to come from federal funds, with the remaining amount coming from state (14%) and municipal (57%) sources [ 13 ]. Thus, while the OAA has established an infrastructure to oversee, plan, and fund CBSS for older Americans, its budget is a fraction of what is needed to pay for all of the services provided by these agencies. The survival of the CBSS network depends on a combination of national, state, and local government support along with private contributions, business support, and other philanthropy.
3. Examples of Services and Supports Provided by Community-Based Organizations
Community-based organizations provide a broad range of programs for older adults and caregivers. Most health care providers are familiar with nutrition, homemaker, and transportation services as well as senior centers but many other services are available, including legal assistance and case management services for clients and counseling and respite services for caregivers. Table 1 lists the primary CBSS available for use by older adults and their caregivers; although broad in scope, the amount of services any one individual receives is often quite limited and not a substitute for formal or family caregiving.
Specific community-based supports and services.
Client services | Description |
---|---|
Home delivered meals | Meals delivered to the home of those who cannot prepare or obtain adequate nutrition |
Congregate meals | Meals served in a community setting to those who cannot prepare or obtain adequate nutrition |
Transportation | Includes subsidized mass transit, curb-to-curb paratransit and other assisted transportation, and driver education |
Personal care | Hands-on or cueing to assist individuals with ADLs or IADLs |
Homemaker services | Services designed to maintain a healthy home environment such as housekeeping, meal preparation, laundry, and shopping |
Information and assistance | Used to help individuals or their representatives identify, access, and use support services (exclusive of case management) |
Nutrition education and counseling | Assessment of and assistance in meeting of an individual’s nutritional needs by a licensed nutritionist or dietician |
Adult day care | Community-based program offering social, recreational, and health-related services in congregate setting |
Case management | Professional management of an individual’s health care; identification and assessment of biopsychosocial needs; monitoring use of services to ensure positive outcomes |
Outreach | To inform and educate the public of the availability of services, benefits, and programs |
Chore | Household tasks such as heavy cleaning and yard work |
Legal assistance | Consultation and representation for consumer issues, housing, benefits, etc. |
Caregiver services | Description |
Respite | Can involve adult day care, in-home or brief periods out of home in a nursing home or assisted living facility |
Access assistance | Assistance to caregivers to gain access to AOA programs |
Counseling, support group, training | Miscellaneous: individual counseling; caregiver support groups; training in caregiving skills |
Supplemental services | Extra services provided on a short term basis |
From Administration on Aging: FY 2012 Report to Congress [ 3 ]. Each column is listed in descending order based on units of service.
The following section summarizes information about four commonly used community-based agencies that provide these services.
3.1. Nutrition Service Programs
Although often colloquially thought of as “Meals on Wheels,” subsidized nutrition encompasses a far broader range of services. Elder nutrition services constitute the largest OAA program [ 14 ]; total federal expenditures for the three main programs (Congregate Nutrition Services, Home Delivered Nutrition Service, and Nutrition Services Incentive Program) totaled $816,289,000 in fiscal year 2012. Despite this significant federal outlay, Title III support provides only 35% of funding for these meals; the rest of the funding comes from state and local government and philanthropic and private sources [ 3 ].
These Title III programs were designed not just to relieve “food insecurity” but also to promote socialization and physical health and well-being [ 14 ]. Socialization occurs in the setting of congregate meals that are served in the community through senior centers, day health programs, and other venues.
Nutritional service programs are directed toward those with significant impairments. A 2009 survey of recipients determined that 41% of those receiving congregate meals and 63% of those receiving home delivered meals had 6 or more chronic conditions. In this same study, 9% of those receiving congregate meals and 31% of those receiving home delivered meals reported at least 3 ADL limitations [ 4 ]. The effectiveness of nutrition support programs has been studied; data suggest that home delivered meals can reduce nursing home admissions [ 15 , 16 ].
3.2. Senior Centers
The first senior center (William Hodson) opened in 1943 in New York City. Over the past 70 years, senior centers have proliferated nationwide (totaling 10,000 in FY 2012 [ 3 ]) and now serve as “community focal points” and gateways to health, educational, social, and recreational services for as many as 1 million older adults every day [ 17 ].
Although there is a general sense that senior centers improve physical and mental health, this is not well investigated. Many studies examining the effectiveness of senior centers have been cross-sectional or had methodological weaknesses [ 18 ]; a few controlled trials have examined specific interventions (e.g., exercise, education) delivered at senior centers and suggested improved outcomes [ 18 , 19 ].
Over the past decade participation in senior centers has declined, especially for the younger, healthier segments of the older population [ 19 ], giving rise to a movement to create more flexible and responsive models that will attract a broader range of individuals and be able to meet a diversity of needs. Pardasani and Tompson [ 20 ] have investigated and classified innovative models into six types, reflecting foci on greater age diversity, health promotion, and intellectual stimulation:
- community centers for all ages,
- wellness centers for active adults over 50,
- lifelong learning/arts centers for adults over 50,
- continuum of care/transitions for older people to age in place,
- entrepreneurial centers focusing in employment and productivity,
- café programs for adults 50 years and over that mix age groups and provide a community space for meals, education, and entertainment.
Although locating a convenient senior center is an important first step, it is important to determine if choices are available, and if so, which senior center could most closely serve a patient’s needs.
3.3. Adult Day Services Centers
Adult day services (ADS) centers provide coordinated services in a community setting. There are three types: social, medical/health, and specialized (e.g., providing programs for demented individuals) [ 21 ]. ADS use is growing. As of 2010, there were 4,601 ADS centers in the USA, 98% of which were open Monday–Friday. Two-thirds of participants attend at least 3 days/week [ 22 ]. Three-fourths of these programs offer medication management for mental health disorders [ 23 ].
As with other CBSS, it is difficult to measure effectiveness in the absence of randomized controlled trials. Some studies have failed to demonstrate clearly positive outcomes [ 24 , 25 ], but others suggest that these programs may enhance quality of life and reduce stress [ 26 , 27 ].
3.4. Naturally Occurring Retirement Communities: An Example of a Creative Solution to a Demographic Challenge
Formally known as Naturally Occurring Retirement Community-Supportive Service Program (NORC-SSP), this model of care is geographically based rather than service based. The first NORC was created in 1986 at a housing development (Penn South Houses) in New York City to support a group of the elderly who had aged in place but required a support system to enable them to continue to live independently in the community [ 28 ]. The development partnered with a local social service agency (United Jewish Appeal Federation of New York) to establish the services necessary to convert what was an apartment complex into housing that could meet the needs of those in declining health. NORCs are public-private partnerships and receive support both from local agencies and the federal government, via Title IV of the OAA [ 29 ].
There are approximately 100 NORCs, half in New York and the rest scattered throughout the USA [ 30 ]. NORCs are formal organizations, with paid staff and volunteers who provide services including socialization, care coordination, and transportation, in addition to expedited referrals to other community services such as home health, nutrition, or legal services [ 29 ]. A newer alternative, known as Villages, is membership-driven and privately funded, originating most often in areas of greater wealth [ 30 ]. Another option is independent senior housing programs that employ service coordinators who link residents to CBSS. Coordinators’ positions can be funded locally or federally [ 31 ].
4. Locating and Determining Eligibility for Services and Supports
Navigating CBSS can be challenging. Eligibility for CBSS benefits depends upon a host of factors that are individual and agency/service-related. OAA requires only that clients be 60 and over; locally funded programs may require means testing. A brochure “You Gave, Now Save” published by the National Council on Aging lists the basic services and general information about eligibility and access: http// www.ncoa.org/assets/files/pdf/center-for-benefits/You-Gave-Now-Save-Guide-to-Benefits.pdf . Online, the Benefits Checkup ( https://www.benefitscheckup.org/ ) helps a patient or caregiver determine eligibility for services and benefits by entering personal information about needs, assets, and expenditures.
The services themselves can be located via local or national sites. Nationally, the AoA sponsors the Eldercare Locator, which is accessible via the web or phone. States or regions may have their own government information phone number or website offering assistance. Information about how to access CBSS is shown below.
Accessing Community-Based Services
- by zip code,
- by service,
- toll-free number: 1-800-677-1116.
- Family caregiver alliance navigator: https://caregiver.org/family-care-navigator .
- National Association of Area Agencies on Aging: http://n4a.membershipsoftware.org/content.asp?contentid=146 ,
- Administration on Aging’s AAA finder: http://www.aoa.gov/AoA_programs/OAA/How_To_Find/Agencies/find_agencies.aspx ,
- search at the state level: office or department of aging (which will usually list the AAAs by county),
- search by county (e.g., “Area Agency on Aging, Cayuga County, New York”).
- search globally (rather than just “meals on wheels”) for home delivered or congregate meals or nutrition assistance,
- counseling and other forms of nutrition support may be available,
- these will usually be called senior center but they may be listed as a subcategory under community resources or congregate meals,
- example 3: adult day services centers: http://nadsa.org/consumers/choosing-a-center/ ,
- example 4: naturally occurring retirement communities: https://www.norcs.org/ .
A newer model of agency, already available in 52 states/territories (as of 2013, 70% of the population was covered), is the Aging and Disability Resource Center [ 32 ]. These centers are programs jointly managed by the Administration for Community Living and Centers for Medicare and Medicaid Services (and in some cases, the Department of Veterans Affairs) to expedite and simplify access to long-term care services and hence the motto: “No wrong door.” The programs offer assistance with care transitions in order to help individuals avoid long-term institutionalization; they also help people access benefits such as Medicaid [ 33 ].
5. Counseling Patients and Caregivers Who Would Benefit from Community-Based Supports and Services
CBSS are underutilized by older adults and caregivers for several reasons, including a lack of awareness, reluctance, unavailability, and unaffordability [ 34 ]. Clinicians can address the first two of these barriers directly; social work services are occasionally necessary to help patients gain access to services that may substitute for those that are not local or require payment.
Even when services and programs are available, older patients and caregivers sometimes refuse them. They may lack experience in accessing services or have difficulty accepting that they need them [ 35 ]. They may resist congregating with “old people” or feel that services are not sensitive to their ethnic group. They may resent subjecting themselves to unnecessary requirements or loss of control; they may feel judged or may feel services are not specific to their needs [ 36 ]. It may be useful to anticipate these attitudinal barriers and provide evidence for the usefulness of local programs.
Assessing a patient’s faith community may also help the clinician when thinking about options for community support. Religious institutions are commonly a well-trusted component of affiliated seniors’ lives, especially in ethnic minorities where a level of mistrust of medical institutions can influence their receptiveness to medical senior/social services. Older adults are more likely to be affiliated with religious congregations and attend services (67–69% above age 65), with an even greater percentage participating in ethnic minority communities [ 37 ]. Many of these congregations have some sort of senior outreach, ranging from home visitations to more formalized programs.
Finally, visiting CBSS programs and meeting the staff can be invaluable for the clinician to provide personal experience and anecdote to go along with the generic advice. Local programs generally welcome the opportunity to have clinicians come in to do presentations on specific topics related to health; both the clinician and the CBSS can establish a mutually beneficial collaborative relationship.
6. Conclusion
Clinicians should develop familiarity with CBSS and the agencies that provide them. Knowledge of and coordination with CBSS are essential if clinicians are to create more flexible and responsive models of care (e.g., medical homes) for their older patients [ 38 , 39 ]. Services and supports provided by these agencies can be a critical link in helping older adults remain in the community.
Acknowledgments
The paper was supported by a grant from the John A. Hartford Foundation and an Edward R. Roybal Center for Translational Research on Aging Award (P30AG22845).
Conflict of Interests
Dr. M. Carrington Reid has been a consultant for Endo Pharmaceuticals. Dr. Eugenia L. Siegler receives royalties from Springer Publishing Company. There is not any other potential conflict of interests reported by the authors.
Authors’ Contribution
Thank you for visiting nature.com. You are using a browser version with limited support for CSS. To obtain the best experience, we recommend you use a more up to date browser (or turn off compatibility mode in Internet Explorer). In the meantime, to ensure continued support, we are displaying the site without styles and JavaScript.
- View all journals
- Explore content
- About the journal
- Publish with us
- Sign up for alerts
- Open access
- Published: 04 September 2024
Predicting anxiety treatment outcome in community mental health services using linked health administrative data
- Kevin E. K. Chai 1 ,
- Kyran Graham-Schmidt 2 ,
- Crystal M. Y. Lee 1 ,
- Daniel Rock 3 , 4 , 5 ,
- Mathew Coleman 6 ,
- Kim S. Betts 1 ,
- Suzanne Robinson 1 , 7 &
- Peter M. McEvoy 1 , 8
Scientific Reports volume 14 , Article number: 20559 ( 2024 ) Cite this article
Metrics details
- Health care
Machine learning
- Psychiatric disorders
Anxiety disorders is ranked as the most common class of mental illness disorders globally, affecting hundreds of millions of people and significantly impacting daily life. Developing reliable predictive models for anxiety treatment outcomes holds immense potential to help guide the development of personalised care, optimise resource allocation and improve patient outcomes. This research investigates whether community mental health treatment for anxiety disorder is associated with reliable changes in Kessler psychological distress scale (K10) scores and whether pre-treatment K10 scores and past health service interactions can accurately predict reliable change (improvement). The K10 assessment was administered to 46,938 public patients in a community setting within the Western Australia dataset in 2005–2022; of whom 3794 in 4067 episodes of care were reassessed at least twice for anxiety disorders, obsessive–compulsive disorder, or reaction to severe stress and adjustment disorders (ICD-10 codes F40–F43). Reliable change on the K10 was calculated and used with the post-treatment score as the outcome variables. Machine learning models were developed using features from a large health service administrative linked dataset that includes the pre-treatment K10 assessment as well as community mental health episodes of care, emergency department presentations, and inpatient admissions for prediction. The classification model achieved an area under the receiver operating characteristic curve of 0.76 as well as an F1 score, precision and recall of 0.69, and the regression model achieved an R 2 of 0.37 with mean absolute error of 5.58 on the test dataset. While the prediction models achieved moderate performance, they also underscore the necessity for regular patient monitoring and the collection of more clinically relevant and contextual patient data to further improve prediction of treatment outcomes.
Similar content being viewed by others
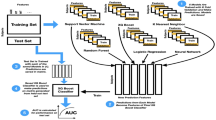
Predictive modeling of depression and anxiety using electronic health records and a novel machine learning approach with artificial intelligence
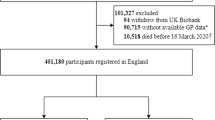
Trends in incident diagnoses and drug prescriptions for anxiety and depression during the COVID-19 pandemic: an 18-month follow-up study based on the UK Biobank
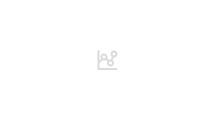
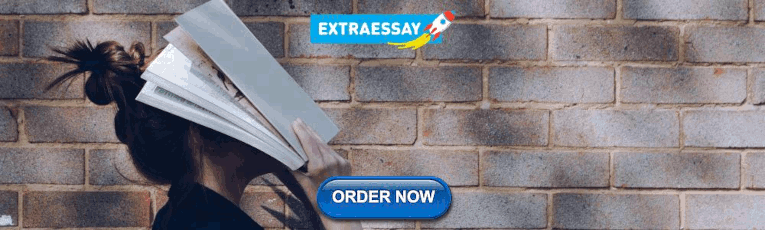
A nationwide cross-sectional study on the association of patient-level factors with financial anxiety in the context of chronic medical conditions
Introduction.
Anxiety disorders are the most common class of mental illness in Australia, affecting 3.4 million adults aged 16 years and older or 17.2% of the population in 2020–2022 1 . Similarly in the United States, anxiety disorders are also the most common estimated to affect 30.6% of the population aged 18 years and older in 2020–2022 2 . These disorders are characterized by excessive worry, fear, and nervousness that can interfere with daily life. There are several different types of anxiety disorders, including generalized anxiety disorder, panic disorder, social anxiety disorder, and specific phobias. Historically, obsessive compulsive disorder and fear and stressor-related disorders (e.g., posttraumatic stress disorder) were considered anxiety disorders in the Diagnostic and Statistical Manual of Mental Disorders (DSM-IV, American Psychiatric Association, APA, 1994) although more recent nosologies consider them separate but related classes of disorders (DSM-5, APA, 2013). Within the International Classification of Diseases (ICD version 10, 2019; ICD version 11, 2023), these disorders are three categories within the mental, behavioural or neurodevelopmental disorders.
Primary care is the main source of treatment for anxiety disorders and, where required, providers more commonly refer patients to private specialist services than to public services 3 . Nonetheless, community mental health services remain important for patients who cannot afford or access private providers 4 . Public services refer to government funded and operated specialised mental health care provided by community and hospital based ambulatory care services, such as outpatient and day clinics 5 and offer a variety of ongoing treatment options including psychotherapy, medication, and support groups. A continuing challenge for clinicians and services in all settings is to predict how well an individual will respond to treatment. There are many factors that can influence outcomes, such as the severity of the disorder, the patient's readiness for change, the quality of the treatment they receive, and external factors that reflect the overall complexity of human lives (e.g., relationship breakdown, financial hardship, workplace redundancy, bereavement) 5 , 6 , 7 .
Being able to accurately predict patient outcomes would be beneficial 7 , 8 , 9 , 10 . First, it would allow clinicians to tailor treatment plans to the individual needs of each patient, for example, by targeting known risk factors for disengagement or poor clinical outcomes. This could improve patient outcomes and reduce the need for patients to try multiple standardised treatments before finding one that works. Second, it would allow clinical planners in mental health services to allocate resources more effectively. For example, services could focus on providing more intensive treatment to patients who are at high risk of deterioration. Third, it could help identify patients who are unlikely to respond to treatment and may need additional support.
Promising methods for predicting patient outcomes for anxiety disorders and other mental illnesses include clinical prediction tools, patient-reported outcome measures, and machine learning 9 , 10 , 11 . These methods are commonly based on predictors such as patient demographics, clinical symptoms, treatment history, from different modes of data such as electronic health records, biometrics, and radiology and machine learning techniques such as logistic regression, random forests, support vector machines, gradient boosting and neural networks on datasets comprising of 4184 undergraduate students 9 and 1249 participants from a mental healthcare provider 11 .
Research on the prediction of treatment outcomes in mental health show that it is difficult, either because treatment outcomes genuinely do not vary based on individual differences or due to a range of methodological limitations, such as investigations of variables based on convenience rather than strong theory; the lack of consideration of the complex interplay between relational and content components of psychotherapy; low statistical power due to studies being designed to evaluate main effects of treatments rather than moderators of symptom change; overly homogenous samples due to exclusion criteria in randomised trials; over-reliance on significance testing without due consideration to effect sizes; failure to probe interactions to understand patterns of effects; and neglecting non-linear relationships within the context of complex relationships for humans in the real world 8 , 12 .
The alternative of relying on clinician intuition is also fraught. The biases clinicians bring to predicting psychotherapy outcomes have been long known 13 , 14 , 15 . Researchers have recently suggested that machine learning approaches that use large databases, theory-informed parameters and include complex relationships with multiple predictors of responder status, could address many of these issues 8 , 16 , 17 . Models that explain patterns in historical data and predict future outcomes, would hold promise for informing and improving the quality of care for people with anxiety disorders.
The aims of this study were to (a) investigate associations between demographic, treatment, and clinical variables and changes in psychological distress while patients were engaged with community mental health services and (b) develop machine learning models to predict reliable change in Kessler (K10) psychological distress scores using a patient’s pre-treatment (K10) scores within a community mental health setting and their past health service interactions for anxiety disorders. No previous research has used a large sample of demographic, clinical, and treatment service data administratively collected within community mental health services over a 17-year period to predict changes in psychological distress using machine learning models.
Study population
This study was approved by the Department of Health Western Australia Human Research Ethics Committee (approval number: RGS0000004782) and the Curtin University Human Research Ethics Committee (approval number: HRE2022-0001) with a waiver of informed consent obtained from the Department of Health Western Australia Human Research Ethics Committee. All methods in this study were performed in accordance with the relevant guidelines and regulations.
The study cohort was collated from a linked mental health dataset provided by the Department of Health Western Australia which is described elsewhere 18 . The linked dataset is comprised of records related to mental health assessments, community mental health service usage, emergency department presentations and inpatient admissions from 2005–2022.
For this study, we restricted the dataset to records from community mental health services where an anxiety disorder (International Statistical Classification of Diseases and Related Health Problems, Tenth Revision, Australian Modification (ICD): F40–F43) 19 was recorded at any time in the episode of care and to episodes of care with at least two assessments (pre and post treatment ≥ 2 weeks and ≤ 4 months apart) for determining the outcome of the treatment. Based on community mental health dataset collection rules, assessments are not to be reported for brief community interventions (< 2 weeks) and that assessments should be completed at least every three months (we adjusted to 4 months to allow delays and scheduling issues). Data is included from eligible patient episodes of care, with the first pre/post assessment used for each individual episode. Allowing multiple care episodes per patient better represents real-world conditions, providing a more accurate evaluation of the predictive model’s performance on each patient encounter. We conducted a sensitivity analysis comparing the use of single and multiple episodes of care in Supplementary Discussion 1 . ICD-10 was used as 99% of records in the community mental health data collection period within the study population used this classification.
The dataset preparation steps for defining the study population (Table 1 ) and the number of records from each anxiety disorder ICD-10 code (Table 2 ) are presented below.
Primary outcome measure
The K10 assessment is a self-reported measure of anxiety and depression symptoms characteristic of the broad construct of psychological distress 20 . It comprises of 10 questions about emotional states assessed on a five-level response scale (1 = none of the time, 2 = a little of the time, 3 = some of the time, 4 = most of the time, 5 = all of the time). The responses from the 10 questions can be summed to a total ranging from 10 to 50, where lower scores represent lower levels of distress. The K10 has high internal reliability (Cronbach's alpha = 0.93) 21 , distinguishes people with and without anxiety disorders 22 , and has been shown to be highly sensitive to change during psychotherapy 23 . We calculated Cronbach’s alpha for each ICD-10 code in our dataset using the Pingouin Python statistical package 24 .
Data analysis plan
Treatment outcome.
The treatment outcome and its effectiveness were determined by subtracting the post-treatment score from the pre-treatment score. Given that changes in scores reflect true change plus measurement error, Jacobson and Traux proposed the Reliable Change Index (RCI) to evaluate the effectiveness of therapies and interventions based on pre/post treatment scores 25 . The RCI estimates the magnitude of change in a measure’s observed score required before assuming that true change has occurred (i.e., not attributable to measurement error). The RCI is calculated by dividing the difference between the two scores by the standard error of the difference. RCI values ≥ 1.96 represent reliable improvement, RCI values ≤ 1.96 represent reliable deterioration and RCI values between − 1.96 and 1.96 represent no reliable change. The K10 was used as both a continuous outcome variable (post-treatment score) and to classify individuals with respect to whether they reliably improved, deteriorated, or remained unchanged between pre-treatment and post-treatment. The calculation of the RCI and subsequent analysis were conducted using Python 3.9.
The dataset of the study population was prepared with the prediction model features restricted to data from the K10 pre-assessment and previous community mental health episodes of care, in addition to emergency department and inpatient mental health service events (Fig. 1 ).
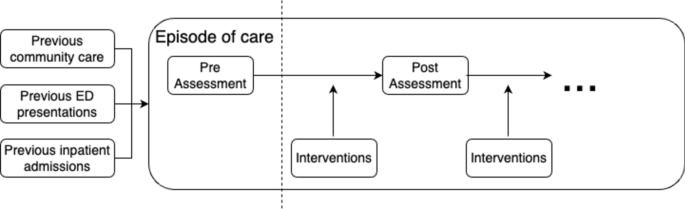
The data sources and features that are available for the prediction model at pre-assessment are depicted to the left of the dashed line. The first pre/post assessment is used for each episode of care and patients may have multiple eligible episodes of care in the dataset. ED emergency department.
The features extracted and created from these data sources are presented in Table 3 with definitions provided in Supplementary Table 1 . The dataset is split into a 70%/30% training and test set using fivefold random subsampling stratified cross validation in machine learning experiments.
Classification and regression models are used to predict the reliable change category (deterioration/no reliable change vs. reliable improvement) and post treatment score as a continuous variable, respectively. Models were trained using the Python scikit-learn library 26 . Training (70%) and testing (30%) datasets were created using a stratified fivefold repeated random sub-sampling cross-validation method.
Model selection
PyCaret 27 , an automated machine learning (AutoML) software library, was used to initially experiment with several machine learning algorithms by splitting only the training dataset into 70/30% using fivefold random sampling cross validation. These initial results will be used to select the most suitable classification and regression methods for subsequent experiments.
Model evaluation
The classification models are evaluated using the Receiver Operating Characteristic (ROC) Area Under the Curve (AUC), precision, recall, F1 score (harmonic mean of precision and recall) and a confusion matrix to identify how often a model gets predictions right (true positives/negatives) and wrong (false positives/negatives) for each reliable change category. An AUC of 1 is considered to have perfect predictive power while an AUC 0.5 suggests no predictive power beyond random chance 28 . The regression models are evaluated using predicted R squared (R 2 ) and the mean absolute error 29 . The predicted post-treatment scores from the regression model were also used to classify episodes of care into the reliable change categories for evaluation.
Feature importance and selection
Shapley Additive Explanations (SHAP) is a game theory inspired technique commonly used to explain the importance and contribution of features in prediction modelling 30 , 31 . It is a model agnostic approach applied to both classification and regression models in our experiments using the SHAP Python library 31 . Furthermore, a greedy forward feature selection method 32 was applied, which involved sequentially adding the feature that provides the largest contribution to the model until a pre-defined stopping criterion was met. The stopping criteria used in experiments for classification were F1 improvement > 0.01 and mean absolute error (MAE) improvement < 0.001 for regression.
The distribution of score changes between pre/post-treatment is shown in Fig. 2 . 2882 (71%) episodes of care showed a reduction in K10 score after treatment, 872 (21%) exhibited an increase in K10 after treatment and 313 (8%) remained unchanged.
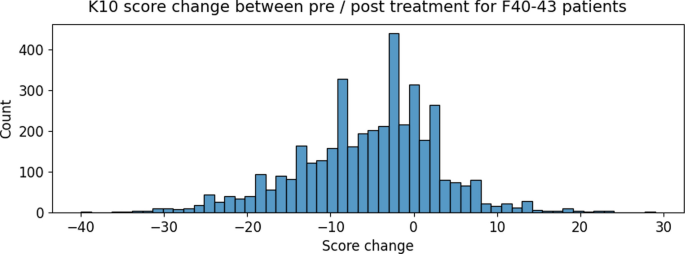
The difference (score change) between pre/post treatment Kessler psychological distress scale (K10) total scores.
The RCI method was applied on the dataset, where K10 reliability coefficients (Cronbach's alphas) of 0.92–0.94 were calculated for each of the ICD codes. The pattern of reliable change for F43 (Reaction to severe stress, and adjustment disorders) is illustrated in Fig. 3 . These boundaries vary for other ICD codes (F40, F41, F42) as the reliable change index was calculated and applied separately for each diagnosis (Supplementary Fig. 1 ).
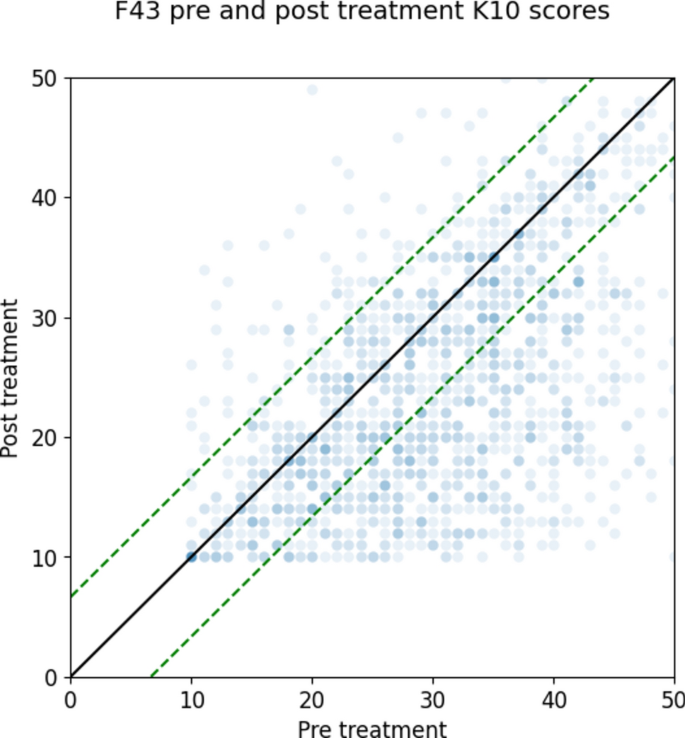
Pre/post treatment scores for F43: Reaction to severe stress, and adjustment disorders. The dashed green lines represent the boundaries of the reliable change index, with the area to the left representing reliable deterioration and the area to the right representing reliable improvement. The area between the green lines represents no reliable change.
Descriptive statistics for the dataset are reported in Table 4 . Altogether, 4067 episodes of care were available for analysis that comprised predominately of females (67%) and a mean (SD) age of 40.2 (17.9) years. The deteriorated reliable change category had low representation (212 records or 5%) and was merged with the no reliable change category (total of 2446 records or 60%) for machine learning experiments.
The machine learning results are presented in two sections (a) classification for predicting the reliable change category and (b) regression for predicting post-assessment scores.
Classification
PyCaret (AutoML) was used to initially experiment with several classification models on the training dataset using cross-validation as presented in Table 5 . Gradient boosting achieved the highest AUC (0.72) and F1 score (0.57). All the models outperform the baseline classifier (AUC = 0.5) that predicts all records as the majority class (deteriorated/no reliable change). Based on these results, gradient boosting was selected for subsequent experiments.
The gradient boosting model was run on both the train and test datasets achieving an average F1 score of 0.66 (0.66–0.69) over fivefold cross validation, with the best model achieving an AUC of 0.77 and F1 of 0.69 (Table 6 ).
The confusion matrix and ROC of the best model is presented in Fig. 4 . The confusion matrix highlighted that the model performed better in classifying episodes of care with deterioration/no reliable change (551 out of 734 (75%) correctly classified) than those that demonstrated reliable improvement (306 out of 487 (63%) correct).
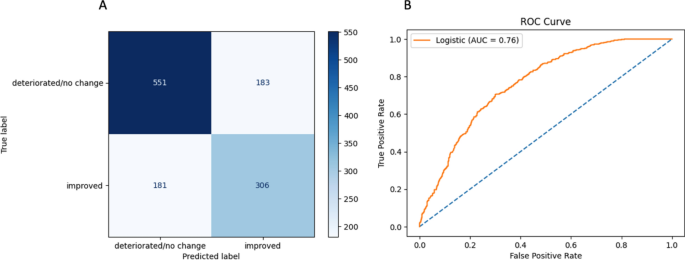
( A ) Classification confusion matrix shows how often the model correctly predicted each class (true positives/negatives) and how often it made mistakes (false positives/negatives). ( B ) The receiver operating characteristic curve on the test dataset shows the sensitivity and specificity at different thresholds for prediction.
The top 20 features based on the SHAP values and feature selection results are shown in Supplementary Table 2 and Supplementary Fig. 2 . The top 2 features from both methods were the pre-assessment score and the collection stage (review). Only using the pre-assessment score achieved a 0.62 F1 score with the admission collection stage increasing the prediction performance to 0.66 and years since the previous emergency contact to 0.69. The additional 4 selected features only improve the model performance to 0.70 (+ 0.1 F1 score).
AutoML was applied to experiment with several regression models on the training dataset using cross-validation as presented in Table 7 . Gradient boosting achieved the top performance with a 0.33 R 2 and 5.82 MAE. All models, except for decision tree, outperformed the baseline regressor that predicts the mean post-treatment score for all records. The gradient boosting model was selected for subsequent experiments.
The gradient boosting model achieved an average MAE of 5.73 (5.58–5.83) over fivefold cross validation with the best model achieving an R 2 of 0.39, 0.37 and MAE values of 5.65, 5.58 on the train and test dataset, respectively (Table 8 ).
The top 20 features based on the absolute SHAP values and feature selection results are shown in Supplementary Table 3 and Supplementary Fig. 3 . Feature selection identified the pre-assessment score and the collection stage (admission) as the top features achieving a 5.75 and 5.59 MAE. The other 5 selected features only reduced the MAE to 5.52 (− 0.07).
Regression applied classification
The regression model predicted the post-assessment score and was used to classify episodes of care into reliable change. The regression applied classification results (Table 9 ) showed a decline when compared to the classification model with an F1 score of 0.69 vs. 0.67 on the test set. The AUC cannot be computed for comparison as the regression model does not generate classification probabilities.
The confusion matrix of the regression applied classification is shown in Fig. 5 . These results when compared to the classification model showed that the regression model performed poorer in predicting improved reliable change (306 vs. 304), and deterioration/no reliable change (551 vs. 533).
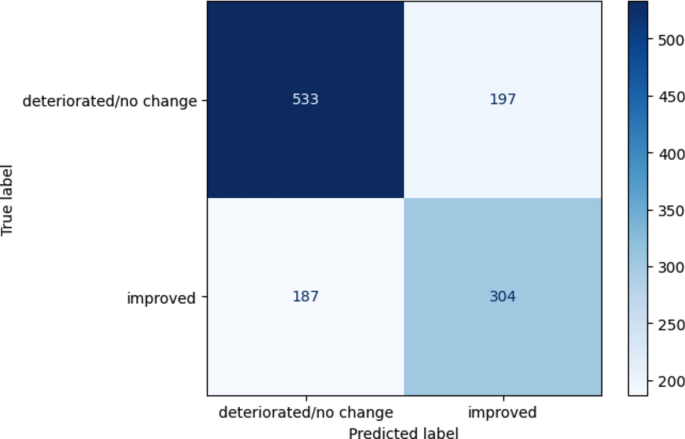
Regression applied classification confusion matrix shows how often the model correctly predicted each class (true positives/negatives) and how often it made mistakes (false positives/negatives).
This study aimed to investigate whether community mental health treatment is related to improvements in psychological distress and develop machine learning models for predicting reliable change and post-treatment scores in anxiety disorder treatments. The discussion will now assess whether the results and findings adequately achieved these aims.
Prediction performance
The classification model achieved an AUC of 0.76 on the test dataset of 1193 patients and an AUC between 0.75 and 0.90 indicates a moderate score in psychology and human behavioural research 33 , 34 . Our results are similar to a study that achieved an AUC of 0.73 on a test dataset of 1255 undergraduate students 9 and outperformed another study that achieved an AUC of 0.60 on 279 patients in their test dataset 11 . The regression model achieved a R 2 on the test dataset and a R 2 between 0.3 and 0.5 is generally considered a weak effect 35 but can be considered as moderate in the context of human behavioural and psychology research 36 . Furthermore, A MAE of 5.58 for the regression model could be interpreted as a relatively large error for downstream tasks such as using the predicted post-treatment scores to classify reliable change. The classification and regression applied classification model achieved similar performance and both outperformed the baseline models. The moderate performance indicates that the models could be further improved with more data and/or better discriminating features. However, there is likely to be an upper limit on prediction performance given the inherent complexity of human lives in predicting the outcome of patient treatments (i.e. Bayes error) 29 .
Classification and regression
The classification model generated probabilities for each class, which helped identify appropriate classification thresholds using the ROC and AUC evaluation metrics. However, a strength of the regression model is that it predicted the post-treatment score, which allowed for the use of classification systems such as reliable change and could potentially be used for other metrics of recovery. Furthermore, the SHAP values of the regression model were easier to interpret as a higher SHAP value indicated a higher predicted post-assessment score (poorer outcomes) compared to classification where a higher SHAP value represents as a lower post-assessment score (improved reliable change). For example, a high pre-assessment score (poor outcome) for classification resulted in the model predicting towards reliable improvement, possibly due to higher pre-assessment scores having more potential to change by post-assessment (i.e. lower scores experiencing a floor effect). However, for regression, a high pre-assessment score (poor outcome) would predict towards high post-assessment scores (poor outcomes).
Model features
The SHAP analysis and feature selection experiments showed that the pre-assessment score was the most important feature, with the assessment collection stage (admission, review) improving prediction with the remaining features providing only a minor contribution to the overall performance. However, a strength of having fewer contributing features is that the model is simpler to implement and translate into clinical software. These top features were, however, not particularly helpful for future treatment-matching, although the challenge of discovering robust predictors of mental health treatment outcomes is well known 8 , 12 . A shift from capturing predominantly health service activity data to capturing more clinically relevant data (e.g., therapeutic process, treatments delivered) along with contextual factors (i.e., non-therapy factors such as life stressors), and implementing more regular patient outcome monitoring 37 to more readily identify when a clinical intervention is not working and could be adapted or stopped, may be required to improve prediction. A cardiologist would not contemplate diagnosing and evaluating interventions for heart disease from single datapoints three months apart, and yet mental health services are expected to do so.
Clinically relevant data
While the study dataset can be seen as a strength (i.e. linked population dataset collected over a 17-year period for training and evaluating prediction models) it is still limited and can be further enhanced. The collection of administrative patient data is often driven by compliance and reporting requirements rather than a clear understanding of its clinical utility. This can lead to the accumulation of vast amounts of data that are difficult to analyse and interpret, providing limited insights into patient care and outcomes. Moreover, the focus on compliance can divert resources away from efforts to collect and curate data that is directly relevant to clinical decision-making while burdening clinicians with onerous data entry administrative tasks. For instance, measures of key individual differences theorised to play a critical role in the aetiology and maintenance of anxiety disorders, such as anxiety sensitivity 38 , intolerance of uncertainty 39 , and experiential avoidance 40 , may help with case formulation, treatment planning, and outcome monitoring. The degree to which interventions successfully modify these factors would be expected to determine downstream impacts on symptom change across the anxiety disorders. Patients’ satisfaction and engagement with the service (e.g., attendance frequency and duration), relational factors between the clinician and patient (e.g., working alliance 41 ), and social determinants (e.g., interpersonal supports and stressors, financial stressors, adverse childhood experiences 42 , 43 ) may also help focus clinicians’ and consumers’ attention on factors likely to have the largest impact on mental health and wellbeing and thereby improve outcomes and their prediction. Outcomes beyond symptom change that capture broader intervention impacts (e.g., quality of life), or monitoring progress on idiographic presenting problems (those specific and of highest priority to the individual), may be particularly valued by consumers 44 , although there is evidence that improvements in quality of life are largely mediated by symptom change 45 . Routine monitoring of known predictors of mental health and wellbeing would facilitate outcome evaluation and benchmarking, whereby novel interventions and service models can be compared over time to previous benchmarks. Without these data, services have no way of knowing if outcomes are worsening, maintaining, or improving over time, which would help with treatment planning. There is evidence that regular and routine outcome monitoring (e.g., session-by-session) that is used collaboratively by consumers and clinicians can improve outcomes, decrease negative outcomes for consumers at risk of not benefiting from treatment, and increase cost-effectiveness of interventions 46 . Future research incorporating and documenting these measures and processes would likely produce more robust and informative predictive models.
The inability of the prediction models to produce higher or more robust performance might suggest that the health administrative data being collected and made available for research lacks clinical relevance, which makes its collection and use difficult to justify. The resources invested in collecting and storing this data could be better utilised towards initiatives that directly improve patient care. Moreover, relying on data that fails to provide meaningful insights could lead to misguided policy decisions and interventions that may not produce the desired outcomes. Administrative data collected solely for service utilisation and planning metrics are insufficient for evaluating quality of care, identifying impacts of service innovations, and ensuring consumer outcomes improve over time. If the priority is maximising patient recovery, then infrastructure (e.g., digital platforms) and measures that routinely, regularly and effectively capture consumer-driven priorities are required to ensure interventions are on track for positive outcomes, or, if not, can be, collaboratively and rapidly responded to by the consumer and healthcare worker to process back on track.
Clinical assessment
A limitation of the model and experiments are features provided by clinicians in their assessments of the patients such as unstructured clinical notes. While these features could aid in prediction, it is noteworthy to highlight that it is also difficult for clinicians to predict, based only from the initial pre-assessment, whether a patient will drop out, be treatment resistant or improve. If this cannot be predicted accurately and reliably by clinical experts 13 , 14 , 15 , then it may be no different when developing and using predictive models. Future research including a combination of clinician, consumer, and administrative data may improve predictive models.
Predicting patient outcomes in mental health is a complex and difficult task but is essential for improving the quality of care for people with anxiety disorders. Research on the prediction of patient outcomes is ongoing and the preliminary findings to date are promising. This study developed classification and regression models that showed moderate prediction performance with features that would be relatively easy to collect and implement in health services organisations and clinics on a linked health administrative dataset collected over a 17-year period. Future research using regular patient outcome monitoring, clinical assessment, consumer and administrative data, may yield more accurate and reliable models for predicting patient outcomes. This will have a significant impact on the lives of people with anxiety disorders and will inform healthcare policy planning.
Data availability
The data that support the findings of this study are available from Government of Western Australia Department of Health ( https://www.datalinkage-wa.org.au/ ) but restrictions apply to the availability of these data, which were used under license for the current study, and so are not publicly available. The corresponding author can provide clarification of the dataset used for the study but for access to the data, contact the Western Australia Department of Health at [email protected].
National Study of Mental Health and Wellbeing. https://www.abs.gov.au/statistics/health/mental-health/national-study-mental-health-and-wellbeing/latest-release (2023).
Villaume, S. C., Chen, S. & Adam, E. K. Age disparities in prevalence of anxiety and depression among US adults during the COVID-19 pandemic. JAMA Netw. Open 6 (11), e2345073 (2023).
Article Google Scholar
Australian Institute of Health and Welfare. Medicare-subsidised mental health-specific services . https://www.aihw.gov.au/mental-health/topic-areas/medicare-subsidised-services (2023).
Castillo, E. G. et al. Community interventions to promote mental health and social equity. Curr. Psychiatry Rep. 21 , 1–14. https://doi.org/10.1007/11920-019-1017-0 (2019).
Australian institute of Health and Welfare. Community Services—Mental health AIHW . https://www.aihw.gov.au/mental-health/topic-areas/community-services (2023).
McMahon, F. J. Prediction of treatment outcomes in psychiatry—Where do we stand?. Dialogues Clin. Neurosci. 16 (4), 455–464 (2014).
Article PubMed PubMed Central Google Scholar
Chekroud, A. M. et al. The promise of machine learning in predicting treatment outcomes in psychiatry. World Psychiatry 20 (2), 154–170 (2021).
Eilertsen, S. E. H. & Eilertsen, T. H. Why is it so hard to identify (consistent) predictors of treatment outcome in psychotherapy? Clinical and research perspectives. BMC Psychol. 11 (1), 198 (2023).
Nemesure, M. D., Heinz, M. V., Huang, R. & Jacobson, N. C. Predictive modeling of depression and anxiety using electronic health records and a novel machine learning approach with artificial intelligence. Sci. Rep. 11 (1), 1980 (2021).
Article CAS PubMed PubMed Central Google Scholar
Stanojevic, M., Norris, L. A., Kendall, P. C. & Obradovic, Z. Predicting anxiety treatment outcomes with machine learning. In 2022 21st IEEE International Conference on Machine Learning and Applications ( ICMLA ) 957–962 (IEEE, 2022).
Hornstein, S., Forman-Hoffman, V., Nazander, A., Ranta, K. & Hilbert, K. Predicting therapy outcome in a digital mental health intervention for depression and anxiety: A machine learning approach. Digit. Health 7 , 20552076211060659 (2021).
PubMed PubMed Central Google Scholar
Erceg-Hurn, D. M., Campbell, B. N. & McEvoy, P. M. What explains the failure to identify replicable moderators of symptom change in social anxiety disorder?. J. Anxiety Disord. 94 , 102676 (2023).
Article PubMed Google Scholar
Meehl, P. E. Clinical versus statistical prediction: A theoretical analysis and a review of the evidence (1954).
Dawes, R. M., Faust, D. & Meehl, P. E. Clinical versus actuarial judgment. Science 243 (4899), 1668–1674 (1989).
Article ADS CAS PubMed Google Scholar
Lilienfeld, S. O., Ritschel, L. A., Lynn, S. J., Cautin, R. L. & Latzman, R. D. Why ineffective psychotherapies appear to work: A taxonomy of causes of spurious therapeutic effectiveness. Perspect. Psychol. Sci. 9 (4), 355–387 (2014).
Mululo, S. C. C., Menezes, G. B. D., Vigne, P. & Fontenelle, L. F. A review on predictors of treatment outcome in social anxiety disorder. Braz. J. Psychiatry 34 , 92–100 (2012).
Ang, Y. S. & Pizzagalli, D. A. Predictors of treatment outcome in adolescent depression. Curr. Treat. Options Psychiatry 8 , 18–28 (2021).
Lee, C. M. Y. et al. Patterns of mental service utilisation: A population-based linkage of over 17 years of health administrative records. Community Ment. Health J. https://doi.org/10.1007/s10597-024-01300-8 (2024).
National Centre for Classification in Health. The International Statistical Classification of Diseases and Related Health Problems, Australian Modification (ICD-10-AM) 10th edn. (Independent Hospital Pricing Authority, 2017).
Google Scholar
Kessler, R. C. M. D. & Mroczek, D. An Update of the Development of Mental Health Screening Scales for the US National Health Interview Stud y (University of Michigan, Survey Research Center of the Institute for Social Research, 1992).
Kessler, R. C. et al. Screening for serious mental illness in the general population. Arch. Gen. Psychiatry 60 (2), 184–189 (2003).
Andrews, G. & Slade, T. Interpreting scores on the Kessler Psychological Distress Scale (K10). Aust. N. Z. J. Public Health 25 , 494–497 (2001).
Article CAS PubMed Google Scholar
McEvoy, P. M. et al. Group metacognitive therapy for repetitive negative thinking in primary and non-primary generalized anxiety disorder: An effectiveness trial. J. Affect. Disord. 175 , 124–132 (2015).
Vallat, R. Pingouin: Statistics in Python. J. Open Source Softw. 3 (31), 1026. https://doi.org/10.21105/joss.01026 (2018).
Article ADS Google Scholar
Jacobson, N. S. & Truax, P. Clinical significance: A statistical approach to defining meaningful change in psychotherapy research. J. Consult. Clin. Psychol. 59 , 12–19 (1992).
Pedregosa, F. et al. Scikit-learn: Machine learning in Python. J. Mach. Learn. Res. 12 , 2825–2830 (2011).
MathSciNet Google Scholar
Ali, M. PyCaret: An open source, low-code machine learning library in Python. https://www.pycaret.org (2020).
Mandrekar, J. N. Receiver operating characteristic curve in diagnostic test assessment. J. Thorac. Oncol. 5 (9), 1315–1316 (2010).
Bishop, C. M. & Nasrabadi, N. M. Pattern Recognition and Machine Learning Vol. 4, 738 (Springer, 2006).
Strumbelj, E. & Kononenko, I. Explaining prediction models and individual predictions with feature contributions. Knowl. Inf. Syst. J. https://doi.org/10.1007/s10115-013-0679-x (2014).
Lundberg, S. M., & Lee, S. I. A unified approach to interpreting model predictions. In Advances in Neural Information Processing Systems ( NeurIPS ) (2017).
Guyon, I. & Elisseeff, A. An introduction to variable and feature selection. J. Mach. Learn. Res. 3 (2), 1157–1182 (2003).
Swets, J. A. Measuring the accuracy of diagnostic systems. Science 240 (4857), 1285–1293 (1988).
Article ADS MathSciNet CAS PubMed Google Scholar
Streiner, D. L. & Cairney, J. What’s under the ROC? An introduction to receiver operating characteristics curves. Can. J. Psychiatry 52 (2), 121–128 (2007).
Moore, D. S., Notz, W. & Fligner, M. A. The Basic Practice of Statistics (W.H. Freeman and Company, 2013).
Cohen, J. Statistical Power Analysis for the Behavioral Sciences 2nd edn. (Lawrence Erlbaum Associates, 1988).
Lambert, M. J. & Harmon, K. L. The merits of implementing routine outcome monitoring in clinical practice. Clin. Psychol. Sci. Pract. 25 (4), e12268 (2018).
Naragon-Gainey, K. Meta-analysis of the relations of anxiety sensitivity to the depressive and anxiety disorders. Psychol. Bull. 136 (1), 128 (2010).
McEvoy, P. M., Hyett, M. P., Shihata, S., Price, J. E. & Strachan, L. The impact of methodological and measurement factors on transdiagnostic associations with intolerance of uncertainty: A meta-analysis. Clin. Psychol. Rev. 73 , 101778 (2019).
Akbari, M., Seydavi, M., Hosseini, Z. S., Krafft, J. & Levin, M. E. Experiential avoidance in depression, anxiety, obsessive-compulsive related, and posttraumatic stress disorders: A comprehensive systematic review and meta-analysis. J. Context. Behav. Sci. 24 , 65–78 (2022).
Vaz, A. M., Ferreira, L. I., Gelso, C. & Janeiro, L. The sister concepts of working alliance and real relationship: A meta-analysis. Counsel. Psychol. Q. 37 (2), 247–268 (2024).
de Graaf, R., ten Have, M., Tuithof, M. & van Dorsselaer, S. First-incidence of DSM-IV mood, anxiety and substance use disorders and its determinants: Results from the Netherlands Mental Health Survey and Incidence Study-2. J. Affect. Disord. 149 (1–3), 100–107 (2013).
Sharma, S., Powers, A., Bradley, B. & Ressler, K. J. Gene × environment determinants of stress-and anxiety-related disorders. Annu. Rev. Psychol. 67 (1), 239–261 (2016).
Cuijpers, P. Targets and outcomes of psychotherapies for mental disorders: An overview. World Psychiatry 18 (3), 276–285 (2019).
Lundqvist, L. O. et al. Influence of mental health service provision on the perceived quality of life among psychiatric outpatients: Associations and mediating factors. Front. Psychiatry 14 , 1282466 (2024).
McAleavey, A. A., de Jong, K., Nissen-Lie, H. A., Boswell, J. F., Moltu, C. & Lutz, W. (2024). Routine outcome monitoring and clinical feedback in psychotherapy: Recent advances and future directions. Administration and Policy in Mental Health and Mental Health Services Research , 1–15.
Download references
Acknowledgements
This work was supported by the Digital Health Cooperative Research Centre (DHCRC) [DHCRC-0076]. DHCRC is funded under the Australian Commonwealth’s Cooperative Research Centres (CRC) Program. The funder had no role in the study design, data collection and analysis, decision to publish, or preparation for the manuscript. The authors wish to thank Justin Manuel from Western Australia Country Health Service for his ongoing contribution to the overall project and to the staff from the Department of Health WA’s Data Linkage Services and the Hospital Morbidity Data Collection, Emergency Department Data Collection, and Mental Health Data Collection.
Author information
Authors and affiliations.
School of Population Health, Curtin University, Perth, WA, Australia
Kevin E. K. Chai, Crystal M. Y. Lee, Kim S. Betts, Suzanne Robinson & Peter M. McEvoy
Department of Health, Perth, WA, Australia
Kyran Graham-Schmidt
Western Australia Primary Health Alliance, Perth, WA, Australia
Daniel Rock
Discipline of Psychiatry, Medical School, University of Western Australia, Perth, WA, Australia
Faculty of Health, Health Research Institute, University of Canberra, Canberra, ACT, Australia
Western Australia Country Health Service, Albany, WA, Australia
Mathew Coleman
Deakin Health Economics, Deakin University, Melbourne, VIC, Australia
Suzanne Robinson
Centre for Clinical Interventions, North Metropolitan Health Service, Perth, WA, Australia
Peter M. McEvoy
You can also search for this author in PubMed Google Scholar
Contributions
K.E.K.C analysed the data, conducted the experiments and drafted the manuscript. K.E.K.C, K.G.S C.M.Y.L, P.M.M., D.R, M.C conceived the design and P.M.M, K.G.S, D.R, M.C provided clinical advice for the project. K.S.B, P.M, D.R, S.R secured funding for the project. All authors contributed to the critical revision of the manuscript and approved the final version of the article to be published.
Corresponding author
Correspondence to Kevin E. K. Chai .
Ethics declarations
Competing interests.
The authors declare no competing interests.
Ethics approval
Additional information, publisher's note.
Springer Nature remains neutral with regard to jurisdictional claims in published maps and institutional affiliations.
Supplementary Information
Supplementary information., rights and permissions.
Open Access This article is licensed under a Creative Commons Attribution-NonCommercial-NoDerivatives 4.0 International License, which permits any non-commercial use, sharing, distribution and reproduction in any medium or format, as long as you give appropriate credit to the original author(s) and the source, provide a link to the Creative Commons licence, and indicate if you modified the licensed material. You do not have permission under this licence to share adapted material derived from this article or parts of it. The images or other third party material in this article are included in the article’s Creative Commons licence, unless indicated otherwise in a credit line to the material. If material is not included in the article’s Creative Commons licence and your intended use is not permitted by statutory regulation or exceeds the permitted use, you will need to obtain permission directly from the copyright holder. To view a copy of this licence, visit http://creativecommons.org/licenses/by-nc-nd/4.0/ .
Reprints and permissions
About this article
Cite this article.
Chai, K.E.K., Graham-Schmidt, K., Lee, C.M.Y. et al. Predicting anxiety treatment outcome in community mental health services using linked health administrative data. Sci Rep 14 , 20559 (2024). https://doi.org/10.1038/s41598-024-71557-2
Download citation
Received : 17 May 2024
Accepted : 29 August 2024
Published : 04 September 2024
DOI : https://doi.org/10.1038/s41598-024-71557-2
Share this article
Anyone you share the following link with will be able to read this content:
Sorry, a shareable link is not currently available for this article.
Provided by the Springer Nature SharedIt content-sharing initiative
By submitting a comment you agree to abide by our Terms and Community Guidelines . If you find something abusive or that does not comply with our terms or guidelines please flag it as inappropriate.
Quick links
- Explore articles by subject
- Guide to authors
- Editorial policies
Sign up for the Nature Briefing newsletter — what matters in science, free to your inbox daily.

- Corpus ID: 51995823
Integrating Community Services and Research: A Livelihood Needs Assessment at the Countryside of the Philippines
- R. C. Garcia
- Published 2017
- Sociology, Environmental Science
- Journal of Education and Practice
Figures from this paper
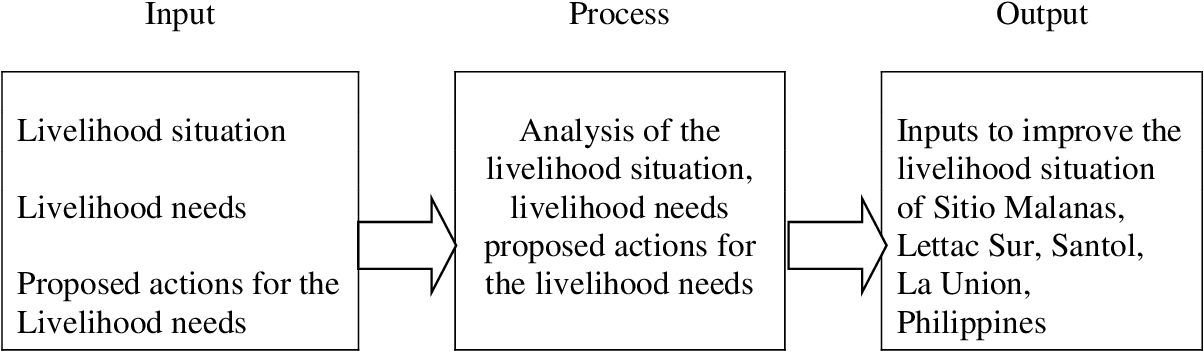
7 Citations
Sources of living: a community needs assessment for livelihood of panaytayan community in mansalay, oriental mindoro, community needs assessment as basis for the extension program of philippine college foundation, acceptability and utilization of livelihood programs in higher education, effectiveness and impact of community extension program of one philippine higher education institution as basis for sustainability, community needs assessment of barangay 694 towards an extension services program, profitability and efficiency evaluation of the financial management of a socio-economic intervention, international journal of evaluation and research in education (ijere), 15 references, ladder of citizen participation, teaching community development to social work students: a critical reflection, qualitative inquiry and research design: choosing among five approaches, methods of research and thesis writing, related papers.
Showing 1 through 3 of 0 Related Papers
Academia.edu no longer supports Internet Explorer.
To browse Academia.edu and the wider internet faster and more securely, please take a few seconds to upgrade your browser .
Enter the email address you signed up with and we'll email you a reset link.
- We're Hiring!
- Help Center
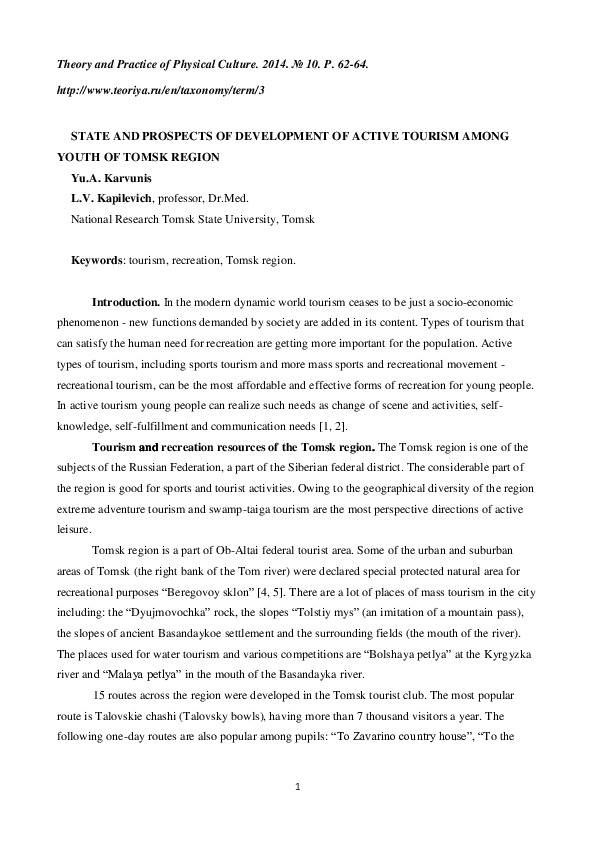
STATE AND PROSPECTS OF DEVELOPMENT OF ACTIVE TOURISM AMONG YOUTH OF TOMSK REGION

The fact that Tomsk is a student city, with a large number of young active people in it, can be assumed the basic factor in the development of sports and recreational tourism in the Tomsk region. This contingent is the best for the formation of the concept of development of sport routes. But with a rather substantial potential for development, currently active forms of tourism are not popular in the Tomsk region, including among young people. There are several obstacles to positive changes. The first is the lack of awareness of potential tourists on existing routes and active leisure activities. Sports tourism is mostly promoted by small, non-governmental organizations and clubs affected by the lack of qualified instructors, effective advertising and promotion of tourism as it is in educational institutions, the lack of image of the Tomsk region as a favorable tourist region.
Related Papers
NETOUR: Network for Excellence in Tourism through Organizations and Universities in Russia
David Ward-Perkins
This report starts with a presentation of the main characteristics that define nature-based tourism, together with an overview of nature-based tourism in Russian protected areas. Some illustrative cases taken in the North-West Federal District of Russia, Mari El Republic, and the Krasnodar territory are provided. The state of the art for Russian nature-based tourism is analysed through those cases. Then, an analysis is conducted to identify the current strengths and weaknesses of this Russian tourism, as well as opportunities and potential risks to consider, for Russia to improve its position as a nature-based tourism destination. The report concludes by offering strategic recommendations for future development, sustainable management, marketing, and guidelines to enhance human resource development through universities.
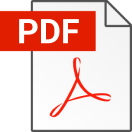
Also in present day development of Polish coastal dunes heavy storms and human activity plays main role. Most of the Polish coastal habitats including dunes are also threatened by human activities (PIOTROWSKA 1995). Major impacts of tourism industry in coastal areas are: (i) infrastructure with hotels, transport system and garbage, (ii) recreation with human activity, and (iii) coast protection against abrasion. If coastal environment will be damaged or destroyed, tourist will not be arriving. Small value of environment is equal to smaller number of tourists and smaller incoming from tourism industry.
Natalie Djohari
Snezana Stetic
International Symposium of Advancements in Tourism, Recreation and Sport Sciences - Book of Abstracts, p. 41, ISBN 978-605-81246-0-8
Tiziano Gasbarro
The purpose is to analyze and confirm the desire to reinterpret local fragments by reaching a "plurality of experiences and landscapes of living" (Lanzani, 2009), that extend beyond the few rigid models of the past, varying more and more the multiple points of view from which the observer chooses to position himself, directly and indirectly, in the digital age we experience. It becomes necessary to introduce a fundamental concept such as the creation of Landscape (Magnaghi, 2010) already discussed and debated in reference to the combination of "constructed or built" and "green and free spaces" (Gambino, 1989). The Landscape is no longer subdivided into an obsolete territorialization and sectorization that refers, to name a few, to rural areas, urban, suburban-residential, post-industrial (Lanzani, 2009) as much as one, from time to time different, land art that make recognizable and as essential as those landmarks of a landscape so reconstructed and planned, which is beautiful to see but especially to live and participate, referring to its different "self-sustainability" (Magnaghi, 2010). With Landscape we mean "an area, as perceived by people, whose character is the result of the action and interaction of natural and/or human factors" (European Landscape Convention, Chapter 1, Art 1). Thus, the thought seems to assert that a Landscape does not exist, except through the eyes of those who benefit from it. In this form, we can speak of city-territory/city-landscape as we refer to an internet network, labyrinthine, widespread and without centers and outskirts (Zagari, 2013), but which is subject to a landscaping as "intelligent space, geographical certification of successful social practices" (Turco, 2017). It is extremely similar to the concept of "infinite city" (Bonomi, Abruzzese, 2004) which inevitably leads to the identification of the "Third Landscape" (Clément, 2014) finally recognized and valued in a context of reappropriation of places. Keywords— shaping, land art, landmarks, point of view
International Symposium of Advancements in Tourism, Recreation and Sport Sciences - Book of Abstracts, p. 29, ISBN 978-605-81246-0-8
Giuliano Bifolchi
The North Caucasus is a Southern Russian region affected by terrorism and jihadist propaganda and considered the most unstable, underdeveloped and unsafe area in the Russian Federation. Since the collapse of the Soviet Union, the North Caucasus has experienced ethnic conflicts, militancy, the rise of radicalism, terrorist attacks, corruption and high unemployment rate. With the aim of contrasting the socioeconomic problems and the recruitment process among the ranks of local militant groups (Imarat Kavkaz) and international terrorist organisations (al-Qaeda and the Islamic State), the Kremlin started a strategy focused on tourism development. This paper wants to study the Russian policy of contrasting terrorism and improving socioeconomic conditions through the analysis of the Kurorti Severnovo Kavkazka (North Caucasus Resorts), a project whose goal is to realise regional tourist clusters to attract FDIs and create more than 31,000 new jobs. In this research, the author will assess the Russian tourism strategy on regional level evaluating at the same time the impact of terrorism and local militancy on the regional tourism development and the effects of the Kurorti Severnovo Kavkaza project in ethnic conflicts management and counterterrorism activities.
Alina Zajadacz
SUMMARY Tourism means traveling through time and space16 in a bid to satisfy diverse needs and desires, which change with the stages of people’s lives. Awareness of permanent tourist characteristics, along with an identification of current trends and development perspectives was the underlying premise in the strategic planning of regional tourist products. The outlined concept proposes a varied offer in: (1) time – seasonal and long- or short-term stays throughout the year; (2) space – separation of the area into zones of intensive, extensive and linear tourist use depending on the tourist assets of the given area, their durability and tourists’ behavior (avoiding conflicts arising from sometimes contradictory interests of different groups) (3) c onnection with tourists’ needs conditioned by social, economic, demographic and health factors; taking into account the needs of organized tourism (mass and small scale) and individual tourism. The vision of tourism development in the Leszno Region reflects current tourist tendencies, which clearly show the desire to seek new unknown places, to choose safe destinations (without the threat of natural disasters or terrorist attacks). The vision was prepared in the process of a broad social dialogue in cooperation with local authorities, entrepreneurs, local inhabitants and tourist experts, which obviously increases the social awareness and acceptance of the implementation of the development plan.
cornetis.pl
Adam R . Szromek
This paper discusses the revitalization of spa services in Poland, which were falling into decline during the 1980s and 1990s. The difficulties faced by entrepreneurs and local administrative leaders, and the scientific studies of specialists in the field have also been analyzed. The author puts forward the hypothesis that spa tourism provides a stimulus for the market revitalization of spa facilities and an opportunity for the further development of health resorts and related enterprises in the country.
Piotr Dzikowski
The article presents the model of a sectoral system of innovation that is a multidimensional and dynamic picture of tourism sector innovativeness. This approach extends boundaries of the sector by taking into account firms and non-firms, links between them and the process of production and accumulation of knowledge. It helps to identify a set of key factors affecting the intensity of innovation, economic potential and international competitiveness of the sector, including companies operating within a given geographical territory. The goal of this paper is to adapt the idea of a sectoral systems of innovation to analyze a tourism sector in Poland. The paper is divided into 6 sections. In Section 2, basic concepts of innovation and innovation activity are presented. In Section 3, a sectoral innovation systems framework is proposed. In Section 4, the basic building blocks of the SSI in the tourism sector are examined. Then in section 5, the basics of tourism innovation system in Poland are discussed. Last part includes conclusions and suggestions of future research.
Lifestyle in Siberia and the Russian North
Joachim Otto Habeck
Lifestyle in Siberia and the Russian North Joachim Otto Habeck (editor) Lifestyle in Siberia and the Russian North breaks new ground by exploring the concept of lifestyle from a distinctly anthropological perspective. Showcasing the collective work of ten experienced scholars in the field, the book goes beyond concepts of tradition that have often been the focus of previous research, to explain how political, economic and technological changes in Russia have created a wide range of new possibilities and constraints in the pursuit of different ways of life. Each contribution is drawn from meticulous first-hand field research, and the authors engage with theoretical questions such as whether and how the concept of lifestyle can be extended beyond its conventionally urban, Euro-American context and employed in a markedly different setting. Lifestyle in Siberia and the Russian North builds on the contributors’ clear commitment to diversifying the field and providing a novel and intimate insight into this vast and dynamic region. This book provides inspiring reading for students and teachers of Anthropology, Sociology and Cultural Studies and for anyone interested in Russia and its regions. By providing ethnographic case studies, it is also a useful basis for teaching anthropological methods and concepts, both at graduate and undergraduate level. Rigorous and innovative, it marks an important contribution to the study of Siberia and the Russian North.
Loading Preview
Sorry, preview is currently unavailable. You can download the paper by clicking the button above.
RELATED TOPICS
- We're Hiring!
- Help Center
- Find new research papers in:
- Health Sciences
- Earth Sciences
- Cognitive Science
- Mathematics
- Computer Science
- Academia ©2024

- Article Databases
- Dictionaries, Encyclopedias, & Handbooks
- Taxonomic Guides
- Manuals & Guides
- Protocols & Methods
- The Ins & Outs of Citing
- Journal Level Measures
- Author Level Measures
Meet Your Librarian
Biology Research at the UMD Libraries
Welcome to the Biology Research Guide!
This guide is designed to help you navigate the many resources offered by the University Libraries. From journal databases to research data management, we are here to aid in your learning and research in Biology. From this page you can:
- Explore library resources that may be helpful to your learning or research.
- Find research information for specific courses in Biology.
- Learn about important scholarly skills such as research data management and citation managers.
As always, if you have any questions about this guide or about library services in general, please reach out to me, your subject specialist by emailing me from the box on the left, or stopping by my office in the STEM Library.
VPN & Proxy Services at UMD
The Libraries' online subscription resources can be accessed from computers and devices on campus and off-campus. When using devices off-campus, access is restricted to current UMD students, faculty, and staff. Below are methods for connecting to the Libraries' resources from off-campus locations.
If you still need help, you can contact the UMD IT Service Desk at 301-405-1500 or [email protected] .
Download and install UMD's VPN to connect to online services. You will need to log in manually to access a restricted site. The connection will remain live until you manually disconnect.
A guide to create proxy links by simply entering in the original URL into a proxy creator box. The output can then be pasted into your browser to gain access to a restricted site.
A browser extension that automatically adds the UMD proxy to your URL. Drag the reload button to your bookmarks to use whenever you need access.
Biology News
- Next: Article Databases >>
- Last Updated: Sep 5, 2024 12:25 AM
- URL: https://lib.guides.umd.edu/bio
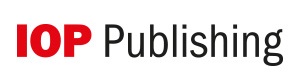
We apologize for the inconvenience...
To ensure we keep this website safe, please can you confirm you are a human by ticking the box below.
If you are unable to complete the above request please contact us using the below link, providing a screenshot of your experience.
https://ioppublishing.org/contacts/
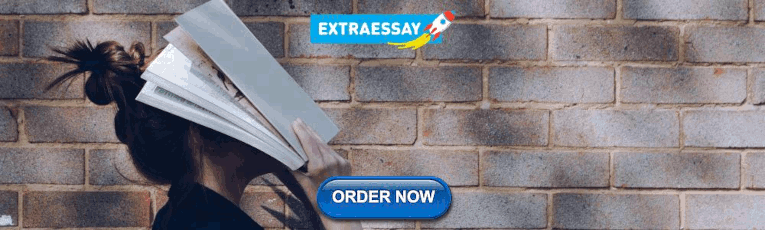
IMAGES
VIDEO
COMMENTS
Opportunities to engage in community service have steadily increased during the last decades (Griffith, 2012).Not all students enrolled in such programs, however, are indeed transformed by their experiences (Jones et al., 2005).First, this is because the impact of such programs may be moderated by participants' characteristics, as a meta-analysis of 49 single studies reveals (van Goethem et ...
activities-community service being one of them (McCarthy & Tucker 1999). By integrating community service into the core curriculum, it provides students life skills that benefit the community and society as a whole. Besides, it also supports teachers to integrate education, learning and humanity (Andrews, 2007; Easterling & Rudell, 1997).
Abstract. This study assessed the integration of community services and research through the livelihood needs assessment of a countryside in the Philippines particularly Sitio Malanas, Lettac Sur, Santol, La Union as basis of the actions in addressing the needs. Specifically, the livelihood situation and needs were determined and proposed ...
However, there is little emphasis and inconsistent research findings within a research field. Therefore, this review paper explores how student volunteerism will contribute to community service ...
community service had higher GPA scores and higher mandated Massachusetts State testing scores in grades 6, 7, and all but one category in grade 8. The research study was undertaken in hopes that the research findings will be of value to the school administration, school committee, and parents when deciding on possibly mandating
PDF | This study aims to determine the student's community service involvement at a local higher education institution in Olongapo City, Philippines. We... | Find, read and cite all the research ...
Explore the latest full-text research PDFs, articles, conference papers, preprints and more on COMMUNITY SERVICES. Find methods information, sources, references or conduct a literature review on ...
The role of community engagement in the field of community practice and generally in social work have continuously evolved over the last century. Included in this issue is "From the archives: Jesse Frederick Steiner assesses community organization in 1922" by Paul Stuart (Stuart, 2022). Stein's 1922 article "Community organization: A ...
DEFINING SERVICE LEARNING AND ANCILLARY CONCEPTS AND CONTEXT. Service learning remains an essential pedagogy for building connections between campus and community, while enriching learning for students (Citation Sullivan, 2000; Citation Zlutkowski, 1996).For this special issue, the editors have looked to the National Service-Learning Clearinghouse for Learn and Serve America, a component of ...
Smart community enables a sustainable and livable community future, in which residents' demands play an important role in its success. Though great efforts have been made to encourage residents' participation in the implementation of smart communities, inefficient service supply still exists. Thus, this study aimed to classify residents' demands for community services in smart ...
1. Introduction. Delivering community service is among the integral objectives of higher educa-tion institutions (HEIs) of Ethiopia together with the teaching-learning and research process [1]. This research titled practices and challenges of community services at Debre Markos University, Ethiopia, was conducted in 2020.
That is, research with the community beyond community participation or 'community-based' research (e.g., collaboratively developed interventions, research questions, methods, analyses). Subsequently, data were extracted from each article by two reviewers from the research team (see additional file 2 for data extraction).
The epistemology of community-based research can be traced back to many roots—Karl Marx, John Dewey, Paulo Freire, C.W. Mills, Thomas Kuhn, and Jane Addams to name but a few. Community-based research as it is practiced today has been enriched by the diversity of thoughts, methodologies, and practices that has been its foundation.
The types of community services in smart communities are clarified and fresh insights are provided into associated factors related to residents' demands for these services in this study, through which enhanced provision of community services and effective implementation of smart communities can be achieved. ... Research Support, Non-U.S. Gov't ...
This paper attempts a parsimonious definition of community development. It proposes that the purpose of community development is the pursuit of solidarity and agency by adhering to the principles ...
View Community and Social Services Research Papers on Academia.edu for free. Skip to main content ... This project was done in partnership with Sonshine Community Services in Calgary and examined the leadership style that is inducive of creating a trauma-informed workplace for all staff. To answer this question, the project captured the ...
Introduction. Community-based supports and services (CBSS) are designed to help community-dwelling older adults remain safely in their homes and delay or prevent institutionalization. CBSS provide (and act as a link to) specific resources for older adults and their caregivers that include wellness programs, nutritional support, educational ...
Recent studies characterizing social activity profiles of older adults in the community have been conducted in Japan (Amagasa et al., 2017), Korea (Katagiri & Kim, 2018), Switzerland (Dawson-Townsend, 2019), and among partnered-older adults in Australia (Lam & Bolano, 2019).These studies have shown some individuals engage more in formal activities (e.g., club memberships, volunteering) and ...
Anxiety disorders are the most common class of mental illness in Australia, affecting 3.4 million adults aged 16 years and older or 17.2% of the population in 2020-2022 1.Similarly in the United ...
This study assessed the integration of community services and research through the livelihood needs assessment of a countryside in the Philippines particularly Sitio Malanas, Lettac Sur, Santol, La Union as basis of the actions in addressing the needs. Specifically, the livelihood situation and needs were determined and proposed actions for the improvement of the livelihood situation of the ...
The fact that Tomsk is a student city, with a large number of young active people in it, can be assumed the basic factor in the development of sports and recreational tourism in the Tomsk region. This contingent is the best for the formation of the
Welcome to the Biology Research Guide! This guide is designed to help you navigate the many resources offered by the University Libraries. From journal databases to research data management, we are here to aid in your learning and research in Biology. From this page you can: Explore library resources that may be helpful to your learning or ...
National Research Tomsk Polytechnic University, 30 Lenin Ave., Tomsk, 634050, Russia . E-mail: 4 [email protected], 5 [email protected]. Abstract. The paper describes the results of the characteristic structure features of oil-bearing rocks via paleomagnetic oriented cores. Volume core model is plotted on the basis of circular panoramic images.
The paper discusses the possibility of selecting relevant data from the pool of the official state statistics indicators to assess the elderly generation's wellbeing in 85 regions of the Russian ...
Our research strategy was to compare the formal property rights introduced in two resource-based sectors during the transition period: the forest sector and the cod fishery sector in the Barents Sea.