How to Write Limitations of the Study (with examples)
This blog emphasizes the importance of recognizing and effectively writing about limitations in research. It discusses the types of limitations, their significance, and provides guidelines for writing about them, highlighting their role in advancing scholarly research.
Updated on August 24, 2023

No matter how well thought out, every research endeavor encounters challenges. There is simply no way to predict all possible variances throughout the process.
These uncharted boundaries and abrupt constraints are known as limitations in research . Identifying and acknowledging limitations is crucial for conducting rigorous studies. Limitations provide context and shed light on gaps in the prevailing inquiry and literature.
This article explores the importance of recognizing limitations and discusses how to write them effectively. By interpreting limitations in research and considering prevalent examples, we aim to reframe the perception from shameful mistakes to respectable revelations.
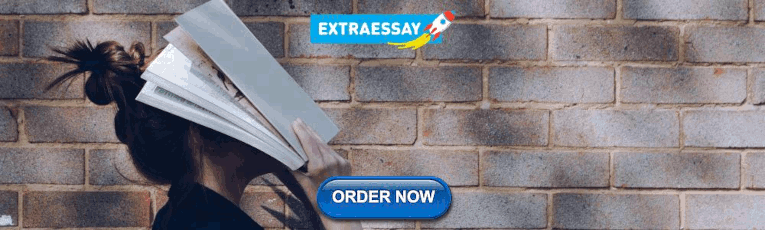
What are limitations in research?
In the clearest terms, research limitations are the practical or theoretical shortcomings of a study that are often outside of the researcher’s control . While these weaknesses limit the generalizability of a study’s conclusions, they also present a foundation for future research.
Sometimes limitations arise from tangible circumstances like time and funding constraints, or equipment and participant availability. Other times the rationale is more obscure and buried within the research design. Common types of limitations and their ramifications include:
- Theoretical: limits the scope, depth, or applicability of a study.
- Methodological: limits the quality, quantity, or diversity of the data.
- Empirical: limits the representativeness, validity, or reliability of the data.
- Analytical: limits the accuracy, completeness, or significance of the findings.
- Ethical: limits the access, consent, or confidentiality of the data.
Regardless of how, when, or why they arise, limitations are a natural part of the research process and should never be ignored . Like all other aspects, they are vital in their own purpose.
Why is identifying limitations important?
Whether to seek acceptance or avoid struggle, humans often instinctively hide flaws and mistakes. Merging this thought process into research by attempting to hide limitations, however, is a bad idea. It has the potential to negate the validity of outcomes and damage the reputation of scholars.
By identifying and addressing limitations throughout a project, researchers strengthen their arguments and curtail the chance of peer censure based on overlooked mistakes. Pointing out these flaws shows an understanding of variable limits and a scrupulous research process.
Showing awareness of and taking responsibility for a project’s boundaries and challenges validates the integrity and transparency of a researcher. It further demonstrates the researchers understand the applicable literature and have thoroughly evaluated their chosen research methods.
Presenting limitations also benefits the readers by providing context for research findings. It guides them to interpret the project’s conclusions only within the scope of very specific conditions. By allowing for an appropriate generalization of the findings that is accurately confined by research boundaries and is not too broad, limitations boost a study’s credibility .
Limitations are true assets to the research process. They highlight opportunities for future research. When researchers identify the limitations of their particular approach to a study question, they enable precise transferability and improve chances for reproducibility.
Simply stating a project’s limitations is not adequate for spurring further research, though. To spark the interest of other researchers, these acknowledgements must come with thorough explanations regarding how the limitations affected the current study and how they can potentially be overcome with amended methods.
How to write limitations
Typically, the information about a study’s limitations is situated either at the beginning of the discussion section to provide context for readers or at the conclusion of the discussion section to acknowledge the need for further research. However, it varies depending upon the target journal or publication guidelines.
Don’t hide your limitations
It is also important to not bury a limitation in the body of the paper unless it has a unique connection to a topic in that section. If so, it needs to be reiterated with the other limitations or at the conclusion of the discussion section. Wherever it is included in the manuscript, ensure that the limitations section is prominently positioned and clearly introduced.
While maintaining transparency by disclosing limitations means taking a comprehensive approach, it is not necessary to discuss everything that could have potentially gone wrong during the research study. If there is no commitment to investigation in the introduction, it is unnecessary to consider the issue a limitation to the research. Wholly consider the term ‘limitations’ and ask, “Did it significantly change or limit the possible outcomes?” Then, qualify the occurrence as either a limitation to include in the current manuscript or as an idea to note for other projects.
Writing limitations
Once the limitations are concretely identified and it is decided where they will be included in the paper, researchers are ready for the writing task. Including only what is pertinent, keeping explanations detailed but concise, and employing the following guidelines is key for crafting valuable limitations:
1) Identify and describe the limitations : Clearly introduce the limitation by classifying its form and specifying its origin. For example:
- An unintentional bias encountered during data collection
- An intentional use of unplanned post-hoc data analysis
2) Explain the implications : Describe how the limitation potentially influences the study’s findings and how the validity and generalizability are subsequently impacted. Provide examples and evidence to support claims of the limitations’ effects without making excuses or exaggerating their impact. Overall, be transparent and objective in presenting the limitations, without undermining the significance of the research.
3) Provide alternative approaches for future studies : Offer specific suggestions for potential improvements or avenues for further investigation. Demonstrate a proactive approach by encouraging future research that addresses the identified gaps and, therefore, expands the knowledge base.
Whether presenting limitations as an individual section within the manuscript or as a subtopic in the discussion area, authors should use clear headings and straightforward language to facilitate readability. There is no need to complicate limitations with jargon, computations, or complex datasets.
Examples of common limitations
Limitations are generally grouped into two categories , methodology and research process .
Methodology limitations
Methodology may include limitations due to:
- Sample size
- Lack of available or reliable data
- Lack of prior research studies on the topic
- Measure used to collect the data
- Self-reported data

The researcher is addressing how the large sample size requires a reassessment of the measures used to collect and analyze the data.
Research process limitations
Limitations during the research process may arise from:
- Access to information
- Longitudinal effects
- Cultural and other biases
- Language fluency
- Time constraints

The author is pointing out that the model’s estimates are based on potentially biased observational studies.
Final thoughts
Successfully proving theories and touting great achievements are only two very narrow goals of scholarly research. The true passion and greatest efforts of researchers comes more in the form of confronting assumptions and exploring the obscure.
In many ways, recognizing and sharing the limitations of a research study both allows for and encourages this type of discovery that continuously pushes research forward. By using limitations to provide a transparent account of the project's boundaries and to contextualize the findings, researchers pave the way for even more robust and impactful research in the future.
Charla Viera, MS
See our "Privacy Policy"
Ensure your structure and ideas are consistent and clearly communicated
Pair your Premium Editing with our add-on service Presubmission Review for an overall assessment of your manuscript.
- USC Libraries
- Research Guides
Organizing Your Social Sciences Research Paper
- Limitations of the Study
- Purpose of Guide
- Design Flaws to Avoid
- Independent and Dependent Variables
- Glossary of Research Terms
- Reading Research Effectively
- Narrowing a Topic Idea
- Broadening a Topic Idea
- Extending the Timeliness of a Topic Idea
- Academic Writing Style
- Applying Critical Thinking
- Choosing a Title
- Making an Outline
- Paragraph Development
- Research Process Video Series
- Executive Summary
- The C.A.R.S. Model
- Background Information
- The Research Problem/Question
- Theoretical Framework
- Citation Tracking
- Content Alert Services
- Evaluating Sources
- Primary Sources
- Secondary Sources
- Tiertiary Sources
- Scholarly vs. Popular Publications
- Qualitative Methods
- Quantitative Methods
- Insiderness
- Using Non-Textual Elements
- Common Grammar Mistakes
- Writing Concisely
- Avoiding Plagiarism
- Footnotes or Endnotes?
- Further Readings
- Generative AI and Writing
- USC Libraries Tutorials and Other Guides
- Bibliography
The limitations of the study are those characteristics of design or methodology that impacted or influenced the interpretation of the findings from your research. Study limitations are the constraints placed on the ability to generalize from the results, to further describe applications to practice, and/or related to the utility of findings that are the result of the ways in which you initially chose to design the study or the method used to establish internal and external validity or the result of unanticipated challenges that emerged during the study.
Price, James H. and Judy Murnan. “Research Limitations and the Necessity of Reporting Them.” American Journal of Health Education 35 (2004): 66-67; Theofanidis, Dimitrios and Antigoni Fountouki. "Limitations and Delimitations in the Research Process." Perioperative Nursing 7 (September-December 2018): 155-163. .
Importance of...
Always acknowledge a study's limitations. It is far better that you identify and acknowledge your study’s limitations than to have them pointed out by your professor and have your grade lowered because you appeared to have ignored them or didn't realize they existed.
Keep in mind that acknowledgment of a study's limitations is an opportunity to make suggestions for further research. If you do connect your study's limitations to suggestions for further research, be sure to explain the ways in which these unanswered questions may become more focused because of your study.
Acknowledgment of a study's limitations also provides you with opportunities to demonstrate that you have thought critically about the research problem, understood the relevant literature published about it, and correctly assessed the methods chosen for studying the problem. A key objective of the research process is not only discovering new knowledge but also to confront assumptions and explore what we don't know.
Claiming limitations is a subjective process because you must evaluate the impact of those limitations . Don't just list key weaknesses and the magnitude of a study's limitations. To do so diminishes the validity of your research because it leaves the reader wondering whether, or in what ways, limitation(s) in your study may have impacted the results and conclusions. Limitations require a critical, overall appraisal and interpretation of their impact. You should answer the question: do these problems with errors, methods, validity, etc. eventually matter and, if so, to what extent?
Price, James H. and Judy Murnan. “Research Limitations and the Necessity of Reporting Them.” American Journal of Health Education 35 (2004): 66-67; Structure: How to Structure the Research Limitations Section of Your Dissertation. Dissertations and Theses: An Online Textbook. Laerd.com.
Descriptions of Possible Limitations
All studies have limitations . However, it is important that you restrict your discussion to limitations related to the research problem under investigation. For example, if a meta-analysis of existing literature is not a stated purpose of your research, it should not be discussed as a limitation. Do not apologize for not addressing issues that you did not promise to investigate in the introduction of your paper.
Here are examples of limitations related to methodology and the research process you may need to describe and discuss how they possibly impacted your results. Note that descriptions of limitations should be stated in the past tense because they were discovered after you completed your research.
Possible Methodological Limitations
- Sample size -- the number of the units of analysis you use in your study is dictated by the type of research problem you are investigating. Note that, if your sample size is too small, it will be difficult to find significant relationships from the data, as statistical tests normally require a larger sample size to ensure a representative distribution of the population and to be considered representative of groups of people to whom results will be generalized or transferred. Note that sample size is generally less relevant in qualitative research if explained in the context of the research problem.
- Lack of available and/or reliable data -- a lack of data or of reliable data will likely require you to limit the scope of your analysis, the size of your sample, or it can be a significant obstacle in finding a trend and a meaningful relationship. You need to not only describe these limitations but provide cogent reasons why you believe data is missing or is unreliable. However, don’t just throw up your hands in frustration; use this as an opportunity to describe a need for future research based on designing a different method for gathering data.
- Lack of prior research studies on the topic -- citing prior research studies forms the basis of your literature review and helps lay a foundation for understanding the research problem you are investigating. Depending on the currency or scope of your research topic, there may be little, if any, prior research on your topic. Before assuming this to be true, though, consult with a librarian! In cases when a librarian has confirmed that there is little or no prior research, you may be required to develop an entirely new research typology [for example, using an exploratory rather than an explanatory research design ]. Note again that discovering a limitation can serve as an important opportunity to identify new gaps in the literature and to describe the need for further research.
- Measure used to collect the data -- sometimes it is the case that, after completing your interpretation of the findings, you discover that the way in which you gathered data inhibited your ability to conduct a thorough analysis of the results. For example, you regret not including a specific question in a survey that, in retrospect, could have helped address a particular issue that emerged later in the study. Acknowledge the deficiency by stating a need for future researchers to revise the specific method for gathering data.
- Self-reported data -- whether you are relying on pre-existing data or you are conducting a qualitative research study and gathering the data yourself, self-reported data is limited by the fact that it rarely can be independently verified. In other words, you have to the accuracy of what people say, whether in interviews, focus groups, or on questionnaires, at face value. However, self-reported data can contain several potential sources of bias that you should be alert to and note as limitations. These biases become apparent if they are incongruent with data from other sources. These are: (1) selective memory [remembering or not remembering experiences or events that occurred at some point in the past]; (2) telescoping [recalling events that occurred at one time as if they occurred at another time]; (3) attribution [the act of attributing positive events and outcomes to one's own agency, but attributing negative events and outcomes to external forces]; and, (4) exaggeration [the act of representing outcomes or embellishing events as more significant than is actually suggested from other data].
Possible Limitations of the Researcher
- Access -- if your study depends on having access to people, organizations, data, or documents and, for whatever reason, access is denied or limited in some way, the reasons for this needs to be described. Also, include an explanation why being denied or limited access did not prevent you from following through on your study.
- Longitudinal effects -- unlike your professor, who can literally devote years [even a lifetime] to studying a single topic, the time available to investigate a research problem and to measure change or stability over time is constrained by the due date of your assignment. Be sure to choose a research problem that does not require an excessive amount of time to complete the literature review, apply the methodology, and gather and interpret the results. If you're unsure whether you can complete your research within the confines of the assignment's due date, talk to your professor.
- Cultural and other type of bias -- we all have biases, whether we are conscience of them or not. Bias is when a person, place, event, or thing is viewed or shown in a consistently inaccurate way. Bias is usually negative, though one can have a positive bias as well, especially if that bias reflects your reliance on research that only support your hypothesis. When proof-reading your paper, be especially critical in reviewing how you have stated a problem, selected the data to be studied, what may have been omitted, the manner in which you have ordered events, people, or places, how you have chosen to represent a person, place, or thing, to name a phenomenon, or to use possible words with a positive or negative connotation. NOTE : If you detect bias in prior research, it must be acknowledged and you should explain what measures were taken to avoid perpetuating that bias. For example, if a previous study only used boys to examine how music education supports effective math skills, describe how your research expands the study to include girls.
- Fluency in a language -- if your research focuses , for example, on measuring the perceived value of after-school tutoring among Mexican-American ESL [English as a Second Language] students and you are not fluent in Spanish, you are limited in being able to read and interpret Spanish language research studies on the topic or to speak with these students in their primary language. This deficiency should be acknowledged.
Aguinis, Hermam and Jeffrey R. Edwards. “Methodological Wishes for the Next Decade and How to Make Wishes Come True.” Journal of Management Studies 51 (January 2014): 143-174; Brutus, Stéphane et al. "Self-Reported Limitations and Future Directions in Scholarly Reports: Analysis and Recommendations." Journal of Management 39 (January 2013): 48-75; Senunyeme, Emmanuel K. Business Research Methods. Powerpoint Presentation. Regent University of Science and Technology; ter Riet, Gerben et al. “All That Glitters Isn't Gold: A Survey on Acknowledgment of Limitations in Biomedical Studies.” PLOS One 8 (November 2013): 1-6.
Structure and Writing Style
Information about the limitations of your study are generally placed either at the beginning of the discussion section of your paper so the reader knows and understands the limitations before reading the rest of your analysis of the findings, or, the limitations are outlined at the conclusion of the discussion section as an acknowledgement of the need for further study. Statements about a study's limitations should not be buried in the body [middle] of the discussion section unless a limitation is specific to something covered in that part of the paper. If this is the case, though, the limitation should be reiterated at the conclusion of the section.
If you determine that your study is seriously flawed due to important limitations , such as, an inability to acquire critical data, consider reframing it as an exploratory study intended to lay the groundwork for a more complete research study in the future. Be sure, though, to specifically explain the ways that these flaws can be successfully overcome in a new study.
But, do not use this as an excuse for not developing a thorough research paper! Review the tab in this guide for developing a research topic . If serious limitations exist, it generally indicates a likelihood that your research problem is too narrowly defined or that the issue or event under study is too recent and, thus, very little research has been written about it. If serious limitations do emerge, consult with your professor about possible ways to overcome them or how to revise your study.
When discussing the limitations of your research, be sure to:
- Describe each limitation in detailed but concise terms;
- Explain why each limitation exists;
- Provide the reasons why each limitation could not be overcome using the method(s) chosen to acquire or gather the data [cite to other studies that had similar problems when possible];
- Assess the impact of each limitation in relation to the overall findings and conclusions of your study; and,
- If appropriate, describe how these limitations could point to the need for further research.
Remember that the method you chose may be the source of a significant limitation that has emerged during your interpretation of the results [for example, you didn't interview a group of people that you later wish you had]. If this is the case, don't panic. Acknowledge it, and explain how applying a different or more robust methodology might address the research problem more effectively in a future study. A underlying goal of scholarly research is not only to show what works, but to demonstrate what doesn't work or what needs further clarification.
Aguinis, Hermam and Jeffrey R. Edwards. “Methodological Wishes for the Next Decade and How to Make Wishes Come True.” Journal of Management Studies 51 (January 2014): 143-174; Brutus, Stéphane et al. "Self-Reported Limitations and Future Directions in Scholarly Reports: Analysis and Recommendations." Journal of Management 39 (January 2013): 48-75; Ioannidis, John P.A. "Limitations are not Properly Acknowledged in the Scientific Literature." Journal of Clinical Epidemiology 60 (2007): 324-329; Pasek, Josh. Writing the Empirical Social Science Research Paper: A Guide for the Perplexed. January 24, 2012. Academia.edu; Structure: How to Structure the Research Limitations Section of Your Dissertation. Dissertations and Theses: An Online Textbook. Laerd.com; What Is an Academic Paper? Institute for Writing Rhetoric. Dartmouth College; Writing the Experimental Report: Methods, Results, and Discussion. The Writing Lab and The OWL. Purdue University.
Writing Tip
Don't Inflate the Importance of Your Findings!
After all the hard work and long hours devoted to writing your research paper, it is easy to get carried away with attributing unwarranted importance to what you’ve done. We all want our academic work to be viewed as excellent and worthy of a good grade, but it is important that you understand and openly acknowledge the limitations of your study. Inflating the importance of your study's findings could be perceived by your readers as an attempt hide its flaws or encourage a biased interpretation of the results. A small measure of humility goes a long way!
Another Writing Tip
Negative Results are Not a Limitation!
Negative evidence refers to findings that unexpectedly challenge rather than support your hypothesis. If you didn't get the results you anticipated, it may mean your hypothesis was incorrect and needs to be reformulated. Or, perhaps you have stumbled onto something unexpected that warrants further study. Moreover, the absence of an effect may be very telling in many situations, particularly in experimental research designs. In any case, your results may very well be of importance to others even though they did not support your hypothesis. Do not fall into the trap of thinking that results contrary to what you expected is a limitation to your study. If you carried out the research well, they are simply your results and only require additional interpretation.
Lewis, George H. and Jonathan F. Lewis. “The Dog in the Night-Time: Negative Evidence in Social Research.” The British Journal of Sociology 31 (December 1980): 544-558.
Yet Another Writing Tip
Sample Size Limitations in Qualitative Research
Sample sizes are typically smaller in qualitative research because, as the study goes on, acquiring more data does not necessarily lead to more information. This is because one occurrence of a piece of data, or a code, is all that is necessary to ensure that it becomes part of the analysis framework. However, it remains true that sample sizes that are too small cannot adequately support claims of having achieved valid conclusions and sample sizes that are too large do not permit the deep, naturalistic, and inductive analysis that defines qualitative inquiry. Determining adequate sample size in qualitative research is ultimately a matter of judgment and experience in evaluating the quality of the information collected against the uses to which it will be applied and the particular research method and purposeful sampling strategy employed. If the sample size is found to be a limitation, it may reflect your judgment about the methodological technique chosen [e.g., single life history study versus focus group interviews] rather than the number of respondents used.
Boddy, Clive Roland. "Sample Size for Qualitative Research." Qualitative Market Research: An International Journal 19 (2016): 426-432; Huberman, A. Michael and Matthew B. Miles. "Data Management and Analysis Methods." In Handbook of Qualitative Research . Norman K. Denzin and Yvonna S. Lincoln, eds. (Thousand Oaks, CA: Sage, 1994), pp. 428-444; Blaikie, Norman. "Confounding Issues Related to Determining Sample Size in Qualitative Research." International Journal of Social Research Methodology 21 (2018): 635-641; Oppong, Steward Harrison. "The Problem of Sampling in qualitative Research." Asian Journal of Management Sciences and Education 2 (2013): 202-210.
- << Previous: 8. The Discussion
- Next: 9. The Conclusion >>
- Last Updated: Sep 4, 2024 9:40 AM
- URL: https://libguides.usc.edu/writingguide
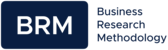
Research Limitations
It is for sure that your research will have some limitations and it is normal. However, it is critically important for you to be striving to minimize the range of scope of limitations throughout the research process. Also, you need to provide the acknowledgement of your research limitations in conclusions chapter honestly.
It is always better to identify and acknowledge shortcomings of your work, rather than to leave them pointed out to your by your dissertation assessor. While discussing your research limitations, don’t just provide the list and description of shortcomings of your work. It is also important for you to explain how these limitations have impacted your research findings.
Your research may have multiple limitations, but you need to discuss only those limitations that directly relate to your research problems. For example, if conducting a meta-analysis of the secondary data has not been stated as your research objective, no need to mention it as your research limitation.
Research limitations in a typical dissertation may relate to the following points:
1. Formulation of research aims and objectives . You might have formulated research aims and objectives too broadly. You can specify in which ways the formulation of research aims and objectives could be narrowed so that the level of focus of the study could be increased.
2. Implementation of data collection method . Because you do not have an extensive experience in primary data collection (otherwise you would not be reading this book), there is a great chance that the nature of implementation of data collection method is flawed.
3. Sample size. Sample size depends on the nature of the research problem. If sample size is too small, statistical tests would not be able to identify significant relationships within data set. You can state that basing your study in larger sample size could have generated more accurate results. The importance of sample size is greater in quantitative studies compared to qualitative studies.
4. Lack of previous studies in the research area . Literature review is an important part of any research, because it helps to identify the scope of works that have been done so far in research area. Literature review findings are used as the foundation for the researcher to be built upon to achieve her research objectives.
However, there may be little, if any, prior research on your topic if you have focused on the most contemporary and evolving research problem or too narrow research problem. For example, if you have chosen to explore the role of Bitcoins as the future currency, you may not be able to find tons of scholarly paper addressing the research problem, because Bitcoins are only a recent phenomenon.
5. Scope of discussions . You can include this point as a limitation of your research regardless of the choice of the research area. Because (most likely) you don’t have many years of experience of conducing researches and producing academic papers of such a large size individually, the scope and depth of discussions in your paper is compromised in many levels compared to the works of experienced scholars.
You can discuss certain points from your research limitations as the suggestion for further research at conclusions chapter of your dissertation.
My e-book, The Ultimate Guide to Writing a Dissertation in Business Studies: a step by step assistance offers practical assistance to complete a dissertation with minimum or no stress. The e-book covers all stages of writing a dissertation starting from the selection to the research area to submitting the completed version of the work within the deadline. John Dudovskiy
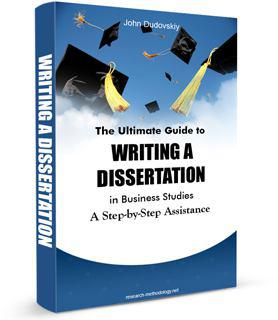
- Privacy Policy

Home » Research Methodology – Types, Examples and writing Guide
Research Methodology – Types, Examples and writing Guide
Table of Contents

Research Methodology
Definition:
Research Methodology refers to the systematic and scientific approach used to conduct research, investigate problems, and gather data and information for a specific purpose. It involves the techniques and procedures used to identify, collect , analyze , and interpret data to answer research questions or solve research problems . Moreover, They are philosophical and theoretical frameworks that guide the research process.
Structure of Research Methodology
Research methodology formats can vary depending on the specific requirements of the research project, but the following is a basic example of a structure for a research methodology section:
I. Introduction
- Provide an overview of the research problem and the need for a research methodology section
- Outline the main research questions and objectives
II. Research Design
- Explain the research design chosen and why it is appropriate for the research question(s) and objectives
- Discuss any alternative research designs considered and why they were not chosen
- Describe the research setting and participants (if applicable)
III. Data Collection Methods
- Describe the methods used to collect data (e.g., surveys, interviews, observations)
- Explain how the data collection methods were chosen and why they are appropriate for the research question(s) and objectives
- Detail any procedures or instruments used for data collection
IV. Data Analysis Methods
- Describe the methods used to analyze the data (e.g., statistical analysis, content analysis )
- Explain how the data analysis methods were chosen and why they are appropriate for the research question(s) and objectives
- Detail any procedures or software used for data analysis
V. Ethical Considerations
- Discuss any ethical issues that may arise from the research and how they were addressed
- Explain how informed consent was obtained (if applicable)
- Detail any measures taken to ensure confidentiality and anonymity
VI. Limitations
- Identify any potential limitations of the research methodology and how they may impact the results and conclusions
VII. Conclusion
- Summarize the key aspects of the research methodology section
- Explain how the research methodology addresses the research question(s) and objectives
Research Methodology Types
Types of Research Methodology are as follows:
Quantitative Research Methodology
This is a research methodology that involves the collection and analysis of numerical data using statistical methods. This type of research is often used to study cause-and-effect relationships and to make predictions.
Qualitative Research Methodology
This is a research methodology that involves the collection and analysis of non-numerical data such as words, images, and observations. This type of research is often used to explore complex phenomena, to gain an in-depth understanding of a particular topic, and to generate hypotheses.
Mixed-Methods Research Methodology
This is a research methodology that combines elements of both quantitative and qualitative research. This approach can be particularly useful for studies that aim to explore complex phenomena and to provide a more comprehensive understanding of a particular topic.
Case Study Research Methodology
This is a research methodology that involves in-depth examination of a single case or a small number of cases. Case studies are often used in psychology, sociology, and anthropology to gain a detailed understanding of a particular individual or group.
Action Research Methodology
This is a research methodology that involves a collaborative process between researchers and practitioners to identify and solve real-world problems. Action research is often used in education, healthcare, and social work.
Experimental Research Methodology
This is a research methodology that involves the manipulation of one or more independent variables to observe their effects on a dependent variable. Experimental research is often used to study cause-and-effect relationships and to make predictions.
Survey Research Methodology
This is a research methodology that involves the collection of data from a sample of individuals using questionnaires or interviews. Survey research is often used to study attitudes, opinions, and behaviors.
Grounded Theory Research Methodology
This is a research methodology that involves the development of theories based on the data collected during the research process. Grounded theory is often used in sociology and anthropology to generate theories about social phenomena.
Research Methodology Example
An Example of Research Methodology could be the following:
Research Methodology for Investigating the Effectiveness of Cognitive Behavioral Therapy in Reducing Symptoms of Depression in Adults
Introduction:
The aim of this research is to investigate the effectiveness of cognitive-behavioral therapy (CBT) in reducing symptoms of depression in adults. To achieve this objective, a randomized controlled trial (RCT) will be conducted using a mixed-methods approach.
Research Design:
The study will follow a pre-test and post-test design with two groups: an experimental group receiving CBT and a control group receiving no intervention. The study will also include a qualitative component, in which semi-structured interviews will be conducted with a subset of participants to explore their experiences of receiving CBT.
Participants:
Participants will be recruited from community mental health clinics in the local area. The sample will consist of 100 adults aged 18-65 years old who meet the diagnostic criteria for major depressive disorder. Participants will be randomly assigned to either the experimental group or the control group.
Intervention :
The experimental group will receive 12 weekly sessions of CBT, each lasting 60 minutes. The intervention will be delivered by licensed mental health professionals who have been trained in CBT. The control group will receive no intervention during the study period.
Data Collection:
Quantitative data will be collected through the use of standardized measures such as the Beck Depression Inventory-II (BDI-II) and the Generalized Anxiety Disorder-7 (GAD-7). Data will be collected at baseline, immediately after the intervention, and at a 3-month follow-up. Qualitative data will be collected through semi-structured interviews with a subset of participants from the experimental group. The interviews will be conducted at the end of the intervention period, and will explore participants’ experiences of receiving CBT.
Data Analysis:
Quantitative data will be analyzed using descriptive statistics, t-tests, and mixed-model analyses of variance (ANOVA) to assess the effectiveness of the intervention. Qualitative data will be analyzed using thematic analysis to identify common themes and patterns in participants’ experiences of receiving CBT.
Ethical Considerations:
This study will comply with ethical guidelines for research involving human subjects. Participants will provide informed consent before participating in the study, and their privacy and confidentiality will be protected throughout the study. Any adverse events or reactions will be reported and managed appropriately.
Data Management:
All data collected will be kept confidential and stored securely using password-protected databases. Identifying information will be removed from qualitative data transcripts to ensure participants’ anonymity.
Limitations:
One potential limitation of this study is that it only focuses on one type of psychotherapy, CBT, and may not generalize to other types of therapy or interventions. Another limitation is that the study will only include participants from community mental health clinics, which may not be representative of the general population.
Conclusion:
This research aims to investigate the effectiveness of CBT in reducing symptoms of depression in adults. By using a randomized controlled trial and a mixed-methods approach, the study will provide valuable insights into the mechanisms underlying the relationship between CBT and depression. The results of this study will have important implications for the development of effective treatments for depression in clinical settings.
How to Write Research Methodology
Writing a research methodology involves explaining the methods and techniques you used to conduct research, collect data, and analyze results. It’s an essential section of any research paper or thesis, as it helps readers understand the validity and reliability of your findings. Here are the steps to write a research methodology:
- Start by explaining your research question: Begin the methodology section by restating your research question and explaining why it’s important. This helps readers understand the purpose of your research and the rationale behind your methods.
- Describe your research design: Explain the overall approach you used to conduct research. This could be a qualitative or quantitative research design, experimental or non-experimental, case study or survey, etc. Discuss the advantages and limitations of the chosen design.
- Discuss your sample: Describe the participants or subjects you included in your study. Include details such as their demographics, sampling method, sample size, and any exclusion criteria used.
- Describe your data collection methods : Explain how you collected data from your participants. This could include surveys, interviews, observations, questionnaires, or experiments. Include details on how you obtained informed consent, how you administered the tools, and how you minimized the risk of bias.
- Explain your data analysis techniques: Describe the methods you used to analyze the data you collected. This could include statistical analysis, content analysis, thematic analysis, or discourse analysis. Explain how you dealt with missing data, outliers, and any other issues that arose during the analysis.
- Discuss the validity and reliability of your research : Explain how you ensured the validity and reliability of your study. This could include measures such as triangulation, member checking, peer review, or inter-coder reliability.
- Acknowledge any limitations of your research: Discuss any limitations of your study, including any potential threats to validity or generalizability. This helps readers understand the scope of your findings and how they might apply to other contexts.
- Provide a summary: End the methodology section by summarizing the methods and techniques you used to conduct your research. This provides a clear overview of your research methodology and helps readers understand the process you followed to arrive at your findings.
When to Write Research Methodology
Research methodology is typically written after the research proposal has been approved and before the actual research is conducted. It should be written prior to data collection and analysis, as it provides a clear roadmap for the research project.
The research methodology is an important section of any research paper or thesis, as it describes the methods and procedures that will be used to conduct the research. It should include details about the research design, data collection methods, data analysis techniques, and any ethical considerations.
The methodology should be written in a clear and concise manner, and it should be based on established research practices and standards. It is important to provide enough detail so that the reader can understand how the research was conducted and evaluate the validity of the results.
Applications of Research Methodology
Here are some of the applications of research methodology:
- To identify the research problem: Research methodology is used to identify the research problem, which is the first step in conducting any research.
- To design the research: Research methodology helps in designing the research by selecting the appropriate research method, research design, and sampling technique.
- To collect data: Research methodology provides a systematic approach to collect data from primary and secondary sources.
- To analyze data: Research methodology helps in analyzing the collected data using various statistical and non-statistical techniques.
- To test hypotheses: Research methodology provides a framework for testing hypotheses and drawing conclusions based on the analysis of data.
- To generalize findings: Research methodology helps in generalizing the findings of the research to the target population.
- To develop theories : Research methodology is used to develop new theories and modify existing theories based on the findings of the research.
- To evaluate programs and policies : Research methodology is used to evaluate the effectiveness of programs and policies by collecting data and analyzing it.
- To improve decision-making: Research methodology helps in making informed decisions by providing reliable and valid data.
Purpose of Research Methodology
Research methodology serves several important purposes, including:
- To guide the research process: Research methodology provides a systematic framework for conducting research. It helps researchers to plan their research, define their research questions, and select appropriate methods and techniques for collecting and analyzing data.
- To ensure research quality: Research methodology helps researchers to ensure that their research is rigorous, reliable, and valid. It provides guidelines for minimizing bias and error in data collection and analysis, and for ensuring that research findings are accurate and trustworthy.
- To replicate research: Research methodology provides a clear and detailed account of the research process, making it possible for other researchers to replicate the study and verify its findings.
- To advance knowledge: Research methodology enables researchers to generate new knowledge and to contribute to the body of knowledge in their field. It provides a means for testing hypotheses, exploring new ideas, and discovering new insights.
- To inform decision-making: Research methodology provides evidence-based information that can inform policy and decision-making in a variety of fields, including medicine, public health, education, and business.
Advantages of Research Methodology
Research methodology has several advantages that make it a valuable tool for conducting research in various fields. Here are some of the key advantages of research methodology:
- Systematic and structured approach : Research methodology provides a systematic and structured approach to conducting research, which ensures that the research is conducted in a rigorous and comprehensive manner.
- Objectivity : Research methodology aims to ensure objectivity in the research process, which means that the research findings are based on evidence and not influenced by personal bias or subjective opinions.
- Replicability : Research methodology ensures that research can be replicated by other researchers, which is essential for validating research findings and ensuring their accuracy.
- Reliability : Research methodology aims to ensure that the research findings are reliable, which means that they are consistent and can be depended upon.
- Validity : Research methodology ensures that the research findings are valid, which means that they accurately reflect the research question or hypothesis being tested.
- Efficiency : Research methodology provides a structured and efficient way of conducting research, which helps to save time and resources.
- Flexibility : Research methodology allows researchers to choose the most appropriate research methods and techniques based on the research question, data availability, and other relevant factors.
- Scope for innovation: Research methodology provides scope for innovation and creativity in designing research studies and developing new research techniques.
Research Methodology Vs Research Methods
Research Methodology | Research Methods |
---|---|
Research methodology refers to the philosophical and theoretical frameworks that guide the research process. | refer to the techniques and procedures used to collect and analyze data. |
It is concerned with the underlying principles and assumptions of research. | It is concerned with the practical aspects of research. |
It provides a rationale for why certain research methods are used. | It determines the specific steps that will be taken to conduct research. |
It is broader in scope and involves understanding the overall approach to research. | It is narrower in scope and focuses on specific techniques and tools used in research. |
It is concerned with identifying research questions, defining the research problem, and formulating hypotheses. | It is concerned with collecting data, analyzing data, and interpreting results. |
It is concerned with the validity and reliability of research. | It is concerned with the accuracy and precision of data. |
It is concerned with the ethical considerations of research. | It is concerned with the practical considerations of research. |
About the author
Muhammad Hassan
Researcher, Academic Writer, Web developer
You may also like

Thesis Outline – Example, Template and Writing...

Research Design – Types, Methods and Examples

Chapter Summary & Overview – Writing Guide...

Research Gap – Types, Examples and How to...

Thesis Statement – Examples, Writing Guide

Thesis Format – Templates and Samples

Research Limitations 101 📖
A Plain-Language Explainer (With Practical Examples)
By: Derek Jansen (MBA) | Expert Reviewer: Dr. Eunice Rautenbach | May 2024
Research limitations are one of those things that students tend to avoid digging into, and understandably so. No one likes to critique their own study and point out weaknesses. Nevertheless, being able to understand the limitations of your study – and, just as importantly, the implications thereof – a is a critically important skill.
In this post, we’ll unpack some of the most common research limitations you’re likely to encounter, so that you can approach your project with confidence.
Overview: Research Limitations 101
- What are research limitations ?
- Access – based limitations
- Temporal & financial limitations
- Sample & sampling limitations
- Design limitations
- Researcher limitations
- Key takeaways
What (exactly) are “research limitations”?
At the simplest level, research limitations (also referred to as “the limitations of the study”) are the constraints and challenges that will invariably influence your ability to conduct your study and draw reliable conclusions .
Research limitations are inevitable. Absolutely no study is perfect and limitations are an inherent part of any research design. These limitations can stem from a variety of sources , including access to data, methodological choices, and the more mundane constraints of budget and time. So, there’s no use trying to escape them – what matters is that you can recognise them.
Acknowledging and understanding these limitations is crucial, not just for the integrity of your research, but also for your development as a scholar. That probably sounds a bit rich, but realistically, having a strong understanding of the limitations of any given study helps you handle the inevitable obstacles professionally and transparently, which in turn builds trust with your audience and academic peers.
Simply put, recognising and discussing the limitations of your study demonstrates that you know what you’re doing , and that you’ve considered the results of your project within the context of these limitations. In other words, discussing the limitations is a sign of credibility and strength – not weakness. Contrary to the common misconception, highlighting your limitations (or rather, your study’s limitations) will earn you (rather than cost you) marks.
So, with that foundation laid, let’s have a look at some of the most common research limitations you’re likely to encounter – and how to go about managing them as effectively as possible.
Need a helping hand?
Limitation #1: Access To Information
One of the first hurdles you might encounter is limited access to necessary information. For example, you may have trouble getting access to specific literature or niche data sets. This situation can manifest due to several reasons, including paywalls, copyright and licensing issues or language barriers.
To minimise situations like these, it’s useful to try to leverage your university’s resource pool to the greatest extent possible. In practical terms, this means engaging with your university’s librarian and/or potentially utilising interlibrary loans to get access to restricted resources. If this sounds foreign to you, have a chat with your librarian 🙃
In emerging fields or highly specific study areas, you might find that there’s very little existing research (i.e., literature) on your topic. This scenario, while challenging, also offers a unique opportunity to contribute significantly to your field , as it indicates that there’s a significant research gap .
All of that said, be sure to conduct an exhaustive search using a variety of keywords and Boolean operators before assuming that there’s a lack of literature. Also, remember to snowball your literature base . In other words, scan the reference lists of the handful of papers that are directly relevant and then scan those references for more sources. You can also consider using tools like Litmaps and Connected Papers (see video below).
Limitation #2: Time & Money
Almost every researcher will face time and budget constraints at some point. Naturally, these limitations can affect the depth and breadth of your research – but they don’t need to be a death sentence.
Effective planning is crucial to managing both the temporal and financial aspects of your study. In practical terms, utilising tools like Gantt charts can help you visualise and plan your research timeline realistically, thereby reducing the risk of any nasty surprises. Always take a conservative stance when it comes to timelines, especially if you’re new to academic research. As a rule of thumb, things will generally take twice as long as you expect – so, prepare for the worst-case scenario.
If budget is a concern, you might want to consider exploring small research grants or adjusting the scope of your study so that it fits within a realistic budget. Trimming back might sound unattractive, but keep in mind that a smaller, well-planned study can often be more impactful than a larger, poorly planned project.
If you find yourself in a position where you’ve already run out of cash, don’t panic. There’s usually a pivot opportunity hidden somewhere within your project. Engage with your research advisor or faculty to explore potential solutions – don’t make any major changes without first consulting your institution.
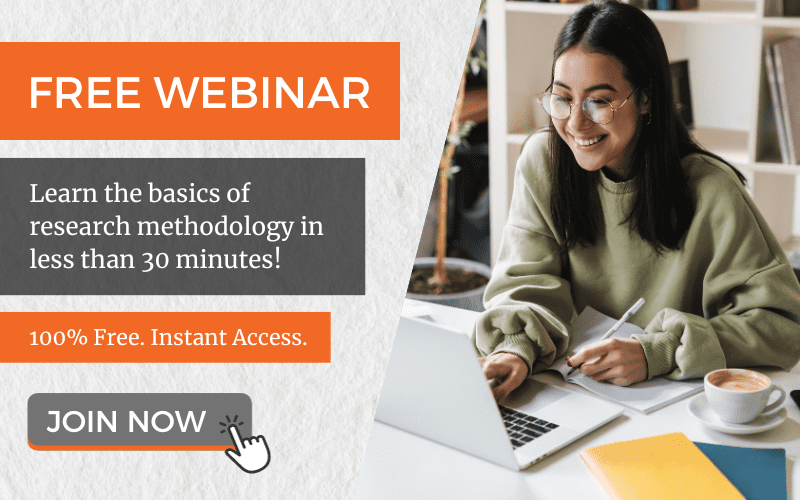
Limitation #3: Sample Size & Composition
As we’ve discussed before , the size and representativeness of your sample are crucial , especially in quantitative research where the robustness of your conclusions often depends on these factors. All too often though, students run into issues achieving a sufficient sample size and composition.
To ensure adequacy in terms of your sample size, it’s important to plan for potential dropouts by oversampling from the outset . In other words, if you aim for a final sample size of 100 participants, aim to recruit 120-140 to account for unexpected challenges. If you still find yourself short on participants, consider whether you could complement your dataset with secondary data or data from an adjacent sample – for example, participants from another city or country. That said, be sure to engage with your research advisor before making any changes to your approach.
A related issue that you may run into is sample composition. In other words, you may have trouble securing a random sample that’s representative of your population of interest. In cases like this, you might again want to look at ways to complement your dataset with other sources, but if that’s not possible, it’s not the end of the world. As with all limitations, you’ll just need to recognise this limitation in your final write-up and be sure to interpret your results accordingly. In other words, don’t claim generalisability of your results if your sample isn’t random.
Limitation #4: Methodological Limitations
As we alluded earlier, every methodological choice comes with its own set of limitations . For example, you can’t claim causality if you’re using a descriptive or correlational research design. Similarly, as we saw in the previous example, you can’t claim generalisability if you’re using a non-random sampling approach.
Making good methodological choices is all about understanding (and accepting) the inherent trade-offs . In the vast majority of cases, you won’t be able to adopt the “perfect” methodology – and that’s okay. What’s important is that you select a methodology that aligns with your research aims and research questions , as well as the practical constraints at play (e.g., time, money, equipment access, etc.). Just as importantly, you must recognise and articulate the limitations of your chosen methods, and justify why they were the most suitable, given your specific context.
Limitation #5: Researcher (In)experience
A discussion about research limitations would not be complete without mentioning the researcher (that’s you!). Whether we like to admit it or not, researcher inexperience and personal biases can subtly (and sometimes not so subtly) influence the interpretation and presentation of data within a study. This is especially true when it comes to dissertations and theses , as these are most commonly undertaken by first-time (or relatively fresh) researchers.
When it comes to dealing with this specific limitation, it’s important to remember the adage “ We don’t know what we don’t know ”. In other words, recognise and embrace your (relative) ignorance and subjectivity – and interpret your study’s results within that context . Simply put, don’t be overly confident in drawing conclusions from your study – especially when they contradict existing literature.
Cultivating a culture of reflexivity within your research practices can help reduce subjectivity and keep you a bit more “rooted” in the data. In practical terms, this simply means making an effort to become aware of how your perspectives and experiences may have shaped the research process and outcomes.
As with any new endeavour in life, it’s useful to garner as many outsider perspectives as possible. Of course, your university-assigned research advisor will play a large role in this respect, but it’s also a good idea to seek out feedback and critique from other academics. To this end, you might consider approaching other faculty at your institution, joining an online group, or even working with a private coach .
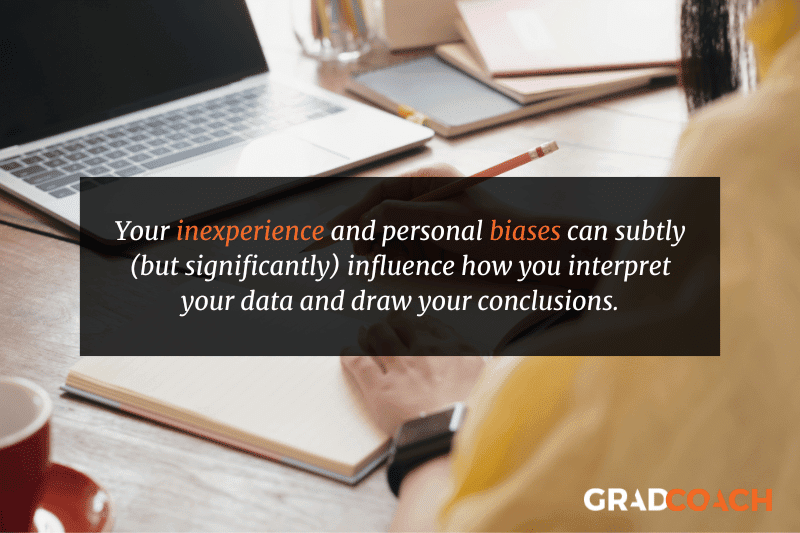
Key Takeaways
Understanding and effectively navigating research limitations is key to conducting credible and reliable academic work. By acknowledging and addressing these limitations upfront, you not only enhance the integrity of your research, but also demonstrate your academic maturity and professionalism.
Whether you’re working on a dissertation, thesis or any other type of formal academic research, remember the five most common research limitations and interpret your data while keeping them in mind.
- Access to Information (literature and data)
- Time and money
- Sample size and composition
- Research design and methodology
- Researcher (in)experience and bias
If you need a hand identifying and mitigating the limitations within your study, check out our 1:1 private coaching service .
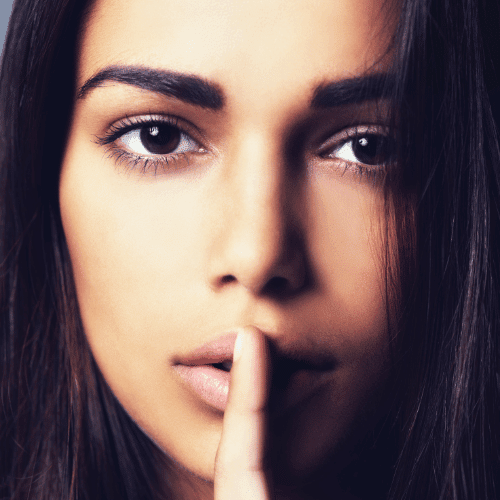
Psst… there’s more!
This post is an extract from our bestselling short course, Methodology Bootcamp . If you want to work smart, you don't want to miss this .
Submit a Comment Cancel reply
Your email address will not be published. Required fields are marked *
Save my name, email, and website in this browser for the next time I comment.
- Print Friendly
Instant insights, infinite possibilities
How to present limitations in research
Last updated
30 January 2024
Reviewed by
Short on time? Get an AI generated summary of this article instead
Limitations don’t invalidate or diminish your results, but it’s best to acknowledge them. This will enable you to address any questions your study failed to answer because of them.
In this guide, learn how to recognize, present, and overcome limitations in research.
- What is a research limitation?
Research limitations are weaknesses in your research design or execution that may have impacted outcomes and conclusions. Uncovering limitations doesn’t necessarily indicate poor research design—it just means you encountered challenges you couldn’t have anticipated that limited your research efforts.
Does basic research have limitations?
Basic research aims to provide more information about your research topic . It requires the same standard research methodology and data collection efforts as any other research type, and it can also have limitations.
- Common research limitations
Researchers encounter common limitations when embarking on a study. Limitations can occur in relation to the methods you apply or the research process you design. They could also be connected to you as the researcher.
Methodology limitations
Not having access to data or reliable information can impact the methods used to facilitate your research. A lack of data or reliability may limit the parameters of your study area and the extent of your exploration.
Your sample size may also be affected because you won’t have any direction on how big or small it should be and who or what you should include. Having too few participants won’t adequately represent the population or groups of people needed to draw meaningful conclusions.
Research process limitations
The study’s design can impose constraints on the process. For example, as you’re conducting the research, issues may arise that don’t conform to the data collection methodology you developed. You may not realize until well into the process that you should have incorporated more specific questions or comprehensive experiments to generate the data you need to have confidence in your results.
Constraints on resources can also have an impact. Being limited on participants or participation incentives may limit your sample sizes. Insufficient tools, equipment, and materials to conduct a thorough study may also be a factor.
Common researcher limitations
Here are some of the common researcher limitations you may encounter:
Time: some research areas require multi-year longitudinal approaches, but you might not be able to dedicate that much time. Imagine you want to measure how much memory a person loses as they age. This may involve conducting multiple tests on a sample of participants over 20–30 years, which may be impossible.
Bias: researchers can consciously or unconsciously apply bias to their research. Biases can contribute to relying on research sources and methodologies that will only support your beliefs about the research you’re embarking on. You might also omit relevant issues or participants from the scope of your study because of your biases.
Limited access to data : you may need to pay to access specific databases or journals that would be helpful to your research process. You might also need to gain information from certain people or organizations but have limited access to them. These cases require readjusting your process and explaining why your findings are still reliable.
- Why is it important to identify limitations?
Identifying limitations adds credibility to research and provides a deeper understanding of how you arrived at your conclusions.
Constraints may have prevented you from collecting specific data or information you hoped would prove or disprove your hypothesis or provide a more comprehensive understanding of your research topic.
However, identifying the limitations contributing to your conclusions can inspire further research efforts that help gather more substantial information and data.
- Where to put limitations in a research paper
A research paper is broken up into different sections that appear in the following order:
Introduction
Methodology
The discussion portion of your paper explores your findings and puts them in the context of the overall research. Either place research limitations at the beginning of the discussion section before the analysis of your findings or at the end of the section to indicate that further research needs to be pursued.
What not to include in the limitations section
Evidence that doesn’t support your hypothesis is not a limitation, so you shouldn’t include it in the limitation section. Don’t just list limitations and their degree of severity without further explanation.
- How to present limitations
You’ll want to present the limitations of your study in a way that doesn’t diminish the validity of your research and leave the reader wondering if your results and conclusions have been compromised.
Include only the limitations that directly relate to and impact how you addressed your research questions. Following a specific format enables the reader to develop an understanding of the weaknesses within the context of your findings without doubting the quality and integrity of your research.
Identify the limitations specific to your study
You don’t have to identify every possible limitation that might have occurred during your research process. Only identify those that may have influenced the quality of your findings and your ability to answer your research question.
Explain study limitations in detail
This explanation should be the most significant portion of your limitation section.
Link each limitation with an interpretation and appraisal of their impact on the study. You’ll have to evaluate and explain whether the error, method, or validity issues influenced the study’s outcome and how.
Propose a direction for future studies and present alternatives
In this section, suggest how researchers can avoid the pitfalls you experienced during your research process.
If an issue with methodology was a limitation, propose alternate methods that may help with a smoother and more conclusive research project . Discuss the pros and cons of your alternate recommendation.
Describe steps taken to minimize each limitation
You probably took steps to try to address or mitigate limitations when you noticed them throughout the course of your research project. Describe these steps in the limitation section.
- Limitation example
“Approaches like stem cell transplantation and vaccination in AD [Alzheimer’s disease] work on a cellular or molecular level in the laboratory. However, translation into clinical settings will remain a challenge for the next decade.”
The authors are saying that even though these methods showed promise in helping people with memory loss when conducted in the lab (in other words, using animal studies), more studies are needed. These may be controlled clinical trials, for example.
However, the short life span of stem cells outside the lab and the vaccination’s severe inflammatory side effects are limitations. Researchers won’t be able to conduct clinical trials until these issues are overcome.
- How to overcome limitations in research
You’ve already started on the road to overcoming limitations in research by acknowledging that they exist. However, you need to ensure readers don’t mistake weaknesses for errors within your research design.
To do this, you’ll need to justify and explain your rationale for the methods, research design, and analysis tools you chose and how you noticed they may have presented limitations.
Your readers need to know that even when limitations presented themselves, you followed best practices and the ethical standards of your field. You didn’t violate any rules and regulations during your research process.
You’ll also want to reinforce the validity of your conclusions and results with multiple sources, methods, and perspectives. This prevents readers from assuming your findings were derived from a single or biased source.
- Learning and improving starts with limitations in research
Dealing with limitations with transparency and integrity helps identify areas for future improvements and developments. It’s a learning process, providing valuable insights into how you can improve methodologies, expand sample sizes, or explore alternate approaches to further support the validity of your findings.
Should you be using a customer insights hub?
Do you want to discover previous research faster?
Do you share your research findings with others?
Do you analyze research data?
Start for free today, add your research, and get to key insights faster
Editor’s picks
Last updated: 18 April 2023
Last updated: 27 February 2023
Last updated: 22 August 2024
Last updated: 5 February 2023
Last updated: 16 August 2024
Last updated: 9 March 2023
Last updated: 30 April 2024
Last updated: 12 December 2023
Last updated: 11 March 2024
Last updated: 4 July 2024
Last updated: 6 March 2024
Last updated: 5 March 2024
Last updated: 13 May 2024
Latest articles
Related topics, .css-je19u9{-webkit-align-items:flex-end;-webkit-box-align:flex-end;-ms-flex-align:flex-end;align-items:flex-end;display:-webkit-box;display:-webkit-flex;display:-ms-flexbox;display:flex;-webkit-flex-direction:row;-ms-flex-direction:row;flex-direction:row;-webkit-box-flex-wrap:wrap;-webkit-flex-wrap:wrap;-ms-flex-wrap:wrap;flex-wrap:wrap;-webkit-box-pack:center;-ms-flex-pack:center;-webkit-justify-content:center;justify-content:center;row-gap:0;text-align:center;max-width:671px;}@media (max-width: 1079px){.css-je19u9{max-width:400px;}.css-je19u9>span{white-space:pre;}}@media (max-width: 799px){.css-je19u9{max-width:400px;}.css-je19u9>span{white-space:pre;}} decide what to .css-1kiodld{max-height:56px;display:-webkit-box;display:-webkit-flex;display:-ms-flexbox;display:flex;-webkit-align-items:center;-webkit-box-align:center;-ms-flex-align:center;align-items:center;}@media (max-width: 1079px){.css-1kiodld{display:none;}} build next, decide what to build next, log in or sign up.
Get started for free

An official website of the United States government
The .gov means it’s official. Federal government websites often end in .gov or .mil. Before sharing sensitive information, make sure you’re on a federal government site.
The site is secure. The https:// ensures that you are connecting to the official website and that any information you provide is encrypted and transmitted securely.
- Publications
- Account settings
Preview improvements coming to the PMC website in October 2024. Learn More or Try it out now .
- Advanced Search
- Journal List
- BMC Med Res Methodol

A tutorial on methodological studies: the what, when, how and why
Lawrence mbuagbaw.
1 Department of Health Research Methods, Evidence and Impact, McMaster University, Hamilton, ON Canada
2 Biostatistics Unit/FSORC, 50 Charlton Avenue East, St Joseph’s Healthcare—Hamilton, 3rd Floor Martha Wing, Room H321, Hamilton, Ontario L8N 4A6 Canada
3 Centre for the Development of Best Practices in Health, Yaoundé, Cameroon
Daeria O. Lawson
Livia puljak.
4 Center for Evidence-Based Medicine and Health Care, Catholic University of Croatia, Ilica 242, 10000 Zagreb, Croatia
David B. Allison
5 Department of Epidemiology and Biostatistics, School of Public Health – Bloomington, Indiana University, Bloomington, IN 47405 USA
Lehana Thabane
6 Departments of Paediatrics and Anaesthesia, McMaster University, Hamilton, ON Canada
7 Centre for Evaluation of Medicine, St. Joseph’s Healthcare-Hamilton, Hamilton, ON Canada
8 Population Health Research Institute, Hamilton Health Sciences, Hamilton, ON Canada
Associated Data
Data sharing is not applicable to this article as no new data were created or analyzed in this study.
Methodological studies – studies that evaluate the design, analysis or reporting of other research-related reports – play an important role in health research. They help to highlight issues in the conduct of research with the aim of improving health research methodology, and ultimately reducing research waste.
We provide an overview of some of the key aspects of methodological studies such as what they are, and when, how and why they are done. We adopt a “frequently asked questions” format to facilitate reading this paper and provide multiple examples to help guide researchers interested in conducting methodological studies. Some of the topics addressed include: is it necessary to publish a study protocol? How to select relevant research reports and databases for a methodological study? What approaches to data extraction and statistical analysis should be considered when conducting a methodological study? What are potential threats to validity and is there a way to appraise the quality of methodological studies?
Appropriate reflection and application of basic principles of epidemiology and biostatistics are required in the design and analysis of methodological studies. This paper provides an introduction for further discussion about the conduct of methodological studies.
The field of meta-research (or research-on-research) has proliferated in recent years in response to issues with research quality and conduct [ 1 – 3 ]. As the name suggests, this field targets issues with research design, conduct, analysis and reporting. Various types of research reports are often examined as the unit of analysis in these studies (e.g. abstracts, full manuscripts, trial registry entries). Like many other novel fields of research, meta-research has seen a proliferation of use before the development of reporting guidance. For example, this was the case with randomized trials for which risk of bias tools and reporting guidelines were only developed much later – after many trials had been published and noted to have limitations [ 4 , 5 ]; and for systematic reviews as well [ 6 – 8 ]. However, in the absence of formal guidance, studies that report on research differ substantially in how they are named, conducted and reported [ 9 , 10 ]. This creates challenges in identifying, summarizing and comparing them. In this tutorial paper, we will use the term methodological study to refer to any study that reports on the design, conduct, analysis or reporting of primary or secondary research-related reports (such as trial registry entries and conference abstracts).
In the past 10 years, there has been an increase in the use of terms related to methodological studies (based on records retrieved with a keyword search [in the title and abstract] for “methodological review” and “meta-epidemiological study” in PubMed up to December 2019), suggesting that these studies may be appearing more frequently in the literature. See Fig. 1 .

Trends in the number studies that mention “methodological review” or “meta-
epidemiological study” in PubMed.
The methods used in many methodological studies have been borrowed from systematic and scoping reviews. This practice has influenced the direction of the field, with many methodological studies including searches of electronic databases, screening of records, duplicate data extraction and assessments of risk of bias in the included studies. However, the research questions posed in methodological studies do not always require the approaches listed above, and guidance is needed on when and how to apply these methods to a methodological study. Even though methodological studies can be conducted on qualitative or mixed methods research, this paper focuses on and draws examples exclusively from quantitative research.
The objectives of this paper are to provide some insights on how to conduct methodological studies so that there is greater consistency between the research questions posed, and the design, analysis and reporting of findings. We provide multiple examples to illustrate concepts and a proposed framework for categorizing methodological studies in quantitative research.
What is a methodological study?
Any study that describes or analyzes methods (design, conduct, analysis or reporting) in published (or unpublished) literature is a methodological study. Consequently, the scope of methodological studies is quite extensive and includes, but is not limited to, topics as diverse as: research question formulation [ 11 ]; adherence to reporting guidelines [ 12 – 14 ] and consistency in reporting [ 15 ]; approaches to study analysis [ 16 ]; investigating the credibility of analyses [ 17 ]; and studies that synthesize these methodological studies [ 18 ]. While the nomenclature of methodological studies is not uniform, the intents and purposes of these studies remain fairly consistent – to describe or analyze methods in primary or secondary studies. As such, methodological studies may also be classified as a subtype of observational studies.
Parallel to this are experimental studies that compare different methods. Even though they play an important role in informing optimal research methods, experimental methodological studies are beyond the scope of this paper. Examples of such studies include the randomized trials by Buscemi et al., comparing single data extraction to double data extraction [ 19 ], and Carrasco-Labra et al., comparing approaches to presenting findings in Grading of Recommendations, Assessment, Development and Evaluations (GRADE) summary of findings tables [ 20 ]. In these studies, the unit of analysis is the person or groups of individuals applying the methods. We also direct readers to the Studies Within a Trial (SWAT) and Studies Within a Review (SWAR) programme operated through the Hub for Trials Methodology Research, for further reading as a potential useful resource for these types of experimental studies [ 21 ]. Lastly, this paper is not meant to inform the conduct of research using computational simulation and mathematical modeling for which some guidance already exists [ 22 ], or studies on the development of methods using consensus-based approaches.
When should we conduct a methodological study?
Methodological studies occupy a unique niche in health research that allows them to inform methodological advances. Methodological studies should also be conducted as pre-cursors to reporting guideline development, as they provide an opportunity to understand current practices, and help to identify the need for guidance and gaps in methodological or reporting quality. For example, the development of the popular Preferred Reporting Items of Systematic reviews and Meta-Analyses (PRISMA) guidelines were preceded by methodological studies identifying poor reporting practices [ 23 , 24 ]. In these instances, after the reporting guidelines are published, methodological studies can also be used to monitor uptake of the guidelines.
These studies can also be conducted to inform the state of the art for design, analysis and reporting practices across different types of health research fields, with the aim of improving research practices, and preventing or reducing research waste. For example, Samaan et al. conducted a scoping review of adherence to different reporting guidelines in health care literature [ 18 ]. Methodological studies can also be used to determine the factors associated with reporting practices. For example, Abbade et al. investigated journal characteristics associated with the use of the Participants, Intervention, Comparison, Outcome, Timeframe (PICOT) format in framing research questions in trials of venous ulcer disease [ 11 ].
How often are methodological studies conducted?
There is no clear answer to this question. Based on a search of PubMed, the use of related terms (“methodological review” and “meta-epidemiological study”) – and therefore, the number of methodological studies – is on the rise. However, many other terms are used to describe methodological studies. There are also many studies that explore design, conduct, analysis or reporting of research reports, but that do not use any specific terms to describe or label their study design in terms of “methodology”. This diversity in nomenclature makes a census of methodological studies elusive. Appropriate terminology and key words for methodological studies are needed to facilitate improved accessibility for end-users.
Why do we conduct methodological studies?
Methodological studies provide information on the design, conduct, analysis or reporting of primary and secondary research and can be used to appraise quality, quantity, completeness, accuracy and consistency of health research. These issues can be explored in specific fields, journals, databases, geographical regions and time periods. For example, Areia et al. explored the quality of reporting of endoscopic diagnostic studies in gastroenterology [ 25 ]; Knol et al. investigated the reporting of p -values in baseline tables in randomized trial published in high impact journals [ 26 ]; Chen et al. describe adherence to the Consolidated Standards of Reporting Trials (CONSORT) statement in Chinese Journals [ 27 ]; and Hopewell et al. describe the effect of editors’ implementation of CONSORT guidelines on reporting of abstracts over time [ 28 ]. Methodological studies provide useful information to researchers, clinicians, editors, publishers and users of health literature. As a result, these studies have been at the cornerstone of important methodological developments in the past two decades and have informed the development of many health research guidelines including the highly cited CONSORT statement [ 5 ].
Where can we find methodological studies?
Methodological studies can be found in most common biomedical bibliographic databases (e.g. Embase, MEDLINE, PubMed, Web of Science). However, the biggest caveat is that methodological studies are hard to identify in the literature due to the wide variety of names used and the lack of comprehensive databases dedicated to them. A handful can be found in the Cochrane Library as “Cochrane Methodology Reviews”, but these studies only cover methodological issues related to systematic reviews. Previous attempts to catalogue all empirical studies of methods used in reviews were abandoned 10 years ago [ 29 ]. In other databases, a variety of search terms may be applied with different levels of sensitivity and specificity.
Some frequently asked questions about methodological studies
In this section, we have outlined responses to questions that might help inform the conduct of methodological studies.
Q: How should I select research reports for my methodological study?
A: Selection of research reports for a methodological study depends on the research question and eligibility criteria. Once a clear research question is set and the nature of literature one desires to review is known, one can then begin the selection process. Selection may begin with a broad search, especially if the eligibility criteria are not apparent. For example, a methodological study of Cochrane Reviews of HIV would not require a complex search as all eligible studies can easily be retrieved from the Cochrane Library after checking a few boxes [ 30 ]. On the other hand, a methodological study of subgroup analyses in trials of gastrointestinal oncology would require a search to find such trials, and further screening to identify trials that conducted a subgroup analysis [ 31 ].
The strategies used for identifying participants in observational studies can apply here. One may use a systematic search to identify all eligible studies. If the number of eligible studies is unmanageable, a random sample of articles can be expected to provide comparable results if it is sufficiently large [ 32 ]. For example, Wilson et al. used a random sample of trials from the Cochrane Stroke Group’s Trial Register to investigate completeness of reporting [ 33 ]. It is possible that a simple random sample would lead to underrepresentation of units (i.e. research reports) that are smaller in number. This is relevant if the investigators wish to compare multiple groups but have too few units in one group. In this case a stratified sample would help to create equal groups. For example, in a methodological study comparing Cochrane and non-Cochrane reviews, Kahale et al. drew random samples from both groups [ 34 ]. Alternatively, systematic or purposeful sampling strategies can be used and we encourage researchers to justify their selected approaches based on the study objective.
Q: How many databases should I search?
A: The number of databases one should search would depend on the approach to sampling, which can include targeting the entire “population” of interest or a sample of that population. If you are interested in including the entire target population for your research question, or drawing a random or systematic sample from it, then a comprehensive and exhaustive search for relevant articles is required. In this case, we recommend using systematic approaches for searching electronic databases (i.e. at least 2 databases with a replicable and time stamped search strategy). The results of your search will constitute a sampling frame from which eligible studies can be drawn.
Alternatively, if your approach to sampling is purposeful, then we recommend targeting the database(s) or data sources (e.g. journals, registries) that include the information you need. For example, if you are conducting a methodological study of high impact journals in plastic surgery and they are all indexed in PubMed, you likely do not need to search any other databases. You may also have a comprehensive list of all journals of interest and can approach your search using the journal names in your database search (or by accessing the journal archives directly from the journal’s website). Even though one could also search journals’ web pages directly, using a database such as PubMed has multiple advantages, such as the use of filters, so the search can be narrowed down to a certain period, or study types of interest. Furthermore, individual journals’ web sites may have different search functionalities, which do not necessarily yield a consistent output.
Q: Should I publish a protocol for my methodological study?
A: A protocol is a description of intended research methods. Currently, only protocols for clinical trials require registration [ 35 ]. Protocols for systematic reviews are encouraged but no formal recommendation exists. The scientific community welcomes the publication of protocols because they help protect against selective outcome reporting, the use of post hoc methodologies to embellish results, and to help avoid duplication of efforts [ 36 ]. While the latter two risks exist in methodological research, the negative consequences may be substantially less than for clinical outcomes. In a sample of 31 methodological studies, 7 (22.6%) referenced a published protocol [ 9 ]. In the Cochrane Library, there are 15 protocols for methodological reviews (21 July 2020). This suggests that publishing protocols for methodological studies is not uncommon.
Authors can consider publishing their study protocol in a scholarly journal as a manuscript. Advantages of such publication include obtaining peer-review feedback about the planned study, and easy retrieval by searching databases such as PubMed. The disadvantages in trying to publish protocols includes delays associated with manuscript handling and peer review, as well as costs, as few journals publish study protocols, and those journals mostly charge article-processing fees [ 37 ]. Authors who would like to make their protocol publicly available without publishing it in scholarly journals, could deposit their study protocols in publicly available repositories, such as the Open Science Framework ( https://osf.io/ ).
Q: How to appraise the quality of a methodological study?
A: To date, there is no published tool for appraising the risk of bias in a methodological study, but in principle, a methodological study could be considered as a type of observational study. Therefore, during conduct or appraisal, care should be taken to avoid the biases common in observational studies [ 38 ]. These biases include selection bias, comparability of groups, and ascertainment of exposure or outcome. In other words, to generate a representative sample, a comprehensive reproducible search may be necessary to build a sampling frame. Additionally, random sampling may be necessary to ensure that all the included research reports have the same probability of being selected, and the screening and selection processes should be transparent and reproducible. To ensure that the groups compared are similar in all characteristics, matching, random sampling or stratified sampling can be used. Statistical adjustments for between-group differences can also be applied at the analysis stage. Finally, duplicate data extraction can reduce errors in assessment of exposures or outcomes.
Q: Should I justify a sample size?
A: In all instances where one is not using the target population (i.e. the group to which inferences from the research report are directed) [ 39 ], a sample size justification is good practice. The sample size justification may take the form of a description of what is expected to be achieved with the number of articles selected, or a formal sample size estimation that outlines the number of articles required to answer the research question with a certain precision and power. Sample size justifications in methodological studies are reasonable in the following instances:
- Comparing two groups
- Determining a proportion, mean or another quantifier
- Determining factors associated with an outcome using regression-based analyses
For example, El Dib et al. computed a sample size requirement for a methodological study of diagnostic strategies in randomized trials, based on a confidence interval approach [ 40 ].
Q: What should I call my study?
A: Other terms which have been used to describe/label methodological studies include “ methodological review ”, “methodological survey” , “meta-epidemiological study” , “systematic review” , “systematic survey”, “meta-research”, “research-on-research” and many others. We recommend that the study nomenclature be clear, unambiguous, informative and allow for appropriate indexing. Methodological study nomenclature that should be avoided includes “ systematic review” – as this will likely be confused with a systematic review of a clinical question. “ Systematic survey” may also lead to confusion about whether the survey was systematic (i.e. using a preplanned methodology) or a survey using “ systematic” sampling (i.e. a sampling approach using specific intervals to determine who is selected) [ 32 ]. Any of the above meanings of the words “ systematic” may be true for methodological studies and could be potentially misleading. “ Meta-epidemiological study” is ideal for indexing, but not very informative as it describes an entire field. The term “ review ” may point towards an appraisal or “review” of the design, conduct, analysis or reporting (or methodological components) of the targeted research reports, yet it has also been used to describe narrative reviews [ 41 , 42 ]. The term “ survey ” is also in line with the approaches used in many methodological studies [ 9 ], and would be indicative of the sampling procedures of this study design. However, in the absence of guidelines on nomenclature, the term “ methodological study ” is broad enough to capture most of the scenarios of such studies.
Q: Should I account for clustering in my methodological study?
A: Data from methodological studies are often clustered. For example, articles coming from a specific source may have different reporting standards (e.g. the Cochrane Library). Articles within the same journal may be similar due to editorial practices and policies, reporting requirements and endorsement of guidelines. There is emerging evidence that these are real concerns that should be accounted for in analyses [ 43 ]. Some cluster variables are described in the section: “ What variables are relevant to methodological studies?”
A variety of modelling approaches can be used to account for correlated data, including the use of marginal, fixed or mixed effects regression models with appropriate computation of standard errors [ 44 ]. For example, Kosa et al. used generalized estimation equations to account for correlation of articles within journals [ 15 ]. Not accounting for clustering could lead to incorrect p -values, unduly narrow confidence intervals, and biased estimates [ 45 ].
Q: Should I extract data in duplicate?
A: Yes. Duplicate data extraction takes more time but results in less errors [ 19 ]. Data extraction errors in turn affect the effect estimate [ 46 ], and therefore should be mitigated. Duplicate data extraction should be considered in the absence of other approaches to minimize extraction errors. However, much like systematic reviews, this area will likely see rapid new advances with machine learning and natural language processing technologies to support researchers with screening and data extraction [ 47 , 48 ]. However, experience plays an important role in the quality of extracted data and inexperienced extractors should be paired with experienced extractors [ 46 , 49 ].
Q: Should I assess the risk of bias of research reports included in my methodological study?
A : Risk of bias is most useful in determining the certainty that can be placed in the effect measure from a study. In methodological studies, risk of bias may not serve the purpose of determining the trustworthiness of results, as effect measures are often not the primary goal of methodological studies. Determining risk of bias in methodological studies is likely a practice borrowed from systematic review methodology, but whose intrinsic value is not obvious in methodological studies. When it is part of the research question, investigators often focus on one aspect of risk of bias. For example, Speich investigated how blinding was reported in surgical trials [ 50 ], and Abraha et al., investigated the application of intention-to-treat analyses in systematic reviews and trials [ 51 ].
Q: What variables are relevant to methodological studies?
A: There is empirical evidence that certain variables may inform the findings in a methodological study. We outline some of these and provide a brief overview below:
- Country: Countries and regions differ in their research cultures, and the resources available to conduct research. Therefore, it is reasonable to believe that there may be differences in methodological features across countries. Methodological studies have reported loco-regional differences in reporting quality [ 52 , 53 ]. This may also be related to challenges non-English speakers face in publishing papers in English.
- Authors’ expertise: The inclusion of authors with expertise in research methodology, biostatistics, and scientific writing is likely to influence the end-product. Oltean et al. found that among randomized trials in orthopaedic surgery, the use of analyses that accounted for clustering was more likely when specialists (e.g. statistician, epidemiologist or clinical trials methodologist) were included on the study team [ 54 ]. Fleming et al. found that including methodologists in the review team was associated with appropriate use of reporting guidelines [ 55 ].
- Source of funding and conflicts of interest: Some studies have found that funded studies report better [ 56 , 57 ], while others do not [ 53 , 58 ]. The presence of funding would indicate the availability of resources deployed to ensure optimal design, conduct, analysis and reporting. However, the source of funding may introduce conflicts of interest and warrant assessment. For example, Kaiser et al. investigated the effect of industry funding on obesity or nutrition randomized trials and found that reporting quality was similar [ 59 ]. Thomas et al. looked at reporting quality of long-term weight loss trials and found that industry funded studies were better [ 60 ]. Kan et al. examined the association between industry funding and “positive trials” (trials reporting a significant intervention effect) and found that industry funding was highly predictive of a positive trial [ 61 ]. This finding is similar to that of a recent Cochrane Methodology Review by Hansen et al. [ 62 ]
- Journal characteristics: Certain journals’ characteristics may influence the study design, analysis or reporting. Characteristics such as journal endorsement of guidelines [ 63 , 64 ], and Journal Impact Factor (JIF) have been shown to be associated with reporting [ 63 , 65 – 67 ].
- Study size (sample size/number of sites): Some studies have shown that reporting is better in larger studies [ 53 , 56 , 58 ].
- Year of publication: It is reasonable to assume that design, conduct, analysis and reporting of research will change over time. Many studies have demonstrated improvements in reporting over time or after the publication of reporting guidelines [ 68 , 69 ].
- Type of intervention: In a methodological study of reporting quality of weight loss intervention studies, Thabane et al. found that trials of pharmacologic interventions were reported better than trials of non-pharmacologic interventions [ 70 ].
- Interactions between variables: Complex interactions between the previously listed variables are possible. High income countries with more resources may be more likely to conduct larger studies and incorporate a variety of experts. Authors in certain countries may prefer certain journals, and journal endorsement of guidelines and editorial policies may change over time.
Q: Should I focus only on high impact journals?
A: Investigators may choose to investigate only high impact journals because they are more likely to influence practice and policy, or because they assume that methodological standards would be higher. However, the JIF may severely limit the scope of articles included and may skew the sample towards articles with positive findings. The generalizability and applicability of findings from a handful of journals must be examined carefully, especially since the JIF varies over time. Even among journals that are all “high impact”, variations exist in methodological standards.
Q: Can I conduct a methodological study of qualitative research?
A: Yes. Even though a lot of methodological research has been conducted in the quantitative research field, methodological studies of qualitative studies are feasible. Certain databases that catalogue qualitative research including the Cumulative Index to Nursing & Allied Health Literature (CINAHL) have defined subject headings that are specific to methodological research (e.g. “research methodology”). Alternatively, one could also conduct a qualitative methodological review; that is, use qualitative approaches to synthesize methodological issues in qualitative studies.
Q: What reporting guidelines should I use for my methodological study?
A: There is no guideline that covers the entire scope of methodological studies. One adaptation of the PRISMA guidelines has been published, which works well for studies that aim to use the entire target population of research reports [ 71 ]. However, it is not widely used (40 citations in 2 years as of 09 December 2019), and methodological studies that are designed as cross-sectional or before-after studies require a more fit-for purpose guideline. A more encompassing reporting guideline for a broad range of methodological studies is currently under development [ 72 ]. However, in the absence of formal guidance, the requirements for scientific reporting should be respected, and authors of methodological studies should focus on transparency and reproducibility.
Q: What are the potential threats to validity and how can I avoid them?
A: Methodological studies may be compromised by a lack of internal or external validity. The main threats to internal validity in methodological studies are selection and confounding bias. Investigators must ensure that the methods used to select articles does not make them differ systematically from the set of articles to which they would like to make inferences. For example, attempting to make extrapolations to all journals after analyzing high-impact journals would be misleading.
Many factors (confounders) may distort the association between the exposure and outcome if the included research reports differ with respect to these factors [ 73 ]. For example, when examining the association between source of funding and completeness of reporting, it may be necessary to account for journals that endorse the guidelines. Confounding bias can be addressed by restriction, matching and statistical adjustment [ 73 ]. Restriction appears to be the method of choice for many investigators who choose to include only high impact journals or articles in a specific field. For example, Knol et al. examined the reporting of p -values in baseline tables of high impact journals [ 26 ]. Matching is also sometimes used. In the methodological study of non-randomized interventional studies of elective ventral hernia repair, Parker et al. matched prospective studies with retrospective studies and compared reporting standards [ 74 ]. Some other methodological studies use statistical adjustments. For example, Zhang et al. used regression techniques to determine the factors associated with missing participant data in trials [ 16 ].
With regard to external validity, researchers interested in conducting methodological studies must consider how generalizable or applicable their findings are. This should tie in closely with the research question and should be explicit. For example. Findings from methodological studies on trials published in high impact cardiology journals cannot be assumed to be applicable to trials in other fields. However, investigators must ensure that their sample truly represents the target sample either by a) conducting a comprehensive and exhaustive search, or b) using an appropriate and justified, randomly selected sample of research reports.
Even applicability to high impact journals may vary based on the investigators’ definition, and over time. For example, for high impact journals in the field of general medicine, Bouwmeester et al. included the Annals of Internal Medicine (AIM), BMJ, the Journal of the American Medical Association (JAMA), Lancet, the New England Journal of Medicine (NEJM), and PLoS Medicine ( n = 6) [ 75 ]. In contrast, the high impact journals selected in the methodological study by Schiller et al. were BMJ, JAMA, Lancet, and NEJM ( n = 4) [ 76 ]. Another methodological study by Kosa et al. included AIM, BMJ, JAMA, Lancet and NEJM ( n = 5). In the methodological study by Thabut et al., journals with a JIF greater than 5 were considered to be high impact. Riado Minguez et al. used first quartile journals in the Journal Citation Reports (JCR) for a specific year to determine “high impact” [ 77 ]. Ultimately, the definition of high impact will be based on the number of journals the investigators are willing to include, the year of impact and the JIF cut-off [ 78 ]. We acknowledge that the term “generalizability” may apply differently for methodological studies, especially when in many instances it is possible to include the entire target population in the sample studied.
Finally, methodological studies are not exempt from information bias which may stem from discrepancies in the included research reports [ 79 ], errors in data extraction, or inappropriate interpretation of the information extracted. Likewise, publication bias may also be a concern in methodological studies, but such concepts have not yet been explored.
A proposed framework
In order to inform discussions about methodological studies, the development of guidance for what should be reported, we have outlined some key features of methodological studies that can be used to classify them. For each of the categories outlined below, we provide an example. In our experience, the choice of approach to completing a methodological study can be informed by asking the following four questions:
- What is the aim?
A methodological study may be focused on exploring sources of bias in primary or secondary studies (meta-bias), or how bias is analyzed. We have taken care to distinguish bias (i.e. systematic deviations from the truth irrespective of the source) from reporting quality or completeness (i.e. not adhering to a specific reporting guideline or norm). An example of where this distinction would be important is in the case of a randomized trial with no blinding. This study (depending on the nature of the intervention) would be at risk of performance bias. However, if the authors report that their study was not blinded, they would have reported adequately. In fact, some methodological studies attempt to capture both “quality of conduct” and “quality of reporting”, such as Richie et al., who reported on the risk of bias in randomized trials of pharmacy practice interventions [ 80 ]. Babic et al. investigated how risk of bias was used to inform sensitivity analyses in Cochrane reviews [ 81 ]. Further, biases related to choice of outcomes can also be explored. For example, Tan et al investigated differences in treatment effect size based on the outcome reported [ 82 ].
Methodological studies may report quality of reporting against a reporting checklist (i.e. adherence to guidelines) or against expected norms. For example, Croituro et al. report on the quality of reporting in systematic reviews published in dermatology journals based on their adherence to the PRISMA statement [ 83 ], and Khan et al. described the quality of reporting of harms in randomized controlled trials published in high impact cardiovascular journals based on the CONSORT extension for harms [ 84 ]. Other methodological studies investigate reporting of certain features of interest that may not be part of formally published checklists or guidelines. For example, Mbuagbaw et al. described how often the implications for research are elaborated using the Evidence, Participants, Intervention, Comparison, Outcome, Timeframe (EPICOT) format [ 30 ].
Sometimes investigators may be interested in how consistent reports of the same research are, as it is expected that there should be consistency between: conference abstracts and published manuscripts; manuscript abstracts and manuscript main text; and trial registration and published manuscript. For example, Rosmarakis et al. investigated consistency between conference abstracts and full text manuscripts [ 85 ].
In addition to identifying issues with reporting in primary and secondary studies, authors of methodological studies may be interested in determining the factors that are associated with certain reporting practices. Many methodological studies incorporate this, albeit as a secondary outcome. For example, Farrokhyar et al. investigated the factors associated with reporting quality in randomized trials of coronary artery bypass grafting surgery [ 53 ].
Methodological studies may also be used to describe methods or compare methods, and the factors associated with methods. Muller et al. described the methods used for systematic reviews and meta-analyses of observational studies [ 86 ].
Some methodological studies synthesize results from other methodological studies. For example, Li et al. conducted a scoping review of methodological reviews that investigated consistency between full text and abstracts in primary biomedical research [ 87 ].
Some methodological studies may investigate the use of names and terms in health research. For example, Martinic et al. investigated the definitions of systematic reviews used in overviews of systematic reviews (OSRs), meta-epidemiological studies and epidemiology textbooks [ 88 ].
In addition to the previously mentioned experimental methodological studies, there may exist other types of methodological studies not captured here.
- 2. What is the design?
Most methodological studies are purely descriptive and report their findings as counts (percent) and means (standard deviation) or medians (interquartile range). For example, Mbuagbaw et al. described the reporting of research recommendations in Cochrane HIV systematic reviews [ 30 ]. Gohari et al. described the quality of reporting of randomized trials in diabetes in Iran [ 12 ].
Some methodological studies are analytical wherein “analytical studies identify and quantify associations, test hypotheses, identify causes and determine whether an association exists between variables, such as between an exposure and a disease.” [ 89 ] In the case of methodological studies all these investigations are possible. For example, Kosa et al. investigated the association between agreement in primary outcome from trial registry to published manuscript and study covariates. They found that larger and more recent studies were more likely to have agreement [ 15 ]. Tricco et al. compared the conclusion statements from Cochrane and non-Cochrane systematic reviews with a meta-analysis of the primary outcome and found that non-Cochrane reviews were more likely to report positive findings. These results are a test of the null hypothesis that the proportions of Cochrane and non-Cochrane reviews that report positive results are equal [ 90 ].
- 3. What is the sampling strategy?
Methodological reviews with narrow research questions may be able to include the entire target population. For example, in the methodological study of Cochrane HIV systematic reviews, Mbuagbaw et al. included all of the available studies ( n = 103) [ 30 ].
Many methodological studies use random samples of the target population [ 33 , 91 , 92 ]. Alternatively, purposeful sampling may be used, limiting the sample to a subset of research-related reports published within a certain time period, or in journals with a certain ranking or on a topic. Systematic sampling can also be used when random sampling may be challenging to implement.
- 4. What is the unit of analysis?
Many methodological studies use a research report (e.g. full manuscript of study, abstract portion of the study) as the unit of analysis, and inferences can be made at the study-level. However, both published and unpublished research-related reports can be studied. These may include articles, conference abstracts, registry entries etc.
Some methodological studies report on items which may occur more than once per article. For example, Paquette et al. report on subgroup analyses in Cochrane reviews of atrial fibrillation in which 17 systematic reviews planned 56 subgroup analyses [ 93 ].
This framework is outlined in Fig. 2 .

A proposed framework for methodological studies
Conclusions
Methodological studies have examined different aspects of reporting such as quality, completeness, consistency and adherence to reporting guidelines. As such, many of the methodological study examples cited in this tutorial are related to reporting. However, as an evolving field, the scope of research questions that can be addressed by methodological studies is expected to increase.
In this paper we have outlined the scope and purpose of methodological studies, along with examples of instances in which various approaches have been used. In the absence of formal guidance on the design, conduct, analysis and reporting of methodological studies, we have provided some advice to help make methodological studies consistent. This advice is grounded in good contemporary scientific practice. Generally, the research question should tie in with the sampling approach and planned analysis. We have also highlighted the variables that may inform findings from methodological studies. Lastly, we have provided suggestions for ways in which authors can categorize their methodological studies to inform their design and analysis.
Acknowledgements
Abbreviations.
CONSORT | Consolidated Standards of Reporting Trials |
EPICOT | Evidence, Participants, Intervention, Comparison, Outcome, Timeframe |
GRADE | Grading of Recommendations, Assessment, Development and Evaluations |
PICOT | Participants, Intervention, Comparison, Outcome, Timeframe |
PRISMA | Preferred Reporting Items of Systematic reviews and Meta-Analyses |
SWAR | Studies Within a Review |
SWAT | Studies Within a Trial |
Authors’ contributions
LM conceived the idea and drafted the outline and paper. DOL and LT commented on the idea and draft outline. LM, LP and DOL performed literature searches and data extraction. All authors (LM, DOL, LT, LP, DBA) reviewed several draft versions of the manuscript and approved the final manuscript.
This work did not receive any dedicated funding.
Availability of data and materials
Ethics approval and consent to participate.
Not applicable.
Consent for publication
Competing interests.
DOL, DBA, LM, LP and LT are involved in the development of a reporting guideline for methodological studies.
Publisher’s Note
Springer Nature remains neutral with regard to jurisdictional claims in published maps and institutional affiliations.
Have a language expert improve your writing
Run a free plagiarism check in 10 minutes, generate accurate citations for free.
- Knowledge Base
- Dissertation
- What Is a Research Methodology? | Steps & Tips
What Is a Research Methodology? | Steps & Tips
Published on August 25, 2022 by Shona McCombes and Tegan George. Revised on September 5, 2024.
Your research methodology discusses and explains the data collection and analysis methods you used in your research. A key part of your thesis, dissertation , or research paper , the methodology chapter explains what you did and how you did it, allowing readers to evaluate the reliability and validity of your research and your dissertation topic .
It should include:
- The type of research you conducted
- How you collected and analyzed your data
- Any tools or materials you used in the research
- How you mitigated or avoided research biases
- Why you chose these methods
- Your methodology section should generally be written in the past tense . Our grammar checker can help ensure consistency in your writing.
- Academic style guides in your field may provide detailed guidelines on what to include for different types of studies.
- Your citation style might provide guidelines for your methodology section (e.g., an APA Style methods section ).
Instantly correct all language mistakes in your text
Upload your document to correct all your mistakes in minutes
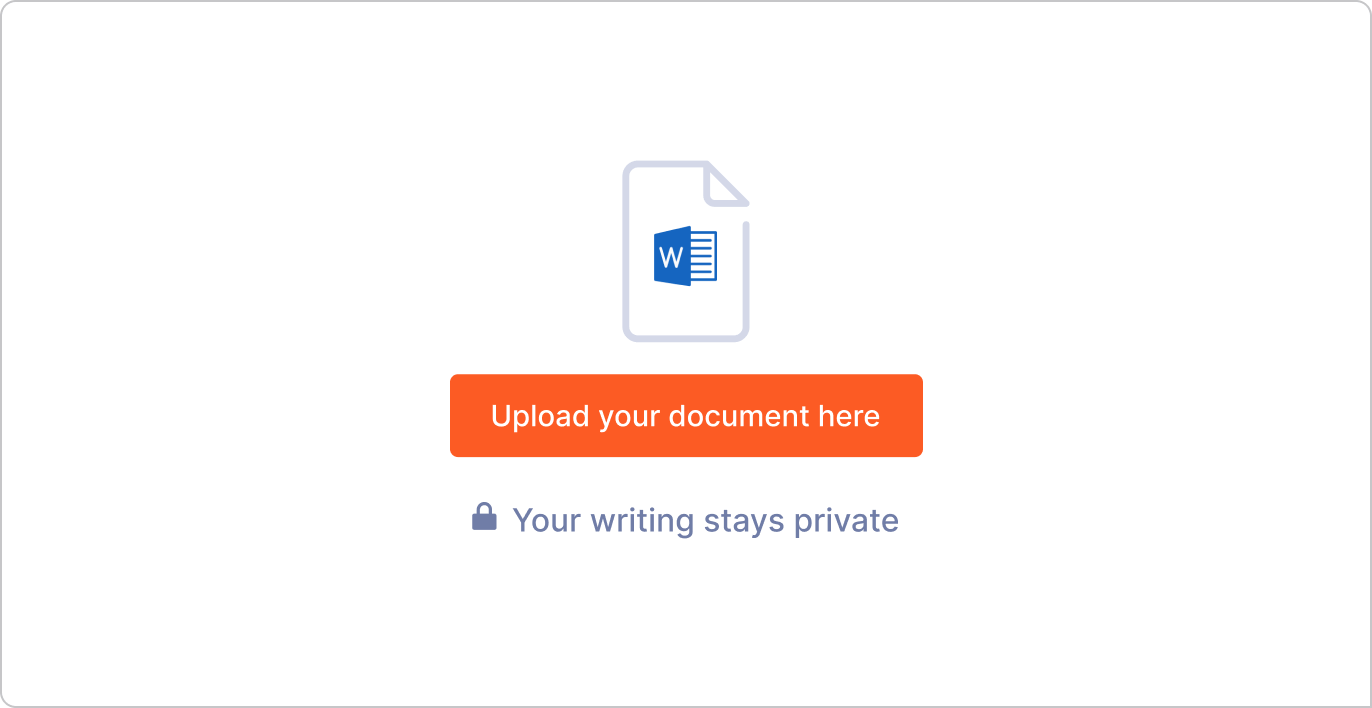
Table of contents
How to write a research methodology, why is a methods section important, step 1: explain your methodological approach, step 2: describe your data collection methods, step 3: describe your analysis method, step 4: evaluate and justify the methodological choices you made, tips for writing a strong methodology chapter, other interesting articles, frequently asked questions about methodology.
Here's why students love Scribbr's proofreading services
Discover proofreading & editing
Your methods section is your opportunity to share how you conducted your research and why you chose the methods you chose. It’s also the place to show that your research was rigorously conducted and can be replicated .
It gives your research legitimacy and situates it within your field, and also gives your readers a place to refer to if they have any questions or critiques in other sections.
You can start by introducing your overall approach to your research. You have two options here.
Option 1: Start with your “what”
What research problem or question did you investigate?
- Aim to describe the characteristics of something?
- Explore an under-researched topic?
- Establish a causal relationship?
And what type of data did you need to achieve this aim?
- Quantitative data , qualitative data , or a mix of both?
- Primary data collected yourself, or secondary data collected by someone else?
- Experimental data gathered by controlling and manipulating variables, or descriptive data gathered via observations?
Option 2: Start with your “why”
Depending on your discipline, you can also start with a discussion of the rationale and assumptions underpinning your methodology. In other words, why did you choose these methods for your study?
- Why is this the best way to answer your research question?
- Is this a standard methodology in your field, or does it require justification?
- Were there any ethical considerations involved in your choices?
- What are the criteria for validity and reliability in this type of research ? How did you prevent bias from affecting your data?
Once you have introduced your reader to your methodological approach, you should share full details about your data collection methods .
Quantitative methods
In order to be considered generalizable, you should describe quantitative research methods in enough detail for another researcher to replicate your study.
Here, explain how you operationalized your concepts and measured your variables. Discuss your sampling method or inclusion and exclusion criteria , as well as any tools, procedures, and materials you used to gather your data.
Surveys Describe where, when, and how the survey was conducted.
- How did you design the questionnaire?
- What form did your questions take (e.g., multiple choice, Likert scale )?
- Were your surveys conducted in-person or virtually?
- What sampling method did you use to select participants?
- What was your sample size and response rate?
Experiments Share full details of the tools, techniques, and procedures you used to conduct your experiment.
- How did you design the experiment ?
- How did you recruit participants?
- How did you manipulate and measure the variables ?
- What tools did you use?
Existing data Explain how you gathered and selected the material (such as datasets or archival data) that you used in your analysis.
- Where did you source the material?
- How was the data originally produced?
- What criteria did you use to select material (e.g., date range)?
The survey consisted of 5 multiple-choice questions and 10 questions measured on a 7-point Likert scale.
The goal was to collect survey responses from 350 customers visiting the fitness apparel company’s brick-and-mortar location in Boston on July 4–8, 2022, between 11:00 and 15:00.
Here, a customer was defined as a person who had purchased a product from the company on the day they took the survey. Participants were given 5 minutes to fill in the survey anonymously. In total, 408 customers responded, but not all surveys were fully completed. Due to this, 371 survey results were included in the analysis.
- Information bias
- Omitted variable bias
- Regression to the mean
- Survivorship bias
- Undercoverage bias
- Sampling bias
Qualitative methods
In qualitative research , methods are often more flexible and subjective. For this reason, it’s crucial to robustly explain the methodology choices you made.
Be sure to discuss the criteria you used to select your data, the context in which your research was conducted, and the role you played in collecting your data (e.g., were you an active participant, or a passive observer?)
Interviews or focus groups Describe where, when, and how the interviews were conducted.
- How did you find and select participants?
- How many participants took part?
- What form did the interviews take ( structured , semi-structured , or unstructured )?
- How long were the interviews?
- How were they recorded?
Participant observation Describe where, when, and how you conducted the observation or ethnography .
- What group or community did you observe? How long did you spend there?
- How did you gain access to this group? What role did you play in the community?
- How long did you spend conducting the research? Where was it located?
- How did you record your data (e.g., audiovisual recordings, note-taking)?
Existing data Explain how you selected case study materials for your analysis.
- What type of materials did you analyze?
- How did you select them?
In order to gain better insight into possibilities for future improvement of the fitness store’s product range, semi-structured interviews were conducted with 8 returning customers.
Here, a returning customer was defined as someone who usually bought products at least twice a week from the store.
Surveys were used to select participants. Interviews were conducted in a small office next to the cash register and lasted approximately 20 minutes each. Answers were recorded by note-taking, and seven interviews were also filmed with consent. One interviewee preferred not to be filmed.
- The Hawthorne effect
- Observer bias
- The placebo effect
- Response bias and Nonresponse bias
- The Pygmalion effect
- Recall bias
- Social desirability bias
- Self-selection bias
Mixed methods
Mixed methods research combines quantitative and qualitative approaches. If a standalone quantitative or qualitative study is insufficient to answer your research question, mixed methods may be a good fit for you.
Mixed methods are less common than standalone analyses, largely because they require a great deal of effort to pull off successfully. If you choose to pursue mixed methods, it’s especially important to robustly justify your methods.
Receive feedback on language, structure, and formatting
Professional editors proofread and edit your paper by focusing on:
- Academic style
- Vague sentences
- Style consistency
See an example
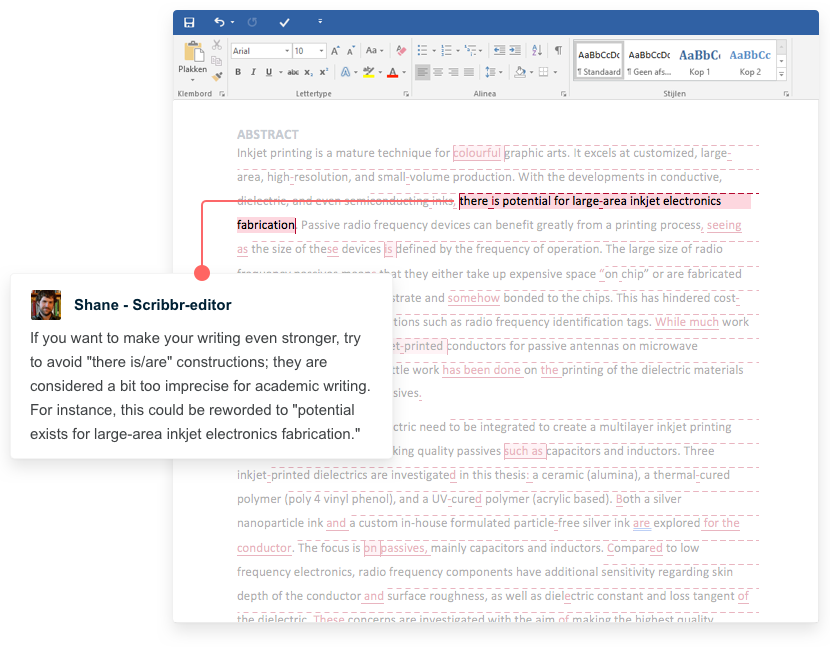
Next, you should indicate how you processed and analyzed your data. Avoid going into too much detail: you should not start introducing or discussing any of your results at this stage.
In quantitative research , your analysis will be based on numbers. In your methods section, you can include:
- How you prepared the data before analyzing it (e.g., checking for missing data , removing outliers , transforming variables)
- Which software you used (e.g., SPSS, Stata or R)
- Which statistical tests you used (e.g., two-tailed t test , simple linear regression )
In qualitative research, your analysis will be based on language, images, and observations (often involving some form of textual analysis ).
Specific methods might include:
- Content analysis : Categorizing and discussing the meaning of words, phrases and sentences
- Thematic analysis : Coding and closely examining the data to identify broad themes and patterns
- Discourse analysis : Studying communication and meaning in relation to their social context
Mixed methods combine the above two research methods, integrating both qualitative and quantitative approaches into one coherent analytical process.
Above all, your methodology section should clearly make the case for why you chose the methods you did. This is especially true if you did not take the most standard approach to your topic. In this case, discuss why other methods were not suitable for your objectives, and show how this approach contributes new knowledge or understanding.
In any case, it should be overwhelmingly clear to your reader that you set yourself up for success in terms of your methodology’s design. Show how your methods should lead to results that are valid and reliable, while leaving the analysis of the meaning, importance, and relevance of your results for your discussion section .
- Quantitative: Lab-based experiments cannot always accurately simulate real-life situations and behaviors, but they are effective for testing causal relationships between variables .
- Qualitative: Unstructured interviews usually produce results that cannot be generalized beyond the sample group , but they provide a more in-depth understanding of participants’ perceptions, motivations, and emotions.
- Mixed methods: Despite issues systematically comparing differing types of data, a solely quantitative study would not sufficiently incorporate the lived experience of each participant, while a solely qualitative study would be insufficiently generalizable.
Remember that your aim is not just to describe your methods, but to show how and why you applied them. Again, it’s critical to demonstrate that your research was rigorously conducted and can be replicated.
1. Focus on your objectives and research questions
The methodology section should clearly show why your methods suit your objectives and convince the reader that you chose the best possible approach to answering your problem statement and research questions .
2. Cite relevant sources
Your methodology can be strengthened by referencing existing research in your field. This can help you to:
- Show that you followed established practice for your type of research
- Discuss how you decided on your approach by evaluating existing research
- Present a novel methodological approach to address a gap in the literature
3. Write for your audience
Consider how much information you need to give, and avoid getting too lengthy. If you are using methods that are standard for your discipline, you probably don’t need to give a lot of background or justification.
Regardless, your methodology should be a clear, well-structured text that makes an argument for your approach, not just a list of technical details and procedures.
If you want to know more about statistics , methodology , or research bias , make sure to check out some of our other articles with explanations and examples.
- Normal distribution
- Measures of central tendency
- Chi square tests
- Confidence interval
- Quartiles & Quantiles
Methodology
- Cluster sampling
- Stratified sampling
- Thematic analysis
- Cohort study
- Peer review
- Ethnography
Research bias
- Implicit bias
- Cognitive bias
- Conformity bias
- Hawthorne effect
- Availability heuristic
- Attrition bias
Methodology refers to the overarching strategy and rationale of your research project . It involves studying the methods used in your field and the theories or principles behind them, in order to develop an approach that matches your objectives.
Methods are the specific tools and procedures you use to collect and analyze data (for example, experiments, surveys , and statistical tests ).
In shorter scientific papers, where the aim is to report the findings of a specific study, you might simply describe what you did in a methods section .
In a longer or more complex research project, such as a thesis or dissertation , you will probably include a methodology section , where you explain your approach to answering the research questions and cite relevant sources to support your choice of methods.
In a scientific paper, the methodology always comes after the introduction and before the results , discussion and conclusion . The same basic structure also applies to a thesis, dissertation , or research proposal .
Depending on the length and type of document, you might also include a literature review or theoretical framework before the methodology.
Quantitative research deals with numbers and statistics, while qualitative research deals with words and meanings.
Quantitative methods allow you to systematically measure variables and test hypotheses . Qualitative methods allow you to explore concepts and experiences in more detail.
Reliability and validity are both about how well a method measures something:
- Reliability refers to the consistency of a measure (whether the results can be reproduced under the same conditions).
- Validity refers to the accuracy of a measure (whether the results really do represent what they are supposed to measure).
If you are doing experimental research, you also have to consider the internal and external validity of your experiment.
A sample is a subset of individuals from a larger population . Sampling means selecting the group that you will actually collect data from in your research. For example, if you are researching the opinions of students in your university, you could survey a sample of 100 students.
In statistics, sampling allows you to test a hypothesis about the characteristics of a population.
Cite this Scribbr article
If you want to cite this source, you can copy and paste the citation or click the “Cite this Scribbr article” button to automatically add the citation to our free Citation Generator.
McCombes, S. & George, T. (2024, September 05). What Is a Research Methodology? | Steps & Tips. Scribbr. Retrieved September 9, 2024, from https://www.scribbr.com/dissertation/methodology/
Is this article helpful?
Shona McCombes
Other students also liked, what is a theoretical framework | guide to organizing, what is a research design | types, guide & examples, qualitative vs. quantitative research | differences, examples & methods, "i thought ai proofreading was useless but..".
I've been using Scribbr for years now and I know it's a service that won't disappoint. It does a good job spotting mistakes”
What are the limitations in research and how to write them?
Learn about the potential limitations in research and how to appropriately address them in order to deliver honest and ethical research.
It is fairly uncommon for researchers to stumble into the term research limitations when working on their research paper. Limitations in research can arise owing to constraints on design, methods, materials, and so on, and these aspects, unfortunately, may have an influence on your subject’s findings.
In this Mind The Graph’s article, we’ll discuss some recommendations for writing limitations in research , provide examples of various common types of limitations, and suggest how to properly present this information.
What are the limitations in research?
The limitations in research are the constraints in design, methods or even researchers’ limitations that affect and influence the interpretation of your research’s ultimate findings. These are limitations on the generalization and usability of findings that emerge from the design of the research and/or the method employed to ensure validity both internally and externally.
Researchers are usually cautious to acknowledge the limitations of their research in their publications for fear of undermining the research’s scientific validity. No research is faultless or covers every possible angle. As a result, addressing the constraints of your research exhibits honesty and integrity .
Why should include limitations of research in my paper?
Though limitations tackle potential flaws in research, commenting on them at the conclusion of your paper, by demonstrating that you are aware of these limitations and explaining how they impact the conclusions that may be taken from the research, improves your research by disclosing any issues before other researchers or reviewers do .
Additionally, emphasizing research constraints implies that you have thoroughly investigated the ramifications of research shortcomings and have a thorough understanding of your research problem.
Limits exist in any research; being honest about them and explaining them would impress researchers and reviewers more than disregarding them.
Remember that acknowledging a research’s shortcomings offers a chance to provide ideas for future research, but be careful to describe how your study may help to concentrate on these outstanding problems.
Possible limitations examples
Here are some limitations connected to methodology and the research procedure that you may need to explain and discuss in connection to your findings.
Methodological limitations
Sample size.
The number of units of analysis used in your study is determined by the sort of research issue being investigated. It is important to note that if your sample is too small, finding significant connections in the data will be challenging, as statistical tests typically require a larger sample size to ensure a fair representation and this can be limiting.
Lack of available or reliable data
A lack of data or trustworthy data will almost certainly necessitate limiting the scope of your research or the size of your sample, or it can be a substantial impediment to identifying a pattern and a relevant connection.
Lack of prior research on the subject
Citing previous research papers forms the basis of your literature review and aids in comprehending the research subject you are researching. Yet there may be little if any, past research on your issue.
The measure used to collect data
After finishing your analysis of the findings, you realize that the method you used to collect data limited your capacity to undertake a comprehensive evaluation of the findings. Recognize the flaw by mentioning that future researchers should change the specific approach for data collection.
Issues with research samples and selection
Sampling inaccuracies arise when a probability sampling method is employed to choose a sample, but that sample does not accurately represent the overall population or the relevant group. As a result, your study suffers from “sampling bias” or “selection bias.”
Limitations of the research
When your research requires polling certain persons or a specific group, you may have encountered the issue of limited access to these interviewees. Because of the limited access, you may need to reorganize or rearrange your research. In this scenario, explain why access is restricted and ensure that your findings are still trustworthy and valid despite the constraint.
Time constraints
Practical difficulties may limit the amount of time available to explore a research issue and monitor changes as they occur. If time restrictions have any detrimental influence on your research, recognize this impact by expressing the necessity for a future investigation.
Due to their cultural origins or opinions on observed events, researchers may carry biased opinions, which can influence the credibility of a research. Furthermore, researchers may exhibit biases toward data and conclusions that only support their hypotheses or arguments.
The structure of the limitations section
The limitations of your research are usually stated at the beginning of the discussion section of your paper so that the reader is aware of and comprehends the limitations prior to actually reading the rest of your findings, or they are stated at the end of the discussion section as an acknowledgment of the need for further research.
The ideal way is to divide your limitations section into three steps:
1. Identify the research constraints;
2. Describe in great detail how they affect your research;
3. Mention the opportunity for future investigations and give possibilities.
By following this method while addressing the constraints of your research, you will be able to effectively highlight your research’s shortcomings without jeopardizing the quality and integrity of your research.
Present your research or paper in an innovative way
If you want your readers to be engaged and participate in your research, try Mind The Graph tool to add visual assets to your content. Infographics may improve comprehension and are easy to read, just as the Mind The Graph tool is simple to use and offers a variety of templates from which you can select the one that best suits your information.
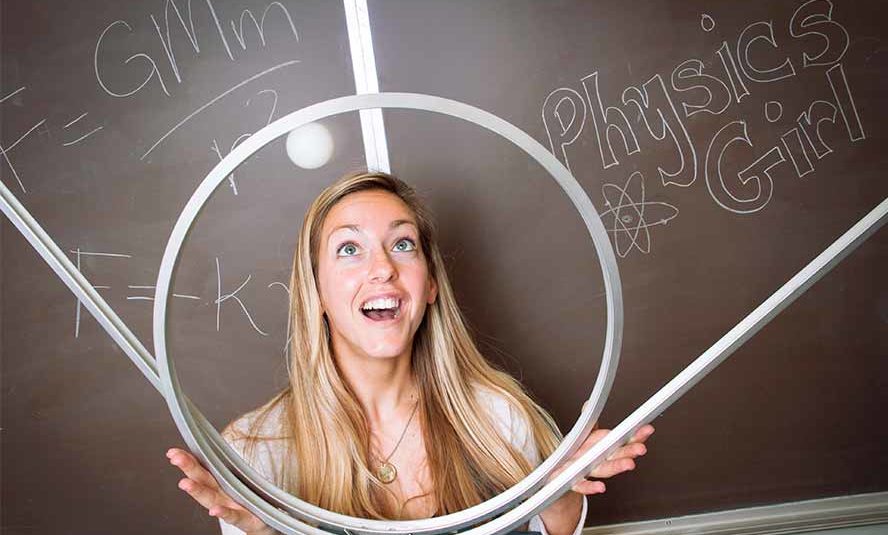
Subscribe to our newsletter
Exclusive high quality content about effective visual communication in science.
Sign Up for Free
Try the best infographic maker and promote your research with scientifically-accurate beautiful figures
no credit card required
About Jessica Abbadia
Jessica Abbadia is a lawyer that has been working in Digital Marketing since 2020, improving organic performance for apps and websites in various regions through ASO and SEO. Currently developing scientific and intellectual knowledge for the community's benefit. Jessica is an animal rights activist who enjoys reading and drinking strong coffee.
Content tags

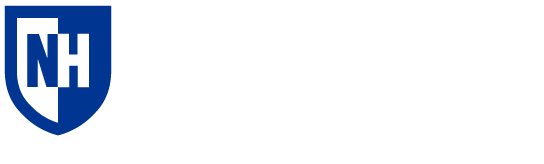
CPS Online Graduate Studies Research Paper (UNH Manchester Library): Limitations of the Study
- Overview of the Research Process for Capstone Projects
- Types of Research Design
- Selecting a Research Problem
- The Title of Your Research Paper
- Before You Begin Writing
- 7 Parts of the Research Paper
- Background Information
- Quanitative and Qualitative Methods
- Qualitative Methods
- Quanitative Methods
- Resources to Help You With the Literature Review
- Non-Textual Elements
Limitations of the Study
- Format of Capstone Research Projects at GSC
- Editing and Proofreading Your Paper
- Acknowledgements
- UNH Scholar's Repository
The limitations of the study are those characteristics of design or methodology that impacted or influenced the interpretation of the findings from your research. They are the constraints on generalizability, applications to practice, and/or utility of findings that are the result of the ways in which you initially chose to design the study and/or the method used to establish internal and external validity.
Price, James H. and Judy Murnan. “Research Limitations and the Necessity of Reporting Them.” American Journal of Health Education 35 (2004): 66-67.
Always acknowledge a study's limitations. It is far better that you identify and acknowledge your study’s limitations than to have them pointed out by your professor and be graded down because you appear to have ignored them.
Keep in mind that acknowledgement of a study's limitations is an opportunity to make suggestions for further research. If you do connect your study's limitations to suggestions for further research, be sure to explain the ways in which these unanswered questions may become more focused because of your study.
Acknowledgement of a study's limitations also provides you with an opportunity to demonstrate that you have thought critically about the research problem, understood the relevant literature published about it, and correctly assessed the methods chosen for studying the problem. A key objective of the research process is not only discovering new knowledge but to also confront assumptions and explore what we don't know.
Claiming limitations is a subjective process because you must evaluate the impact of those limitations . Don't just list key weaknesses and the magnitude of a study's limitations. To do so diminishes the validity of your research because it leaves the reader wondering whether, or in what ways, limitation(s) in your study may have impacted the results and conclusions. Limitations require a critical, overall appraisal and interpretation of their impact. You should answer the question: do these problems with errors, methods, validity, etc. eventually matter and, if so, to what extent?
Price, James H. and Judy Murnan. “Research Limitations and the Necessity of Reporting Them.” American Journal of Health Education 35 (2004): 66-67; Structure: How to Structure the Research Limitations Section of Your Dissertation . Dissertations and Theses: An Online Textbook. Laerd.com.
Descriptions of Possible Limitations
All studies have limitations . However, it is important that you restrict your discussion to limitations related to the research problem under investigation. For example, if a meta-analysis of existing literature is not a stated purpose of your research, it should not be discussed as a limitation. Do not apologize for not addressing issues that you did not promise to investigate in the introduction of your paper.
Here are examples of limitations related to methodology and the research process you may need to describe and to discuss how they possibly impacted your results. Descriptions of limitations should be stated in the past tense because they were discovered after you completed your research.
Possible Methodological Limitations
- Sample size -- the number of the units of analysis you use in your study is dictated by the type of research problem you are investigating. Note that, if your sample size is too small, it will be difficult to find significant relationships from the data, as statistical tests normally require a larger sample size to ensure a representative distribution of the population and to be considered representative of groups of people to whom results will be generalized or transferred. Note that sample size is less relevant in qualitative research.
- Lack of available and/or reliable data -- a lack of data or of reliable data will likely require you to limit the scope of your analysis, the size of your sample, or it can be a significant obstacle in finding a trend and a meaningful relationship. You need to not only describe these limitations but to offer reasons why you believe data is missing or is unreliable. However, don’t just throw up your hands in frustration; use this as an opportunity to describe the need for future research.
- Lack of prior research studies on the topic -- citing prior research studies forms the basis of your literature review and helps lay a foundation for understanding the research problem you are investigating. Depending on the currency or scope of your research topic, there may be little, if any, prior research on your topic. Before assuming this to be true, though, consult with a librarian. In cases when a librarian has confirmed that there is no prior research, you may be required to develop an entirely new research typology [for example, using an exploratory rather than an explanatory research design]. Note again that discovering a limitation can serve as an important opportunity to identify new gaps in the literature and to describe the need for further research.
- Measure used to collect the data -- sometimes it is the case that, after completing your interpretation of the findings, you discover that the way in which you gathered data inhibited your ability to conduct a thorough analysis of the results. For example, you regret not including a specific question in a survey that, in retrospect, could have helped address a particular issue that emerged later in the study. Acknowledge the deficiency by stating a need for future researchers to revise the specific method for gathering data.
- Self-reported data -- whether you are relying on pre-existing data or you are conducting a qualitative research study and gathering the data yourself, self-reported data is limited by the fact that it rarely can be independently verified. In other words, you have to take what people say, whether in interviews, focus groups, or on questionnaires, at face value. However, self-reported data can contain several potential sources of bias that you should be alert to and note as limitations. These biases become apparent if they are incongruent with data from other sources. These are: (1) selective memory [remembering or not remembering experiences or events that occurred at some point in the past]; (2) telescoping [recalling events that occurred at one time as if they occurred at another time]; (3) attribution [the act of attributing positive events and outcomes to one's own agency but attributing negative events and outcomes to external forces]; and, (4) exaggeration [the act of representing outcomes or embellishing events as more significant than is actually suggested from other data].
Possible Limitations of the Researcher
- Access -- if your study depends on having access to people, organizations, or documents and, for whatever reason, access is denied or limited in some way, the reasons for this need to be described.
- Longitudinal effects -- unlike your professor, who can literally devote years [even a lifetime] to studying a single topic, the time available to investigate a research problem and to measure change or stability over time is pretty much constrained by the due date of your assignment. Be sure to choose a research problem that does not require an excessive amount of time to complete the literature review, apply the methodology, and gather and interpret the results. If you're unsure whether you can complete your research within the confines of the assignment's due date, talk to your professor.
- Cultural and other type of bias -- we all have biases, whether we are conscience of them or not. Bias is when a person, place, or thing is viewed or shown in a consistently inaccurate way. Bias is usually negative, though one can have a positive bias as well, especially if that bias reflects your reliance on research that only support for your hypothesis. When proof-reading your paper, be especially critical in reviewing how you have stated a problem, selected the data to be studied, what may have been omitted, the manner in which you have ordered events, people, or places, how you have chosen to represent a person, place, or thing, to name a phenomenon, or to use possible words with a positive or negative connotation.
NOTE: If you detect bias in prior research, it must be acknowledged and you should explain what measures were taken to avoid perpetuating that bias.
- Fluency in a language -- if your research focuses on measuring the perceived value of after-school tutoring among Mexican-American ESL [English as a Second Language] students, for example, and you are not fluent in Spanish, you are limited in being able to read and interpret Spanish language research studies on the topic. This deficiency should be acknowledged.
Aguinis, Hermam and Jeffrey R. Edwards. “Methodological Wishes for the Next Decade and How to Make Wishes Come True.” Journal of Management Studies 51 (January 2014): 143-174; Brutus, Stéphane et al. "Self-Reported Limitations and Future Directions in Scholarly Reports: Analysis and Recommendations." Journal of Management 39 (January 2013): 48-75; Senunyeme, Emmanuel K. Business Research Methods . Powerpoint Presentation. Regent University of Science and Technology; ter Riet, Gerben et al. “All That Glitters Isn't Gold: A Survey on Acknowledgment of Limitations in Biomedical Studies.” PLOS One 8 (November 2013): 1-6.
Structure and Writing Style
Information about the limitations of your study are generally placed either at the beginning of the discussion section of your paper so the reader knows and understands the limitations before reading the rest of your analysis of the findings, or, the limitations are outlined at the conclusion of the discussion section as an acknowledgement of the need for further study. Statements about a study's limitations should not be buried in the body [middle] of the discussion section unless a limitation is specific to something covered in that part of the paper. If this is the case, though, the limitation should be reiterated at the conclusion of the section. If you determine that your study is seriously flawed due to important limitations, such as, an inability to acquire critical data, consider reframing it as an exploratory study intended to lay the groundwork for a more complete research study in the future. Be sure, though, to specifically explain the ways that these flaws can be successfully overcome in a new study. But, do not use this as an excuse for not developing a thorough research paper! Review the tab in this guide for developing a research topic. If serious limitations exist, it generally indicates a likelihood that your research problem is too narrowly defined or that the issue or event under study is too recent and, thus, very little research has been written about it. If serious limitations do emerge, consult with your professor about possible ways to overcome them or how to revise your study. When discussing the limitations of your research, be sure to: Describe each limitation in detailed but concise terms; Explain why each limitation exists; Provide the reasons why each limitation could not be overcome using the method(s) chosen to acquire or gather the data [cite to other studies that had similar problems when possible]; Assess the impact of each limitation in relation to the overall findings and conclusions of your study; and, If appropriate, describe how these limitations could point to the need for further research. Remember that the method you chose may be the source of a significant limitation that has emerged during your interpretation of the results [for example, you didn't interview a group of people that you later wish you had]. If this is the case, don't panic. Acknowledge it, and explain how applying a different or more robust methodology might address the research problem more effectively in a future study. A underlying goal of scholarly research is not only to show what works, but to demonstrate what doesn't work or what needs further clarification. Aguinis, Hermam and Jeffrey R. Edwards. “Methodological Wishes for the Next Decade and How to Make Wishes Come True.” Journal of Management Studies 51 (January 2014): 143-174; Brutus, Stéphane et al. "Self-Reported Limitations and Future Directions in Scholarly Reports: Analysis and Recommendations." Journal of Management 39 (January 2013): 48-75; Ioannidis, John P.A. "Limitations are not Properly Acknowledged in the Scientific Literature." Journal of Clinical Epidemiology 60 (2007): 324-329; Pasek, Josh. Writing the Empirical Social Science Research Paper: A Guide for the Perplexed. January 24, 2012. Academia.edu; Structure: How to Structure the Research Limitations Section of Your Dissertation. Dissertations and Theses: An Online Textbook. Laerd.com; What Is an Academic Paper? Institute for Writing Rhetoric. Dartmouth College; Writing the Experimental Report: Methods, Results, and Discussion. The Writing Lab and The OWL. Purdue University.
Information about the limitations of your study are generally placed either at the beginning of the discussion section of your paper so the reader knows and understands the limitations before reading the rest of your analysis of the findings, or, the limitations are outlined at the conclusion of the discussion section as an acknowledgement of the need for further study. Statements about a study's limitations should not be buried in the body [middle] of the discussion section unless a limitation is specific to something covered in that part of the paper. If this is the case, though, the limitation should be reiterated at the conclusion of the section.
If you determine that your study is seriously flawed due to important limitations , such as, an inability to acquire critical data, consider reframing it as an exploratory study intended to lay the groundwork for a more complete research study in the future. Be sure, though, to specifically explain the ways that these flaws can be successfully overcome in a new study.
But, do not use this as an excuse for not developing a thorough research paper! Review the tab in this guide for developing a research topic . If serious limitations exist, it generally indicates a likelihood that your research problem is too narrowly defined or that the issue or event under study is too recent and, thus, very little research has been written about it. If serious limitations do emerge, consult with your professor about possible ways to overcome them or how to revise your study.
When discussing the limitations of your research, be sure to:
- Describe each limitation in detailed but concise terms;
- Explain why each limitation exists;
- Provide the reasons why each limitation could not be overcome using the method(s) chosen to acquire or gather the data [cite to other studies that had similar problems when possible];
- Assess the impact of each limitation in relation to the overall findings and conclusions of your study; and,
- If appropriate, describe how these limitations could point to the need for further research.
Remember that the method you chose may be the source of a significant limitation that has emerged during your interpretation of the results [for example, you didn't interview a group of people that you later wish you had]. If this is the case, don't panic. Acknowledge it, and explain how applying a different or more robust methodology might address the research problem more effectively in a future study. A underlying goal of scholarly research is not only to show what works, but to demonstrate what doesn't work or what needs further clarification.
Aguinis, Hermam and Jeffrey R. Edwards. “Methodological Wishes for the Next Decade and How to Make Wishes Come True.” Journal of Management Studies 51 (January 2014): 143-174; Brutus, Stéphane et al. "Self-Reported Limitations and Future Directions in Scholarly Reports: Analysis and Recommendations." Journal of Management 39 (January 2013): 48-75; Ioannidis, John P.A. "Limitations are not Properly Acknowledged in the Scientific Literature." Journal of Clinical Epidemiology 60 (2007): 324-329; Pasek, Josh. Writing the Empirical Social Science Research Paper: A Guide for the Perplexed . January 24, 2012. Academia.edu; Structure: How to Structure the Research Limitations Section of Your Dissertation . Dissertations and Theses: An Online Textbook. Laerd.com; What Is an Academic Paper? Institute for Writing Rhetoric. Dartmouth College; Writing the Experimental Report: Methods, Results, and Discussion . The Writing Lab and The OWL. Purdue University.
- << Previous: The Discussion
- Next: Conclusion >>
- Last Updated: Nov 6, 2023 1:43 PM
- URL: https://libraryguides.unh.edu/cpsonlinegradpaper
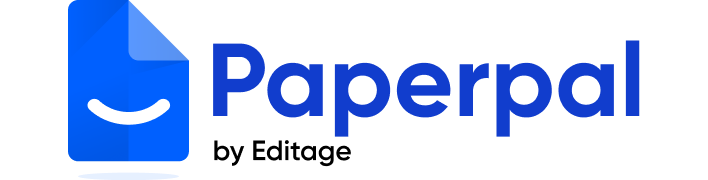
What is Research Methodology? Definition, Types, and Examples
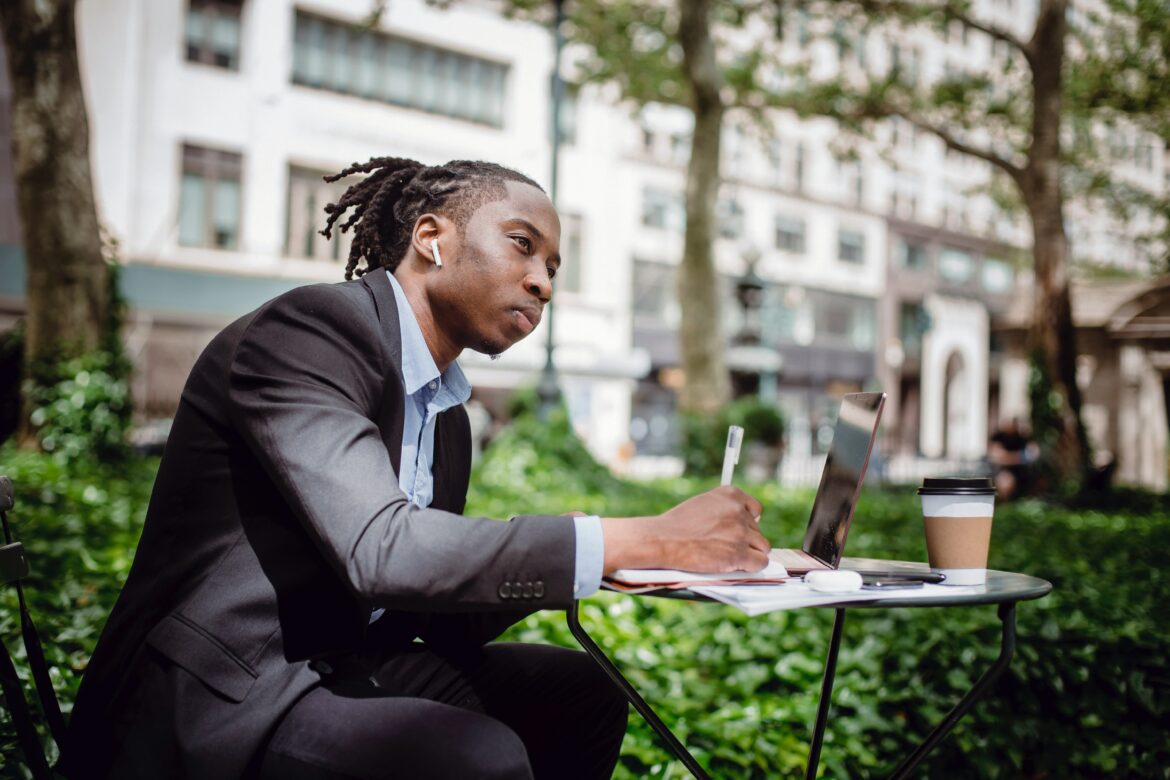
Research methodology 1,2 is a structured and scientific approach used to collect, analyze, and interpret quantitative or qualitative data to answer research questions or test hypotheses. A research methodology is like a plan for carrying out research and helps keep researchers on track by limiting the scope of the research. Several aspects must be considered before selecting an appropriate research methodology, such as research limitations and ethical concerns that may affect your research.
The research methodology section in a scientific paper describes the different methodological choices made, such as the data collection and analysis methods, and why these choices were selected. The reasons should explain why the methods chosen are the most appropriate to answer the research question. A good research methodology also helps ensure the reliability and validity of the research findings. There are three types of research methodology—quantitative, qualitative, and mixed-method, which can be chosen based on the research objectives.
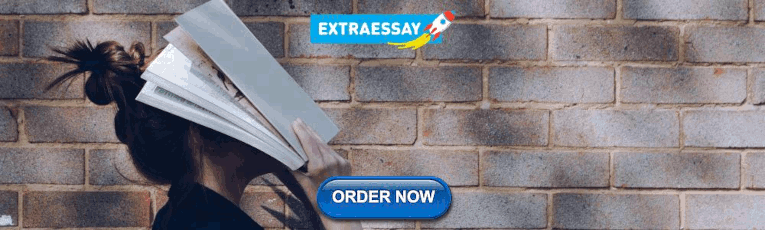
What is research methodology ?
A research methodology describes the techniques and procedures used to identify and analyze information regarding a specific research topic. It is a process by which researchers design their study so that they can achieve their objectives using the selected research instruments. It includes all the important aspects of research, including research design, data collection methods, data analysis methods, and the overall framework within which the research is conducted. While these points can help you understand what is research methodology, you also need to know why it is important to pick the right methodology.
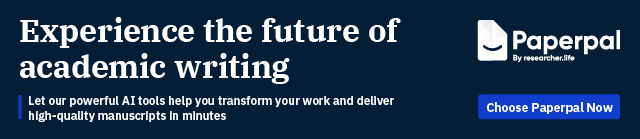
Having a good research methodology in place has the following advantages: 3
- Helps other researchers who may want to replicate your research; the explanations will be of benefit to them.
- You can easily answer any questions about your research if they arise at a later stage.
- A research methodology provides a framework and guidelines for researchers to clearly define research questions, hypotheses, and objectives.
- It helps researchers identify the most appropriate research design, sampling technique, and data collection and analysis methods.
- A sound research methodology helps researchers ensure that their findings are valid and reliable and free from biases and errors.
- It also helps ensure that ethical guidelines are followed while conducting research.
- A good research methodology helps researchers in planning their research efficiently, by ensuring optimum usage of their time and resources.
Writing the methods section of a research paper? Let Paperpal help you achieve perfection
Types of research methodology.
There are three types of research methodology based on the type of research and the data required. 1
- Quantitative research methodology focuses on measuring and testing numerical data. This approach is good for reaching a large number of people in a short amount of time. This type of research helps in testing the causal relationships between variables, making predictions, and generalizing results to wider populations.
- Qualitative research methodology examines the opinions, behaviors, and experiences of people. It collects and analyzes words and textual data. This research methodology requires fewer participants but is still more time consuming because the time spent per participant is quite large. This method is used in exploratory research where the research problem being investigated is not clearly defined.
- Mixed-method research methodology uses the characteristics of both quantitative and qualitative research methodologies in the same study. This method allows researchers to validate their findings, verify if the results observed using both methods are complementary, and explain any unexpected results obtained from one method by using the other method.
What are the types of sampling designs in research methodology?
Sampling 4 is an important part of a research methodology and involves selecting a representative sample of the population to conduct the study, making statistical inferences about them, and estimating the characteristics of the whole population based on these inferences. There are two types of sampling designs in research methodology—probability and nonprobability.
- Probability sampling
In this type of sampling design, a sample is chosen from a larger population using some form of random selection, that is, every member of the population has an equal chance of being selected. The different types of probability sampling are:
- Systematic —sample members are chosen at regular intervals. It requires selecting a starting point for the sample and sample size determination that can be repeated at regular intervals. This type of sampling method has a predefined range; hence, it is the least time consuming.
- Stratified —researchers divide the population into smaller groups that don’t overlap but represent the entire population. While sampling, these groups can be organized, and then a sample can be drawn from each group separately.
- Cluster —the population is divided into clusters based on demographic parameters like age, sex, location, etc.
- Convenience —selects participants who are most easily accessible to researchers due to geographical proximity, availability at a particular time, etc.
- Purposive —participants are selected at the researcher’s discretion. Researchers consider the purpose of the study and the understanding of the target audience.
- Snowball —already selected participants use their social networks to refer the researcher to other potential participants.
- Quota —while designing the study, the researchers decide how many people with which characteristics to include as participants. The characteristics help in choosing people most likely to provide insights into the subject.
What are data collection methods?
During research, data are collected using various methods depending on the research methodology being followed and the research methods being undertaken. Both qualitative and quantitative research have different data collection methods, as listed below.
Qualitative research 5
- One-on-one interviews: Helps the interviewers understand a respondent’s subjective opinion and experience pertaining to a specific topic or event
- Document study/literature review/record keeping: Researchers’ review of already existing written materials such as archives, annual reports, research articles, guidelines, policy documents, etc.
- Focus groups: Constructive discussions that usually include a small sample of about 6-10 people and a moderator, to understand the participants’ opinion on a given topic.
- Qualitative observation : Researchers collect data using their five senses (sight, smell, touch, taste, and hearing).
Quantitative research 6
- Sampling: The most common type is probability sampling.
- Interviews: Commonly telephonic or done in-person.
- Observations: Structured observations are most commonly used in quantitative research. In this method, researchers make observations about specific behaviors of individuals in a structured setting.
- Document review: Reviewing existing research or documents to collect evidence for supporting the research.
- Surveys and questionnaires. Surveys can be administered both online and offline depending on the requirement and sample size.
Let Paperpal help you write the perfect research methods section. Start now!
What are data analysis methods.
The data collected using the various methods for qualitative and quantitative research need to be analyzed to generate meaningful conclusions. These data analysis methods 7 also differ between quantitative and qualitative research.
Quantitative research involves a deductive method for data analysis where hypotheses are developed at the beginning of the research and precise measurement is required. The methods include statistical analysis applications to analyze numerical data and are grouped into two categories—descriptive and inferential.
Descriptive analysis is used to describe the basic features of different types of data to present it in a way that ensures the patterns become meaningful. The different types of descriptive analysis methods are:
- Measures of frequency (count, percent, frequency)
- Measures of central tendency (mean, median, mode)
- Measures of dispersion or variation (range, variance, standard deviation)
- Measure of position (percentile ranks, quartile ranks)
Inferential analysis is used to make predictions about a larger population based on the analysis of the data collected from a smaller population. This analysis is used to study the relationships between different variables. Some commonly used inferential data analysis methods are:
- Correlation: To understand the relationship between two or more variables.
- Cross-tabulation: Analyze the relationship between multiple variables.
- Regression analysis: Study the impact of independent variables on the dependent variable.
- Frequency tables: To understand the frequency of data.
- Analysis of variance: To test the degree to which two or more variables differ in an experiment.
Qualitative research involves an inductive method for data analysis where hypotheses are developed after data collection. The methods include:
- Content analysis: For analyzing documented information from text and images by determining the presence of certain words or concepts in texts.
- Narrative analysis: For analyzing content obtained from sources such as interviews, field observations, and surveys. The stories and opinions shared by people are used to answer research questions.
- Discourse analysis: For analyzing interactions with people considering the social context, that is, the lifestyle and environment, under which the interaction occurs.
- Grounded theory: Involves hypothesis creation by data collection and analysis to explain why a phenomenon occurred.
- Thematic analysis: To identify important themes or patterns in data and use these to address an issue.
How to choose a research methodology?
Here are some important factors to consider when choosing a research methodology: 8
- Research objectives, aims, and questions —these would help structure the research design.
- Review existing literature to identify any gaps in knowledge.
- Check the statistical requirements —if data-driven or statistical results are needed then quantitative research is the best. If the research questions can be answered based on people’s opinions and perceptions, then qualitative research is most suitable.
- Sample size —sample size can often determine the feasibility of a research methodology. For a large sample, less effort- and time-intensive methods are appropriate.
- Constraints —constraints of time, geography, and resources can help define the appropriate methodology.
Got writer’s block? Kickstart your research paper writing with Paperpal now!
How to write a research methodology .
A research methodology should include the following components: 3,9
- Research design —should be selected based on the research question and the data required. Common research designs include experimental, quasi-experimental, correlational, descriptive, and exploratory.
- Research method —this can be quantitative, qualitative, or mixed-method.
- Reason for selecting a specific methodology —explain why this methodology is the most suitable to answer your research problem.
- Research instruments —explain the research instruments you plan to use, mainly referring to the data collection methods such as interviews, surveys, etc. Here as well, a reason should be mentioned for selecting the particular instrument.
- Sampling —this involves selecting a representative subset of the population being studied.
- Data collection —involves gathering data using several data collection methods, such as surveys, interviews, etc.
- Data analysis —describe the data analysis methods you will use once you’ve collected the data.
- Research limitations —mention any limitations you foresee while conducting your research.
- Validity and reliability —validity helps identify the accuracy and truthfulness of the findings; reliability refers to the consistency and stability of the results over time and across different conditions.
- Ethical considerations —research should be conducted ethically. The considerations include obtaining consent from participants, maintaining confidentiality, and addressing conflicts of interest.
Streamline Your Research Paper Writing Process with Paperpal
The methods section is a critical part of the research papers, allowing researchers to use this to understand your findings and replicate your work when pursuing their own research. However, it is usually also the most difficult section to write. This is where Paperpal can help you overcome the writer’s block and create the first draft in minutes with Paperpal Copilot, its secure generative AI feature suite.
With Paperpal you can get research advice, write and refine your work, rephrase and verify the writing, and ensure submission readiness, all in one place. Here’s how you can use Paperpal to develop the first draft of your methods section.
- Generate an outline: Input some details about your research to instantly generate an outline for your methods section
- Develop the section: Use the outline and suggested sentence templates to expand your ideas and develop the first draft.
- P araph ras e and trim : Get clear, concise academic text with paraphrasing that conveys your work effectively and word reduction to fix redundancies.
- Choose the right words: Enhance text by choosing contextual synonyms based on how the words have been used in previously published work.
- Check and verify text : Make sure the generated text showcases your methods correctly, has all the right citations, and is original and authentic. .
You can repeat this process to develop each section of your research manuscript, including the title, abstract and keywords. Ready to write your research papers faster, better, and without the stress? Sign up for Paperpal and start writing today!
Frequently Asked Questions
Q1. What are the key components of research methodology?
A1. A good research methodology has the following key components:
- Research design
- Data collection procedures
- Data analysis methods
- Ethical considerations
Q2. Why is ethical consideration important in research methodology?
A2. Ethical consideration is important in research methodology to ensure the readers of the reliability and validity of the study. Researchers must clearly mention the ethical norms and standards followed during the conduct of the research and also mention if the research has been cleared by any institutional board. The following 10 points are the important principles related to ethical considerations: 10
- Participants should not be subjected to harm.
- Respect for the dignity of participants should be prioritized.
- Full consent should be obtained from participants before the study.
- Participants’ privacy should be ensured.
- Confidentiality of the research data should be ensured.
- Anonymity of individuals and organizations participating in the research should be maintained.
- The aims and objectives of the research should not be exaggerated.
- Affiliations, sources of funding, and any possible conflicts of interest should be declared.
- Communication in relation to the research should be honest and transparent.
- Misleading information and biased representation of primary data findings should be avoided.
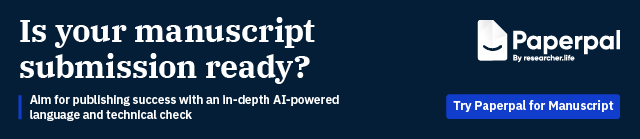
Q3. What is the difference between methodology and method?
A3. Research methodology is different from a research method, although both terms are often confused. Research methods are the tools used to gather data, while the research methodology provides a framework for how research is planned, conducted, and analyzed. The latter guides researchers in making decisions about the most appropriate methods for their research. Research methods refer to the specific techniques, procedures, and tools used by researchers to collect, analyze, and interpret data, for instance surveys, questionnaires, interviews, etc.
Research methodology is, thus, an integral part of a research study. It helps ensure that you stay on track to meet your research objectives and answer your research questions using the most appropriate data collection and analysis tools based on your research design.
Accelerate your research paper writing with Paperpal. Try for free now!
- Research methodologies. Pfeiffer Library website. Accessed August 15, 2023. https://library.tiffin.edu/researchmethodologies/whatareresearchmethodologies
- Types of research methodology. Eduvoice website. Accessed August 16, 2023. https://eduvoice.in/types-research-methodology/
- The basics of research methodology: A key to quality research. Voxco. Accessed August 16, 2023. https://www.voxco.com/blog/what-is-research-methodology/
- Sampling methods: Types with examples. QuestionPro website. Accessed August 16, 2023. https://www.questionpro.com/blog/types-of-sampling-for-social-research/
- What is qualitative research? Methods, types, approaches, examples. Researcher.Life blog. Accessed August 15, 2023. https://researcher.life/blog/article/what-is-qualitative-research-methods-types-examples/
- What is quantitative research? Definition, methods, types, and examples. Researcher.Life blog. Accessed August 15, 2023. https://researcher.life/blog/article/what-is-quantitative-research-types-and-examples/
- Data analysis in research: Types & methods. QuestionPro website. Accessed August 16, 2023. https://www.questionpro.com/blog/data-analysis-in-research/#Data_analysis_in_qualitative_research
- Factors to consider while choosing the right research methodology. PhD Monster website. Accessed August 17, 2023. https://www.phdmonster.com/factors-to-consider-while-choosing-the-right-research-methodology/
- What is research methodology? Research and writing guides. Accessed August 14, 2023. https://paperpile.com/g/what-is-research-methodology/
- Ethical considerations. Business research methodology website. Accessed August 17, 2023. https://research-methodology.net/research-methodology/ethical-considerations/
Paperpal is a comprehensive AI writing toolkit that helps students and researchers achieve 2x the writing in half the time. It leverages 21+ years of STM experience and insights from millions of research articles to provide in-depth academic writing, language editing, and submission readiness support to help you write better, faster.
Get accurate academic translations, rewriting support, grammar checks, vocabulary suggestions, and generative AI assistance that delivers human precision at machine speed. Try for free or upgrade to Paperpal Prime starting at US$19 a month to access premium features, including consistency, plagiarism, and 30+ submission readiness checks to help you succeed.
Experience the future of academic writing – Sign up to Paperpal and start writing for free!
Related Reads:
- Dangling Modifiers and How to Avoid Them in Your Writing
- Research Outlines: How to Write An Introduction Section in Minutes with Paperpal Copilot
- How to Paraphrase Research Papers Effectively
- What is a Literature Review? How to Write It (with Examples)
Language and Grammar Rules for Academic Writing
Climatic vs. climactic: difference and examples, you may also like, dissertation printing and binding | types & comparison , what is a dissertation preface definition and examples , how to write a research proposal: (with examples..., how to write your research paper in apa..., how to choose a dissertation topic, how to write a phd research proposal, how to write an academic paragraph (step-by-step guide), maintaining academic integrity with paperpal’s generative ai writing..., research funding basics: what should a grant proposal..., how to write an abstract in research papers....

21 Research Limitations Examples
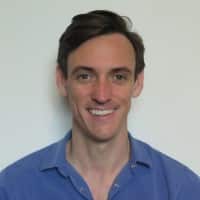
Chris Drew (PhD)
Dr. Chris Drew is the founder of the Helpful Professor. He holds a PhD in education and has published over 20 articles in scholarly journals. He is the former editor of the Journal of Learning Development in Higher Education. [Image Descriptor: Photo of Chris]
Learn about our Editorial Process
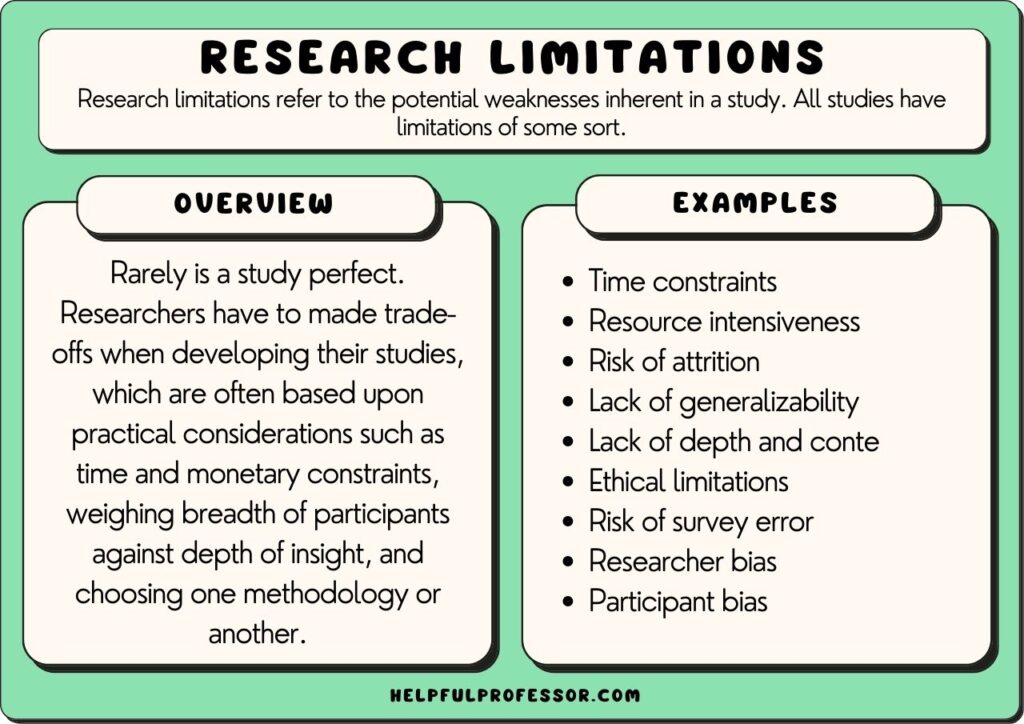
Research limitations refer to the potential weaknesses inherent in a study. All studies have limitations of some sort, meaning declaring limitations doesn’t necessarily need to be a bad thing, so long as your declaration of limitations is well thought-out and explained.
Rarely is a study perfect. Researchers have to make trade-offs when developing their studies, which are often based upon practical considerations such as time and monetary constraints, weighing the breadth of participants against the depth of insight, and choosing one methodology or another.
In research, studies can have limitations such as limited scope, researcher subjectivity, and lack of available research tools.
Acknowledging the limitations of your study should be seen as a strength. It demonstrates your willingness for transparency, humility, and submission to the scientific method and can bolster the integrity of the study. It can also inform future research direction.
Typically, scholars will explore the limitations of their study in either their methodology section, their conclusion section, or both.
Research Limitations Examples
Qualitative and quantitative research offer different perspectives and methods in exploring phenomena, each with its own strengths and limitations. So, I’ve split the limitations examples sections into qualitative and quantitative below.
Qualitative Research Limitations
Qualitative research seeks to understand phenomena in-depth and in context. It focuses on the ‘why’ and ‘how’ questions.
It’s often used to explore new or complex issues, and it provides rich, detailed insights into participants’ experiences, behaviors, and attitudes. However, these strengths also create certain limitations, as explained below.
1. Subjectivity
Qualitative research often requires the researcher to interpret subjective data. One researcher may examine a text and identify different themes or concepts as more dominant than others.
Close qualitative readings of texts are necessarily subjective – and while this may be a limitation, qualitative researchers argue this is the best way to deeply understand everything in context.
Suggested Solution and Response: To minimize subjectivity bias, you could consider cross-checking your own readings of themes and data against other scholars’ readings and interpretations. This may involve giving the raw data to a supervisor or colleague and asking them to code the data separately, then coming together to compare and contrast results.
2. Researcher Bias
The concept of researcher bias is related to, but slightly different from, subjectivity.
Researcher bias refers to the perspectives and opinions you bring with you when doing your research.
For example, a researcher who is explicitly of a certain philosophical or political persuasion may bring that persuasion to bear when interpreting data.
In many scholarly traditions, we will attempt to minimize researcher bias through the utilization of clear procedures that are set out in advance or through the use of statistical analysis tools.
However, in other traditions, such as in postmodern feminist research , declaration of bias is expected, and acknowledgment of bias is seen as a positive because, in those traditions, it is believed that bias cannot be eliminated from research, so instead, it is a matter of integrity to present it upfront.
Suggested Solution and Response: Acknowledge the potential for researcher bias and, depending on your theoretical framework , accept this, or identify procedures you have taken to seek a closer approximation to objectivity in your coding and analysis.
3. Generalizability
If you’re struggling to find a limitation to discuss in your own qualitative research study, then this one is for you: all qualitative research, of all persuasions and perspectives, cannot be generalized.
This is a core feature that sets qualitative data and quantitative data apart.
The point of qualitative data is to select case studies and similarly small corpora and dig deep through in-depth analysis and thick description of data.
Often, this will also mean that you have a non-randomized sample size.
While this is a positive – you’re going to get some really deep, contextualized, interesting insights – it also means that the findings may not be generalizable to a larger population that may not be representative of the small group of people in your study.
Suggested Solution and Response: Suggest future studies that take a quantitative approach to the question.
4. The Hawthorne Effect
The Hawthorne effect refers to the phenomenon where research participants change their ‘observed behavior’ when they’re aware that they are being observed.
This effect was first identified by Elton Mayo who conducted studies of the effects of various factors ton workers’ productivity. He noticed that no matter what he did – turning up the lights, turning down the lights, etc. – there was an increase in worker outputs compared to prior to the study taking place.
Mayo realized that the mere act of observing the workers made them work harder – his observation was what was changing behavior.
So, if you’re looking for a potential limitation to name for your observational research study , highlight the possible impact of the Hawthorne effect (and how you could reduce your footprint or visibility in order to decrease its likelihood).
Suggested Solution and Response: Highlight ways you have attempted to reduce your footprint while in the field, and guarantee anonymity to your research participants.
5. Replicability
Quantitative research has a great benefit in that the studies are replicable – a researcher can get a similar sample size, duplicate the variables, and re-test a study. But you can’t do that in qualitative research.
Qualitative research relies heavily on context – a specific case study or specific variables that make a certain instance worthy of analysis. As a result, it’s often difficult to re-enter the same setting with the same variables and repeat the study.
Furthermore, the individual researcher’s interpretation is more influential in qualitative research, meaning even if a new researcher enters an environment and makes observations, their observations may be different because subjectivity comes into play much more. This doesn’t make the research bad necessarily (great insights can be made in qualitative research), but it certainly does demonstrate a weakness of qualitative research.
6. Limited Scope
“Limited scope” is perhaps one of the most common limitations listed by researchers – and while this is often a catch-all way of saying, “well, I’m not studying that in this study”, it’s also a valid point.
No study can explore everything related to a topic. At some point, we have to make decisions about what’s included in the study and what is excluded from the study.
So, you could say that a limitation of your study is that it doesn’t look at an extra variable or concept that’s certainly worthy of study but will have to be explored in your next project because this project has a clearly and narrowly defined goal.
Suggested Solution and Response: Be clear about what’s in and out of the study when writing your research question.
7. Time Constraints
This is also a catch-all claim you can make about your research project: that you would have included more people in the study, looked at more variables, and so on. But you’ve got to submit this thing by the end of next semester! You’ve got time constraints.
And time constraints are a recognized reality in all research.
But this means you’ll need to explain how time has limited your decisions. As with “limited scope”, this may mean that you had to study a smaller group of subjects, limit the amount of time you spent in the field, and so forth.
Suggested Solution and Response: Suggest future studies that will build on your current work, possibly as a PhD project.
8. Resource Intensiveness
Qualitative research can be expensive due to the cost of transcription, the involvement of trained researchers, and potential travel for interviews or observations.
So, resource intensiveness is similar to the time constraints concept. If you don’t have the funds, you have to make decisions about which tools to use, which statistical software to employ, and how many research assistants you can dedicate to the study.
Suggested Solution and Response: Suggest future studies that will gain more funding on the back of this ‘ exploratory study ‘.
9. Coding Difficulties
Data analysis in qualitative research often involves coding, which can be subjective and complex, especially when dealing with ambiguous or contradicting data.
After naming this as a limitation in your research, it’s important to explain how you’ve attempted to address this. Some ways to ‘limit the limitation’ include:
- Triangulation: Have 2 other researchers code the data as well and cross-check your results with theirs to identify outliers that may need to be re-examined, debated with the other researchers, or removed altogether.
- Procedure: Use a clear coding procedure to demonstrate reliability in your coding process. I personally use the thematic network analysis method outlined in this academic article by Attride-Stirling (2001).
Suggested Solution and Response: Triangulate your coding findings with colleagues, and follow a thematic network analysis procedure.
10. Risk of Non-Responsiveness
There is always a risk in research that research participants will be unwilling or uncomfortable sharing their genuine thoughts and feelings in the study.
This is particularly true when you’re conducting research on sensitive topics, politicized topics, or topics where the participant is expressing vulnerability .
This is similar to the Hawthorne effect (aka participant bias), where participants change their behaviors in your presence; but it goes a step further, where participants actively hide their true thoughts and feelings from you.
Suggested Solution and Response: One way to manage this is to try to include a wider group of people with the expectation that there will be non-responsiveness from some participants.
11. Risk of Attrition
Attrition refers to the process of losing research participants throughout the study.
This occurs most commonly in longitudinal studies , where a researcher must return to conduct their analysis over spaced periods of time, often over a period of years.
Things happen to people over time – they move overseas, their life experiences change, they get sick, change their minds, and even die. The more time that passes, the greater the risk of attrition.
Suggested Solution and Response: One way to manage this is to try to include a wider group of people with the expectation that there will be attrition over time.
12. Difficulty in Maintaining Confidentiality and Anonymity
Given the detailed nature of qualitative data , ensuring participant anonymity can be challenging.
If you have a sensitive topic in a specific case study, even anonymizing research participants sometimes isn’t enough. People might be able to induce who you’re talking about.
Sometimes, this will mean you have to exclude some interesting data that you collected from your final report. Confidentiality and anonymity come before your findings in research ethics – and this is a necessary limiting factor.
Suggested Solution and Response: Highlight the efforts you have taken to anonymize data, and accept that confidentiality and accountability place extremely important constraints on academic research.
13. Difficulty in Finding Research Participants
A study that looks at a very specific phenomenon or even a specific set of cases within a phenomenon means that the pool of potential research participants can be very low.
Compile on top of this the fact that many people you approach may choose not to participate, and you could end up with a very small corpus of subjects to explore. This may limit your ability to make complete findings, even in a quantitative sense.
You may need to therefore limit your research question and objectives to something more realistic.
Suggested Solution and Response: Highlight that this is going to limit the study’s generalizability significantly.
14. Ethical Limitations
Ethical limitations refer to the things you cannot do based on ethical concerns identified either by yourself or your institution’s ethics review board.
This might include threats to the physical or psychological well-being of your research subjects, the potential of releasing data that could harm a person’s reputation, and so on.
Furthermore, even if your study follows all expected standards of ethics, you still, as an ethical researcher, need to allow a research participant to pull out at any point in time, after which you cannot use their data, which demonstrates an overlap between ethical constraints and participant attrition.
Suggested Solution and Response: Highlight that these ethical limitations are inevitable but important to sustain the integrity of the research.
For more on Qualitative Research, Explore my Qualitative Research Guide
Quantitative Research Limitations
Quantitative research focuses on quantifiable data and statistical, mathematical, or computational techniques. It’s often used to test hypotheses, assess relationships and causality, and generalize findings across larger populations.
Quantitative research is widely respected for its ability to provide reliable, measurable, and generalizable data (if done well!). Its structured methodology has strengths over qualitative research, such as the fact it allows for replication of the study, which underpins the validity of the research.
However, this approach is not without it limitations, explained below.
1. Over-Simplification
Quantitative research is powerful because it allows you to measure and analyze data in a systematic and standardized way. However, one of its limitations is that it can sometimes simplify complex phenomena or situations.
In other words, it might miss the subtleties or nuances of the research subject.
For example, if you’re studying why people choose a particular diet, a quantitative study might identify factors like age, income, or health status. But it might miss other aspects, such as cultural influences or personal beliefs, that can also significantly impact dietary choices.
When writing about this limitation, you can say that your quantitative approach, while providing precise measurements and comparisons, may not capture the full complexity of your subjects of study.
Suggested Solution and Response: Suggest a follow-up case study using the same research participants in order to gain additional context and depth.
2. Lack of Context
Another potential issue with quantitative research is that it often focuses on numbers and statistics at the expense of context or qualitative information.
Let’s say you’re studying the effect of classroom size on student performance. You might find that students in smaller classes generally perform better. However, this doesn’t take into account other variables, like teaching style , student motivation, or family support.
When describing this limitation, you might say, “Although our research provides important insights into the relationship between class size and student performance, it does not incorporate the impact of other potentially influential variables. Future research could benefit from a mixed-methods approach that combines quantitative analysis with qualitative insights.”
3. Applicability to Real-World Settings
Oftentimes, experimental research takes place in controlled environments to limit the influence of outside factors.
This control is great for isolation and understanding the specific phenomenon but can limit the applicability or “external validity” of the research to real-world settings.
For example, if you conduct a lab experiment to see how sleep deprivation impacts cognitive performance, the sterile, controlled lab environment might not reflect real-world conditions where people are dealing with multiple stressors.
Therefore, when explaining the limitations of your quantitative study in your methodology section, you could state:
“While our findings provide valuable information about [topic], the controlled conditions of the experiment may not accurately represent real-world scenarios where extraneous variables will exist. As such, the direct applicability of our results to broader contexts may be limited.”
Suggested Solution and Response: Suggest future studies that will engage in real-world observational research, such as ethnographic research.
4. Limited Flexibility
Once a quantitative study is underway, it can be challenging to make changes to it. This is because, unlike in grounded research, you’re putting in place your study in advance, and you can’t make changes part-way through.
Your study design, data collection methods, and analysis techniques need to be decided upon before you start collecting data.
For example, if you are conducting a survey on the impact of social media on teenage mental health, and halfway through, you realize that you should have included a question about their screen time, it’s generally too late to add it.
When discussing this limitation, you could write something like, “The structured nature of our quantitative approach allows for consistent data collection and analysis but also limits our flexibility to adapt and modify the research process in response to emerging insights and ideas.”
Suggested Solution and Response: Suggest future studies that will use mixed-methods or qualitative research methods to gain additional depth of insight.
5. Risk of Survey Error
Surveys are a common tool in quantitative research, but they carry risks of error.
There can be measurement errors (if a question is misunderstood), coverage errors (if some groups aren’t adequately represented), non-response errors (if certain people don’t respond), and sampling errors (if your sample isn’t representative of the population).
For instance, if you’re surveying college students about their study habits , but only daytime students respond because you conduct the survey during the day, your results will be skewed.
In discussing this limitation, you might say, “Despite our best efforts to develop a comprehensive survey, there remains a risk of survey error, including measurement, coverage, non-response, and sampling errors. These could potentially impact the reliability and generalizability of our findings.”
Suggested Solution and Response: Suggest future studies that will use other survey tools to compare and contrast results.
6. Limited Ability to Probe Answers
With quantitative research, you typically can’t ask follow-up questions or delve deeper into participants’ responses like you could in a qualitative interview.
For instance, imagine you are surveying 500 students about study habits in a questionnaire. A respondent might indicate that they study for two hours each night. You might want to follow up by asking them to elaborate on what those study sessions involve or how effective they feel their habits are.
However, quantitative research generally disallows this in the way a qualitative semi-structured interview could.
When discussing this limitation, you might write, “Given the structured nature of our survey, our ability to probe deeper into individual responses is limited. This means we may not fully understand the context or reasoning behind the responses, potentially limiting the depth of our findings.”
Suggested Solution and Response: Suggest future studies that engage in mixed-method or qualitative methodologies to address the issue from another angle.
7. Reliance on Instruments for Data Collection
In quantitative research, the collection of data heavily relies on instruments like questionnaires, surveys, or machines.
The limitation here is that the data you get is only as good as the instrument you’re using. If the instrument isn’t designed or calibrated well, your data can be flawed.
For instance, if you’re using a questionnaire to study customer satisfaction and the questions are vague, confusing, or biased, the responses may not accurately reflect the customers’ true feelings.
When discussing this limitation, you could say, “Our study depends on the use of questionnaires for data collection. Although we have put significant effort into designing and testing the instrument, it’s possible that inaccuracies or misunderstandings could potentially affect the validity of the data collected.”
Suggested Solution and Response: Suggest future studies that will use different instruments but examine the same variables to triangulate results.
8. Time and Resource Constraints (Specific to Quantitative Research)
Quantitative research can be time-consuming and resource-intensive, especially when dealing with large samples.
It often involves systematic sampling, rigorous design, and sometimes complex statistical analysis.
If resources and time are limited, it can restrict the scale of your research, the techniques you can employ, or the extent of your data analysis.
For example, you may want to conduct a nationwide survey on public opinion about a certain policy. However, due to limited resources, you might only be able to survey people in one city.
When writing about this limitation, you could say, “Given the scope of our research and the resources available, we are limited to conducting our survey within one city, which may not fully represent the nationwide public opinion. Hence, the generalizability of the results may be limited.”
Suggested Solution and Response: Suggest future studies that will have more funding or longer timeframes.
How to Discuss Your Research Limitations
1. in your research proposal and methodology section.
In the research proposal, which will become the methodology section of your dissertation, I would recommend taking the four following steps, in order:
- Be Explicit about your Scope – If you limit the scope of your study in your research question, aims, and objectives, then you can set yourself up well later in the methodology to say that certain questions are “outside the scope of the study.” For example, you may identify the fact that the study doesn’t address a certain variable, but you can follow up by stating that the research question is specifically focused on the variable that you are examining, so this limitation would need to be looked at in future studies.
- Acknowledge the Limitation – Acknowledging the limitations of your study demonstrates reflexivity and humility and can make your research more reliable and valid. It also pre-empts questions the people grading your paper may have, so instead of them down-grading you for your limitations; they will congratulate you on explaining the limitations and how you have addressed them!
- Explain your Decisions – You may have chosen your approach (despite its limitations) for a very specific reason. This might be because your approach remains, on balance, the best one to answer your research question. Or, it might be because of time and monetary constraints that are outside of your control.
- Highlight the Strengths of your Approach – Conclude your limitations section by strongly demonstrating that, despite limitations, you’ve worked hard to minimize the effects of the limitations and that you have chosen your specific approach and methodology because it’s also got some terrific strengths. Name the strengths.
Overall, you’ll want to acknowledge your own limitations but also explain that the limitations don’t detract from the value of your study as it stands.
2. In the Conclusion Section or Chapter
In the conclusion of your study, it is generally expected that you return to a discussion of the study’s limitations. Here, I recommend the following steps:
- Acknowledge issues faced – After completing your study, you will be increasingly aware of issues you may have faced that, if you re-did the study, you may have addressed earlier in order to avoid those issues. Acknowledge these issues as limitations, and frame them as recommendations for subsequent studies.
- Suggest further research – Scholarly research aims to fill gaps in the current literature and knowledge. Having established your expertise through your study, suggest lines of inquiry for future researchers. You could state that your study had certain limitations, and “future studies” can address those limitations.
- Suggest a mixed methods approach – Qualitative and quantitative research each have pros and cons. So, note those ‘cons’ of your approach, then say the next study should approach the topic using the opposite methodology or could approach it using a mixed-methods approach that could achieve the benefits of quantitative studies with the nuanced insights of associated qualitative insights as part of an in-study case-study.
Overall, be clear about both your limitations and how those limitations can inform future studies.
In sum, each type of research method has its own strengths and limitations. Qualitative research excels in exploring depth, context, and complexity, while quantitative research excels in examining breadth, generalizability, and quantifiable measures. Despite their individual limitations, each method contributes unique and valuable insights, and researchers often use them together to provide a more comprehensive understanding of the phenomenon being studied.
Attride-Stirling, J. (2001). Thematic networks: an analytic tool for qualitative research. Qualitative research , 1 (3), 385-405. ( Source )
Atkinson, P., Delamont, S., Cernat, A., Sakshaug, J., & Williams, R. A. (2021). SAGE research methods foundations . London: Sage Publications.
Clark, T., Foster, L., Bryman, A., & Sloan, L. (2021). Bryman’s social research methods . Oxford: Oxford University Press.
Köhler, T., Smith, A., & Bhakoo, V. (2022). Templates in qualitative research methods: Origins, limitations, and new directions. Organizational Research Methods , 25 (2), 183-210. ( Source )
Lenger, A. (2019). The rejection of qualitative research methods in economics. Journal of Economic Issues , 53 (4), 946-965. ( Source )
Taherdoost, H. (2022). What are different research approaches? Comprehensive review of qualitative, quantitative, and mixed method research, their applications, types, and limitations. Journal of Management Science & Engineering Research , 5 (1), 53-63. ( Source )
Walliman, N. (2021). Research methods: The basics . New York: Routledge.
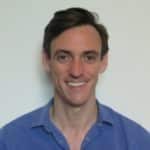
- Chris Drew (PhD) https://helpfulprofessor.com/author/chris-drew-phd-2/ 10 Reasons you’re Perpetually Single
- Chris Drew (PhD) https://helpfulprofessor.com/author/chris-drew-phd-2/ 20 Montessori Toddler Bedrooms (Design Inspiration)
- Chris Drew (PhD) https://helpfulprofessor.com/author/chris-drew-phd-2/ 21 Montessori Homeschool Setups
- Chris Drew (PhD) https://helpfulprofessor.com/author/chris-drew-phd-2/ 101 Hidden Talents Examples
Leave a Comment Cancel Reply
Your email address will not be published. Required fields are marked *

Organizing Academic Research Papers: Limitations of the Study
- Purpose of Guide
- Design Flaws to Avoid
- Glossary of Research Terms
- Narrowing a Topic Idea
- Broadening a Topic Idea
- Extending the Timeliness of a Topic Idea
- Academic Writing Style
- Choosing a Title
- Making an Outline
- Paragraph Development
- Executive Summary
- Background Information
- The Research Problem/Question
- Theoretical Framework
- Citation Tracking
- Content Alert Services
- Evaluating Sources
- Primary Sources
- Secondary Sources
- Tertiary Sources
- What Is Scholarly vs. Popular?
- Qualitative Methods
- Quantitative Methods
- Using Non-Textual Elements
- Limitations of the Study
- Common Grammar Mistakes
- Avoiding Plagiarism
- Footnotes or Endnotes?
- Further Readings
- Annotated Bibliography
- Dealing with Nervousness
- Using Visual Aids
- Grading Someone Else's Paper
- How to Manage Group Projects
- Multiple Book Review Essay
- Reviewing Collected Essays
- About Informed Consent
- Writing Field Notes
- Writing a Policy Memo
- Writing a Research Proposal
- Acknowledgements
The limitations of the study are those characteristics of design or methodology that impacted or influenced the application or interpretation of the results of your study. They are the constraints on generalizability and utility of findings that are the result of the ways in which you chose to design the study and/or the method used to establish internal and external validity.
Importance of...
Always acknowledge a study's limitations. It is far better for you to identify and acknowledge your study’s limitations than to have them pointed out by your professor and be graded down because you appear to have ignored them.
Keep in mind that acknowledgement of a study's limitations is an opportunity to make suggestions for further research. If you do connect your study's limitations to suggestions for further research, be sure to explain the ways in which these unanswered questions may become more focused because of your study.
Acknowledgement of a study's limitations also provides you with an opportunity to demonstrate to your professor that you have thought critically about the research problem, understood the relevant literature published about it, and correctly assessed the methods chosen for studying the problem. A key objective of the research process is not only discovering new knowledge but also to confront assumptions and explore what we don't know.
Claiming limitiations is a subjective process because you must evaluate the impact of those limitations . Don't just list key weaknesses and the magnitude of a study's limitations. To do so diminishes the validity of your research because it leaves the reader wondering whether, or in what ways, limitation(s) in your study may have impacted the findings and conclusions. Limitations require a critical, overall appraisal and interpretation of their impact. You should answer the question: do these problems with errors, methods, validity, etc. eventually matter and, if so, to what extent?
Structure: How to Structure the Research Limitations Section of Your Dissertation . Dissertations and Theses: An Online Textbook. Laerd.com.
Descriptions of Possible Limitations
All studies have limitations . However, it is important that you restrict your discussion to limitations related to the research problem under investigation. For example, if a meta-analysis of existing literature is not a stated purpose of your research, it should not be discussed as a limitation. Do not apologize for not addressing issues that you did not promise to investigate in your paper.
Here are examples of limitations you may need to describe and to discuss how they possibly impacted your findings. Descriptions of limitations should be stated in the past tense.
Possible Methodological Limitations
- Sample size -- the number of the units of analysis you use in your study is dictated by the type of research problem you are investigating. Note that, if your sample size is too small, it will be difficult to find significant relationships from the data, as statistical tests normally require a larger sample size to ensure a representative distribution of the population and to be considered representative of groups of people to whom results will be generalized or transferred.
- Lack of available and/or reliable data -- a lack of data or of reliable data will likely require you to limit the scope of your analysis, the size of your sample, or it can be a significant obstacle in finding a trend and a meaningful relationship. You need to not only describe these limitations but to offer reasons why you believe data is missing or is unreliable. However, don’t just throw up your hands in frustration; use this as an opportunity to describe the need for future research.
- Lack of prior research studies on the topic -- citing prior research studies forms the basis of your literature review and helps lay a foundation for understanding the research problem you are investigating. Depending on the currency or scope of your research topic, there may be little, if any, prior research on your topic. Before assuming this to be true, consult with a librarian! In cases when a librarian has confirmed that there is a lack of prior research, you may be required to develop an entirely new research typology [for example, using an exploratory rather than an explanatory research design]. Note that this limitation can serve as an important opportunity to describe the need for further research.
- Measure used to collect the data -- sometimes it is the case that, after completing your interpretation of the findings, you discover that the way in which you gathered data inhibited your ability to conduct a thorough analysis of the results. For example, you regret not including a specific question in a survey that, in retrospect, could have helped address a particular issue that emerged later in the study. Acknowledge the deficiency by stating a need in future research to revise the specific method for gathering data.
- Self-reported data -- whether you are relying on pre-existing self-reported data or you are conducting a qualitative research study and gathering the data yourself, self-reported data is limited by the fact that it rarely can be independently verified. In other words, you have to take what people say, whether in interviews, focus groups, or on questionnaires, at face value. However, self-reported data contain several potential sources of bias that should be noted as limitations: (1) selective memory (remembering or not remembering experiences or events that occurred at some point in the past); (2) telescoping [recalling events that occurred at one time as if they occurred at another time]; (3) attribution [the act of attributing positive events and outcomes to one's own agency but attributing negative events and outcomes to external forces]; and, (4) exaggeration [the act of representing outcomes or embellishing events as more significant than is actually suggested from other data].
Possible Limitations of the Researcher
- Access -- if your study depends on having access to people, organizations, or documents and, for whatever reason, access is denied or otherwise limited, the reasons for this need to be described.
- Longitudinal effects -- unlike your professor, who can literally devote years [even a lifetime] to studying a single research problem, the time available to investigate a research problem and to measure change or stability within a sample is constrained by the due date of your assignment. Be sure to choose a topic that does not require an excessive amount of time to complete the literature review, apply the methodology, and gather and interpret the results. If you're unsure, talk to your professor.
- Cultural and other type of bias -- we all have biases, whether we are conscience of them or not. Bias is when a person, place, or thing is viewed or shown in a consistently inaccurate way. It is usually negative, though one can have a positive bias as well. When proof-reading your paper, be especially critical in reviewing how you have stated a problem, selected the data to be studied, what may have been omitted, the manner in which you have ordered events, people, or places and how you have chosen to represent a person, place, or thing, to name a phenomenon, or to use possible words with a positive or negative connotation. Note that if you detect bias in prior research, it must be acknowledged and you should explain what measures were taken to avoid perpetuating bias.
- Fluency in a language -- if your research focuses on measuring the perceived value of after-school tutoring among Mexican-American ESL [English as a Second Language] students, for example, and you are not fluent in Spanish, you are limited in being able to read and interpret Spanish language research studies on the topic. This deficiency should be acknowledged.
Brutus, Stéphane et al. Self-Reported Limitations and Future Directions in Scholarly Reports: Analysis and Recommendations. Journal of Management 39 (January 2013): 48-75; Senunyeme, Emmanuel K. Business Research Methods . Powerpoint Presentation. Regent University of Science and Technology.
Structure and Writing Style
Information about the limitations of your study are generally placed either at the beginning of the discussion section of your paper so the reader knows and understands the limitations before reading the rest of your analysis of the findings, or, the limitations are outlined at the conclusion of the discussion section as an acknowledgement of the need for further study. Statements about a study's limitations should not be buried in the body [middle] of the discussion section unless a limitation is specific to something covered in that part of the paper. If this is the case, though, the limitation should be reiterated at the conclusion of the section.
If you determine that your study is seriously flawed due to important limitations , such as, an inability to acquire critical data, consider reframing it as a pilot study intended to lay the groundwork for a more complete research study in the future. Be sure, though, to specifically explain the ways that these flaws can be successfully overcome in later studies.
But, do not use this as an excuse for not developing a thorough research paper! Review the tab in this guide for developing a research topic . If serious limitations exist, it generally indicates a likelihood that your research problem is too narrowly defined or that the issue or event under study is too recent and, thus, very little research has been written about it. If serious limitations do emerge, consult with your professor about possible ways to overcome them or how to reframe your study.
When discussing the limitations of your research, be sure to:
- Describe each limitation in detailed but concise terms;
- Explain why each limitation exists;
- Provide the reasons why each limitation could not be overcome using the method(s) chosen to gather the data [cite to other studies that had similar problems when possible];
- Assess the impact of each limitation in relation to the overall findings and conclusions of your study; and,
- If appropriate, describe how these limitations could point to the need for further research.
Remember that the method you chose may be the source of a significant limitation that has emerged during your interpretation of the results [for example, you didn't ask a particular question in a survey that you later wish you had]. If this is the case, don't panic. Acknowledge it, and explain how applying a different or more robust methodology might address the research problem more effectively in any future study. A underlying goal of scholarly research is not only to prove what works, but to demonstrate what doesn't work or what needs further clarification.
Brutus, Stéphane et al. Self-Reported Limitations and Future Directions in Scholarly Reports: Analysis and Recommendations. Journal of Management 39 (January 2013): 48-75; Ioannidis, John P.A. Limitations are not Properly Acknowledged in the Scientific Literature. Journal of Clinical Epidemiology 60 (2007): 324-329; Pasek, Josh. Writing the Empirical Social Science Research Paper: A Guide for the Perplexed . January 24, 2012. Academia.edu; Structure: How to Structure the Research Limitations Section of Your Dissertation . Dissertations and Theses: An Online Textbook. Laerd.com; What Is an Academic Paper? Institute for Writing Rhetoric. Dartmouth College; Writing the Experimental Report: Methods, Results, and Discussion. The Writing Lab and The OWL. Purdue University.
Writing Tip
Don't Inflate the Importance of Your Findings! After all the hard work and long hours devoted to writing your research paper, it is easy to get carried away with attributing unwarranted importance to what you’ve done. We all want our academic work to be viewed as excellent and worthy of a good grade, but it is important that you understand and openly acknowledge the limitiations of your study. Inflating of the importance of your study's findings in an attempt hide its flaws is a big turn off to your readers. A measure of humility goes a long way!
Another Writing Tip
Negative Results are Not a Limitation!
Negative evidence refers to findings that unexpectedly challenge rather than support your hypothesis. If you didn't get the results you anticipated, it may mean your hypothesis was incorrect and needs to be reformulated, or, perhaps you have stumbled onto something unexpected that warrants further study. Moreover, the absence of an effect may be very telling in many situations, particularly in experimental research designs. In any case, your results may be of importance to others even though they did not support your hypothesis. Do not fall into the trap of thinking that results contrary to what you expected is a limitation to your study. If you carried out the research well, they are simply your results and only require additional interpretation.
Yet Another Writing Tip
A Note about Sample Size Limitations in Qualitative Research
Sample sizes are typically smaller in qualitative research because, as the study goes on, acquiring more data does not necessarily lead to more information. This is because one occurrence of a piece of data, or a code, is all that is necessary to ensure that it becomes part of the analysis framework. However, it remains true that sample sizes that are too small cannot adequately support claims of having achieved valid conclusions and sample sizes that are too large do not permit the deep, naturalistic, and inductive analysis that defines qualitative inquiry. Determining adequate sample size in qualitative research is ultimately a matter of judgment and experience in evaluating the quality of the information collected against the uses to which it will be applied and the particular research method and purposeful sampling strategy employed. If the sample size is found to be a limitation, it may reflect your judgement about the methodological technique chosen [e.g., single life history study versus focus group interviews] rather than the number of respondents used.
Huberman, A. Michael and Matthew B. Miles. Data Management and Analysis Methods. In Handbook of Qualitative Research. Norman K. Denzin and Yvonna S. Lincoln, eds. (Thousand Oaks, CA: Sage, 1994), pp. 428-444.
- << Previous: 8. The Discussion
- Next: 9. The Conclusion >>
- Last Updated: Jul 18, 2023 11:58 AM
- URL: https://library.sacredheart.edu/c.php?g=29803
- QuickSearch
- Library Catalog
- Databases A-Z
- Publication Finder
- Course Reserves
- Citation Linker
- Digital Commons
- Our Website
Research Support
- Ask a Librarian
- Appointments
- Interlibrary Loan (ILL)
- Research Guides
- Databases by Subject
- Citation Help
Using the Library
- Reserve a Group Study Room
- Renew Books
- Honors Study Rooms
- Off-Campus Access
- Library Policies
- Library Technology
User Information
- Grad Students
- Online Students
- COVID-19 Updates
- Staff Directory
- News & Announcements
- Library Newsletter
My Accounts
- Interlibrary Loan
- Staff Site Login

FIND US ON
Educational resources and simple solutions for your research journey
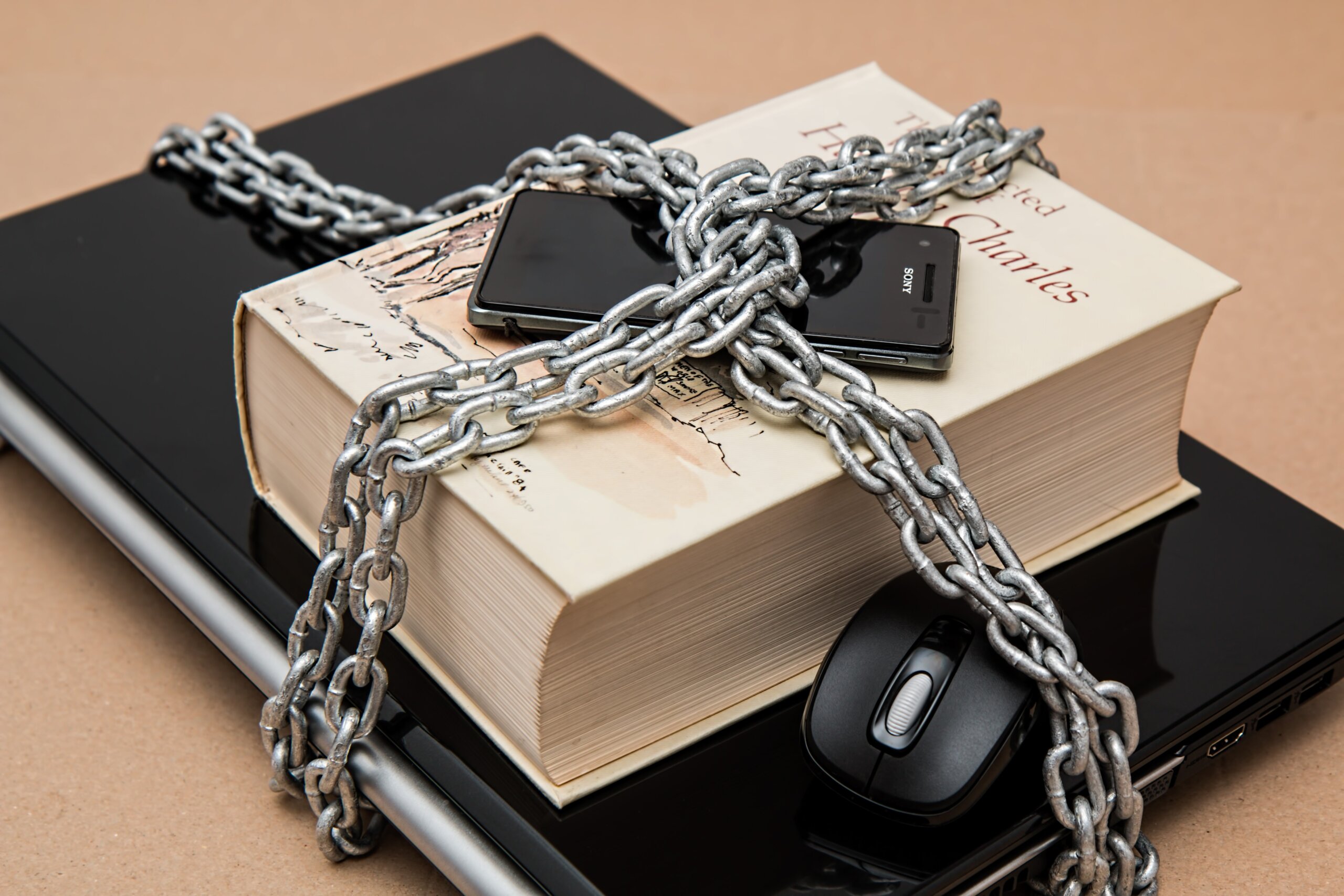
How to Present the Limitations of a Study in Research?
The limitations of the study convey to the reader how and under which conditions your study results will be evaluated. Scientific research involves investigating research topics, both known and unknown, which inherently includes an element of risk. The risk could arise due to human errors, barriers to data gathering, limited availability of resources, and researcher bias. Researchers are encouraged to discuss the limitations of their research to enhance the process of research, as well as to allow readers to gain an understanding of the study’s framework and value.
Limitations of the research are the constraints placed on the ability to generalize from the results and to further describe applications to practice. It is related to the utility value of the findings based on how you initially chose to design the study, the method used to establish internal and external validity, or the result of unanticipated challenges that emerged during the study. Knowing about these limitations and their impact can explain how the limitations of your study can affect the conclusions and thoughts drawn from your research. 1
Table of Contents
What are the limitations of a study
Researchers are probably cautious to acknowledge what the limitations of the research can be for fear of undermining the validity of the research findings. No research can be faultless or cover all possible conditions. These limitations of your research appear probably due to constraints on methodology or research design and influence the interpretation of your research’s ultimate findings. 2 These are limitations on the generalization and usability of findings that emerge from the design of the research and/or the method employed to ensure validity internally and externally. But such limitations of the study can impact the whole study or research paper. However, most researchers prefer not to discuss the different types of limitations in research for fear of decreasing the value of their paper amongst the reviewers or readers.
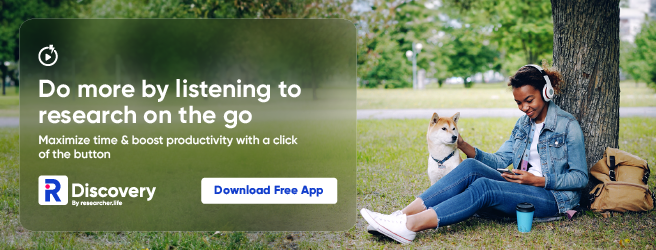
Importance of limitations of a study
Writing the limitations of the research papers is often assumed to require lots of effort. However, identifying the limitations of the study can help structure the research better. Therefore, do not underestimate the importance of research study limitations. 3
- Opportunity to make suggestions for further research. Suggestions for future research and avenues for further exploration can be developed based on the limitations of the study.
- Opportunity to demonstrate critical thinking. A key objective of the research process is to discover new knowledge while questioning existing assumptions and exploring what is new in the particular field. Describing the limitation of the research shows that you have critically thought about the research problem, reviewed relevant literature, and correctly assessed the methods chosen for studying the problem.
- Demonstrate Subjective learning process. Writing limitations of the research helps to critically evaluate the impact of the said limitations, assess the strength of the research, and consider alternative explanations or interpretations. Subjective evaluation contributes to a more complex and comprehensive knowledge of the issue under study.
Why should I include limitations of research in my paper
All studies have limitations to some extent. Including limitations of the study in your paper demonstrates the researchers’ comprehensive and holistic understanding of the research process and topic. The major advantages are the following:
- Understand the study conditions and challenges encountered . It establishes a complete and potentially logical depiction of the research. The boundaries of the study can be established, and realistic expectations for the findings can be set. They can also help to clarify what the study is not intended to address.
- Improve the quality and validity of the research findings. Mentioning limitations of the research creates opportunities for the original author and other researchers to undertake future studies to improve the research outcomes.
- Transparency and accountability. Including limitations of the research helps maintain mutual integrity and promote further progress in similar studies.
- Identify potential bias sources. Identifying the limitations of the study can help researchers identify potential sources of bias in their research design, data collection, or analysis. This can help to improve the validity and reliability of the findings.
Where do I need to add the limitations of the study in my paper
The limitations of your research can be stated at the beginning of the discussion section, which allows the reader to comprehend the limitations of the study prior to reading the rest of your findings or at the end of the discussion section as an acknowledgment of the need for further research.
Types of limitations in research
There are different types of limitations in research that researchers may encounter. These are listed below:
- Research Design Limitations : Restrictions on your research or available procedures may affect the research outputs. If the research goals and objectives are too broad, explain how they should be narrowed down to enhance the focus of your study. If there was a selection bias in your sample, explain how this may affect the generalizability of your findings. This can help readers understand the limitations of the study in terms of their impact on the overall validity of your research.
- Impact Limitations : Your study might be limited by a strong regional-, national-, or species-based impact or population- or experimental-specific impact. These inherent limitations on impact affect the extendibility and generalizability of the findings.
- Data or statistical limitations : Data or statistical limitations in research are extremely common in experimental (such as medicine, physics, and chemistry) or field-based (such as ecology and qualitative clinical research) studies. Sometimes, it is either extremely difficult to acquire sufficient data or gain access to the data. These limitations of the research might also be the result of your study’s design and might result in an incomplete conclusion to your research.
Limitations of study examples
All possible limitations of the study cannot be included in the discussion section of the research paper or dissertation. It will vary greatly depending on the type and nature of the study. These include types of research limitations that are related to methodology and the research process and that of the researcher as well that you need to describe and discuss how they possibly impacted your results.
Common methodological limitations of the study
Limitations of research due to methodological problems are addressed by identifying the potential problem and suggesting ways in which this should have been addressed. Some potential methodological limitations of the study are as follows. 1
- Sample size: The sample size 4 is dictated by the type of research problem investigated. If the sample size is too small, finding a significant relationship from the data will be difficult, as statistical tests require a large sample size to ensure a representative population distribution and generalize the study findings.
- Lack of available/reliable data: A lack of available/reliable data will limit the scope of your analysis and the size of your sample or present obstacles in finding a trend or meaningful relationship. So, when writing about the limitations of the study, give convincing reasons why you feel data is absent or untrustworthy and highlight the necessity for a future study focused on developing a new data-gathering strategy.
- Lack of prior research studies: Citing prior research studies is required to help understand the research problem being investigated. If there is little or no prior research, an exploratory rather than an explanatory research design will be required. Also, discovering the limitations of the study presents an opportunity to identify gaps in the literature and describe the need for additional study.
- Measure used to collect the data: Sometimes, the data gathered will be insufficient to conduct a thorough analysis of the results. A limitation of the study example, for instance, is identifying in retrospect that a specific question could have helped address a particular issue that emerged during data analysis. You can acknowledge the limitation of the research by stating the need to revise the specific method for gathering data in the future.
- Self-reported data: Self-reported data cannot be independently verified and can contain several potential bias sources, such as selective memory, attribution, and exaggeration. These biases become apparent if they are incongruent with data from other sources.
General limitations of researchers
Limitations related to the researcher can also influence the study outcomes. These should be addressed, and related remedies should be proposed.
- Limited access to data : If your study requires access to people, organizations, data, or documents whose access is denied or limited, the reasons need to be described. An additional explanation stating why this limitation of research did not prevent you from following through on your study is also needed.
- Time constraints : Researchers might also face challenges in meeting research deadlines due to a lack of timely participant availability or funds, among others. The impacts of time constraints must be acknowledged by mentioning the need for a future study addressing this research problem.
- Conflicts due to biased views and personal issues : Differences in culture or personal views can contribute to researcher bias, as they focus only on the results and data that support their main arguments. To avoid this, pay attention to the problem statement and data gathering.
Steps for structuring the limitations section
Limitations are an inherent part of any research study. Issues may vary, ranging from sampling and literature review to methodology and bias. However, there is a structure for identifying these elements, discussing them, and offering insight or alternatives on how the limitations of the study can be mitigated. This enhances the process of the research and helps readers gain a comprehensive understanding of a study’s conditions.
- Identify the research constraints : Identify those limitations having the greatest impact on the quality of the research findings and your ability to effectively answer your research questions and/or hypotheses. These include sample size, selection bias, measurement error, or other issues affecting the validity and reliability of your research.
- Describe their impact on your research : Reflect on the nature of the identified limitations and justify the choices made during the research to identify the impact of the study’s limitations on the research outcomes. Explanations can be offered if needed, but without being defensive or exaggerating them. Provide context for the limitations of your research to understand them in a broader context. Any specific limitations due to real-world considerations need to be pointed out critically rather than justifying them as done by some other author group or groups.
- Mention the opportunity for future investigations : Suggest ways to overcome the limitations of the present study through future research. This can help readers understand how the research fits into the broader context and offer a roadmap for future studies.
Frequently Asked Questions
- Should I mention all the limitations of my study in the research report?
Restrict limitations to what is pertinent to the research question under investigation. The specific limitations you include will depend on the nature of the study, the research question investigated, and the data collected.
- Can the limitations of a study affect its credibility?
Stating the limitations of the research is considered favorable by editors and peer reviewers. Connecting your study’s limitations with future possible research can help increase the focus of unanswered questions in this area. In addition, admitting limitations openly and validating that they do not affect the main findings of the study increases the credibility of your study. However, if you determine that your study is seriously flawed, explain ways to successfully overcome such flaws in a future study. For example, if your study fails to acquire critical data, consider reframing the research question as an exploratory study to lay the groundwork for more complete research in the future.
- How can I mitigate the limitations of my study?
Strategies to minimize limitations of the research should focus on convincing reviewers and readers that the limitations do not affect the conclusions of the study by showing that the methods are appropriate and that the logic is sound. Here are some steps to follow to achieve this:
- Use data that are valid.
- Use methods that are appropriate and sound logic to draw inferences.
- Use adequate statistical methods for drawing inferences from the data that studies with similar limitations have been published before.
Admit limitations openly and, at the same time, show how they do not affect the main conclusions of the study.
- Can the limitations of a study impact its publication chances?
Limitations in your research can arise owing to restrictions in methodology or research design. Although this could impact your chances of publishing your research paper, it is critical to explain your study’s limitations to your intended audience. For example, it can explain how your study constraints may impact the results and views generated from your investigation. It also shows that you have researched the flaws of your study and have a thorough understanding of the subject.
- How can limitations in research be used for future studies?
The limitations of a study give you an opportunity to offer suggestions for further research. Your study’s limitations, including problems experienced during the study and the additional study perspectives developed, are a great opportunity to take on a new challenge and help advance knowledge in a particular field.
References:
- Brutus, S., Aguinis, H., & Wassmer, U. (2013). Self-reported limitations and future directions in scholarly reports: Analysis and recommendations. Journal of Management , 39 (1), 48-75.
- Ioannidis, J. P. (2007). Limitations are not properly acknowledged in the scientific literature. Journal of Clinical Epidemiology , 60 (4), 324-329.
- Price, J. H., & Murnan, J. (2004). Research limitations and the necessity of reporting them. American Journal of Health Education , 35 (2), 66.
- Boddy, C. R. (2016). Sample size for qualitative research. Qualitative Market Research: An International Journal , 19 (4), 426-432.
R Discovery is a literature search and research reading platform that accelerates your research discovery journey by keeping you updated on the latest, most relevant scholarly content. With 250M+ research articles sourced from trusted aggregators like CrossRef, Unpaywall, PubMed, PubMed Central, Open Alex and top publishing houses like Springer Nature, JAMA, IOP, Taylor & Francis, NEJM, BMJ, Karger, SAGE, Emerald Publishing and more, R Discovery puts a world of research at your fingertips.
Try R Discovery Prime FREE for 1 week or upgrade at just US$72 a year to access premium features that let you listen to research on the go, read in your language, collaborate with peers, auto sync with reference managers, and much more. Choose a simpler, smarter way to find and read research – Download the app and start your free 7-day trial today !
Related Posts

What is Research Impact: Types and Tips for Academics

Research in Shorts: R Discovery’s New Feature Helps Academics Assess Relevant Papers in 2mins
- Open access
- Published: 05 September 2024
The goldmine of GWAS summary statistics: a systematic review of methods and tools
- Panagiota I. Kontou 1 &
- Pantelis G. Bagos 2
BioData Mining volume 17 , Article number: 31 ( 2024 ) Cite this article
3 Altmetric
Metrics details
Genome-wide association studies (GWAS) have revolutionized our understanding of the genetic architecture of complex traits and diseases. GWAS summary statistics have become essential tools for various genetic analyses, including meta-analysis, fine-mapping, and risk prediction. However, the increasing number of GWAS summary statistics and the diversity of software tools available for their analysis can make it challenging for researchers to select the most appropriate tools for their specific needs. This systematic review aims to provide a comprehensive overview of the currently available software tools and databases for GWAS summary statistics analysis. We conducted a comprehensive literature search to identify relevant software tools and databases. We categorized the tools and databases by their functionality, including data management, quality control, single-trait analysis, and multiple-trait analysis. We also compared the tools and databases based on their features, limitations, and user-friendliness. Our review identified a total of 305 functioning software tools and databases dedicated to GWAS summary statistics, each with unique strengths and limitations. We provide descriptions of the key features of each tool and database, including their input/output formats, data types, and computational requirements. We also discuss the overall usability and applicability of each tool for different research scenarios. This comprehensive review will serve as a valuable resource for researchers who are interested in using GWAS summary statistics to investigate the genetic basis of complex traits and diseases. By providing a detailed overview of the available tools and databases, we aim to facilitate informed tool selection and maximize the effectiveness of GWAS summary statistics analysis.
Peer Review reports
Genome-wide association studies (GWAS) enable the simultaneous testing of thousands of genetic variants, usually SNPs, across the genome in order to find variants associated with a trait or a disease [ 1 ]. The GWAS methodology, so far, has generated many robust associations for various traits and diseases and has revolutionized our understanding of the genetic architecture of complex traits. With increasing sample sizes, new sequencing technologies and the accumulation of large biobanks it is expected that our ability to investigate the effects of human genetic variation in complex traits will increase in the near future [ 2 ]. In the first years of the development of the field, efforts were oriented towards the statistical aspects of the analysis [ 3 ], which involved thousands of SNPs simultaneously, including the methodology for multiple testing and quality control. This task was successful and enabled the discovery of associations replicated in subsequent studies, and in several cases, validated experimentally and functionally using a wide variety of methods [ 4 ]. However, it was soon clear that most variants discovered via GWAS have small overall effects on disease susceptibility [ 5 ]. Thus, it became evident that integrating data from multiple sources and developing reliable bioinformatics tools was a necessary step in order to address the complexity of the underlying genetic basis of common human diseases [ 5 ].
Soon after the publication of the first GWAS it also became evident that, at least theoretically, individuals could be identified in such cohorts even if only the summary statistics are available [ 6 ]. This led to imposing strict control access for sharing individual patients’ data (IPD) from GWAS. Subsequent works found that privacy attacks are possible in theory but unsuccessful and unconvincing in real practice. For instance, even sharing 1,000 SNPs for datasets with more than 500 individuals generally leads to a low power of the “attack” [ 7 ]. A more thorough investigation is given in [ 8 ]. In practice, however, not all studies share their data, at least when it comes to the studies published in the first decade of GWAS. It has been estimated that the proportion is only 13%, which increased from 3% in 2010 to 23% in 2017 [ 9 ]. On the contrary, researchers sharing their summary data has been shown to receive on average 81.8% more citations, an effect that probably is related, at least partially, to the usability of the data in downstream analyses [ 10 ]. Summary statistics do not only offer the additional protection of privacy, but also offer significant advantages in computational cost when using the data in downstream analyses, which does not scale with the number of participants in the study [ 11 ]. Thus, it is of no surprise that during the last years a large variety of methods have been developed to perform a so-called post-GWAS analysis using the summary results of a single study, or of several studies, and in most cases integrating data from other sources [ 11 ]. The majority of these methods use the summary data in the form of per-allele SNP effect sizes (log odds ratios or betas) along with their standard errors, or equivalently the z-scores (per-allele effect sizes divided by their standard errors). These methods seek to go a step further from the simple analysis, or re-analysis of a study, and aim to improve our understanding about the functional role of the identified variants [ 12 ]. The most important factors that played significant role in the development of such methods, in this so-called post-GWAS era, is the linkage disequilibrium (LD) information from a population reference panel such as HapMap or 1000 Genomes Project, the gene expression variation in the form of eQTL, and the integration of functional information on biological pathways [ 13 , 14 , 15 ].
The methods developed so far cover a broad range of different types of analysis, either in the study of a single trait or in the combined analysis of multiple traits. For a single trait, we may have methods for meta-analysis [ 16 , 17 ], methods for inferring heritability [ 18 , 19 ], gene-based tests [ 20 ], methods for Gene Set (or Pathway) Analysis [ 21 ], or methods for fine-mapping causal variants [ 22 ]. Regarding the analysis of multiple traits there is also a variety of methods [ 23 ], ranging from those that estimate the genetic correlation between traits [ 24 ], the joint analysis of multiple traits [ 25 ], or the methods that try to estimate causality between traits such as Mendelian Randomization [ 26 ], transcriptome-wide association studies [ 27 ], or colocalization [ 28 ]. Of course, the data standards [ 29 ] used to facilitate these analyses and the databases that the results are stored in, are also of great importance for the community.
In order to provide a comprehensive overview of the currently available software tools and databases for GWAS summary statistics we performed a systematic review following the PRISMA guidelines [ 30 ]. We conducted a comprehensive search of the literature to identify relevant software tools and databases. We categorized the tools and databases by their functionality, in categories related to data, single-trait analysis, and multiple-trait analysis, along with their sub-categories mentioned in the previous paragraph. We also compared the tools and databases based on their features, limitations, and user-friendliness. Our review identified a wide range of software tools and databases for GWAS summary statistics analysis, each with unique strengths and limitations. We provide descriptions of the key features of each tool and database, including their input/output formats, data types, and computational requirements. We also discuss the overall usability and applicability of each tool for different research scenarios. This comprehensive review will serve as a valuable resource for researchers who are interested in using GWAS summary statistics to investigate the genetic basis of complex traits and diseases. By providing a detailed overview of the available tools and databases, we aim to facilitate informed tool selection and maximize the effectiveness of using GWAS summary statistics.
The systematic review
In order to collect all the available published papers, we performed a systematic review of the literature following the PRISMA guidelines [ 30 ]. The search was performed in PubMed ( https://pubmed.ncbi.nlm.nih.gov ) with the following query: ("Summary Statistics" OR "Summary Data" OR "Summary Association Statistics" OR "Summary Association Data") AND (GWAS OR genomewide OR genome-wide ). The abstracts initially, and then the full articles were scrutinized in order to collect the necessary information. The inclusion criteria state that methods, software tools and databases, suitable for the analysis of GWAS summary data are suitable for inclusion. Methods papers that do not report software, or software pages not currently available are excluded. Additional searches were performed in the reference lists of the identified articles in order to identify additional studies that were missing. In many cases multiple articles regarding a single tool were found, so we kept only one. We decided to include reports deposited in preprint servers like medRxiv/bioRxiv, but some of these papers were eventually published in peer-review journals, so in such cases we retained only the latter reference. Tools regarding Polygenic Risk Scores (PRSs) and visualization were excluded. For all included tools we recorded the URL, the PMID, and the main functionality/es along with comments regarding its main methodological features. The initial search identified 2942 articles (22/12/2023).
In total we identified 305 tools and databases (Fig. 1 ). We classified them in three broad categories: data , tools for single traits and tools for multiple traits , along with the various sub-categories. The total breakdown is given in Table 1 . Several tools may perform different tasks and thus they can be considered for more than one category; so, we classified them to the one most closely related to the primary goal of the analysis they claim to perform. Other tools do not fit exactly to the general description of the category, but we nevertheless classified them to the most similar one. The largest sub-category consists of the tools for pleiotropy analysis, whereas the smallest one is related to reconstruction of genotypes and effect sizes. Most tools are written in R (56.4%) with the largest proportion being in the multiple traits category, followed by Python (12.5%) and C/C + + (8.2%) (Fig. 2 ). Apart from the publicly available databases only a handful of tools are offered as webservers (6.95%). Most of the tools were published after 2015 (Fig. 3 ). Nearly 60% of the tools and databases were published in: Bioinformatics, American Journal of Human Genetics, Nature Genetics, Nature Communications, Nucleic Acids Research and PloS Genetics (Fig. 4 ). In the following sections we proceed with the detailed description of the various tools identified, classified in the different categories and sub-categories. The complete list of identified tools along with the relevant information is given in Supplementary Table 1.
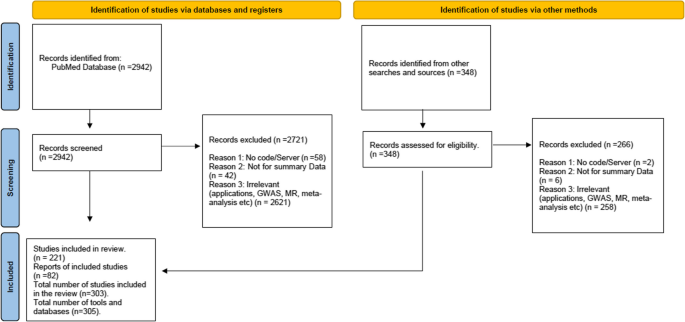
PRISMA Flow diagram for systematic review
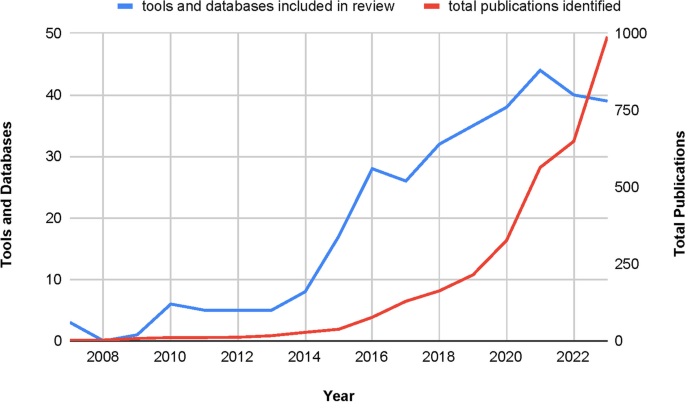
Number of Tools and Databases included in the review Published Per Year
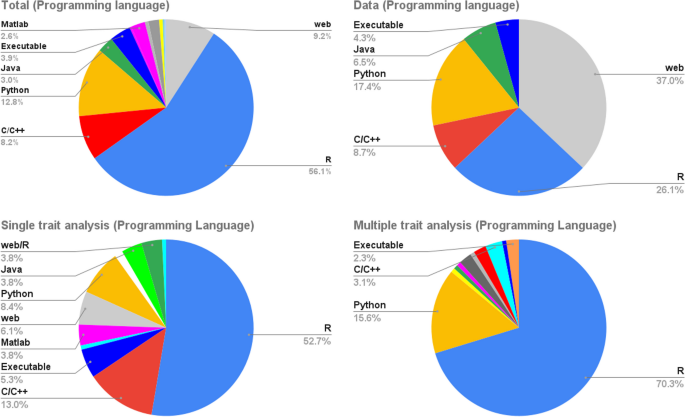
The programming languages used in the various categories of identified tools
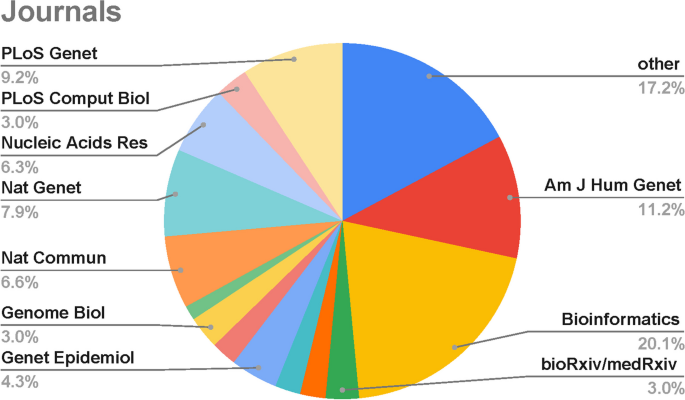
The journals in which the studies including in the review were published
Firstly, we are going to present the tools dedicated to the data themselves. We include here tools for quality control of GWA summary statistics, tools for imputation and genotype reconstruction as well as the publicly available databases of summary results.
Standards and quality control
The need for sharing and re-using GWAS summary statistics has been an issue for the community during the last years. Generally, it is acceptable that the minimum information (“mandatory”) contained in GWAS summary statistics should include: the chromosome and the base-pair location, the p-value of the association, the risk allele and the other allele, the risk allele frequency, and an estimate of the effect size (odds ratio or beta) along with its standard error [ 29 ]. Other important summary statistics that nevertheless termed as “encouraged” ones include the sample size, the variant ID, the rsID, the confidence interval of the effect size and so on. Such specifications were considered for the GWAS-SSF format [ 31 ], which was developed to meet the requirements settled by the community. GWAS-SSF consists of a tab-separated data file with well-defined fields and an accompanying metadata file. Most repositories and programs use some variant of the GWAS-SSF. However, such tabular formats in several cases lead to ambiguity or incomplete storage of information, or other times lack essential metadata. This leads to poor performance and increased risk of possible errors in downstream analyses. To address these issues, an adaptation of the well-known variant call format [ 32 ] was developed, capable of storing GWAS summary statistics which was called GWAS-VCF along with software tools to apply it in downstream analyses [ 33 ]. The VCF contains a file header with metadata and a main file containing variant-level (one locus per row with one or more alternative alleles/variants) and sample-level (one sample per column) information. This way, the VCF was adapted to include GWAS-specific metadata utilizing the sample column to store variant-trait association data. The GWAS-VCF is the standard used by the MRC-IEU OpenGWAS database [ 34 ] and it comes with appropriate tools to map GWAS summary statistics to VCF with on-the-fly harmonization ( https://github.com/mrcieu/gwas2vcf ).
Despite these efforts, not all available data are in line with the standards, especially when dealing with data from older studies. Thus, there is a need for additional tools to harmonize the data, as well as to identify and correct errors. Tools belonging to the former class were developed early and were focused mainly on harmonizing data in preparation of a meta-analysis. These include QCGWAS [ 35 ], GWAtoolbox [ 36 ] and EasyQC [ 37 ]. GEAR [ 38 ] is very interesting in that it incorporates ideas from population genetics which allow verification of the genetic origin and geographic location of each cohort and identifying significant sample overlap. More recent tools like MungeSumstats [ 39 ] and GWASlab [ 40 ] perform standardization and quality control handling the most common formats, SumStatsRehab [ 41 ] can be used for data validation, restoration of missing data, correction of errors or formatting, and GWASinspector [ 42 ] provides extensive QC reports and perform harmonization being compatible with recent reference panels and by handling insertion/deletion and multi-allelic variants. The latter class of methods, additionally, leverages information from the LD among SNPs. One such tool is GQS [ 43 ] which identifies suspicious regions and prevents erroneous interpretations by comparing the significance of the association for each SNP to its LD value for the reported index SNP. Similar functionalities are offered by DENTIST [ 44 ] which uses LD to detect and eliminate errors and disagreements between GWAS data and the LD reference panel. EXTminus23andMe [ 45 ] evaluates the quality of summary statistics after data removal and the suitability of the down sampled summary statistics for typical follow-up genetic analyses.
The publicly available biological databases played and continue to play a central role in bioinformatics and in biological research in general [ 46 , 47 , 48 ]. The same is the case for databases related to human research [ 49 ] and in particular those involved in GWAS [ 50 ]. The databases we identified can be roughly divided in two categories: databases that contain summary statistics from GWAS and databases that contain important secondary analyses on those data with some of the methods that we will describe in later sections.
Regarding the databases of the first category, NCBI’s dbGAP [ 51 ] was developed to contain the results of studies investigating the interaction of genotype and phenotype, which include GWAS. One of the dbGAP’s primary objectives was to house individual level GWAS data, but the database also contains summary data as well. Summary statistics are generally available to the public, whereas access to IPD requires varying levels of authorization. The NHGRI-EBI GWAS Catalog [ 52 ], which was established in 2008 is considered for years the central repository of GWAS summary statistics. It is a high-quality curated collection of all published GWAS and as of 2023–12-20, contains 6,680 publications, 566,798 top associations and 66,825 full summary statistics (Fig. 5 ). The database played an important role in the community efforts leading to the development of GWAS-SSF format. GWAScentral [ 53 ] previously known as the Human Genome Variation (HGV) database of Genotype-to-Phenotype information is a database that contains over 72.5 million P-values for over 5,000 studies, with over 7.4 million unique genetic markers involved in more than 1,700 unique phenotypes. The database contains data from several sources (including NHGRI-EBI GWAS Catalog, OpenGWAS, Japanese GWASdb, dbGaP, WTCCC and so on). The IEU MRC OpenGWAS [ 34 ] is a new addition and contains 346 million genetic associations from 50,037 GWAS summary datasets. It contains complete data from various consortia and the UK Biobank and comes with a lot of tools for harmonizing the data and storing them in the GWAS-VCF format. At the time of writing there are 4,126 binary traits, 725 metabolites, 3,371 proteins, 3,143 brain imaging phenotypes, and 3,217 other continuous phenotypes. In addition to the complete GWAS summary data, it also contains independent top hits for every dataset, totaling 116,918 independent signals in which 7,109 datasets have at least one hit. GeneATLAS [ 54 ] and GBE [ 55 ] contain associations from the UK Biobank cohort. GeneATLAS currently contains data for 452,264 individuals, 778 traits and 30 million variants, whereas GBE contains summary statistics from over 750,000 individuals combining data from the UK Biobank, the Million Veterans Program and the Biobank Japan. GTEx [ 56 ] and QTLbase [ 57 ] are the primary resources for xQTL data. The GTEx project has been expanded over time, and currently contains data of genetic associations for gene expression and splicing in 838 individuals in 49 tissues. QTLbase, similarly, contains genome-wide QTL summary statistics for many molecular traits across 95 tissue/cell types and multiple conditions. Contains tens of millions of significant genotype-molecular trait associations under different conditions. Other resources of this category, related to various large consortia (GIANT, WTCC, PGC etc.) as well as other biobanks (FinnGen etc.) can be found in Supplementary Table 2.
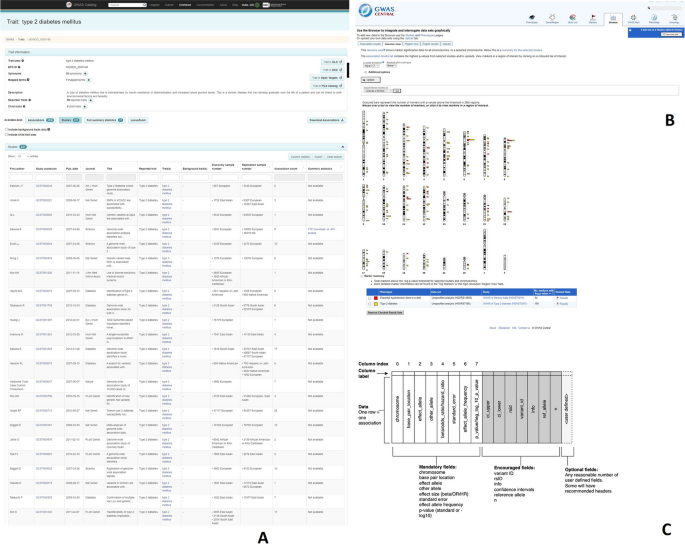
A snapshot of the data. A A view of the Type 2 Diabetes Mellitus studies deposited in NHGRI-EBI GWAS Catalog. B Type 2 Diabetes Mellitus studies contained in GWAS Central, depicting the significant hits in the chromosomes. C The SFF format
The second category contains databases of important secondary analyses performed on GWAS summary statistics with some of the methods that we describe in detail in later sections, such as gene-based tests, heritability analysis, TWAS, colocalization and so on. TSEA-DB [ 58 ] and PCGA [ 59 ] use information from gene-expression in various tissues to perform tissue or cell-type enrichment analysis of the GWAS association statistics. webTWAS [ 60 ] and COLOCdb [ 61 ] also use information on eQTL but in different fashion. webTWAS currently contains data for over 1,389 full GWAS for which it calculates the causal genes using single tissue expression imputation (using MetaXcan and FUSION), or cross-tissue expression imputation (using UTMOST). COLOCdb on the other hand is the most comprehensive colocalization analysis by integrating publicly available GWASs with different types of xQTL and different algorithms (COLOC, SMR). GWAS ATLAS [ 62 ] contains results of 4,756 GWAS from 473 unique studies across 3,302 unique traits accompanied by useful information obtained from downstream analysis. Each study is accompanied by MAGMA results (see also “gene-based tests”), SNP heritability estimation and genetic correlations with other traits in the database. GWASROCS [ 63 ], on the other hand, contains a large and comprehensive set of SNP-derived AUROCs and heritabilities. Currently includes 579 simulated populations (corresponding to 219 traits) and SNP data (odds ratio, risk allele frequency, and p-values) for 2,886 unique SNPs. Phenome-wide association studies (PheWAS) invert the idea of a GWAS by searching for phenotypes associated with specific variants across the range of thousands of human phenotypes, or the “phenome [ 64 , 65 , 66 ]. Thus, it is expected that a PheWAS will need large databases of GWAS results. PhenoScanner [ 67 ] is the most complete such database with publicly available results from over 65 billion associations and more than 150 million unique genetic variants. Similar functionalities are offered also by OpenGWAS, GWAS ATLAS and PheWAS Catalog [ 68 ]. Lastly, we need to mention LD Hub [ 69 ], a centralized database of publicly available GWAS results for 173 diseases/traits which offers a web interface that automates the LD score regression (LDSC) analysis pipeline (see also “Genetic correlation”).
Imputation and genotype reconstruction
Although some of the methods for quality control mentioned previously can correct errors and alter the data, the methods used for imputation go one step further. As expected, imputation methods were developed initially for individual data for handling studies genotyped with different platforms [ 70 , 71 , 72 ]. Such methods can infer missing genotypes using LD information from reference samples genotyped using denser arrays or sequencing. Genotype imputation increases the coverage of SNPs and thus can be used to increase statistical power, increase the accuracy of fine-mapping and harmonize the data in order to facilitate meta-analysis [ 70 ]. Several factors can influence the imputation accuracy: the sample size, the suitability of the reference panel for the particular sample, the genotyping chip and the allele frequency [ 71 ]. In general, however, these methods are time-consuming since they process individuals one at a time, and thus methods that impute directly the summary statistics were developed. These methods utilize only the information provided in the sample regarding the studied population (p-value, z-score or odds-ratio/beta) and require additional information regarding the LD structure. Nearly all methods perform a kind of multiple regression assuming the multivariate normal distribution for the test statistics and utilizing the theoretical result pointing that the correlation of such test statistics equals the correlation of the corresponding variables [ 73 ], that is the genotype correlation, available through the reference panel. Such methods include FAPI [ 74 ], ImpG [ 75 ], RAISS [ 76 ], DIST [ 77 ] and SSimp [ 78 ] with most of the differences lying in the choice of the reference panel and the exact details of the mathematical methods used to handle matrix inversions in the multivariate normal. DISSCO [ 79 ] uses a similar framework but allows for covariates. Such methods may perform poorly in cases where the sample has a different LD structure compared to the reference panel. Thus, extensions such as DISTMIX [ 80 ] and ARDISS [ 81 ] were developed to handle mixed ethnicity cohorts, improving the imputation performance. Adapt-Mix [ 82 ] estimates the correlation structure in both admixed and non-admixed individuals using simulated and real data and allows the use of this matrix with other imputation methods. Other methods such LS-meta [ 83 ] and LSimputing [ 84 ] offer additional advantages; LS-meta imputes both genetic and environmental components using information from additional omics-trait association summary data, whereas LSimputing implements a non-parametric method that allows for nonlinear SNP-trait associations and predictions in case a sample of IPD is available. Using the same principles, simGWAS [ 84 ] allows simulation of whole GWAS summary data, without generating individual data as an intermediate step.
Genotype reconstruction methods take a different approach. Given the summary statistics for a SNP (either directly measured or imputed), one can reconstruct the genotype counts that produced it. This will offer many advantages, since with the reconstructed genotypes the researchers could perform additional analyses using other statistical methods suitable for grouped data and test different hypotheses [ 85 ]. For instance, one can calculate grouped Polygenic Risk Scores (PRS) [ 85 ], perform logistic regression for grouped data [ 85 , 86 ], perform multivariate meta-analysis [ 87 ], or implement robust tests for association that is expected to work better when the underlying model of inheritance deviates from the additive which is usually assumed [ 88 , 89 ]. The details and the success of the reconstruction depend heavily on available summary statistics. As one can easily understand, p-values and z-scores cannot be used, and one must rely on available effect sizes such as the odds ratio (OR). When the OR, the standard error and the sample size is given, methods are available in epidemiology that allow the reconstruction of the allelic 2X2 table [ 90 ]. If z-scores, confidence intervals or p-values are available one can use them to obtain the standard error. React [ 85 ] uses an equivalent method relying on solving a system of nonlinear equations. If the allele frequency in one group (usually the controls) is also known, the allelic counts may easily be obtained with a simple calculation. In all cases the accuracy of the reconstruction may depend on the precision of the available summary statistics. After the allelic 2X2 table is reconstructed, it is straightforward to obtain the genotype counts, assuming HWE (which as one might expect adds another source of potential bias). MetaSustract [ 91 ] is a tool that recreates analytically the results of the validation cohort from meta-analysis summary statistics, allowing the researchers to compute meta-analysis summary statistics that are independent of the validation cohort, without requiring access to the IPD. Spkmt [ 92 ] works in similar fashion but in families; it can be used to derive the summary statistics of one parent from the data of the offspring and the other parent. Finally, we need to mention two tools that work in somewhat different modes. OATH [ 93 ] is used to reproduce reported results from a GWAS and recover underreported results from other alternative models with a different combination of nuisance parameters, whereas LMOR [ 94 ] performs transformations from the genetic effects estimated under the Linear Mixed Model to the Odds Ratio that only rely on summary statistics.
Analysis of a single trait
In this section we are going to present the various types of methods and tools dedicated to the analysis of a single trait. These include tools for meta-analysis , tools for the estimation of heritability , tools for implementing gene-based tests , gene set methods and fine mapping methods.
Meta -analysis
One of the most obvious uses of GWAS summary data is to combine them and perform a meta-analysis. Meta-analysis is the statistical procedure used to combine evidence from multiple studies in order to increase statistical power and it is a methodology widely used in medical research for decades [ 95 ]. A meta-analysis can be performed with various methods [ 16 ] using IPD or summary data; the former offers many advantages, but the latter is far more easy to be performed taking into account the various restrictions imposed on sharing GWAS IPD and the difficulties in the logistics of such a project [ 17 ]. Moreover, given the large samples usually encountered in GWAS it has been shown, both theoretically and empirically, that meta-analysis using summary statistics has the same efficiency as the joint analysis of IPD [ 96 ]. A compromise between these two extremes arises when a research group has access to individual-level genotype data of a limited sample size and wants to integrate these with existing summary data available in the databases. Such methods are in use in epidemiology for years [ 97 ] and several tools have been developed especially for handling GWAS data, for instance IGESS [ 98 ], metaGIM [ 99 ] and LEP [ 100 ]. PolyGIM [ 101 ] can be applied with or without IPD and uses polytomous logistic regression to investigate disease subtype heterogeneity in situations when only summary data is available.
Regarding summary-data meta-analysis of GWAS, the most commonly used methods includes standard methods, such as combining p-values, z-statistics or effects sizes like Odds Ratio (for binary traits) or mean differences (for continuous traits) using fixed or random effects models [ 16 , 102 ]. These statistical methods are straightforward to implement, and are available in general purpose statistical packages such as STATA and R. However, there are several specialized tools that facilitate the process and provide integration with useful bioinformatics or visualization functions. Such widely used tools include METAL [ 103 ], GWAMA [ 104 ] and PLINK [ 105 ]. Other tools are oriented to more specialized cases offering advanced options. For instance, YAMAS performs meta-analysis including missing SNPs identified with LD without performing imputation [ 106 ] and rareMETALS [ 107 ] uses a partial correlation based score to perform meta-analysis in the presence of large amounts of missing values. There is also a class of tools which focus on the replication of GWAS and the combined analysis of data from primary and replication studies. Such tools include rfdr [ 108 ] and Jlfdr [ 109 ] which control for False Discovery Rate (FDR), Rrate [ 110 ], which determines the sample size of the replication study and checks the consistency between the primary and the replication study, and MAJAR [ 111 ] which jointly test prognostic and predictive effects in meta-analysis without the need of using an independent cohort. metaGAP [ 112 ] is an online tool for calculating the statistical power of a meta-analysis of GWAS (Fig. 6 ). METACARPA works with overlapping or related samples, even when details of the overlap or relatedness are unknown [ 113 ], MAGENTA [ 114 ] performs meta-analysis with gene set enrichment analysis (GSEA), whereas GWASmeta [ 115 ] and MetABF [ 116 ] work in a bayesian framework calculating the Approximate Bayes Factor (ABF). Other tools offer more advanced options such as meta-analysis with multiple traits (see also “multiple traits”), like nGWAMA [ 117 ], metaCCA [ 118 ], CPASSOC [ 119 ], metaUSAT [ 120 ] and CPBayes [ 114 ] (and its extension GCPBayes [ 121 ]), and others are designed for meta-analysis under different genetic models, like GWAR [ 89 ] which uses robust methods (like MIN2 or MAX) in order to handle the uncertainty in the underlying genetic model, or like the simulation tool [ 122 ] which implements an alternate strategy for the additive genetic model simulating data for the individual studies. Finally, we need to mention sPLINK [ 123 ] which performs privacy-aware GWAS on distributed datasets, and XPEB [ 124 ] which is an empirical Bayes approach designed to improve the power GWAS in minority populations by exploiting information from GWASs performed in populations of different origin.
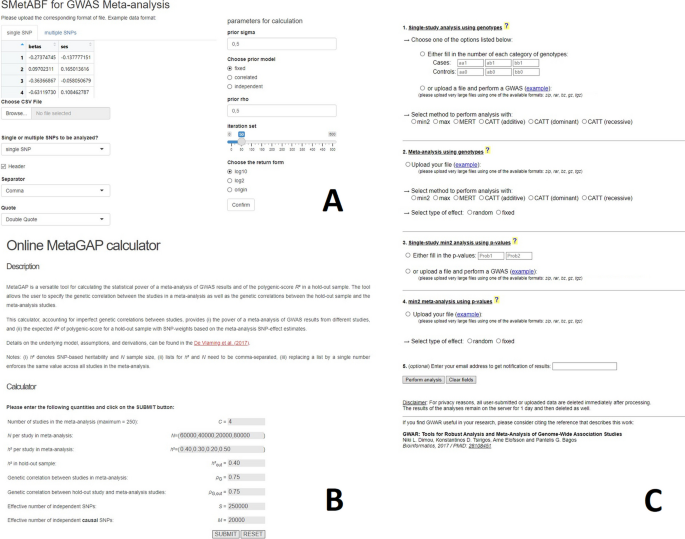
Tools for meta-analysis. A GWASmeta (SMetABF) for performing Bayesian meta-analysis. B The MetaGAP power calculator. C GWAR for robust analysis and meta-analysis of GWAS
Inferring heritability
Heritability is generally defined as the fraction of phenotypic variation explained by genetic variation. Heritability is a dimensionless parameter of the population, and it was introduced by Sewall Wright and Ronald Fisher in the previous century. Traditionally, heritability is estimated using family-based designs such as twin studies. However, there are controversies regarding the various methodologies for estimation and interpretation of the results [ 125 ]. Despite all these, heritability is an important aspect of research in modern genetics, and regarding the prediction of disease risk from genomic data [ 126 ]. The technological advancements have facilitated the development of methods that use large samples of unrelated, or related, individuals. Thus, family-based designs using genomic data (trio-genome-wide complex trait analysis, and so on) have emerged. Such methods are discussed and compared in [ 127 ]. Of course, heritability can also be estimated via the results obtained in a traditional GWAS using unrelated individuals. The gap between these estimates and those obtained from classical heritability estimation methods has been termed the "missing heritability problem" and it is an important open question in current research [ 128 ]. Recent reviews of the methods that use GWAS data, are given in [ 18 , 19 ] focusing on their modeling assumptions, their similarities, and their applicability.
One of the first and simplest methods to calculate heritability from allele frequency, odds ratio and prevalence of the disease was implemented in the SumVg package [ 129 ]. This method, however, utilizes only the significant SNPs. The same authors extended the method later in order to allow calculation using the z-statistics from the whole GWAS sample [ 130 ]. A disadvantage of this method is that LD is not taken care of, and highly correlated SNPs need to be filtered manually. AVENGEME [ 131 ] is a tool that treats causal effect sizes as fixed effects and models the genotypes as random correlated variables. HESS [ 132 ] which was presented later built upon the same ideas and can be viewed as a weighted sum of the squares of the projection of effect sizes onto the eigenvectors of the LD matrix at the particular locus, with weights inversely proportional to the corresponding eigenvalues. LD Score Regression (LDSC) has been frequently applied to summary statistics from GWAS and one of its functionalities is to estimate the SNP heritability of a trait [ 133 ]. LDER [ 134 ] extends LDSC making full use of the information from the LD matrix providing more accurate estimates, whereas s-LDSC [ 135 ] is an extension suitable for partitioning heritability. SumHer [ 136 ] presented later and offers the same functionalities, with the main difference being that it allows for different so called “heritability models”. According to these, a SNP with high MAF is expected to contribute more to the total heritability compared to one with low MAF, whereas on the other hand, a SNP in a region of low LD is expected to contribute more compared to one in a region of high LD. On the contrary, LDSC estimates are obtained by assuming that all SNPs contribute equally. HEELS [ 137 ] is a new tool using REML to produce accurate and precise local heritability estimates and RSS, is a multiple regression-based fine-mapping tool (see “Fine-mapping”), can also calculate SNP heritability from the regression model. VarExp [ 138 ] and GxESum [ 139 ] are methods for estimating the phenotypic variance explained by genome-wide gene-environment (GxE) interactions. There are also tools like GWIZ [ 63 ] and SummaryAUC [ 140 ] that calculate the Receivers Operator’s Characteristic (ROC) curve and the associated Area Under the Curve (AUC). GWIZ generates ROC curves and the AUC using simulations and then estimates heritability using the square of the Somers’ rank correlation D. SummaryAUC on the other hand approximates the AUC of a PRS and its variance. HAMSTA [ 141 ] is a tool that, among others, estimates heritability explained by local ancestry using data from admixture mapping studies. Estimating the Effect size distribution is also a related important concept. GENESIS [ 142 ] uses LD and a Likelihood-based approach to estimate effect-size distributions. It also allows predictions regarding yield of future GWAS with larger sample sizes. GWEHS [ 143 ] calculates the distribution of effect sizes of SNPs, as well as their contribution to trait heritability. Furthermore, it performs predictions for the change in the effect size as well as in the heritability when new variants are identified. FMR [ 144 ] is a method-of-moments for calculating the effect-size distribution and GWAS-Causal-Effects-Model [ 145 ] is a random effects model for estimating the causal variants and their effect size distribution. Finally, there are tools to implicate gene-expression in heritability analysis: MESC [ 146 ] which estimates the proportion of heritability mediated by gene expression levels using linkage disequilibrium (LD) scores and eQTL, and GCSC [ 147 ] which uses results from a TWAS (see “TWAS and Colocalization”) in the so-called gene co-regulation score regression, to identify gene sets enriched for disease heritability.
Gene-based tests
Historically, association tests are oriented towards single variants, and this was the case for both traditional association studies as well as for GWAS. However this approach has some limitations that were noted earlier and a call for a shift towards gene-based tests was made [ 148 ]. Gene-based tests aggregate individual variant associations within a gene, providing a more comprehensive assessment of the gene's overall contribution to a trait or disease. This approach helps prioritize genes with multiple associated variants, enhancing the biological relevance of findings, and it has proven to be useful particularly in case of low frequency variants [ 148 ]. There are plenty of different methods for combining the association statistics or p-values within a gene, ranging from simple Fisher’s method or the minimum p-value approach, to more advanced methods like the Burden Test (BT) [ 149 ] or quadratic tests like SKAT [ 150 ] with variations in power [ 151 ]. Nevertheless, there is a consensus regarding the importance of incorporating LD information of the nearby variants into the methods for controlling the type I error rate at the desired level [ 20 ].
VEGAS, GATES, fastBAT and GCTA are among the oldest tools available for summary data, which remain efficient and widely used. SKAT (Sequence Kernel Association Test) is a well-known regression method for testing association between variants and traits adjusting for covariates. As a score-based variance-component test, it calculates p-values analytically by fitting the null model containing only the covariates [ 150 ]. The original SKAT method uses only IPD, but later implementations like metaSKAT or SKAT-O have been extended to handle summary data. GCTA and VEGAS also use the multivariate normal framework adjusting the estimates for LD using a reference panel [ 152 , 153 ]. Of note, GCTA also offers methods for conditional analysis (see “Fine mapping”), and same also holds for KGG [ 154 ], whereas VEGAS’s new version allows for mixed ethnicity populations. GATES [ 155 ], on the other hand, uses an extended Simes procedure that integrates functional information and association evidence to combine p-values, whereas fastBAT [ 156 ] offers fast analytical p-value computations. The gene analysis in MAGMA (Multi-marker Analysis of GenoMic Annotation) is based on a multiple linear principal components’ regression model to account for LD and uses an F-test to compute the overall gene p-value [ 157 ]. Its extension, nMAGMA, extends the lists of genes that can be annotated by integrating local signals, long-range regulation signals, and tissue-specific gene networks. It also provides tissue-specific risk signals, which are useful for understanding disorders with multi-tissue origins [ 158 ]. H-MAGMA [ 159 ] and eMAGMA [ 160 ] are two other extensions. The former integrates 3D chromatin configuration, whereas the latter leverages significant tissue-specific cis-eQTL information to assign SNPs to putative genes. EPIC [ 161 ] and GAMBIT [ 162 ] also utilize functional data for gene-based analysis; the former using cell-type-specific gene expression data obtained from single-cell RNA sequencing and the latter using coding and tissue-specific regulatory annotations. Such methods share several features in common with TWAS methods (see respective section). AgglomerativLD [ 163 ] also captures LD between SNPs of nearby genes, which induces correlation of the gene-based test statistics. DOT [ 164 ] is one of the few methods that applies a decorrelation-based approach before combining SNP-level statistics or p-values. Tools like GPA [ 165 ], oTFisher [ 166 ], TS [ 167 ] and aSPU [ 168 ] implement some type of so-called adaptive tests (AT), that is, they account for possibly varying association patterns across SNPs, whereas some modern tools like MKATR [ 169 ], COMBAT [ 170 ], MCA [ 171 ], OWC [ 172 ], FST [ 173 ], ACAT [ 174 ], HYST [ 175 ], GBJ [ 176 ] and sumFREGAT [ 177 ] perform analysis with multiple statistical methods and test and combine the results. Notably, tools like aSPU [ 168 ], snpGeneSets [ 178 ], Pascal/PascalX [ 179 , 180 ], MAGMA, chromMAGMA [ 181 ] and FUMA [ 182 ], also offer the option of performing gene-set analysis after performing the gene-based analysis (see next section), whereas HSVS-M [ 168 , 183 ] tests the association of a gene with multiple correlated traits.
Gene Set analysis
Gene set analysis (GSA), or Pathway Analysis, extends the concept of gene-based methods by jointly analyzing groups of functionally related genes and identifying biological pathways enriched with trait-associated genes. By considering the collective impact of multiple genes within a pathway, researchers can obtain a clearer picture of the underlying biological mechanisms influencing the phenotype under investigation. The first applications of such methods borrowed ideas from the microarray data analysis literature, and since then they became widespread in analysis of GWAS [ 184 ]. Any GSA method needs to address some issues. Firstly, how to handle SNPs of the same gene; secondly, how to define the appropriate gene-set or pathway, and finally how to combine the effects from multiple SNPs/genes within the same set/pathway [ 185 ]. Thus, the choices made by different methods can be very diverse leading to a wide variety of different approaches. For instance, some methods operate with SNP-level statistics (effect sizes, z, or p-values) assigning the SNP to the closest gene (usually within a range of ± 20 K bases), whereas others take as input a gene-level statistic or simply a gene list obtained by a gene-based method (of course, several tools allow for both a gene-based and a GSA approach). Regarding the choice of set there is a plethora of databases containing biological pathways (KEGG, PANTHER etc.), or other types of gene-set representation like PPI interactions, ontologies and so on [ 186 ]. Finally, regarding the statistical method used to aggregate evidence there is also a wide range of different methods that handle with different approaches the gene set size and gene length, the LD patterns and the presence of overlapping genes within pathways, or apply different statistical approaches such as those using the so-called competitive null hypothesis, or those using the self-containing one [ 14 , 187 ]. A tutorial regarding the use of such methods is given in [ 21 ].
Among the most easily used and frequently cited are the tools that utilize a webserver. FUMA [ 182 ] and iGSE4GWAS [ 188 ] are tools specialized in GWAS and use SNP-level statistics as inputs, differing in the subsequent analyses: FUMA uses MAGMA for gene-based testing and allows for ORA and Kologorov-Smirnov test (GSEA), whereas iGSE4GWAS maps the most significant SNP to a gene and then performs an improved GSEA with label permutation to obtain accurate p-values. Tools like Enrichr [ 189 ], g:Profiler [ 190 ], DAVID [ 191 ], WebGestalt [ 192 ] and PANTHER [ 193 ] are general purpose enrichment tools that provide functionalities for different types of omics data (Fig. 7 ). They accept gene or SNP-list as input and provide Application Programming Interface (API) ensuring interoperability, whereas for the statistical analysis they all use some version of ORA and/or GSEA (WebGestalt also uses Network Topology-based Analysis). A major feature of these tools is that they incorporate a large number of biological and pathway databases, with g:Profiler and Enrichr offering the most complete collection. GSA-SNP2 is one of the first methods to be developed for GWAS and has seen several improvements regarding the calculation of the combined gene score and the execution time, being among the fastest methods [ 194 ]. aSPUpath2 [ 195 ] and GIGSEA [ 196 ] are two methods that integrate expression data (eQTL) in the pathway analysis. The former uses an adaptive test that extends the aSPU methodology based on chi-square, whereas the latter uses a regression-based approach coupled with permutations to calculate accurate p-values. In a similar fashion, deTS [ 197 ] and PGCA perform tissue-specific enrichment analysis (TSEA) for detecting tissue-specific genes and for enrichment test of different forms of query data. Other methods use different definitions of the gene-sets, in some cases utilizing additional information. For instance, dmGWAS [ 198 ] integrates PPI networks and uses a search method to identify subnetworks. Compared with standard pathway methods it offers to the users the flexibility in the definition of a gene set and can utilize local PPI information. GEMB [ 199 ] defines the gene-sets using gene weights from model predictions and gene ranks from GWAS, and GENOMICper [ 200 ] uses permutations of the identified SNPs by rotation with respect to the genomic locations. GWAB [ 201 ] uses network connections to reprioritize candidate genes by integrating the GWAS and network data, whereas GenToS [ 202 ] searches for trait-associated variants in existing human GWAS. We also need to mention PAPA [ 203 ] which is a flexible tool for pleiotropic pathway analysis. As we already mentioned, aSPU, snpGeneSets, PascalX/PASCAL and MAGMA/chromMAGMA are gene-based methods that also perform GSA, whereas MAGENTA is a tool that performs meta-analysis and subsequently GSA (see “meta-analysis”). Lastly, we need to mention Inferno [ 204 ] and Mergeomics [ 205 ] which are webservers offering a variety of options, extending typical GSA applications. Inferno integrates a variety of functional genomics sources to identify causal noncoding variants using COLOC, WebGestalt, LDSC and MetaXcan. Mergeomics uses summary statistics of multi-omics association studies (GWAS, EWAS, TWAS, PWAS, etc.) and performs correction for LD, GSEA, meta-analysis and identification of regulators of disease-associated pathways and networks.
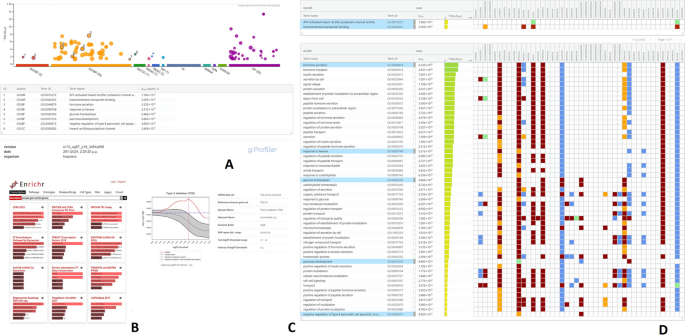
Enrichment. A Summary view in g:Profiler of the significant SNPs for Type 2 Diabetes Mellitus. B Enrichr results for the same set. C Output of GWAB for Type 2 Diabetes Mellitus SNPs. D Detailed results from g:Profiler
Fine-mapping
While GWAS can identify broad genomic regions associated with the trait, it doesn't pinpoint the exact causal variant within those regions. Fine mapping, working in the opposite direction of that of the gene-based approaches, is a process aimed at narrowing down and identifying causal variants, that is the specific genetic variants responsible for the observed associations between genomic regions and traits of interest. The plethora of statistical methods and study designs makes it difficult to choose an optimal approach. The different approaches that have been proposed to perform fine-mapping can be divided in three broad categories: heuristic methods that select SNPs based on LD patterns, conditional or penalized regression models that perform variable selection, and Bayesian methods that calculate posterior probabilities or Bayes Factors. Based on theoretical and empirical evidence it seems that Bayesian methods have superior performance [ 22 ]. Several factors may influence the performance of fine-mapping approaches, including the true number of causal SNPs in a region and their effect sizes, the local LD structure, the sample size, and the SNP density [ 22 , 206 ]. Functional annotations are also of great importance leading to the so-called functionally informed fine-mapping (FIFM) methods [ 206 ]. The hypothesis of a single causal variant is also very restrictive, and several methods have been developed to allow multiple causal variants in a region as well as to incorporate additional layers of functional annotations, like eQTL [ 207 ]. Moreover, methods for fine-mapping of multiple datasets have been proposed, either exploiting different LD patterns across ethnic groups or borrowing information between different traits [ 207 ].
As we already noted Bayesian methods seem to have superior performance [ 22 ] and thus it is of no surprise that most of the currently available methods operate in a Bayesian framework calculating Posterior Inclusion Probabilities (PIP) and/or Bayes Factors (BFs) in various settings: PAINTOR [ 208 ], DAP [ 209 ], fgwas [ 210 ], FINEMAP [ 211 ], flashfm [ 212 ], FINMOM [ 213 ], CARMA [ 214 ] and CAVIAR/CAVIARBF [ 215 ]. MsCAVIAR [ 216 ] is an extension of the latter method leveraging information from multiple studies, useful in trans-ethnic fine mapping. Similarly, XMAP [ 217 ] performs cross-population fine-mapping by leveraging genetic diversity and accounting for confounding bias. BEATRICE [ 218 ] is a unique method that combines a hierarchical Bayesian model with a deep learning-based inference procedure, whereas RIVIERA-beta [ 219 ] performs Bayesian fine-mapping using Epigenomic Reference Annotation. On a different level, PolyFun/PolyLoc [ 220 ] do not perform fine-mapping per se but are used for estimating the prior causal probabilities of SNPs, which can then be used by other Bayesian fine-mapping methods. SusieR [ 221 ], BVS-PICA [ 222 ] and JAM [ 223 ], operate also in a Bayesian regression framework performing variable selection and penalized regression. Other regression-based methods, like SOJO [ 224 ] and ANNORE [ 225 ] work in a frequentist framework and perform lasso-type and differential shrinkage via random effects, respectively, whereas GSR utilizes a gene score regression approach [ 226 ] and RSS performs multiple regression utilizing the so-called summary statistics likelihood [ 227 ]. AHIUT [ 228 ] performs an intersection–union test based on a joint/conditional regression model with all the SNPs in a region. Lastly, we need to mention PICS2 [ 229 ], which performs probabilistic identification of causal SNPs and is the only of the methods that is available as a web-server, and echocolatoR [ 230 ] which requires minimal input from users and integrates a suite of fine-mapping tools to identify consensus variants, test enrichment and visualize the results.
Analysis of multiple traits
In this section we analyze methods developed for handling multiple traits. Depending on the type of data and the purpose of the analysis the methods can be divided into pleiotropy methods, methods that calculate the genetic correlation , methods for mendelian randomization, transcriptome-wide association and colocalization methods.
Pleiotropy is the phenomenon in which a single variant influences several traits [ 231 ]. Such methods are of great importance in genetic research and several methods have been developed during the last years. A major goal of such methods is to increase the statistical power over single trait methods. Imagine for instance a variant that produces a near-significant effect when analyzed separately for two or three traits. A method that can combine these estimates may produce significant results. Another application of a joint analysis would be to identify variants that influence both traits, or variants that influence only one of them. When all the relevant variants are considered, one can also estimate the kind of relationship between the traits (see “genetic correlation”). A review of the statistical methods to detect pleiotropy in complex traits can be found in [ 25 ]. Usually, the methods that allow for multiple trait analysis are oriented toward quantitative traits like BMI, SBP, DBP and so on, that traditionally are measured on a single cohort, resulting in the existence of cross-trait correlation that needs to be taken into account in the analysis. However, there are also methods for performing the same analysis with summary estimates derived from different cohorts, as well as methods that allow for binary traits with the case–control design, using overlapped or non-overlapped controls.
All methods base their inference on the assumption that the z-statistics follow a multivariate normal distribution (MVN) and perform different types of tests and/or different procedures to estimate or approximate the correlation structure. ACA [ 232 ] one of the first methods, estimates the traits covariance from a subset of the phenotypic data or from published studies, p_ACT [ 233 ] integrates the MVN using the trait correlation, PAT [ 234 ] uses a likelihood-ratio test, and PLEI [ 235 ] uses the union-intersection testing method, but in addition to the likelihood ratio test, it also applies generalized estimating equations under the working independence model; it can be applied for both marginal analysis and conditional analysis. USAT [ 236 ] uses a score-based test, JaSPU [ 237 ] uses an adaptive test which is robust to violations of the MVN assumptions and MTAR [ 238 ] uses a Principal Components (PC)-based test. BMASS [ 239 ] on the other hand is a Bayesian multivariate method, whereas TWT [ 240 ], MTAFS [ 241 ] and EBMMT [ 242 ], which are among the newer tools, perform a Cauchy Combined Test (CCT) to handle the correlation structure and obtain accurate p-values. SHAHER [ 243 ] uses a linear combination of traits by maximizing the proportion of its genetic variance explained by the shared variants and allows both shared and unshared variants to be effectively analyzed and HIPO [ 244 ] performs heritability-informed power optimization for conducting multi-trait association analysis. HOPS [ 245 ] computes a horizontal pleiotropy score by removing correlations between traits caused by vertical pleiotropy and normalizing effect sizes across all traits and PDR [ 246 ] performs a pleiotropic decomposition regression to identify shared components and their underlying genetic variants. We also need to mention methods like MTAG [ 247 ] and PLEIO [ 248 ] which use LDSC and apart from sample overlap also allow data from multiple studies, something that can be considered meta-analysis and methods like MSKAT [ 249 ], multiSKAT [ 250 ], MGAS [ 251 ], MAIUP [ 252 ] and MTAR (multi-trait analysis of rare variants) [ 253 ] which are gene-based methods specialized for multiple traits. Finally, methods like iMAP [ 254 ] and graphGPA2 [ 255 ] use graphical models and are capable of performing analysis of large number of traits.
On the other hand, there are several methods that assume independence of the studied samples. Most of them are designed for larger analyses of many traits from multiple studies, for instance PolarMorphism [ 256 ], JASS [ 257 ], gwas-pw [ 258 ] and FactorGo [ 259 ], sumDAG [ 260 ], combGWAS [ 261 ] and GCPBayes pipeline [ 262 ]. GCPBayes_pipeline uses the functionality of GCPBayes to perform cross-phenotype gene-set analysis between two traits. gwas-pw is used for the joint analysis of two GWAS in order to identify variants influencing both traits. PolarMorphism is based on a transform from Cartesian to polar coordinates and reports a per variant degree of 'sharedness' across traits, whereas FactorGo provides scalable variational factor analysis model that is computationally efficient for large number of traits. JASS provides interactive exploration and visualization of the results of comparison of many traits through a web interface (Fig. 8 A-C), sumDAG goes one step further and constructs phenotype networks by using a Gaussian linear model and a directed acyclic graph, and combGWAS identifies susceptibility variants for comorbid disorders and calculate genetic correlations. EPS [ 263 ] and GPA [ 264 ] differ in integrating Pleiotropy and functional annotation from eQTL.
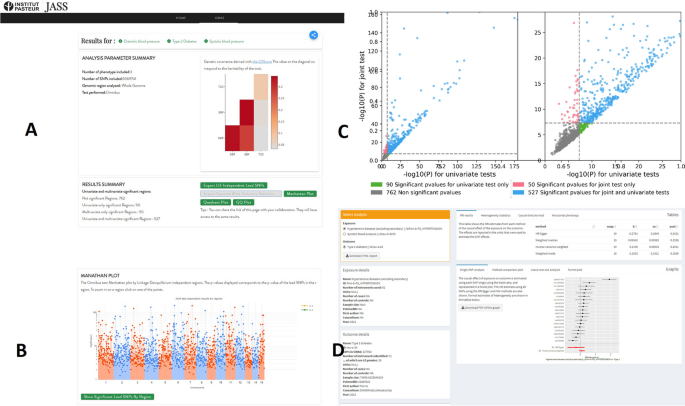
Analysis of multiple traits. A JASS analysis for Type 2 Diabetes Mellitus (T2DM), Systolic Blood Pressure (SBP) and Diastolic Blood Pressure (SBP), indicating the pairwise genetic correlations between the traits. B Manhattan Plot from JASS for the combined analysis of the three traits. C Pairwise analysis of the SNPs identified as significant in the univariate analysis and in the combined analysis. D Two-sample Mendelian Randomization analysis for the association of SBP and T2DM obtained by MR-BASE
Genetic correlation
Genetic correlation is related to pleiotropy and describes the relationship between two traits, that is, the extent to which the genetic variants influencing one trait overlap with the genetic variants associated with the other. It thus can quantify the overall genetic similarity and provide insights into the polygenic genetic architecture of complex traits [ 23 ]. As we already saw, analyzing simultaneously multiple traits may increase power in case of horizontal pleiotropy; an additional potential application is to use the estimated correlation in order to establish causality between traits in case of vertical pleiotropy (see also next sections). Since heritability is the proportion of the phenotypic variance explained by genotypic variation it is of no surprise that genetic correlation (or, the genetic covariance) is related to the traits’ heritabilities. Thus, several of the methods for estimating heritability discussed earlier, like HESS and SumHer can also calculate the correlation between traits. The most commonly used method, however, for calculating genetic correlation is LDSC (LD Score Regression). The method originally developed for distinguishing polygenicity from bias by examining the relationship between test statistics and LD score, but it is also used for estimating heritability and genetic correlation [ 133 ]. LDSC is also available through the LD Hub server. PCGC-s [ 265 ] is an adaptation of stratified LDSC for case–control studies and can also estimate genetic heritability, genetic correlation, and functional enrichment. Another popular tool is GNOVA [ 266 ] which calculates annotation-stratified covariance using the method of moments and allows for sample overlap. Its extension, SUPERGNOVA [ 267 ] identifies global and local genetic correlations that could provide new insights into the shared genetic basis of many phenotypes. Local correlations, among others, can be also computed using LAVA [ 268 ]. HDL [ 269 ] is a likelihood-based method which produces more precise estimates. A recent comparison found that LDSC and GNOVA are more similar and robust to LD and sample overlap compared to HDL. HDL provides biased estimates of the genetic covariance in most cases and could not distinguish genetic from non-genetic correlation. Moreover, HDL restricts the users to using the built-in reference panel, and its performs poorly when the number of shared SNPs between reference panel and GWAS is small [ 24 ]. Other tools provide somewhat different types of analyses. For instance Popcorn [ 270 ] estimates transethnic genetic correlation, GECKO [ 271 ] estimates both genetic and environmental covariances, PhenoSD [ 272 ] uses LDSC for estimating phenotypic correlations and then performs correction for multiple testing using the spectral decomposition of matrices, whereas LPM [ 273 ] is a latent probit model scalable to hundreds of annotations and phenotypes that integrates functional annotations. ccGWAS [ 274 ] is a tool for comparing two different disorders with small genetic correlation providing a case-case association test, and RHOGE [ 275 ] estimates the genetic correlation between two traits as a function of predicted gene expression effect. LOGOdetect [ 276 ] uses scan statistics with an LD score-weighted inner product of local z-scores to identify small segments that harbor local genetic correlation between two traits. DONUTS [ 277 ] is a unique method since it operates on summary statistics from families.
Mendelian randomization
Mendelian Randomization (MR) is a method suggested in the pre-GWAS era to investigate causal relationships between two traits, usually a phenotype and a disease [ 278 ] using genotype–trait associations to make inferences about environmentally modifiable causes of the traits. In technical terms, MR uses genetic variants as instrumental variables [ 279 ] to mimic the random assignment of exposures in a randomized controlled trial, similar to the way Mendel's laws of inheritance dictate the random assortment of alleles during gamete formation. By utilizing the natural randomization of genetic inheritance, MR aims to minimize biases introduced by confounding factors that usually affect observational studies when investigating the association of two traits. Usually, we are interested in a disease and some other intermediate phenotype, or another disease. For instance, the MR approach may involve the relationship between hypertension and BMI, or between hypertension and diabetes. Traditionally MR was performed with one sample (1SMR) using a single variant (usually referred to IPD methods), and subsequently multivariate methods for MR meta-analysis were developed [ 280 ]. With the emergence of GWAS these methods evolved to the most commonly used two-sample MR (2SMR) methods that utilize summary data estimates from several variants regarding the genotype–phenotype and genotype-disease association from different samples [ 26 , 281 ]. To establish connection with the previous sections, MR seeks to analyze correlated traits [ 282 ] and to provide evidence for causation, in other words to distinguish vertical from horizontal pleiotropy.
Several standard methods for MR in GWAS with summary data have been made available during the last years: the inverse-variance weighted method (IVW), the various types of median estimators (simple or weighted) and the MR-Egger regression approach. IVW gives consistent estimates only if all the genetic variants in the analysis are valid instruments. The median estimator is consistent even when up to 50% of the information comes from invalid instrumental variables, whereas MR-Egger performs equally well but provides somewhat less precise estimates [ 283 ]. These methods are readily available in standard packages like TwoSampleMR [ 284 ] and MR [ 285 ]. The functionalities of TwoSampleMR are also offered, at least partially, through the webserver of MRBASE [ 284 ], which is the only method available as such (see Fig. 8 , D). BWMR [ 286 ] is a tool that performs MR in a Bayesian framework. Besides the issue of weak instruments which is of importance, most modern methods also aim to perform the MR analysis accounting or correcting for horizontal pleiotropy. For instance, pIVW [ 287 ] is an extension of the IVW that accounts simultaneously for weak instruments and balanced horizontal pleiotropy and MRmix [ 288 ] uses a mixture approach allowing a fraction of the instruments to have pleiotropic effect on the outcome. Similarly, MRcML [ 289 ], MR-LDP [ 290 ], MR-Corr2 [ 291 ] and MR-PRESSO [ 292 ] provide functionalities to account for horizontal pleiotropy, whereas IMRP [ 293 ] takes a different approach and searches iteratively for horizontally pleiotropic variants and causal effects. MR-APSS [ 294 ] differs in that it performs MR accounting for both pleiotropy and sample structure which seems to be another important confounder (and includes population stratification, cryptic relatedness, and sample overlap); MRlap [ 295 ] considers both weak instrument bias and winner's curse, accounting for sample overlap. MR.CUE [ 296 ] and TS_LMM [ 297 ] offer additional functionality for handling variability of the estimates. LCV [ 298 ] is a method that estimates causal associations between traits avoiding confounding by genetic correlation, whereas OMR [ 299 ] uses information from all GWAS SNPs for causal inference and JAM-MR [ 300 ] performs variable selection and causal effect estimation in MR. CS [ 301 ], BiDirectCausal [ 302 ], MRCI [ 303 ] and LHC-MR [ 304 ] constitute another important class of methods since they can identify bidirectional causal effects. Another important extension is offered by methods like MR2 [ 305 ], MV-MR [ 306 ], MRBEE [ 307 ], MVMR-cML [ 308 ] and adOMICs [ 309 ] which extend the MR framework in the multivariate setting allowing more than one exposures or outcomes, as well as MR-BMA [ 310 ] which go one step further performing multivariate MR in a Bayesian framework. Finally, other methods like hJAM [ 311 ], MR.RAPS [ 312 ] and MRPEA [ 313 ] offer more advanced options. hJAM unifies the framework of MR and TWAS and can be applied to correlated instruments and multiple intermediates, MR.RAPS uses a three-sample genome-wide design with many independent genetic instruments across the genome to handle many weak genetic instruments and pleiotropy, whereas MRPEA uses pathway association MR analysis approach using data of environmental exposures.
Colocalization and TWAS
As we already described, the MR approach involves the combination of two types of data, a genotype-disease association, and a genotype–phenotype association. If the phenotype involves gene-expression, that is the result of an eQTL study, then we have two distinct but fundamentally related methods, the Transcriptome-wide association study (TWAS) and the colocalization approach (Fig. 9 ). TWAS is based on the idea that genetic variants can influence gene expression, which subsequently can affect complex traits or diseases [ 27 ]. Thus, the approach uses information from eQTL to identify associations between predicted gene expression levels and complex traits/diseases [ 314 ]. Even though there are several different methods, the resemblance to MR is obvious; in fact several methods like SMR that uses a single variant [ 315 ], GSMR that uses multiple variants [ 310 ], and PMR [ 316 ] which can account for correlated instruments, horizontal pleiotropy, and can accommodate both single traits and multiple correlated outcomes, all use the term MR, whereas the authors of TScML [ 317 ], which uses two-stage constrained maximum likelihood, which is an extension of 2SLS, explicitly state that can be used for both MR and TWAS analyses. FUSION and S-PrediXcan are the oldest and most widely known methods. FUSION is the current implementation of the first TWAS method [ 318 ], whereas S-PrediXcan [ 319 ] is the summary-data version of PrediXcan. Xu et al. [ 320 ] noted that PrediXcan and TWAS can be viewed as a special case of general association testing with multiple SNPs in a GLM and proposed the so-called sum of powered score (SPU) test implemented in aSPU-TWAS [ 320 ]. A subsequent evaluation has shown that the original TWAS statistic is equivalent to an LD-aware version of standard MR [ 321 ]. iFunMed [ 322 ] and sMIST [ 323 ] formulate the problem within the framework of mediator analysis, and similarly PTWAS [ 324 ] applies principles from instrumental variables analysis. Comm-S* [ 325 ] uses a variational Bayesian EM algorithm and a likelihood ratio test to assess expression-trait association. Its extension Tiss-Comm [ 326 ] leverages the co-regulation of genetic variations across different tissues explicitly via a unified probabilistic model and also detects the tissue-specific role of candidate target genes in complex traits. Similar multi-tissue approaches are followed by fQTL [ 327 ], sCCA [ 328 ] and UTMOST [ 329 ]. Primo [ 330 ], and OPERA [ 331 ] extend further the integration by allowing different types of xQTL data (eQTL, pQTL, mQTL etc.) to allow estimation under different conditions, whereas SUMMIT [ 332 ] uses a large eQTL summary-level dataset, penalized regression and Cauchy Combination Test and HMAT [ 333 ] aggregates TWAS association tests obtained across multiple gene expression prediction models using the harmonic mean P-value combination (HMP). BGW [ 334 ] and ARCHIE [ 335 ] are two methods that utilize trans-regulated eQTLs. Other tools use combination of methods, like TIGAR [ 336 ] which combines DPR and PrediXcan, whereas others, like JEPEGMIX2‐P [ 337 ] or FOCUS [ 338 ], perform TWAS using pathway information, or use LD to perform fine-mapping over the gene–trait association signals obtained from TWAS, respectively. Even though the various methods discussed here have different modeling assumptions and many were initially developed to answer different biological questions, a recent technical review of the TWAS methods showed that all can be viewed as versions of the two-sample MR analysis [ 339 ]. Indeed, several recent tools like MRLocus [ 340 ], TWMR [ 341 ], and Mr.MtRobin [ 342 ] make explicit use of the MR methodology and jargon in order to perform a sophisticated TWAS. MRLocus performs first a colocalization step to each nearly-LD-independent eQTL, and then performs an MR analysis step across eQTLs. TWMR performs a multi-gene multi-instrument MR approach to identify genes whose expression influence the phenotype. Finally, Mr.MtRobin uses multi-tissue eQTL and a reverse regression random slope mixed model to infer whether a gene is associated with a complex trait. As we have already noticed, webTWAS, apart from the database, also offers a webserver for accessing S-PrediXcan, SMR and UTMOST with user supplied datasets.
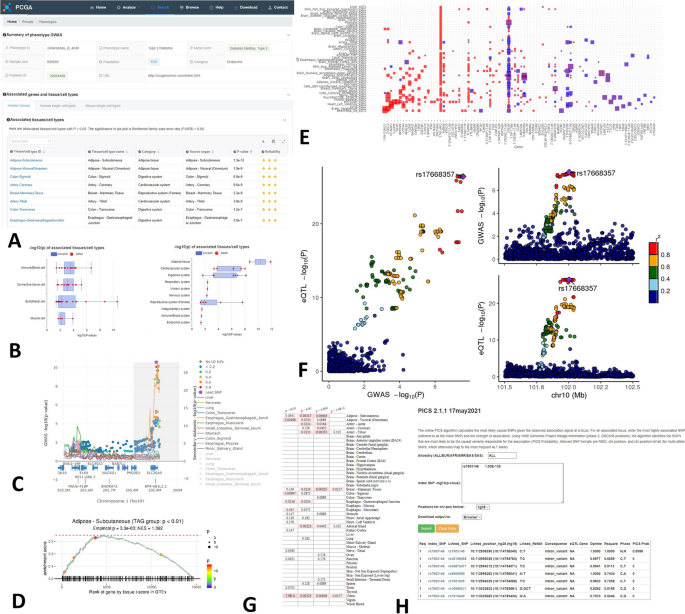
Incorporation of eQTL data. A Overview of the gene-expression patterns in T2DM obtained by PCGA. B Top associated tissues and cells for T2DM (PCGA). C An example of colocalization output perform by LocusFocus. D TSEA-DB view of the analysis of significant SNPs involved in T2DM. E Heat-map for the tissues involved in T2DM significant hits obtained by COLOC. F Plots of the genome-wide significant hits obtained from GWAS and eQTL (COLOC). G Heat-map for the tissues involved in T2DM (TSEA-DB). H Example of fine-mapping regarding a SNP indicated in T2DM obtained by PICS2
Another method that also uses GWAS results along with eQTL data is colocalization. Colocalization approaches are used to assess whether two different traits or diseases share a common causal genetic variant or set of variants at a specific genomic locus [ 13 ]. Colocalization analysis identifies genetic variants that show significant association in both GWAS and eQTL studies. However, unlike TWAS, it does not perform gene expression prediction and gene-trait association tests, but it focuses on the colocalized SNPs [ 28 ]. TWAS and colocalization are related approaches but not identical, since it has been shown that may give different results under different conditions (for instance in case of horizontal pleiotropy) and thus it has been suggested that they should be used complementary [ 28 , 343 ]. COLOC was one of the first methods for colocalization and has seen several improvements [ 344 , 345 ] (see also Fig. 9 ). The latest version uses SuSiE and allows evidence for association at multiple causal variants to be evaluated simultaneously, while at the same time separating the statistical support for each variant conditional on the causal signal being considered. MOLOC [ 346 ] is multiple-trait version of COLOC, operating in a Bayesian framework that integrates GWAS summary data with multiple xQTL data to identify regulatory effects, HyPrColoc [ 347 ] is a deterministic Bayesian method that detects colocalization across large numbers of traits, and SS2 [ 348 ] operates across any number of gene-tissue pairs allowing for sample overlap. LLR [ 349 ] works for colocalizing genetic risk variants in multiple GWAS and phenotypes, whereas POEMcoloc [ 350 ] is an approximation to the COLOC method that can be applied when limited data are available. SparkINFERNO [ 351 ], PwCoCo [ 352 ] and ColocQuiaL [ 353 ] are pipelines offering additional functionalities, all using COLOC. eCAVIAR is another popular method [ 354 ] that uses a probabilistic model that accounts for more than one causal variant at a given locus. MSG [ 355 ] increases the power using a spliced gene approach and SharePro [ 356 ] integrates LD modeling and colocalization assessment to account for multiple causal variants in colocalization analysis. PESCA [ 357 ] uses estimates of LD that are ancestry-matched, in order to infer proportions of population-specific and shared causal variants in two populations. These estimates are then used as priors in an empirical Bayes framework for colocalization and test for enrichment of these causal variants in loci of interest. Lastly, we have to mention the methods that operate as webservers offering ease of use. Sherlock [ 358 ] which is also one of the oldest methods, uses a database of eQTL associations from different tissues to identify genetic signatures that match those for specific genes. Unlike other methods it incorporates information from both cis- and trans- eQTL SNPs. LocusFocus [ 359 ] is a web-based colocalization tool that tests colocalization using the Simple Sum method to identify relevant genes and tissues for a particular GWAS locus in the presence of high linkage disequilibrium and/or allelic heterogeneity. Regarding the analysis of eQTL data, ezQTL [ 360 ] is a webserver performing various tasks like data quality control for variants matched between different datasets, LD visualization, and colocalization analysis using eCAVIAR and HyPrColoc, whereas BAGEA [ 361 ] uses a variational Bayes framework to model cis-eQTLs using directed and undirected genomic annotations.
Conclusions
Summary statistics offer protection of privacy over IPD, as well as significant advantages in computational cost, which does not scale with the number of individuals in the study [ 11 ]. Naturally, in the post-GWAS era it is expected that a large number of methods would be developed to perform analysis using the summary results of GWAS [ 11 ]. The particular methods, integrating data from multiple sources such as LD, gene expression and biological pathways, aim to provide biological insight and improve our understanding about the functional role of identified variants [ 12 , 13 , 14 , 15 ]. One thing which we should emphasize is the fact that GWAS summary statistics are not mere replacements for IPD. Of course, some types of analysis can be applied using both summary data or IPD, like meta-analysis, heritability analysis, fine-mapping and so on. In such cases the summary data methods greatly enhance the applicability and the ease of use overcoming the limitations of IPD mentioned earlier. However, methods for other types of analysis, and particularly those that use multiple datasets, like TWAS, colocalization or Mendelian Randomization were designed having in mind the summary data and the integration of data from multiple sources. This is exactly the spirit of the so-called post-GWAS analysis that brought bioinformatics into a central role in genetics research [ 11 ]. Most of the “success stories” in GWAS during the last years can be attributed to the development and the application of such methods in identifying new variants, in functional annotation, causal discovery or even in medical applications [ 2 , 12 , 362 ].
In this work we conducted, for the first time in the literature, a systematic review in order to identify software tools and databases dedicated to GWAS summary data analysis. We categorized the tools and databases by their functionality, in categories related to data, single-trait analysis, and multiple-trait analysis, along with their sub-categories which we analyzed and reviewed. We also compared the tools and databases based on their features, limitations, and user-friendliness. Our review identified a wide range of tools, each with unique strengths and limitations. We provided descriptions of the key features of each tool and database, including their input/output formats, data types, and computational requirements. We also discussed the overall usability and applicability of each tool for different research scenarios. We identified families of related tools for performing different or complementary tasks, for instance the CAVIAR tools (CAVIAR, CAVIARBF, msCAVIAR, eCAVIAR), the EpiXcan tools (S-MultiXcan, S-PrediXcan), the LDAK programs (SumHer, GBAT), the MAGMA tools (nMAGMA, H-MAGMA, eMAGMA) and so on. We need to emphasize that in many cases a tool, originally developed for IPD, is later adapted to handle summary data, whereas in other cases a tool is succeeded by a newer version with added capabilities. For instance, the original PrediXcan method uses only IPD, but it is now considered deprecated. S-PrediXcan and S-MultiXcan are later versions that are designed to be used with summary data. The same is the case regarding SKAT. The original method uses only IPD, but later implementations like metaSKAT or SKAT-O allow for summary data as well. At the same time, it is of importance that there are several tools that combine different functionalities. For instance there are tools that can perform meta-analysis and GSA (MAGENTA), gene-based methods that also offer functionalities for conditional analysis (GCTA), methods for analysis of multiple traits with gene-based tests (multiSKAT, MSKAT), methods that can be seen both as methods for multiple-traits or as meta-analysis (PLEIO, PASCAL), methods that perform both GSA and gene-based tests (aSPU, snpGeneSets, PascalX, PASCAL,MAGMA, FUMA). Of course, there are several single-purpose methods that use and combine different statistical tests or different methods (OWC, MCA, TWT, EBMMT, COMBAT, sumFREGAT, MKATR), and we may not forget methods like LDSC, with its variants, which was originally developed for distinguishing polygenicity from bias, but it is also used for estimating heritability and genetic correlation being integrated in many other tools and pipelines.
As we already mentioned, the tools and databases included in the study were those with a functioning URL. In many publications identified through the literature search the URL was not working. In some situations, we recovered a valid link by performing google searches, or by identifying the authors’ websites, but in many cases, this was not enough. Similarly, several tools deposited in CRAN had been removed or archived. This kind of problem is something already known in the scientific community for years [ 363 , 364 , 365 ]. However, there is more to it. Even for the tools included in the review we could not verify without proper testing that they all work seamlessly, especially for the older ones [ 366 ]. Operating systems evolve, programming languages change, and with these the dependencies of each software also change. Even though there are available best practices [ 367 ], it is not always realistic to expect complex software to work forever without maintenance. Even for some of the tools having valid URLs, for instance deposited on GitHub, or on personal web pages, we found statements by the authors indicating that the software is no longer maintained and that it is not easy to provide technical support. It is clear that more advanced solutions should be pursued. For instance, among the tools we identified the majority are written in R and Python, but only a handful is available as a webserver: ten of the tools for GSA, three tools for colocalization, two tools for meta-analysis, and one for pleiotropy analysis, MR and fine-mapping. Of course, several of the secondary databases we identified also provide the functionality of performing the analysis using data provided by the user (webTWAS, TSEA-DB, PCGA), but even counting these the proportion of web-tools is rather low (< 10%). Web servers and web services have become of high relevance to the field of bioinformatics during the last 20 years [ 368 ], so it is expected to have an increasing number of relevant webservers in the near future as relevant tools are available to facilitate the incorporation of existing applications [ 369 , 370 , 371 , 372 ]. On the other hand, some tools may be too computationally demanding, so other solutions must be found. Container-based applications [ 373 , 374 ] such as Docker can simplify maintenance procedures and add to the reproducibility of research [ 375 ]. Community efforts such as udocker [ 376 ] may promote usability of complex software tools by non-experts in multi-user environments.
As data accumulates it is unavoidable to head to analyses on an even larger scale. Traditionally the large-scale analysis of many gene-disease associations is modeled by the so-called diseasome [ 377 , 378 ] using graph theoretic methods [ 379 , 380 ]. The gene-disease network is composed of pairwise associations obtained from public databases and is a bipartite network [ 379 ] consisting of two separate sets of nodes and the interactions between nodes belonging to the different sets. The projection to the one or the other of the sets may lead to the gene–gene or the disease-disease projected networks that inform us about the associations between members of the same set (for instance, two diseases are connected if they share common genes, and so on). Such methods are available for years, but they treat the associations as fixed inputs to the graph. As data accumulate and even more complex statistical methods are developed that allow cross-trait comparisons and combined analyses of multiple traits, along with the integration of different types of data such as xQTL, it is tempting to speculate that a fusion of these two traditions may come, in which the statistical formalism of the tools presented in this review will merge with the graph theoretic approaches developed in the systems biology literature. For instance, we may see network approaches leading to causal analyses (similar to MR) that consider simultaneously all the diseases and traits for which we have GWAS summary data, or similar approaches that integrate xQTL data of various types, different tissues and so on.
We hope that this comprehensive review will serve as a valuable resource for researchers who are interested in using GWAS summary statistics to investigate the genetic basis of complex traits and diseases, as well as to methodologists that develop and test relevant methods. We provided a detailed overview of the available tools and databases, and we hope that this work will facilitate informed tool selection and will maximize the effectiveness of using GWAS summary statistics.
Availability of data and materials
The data collected in this study are available in Supplementary Material. Supplementary Table 1 contains the list with the identified tools along with the URLs, the references and the descriptions. Supplementary Table 2 contains the list with the additional datasets identified in various consortia.
Uffelmann E, Huang QQ, Munung NS, de Vries J, Okada Y, Martin AR, et al. Genome-wide association studies. Nature Reviews Methods Primers. 2021;1(1):59.
Article CAS Google Scholar
Abdellaoui A, Yengo L, Verweij KJH, Visscher PM. 15 years of GWAS discovery: Realizing the promise. Am J Hum Genet. 2023;110(2):179–94.
Article CAS PubMed PubMed Central Google Scholar
Ziegler A, Konig IR, Thompson JR. Biostatistical aspects of genome-wide association studies. Biom J. 2008;50(1):8–28.
Article PubMed Google Scholar
Alsheikh AJ, Wollenhaupt S, King EA, Reeb J, Ghosh S, Stolzenburg LR, et al. The landscape of GWAS validation; systematic review identifying 309 validated non-coding variants across 130 human diseases. BMC Med Genomics. 2022;15(1):74.
Article PubMed PubMed Central Google Scholar
Moore JH, Asselbergs FW, Williams SM. Bioinformatics challenges for genome-wide association studies. Bioinformatics. 2010;26(4):445–55.
Homer N, Szelinger S, Redman M, Duggan D, Tembe W, Muehling J, et al. Resolving individuals contributing trace amounts of DNA to highly complex mixtures using high-density SNP genotyping microarrays. PLoS Genet. 2008;4(8): e1000167.
Craig DW, Goor RM, Wang Z, Paschall J, Ostell J, Feolo M, et al. Assessing and managing risk when sharing aggregate genetic variant data. Nat Rev Genet. 2011;12(10):730–6.
Cai R, Hao Z, Winslett M, Xiao X, Yang Y, Zhang Z, et al. Deterministic identification of specific individuals from GWAS results. Bioinformatics. 2015;31(11):1701–7.
Thelwall M, Munafo M, Mas-Bleda A, Stuart E, Makita M, Weigert V, et al. Is useful research data usually shared? An investigation of genome-wide association study summary statistics. PLoS ONE. 2020;15(2): e0229578.
Reales G, Wallace C. Sharing GWAS summary statistics results in more citations. Commun Biol. 2023;6(1):116.
Pasaniuc B, Price AL. Dissecting the genetics of complex traits using summary association statistics. Nat Rev Genet. 2017;18(2):117–27.
Article CAS PubMed Google Scholar
Gallagher MD, Chen-Plotkin AS. The Post-GWAS Era: From Association to Function. Am J Hum Genet. 2018;102(5):717–30.
Cano-Gamez E, Trynka G. From GWAS to Function: Using Functional Genomics to Identify the Mechanisms Underlying Complex Diseases. Front Genet. 2020;11:424.
Chimusa ER, Dalvie S, Dandara C, Wonkam A, Mazandu GK. Post genome-wide association analysis: dissecting computational pathway/network-based approaches. Brief Bioinform. 2019;20(2):690–700.
Ishigaki K. Beyond GWAS: from simple associations to functional insights. Semin Immunopathol. 2022;44(1):3–14.
Begum F, Ghosh D, Tseng GC, Feingold E. Comprehensive literature review and statistical considerations for GWAS meta-analysis. Nucleic Acids Res. 2012;40(9):3777–84.
Ioannidis JP, Rosenberg PS, Goedert JJ, O'Brien TR, International Meta-analysis of HIVHG. Commentary: meta-analysis of individual participants' data in genetic epidemiology. Am J Epidemiol. 2002;156(3):204–10.
Tang M, Wang T, Zhang X. A review of SNP heritability estimation methods. Brief Bioinform. 2022;23(3).
Zhu H, Zhou X. Statistical methods for SNP heritability estimation and partition: A review. Comput Struct Biotechnol J. 2020;18:1557–68.
Cinar O, Viechtbauer W. A Comparison of Methods for Gene-Based Testing That Account for Linkage Disequilibrium. Front Genet. 2022;13: 867724.
Mooney MA, Wilmot B. Gene set analysis: A step-by-step guide. Am J Med Genet B Neuropsychiatr Genet. 2015;168(7):517–27.
Schaid DJ, Chen W, Larson NB. From genome-wide associations to candidate causal variants by statistical fine-mapping. Nat Rev Genet. 2018;19(8):491–504.
van Rheenen W, Peyrot WJ, Schork AJ, Lee SH, Wray NR. Genetic correlations of polygenic disease traits: from theory to practice. Nat Rev Genet. 2019;20(10):567–81.
Zhang Y, Cheng Y, Jiang W, Ye Y, Lu Q, Zhao H. Comparison of methods for estimating genetic correlation between complex traits using GWAS summary statistics. Brief Bioinform. 2021;22(5).
Hackinger S, Zeggini E. Statistical methods to detect pleiotropy in human complex traits. Open Biol. 2017;7(11).
Boehm FJ, Zhou X. Statistical methods for Mendelian randomization in genome-wide association studies: A review. Comput Struct Biotechnol J. 2022;20:2338–51.
Wainberg M, Sinnott-Armstrong N, Mancuso N, Barbeira AN, Knowles DA, Golan D, et al. Opportunities and challenges for transcriptome-wide association studies. Nat Genet. 2019;51(4):592–9.
Hukku A, Sampson MG, Luca F, Pique-Regi R, Wen X. Analyzing and reconciling colocalization and transcriptome-wide association studies from the perspective of inferential reproducibility. Am J Hum Genet. 2022;109(5):825–37.
MacArthur JAL, Buniello A, Harris LW, Hayhurst J, McMahon A, Sollis E, et al. Workshop proceedings: GWAS summary statistics standards and sharing. Cell Genom. 2021;1(1).
Page MJ, McKenzie JE, Bossuyt PM, Boutron I, Hoffmann TC, Mulrow CD, et al. The PRISMA 2020 statement: an updated guideline for reporting systematic reviews. BMJ. 2021;372: n71.
Hayhurst J, Buniello A, Harris L, Mosaku A, Chang C, Gignoux CR, et al. A community driven GWAS summary statistics standard. bioRxiv. 2023:2022.07.15.500230.
Danecek P, Auton A, Abecasis G, Albers CA, Banks E, DePristo MA, et al. The variant call format and VCFtools. Bioinformatics. 2011;27(15):2156–8.
Lyon MS, Andrews SJ, Elsworth B, Gaunt TR, Hemani G, Marcora E. The variant call format provides efficient and robust storage of GWAS summary statistics. Genome Biol. 2021;22(1):32.
Elsworth B, Lyon M, Alexander T, Liu Y, Matthews P, Hallett J, et al. The MRC IEU OpenGWAS data infrastructure. bioRxiv. 2020:2020.08.10.244293.
van der Most PJ, Vaez A, Prins BP, Munoz ML, Snieder H, Alizadeh BZ, et al. QCGWAS: A flexible R package for automated quality control of genome-wide association results. Bioinformatics. 2014;30(8):1185–6.
Fuchsberger C, Taliun D, Pramstaller PP, Pattaro C. GWAtoolbox: an R package for fast quality control and handling of genome-wide association studies meta-analysis data. Bioinformatics. 2012;28(3):444–5.
Winkler TW, Day FR, Croteau-Chonka DC, Wood AR, Locke AE, Mägi R, et al. Quality control and conduct of genome-wide association meta-analyses. Nat Protoc. 2014;9(5):1192–212.
Chen GB, Lee SH, Robinson MR, Trzaskowski M, Zhu ZX, Winkler TW, et al. Across-cohort QC analyses of GWAS summary statistics from complex traits. Eur J Hum Genet. 2016;25(1):137–46.
Murphy AE, Schilder BM, Skene NG. MungeSumstats: a Bioconductor package for the standardization and quality control of many GWAS summary statistics. Bioinformatics. 2021;37(23):4593–6.
He Y, Koido M, Shimmori Y, Kamatani Y. GWASLab: a Python package for processing and visualizing GWAS summary statistics. 2023.
Matushyn M, Bose M, Mahmoud AA, Cuthbertson L, Tello C, Bircan KO, et al. SumStatsRehab: an efficient algorithm for GWAS summary statistics assessment and restoration. BMC Bioinformatics. 2022;23(1):443.
Ani A, van der Most PJ, Snieder H, Vaez A, Nolte IM. GWASinspector: comprehensive quality control of genome-wide association study results. Bioinformatics. 2021;37(1):129–30.
Awasthi S, Chen CY, Lam M, Huang H, Ripke S, Altar CA. GWAS quality score for evaluating associated regions in GWAS analyses. Bioinformatics. 2023;39(1).
Chen W, Wu Y, Zheng Z, Qi T, Visscher PM, Zhu Z, et al. Improved analyses of GWAS summary statistics by reducing data heterogeneity and errors. Nat Commun. 2021;12(1):7117.
Williams CM, Poore H, Tanksley PT, Kweon H, Courchesne-Krak NS, Londono-Correa D, et al. Guidelines for Evaluating the Comparability of Down-Sampled GWAS Summary Statistics. Behav Genet. 2023;53(5–6):404–15.
Baxevanis AD, Bateman A. The Importance of Biological Databases in Biological Discovery. Curr Protoc Bioinformatics. 2015;50:1–8.
Article Google Scholar
Ison J, Rapacki K, Menager H, Kalas M, Rydza E, Chmura P, et al. Tools and data services registry: a community effort to document bioinformatics resources. Nucleic Acids Res. 2016;44(D1):D38-47.
Rigden DJ, Fernandez XM. The 27th annual Nucleic Acids Research database issue and molecular biology database collection. Nucleic Acids Res. 2020;48(D1):D1–8.
Zou D, Ma L, Yu J, Zhang Z. Biological databases for human research. Genomics Proteomics Bioinformatics. 2015;13(1):55–63.
Hassani-Pak K, Rawlings C. Knowledge Discovery in Biological Databases for Revealing Candidate Genes Linked to Complex Phenotypes. J Integr Bioinform. 2017;14(1).
Mailman MD, Feolo M, Jin Y, Kimura M, Tryka K, Bagoutdinov R, et al. The NCBI dbGaP database of genotypes and phenotypes. Nat Genet. 2007;39(10):1181–6.
Buniello A, MacArthur JAL, Cerezo M, Harris LW, Hayhurst J, Malangone C, et al. The NHGRI-EBI GWAS Catalog of published genome-wide association studies, targeted arrays and summary statistics 2019. Nucleic Acids Res. 2019;47(D1):D1005–12.
Beck T, Rowlands T, Shorter T, Brookes AJ. GWAS Central: an expanding resource for finding and visualising genotype and phenotype data from genome-wide association studies. Nucleic Acids Res. 2023;51(D1):D986–93.
Canela-Xandri O, Rawlik K, Tenesa A. An atlas of genetic associations in UK Biobank. Nat Genet. 2018;50(11):1593–9.
McInnes G, Tanigawa Y, DeBoever C, Lavertu A, Olivieri JE, Aguirre M, et al. Global Biobank Engine: enabling genotype-phenotype browsing for biobank summary statistics. Bioinformatics. 2019;35(14):2495–7.
Consortium GT. The GTEx Consortium atlas of genetic regulatory effects across human tissues. Science. 2020;369(6509):1318–30.
Huang D, Feng X, Yang H, Wang J, Zhang W, Fan X, et al. QTLbase2: an enhanced catalog of human quantitative trait loci on extensive molecular phenotypes. Nucleic Acids Res. 2023;51(D1):D1122–8.
Dai Y, Hu R, Manuel AM, Liu A, Jia P, Zhao Z. CSEA-DB: an omnibus for human complex trait and cell type associations. Nucleic Acids Res. 2021;49(D1):D862–70.
Xue C, Jiang L, Zhou M, Long Q, Chen Y, Li X, et al. PCGA: a comprehensive web server for phenotype-cell-gene association analysis. Nucleic Acids Res. 2022;50(W1):W568–76.
Cao C, Wang J, Kwok D, Cui F, Zhang Z, Zhao D, et al. webTWAS: a resource for disease candidate susceptibility genes identified by transcriptome-wide association study. Nucleic Acids Res. 2022;50(D1):D1123–30.
Pan S, Kang H, Liu X, Li S, Yang P, Wu M, et al. COLOCdb: a comprehensive resource for multi-model colocalization of complex traits. Nucleic Acids Res. 2024;52(D1):D871–81.
Watanabe K, Stringer S, Frei O, Umicevic Mirkov M, de Leeuw C, Polderman TJC, et al. A global overview of pleiotropy and genetic architecture in complex traits. Nat Genet. 2019;51(9):1339–48.
Patron J, Serra-Cayuela A, Han B, Li C, Wishart DS. Assessing the performance of genome-wide association studies for predicting disease risk. PLoS ONE. 2019;14(12): e0220215.
Bastarache L, Denny JC, Roden DM. Phenome-Wide Association Studies. JAMA. 2022;327(1):75–6.
Verma A, Ritchie MD. Current Scope and Challenges in Phenome-Wide Association Studies. Curr Epidemiol Rep. 2017;4(4):321–9.
Wang L, Zhang X, Meng X, Koskeridis F, Georgiou A, Yu L, et al. Methodology in phenome-wide association studies: a systematic review. J Med Genet. 2021;58(11):720–8.
Kamat MA, Blackshaw JA, Young R, Surendran P, Burgess S, Danesh J, et al. PhenoScanner V2: an expanded tool for searching human genotype-phenotype associations. Bioinformatics. 2019;35(22):4851–3.
Denny JC, Bastarache L, Ritchie MD, Carroll RJ, Zink R, Mosley JD, et al. Systematic comparison of phenome-wide association study of electronic medical record data and genome-wide association study data. Nat Biotechnol. 2013;31(12):1102–10.
Zheng J, Erzurumluoglu AM, Elsworth BL, Kemp JP, Howe L, Haycock PC, et al. LD Hub: a centralized database and web interface to perform LD score regression that maximizes the potential of summary level GWAS data for SNP heritability and genetic correlation analysis. Bioinformatics. 2017;33(2):272–9.
Li Y, Willer C, Sanna S, Abecasis G. Genotype imputation. Annu Rev Genomics Hum Genet. 2009;10:387–406.
Marchini J, Howie B. Genotype imputation for genome-wide association studies. Nat Rev Genet. 2010;11(7):499–511.
Naj AC. Genotype Imputation in Genome-Wide Association Studies. Curr Protoc Hum Genet. 2019;102(1): e84.
Dickhaus T, Stange J, Demirhan H. On an extended interpretation of linkage disequilibrium in genetic case-control association studies. Stat Appl Genet Mol Biol. 2015;14(5):497–505.
Kwan JS, Li MX, Deng JE, Sham PC. FAPI: Fast and accurate P-value Imputation for genome-wide association study. Eur J Hum Genet. 2016;24(5):761–6.
Pasaniuc B, Zaitlen N, Shi H, Bhatia G, Gusev A, Pickrell J, et al. Fast and accurate imputation of summary statistics enhances evidence of functional enrichment. Bioinformatics. 2014;30(20):2906–14.
Julienne H, Shi H, Pasaniuc B, Aschard H. RAISS: robust and accurate imputation from summary statistics. Bioinformatics. 2019;35(22):4837–9.
Lee D, Bigdeli TB, Williamson VS, Vladimirov VI, Riley BP, Fanous AH, et al. DISTMIX: direct imputation of summary statistics for unmeasured SNPs from mixed ethnicity cohorts. Bioinformatics. 2015;31(19):3099–104.
Rueger S, McDaid A, Kutalik Z. Evaluation and application of summary statistic imputation to discover new height-associated loci. PLoS Genet. 2018;14(5): e1007371.
Xu Z, Duan Q, Yan S, Chen W, Li M, Lange E, et al. DISSCO: direct imputation of summary statistics allowing covariates. Bioinformatics. 2015;31(15):2434–42.
Lee D, Bigdeli TB, Riley BP, Fanous AH, Bacanu SA. DIST: direct imputation of summary statistics for unmeasured SNPs. Bioinformatics. 2013;29(22):2925–7.
Togninalli M, Roqueiro D, Investigators CO, Borgwardt KM. Accurate and adaptive imputation of summary statistics in mixed-ethnicity cohorts. Bioinformatics. 2018;34(17):i687–96.
Park DS, Brown B, Eng C, Huntsman S, Hu D, Torgerson DG, et al. Adapt-Mix: learning local genetic correlation structure improves summary statistics-based analyses. Bioinformatics. 2015;31(12):i181–9.
Ren J, Lin Z, Pan W. Integrating GWAS summary statistics, individual-level genotypic and omic data to enhance the performance for large-scale trait imputation. Hum Mol Genet. 2023;32(17):2693–703.
Ren J, Lin Z, He R, Shen X, Pan W. Using GWAS summary data to impute traits for genotyped individuals. HGG Adv. 2023;4(3): 100197.
CAS PubMed PubMed Central Google Scholar
Yang Z, Paschou P, Drineas P. Reconstructing SNP allele and genotype frequencies from GWAS summary statistics. Sci Rep. 2022;12(1):8242.
Bagos PG, Nikolopoulos GK. A method for meta-analysis of case-control genetic association studies using logistic regression. Stat Appl Genet Mol Biol. 2007;6:Article17.
Bagos PG. A unification of multivariate methods for meta-analysis of genetic association studies. Stat Appl Genet Mol Biol. 2008;7(1):Article31.
Bagos PG. Genetic model selection in genome-wide association studies: robust methods and the use of meta-analysis. Stat Appl Genet Mol Biol. 2013;12(3):285–308.
Dimou NL, Tsirigos KD, Elofsson A, Bagos PG. GWAR: robust analysis and meta-analysis of genome-wide association studies. Bioinformatics. 2017;33(10):1521–7.
Di Pietrantonj C. Four-fold table cell frequencies imputation in meta analysis. Stat Med. 2006;25(13):2299–322.
Nolte IM. Metasubtract: an R-package to analytically produce leave-one-out meta-analysis GWAS summary statistics. Bioinformatics. 2020;36(16):4521–2.
Woolf B, Sallis HM, Munafò MR, Gill D. Deriving GWAS summary estimates for paternal smoking in UK biobank: a GWAS by subtraction. BMC Res Notes. 2023;16(1):159.
Niu YF, Ye C, He J, Han F, Guo LB, Zheng HF, et al. Reproduction and In-Depth Evaluation of Genome-Wide Association Studies and Genome-Wide Meta-analyses Using Summary Statistics. G3 (Bethesda). 2017;7(3):943–52.
Lloyd-Jones LR, Robinson MR, Yang J, Visscher PM. Transformation of Summary Statistics from Linear Mixed Model Association on All-or-None Traits to Odds Ratio. Genetics. 2018;208(4):1397–408.
Forero DA, Lopez-Leon S, González-Giraldo Y, Bagos PG. Ten simple rules for carrying out and writing meta-analyses. PLoS Comput Biol. 2019;15(5): e1006922.
Lin DY, Zeng D. Meta-analysis of genome-wide association studies: no efficiency gain in using individual participant data. Genet Epidemiol. 2010;34(1):60–6.
Riley RD, Lambert PC, Staessen JA, Wang J, Gueyffier F, Thijs L, et al. Meta-analysis of continuous outcomes combining individual patient data and aggregate data. Stat Med. 2008;27(11):1870–93.
Dai M, Ming J, Cai M, Liu J, Yang C, Wan X, et al. IGESS: a statistical approach to integrating individual-level genotype data and summary statistics in genome-wide association studies. Bioinformatics. 2017;33(18):2882–9.
Fu S, Deng L, Zhang H, Qin J, Yu K. Integrative analysis of individual-level data and high-dimensional summary statistics. Bioinformatics. 2023;39(4).
Dai M, Wan X, Peng H, Wang Y, Liu Y, Liu J, et al. Joint analysis of individual-level and summary-level GWAS data by leveraging pleiotropy. Bioinformatics. 2019;35(10):1729–36.
Fu S, Purdue MP, Zhang H, Qin J, Song L, Berndt SI, et al. Improve the model of disease subtype heterogeneity by leveraging external summary data. PLoS Comput Biol. 2023;19(7): e1011236.
Evangelou E, Ioannidis JP. Meta-analysis methods for genome-wide association studies and beyond. Nat Rev Genet. 2013;14(6):379–89.
Willer CJ, Li Y, Abecasis GR. METAL: fast and efficient meta-analysis of genomewide association scans. Bioinformatics. 2010;26(17):2190–1.
Mägi R, Morris AP. GWAMA: software for genome-wide association meta-analysis. BMC Bioinformatics. 2010;11:288.
Purcell S, Neale B, Todd-Brown K, Thomas L, Ferreira MA, Bender D, et al. PLINK: a tool set for whole-genome association and population-based linkage analyses. Am J Hum Genet. 2007;81(3):559–75.
Meesters C, Leber M, Herold C, Angisch M, Mattheisen M, Drichel D, et al. Quick, “imputation-free” meta-analysis with proxy-SNPs. BMC Bioinformatics. 2012;13:231.
Jiang Y, Chen S, McGuire D, Chen F, Liu M, Iacono WG, et al. Proper conditional analysis in the presence of missing data: Application to large scale meta-analysis of tobacco use phenotypes. PLoS Genet. 2018;14(7): e1007452.
Jiang W, Yu W. Jointly determining significance levels of primary and replication studies by controlling the false discovery rate in two-stage genome-wide association studies. Stat Methods Med Res. 2018;27(9):2795–808.
Jiang W, Yu W. Controlling the joint local false discovery rate is more powerful than meta-analysis methods in joint analysis of summary statistics from multiple genome-wide association studies. Bioinformatics. 2017;33(4):500–7.
Jiang W, Xue JH, Yu W. What is the probability of replicating a statistically significant association in genome-wide association studies? Brief Bioinform. 2017;18(6):928–39.
PubMed Google Scholar
Xie Y, Zhai S, Jiang W, Zhao H, Mehrotra DV, Shen J. Statistical assessment of biomarker replicability using MAJAR method. Stat Methods Med Res. 2023;32(10):1961–72.
de Vlaming R, Okbay A, Rietveld CA, Johannesson M, Magnusson PK, Uitterlinden AG, et al. Meta-GWAS Accuracy and Power (MetaGAP) Calculator Shows that Hiding Heritability Is Partially Due to Imperfect Genetic Correlations across Studies. PLoS Genet. 2017;13(1): e1006495.
Province MA, Borecki IB. A correlated meta-analysis strategy for data mining "OMIC" scans. Pac Symp Biocomput. 2013:236–46.
Segrè AV, Groop L, Mootha VK, Daly MJ, Altshuler D. Common inherited variation in mitochondrial genes is not enriched for associations with type 2 diabetes or related glycemic traits. PLoS Genet. 2010;6(8).
Sun J, Lyu R, Deng L, Li Q, Zhao Y, Zhang Y. SMetABF: A rapid algorithm for Bayesian GWAS meta-analysis with a large number of studies included. PLoS Comput Biol. 2022;18(3): e1009948.
Trochet H, Pirinen M, Band G, Jostins L, McVean G, Spencer CCA. Bayesian meta-analysis across genome-wide association studies of diverse phenotypes. Genet Epidemiol. 2019;43(5):532–47.
Baselmans BML, Jansen R, Ip HF, van Dongen J, Abdellaoui A, van de Weijer MP, et al. Multivariate genome-wide analyses of the well-being spectrum. Nat Genet. 2019;51(3):445–51.
Cichonska A, Rousu J, Marttinen P, Kangas AJ, Soininen P, Lehtimäki T, et al. metaCCA: summary statistics-based multivariate meta-analysis of genome-wide association studies using canonical correlation analysis. Bioinformatics. 2016;32(13):1981–9.
Zhu X, Feng T, Tayo BO, Liang J, Young JH, Franceschini N, et al. Meta-analysis of correlated traits via summary statistics from GWASs with an application in hypertension. Am J Hum Genet. 2015;96(1):21–36.
Ray D, Boehnke M. Methods for meta-analysis of multiple traits using GWAS summary statistics. Genet Epidemiol. 2018;42(2):134–45.
Baghfalaki T, Sugier PE, Truong T, Pettitt AN, Mengersen K, Liquet B. Bayesian meta-analysis models for cross cancer genomic investigation of pleiotropic effects using group structure. Stat Med. 2021;40(6):1498–518.
John M, Lencz T, Malhotra AK, Correll CU, Zhang JP. A simulations approach for meta-analysis of genetic association studies based on additive genetic model. Meta Gene. 2018;16:143–64.
Nasirigerdeh R, Torkzadehmahani R, Matschinske J, Frisch T, List M, Späth J, et al. sPLINK: a hybrid federated tool as a robust alternative to meta-analysis in genome-wide association studies. Genome Biol. 2022;23(1):32.
Coram MA, Candille SI, Duan Q, Chan KH, Li Y, Kooperberg C, et al. Leveraging Multi-ethnic Evidence for Mapping Complex Traits in Minority Populations: An Empirical Bayes Approach. Am J Hum Genet. 2015;96(5):740–52.
Tenesa A, Haley CS. The heritability of human disease: estimation, uses and abuses. Nat Rev Genet. 2013;14(2):139–49.
Visscher PM, Hill WG, Wray NR. Heritability in the genomics era–concepts and misconceptions. Nat Rev Genet. 2008;9(4):255–66.
Barry CS, Walker VM, Cheesman R, Davey Smith G, Morris TT, Davies NM. How to estimate heritability: a guide for genetic epidemiologists. Int J Epidemiol. 2023;52(2):624–32.
Zaitlen N, Kraft P. Heritability in the genome-wide association era. Hum Genet. 2012;131(10):1655–64.
So HC, Gui AH, Cherny SS, Sham PC. Evaluating the heritability explained by known susceptibility variants: a survey of ten complex diseases. Genet Epidemiol. 2011;35(5):310–7.
So HC, Li M, Sham PC. Uncovering the total heritability explained by all true susceptibility variants in a genome-wide association study. Genet Epidemiol. 2011;35(6):447–56.
Palla L, Dudbridge F. A Fast Method that Uses Polygenic Scores to Estimate the Variance Explained by Genome-wide Marker Panels and the Proportion of Variants Affecting a Trait. Am J Hum Genet. 2015;97(2):250–9.
Shi H, Kichaev G, Pasaniuc B. Contrasting the Genetic Architecture of 30 Complex Traits from Summary Association Data. Am J Hum Genet. 2016;99(1):139–53.
Bulik-Sullivan BK, Loh PR, Finucane HK, Ripke S, Yang J, Patterson N, et al. LD Score regression distinguishes confounding from polygenicity in genome-wide association studies. Nat Genet. 2015;47(3):291–5.
Song S, Jiang W, Zhang Y, Hou L, Zhao H. Leveraging LD eigenvalue regression to improve the estimation of SNP heritability and confounding inflation. Am J Hum Genet. 2022;109(5):802–11.
Finucane HK, Bulik-Sullivan B, Gusev A, Trynka G, Reshef Y, Loh PR, et al. Partitioning heritability by functional annotation using genome-wide association summary statistics. Nat Genet. 2015;47(11):1228–35.
Speed D, Balding DJ. SumHer better estimates the SNP heritability of complex traits from summary statistics. Nat Genet. 2019;51(2):277–84.
Li H, Mazumder R, Lin X. Accurate and efficient estimation of local heritability using summary statistics and the linkage disequilibrium matrix. Nat Commun. 2023;14(1):7954.
Laville V, Bentley AR, Privé F, Zhu X, Gauderman J, Winkler TW, et al. VarExp: estimating variance explained by genome-wide GxE summary statistics. Bioinformatics. 2018;34(19):3412–4.
Shin J, Lee SH. GxEsum: a novel approach to estimate the phenotypic variance explained by genome-wide GxE interaction based on GWAS summary statistics for biobank-scale data. Genome Biol. 2021;22(1):183.
Song L, Liu A, Shi J. SummaryAUC: a tool for evaluating the performance of polygenic risk prediction models in validation datasets with only summary level statistics. Bioinformatics. 2019;35(20):4038–44.
Chan TF, Rui X, Conti DV, Fornage M, Graff M, Haessler J, et al. Estimating heritability explained by local ancestry and evaluating stratification bias in admixture mapping from summary statistics. Am J Hum Genet. 2023;110(11):1853–62.
Zhang Y, Qi G, Park JH, Chatterjee N. Estimation of complex effect-size distributions using summary-level statistics from genome-wide association studies across 32 complex traits. Nat Genet. 2018;50(9):1318–26.
López-Cortegano E, Caballero A. GWEHS: A Genome-Wide Effect Sizes and Heritability Screener. Genes (Basel). 2019;10(8).
O’Connor LJ. The distribution of common-variant effect sizes. Nat Genet. 2021;53(8):1243–9.
Holland D, Frei O, Desikan R, Fan CC, Shadrin AA, Smeland OB, et al. Beyond SNP heritability: Polygenicity and discoverability of phenotypes estimated with a univariate Gaussian mixture model. PLoS Genet. 2020;16(5): e1008612.
Yao DW, O’Connor LJ, Price AL, Gusev A. Quantifying genetic effects on disease mediated by assayed gene expression levels. Nat Genet. 2020;52(6):626–33.
Siewert-Rocks KM, Kim SS, Yao DW, Shi H, Price AL. Leveraging gene co-regulation to identify gene sets enriched for disease heritability. Am J Hum Genet. 2022;109(3):393–404.
Neale BM, Sham PC. The future of association studies: gene-based analysis and replication. Am J Hum Genet. 2004;75(3):353–62.
Li B, Leal SM. Methods for detecting associations with rare variants for common diseases: application to analysis of sequence data. Am J Hum Genet. 2008;83(3):311–21.
Wu MC, Lee S, Cai T, Li Y, Boehnke M, Lin X. Rare-variant association testing for sequencing data with the sequence kernel association test. Am J Hum Genet. 2011;89(1):82–93.
Chapman J, Whittaker J. Analysis of multiple SNPs in a candidate gene or region. Genet Epidemiol. 2008;32(6):560–6.
Lee D, Williamson VS, Bigdeli TB, Riley BP, Fanous AH, Vladimirov VI, et al. JEPEG: a summary statistics based tool for gene-level joint testing of functional variants. Bioinformatics. 2015;31(8):1176–82.
Yang J, Ferreira T, Morris AP, Medland SE, Madden PA, Heath AC, et al. Conditional and joint multiple-SNP analysis of GWAS summary statistics identifies additional variants influencing complex traits. Nat Genet. 2012;44(4):369–75, s1–3.
Li M, Jiang L, Mak TSH, Kwan JSH, Xue C, Chen P, et al. A powerful conditional gene-based association approach implicated functionally important genes for schizophrenia. Bioinformatics. 2019;35(4):628–35.
Li MX, Gui HS, Kwan JS, Sham PC. GATES: a rapid and powerful gene-based association test using extended Simes procedure. Am J Hum Genet. 2011;88(3):283–93.
Bakshi A, Zhu Z, Vinkhuyzen AA, Hill WD, McRae AF, Visscher PM, et al. Fast set-based association analysis using summary data from GWAS identifies novel gene loci for human complex traits. Sci Rep. 2016;6:32894.
de Leeuw CA, Mooij JM, Heskes T, Posthuma D. MAGMA: generalized gene-set analysis of GWAS data. PLoS Comput Biol. 2015;11(4): e1004219.
Yang A, Chen J, Zhao XM. nMAGMA: a network-enhanced method for inferring risk genes from GWAS summary statistics and its application to schizophrenia. Brief Bioinform. 2021;22(4).
Sey NYA, Pratt BM, Won H. Annotating genetic variants to target genes using H-MAGMA. Nat Protoc. 2023;18(1):22–35.
Gerring ZF, Mina-Vargas A, Gamazon ER, Derks EM. E-MAGMA: an eQTL-informed method to identify risk genes using genome-wide association study summary statistics. Bioinformatics. 2021;37(16):2245–9.
Wang R, Lin DY, Jiang Y. EPIC: Inferring relevant cell types for complex traits by integrating genome-wide association studies and single-cell RNA sequencing. PLoS Genet. 2022;18(6): e1010251.
Quick C, Wen X, Abecasis G, Boehnke M, Kang HM. Integrating comprehensive functional annotations to boost power and accuracy in gene-based association analysis. PLoS Genet. 2020;16(12): e1009060.
Yurko R, Roeder K, Devlin B, G'Sell M. An approach to gene-based testing accounting for dependence of tests among nearby genes. Brief Bioinform. 2021;22(6).
Vsevolozhskaya OA, Shi M, Hu F, Zaykin DV. DOT: Gene-set analysis by combining decorrelated association statistics. PLoS Comput Biol. 2020;16(4): e1007819.
Zhang J, Zhao Z, Guo X, Guo B, Wu B. Powerful statistical method to detect disease-associated genes using publicly available genome-wide association studies summary data. Genet Epidemiol. 2019;43(8):941–51.
Chen X, Zhang H, Liu M, Deng HW, Wu Z. Simultaneous detection of novel genes and SNPs by adaptive p-value combination. Front Genet. 2022;13:1009428.
Zhang J, Guo X, Gonzales S, Yang J, Wang X. TS: a powerful truncated test to detect novel disease associated genes using publicly available gWAS summary data. BMC Bioinformatics. 2020;21(1):172.
Kwak IY, Pan W. Gene- and pathway-based association tests for multiple traits with GWAS summary statistics. Bioinformatics. 2017;33(1):64–71.
Guo B, Wu B. Statistical methods to detect novel genetic variants using publicly available GWAS summary data. Comput Biol Chem. 2018;74:76–9.
Wang M, Huang J, Liu Y, Ma L, Potash JB, Han S. COMBAT: A Combined Association Test for Genes Using Summary Statistics. Genetics. 2017;207(3):883–91.
Shao Z, Wang T, Qiao J, Zhang Y, Huang S, Zeng P. A comprehensive comparison of multilocus association methods with summary statistics in genome-wide association studies. BMC Bioinformatics. 2022;23(1):359.
Zhang J, Liang X, Gonzales S, Liu J, Gao XR, Wang X. A gene based combination test using GWAS summary data. BMC Bioinformatics. 2023;24(1):2.
He Z, Xu B, Lee S, Ionita-Laza I. Unified Sequence-Based Association Tests Allowing for Multiple Functional Annotations and Meta-analysis of Noncoding Variation in Metabochip Data. Am J Hum Genet. 2017;101(3):340–52.
Liu Y, Chen S, Li Z, Morrison AC, Boerwinkle E, Lin X. ACAT: A Fast and Powerful p Value Combination Method for Rare-Variant Analysis in Sequencing Studies. Am J Hum Genet. 2019;104(3):410–21.
Li MX, Kwan JS, Sham PC. HYST: a hybrid set-based test for genome-wide association studies, with application to protein-protein interaction-based association analysis. Am J Hum Genet. 2012;91(3):478–88.
Sun R, Lin X. Genetic Variant Set-Based Tests Using the Generalized Berk-Jones Statistic with Application to a Genome-Wide Association Study of Breast Cancer. J Am Stat Assoc. 2020;115(531):1079–91.
Berrandou TE, Balding D, Speed D. LDAK-GBAT: Fast and powerful gene-based association testing using summary statistics. Am J Hum Genet. 2023;110(1):23–9.
Mei H, Li L, Jiang F, Simino J, Griswold M, Mosley T, et al. snpGeneSets: An R Package for Genome-Wide Study Annotation. G3 (Bethesda). 2016;6(12):4087–95.
Krefl D, Brandulas Cammarata A, Bergmann S. PascalX: a Python library for GWAS gene and pathway enrichment tests. Bioinformatics. 2023;39(5).
Lamparter D, Marbach D, Rueedi R, Kutalik Z, Bergmann S. Fast and Rigorous Computation of Gene and Pathway Scores from SNP-Based Summary Statistics. PLoS Comput Biol. 2016;12(1): e1004714.
Nameki R, Shetty A, Dareng E, Tyrer J, Lin X, Pharoah P, et al. chromMAGMA: regulatory element-centric interrogation of risk variants. Life Sci Alliance. 2022;5(10).
Watanabe K, Taskesen E, van Bochoven A, Posthuma D. Functional mapping and annotation of genetic associations with FUMA. Nat Commun. 2017;8(1):1826.
Yang Y, Basu S, Zhang L. A Bayesian hierarchically structured prior for gene-based association testing with multiple traits in genome-wide association studies. Genet Epidemiol. 2022;46(1):63–72.
Wang K, Li M, Bucan M. Pathway-based approaches for analysis of genomewide association studies. Am J Hum Genet. 2007;81(6):1278–83.
Mooney MA, Nigg JT, McWeeney SK, Wilmot B. Functional and genomic context in pathway analysis of GWAS data. Trends Genet. 2014;30(9):390–400.
Pers TH. Gene set analysis for interpreting genetic studies. Hum Mol Genet. 2016;25(R2):R133–40.
Wang L, Jia P, Wolfinger RD, Chen X, Zhao Z. Gene set analysis of genome-wide association studies: methodological issues and perspectives. Genomics. 2011;98(1):1–8.
Zhang K, Cui S, Chang S, Zhang L, Wang J. i-GSEA4GWAS: a web server for identification of pathways/gene sets associated with traits by applying an improved gene set enrichment analysis to genome-wide association study. Nucleic Acids Res. 2010;38(Web Server issue):W90–5.
Kuleshov MV, Jones MR, Rouillard AD, Fernandez NF, Duan Q, Wang Z, et al. Enrichr: a comprehensive gene set enrichment analysis web server 2016 update. Nucleic Acids Res. 2016;44(W1):W90–7.
Kolberg L, Raudvere U, Kuzmin I, Adler P, Vilo J, Peterson H. g:Profiler-interoperable web service for functional enrichment analysis and gene identifier mapping (2023 update). Nucleic Acids Res. 2023;51(W1):W207–12.
Sherman BT, Hao M, Qiu J, Jiao X, Baseler MW, Lane HC, et al. DAVID: a web server for functional enrichment analysis and functional annotation of gene lists (2021 update). Nucleic Acids Res. 2022;50(W1):W216–21.
Liao Y, Wang J, Jaehnig EJ, Shi Z, Zhang B. WebGestalt 2019: gene set analysis toolkit with revamped UIs and APIs. Nucleic Acids Res. 2019;47(W1):W199-w205.
Mi H, Ebert D, Muruganujan A, Mills C, Albou LP, Mushayamaha T, et al. PANTHER version 16: a revised family classification, tree-based classification tool, enhancer regions and extensive API. Nucleic Acids Res. 2021;49(D1):D394-d403.
Yoon S, Nguyen HCT, Yoo YJ, Kim J, Baik B, Kim S, et al. Efficient pathway enrichment and network analysis of GWAS summary data using GSA-SNP2. Nucleic Acids Res. 2018;46(10): e60.
Wu C, Pan W. Integrating eQTL data with GWAS summary statistics in pathway-based analysis with application to schizophrenia. Genet Epidemiol. 2018;42(3):303–16.
Zhu S, Qian T, Hoshida Y, Shen Y, Yu J, Hao K. GIGSEA: genotype imputed gene set enrichment analysis using GWAS summary level data. Bioinformatics. 2019;35(1):160–3.
Pei G, Dai Y, Zhao Z, Jia P. deTS: tissue-specific enrichment analysis to decode tissue specificity. Bioinformatics. 2019;35(19):3842–5.
Jia P, Zheng S, Long J, Zheng W, Zhao Z. dmGWAS: dense module searching for genome-wide association studies in protein-protein interaction networks. Bioinformatics. 2011;27(1):95–102.
Cochran AL, Nieser KJ, Forger DB, Zöllner S, McInnis MG. Gene-set Enrichment with Mathematical Biology (GEMB). Gigascience. 2020;9(10).
Cabrera CP, Navarro P, Huffman JE, Wright AF, Hayward C, Campbell H, et al. Uncovering networks from genome-wide association studies via circular genomic permutation. G3 (Bethesda). 2012;2(9):1067–75.
Shim JE, Bang C, Yang S, Lee T, Hwang S, Kim CY, et al. GWAB: a web server for the network-based boosting of human genome-wide association data. Nucleic Acids Res. 2017;45(W1):W154–61.
Hoppmann AS, Schlosser P, Backofen R, Lausch E, Köttgen A. GenToS: Use of Orthologous Gene Information to Prioritize Signals from Human GWAS. PLoS ONE. 2016;11(9): e0162466.
Wen Y, Wang W, Guo X, Zhang F. PAPA: a flexible tool for identifying pleiotropic pathways using genome-wide association study summaries. Bioinformatics. 2016;32(6):946–8.
Amlie-Wolf A, Tang M, Mlynarski EE, Kuksa PP, Valladares O, Katanic Z, et al. INFERNO: inferring the molecular mechanisms of noncoding genetic variants. Nucleic Acids Res. 2018;46(17):8740–53.
Ding J, Blencowe M, Nghiem T, Ha SM, Chen YW, Li G, et al. Mergeomics 2.0: a web server for multi-omics data integration to elucidate disease networks and predict therapeutics. Nucleic Acids Res. 2021;49(W1):W375-w87.
Wang QS, Huang H. Methods for statistical fine-mapping and their applications to auto-immune diseases. Semin Immunopathol. 2022;44(1):101–13.
Hutchinson A, Asimit J, Wallace C. Fine-mapping genetic associations. Hum Mol Genet. 2020;29(R1):R81–8.
Kichaev G, Roytman M, Johnson R, Eskin E, Lindström S, Kraft P, et al. Improved methods for multi-trait fine mapping of pleiotropic risk loci. Bioinformatics. 2017;33(2):248–55.
Wen X, Lee Y, Luca F, Pique-Regi R. Efficient Integrative Multi-SNP Association Analysis via Deterministic Approximation of Posteriors. Am J Hum Genet. 2016;98(6):1114–29.
Pickrell JK. Joint analysis of functional genomic data and genome-wide association studies of 18 human traits. Am J Hum Genet. 2014;94(4):559–73.
Benner C, Spencer CC, Havulinna AS, Salomaa V, Ripatti S, Pirinen M. FINEMAP: efficient variable selection using summary data from genome-wide association studies. Bioinformatics. 2016;32(10):1493–501.
Hernández N, Soenksen J, Newcombe P, Sandhu M, Barroso I, Wallace C, et al. The flashfm approach for fine-mapping multiple quantitative traits. Nat Commun. 2021;12(1):6147.
Karhunen V, Launonen I, Järvelin MR, Sebert S, Sillanpää MJ. Genetic fine-mapping from summary data using a nonlocal prior improves the detection of multiple causal variants. Bioinformatics. 2023;39(7).
Yang Z, Wang C, Liu L, Khan A, Lee A, Vardarajan B, et al. CARMA is a new Bayesian model for fine-mapping in genome-wide association meta-analyses. Nat Genet. 2023;55(6):1057–65.
Chen W, Larrabee BR, Ovsyannikova IG, Kennedy RB, Haralambieva IH, Poland GA, et al. Fine Mapping Causal Variants with an Approximate Bayesian Method Using Marginal Test Statistics. Genetics. 2015;200(3):719–36.
LaPierre N, Taraszka K, Huang H, He R, Hormozdiari F, Eskin E. Identifying causal variants by fine mapping across multiple studies. PLoS Genet. 2021;17(9): e1009733.
Cai M, Wang Z, Xiao J, Hu X, Chen G, Yang C. XMAP: Cross-population fine-mapping by leveraging genetic diversity and accounting for confounding bias. Nat Commun. 2023;14(1):6870.
Ghosal S, Schatz MC, Venkataraman A. BEATRICE: Bayesian Fine-mapping from Summary Data using Deep Variational Inference. bioRxiv. 2023.a
Li Y, Kellis M. Joint Bayesian inference of risk variants and tissue-specific epigenomic enrichments across multiple complex human diseases. Nucleic Acids Res. 2016;44(18): e144.
Weissbrod O, Hormozdiari F, Benner C, Cui R, Ulirsch J, Gazal S, et al. Functionally informed fine-mapping and polygenic localization of complex trait heritability. Nat Genet. 2020;52(12):1355–63.
Zou Y, Carbonetto P, Wang G, Stephens M. Fine-mapping from summary data with the “Sum of Single Effects” model. PLoS Genet. 2022;18(7): e1010299.
Chen S, Nunez S, Reilly MP, Foulkes AS. Bayesian variable selection for post-analytic interrogation of susceptibility loci. Biometrics. 2017;73(2):603–14.
Newcombe PJ, Conti DV, Richardson S. JAM: A Scalable Bayesian Framework for Joint Analysis of Marginal SNP Effects. Genet Epidemiol. 2016;40(3):188–201.
Ning Z, Lee Y, Joshi PK, Wilson JF, Pawitan Y, Shen X. A Selection Operator for Summary Association Statistics Reveals Allelic Heterogeneity of Complex Traits. Am J Hum Genet. 2017;101(6):903–12.
Fisher V, Sebastiani P, Cupples LA, Liu CT. ANNORE: genetic fine-mapping with functional annotation. Hum Mol Genet. 2021;31(1):32–40.
Zhang W, Li SY, Liu T, Li Y. Partitioning gene-based variance of complex traits by gene score regression. PLoS ONE. 2020;15(8): e0237657.
Zhu X, Stephens M. BAYESIAN LARGE-SCALE MULTIPLE REGRESSION WITH SUMMARY STATISTICS FROM GENOME-WIDE ASSOCIATION STUDIES. Ann Appl Stat. 2017;11(3):1561–92.
Deng Y, Pan W. Significance Testing for Allelic Heterogeneity. Genetics. 2018;210(1):25–32.
Taylor KE, Ansel KM, Marson A, Criswell LA, Farh KK. PICS2: next-generation fine mapping via probabilistic identification of causal SNPs. Bioinformatics. 2021;37(18):3004–7.
Schilder BM, Humphrey J, Raj T. echolocatoR: an automated end-to-end statistical and functional genomic fine-mapping pipeline. Bioinformatics. 2022;38(2):536–9.
Tyler AL, Crawford DC, Pendergrass SA. The detection and characterization of pleiotropy: discovery, progress, and promise. Brief Bioinform. 2016;17(1):13–22.
Wu P, Wang B, Lubitz SA, Benjamin EJ, Meigs JB, Dupuis J. Approximate conditional phenotype analysis based on genome wide association summary statistics. Sci Rep. 2021;11(1):2518.
Conneely KN, Boehnke M. So many correlated tests, so little time! Rapid adjustment of P values for multiple correlated tests. Am J Hum Genet. 2007;81(6):1158–68.
Taraszka K, Zaitlen N, Eskin E. Leveraging pleiotropy for joint analysis of genome-wide association studies with per trait interpretations. PLoS Genet. 2022;18(11): e1010447.
Deng Y, Pan W. Testing Genetic Pleiotropy with GWAS Summary Statistics for Marginal and Conditional Analyses. Genetics. 2017;207(4):1285–99.
Ray D, Pankow JS, Basu S. USAT: A Unified Score-Based Association Test for Multiple Phenotype-Genotype Analysis. Genet Epidemiol. 2016;40(1):20–34.
Sitlani CM, Baldassari AR, Highland HM, Hodonsky CJ, McKnight B, Avery CL. Comparison of adaptive multiple phenotype association tests using summary statistics in genome-wide association studies. Hum Mol Genet. 2021;30(15):1371–83.
Guo B, Wu B. Integrate multiple traits to detect novel trait-gene association using GWAS summary data with an adaptive test approach. Bioinformatics. 2019;35(13):2251–7.
Turchin MC, Stephens M. Bayesian multivariate reanalysis of large genetic studies identifies many new associations. PLoS Genet. 2019;15(10): e1008431.
Bu D, Wang X, Li Q. Summary statistics-based association test for identifying the pleiotropic effects with set of genetic variants. Bioinformatics. 2023;39(4).
Deng Q, Song C, Lin S. An adaptive and robust method for multi-trait analysis of genome-wide association studies using summary statistics. Eur J Hum Genet. 2023.
Liu W, Xu Y, Wang A, Huang T, Liu Z. The eigen higher criticism and eigen Berk-Jones tests for multiple trait association studies based on GWAS summary statistics. Genet Epidemiol. 2022;46(2):89–104.
Svishcheva GR, Tiys ES, Elgaeva EE, Feoktistova SG, Timmers P, Sharapov SZ, et al. A Novel Framework for Analysis of the Shared Genetic Background of Correlated Traits. Genes (Basel). 2022;13(10).
Qi G, Chatterjee N. Heritability informed power optimization (HIPO) leads to enhanced detection of genetic associations across multiple traits. PLoS Genet. 2018;14(10): e1007549.
Jordan DM, Verbanck M, Do R. HOPS: a quantitative score reveals pervasive horizontal pleiotropy in human genetic variation is driven by extreme polygenicity of human traits and diseases. Genome Biol. 2019;20(1):222.
Ballard JL, O’Connor LJ. Shared components of heritability across genetically correlated traits. Am J Hum Genet. 2022;109(6):989–1006.
Turley P, Walters RK, Maghzian O, Okbay A, Lee JJ, Fontana MA, et al. Multi-trait analysis of genome-wide association summary statistics using MTAG. Nat Genet. 2018;50(2):229–37.
Lee CH, Shi H, Pasaniuc B, Eskin E, Han B. PLEIO: a method to map and interpret pleiotropic loci with GWAS summary statistics. Am J Hum Genet. 2021;108(1):36–48.
Guo B, Wu B. Powerful and efficient SNP-set association tests across multiple phenotypes using GWAS summary data. Bioinformatics. 2019;35(8):1366–72.
Dutta D, Scott L, Boehnke M, Lee S. Multi-SKAT: General framework to test for rare-variant association with multiple phenotypes. Genet Epidemiol. 2019;43(1):4–23.
Van der Sluis S, Dolan CV, Li J, Song Y, Sham P, Posthuma D, et al. MGAS: a powerful tool for multivariate gene-based genome-wide association analysis. Bioinformatics. 2015;31(7):1007–15.
Wang T, Lu H, Zeng P. Identifying pleiotropic genes for complex phenotypes with summary statistics from a perspective of composite null hypothesis testing. Brief Bioinform. 2022;23(1).
Luo L, Shen J, Zhang H, Chhibber A, Mehrotra DV, Tang ZZ. Multi-trait analysis of rare-variant association summary statistics using MTAR. Nat Commun. 2020;11(1):2850.
Zeng P, Hao X, Zhou X. Pleiotropic mapping and annotation selection in genome-wide association studies with penalized Gaussian mixture models. Bioinformatics. 2018;34(16):2797–807.
Deng Q, Gupta A, Jeon H, Nam JH, Yilmaz AS, Chang W, et al. graph-GPA 2.0: improving multi-disease genetic analysis with integration of functional annotation data. Front Genet. 2023;14:1079198.
von Berg J, Ten Dam M, van der Laan SW, de Ridder J. PolarMorphism enables discovery of shared genetic variants across multiple traits from GWAS summary statistics. Bioinformatics. 2022;38(Suppl 1):i212–9.
Julienne H, Laville V, McCaw ZR, He Z, Guillemot V, Lasry C, et al. Multitrait GWAS to connect disease variants and biological mechanisms. PLoS Genet. 2021;17(8): e1009713.
Pickrell JK, Berisa T, Liu JZ, Ségurel L, Tung JY, Hinds DA. Detection and interpretation of shared genetic influences on 42 human traits. Nat Genet. 2016;48(7):709–17.
Zhang Z, Jung J, Kim A, Suboc N, Gazal S, Mancuso N. A scalable approach to characterize pleiotropy across thousands of human diseases and complex traits using GWAS summary statistics. Am J Hum Genet. 2023;110(11):1863–74.
Zilinskas R, Li C, Shen X, Pan W, Yang T. Inferring a directed acyclic graph of phenotypes from GWAS summary statistics. bioRxiv. 2023.
Yin L, Chau CK, Lin YP, Rao S, Xiang Y, Sham PC, et al. A framework to decipher the genetic architecture of combinations of complex diseases: applications in cardiovascular medicine. Bioinformatics. 2021;37(22):4137–47.
Asgari Y, Sugier PE, Baghfalaki T, Lucotte E, Karimi M, Sedki M, et al. GCPBayes pipeline: a tool for exploring pleiotropy at the gene level. NAR Genom Bioinform. 2023;5(3):lqad065.
Liu J, Wan X, Ma S, Yang C. EPS: an empirical Bayes approach to integrating pleiotropy and tissue-specific information for prioritizing risk genes. Bioinformatics. 2016;32(12):1856–64.
Chung D, Yang C, Li C, Gelernter J, Zhao H. GPA: a statistical approach to prioritizing GWAS results by integrating pleiotropy and annotation. PLoS Genet. 2014;10(11): e1004787.
Weissbrod O, Flint J, Rosset S. Estimating SNP-Based Heritability and Genetic Correlation in Case-Control Studies Directly and with Summary Statistics. Am J Hum Genet. 2018;103(1):89–99.
Lu Q, Li B, Ou D, Erlendsdottir M, Powles RL, Jiang T, et al. A Powerful Approach to Estimating Annotation-Stratified Genetic Covariance via GWAS Summary Statistics. Am J Hum Genet. 2017;101(6):939–64.
Zhang Y, Lu Q, Ye Y, Huang K, Liu W, Wu Y, et al. SUPERGNOVA: local genetic correlation analysis reveals heterogeneous etiologic sharing of complex traits. Genome Biol. 2021;22(1):262.
Werme J, van der Sluis S, Posthuma D, de Leeuw CA. An integrated framework for local genetic correlation analysis. Nat Genet. 2022;54(3):274–82.
Ning Z, Pawitan Y, Shen X. High-definition likelihood inference of genetic correlations across human complex traits. Nat Genet. 2020;52(8):859–64.
Brown BC, Ye CJ, Price AL, Zaitlen N. Transethnic Genetic-Correlation Estimates from Summary Statistics. Am J Hum Genet. 2016;99(1):76–88.
Gao B, Yang C, Liu J, Zhou X. Accurate genetic and environmental covariance estimation with composite likelihood in genome-wide association studies. PLoS Genet. 2021;17(1): e1009293.
Zheng J, Richardson TG, Millard LAC, Hemani G, Elsworth BL, Raistrick CA, et al. PhenoSpD: an integrated toolkit for phenotypic correlation estimation and multiple testing correction using GWAS summary statistics. Gigascience. 2018;7(8).
Ming J, Wang T, Yang C. LPM: a latent probit model to characterize the relationship among complex traits using summary statistics from multiple GWASs and functional annotations. Bioinformatics. 2020;36(8):2506–14.
Peyrot WJ, Price AL. Identifying loci with different allele frequencies among cases of eight psychiatric disorders using CC-GWAS. Nat Genet. 2021;53(4):445–54.
Mancuso N, Shi H, Goddard P, Kichaev G, Gusev A, Pasaniuc B. Integrating Gene Expression with Summary Association Statistics to Identify Genes Associated with 30 Complex Traits. Am J Hum Genet. 2017;100(3):473–87.
Guo H, Li JJ, Lu Q, Hou L. Detecting local genetic correlations with scan statistics. Nat Commun. 2021;12(1):2033.
Wu Y, Zhong X, Lin Y, Zhao Z, Chen J, Zheng B, et al. Estimating genetic nurture with summary statistics of multigenerational genome-wide association studies. Proc Natl Acad Sci U S A. 2021;118(25).
Smith GD, Ebrahim S. Mendelian randomization: prospects, potentials, and limitations. Int J Epidemiol. 2004;33(1):30–42.
Didelez V, Sheehan N. Mendelian randomization as an instrumental variable approach to causal inference. Stat Methods Med Res. 2007;16(4):309–30.
Thompson JR, Minelli C, Abrams KR, Tobin MD, Riley RD. Meta-analysis of genetic studies using Mendelian randomization–a multivariate approach. Stat Med. 2005;24(14):2241–54.
Bowden J, Holmes MV. Meta-analysis and Mendelian randomization: A review. Res Synth Methods. 2019;10(4):486–96.
Kraft P, Chen H, Lindström S. The Use Of Genetic Correlation And Mendelian Randomization Studies To Increase Our Understanding of Relationships Between Complex Traits. Curr Epidemiol Rep. 2020;7(2):104–12.
Bowden J, Davey Smith G, Haycock PC, Burgess S. Consistent Estimation in Mendelian Randomization with Some Invalid Instruments Using a Weighted Median Estimator. Genet Epidemiol. 2016;40(4):304–14.
Hemani G, Zheng J, Elsworth B, Wade KH, Haberland V, Baird D, et al. The MR-Base platform supports systematic causal inference across the human phenome. Elife. 2018;7.
Burgess S, Foley CN, Allara E, Staley JR, Howson JMM. A robust and efficient method for Mendelian randomization with hundreds of genetic variants. Nat Commun. 2020;11(1):376.
Zhao J, Ming J, Hu X, Chen G, Liu J, Yang C. Bayesian weighted Mendelian randomization for causal inference based on summary statistics. Bioinformatics. 2020;36(5):1501–8.
Xu S, Wang P, Fung WK, Liu Z. A novel penalized inverse-variance weighted estimator for Mendelian randomization with applications to COVID-19 outcomes. Biometrics. 2023;79(3):2184–95.
Qi G, Chatterjee N. Mendelian randomization analysis using mixture models for robust and efficient estimation of causal effects. Nat Commun. 2019;10(1):1941.
Xue H, Shen X, Pan W. Constrained maximum likelihood-based Mendelian randomization robust to both correlated and uncorrelated pleiotropic effects. Am J Hum Genet. 2021;108(7):1251–69.
Cheng Q, Yang Y, Shi X, Yeung KF, Yang C, Peng H, et al. MR-LDP: a two-sample Mendelian randomization for GWAS summary statistics accounting for linkage disequilibrium and horizontal pleiotropy. NAR Genom Bioinform. 2020;2(2):lqaa028.
Cheng Q, Qiu T, Chai X, Sun B, Xia Y, Shi X, et al. MR-Corr2: a two-sample Mendelian randomization method that accounts for correlated horizontal pleiotropy using correlated instrumental variants. Bioinformatics. 2022;38(2):303–10.
Verbanck M, Chen CY, Neale B, Do R. Detection of widespread horizontal pleiotropy in causal relationships inferred from Mendelian randomization between complex traits and diseases. Nat Genet. 2018;50(5):693–8.
Zhu X, Li X, Xu R, Wang T. An iterative approach to detect pleiotropy and perform Mendelian Randomization analysis using GWAS summary statistics. Bioinformatics. 2021;37(10):1390–400.
Hu X, Zhao J, Lin Z, Wang Y, Peng H, Zhao H, et al. Mendelian randomization for causal inference accounting for pleiotropy and sample structure using genome-wide summary statistics. Proc Natl Acad Sci U S A. 2022;119(28): e2106858119.
Mounier N, Kutalik Z. Bias correction for inverse variance weighting Mendelian randomization. Genet Epidemiol. 2023;47(4):314–31.
Cheng Q, Zhang X, Chen LS, Liu J. Mendelian randomization accounting for complex correlated horizontal pleiotropy while elucidating shared genetic etiology. Nat Commun. 2022;13(1):6490.
Ding M. A Two-stage Linear Mixed Model (TS-LMM) for Summary-data-based Multivariable Mendelian Randomization. medRxiv. 2023.
O’Connor LJ, Price AL. Distinguishing genetic correlation from causation across 52 diseases and complex traits. Nat Genet. 2018;50(12):1728–34.
Wang L, Gao B, Fan Y, Xue F, Zhou X. Mendelian randomization under the omnigenic architecture. Brief Bioinform. 2021;22(6).
Gkatzionis A, Burgess S, Conti DV, Newcombe PJ. Bayesian variable selection with a pleiotropic loss function in Mendelian randomization. Stat Med. 2021;40(23):5025–45.
Xue H, Pan W. Inferring causal direction between two traits in the presence of horizontal pleiotropy with GWAS summary data. PLoS Genet. 2020;16(11): e1009105.
Xue H, Pan W. Robust inference of bi-directional causal relationships in presence of correlated pleiotropy with GWAS summary data. PLoS Genet. 2022;18(5): e1010205.
Liu Z, Qin Y, Wu T, Tubbs JD, Baum L, Mak TSH, et al. Reciprocal causation mixture model for robust Mendelian randomization analysis using genome-scale summary data. Nat Commun. 2023;14(1):1131.
Darrous L, Mounier N, Kutalik Z. Simultaneous estimation of bi-directional causal effects and heritable confounding from GWAS summary statistics. Nat Commun. 2021;12(1):7274.
Zuber V, Lewin A, Levin MG, Haglund A, Ben-Aicha S, Emanueli C, et al. Multi-response Mendelian randomization: Identification of shared and distinct exposures for multimorbidity and multiple related disease outcomes. Am J Hum Genet. 2023;110(7):1177–99.
Sanderson E, Davey Smith G, Windmeijer F, Bowden J. An examination of multivariable Mendelian randomization in the single-sample and two-sample summary data settings. Int J Epidemiol. 2019;48(3):713–27.
Lorincz-Comi N, Yang Y, Li G, Zhu X. MRBEE: A novel bias-corrected multivariable Mendelian Randomization method. bioRxiv. 2023.
Lin Z, Xue H, Pan W. Robust multivariable Mendelian randomization based on constrained maximum likelihood. Am J Hum Genet. 2023;110(4):592–605.
Jin C, Lee B, Shen L, Long Q. Integrating multi-omics summary data using a Mendelian randomization framework. Brief Bioinform. 2022;23(6).
Zuber V, Colijn JM, Klaver C, Burgess S. Selecting likely causal risk factors from high-throughput experiments using multivariable Mendelian randomization. Nat Commun. 2020;11(1):29.
Jiang L, Xu S, Mancuso N, Newcombe PJ, Conti DV. A Hierarchical Approach Using Marginal Summary Statistics for Multiple Intermediates in a Mendelian Randomization or Transcriptome Analysis. Am J Epidemiol. 2021;190(6):1148–58.
Zhao Q, Chen Y, Wang J, Small DS. Powerful three-sample genome-wide design and robust statistical inference in summary-data Mendelian randomization. Int J Epidemiol. 2019;48(5):1478–92.
Fan Q, Zhang F, Wang W, Xu J, Hao J, He A, et al. GWAS summary-based pathway analysis correcting for the genetic confounding impact of environmental exposures. Brief Bioinform. 2018;19(5):725–30.
Mai J, Lu M, Gao Q, Zeng J, Xiao J. Transcriptome-wide association studies: recent advances in methods, applications and available databases. Commun Biol. 2023;6(1):899.
Zhu Z, Zhang F, Hu H, Bakshi A, Robinson MR, Powell JE, et al. Integration of summary data from GWAS and eQTL studies predicts complex trait gene targets. Nat Genet. 2016;48(5):481–7.
Yuan Z, Zhu H, Zeng P, Yang S, Sun S, Yang C, et al. Testing and controlling for horizontal pleiotropy with probabilistic Mendelian randomization in transcriptome-wide association studies. Nat Commun. 2020;11(1):3861.
Xue H, Shen X, Pan W. Causal Inference in Transcriptome-Wide Association Studies with Invalid Instruments and GWAS Summary Data. J Am Stat Assoc. 2023;118(543):1525–37.
Gusev A, Ko A, Shi H, Bhatia G, Chung W, Penninx BW, et al. Integrative approaches for large-scale transcriptome-wide association studies. Nat Genet. 2016;48(3):245–52.
Barbeira AN, Dickinson SP, Bonazzola R, Zheng J, Wheeler HE, Torres JM, et al. Exploring the phenotypic consequences of tissue specific gene expression variation inferred from GWAS summary statistics. Nat Commun. 2018;9(1):1825.
Xu Z, Wu C, Wei P, Pan W. A Powerful Framework for Integrating eQTL and GWAS Summary Data. Genetics. 2017;207(3):893–902.
Barfield R, Feng H, Gusev A, Wu L, Zheng W, Pasaniuc B, et al. Transcriptome-wide association studies accounting for colocalization using Egger regression. Genet Epidemiol. 2018;42(5):418–33.
Rojo C, Zhang Q, Keleş S. iFunMed: Integrative functional mediation analysis of GWAS and eQTL studies. Genet Epidemiol. 2019;43(7):742–60.
Dong X, Su YR, Barfield R, Bien SA, He Q, Harrison TA, et al. A general framework for functionally informed set-based analysis: Application to a large-scale colorectal cancer study. PLoS Genet. 2020;16(8): e1008947.
Zhang Y, Quick C, Yu K, Barbeira A, Luca F, Pique-Regi R, et al. PTWAS: investigating tissue-relevant causal molecular mechanisms of complex traits using probabilistic TWAS analysis. Genome Biol. 2020;21(1):232.
Yang Y, Yeung KF, Liu J. CoMM-S(4): A Collaborative Mixed Model Using Summary-Level eQTL and GWAS Datasets in Transcriptome-Wide Association Studies. Front Genet. 2021;12: 704538.
Shi X, Chai X, Yang Y, Cheng Q, Jiao Y, Huang J, et al. A tissue-specific collaborative mixed model for jointly analyzing multiple tissues in transcriptome-wide association studies. bioRxiv. 2019:789396.
Park Y, Sarkar A, Bhutani K, Kellis M. Multi-tissue polygenic models for transcriptome-wide association studies. bioRxiv. 2017:107623.
Feng H, Mancuso N, Gusev A, Majumdar A, Major M, Pasaniuc B, et al. Leveraging expression from multiple tissues using sparse canonical correlation analysis and aggregate tests improves the power of transcriptome-wide association studies. PLoS Genet. 2021;17(4): e1008973.
Hu Y, Li M, Lu Q, Weng H, Wang J, Zekavat SM, et al. A statistical framework for cross-tissue transcriptome-wide association analysis. Nat Genet. 2019;51(3):568–76.
Gleason KJ, Yang F, Pierce BL, He X, Chen LS. Primo: integration of multiple GWAS and omics QTL summary statistics for elucidation of molecular mechanisms of trait-associated SNPs and detection of pleiotropy in complex traits. Genome Biol. 2020;21(1):236.
Wu Y, Qi T, Wray NR, Visscher PM, Zeng J, Yang J. Joint analysis of GWAS and multi-omics QTL summary statistics reveals a large fraction of GWAS signals shared with molecular phenotypes. Cell Genom. 2023;3(8): 100344.
Zhang Z, Bae YE, Bradley JR, Wu L, Wu C. SUMMIT: An integrative approach for better transcriptomic data imputation improves causal gene identification. Nat Commun. 2022;13(1):6336.
Zeng P, Dai J, Jin S, Zhou X. Aggregating multiple expression prediction models improves the power of transcriptome-wide association studies. Hum Mol Genet. 2021;30(10):939–51.
Luningham JM, Chen J, Tang S, De Jager PL, Bennett DA, Buchman AS, et al. Bayesian Genome-wide TWAS Method to Leverage both cis- and trans-eQTL Information through Summary Statistics. Am J Hum Genet. 2020;107(4):714–26.
Dutta D, He Y, Saha A, Arvanitis M, Battle A, Chatterjee N. Aggregative trans-eQTL analysis detects trait-specific target gene sets in whole blood. Nat Commun. 2022;13(1):4323.
Nagpal S, Meng X, Epstein MP, Tsoi LC, Patrick M, Gibson G, et al. TIGAR: An Improved Bayesian Tool for Transcriptomic Data Imputation Enhances Gene Mapping of Complex Traits. Am J Hum Genet. 2019;105(2):258–66.
Chatzinakos C, Georgiadis F, Lee D, Cai N, Vladimirov VI, Docherty A, et al. TWAS pathway method greatly enhances the number of leads for uncovering the molecular underpinnings of psychiatric disorders. Am J Med Genet B Neuropsychiatr Genet. 2020;183(8):454–63.
Mancuso N, Freund MK, Johnson R, Shi H, Kichaev G, Gusev A, et al. Probabilistic fine-mapping of transcriptome-wide association studies. Nat Genet. 2019;51(4):675–82.
Zhu H, Zhou X. Transcriptome-wide association studies: a view from Mendelian randomization. Quant Biol. 2021;9(2):107–21.
Zhu A, Matoba N, Wilson EP, Tapia AL, Li Y, Ibrahim JG, et al. MRLocus: Identifying causal genes mediating a trait through Bayesian estimation of allelic heterogeneity. PLoS Genet. 2021;17(4): e1009455.
Porcu E, Rüeger S, Lepik K, Santoni FA, Reymond A, Kutalik Z. Mendelian randomization integrating GWAS and eQTL data reveals genetic determinants of complex and clinical traits. Nat Commun. 2019;10(1):3300.
Gleason KJ, Yang F, Chen LS. A robust two-sample transcriptome-wide Mendelian randomization method integrating GWAS with multi-tissue eQTL summary statistics. Genet Epidemiol. 2021;45(4):353–71.
Al-Barghouthi BM, Rosenow WT, Du KP, Heo J, Maynard R, Mesner L, et al. Transcriptome-wide association study and eQTL colocalization identify potentially causal genes responsible for human bone mineral density GWAS associations. Elife. 2022;11.
Plagnol V, Smyth DJ, Todd JA, Clayton DG. Statistical independence of the colocalized association signals for type 1 diabetes and RPS26 gene expression on chromosome 12q13. Biostatistics. 2009;10(2):327–34.
Wallace C. A more accurate method for colocalisation analysis allowing for multiple causal variants. PLoS Genet. 2021;17(9): e1009440.
Giambartolomei C, Zhenli Liu J, Zhang W, Hauberg M, Shi H, Boocock J, et al. A Bayesian framework for multiple trait colocalization from summary association statistics. Bioinformatics. 2018;34(15):2538–45.
Foley CN, Staley JR, Breen PG, Sun BB, Kirk PDW, Burgess S, et al. A fast and efficient colocalization algorithm for identifying shared genetic risk factors across multiple traits. Nat Commun. 2021;12(1):764.
Wang F, Panjwani N, Wang C, Sun L, Strug LJ. A flexible summary statistics-based colocalization method with application to the mucin cystic fibrosis lung disease modifier locus. Am J Hum Genet. 2022;109(2):253–69.
Liu J, Wan X, Wang C, Yang C, Zhou X, Yang C. LLR: a latent low-rank approach to colocalizing genetic risk variants in multiple GWAS. Bioinformatics. 2017;33(24):3878–86.
King EA, Dunbar F, Davis JW, Degner JF. Estimating colocalization probability from limited summary statistics. BMC Bioinformatics. 2021;22(1):254.
Kuksa PP, Lee CY, Amlie-Wolf A, Gangadharan P, Mlynarski EE, Chou YF, et al. SparkINFERNO: a scalable high-throughput pipeline for inferring molecular mechanisms of non-coding genetic variants. Bioinformatics. 2020;36(12):3879–81.
Zheng J, Haberland V, Baird D, Walker V, Haycock PC, Hurle MR, et al. Phenome-wide Mendelian randomization mapping the influence of the plasma proteome on complex diseases. Nat Genet. 2020;52(10):1122–31.
Chen BY, Bone WP, Lorenz K, Levin M, Ritchie MD, Voight BF. ColocQuiaL: a QTL-GWAS colocalization pipeline. Bioinformatics. 2022;38(18):4409–11.
Hormozdiari F, van de Bunt M, Segrè AV, Li X, Joo JWJ, Bilow M, et al. Colocalization of GWAS and eQTL Signals Detects Target Genes. Am J Hum Genet. 2016;99(6):1245–60.
Ji Y, Wei Q, Chen R, Wang Q, Tao R, Li B. Integration of multidimensional splicing data and GWAS summary statistics for risk gene discovery. PLoS Genet. 2022;18(6): e1009814.
Zhang W, Lu T, Sladek R, Li Y, Najafabadi HS, Dupuis J. SharePro: an accurate and efficient genetic colocalization method accounting for multiple causal signals. bioRxiv. 2023:2023.07.24.550431.
Shi H, Burch KS, Johnson R, Freund MK, Kichaev G, Mancuso N, et al. Localizing Components of Shared Transethnic Genetic Architecture of Complex Traits from GWAS Summary Data. Am J Hum Genet. 2020;106(6):805–17.
He X, Fuller CK, Song Y, Meng Q, Zhang B, Yang X, et al. Sherlock: detecting gene-disease associations by matching patterns of expression QTL and GWAS. Am J Hum Genet. 2013;92(5):667–80.
Panjwani N, Wang F, Mastromatteo S, Bao A, Wang C, He G, et al. LocusFocus: Web-based colocalization for the annotation and functional follow-up of GWAS. PLoS Comput Biol. 2020;16(10): e1008336.
Zhang T, Klein A, Sang J, Choi J, Brown KM. ezQTL: A Web Platform for Interactive Visualization and Colocalization of QTLs and GWAS Loci. Genomics Proteomics Bioinformatics. 2022;20(3):541–8.
Lamparter D, Bhatnagar R, Hebestreit K, Belgard TG, Zhang A, Hanson-Smith V. A framework for integrating directed and undirected annotations to build explanatory models of cis-eQTL data. PLoS Comput Biol. 2020;16(6): e1007770.
Visscher PM, Wray NR, Zhang Q, Sklar P, McCarthy MI, Brown MA, et al. 10 Years of GWAS Discovery: Biology, Function, and Translation. Am J Hum Genet. 2017;101(1):5–22.
Schultheiss SJ, Münch MC, Andreeva GD, Rätsch G. Persistence and availability of Web services in computational biology. PLoS ONE. 2011;6(9): e24914.
Veretnik S, Fink JL, Bourne PE. Computational biology resources lack persistence and usability. PLoS Comput Biol. 2008;4(7): e1000136.
Wren JD. 404 not found: the stability and persistence of URLs published in MEDLINE. Bioinformatics. 2004;20(5):668–72.
Kern F, Fehlmann T, Keller A. On the lifetime of bioinformatics web services. Nucleic Acids Res. 2020;48(22):12523–33.
Taschuk M, Wilson G. Ten simple rules for making research software more robust. PLoS Comput Biol. 2017;13(4): e1005412.
Brazas MD, Yim D, Yeung W, Ouellette BF. A decade of Web Server updates at the Bioinformatics Links Directory: 2003–2012. Nucleic Acids Res. 2012;40(Web Server issue):W3-w12.
Chakiachvili M, Milanesi S, Arigon Chifolleau AM, Lefort V. WAVES: a web application for versatile enhanced bioinformatic services. Bioinformatics. 2019;35(1):140–2.
Daniluk P, Wilczyński B, Lesyng B. WeBIAS: a web server for publishing bioinformatics applications. BMC Res Notes. 2015;8:628.
Jia L, Yao W, Jiang Y, Li Y, Wang Z, Li H, et al. Development of interactive biological web applications with R/Shiny. Brief Bioinform. 2022;23(1).
Joppich M, Zimmer R. From command-line bioinformatics to bioGUI PeerJ. 2019;7: e8111.
Kadri S, Sboner A, Sigaras A, Roy S. Containers in Bioinformatics: Applications, Practical Considerations, and Best Practices in Molecular Pathology. J Mol Diagn. 2022;24(5):442–54.
Williams CL, Sica JC, Killen RT, Balis UG. The growing need for microservices in bioinformatics. J Pathol Inform. 2016;7:45.
Boettiger C. An introduction to Docker for reproducible research. ACM SIGOPS Operating Systems Review. 2015;49(1):71–9.
Gomes J, Bagnaschi E, Campos I, David M, Alves L, Martins J, et al. Enabling rootless Linux Containers in multi-user environments: the udocker tool. Comput Phys Commun. 2018;232:84–97.
Goh KI, Cusick ME, Valle D, Childs B, Vidal M, Barabási AL. The human disease network. Proc Natl Acad Sci U S A. 2007;104(21):8685–90.
Kontou PI, Pavlopoulou A, Dimou NL, Pavlopoulos GA, Bagos PG. Network analysis of genes and their association with diseases. Gene. 2016;590(1):68–78.
Corrigendum to: Bipartite graphs in systems biology and medicine: a survey of methods and applications. Gigascience. 2020;9(1).
Pavlopoulos GA, Secrier M, Moschopoulos CN, Soldatos TG, Kossida S, Aerts J, et al. Using graph theory to analyze biological networks. BioData Min. 2011;4:10.
Download references
Acknowledgements
The authors would like to thank the anonymous reviewers whose comments and constructive criticism helped in improving the quality of the manuscript.
This work is funded by the project “Bridging big omic, genetic and medical data for Precision Medicine implementation in Greece” (TAEDR-0539180) which is carried out within the framework of the National Recovery and Resilience Plan Greece 2.0, funded by the European Union –NextGenerationEU.
Author information
Authors and affiliations.
Department of Mathematics, University of Thessaly, 35131, Lamia, Greece
Panagiota I. Kontou
Department of Computer Science and Biomedical Informatics, University of Thessaly, 35131, Lamia, Greece
Pantelis G. Bagos
You can also search for this author in PubMed Google Scholar
Contributions
PK: Investigation, Methodology, Data Curation, Visualization. PB: Conceptualization, Supervision, Investigation, Methodology, Data Curation, Visualization. PK and PB wrote parts of the manuscript and have read and approved the final manuscript.
Corresponding author
Correspondence to Pantelis G. Bagos .
Ethics declarations
Ethics approval and consent to participate.
Not applicable.
Consent for publication
Competing interests.
The authors declare that they have no competing interests.
Additional information
Publisher’s note.
Springer Nature remains neutral with regard to jurisdictional claims in published maps and institutional affiliations.
Supplementary Information
Supplementary material 1., supplementary material 2., rights and permissions.
Open Access This article is licensed under a Creative Commons Attribution-NonCommercial-NoDerivatives 4.0 International License, which permits any non-commercial use, sharing, distribution and reproduction in any medium or format, as long as you give appropriate credit to the original author(s) and the source, provide a link to the Creative Commons licence, and indicate if you modified the licensed material. You do not have permission under this licence to share adapted material derived from this article or parts of it. The images or other third party material in this article are included in the article’s Creative Commons licence, unless indicated otherwise in a credit line to the material. If material is not included in the article’s Creative Commons licence and your intended use is not permitted by statutory regulation or exceeds the permitted use, you will need to obtain permission directly from the copyright holder. To view a copy of this licence, visit http://creativecommons.org/licenses/by-nc-nd/4.0/ .
Reprints and permissions
About this article
Cite this article.
Kontou, P.I., Bagos, P.G. The goldmine of GWAS summary statistics: a systematic review of methods and tools. BioData Mining 17 , 31 (2024). https://doi.org/10.1186/s13040-024-00385-x
Download citation
Received : 09 February 2024
Accepted : 27 August 2024
Published : 05 September 2024
DOI : https://doi.org/10.1186/s13040-024-00385-x
Share this article
Anyone you share the following link with will be able to read this content:
Sorry, a shareable link is not currently available for this article.
Provided by the Springer Nature SharedIt content-sharing initiative
- Summary statistics
- systematic review
BioData Mining
ISSN: 1756-0381
- General enquiries: [email protected]
Information
- Author Services
Initiatives
You are accessing a machine-readable page. In order to be human-readable, please install an RSS reader.
All articles published by MDPI are made immediately available worldwide under an open access license. No special permission is required to reuse all or part of the article published by MDPI, including figures and tables. For articles published under an open access Creative Common CC BY license, any part of the article may be reused without permission provided that the original article is clearly cited. For more information, please refer to https://www.mdpi.com/openaccess .
Feature papers represent the most advanced research with significant potential for high impact in the field. A Feature Paper should be a substantial original Article that involves several techniques or approaches, provides an outlook for future research directions and describes possible research applications.
Feature papers are submitted upon individual invitation or recommendation by the scientific editors and must receive positive feedback from the reviewers.
Editor’s Choice articles are based on recommendations by the scientific editors of MDPI journals from around the world. Editors select a small number of articles recently published in the journal that they believe will be particularly interesting to readers, or important in the respective research area. The aim is to provide a snapshot of some of the most exciting work published in the various research areas of the journal.
Original Submission Date Received: .
- Active Journals
- Find a Journal
- Proceedings Series
- For Authors
- For Reviewers
- For Editors
- For Librarians
- For Publishers
- For Societies
- For Conference Organizers
- Open Access Policy
- Institutional Open Access Program
- Special Issues Guidelines
- Editorial Process
- Research and Publication Ethics
- Article Processing Charges
- Testimonials
- Preprints.org
- SciProfiles
- Encyclopedia
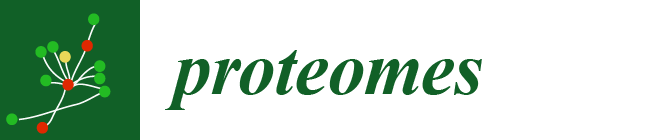
Article Menu
- Subscribe SciFeed
- Recommended Articles
- Google Scholar
- on Google Scholar
- Table of Contents
Find support for a specific problem in the support section of our website.
Please let us know what you think of our products and services.
Visit our dedicated information section to learn more about MDPI.
JSmol Viewer
Transforming clinical research: the power of high-throughput omics integration.

Graphical Abstract
1. Introduction
2. comprehensive frameworks for high-throughput pipeline omics integration, 2.1. key components and technologies.
Aspect | Description | Examples | References |
---|---|---|---|
Concept | High-throughput omics technologies encompass genomics, transcriptomics, proteomics and metabolomics, enabling comprehensive analysis of molecular components. | Genomics: DNA sequencing, Transcriptomics: RNA sequencing, Proteomics: Mass spectrometry, Metabolomics: NMR spectroscopy. | [ ] |
Need | The complexity and heterogeneity of biological systems necessitate advanced methods to capture molecular interactions and dynamics. | Multifactorial diseases like cancer require comprehensive data to understand gene–protein–metabolite interactions. | [ ] |
Benefits | Provides detailed views of biological systems, identifies novel biomarkers and facilitates personalized medicine. | Improves disease understanding, targeted therapeutic strategies and customized treatment plans. | [ ] |
Challenges | Managing and integrating vast, heterogeneous datasets and developing accurate computational models. | Data heterogeneity, computational resource requirements and the need for advanced bioinformatics tools. | [ ] |
2.2. Challenges and Opportunities
3. case studies and applications, 3.1. automatic text mining in biomedical research, 3.2. genomic analysis and biomarkers, 3.3. role of the genome-wide association studies (gwas) catalog.
Disease Area | Genomic Analysis Techniques | Biomarker Identification | Advantages | Limitations | References |
---|---|---|---|---|---|
Cancer | Whole Genome Sequencing (WGS), Whole Exome Sequencing (WES), Targeted Sequencing | Identification of cancer-specific mutations and genes, prognostic and predictive biomarkers for therapy response | Enables personalized treatment plans; early detection and monitoring; comprehensive mutation analysis | High cost; large data volume requires advanced bioinformatics; interpretation of variants can be challenging | [ ] |
Cardiovascular Diseases | Genome-Wide Association Studies (GWAS), WGS, WES | Discovery of genetic variants associated with heart disease, biomarkers for risk assessments and therapeutic targets | Identification of high-risk individuals; potential for new therapeutic targets | Complex interplay of genetics and environment; data integration challenges | [ ] |
Neurodegenerative Diseases | WGS, WES, GWAS, Epigenetic Profiling | Identification of genetic mutations linked to Alzheimer’s, Parkinson’s, and other neurodegenerative diseases | Early diagnosis; understanding disease mechanisms; potential for targeted therapies | Genetic heterogeneity; need for large cohort studies | [ ] |
Diabetes | GWAS, WGS, WES, Transcriptomics | Genetic markers associated with insulin resistance, beta-cell function and complications of diabetes | Improved prediction of disease onset; potential for personalized treatment strategies | Complex genetic architecture; influence of lifestyle factors | [ ] |
Autoimmune Diseases | GWAS, WGS, Single Nucleotide Polymorphism (SNP) Analysis | Biomarkers for disease susceptibility, progression, and response to treatment in conditions like rheumatoid arthritis | Identification of susceptibility genes; tailored therapeutic interventions | Genetic and environmental interactions; variable disease phenotypes | [ ] |
Infectious Diseases | WGS, Pathogen Genomics, Metagenomics | Pathogen-specific genetic markers, host genetic factors influencing susceptibility and treatment response | Rapid pathogen identification; understanding pathogen-host interactions | High cost of sequencing; data complexity; need for rapid analysis turnaround | [ ] |
Rare Genetic Disorders | WGS, WES, Copy Number Variation (CNV) Analysis | Discovery of causative mutations and genes, development of diagnostic tests and personalized treatment approaches | Accurate diagnosis; potential for gene therapy; detailed understanding of rare diseases | High cost; difficulty in obtaining sufficient sample sizes; ethical considerations | [ ] |
Respiratory Diseases | WGS, Transcriptomics, Epigenomics | Identification of genetic variants linked to asthma, COPD and other respiratory condition biomarkers for disease management | Early detection; better disease management; identification of environmental and genetic interactions | Heterogeneous disease mechanisms; influence of environmental factors; data interpretation challenges | [ ] |
3.4. Proteomic Analysis in High-Throughput Pipelines
4. integration and interoperability of omics data.
Omics Type | Integration Approach | Interoperability Tools and Standards | Benefits | Limitations |
---|---|---|---|---|
Genomics | Cross-referencing genetic variants | VCF (Variant Call Format), dbSNP [ ], Ensembl [ ] | Identifies genetic variations and their effects | High data volume; interpretation of variants; privacy issues |
Transcriptomics | Aligning RNA-seq data with genome | FASTQ, SAM/BAM, GTF/GFF, GEO ( , accessed on 1 June 2024) | Reveals gene expression patterns and alternative splicing | Data complexity; variability between samples |
Proteomics | Correlating protein levels with gene expression | mzML [ ], PRIDE [ ], UniProt [ ] | Understands protein abundance and functional roles | Sensitivity to sample preparation; high technical variability |
Metabolomics | Linking metabolites to metabolic pathways | mzTab, HMDB [ ], KEGG [ ] | Provides insights into cellular metabolism and pathway activities | Complex data integration; diverse chemical properties |
Epigenomics | Mapping epigenetic modifications | BED, WIG, GEO, ENCODE ( , accessed on 1 June 2024) | Studies DNA methylation, histone modifications and chromatin accessibility | High data complexity; dynamic nature of epigenetic changes |
Lipidomics | Profiling lipid species | LIPID MAPS [ ], mzXML [ ] | Explores lipid composition and its role in cell biology | Heterogeneity of lipids; difficulty in quantifying low-abundance lipids |
Glycomics | Characterizing glycan structures | GlyTouCan [ ], UniCarb-DB [ ] | Analyzes glycan functions and interactions | Structural complexity of glycans; limited analytical standards |
Microbiomics | Integrating microbiome with host data | QIIME [ ], MG-RAST [ ] | Examines microbial communities and their impact on host health | Variability in sample preparation; complex data interpretation |
Phenomics | Associating phenotypic traits with molecular data | PhenX [ ], PheWAS [ ], dbGaP [ ] | Identifies molecular markers linked to phenotypes | High variability in phenotype data; integration with genomic data |
Pharmacogenomics | Linking drug response to genetic profiles | PharmGKB [ ], CPIC | Personalizes medicine based on genetic profiles | Ethical concerns; variability in drug response among individuals |
5. Future Directions
6. conclusions, institutional review board statement, informed consent statement, data availability statement, conflicts of interest.
- Agamah, F.E.; Bayjanov, J.R.; Niehues, A.; Njoku, K.F.; Skelton, M.; Mazandu, G.K.; Ederveen, T.H.A.; Mulder, N.; Chimusa, E.R.; t Hoen, P.A.C. Computational approaches for network-based integrative multi-omics analysis. Front. Mol. Biosci. 2022 , 9 , 967205. [ Google Scholar ] [ CrossRef ] [ PubMed ]
- Li, Y.; Mansmann, U.; Du, S.; Hornung, R. Benchmark study of feature selection strategies for multi-omics data. BMC Bioinform. 2022 , 23 , 412. [ Google Scholar ] [ CrossRef ]
- Argelaguet, R.; Velten, B.; Arnol, D.; Dietrich, S.; Zenz, T.; Marioni, J.C.; Buettner, F.; Huber, W.; Stegle, O. Multi-Omics Factor Analysis-a framework for unsupervised integration of multi-omics data sets. Mol. Syst. Biol. 2018 , 14 , e8124. [ Google Scholar ] [ CrossRef ] [ PubMed ]
- The Galaxy Community. The Galaxy platform for accessible, reproducible, and collaborative data analyses: 2024 update. Nucleic Acids Res. 2024 , 52 , W83–W94. [ Google Scholar ] [ CrossRef ] [ PubMed ]
- Zhou, G.; Pang, Z.; Lu, Y.; Ewald, J.; Xia, J. OmicsNet 2.0: A web-based platform for multi-omics integration and network visual analytics. Nucleic Acids Res. 2022 , 50 , W527–W533. [ Google Scholar ] [ CrossRef ] [ PubMed ]
- Zhou, G.; Soufan, O.; Ewald, J.; Hancock, R.E.W.; Basu, N.; Xia, J. NetworkAnalyst 3.0: A visual analytics platform for comprehensive gene expression profiling and meta-analysis. Nucleic Acids Res. 2019 , 47 , W234–W241. [ Google Scholar ] [ CrossRef ]
- Dai, X.; Shen, L. Advances and Trends in Omics Technology Development. Front. Med. 2022 , 9 , 911861. [ Google Scholar ] [ CrossRef ]
- Mukherjee, A.; Abraham, S.; Singh, A.; Balaji, S.; Mukunthan, K.S. From Data to Cure: A Comprehensive Exploration of Multi-omics Data Analysis for Targeted Therapies. Mol. Biotechnol. 2024 , 1–21. [ Google Scholar ] [ CrossRef ]
- Fiers, M.W.; van der Burgt, A.; Datema, E.; de Groot, J.C.; van Ham, R.C. High-throughput bioinformatics with the Cyrille2 pipeline system. BMC Bioinform. 2008 , 9 , 96. [ Google Scholar ] [ CrossRef ]
- Tuncbag, N.; Gosline, S.J.; Kedaigle, A.; Soltis, A.R.; Gitter, A.; Fraenkel, E. Network-Based Interpretation of Diverse High-Throughput Datasets through the Omics Integrator Software Package. PLoS Comput. Biol. 2016 , 12 , e1004879. [ Google Scholar ] [ CrossRef ]
- Satam, H.; Joshi, K.; Mangrolia, U.; Waghoo, S.; Zaidi, G.; Rawool, S.; Thakare, R.P.; Banday, S.; Mishra, A.K.; Das, G.; et al. Next-Generation Sequencing Technology: Current Trends and Advancements. Biology 2023 , 12 , 997. [ Google Scholar ] [ CrossRef ]
- Misra, B.B.; Langefeld, C.; Olivier, M.; Cox, L.A. Integrated omics: Tools, advances and future approaches. J. Mol. Endocrinol. 2019 , 62 , R21–R45. [ Google Scholar ] [ CrossRef ]
- Eren, A.M.; Esen, Ö.C.; Quince, C.; Vineis, J.H.; Morrison, H.G.; Sogin, M.L.; Delmont, T.O. Anvi’o: An advanced analysis and visualization platform for ‘omics data. PeerJ 2015 , 3 , e1319. [ Google Scholar ] [ CrossRef ] [ PubMed ]
- Arakawa, K.; Tomita, M. G-language System as a platform for large-scale analysis of high-throughput omics data. J. Pestic. Sci. 2006 , 31 , 282–288. [ Google Scholar ] [ CrossRef ]
- Park, M.; Kim, D.; Moon, K.; Park, T. Integrative Analysis of Multi-Omics Data Based on Blockwise Sparse Principal Components. Int. J. Mol. Sci. 2020 , 21 , 8202. [ Google Scholar ] [ CrossRef ] [ PubMed ]
- Quezada, H.; Guzmán-Ortiz, A.L.; Díaz-Sánchez, H.; Valle-Rios, R.; Aguirre-Hernández, J. Omics-based biomarkers: Current status and potential use in the clinic. Bol. Med. Hosp. Infant. Mex. 2017 , 74 , 219–226. [ Google Scholar ] [ CrossRef ]
- Wekesa, J.S.; Kimwele, M. A review of multi-omics data integration through deep learning approaches for disease diagnosis, prognosis, and treatment. Front. Genet. 2023 , 14 , 1199087. [ Google Scholar ] [ CrossRef ]
- Kaur, P.; Singh, A.; Chana, I. OmicPredict: A framework for omics data prediction using ANOVA-Firefly algorithm for feature selection. Comput. Methods Biomech. Biomed. Engin. 2023 , 1–14. [ Google Scholar ] [ CrossRef ]
- Chen, C.; McGarvey, P.B.; Huang, H.; Wu, C.H. Protein Bioinformatics Infrastructure for the Integration and Analysis of Multiple High-Throughput “omics” Data. Adv. Bioinform. 2010 , 2010 , 423589. [ Google Scholar ] [ CrossRef ]
- Groen, N.; Guvendiren, M.; Rabitz, H.; Welsh, W.J.; Kohn, J.; de Boer, J. Stepping into the omics era: Opportunities and challenges for biomaterials science and engineering. Acta Biomater. 2016 , 34 , 133–142. [ Google Scholar ] [ CrossRef ]
- Pesce, F.; Pathan, S.; Schena, F.P. From-omics to personalized medicine in nephrology: Integration is the key. Nephrol. Dial. Transplant. 2013 , 28 , 24–28. [ Google Scholar ] [ CrossRef ] [ PubMed ]
- Berger, B.; Peng, J.; Singh, M. Computational solutions for omics data. Nat. Rev. Genet. 2013 , 14 , 333–346. [ Google Scholar ] [ CrossRef ] [ PubMed ]
- O’Connor, L.M.; O’Connor, B.A.; Lim, S.B.; Zeng, J.; Lo, C.H. Integrative multi-omics and systems bioinformatics in translational neuroscience: A data mining perspective. J. Pharm. Anal. 2023 , 13 , 836–850. [ Google Scholar ] [ CrossRef ]
- Sun, Y.V.; Hu, Y.J. Integrative Analysis of Multi-omics Data for Discovery and Functional Studies of Complex Human Diseases. Adv. Genet. 2016 , 93 , 147–190. [ Google Scholar ] [ CrossRef ] [ PubMed ]
- Ning, M.; Lo, E.H. Opportunities and challenges in omics. Transl. Stroke Res. 2010 , 1 , 233–237. [ Google Scholar ] [ CrossRef ] [ PubMed ]
- Altelaar, A.F.; Munoz, J.; Heck, A.J. Next-generation proteomics: Towards an integrative view of proteome dynamics. Nat. Rev. Genet. 2013 , 14 , 35–48. [ Google Scholar ] [ CrossRef ]
- Fortino, V.; Scala, G.; Greco, D. Feature set optimization in biomarker discovery from genome-scale data. Bioinformatics 2020 , 36 , 3393–3400. [ Google Scholar ] [ CrossRef ]
- López de Maturana, E.; Alonso, L.; Alarcón, P.; Martín-Antoniano, I.A.; Pineda, S.; Piorno, L.; Calle, M.L.; Malats, N. Challenges in the Integration of Omics and Non-Omics Data. Genes 2019 , 10 , 238. [ Google Scholar ] [ CrossRef ]
- Davis-Turak, J.; Courtney, S.M.; Hazard, E.S.; Glen, W.B., Jr.; da Silveira, W.A.; Wesselman, T.; Harbin, L.P.; Wolf, B.J.; Chung, D.; Hardiman, G. Genomics pipelines and data integration: Challenges and opportunities in the research setting. Expert. Rev. Mol. Diagn. 2017 , 17 , 225–237. [ Google Scholar ] [ CrossRef ]
- Huang, H.; Barker, W.C.; Chen, Y.; Wu, C.H. iProClass: An integrated database of protein family, function and structure information. Nucleic Acids Res. 2003 , 31 , 390–392. [ Google Scholar ] [ CrossRef ]
- Huang, H.; Hu, Z.Z.; Arighi, C.N.; Wu, C.H. Integration of bioinformatics resources for functional analysis of gene expression and proteomic data. Front. Biosci. 2007 , 12 , 5071–5088. [ Google Scholar ] [ CrossRef ]
- Wanichthanarak, K.; Fahrmann, J.F.; Grapov, D. Genomic, Proteomic, and Metabolomic Data Integration Strategies. Biomark. Insights 2015 , 10 , 1–6. [ Google Scholar ] [ CrossRef ] [ PubMed ]
- Bravo, À.; Piñero, J.; Queralt-Rosinach, N.; Rautschka, M.; Furlong, L.I. Extraction of relations between genes and diseases from text and large-scale data analysis: Implications for translational research. BMC Bioinform. 2015 , 16 , 55. [ Google Scholar ] [ CrossRef ]
- Wei, C.H.; Allot, A.; Lai, P.T.; Leaman, R.; Tian, S.; Luo, L.; Jin, Q.; Wang, Z.; Chen, Q.; Lu, Z. PubTator 3.0: An AI-powered literature resource for unlocking biomedical knowledge. Nucleic Acids Res. 2024 , 52 , W540–W546. [ Google Scholar ] [ CrossRef ] [ PubMed ]
- Aronson, A.R.; Lang, F.M. An overview of MetaMap: Historical perspective and recent advances. J. Am. Med. Inf. Assoc. 2010 , 17 , 229–236. [ Google Scholar ] [ CrossRef ]
- Müller, H.M.; Van Auken, K.M.; Li, Y.; Sternberg, P.W. Textpresso Central: A customizable platform for searching, text mining, viewing, and curating biomedical literature. BMC Bioinform. 2018 , 19 , 94. [ Google Scholar ] [ CrossRef ] [ PubMed ]
- Lau, E.; Venkatraman, V.; Thomas, C.T.; Wu, J.C.; Van Eyk, J.E.; Lam, M.P.Y. Identifying High-Priority Proteins Across the Human Diseasome Using Semantic Similarity. J. Proteome Res. 2018 , 17 , 4267–4278. [ Google Scholar ] [ CrossRef ] [ PubMed ]
- van Eck, N.J.; Waltman, L. Visualizing Bibliometric Networks. In Measuring Scholarly Impact: Methods and Practice ; Ding, Y., Rousseau, R., Wolfram, D., Eds.; Springer International Publishing: Cham, Switzerland, 2014; pp. 285–320. [ Google Scholar ]
- Kuntawala, D.H.; Martins, F.; Vitorino, R.; Rebelo, S. Automatic Text-Mining Approach to Identify Molecular Target Candidates Associated with Metabolic Processes for Myotonic Dystrophy Type 1. Int. J. Environ. Res. Public Health 2023 , 20 , 2283. [ Google Scholar ] [ CrossRef ] [ PubMed ]
- Barrett, T.; Suzek, T.O.; Troup, D.B.; Wilhite, S.E.; Ngau, W.-C.; Ledoux, P.; Rudnev, D.; Lash, A.E.; Fujibuchi, W.; Edgar, R. NCBI GEO: Mining millions of expression profiles—Database and tools. Nucleic Acids Res. 2005 , 33 , D562–D566. [ Google Scholar ] [ CrossRef ]
- Lima, T.; Ferreira, R.; Freitas, M.; Henrique, R.; Vitorino, R.; Fardilha, M. Integration of Automatic Text Mining and Genomic and Proteomic Analysis to Unravel Prostate Cancer Biomarkers. J. Proteome Res. 2022 , 21 , 447–458. [ Google Scholar ] [ CrossRef ]
- Ginsburg, G.S.; Haga, S.B. Translating genomic biomarkers into clinically useful diagnostics. Expert. Rev. Mol. Diagn. 2006 , 6 , 179–191. [ Google Scholar ] [ CrossRef ] [ PubMed ]
- Bresalier, R.S.; Grady, W.M.; Markowitz, S.D.; Nielsen, H.J.; Batra, S.K.; Lampe, P.D. Biomarkers for Early Detection of Colorectal Cancer: The Early Detection Research Network, a Framework for Clinical Translation. Cancer Epidemiol. Biomark. Prev. 2020 , 29 , 2431–2440. [ Google Scholar ] [ CrossRef ] [ PubMed ]
- Drouin, A.; Giguère, S.; Déraspe, M.; Marchand, M.; Tyers, M.; Loo, V.G.; Bourgault, A.M.; Laviolette, F.; Corbeil, J. Predictive computational phenotyping and biomarker discovery using reference-free genome comparisons. BMC Genom. 2016 , 17 , 754. [ Google Scholar ] [ CrossRef ]
- Hassan, M.; Awan, F.M.; Naz, A.; deAndrés-Galiana, E.J.; Alvarez, O.; Cernea, A.; Fernández-Brillet, L.; Fernández-Martínez, J.L.; Kloczkowski, A. Innovations in Genomics and Big Data Analytics for Personalized Medicine and Health Care: A Review. Int. J. Mol. Sci. 2022 , 23 , 4645. [ Google Scholar ] [ CrossRef ]
- Fountzilas, E.; Tsimberidou, A.M.; Vo, H.H.; Kurzrock, R. Clinical trial design in the era of precision medicine. Genome Med. 2022 , 14 , 101. [ Google Scholar ] [ CrossRef ] [ PubMed ]
- Simon, R. Genomic biomarkers in predictive medicine: An interim analysis. EMBO Mol. Med. 2011 , 3 , 429–435. [ Google Scholar ] [ CrossRef ] [ PubMed ]
- Bourgey, M.; Dali, R.; Eveleigh, R.; Chen, K.C.; Letourneau, L.; Fillon, J.; Michaud, M.; Caron, M.; Sandoval, J.; Lefebvre, F.; et al. GenPipes: An open-source framework for distributed and scalable genomic analyses. GigaScience 2019 , 8 , giz037. [ Google Scholar ] [ CrossRef ]
- Wratten, L.; Wilm, A.; Göke, J. Reproducible, scalable, and shareable analysis pipelines with bioinformatics workflow managers. Nat. Methods 2021 , 18 , 1161–1168. [ Google Scholar ] [ CrossRef ]
- Ovaska, K.; Lyly, L.; Sahu, B.; Jänne, O.A.; Hautaniemi, S. Genomic region operation kit for flexible processing of deep sequencing data. IEEE/ACM Trans. Comput. Biol. Bioinform. 2013 , 10 , 200–206. [ Google Scholar ] [ CrossRef ]
- Hess, J.F.; Kohl, T.A.; Kotrová, M.; Rönsch, K.; Paprotka, T.; Mohr, V.; Hutzenlaub, T.; Brüggemann, M.; Zengerle, R.; Niemann, S.; et al. Library preparation for next generation sequencing: A review of automation strategies. Biotechnol. Adv. 2020 , 41 , 107537. [ Google Scholar ] [ CrossRef ]
- Rouse, W.B.; Andrews, R.J.; Booher, N.J.; Wang, J.; Woodman, M.E.; Dow, E.R.; Jessop, T.C.; Moss, W.N. Prediction and analysis of functional RNA structures within the integrative genomics viewer. NAR Genom. Bioinform. 2022 , 4 , lqab127. [ Google Scholar ] [ CrossRef ] [ PubMed ]
- Zhang, B.; Wang, J.; Wang, X.; Zhu, J.; Liu, Q.; Shi, Z.; Chambers, M.C.; Zimmerman, L.J.; Shaddox, K.F.; Kim, S.; et al. Proteogenomic characterization of human colon and rectal cancer. Nature 2014 , 513 , 382–387. [ Google Scholar ] [ CrossRef ] [ PubMed ]
- Vasaikar, S.; Huang, C.; Wang, X.; Petyuk, V.A.; Savage, S.R.; Wen, B.; Dou, Y.; Zhang, Y.; Shi, Z.; Arshad, O.A.; et al. Proteogenomic Analysis of Human Colon Cancer Reveals New Therapeutic Opportunities. Cell 2019 , 177 , 1035–1049. [ Google Scholar ] [ CrossRef ]
- Hornbeck, P.V.; Zhang, B.; Murray, B.; Kornhauser, J.M.; Latham, V.; Skrzypek, E. PhosphoSitePlus, 2014: Mutations, PTMs and recalibrations. Nucleic Acids Res. 2015 , 43 , D512–D520. [ Google Scholar ] [ CrossRef ] [ PubMed ]
- Lautenbacher, L.; Samaras, P.; Muller, J.; Grafberger, A.; Shraideh, M.; Rank, J.; Fuchs, S.T.; Schmidt, T.K.; The, M.; Dallago, C.; et al. ProteomicsDB: Toward a FAIR open-source resource for life-science research. Nucleic Acids Res. 2022 , 50 , D1541–D1552. [ Google Scholar ] [ CrossRef ] [ PubMed ]
- Perez-Riverol, Y.; Csordas, A.; Bai, J.; Bernal-Llinares, M.; Hewapathirana, S.; Kundu, D.J.; Inuganti, A.; Griss, J.; Mayer, G.; Eisenacher, M.; et al. The PRIDE database and related tools and resources in 2019: Improving support for quantification data. Nucleic Acids Res. 2018 , 47 , D442–D450. [ Google Scholar ] [ CrossRef ]
- Ma, Y.S.; Huang, T.; Zhong, X.M.; Zhang, H.W.; Cong, X.L.; Xu, H.; Lu, G.X.; Yu, F.; Xue, S.B.; Lv, Z.W.; et al. Proteogenomic characterization and comprehensive integrative genomic analysis of human colorectal cancer liver metastasis. Mol. Cancer 2018 , 17 , 139. [ Google Scholar ] [ CrossRef ]
- Picard, M.; Scott-Boyer, M.P.; Bodein, A.; Périn, O.; Droit, A. Integration strategies of multi-omics data for machine learning analysis. Comput. Struct. Biotechnol. J. 2021 , 19 , 3735–3746. [ Google Scholar ] [ CrossRef ]
- Zhang, X.; Wang, J.; Lu, J.; Su, L.; Wang, C.; Huang, Y.; Zhang, X.; Zhu, X. Robust Prognostic Subtyping of Muscle-Invasive Bladder Cancer Revealed by Deep Learning-Based Multi-Omics Data Integration. Front. Oncol. 2021 , 11 , 689626. [ Google Scholar ] [ CrossRef ]
- Pineda, S.; Real, F.X.; Kogevinas, M.; Carrato, A.; Chanock, S.J.; Malats, N.; Van Steen, K. Integration Analysis of Three Omics Data Using Penalized Regression Methods: An Application to Bladder Cancer. PLoS Genet. 2015 , 11 , e1005689. [ Google Scholar ] [ CrossRef ]
- Adossa, N.; Khan, S.; Rytkönen, K.T.; Elo, L.L. Computational strategies for single-cell multi-omics integration. Comput. Struct. Biotechnol. J. 2021 , 19 , 2588–2596. [ Google Scholar ] [ CrossRef ] [ PubMed ]
- Rodriguez-Tomé, P.; Stoehr, P.; Cameron, G.; Flores, T. The European Bioinformatics Institute (EBI) databases. Nucleic Acids Res. 1996 , 24 , 6–12. [ Google Scholar ] [ CrossRef ] [ PubMed ]
- Sollis, E.; Mosaku, A.; Abid, A.; Buniello, A.; Cerezo, M.; Gil, L.; Groza, T.; Güneş, O.; Hall, P.; Hayhurst, J.; et al. The NHGRI-EBI GWAS Catalog: Knowledgebase and deposition resource. Nucleic Acids Res. 2022 , 51 , D977–D985. [ Google Scholar ] [ CrossRef ]
- Buniello, A.; MacArthur, J.A.L.; Cerezo, M.; Harris, L.W.; Hayhurst, J.; Malangone, C.; McMahon, A.; Morales, J.; Mountjoy, E.; Sollis, E.; et al. The NHGRI-EBI GWAS Catalog of published genome-wide association studies, targeted arrays and summary statistics 2019. Nucleic Acids Res. 2019 , 47 , D1005–D1012. [ Google Scholar ] [ CrossRef ] [ PubMed ]
- Ramos, E.M.; Hoffman, D.; Junkins, H.A.; Maglott, D.; Phan, L.; Sherry, S.T.; Feolo, M.; Hindorff, L.A. Phenotype–Genotype Integrator (PheGenI): Synthesizing genome-wide association study (GWAS) data with existing genomic resources. Eur. J. Hum. Genet. 2014 , 22 , 144–147. [ Google Scholar ] [ CrossRef ] [ PubMed ]
- MacArthur, J.; Bowler, E.; Cerezo, M.; Gil, L.; Hall, P.; Hastings, E.; Junkins, H.; McMahon, A.; Milano, A.; Morales, J.; et al. The new NHGRI-EBI Catalog of published genome-wide association studies (GWAS Catalog). Nucleic Acids Res. 2017 , 45 , D896–D901. [ Google Scholar ] [ CrossRef ]
- Patel, L.; Parker, B.; Yang, D.; Zhang, W. Translational genomics in cancer research: Converting profiles into personalized cancer medicine. Cancer Biol. Med. 2013 , 10 , 214–220. [ Google Scholar ] [ CrossRef ]
- Gliddon, H.D.; Herberg, J.A.; Levin, M.; Kaforou, M. Genome-wide host RNA signatures of infectious diseases: Discovery and clinical translation. Immunology 2018 , 153 , 171–178. [ Google Scholar ] [ CrossRef ]
- Sud, A.; Kinnersley, B.; Houlston, R.S. Genome-wide association studies of cancer: Current insights and future perspectives. Nat. Rev. Cancer 2017 , 17 , 692–704. [ Google Scholar ] [ CrossRef ]
- Davis, M.B. Genomics and Cancer Disparities: The Justice and Power of Inclusion. Cancer Discov. 2021 , 11 , 805–809. [ Google Scholar ] [ CrossRef ]
- Zavala, V.A.; Bracci, P.M.; Carethers, J.M.; Carvajal-Carmona, L.; Coggins, N.B.; Cruz-Correa, M.R.; Davis, M.; de Smith, A.J.; Dutil, J.; Figueiredo, J.C.; et al. Cancer health disparities in racial/ethnic minorities in the United States. Br. J. Cancer 2021 , 124 , 315–332. [ Google Scholar ] [ CrossRef ] [ PubMed ]
- Wojcik, G.L.; Graff, M.; Nishimura, K.K.; Tao, R.; Haessler, J.; Gignoux, C.R.; Highland, H.M.; Patel, Y.M.; Sorokin, E.P.; Avery, C.L.; et al. Genetic analyses of diverse populations improves discovery for complex traits. Nature 2019 , 570 , 514–518. [ Google Scholar ] [ CrossRef ] [ PubMed ]
- da Silva Rosa, S.C.; Barzegar Behrooz, A.; Guedes, S.; Vitorino, R.; Ghavami, S. Prioritization of genes for translation: A computational approach. Expert Rev. Proteom. 2024 , 21 , 125–147. [ Google Scholar ] [ CrossRef ]
- Sonawane, A.R.; Weiss, S.T.; Glass, K.; Sharma, A. Network Medicine in the Age of Biomedical Big Data. Front. Genet. 2019 , 10 , 294. [ Google Scholar ] [ CrossRef ]
- Biswas, S.; Pal, S.; Majumder, P.P.; Bhattacharjee, S. A framework for pathway knowledge driven prioritization in genome-wide association studies. Genet. Epidemiol. 2020 , 44 , 841–853. [ Google Scholar ] [ CrossRef ] [ PubMed ]
- Yu, K.H.; Snyder, M. Omics Profiling in Precision Oncology. Mol. Cell. Proteom. MCP 2016 , 15 , 2525–2536. [ Google Scholar ] [ CrossRef ] [ PubMed ]
- Huang, S.; Chaudhary, K.; Garmire, L.X. More Is Better: Recent Progress in Multi-Omics Data Integration Methods. Front. Genet. 2017 , 8 , 84. [ Google Scholar ] [ CrossRef ] [ PubMed ]
- Qiao, R.; Cong, Y.; Ovais, M.; Cai, R.; Chen, C.; Wang, L. Performance modulation and analysis for catalytic biomedical nanomaterials in biological systems. Cell Rep. Phys. Sci. 2023 , 4 , 101453. [ Google Scholar ] [ CrossRef ]
- Tebani, A.; Afonso, C.; Marret, S.; Bekri, S. Omics-Based Strategies in Precision Medicine: Toward a Paradigm Shift in Inborn Errors of Metabolism Investigations. Int. J. Mol. Sci. 2016 , 17 , 1555. [ Google Scholar ] [ CrossRef ]
- Olivier, M.; Asmis, R.; Hawkins, G.A.; Howard, T.D.; Cox, L.A. The Need for Multi-Omics Biomarker Signatures in Precision Medicine. Int. J. Mol. Sci. 2019 , 20 , 4781. [ Google Scholar ] [ CrossRef ]
- McDaniel, E.A.; Wahl, S.A.; Ishii, S.; Pinto, A.; Ziels, R.; Nielsen, P.H.; McMahon, K.D.; Williams, R.B.H. Prospects for multi-omics in the microbial ecology of water engineering. Water Res. 2021 , 205 , 117608. [ Google Scholar ] [ CrossRef ] [ PubMed ]
- Petti, M.; Farina, L. Network medicine for patients’ stratification: From single-layer to multi-omics. WIREs Mech. Dis. 2023 , 15 , e1623. [ Google Scholar ] [ CrossRef ] [ PubMed ]
- Roychowdhury, R.; Das, S.P.; Gupta, A.; Parihar, P.; Chandrasekhar, K.; Sarker, U.; Kumar, A.; Ramrao, D.P.; Sudhakar, C. Multi-Omics Pipeline and Omics-Integration Approach to Decipher Plant’s Abiotic Stress Tolerance Responses. Genes 2023 , 14 , 1281. [ Google Scholar ] [ CrossRef ] [ PubMed ]
- Aslam, B.; Basit, M.; Nisar, M.A.; Khurshid, M.; Rasool, M.H. Proteomics: Technologies and Their Applications. J. Chromatogr. Sci. 2017 , 55 , 182–196. [ Google Scholar ] [ CrossRef ] [ PubMed ]
- Cho, W.C. Proteomics technologies and challenges. Genom. Proteom. Bioinform. 2007 , 5 , 77–85. [ Google Scholar ] [ CrossRef ]
- Neverova, I.; Van Eyk, J.E. Role of chromatographic techniques in proteomic analysis. J. Chromatogr. B 2005 , 815 , 51–63. [ Google Scholar ] [ CrossRef ]
- Ackermann, B.L.; Hale, J.E.; Duffin, K.L. The role of mass spectrometry in biomarker discovery and measurement. Curr. Drug Metab. 2006 , 7 , 525–539. [ Google Scholar ] [ CrossRef ]
- Parker, C.E.; Domanski, D.; Percy, A.J.; Chambers, A.G.; Camenzind, A.G.; Smith, D.S.; Borchers, C.H. Mass spectrometry in high-throughput clinical biomarker assays: Multiple reaction monitoring. Top. Curr. Chem. 2014 , 336 , 117–137. [ Google Scholar ] [ CrossRef ]
- Bichmann, L.; Gupta, S.; Rosenberger, G.; Kuchenbecker, L.; Sachsenberg, T.; Ewels, P.; Alka, O.; Pfeuffer, J.; Kohlbacher, O.; Röst, H. DIAproteomics: A Multifunctional Data Analysis Pipeline for Data-Independent Acquisition Proteomics and Peptidomics. J. Proteome Res. 2021 , 20 , 3758–3766. [ Google Scholar ] [ CrossRef ]
- Demichev, V.; Messner, C.B.; Vernardis, S.I.; Lilley, K.S.; Ralser, M. DIA-NN: Neural networks and interference correction enable deep proteome coverage in high throughput. Nat. Methods 2020 , 17 , 41–44. [ Google Scholar ] [ CrossRef ]
- Caldwell, R.L.; Caprioli, R.M. Tissue profiling by mass spectrometry: A review of methodology and applications. Mol. Cell. Proteom. MCP 2005 , 4 , 394–401. [ Google Scholar ] [ CrossRef ]
- Karlsson, O.; Hanrieder, J. Imaging mass spectrometry in drug development and toxicology. Arch. Toxicol. 2017 , 91 , 2283–2294. [ Google Scholar ] [ CrossRef ] [ PubMed ]
- Wei, X.; Li, L. Mass spectrometry-based proteomics and peptidomics for biomarker discovery in neurodegenerative diseases. Int. J. Clin. Exp. Pathol. 2009 , 2 , 132–148. [ Google Scholar ] [ PubMed ]
- Cafe-Mendes, C.C.; Ferro, E.S.; Britto, L.R.; Martins-de-Souza, D. Using mass spectrometry-based peptidomics to understand the brain and disorders such as Parkinson’s disease and schizophrenia. Curr. Top. Med. Chem. 2014 , 14 , 369–381. [ Google Scholar ] [ CrossRef ]
- Yu, K.; Salomon, A.R. HTAPP: High-throughput autonomous proteomic pipeline. Proteomics 2010 , 10 , 2113–2122. [ Google Scholar ] [ CrossRef ]
- Lin, Z.; Gongora, J.; Liu, X.; Xie, Y.; Zhao, C.; Lv, D.; Garcia, B.A. Automation to Enable High-Throughput Chemical Proteomics. J. Proteome Res. 2023 , 22 , 3676–3682. [ Google Scholar ] [ CrossRef ]
- Reilly, L.; Lara, E.; Ramos, D.; Li, Z.; Pantazis, C.B.; Stadler, J.; Santiana, M.; Roberts, J.; Faghri, F.; Hao, Y.; et al. A fully automated FAIMS-DIA mass spectrometry-based proteomic pipeline. Cell Rep. Methods 2023 , 3 , 100593. [ Google Scholar ] [ CrossRef ]
- Wu, M.; Jiang, Y.; Ma, S. Integration of Proteomics and Other Omics Data. Methods Mol. Biol. 2021 , 2361 , 307–324. [ Google Scholar ] [ CrossRef ]
- Rohart, F.; Gautier, B.; Singh, A.; KA, L.C. mixOmics: An R package for ‘omics feature selection and multiple data integration. PLoS Comput. Biol. 2017 , 13 , e1005752. [ Google Scholar ] [ CrossRef ] [ PubMed ]
- Vasaikar, S.V.; Straub, P.; Wang, J.; Zhang, B. LinkedOmics: Analyzing multi-omics data within and across 32 cancer types. Nucleic Acids Res. 2018 , 46 , D956–D963. [ Google Scholar ] [ CrossRef ]
- Zhang, B.; Kuster, B. Proteomics Is Not an Island: Multi-omics Integration Is the Key to Understanding Biological Systems. Mol. Cell. Proteom. 2019 , 18 , S1–S4. [ Google Scholar ] [ CrossRef ]
- Krasnov, G.S.; Dmitriev, A.A.; Kudryavtseva, A.V.; Shargunov, A.V.; Karpov, D.S.; Uroshlev, L.A.; Melnikova, N.V.; Blinov, V.M.; Poverennaya, E.V.; Archakov, A.I.; et al. PPLine: An Automated Pipeline for SNP, SAP, and Splice Variant Detection in the Context of Proteogenomics. J. Proteome Res. 2015 , 14 , 3729–3737. [ Google Scholar ] [ CrossRef ]
- Posada-Céspedes, S.; Seifert, D.; Topolsky, I.; Jablonski, K.P.; Metzner, K.J.; Beerenwinkel, N. V-pipe: A computational pipeline for assessing viral genetic diversity from high-throughput data. Bioinformatics 2021 , 37 , 1673–1680. [ Google Scholar ] [ CrossRef ]
- Cheng, C. From Transcription Factor Binding and Histone Modification to Gene Expression: Integrative Quantitative Models. In Integrating Omics Data ; Tseng, G., Ghosh, D., Zhou, X.J., Eds.; Cambridge University Press: Cambridge, UK, 2015; pp. 380–402. [ Google Scholar ]
- Kang, M.; Ko, E.; Mersha, T.B. A roadmap for multi-omics data integration using deep learning. Brief. Bioinform. 2022 , 23 , bbab454. [ Google Scholar ] [ CrossRef ] [ PubMed ]
- Zuo, Y.; Yu, G.; Zhang, C.; Ressom, H. A New Approach for Multi-Omic Data Integration. In Proceedings of the 2014 IEEE International Conference on Bioinformatics and Biomedicine (BIBM), Belfast, UK, 2–5 November 2014; pp. 214–217. [ Google Scholar ]
- Bouhaddani, S.E.; Uh, H.W.; Jongbloed, G.; Hayward, C.; Klarić, L.; Kiełbasa, S.M.; Houwing-Duistermaat, J. Integrating omics datasets with the OmicsPLS package. BMC Bioinform. 2018 , 19 , 371. [ Google Scholar ] [ CrossRef ] [ PubMed ]
- Sherry, S.T.; Ward, M.H.; Kholodov, M.; Baker, J.; Phan, L.; Smigielski, E.M.; Sirotkin, K. dbSNP: The NCBI database of genetic variation. Nucleic Acids Res. 2001 , 29 , 308–311. [ Google Scholar ] [ CrossRef ] [ PubMed ]
- Birney, E.; Andrews, T.D.; Bevan, P.; Caccamo, M.; Chen, Y.; Clarke, L.; Coates, G.; Cuff, J.; Curwen, V.; Cutts, T.; et al. An overview of Ensembl. Genome Res. 2004 , 14 , 925–928. [ Google Scholar ] [ CrossRef ]
- Deutsch, E.W. File formats commonly used in mass spectrometry proteomics. Mol. Cell. Proteom. MCP 2012 , 11 , 1612–1621. [ Google Scholar ] [ CrossRef ]
- The UniProt Consortium. UniProt: The universal protein knowledgebase. Nucleic Acids Res. 2016 , 45 , D158–D169. [ Google Scholar ] [ CrossRef ]
- Wishart, D.S.; Tzur, D.; Knox, C.; Eisner, R.; Guo, A.C.; Young, N.; Cheng, D.; Jewell, K.; Arndt, D.; Sawhney, S.; et al. HMDB: The Human Metabolome Database. Nucleic Acids Res. 2007 , 35 , D521–D526. [ Google Scholar ] [ CrossRef ]
- Kanehisa, M.; Goto, S. KEGG: Kyoto encyclopedia of genes and genomes. Nucleic Acids Res. 2000 , 28 , 27–30. [ Google Scholar ] [ CrossRef ] [ PubMed ]
- Conroy, M.J.; Andrews, R.M.; Andrews, S.; Cockayne, L.; Dennis, E.A.; Fahy, E.; Gaud, C.; Griffiths, W.J.; Jukes, G.; Kolchin, M.; et al. LIPID MAPS: Update to databases and tools for the lipidomics community. Nucleic Acids Res. 2023 , 52 , D1677–D1682. [ Google Scholar ] [ CrossRef ]
- Tiemeyer, M.; Aoki, K.; Paulson, J.; Cummings, R.D.; York, W.S.; Karlsson, N.G.; Lisacek, F.; Packer, N.H.; Campbell, M.P.; Aoki, N.P.; et al. GlyTouCan: An accessible glycan structure repository. Glycobiology 2017 , 27 , 915–919. [ Google Scholar ] [ CrossRef ] [ PubMed ]
- Hayes, C.A.; Karlsson, N.G.; Struwe, W.B.; Lisacek, F.; Rudd, P.M.; Packer, N.H.; Campbell, M.P. UniCarb-DB: A database resource for glycomic discovery. Bioinformatics 2011 , 27 , 1343–1344. [ Google Scholar ] [ CrossRef ] [ PubMed ]
- Bolyen, E.; Rideout, J.R.; Dillon, M.R.; Bokulich, N.A.; Abnet, C.C.; Al-Ghalith, G.A.; Alexander, H.; Alm, E.J.; Arumugam, M.; Asnicar, F.; et al. Reproducible, interactive, scalable and extensible microbiome data science using QIIME 2. Nat. Biotechnol. 2019 , 37 , 852–857. [ Google Scholar ] [ CrossRef ] [ PubMed ]
- Meyer, F.; Paarmann, D.; D’Souza, M.; Olson, R.; Glass, E.M.; Kubal, M.; Paczian, T.; Rodriguez, A.; Stevens, R.; Wilke, A.; et al. The metagenomics RAST server—A public resource for the automatic phylogenetic and functional analysis of metagenomes. BMC Bioinform. 2008 , 9 , 386. [ Google Scholar ] [ CrossRef ]
- Hamilton, C.M.; Strader, L.C.; Pratt, J.G.; Maiese, D.; Hendershot, T.; Kwok, R.K.; Hammond, J.A.; Huggins, W.; Jackman, D.; Pan, H.; et al. The PhenX Toolkit: Get the most from your measures. Am. J. Epidemiol. 2011 , 174 , 253–260. [ Google Scholar ] [ CrossRef ]
- Carroll, R.J.; Bastarache, L.; Denny, J.C. R PheWAS: Data analysis and plotting tools for phenome-wide association studies in the R environment. Bioinformatics 2014 , 30 , 2375–2376. [ Google Scholar ] [ CrossRef ]
- Tryka, K.A.; Hao, L.; Sturcke, A.; Jin, Y.; Wang, Z.Y.; Ziyabari, L.; Lee, M.; Popova, N.; Sharopova, N.; Kimura, M.; et al. NCBI’s Database of Genotypes and Phenotypes: dbGaP. Nucleic Acids Res. 2013 , 42 , D975–D979. [ Google Scholar ] [ CrossRef ]
- Thorn, C.F.; Klein, T.E.; Altman, R.B. PharmGKB: The Pharmacogenomics Knowledge Base. Methods Mol. Biol. 2013 , 1015 , 311–320. [ Google Scholar ] [ CrossRef ]
- Mayer, G. Data management in systems biology I-Overview and bibliography. arXiv 2009 , arXiv:0908.0411. [ Google Scholar ]
- Gomez-Cabrero, D.; Abugessaisa, I.; Maier, D.; Teschendorff, A.; Merkenschlager, M.; Gisel, A.; Ballestar, E.; Bongcam-Rudloff, E.; Conesa, A.; Tegnér, J. Data integration in the era of omics: Current and future challenges. BMC Syst. Biol. 2014 , 8 (Suppl. S2), I1. [ Google Scholar ] [ CrossRef ] [ PubMed ]
- Benkirane, H.; Pradat, Y.; Michiels, S.; Cournède, P.H. CustOmics: A versatile deep-learning based strategy for multi-omics integration. PLoS Comput. Biol. 2023 , 19 , e1010921. [ Google Scholar ] [ CrossRef ]
- Sadegh, S.; Skelton, J.; Anastasi, E.; Bernett, J.; Blumenthal, D.B.; Galindez, G.; Salgado-Albarrán, M.; Lazareva, O.; Flanagan, K.; Cockell, S.; et al. Network medicine for disease module identification and drug repurposing with the NeDRex platform. Nat. Commun. 2021 , 12 , 6848. [ Google Scholar ] [ CrossRef ] [ PubMed ]
- Hamosh, A.; Scott, A.F.; Amberger, J.S.; Bocchini, C.A.; McKusick, V.A. Online Mendelian Inheritance in Man (OMIM), a knowledgebase of human genes and genetic disorders. Nucleic Acids Res. 2005 , 33 , D514–D517. [ Google Scholar ] [ CrossRef ]
- Piñero, J.; Ramírez-Anguita, J.M.; Saüch-Pitarch, J.; Ronzano, F.; Centeno, E.; Sanz, F.; Furlong, L.I. The DisGeNET knowledge platform for disease genomics: 2019 update. Nucleic Acids Res. 2019 , 48 , D845–D855. [ Google Scholar ] [ CrossRef ]
- O’Leary, N.A.; Wright, M.W.; Brister, J.R.; Ciufo, S.; Haddad, D.; McVeigh, R.; Rajput, B.; Robbertse, B.; Smith-White, B.; Ako-Adjei, D.; et al. Reference sequence (RefSeq) database at NCBI: Current status, taxonomic expansion, and functional annotation. Nucleic Acids Res. 2016 , 44 , D733–D745. [ Google Scholar ] [ CrossRef ]
- Milacic, M.; Beavers, D.; Conley, P.; Gong, C.; Gillespie, M.; Griss, J.; Haw, R.; Jassal, B.; Matthews, L.; May, B.; et al. The Reactome Pathway Knowledgebase 2024. Nucleic Acids Res. 2023 , 52 , D672–D678. [ Google Scholar ] [ CrossRef ]
- Vasilevsky, N.A.; Matentzoglu, N.A.; Toro, S.; Flack, J.E.; Hegde, H.; Unni, D.R.; Alyea, G.F.; Amberger, J.S.; Babb, L.; Balhoff, J.P.; et al. Mondo: Unifying diseases for the world, by the world. medRxiv 2022 . [ Google Scholar ] [ CrossRef ]
- Knox, C.; Wilson, M.; Klinger, C.M.; Franklin, M.; Oler, E.; Wilson, A.; Pon, A.; Cox, J.; Chin, N.E.L.; Strawbridge, S.A.; et al. DrugBank 6.0: The DrugBank Knowledgebase for 2024. Nucleic Acids Res. 2024 , 52 , D1265–D1275. [ Google Scholar ] [ CrossRef ]
- Ursu, O.; Holmes, J.; Knockel, J.; Bologa, C.G.; Yang, J.J.; Mathias, S.L.; Nelson, S.J.; Oprea, T.I. DrugCentral: Online drug compendium. Nucleic Acids Res. 2017 , 45 , D932–D939. [ Google Scholar ] [ CrossRef ]
- Technology, Inc. Neo4j, the World’s Leading Graph Database. Available online: https://neo4j.com/ (accessed on 1 June 2024).
- Shannon, P.; Markiel, A.; Ozier, O.; Baliga, N.S.; Wang, J.T.; Ramage, D.; Amin, N.; Schwikowski, B.; Ideker, T. Cytoscape: A software environment for integrated models of biomolecular interaction networks. Genome Res. 2003 , 13 , 2498–2504. [ Google Scholar ] [ CrossRef ]
- Ahmed, R.; Angelini, P.; Darabi Sahneh, F.; Efrat, A.; Glickenstein, D.; Gronemann, M.; Heinsohn, N.; Kobourov, S.; Spence, R.; Watkins, J.; et al. Multi-Level Steiner Trees. J. Exp. Algorithmics 2018 , 24 , 1–22. [ Google Scholar ] [ CrossRef ]
- Kumar, R.; Singh, A.; Mohan, A.; Goh, K.L. Link Based Spam Algorithms in Adversarial Information Retrieval. Cybern. Syst. 2012 , 43 , 459–475. [ Google Scholar ] [ CrossRef ]
- Lazareva, O.; Canzar, S.; Yuan, K.; Baumbach, J.; Blumenthal, D.B.; Tieri, P.; Kacprowski, T.; List, M. BiCoN: Network-constrained biclustering of patients and omics data. Bioinformatics 2020 , 37 , 2398–2404. [ Google Scholar ] [ CrossRef ] [ PubMed ]
- Ghiassian, S.D.; Menche, J.; Barabási, A.L. A DIseAse MOdule Detection (DIAMOnD) algorithm derived from a systematic analysis of connectivity patterns of disease proteins in the human interactome. PLoS Comput. Biol. 2015 , 11 , e1004120. [ Google Scholar ] [ CrossRef ] [ PubMed ]
- Barzegar Behrooz, A.; Latifi-Navid, H.; da Silva Rosa, S.C.; Swiat, M.; Wiechec, E.; Vitorino, C.; Vitorino, R.; Jamalpoor, Z.; Ghavami, S. Integrating Multi-Omics Analysis for Enhanced Diagnosis and Treatment of Glioblastoma: A Comprehensive Data-Driven Approach. Cancers 2023 , 15 , 3158. [ Google Scholar ] [ CrossRef ]
- Correa-Aguila, R.; Alonso-Pupo, N.; Hernández-Rodríguez, E.W. Multi-omics data integration approaches for precision oncology. Mol. Omics 2022 , 18 , 469–479. [ Google Scholar ] [ CrossRef ] [ PubMed ]
- Vougas, K.; Krochmal, M.; Jackson, T.; Polyzos, A.; Aggelopoulos, A.; Pateras, I.; Liontos, M.; Varvarigou, A.; Johnson, E.; Georgoulias, V.; et al. Deep Learning and Association Rule Mining for Predicting Drug Response in Cancer. A Personalised Medicine Approach. BioRxiv 2017 . [ Google Scholar ] [ CrossRef ]
- Tong, L.; Shi, W.; Isgut, M.; Zhong, Y.; Lais, P.; Gloster, L.; Sun, J.; Swain, A.; Giuste, F.; Wang, M.D. Integrating Multi-Omics Data with EHR for Precision Medicine Using Advanced Artificial Intelligence. IEEE Rev. Biomed. Eng. 2024 , 17 , 80–97. [ Google Scholar ] [ CrossRef ]
- Madrid-Márquez, L.; Rubio-Escudero, C.; Pontes, B.; González-Pérez, A.; Riquelme, J.C.; Sáez, M.E. MOMIC: A Multi-Omics Pipeline for Data Analysis, Integration and Interpretation. Appl. Sci. 2022 , 12 , 3987. [ Google Scholar ] [ CrossRef ]
- Miao, B.B.; Dong, W.; Gu, Y.X.; Han, Z.F.; Luo, X.; Ke, C.H.; You, W.W. OmicsSuite: A customized and pipelined suite for analysis and visualization of multi-omics big data. Hortic. Res. 2023 , 10 , uhad195. [ Google Scholar ] [ CrossRef ]
- Merelli, I.; Pérez-Sánchez, H.; Gesing, S.; D’Agostino, D. High-performance computing and big data in omics-based medicine. BioMed Res. Int. 2014 , 2014 , 825649. [ Google Scholar ] [ CrossRef ]
Click here to enlarge figure
Omics Type | Approaches | Outputs | Goals | Practical Suggestions |
---|---|---|---|---|
Genomics | High-throughput sequencing, annotation tools | Genome sequences, genetic variants | Identify genetic mutations, understand disease genetics | Use integrated databases (e.g., Ensembl) for annotation |
Transcriptomics | RNA sequencing, normalization, analysis tools | Gene expression profiles, splicing variants | Analyze gene expression changes, understand regulatory mechanisms | Combine with single-cell RNA-seq for detailed insights |
Proteomics | Mass spectrometry, protein databases | Protein identification, quantification | Understand protein functions, identify biomarkers and targets | Employ bioinformatics tools (e.g., MaxQuant) for data processing |
Metabolomics | NMR spectroscopy, mass spectrometry | Metabolite profiles, metabolic pathways | Identify metabolic changes, understand pathways and disease mechanisms | Use MetaboAnalyst for comprehensive analysis |
Epigenomics | DNA methylation analysis, ChIP-Seq | Epigenetic modification maps | Study gene regulation, understand epigenetic influences on disease | Integrate data with ENCODE for broader insights |
Lipidomics | Mass spectrometry, chromatography | Lipid profiles, lipid interactions | Investigate lipid metabolism, understand lipid-related diseases | Utilize LipidSearch for detailed lipid analysis |
Glycomics | Mass spectrometry, lectin microarrays | Glycan structures, glycosylation patterns | Study glycosylation processes, understand glycan roles in health | Use GlycoWorkbench for structural analysis |
Microbiomics | 16S rRNA sequencing, metagenomics | Microbial community composition | Understand microbiome contributions to health, study microbial interactions | Integrate with QIIME for diversity analysis |
Metagenomics | High-throughput sequencing, data integration tools | Community genomic data, functional profiles | Analyze microbial communities, understand environmental impacts | Use MG-RAST for metagenomic analysis |
Pharmacogenomics | Genotyping, pharmacokinetic studies | Genetic markers for drug response | Personalize medicine, predict drug response and side effects | Apply PharmGKB resources for clinical interpretation |
Toxinomics | Toxicogenomics, proteomics, metabolomics | Toxicity profiles, biomarker identification | Assess environmental and drug toxicities, identify toxicity mechanisms | Utilize ToxPi for visualizing risk assessments |
Exposomics | Environmental monitoring, high-throughput sequencing | Exposure biomarkers, environmental impacts | Understand effects of environmental exposures on health | Integrate exposomic data with health records |
Single-cell Omics | Single-cell sequencing, advanced imaging | Single-cell gene expression, protein profiles | Investigate cellular heterogeneity, understand cell functions | Use Seurat for single-cell data analysis |
Spatial Omics | Spatial transcriptomics, proteomics imaging | Spatial maps of gene/protein expression | Analyze tissue architecture, understand spatial organization | Apply 10× Genomics Visium for spatial transcriptomics |
Nutrigenomics | Diet records, genotyping | Gene-diet interactions, nutritional biomarkers | Understand diet impact on gene expression, personalize nutrition | Combine with dietary intake software for comprehensive plans |
Immunomics | Immune profiling, sequencing | Immune cell profiles, cytokine levels | Study immune responses, understand autoimmune diseases | Utilize Cytobank for immune profiling analysis |
Aspect | Challenges | Opportunities | Requirements | Pitfalls |
---|---|---|---|---|
Data Complexity | Managing large volumes of heterogeneous data | Development of advanced bioinformatics tools for data integration | Sophisticated bioinformatics tools and infrastructures | Data heterogeneity and lack of standardized data formats |
Technical Limitations | Sensitivity, specificity and accuracy of high-throughput technologies | Continuous technological advancements improving precision | Advanced instrumentation and accurate measurement techniques | Overfitting and varying samples/instrument quality |
Cost and Accessibility | High costs of equipment and reagents | Reduction in costs through technological innovations and economies of scale | Cost-effective technological solutions | High initial investment and maintenance costs |
Data Storage and Management | Efficient storage, retrieval and sharing of large datasets | Cloud-based storage solutions and standardized data formats | Robust data storage and management systems | Data losses and breaches in data security |
Data Interpretation | Complexity in analyzing and interpreting multi-omics data | Use of machine learning and AI for comprehensive data analysis | Expertise in machine learning and data analytics | Misinterpretations due to data complexity |
Ethical and Privacy Concerns | Ensuring privacy and security of sensitive genetic information | Development of ethical guidelines and robust security measures | Adherence to ethical standards and data protection protocols | Privacy breaches and ethical dilemmas |
Interdisciplinary Collaboration | Need for expertise in multiple disciplines (biology, chemistry and bioinformatics) | Fostering collaborations across diverse fields to drive innovation | Strong collaborative frameworks and interdisciplinary training | Miscommunications and integration issues among diverse teams |
Standardization | Lack of standardized protocols and methodologies | Establishing universal standards for reproducibility and comparability | Universal standards and protocols | Inconsistent results and lack of comparability across studies |
Tool | Description | Key Applications |
---|---|---|
MetaMap [ ] | Maps biomedical text to the Unified Medical Language System (UMLS) Metathesaurus, identifies concepts in biomedical literature | Data annotation and information retrieval |
Textpresso [ ] | Information retrieval and extraction system for biological literature, categorizes and indexes text based on biological concepts | Facilitates extraction of specific information from biological literature |
Pubpular [ ] | Identifies high-priority proteins in human diseases based on semantic similarity, analyzes text data from biomedical literature | Prioritizes proteins relevant to disease pathology by calculating semantic similarities between protein and disease terms |
VoSviewer [ ] | Software tool for creating and visualizing bibliometric networks, analyzes co-occurrence of terms in scientific literature | Identifies research trends, collaborations and key areas of interest in the biomedical field |
PubTator [ ] | Provides annotations for biomedical entities such as genes, diseases and chemicals in text | Extracts relevant information from large corpora of biomedical literature |
Application Area | Proteomics | Peptidomics | Advantages | Limitations |
---|---|---|---|---|
Protein Identification | Identifying proteins in complex mixtures | Identifying peptide sequences and modifications | Comprehensive identification of proteins and their functions | Complex sample preparation and high data complexity |
Quantitative Analysis | Measuring protein expression levels | Quantifying peptide abundances | Accurate quantification of protein expression levels | Requires high sensitivity and precision in measurement |
Post-Translational Modifications | Detecting phosphorylation, glycosylation and acetylation | Characterizing modifications on peptides | Detailed analysis of protein modifications | Complex detection and interpretation of multiple modifications |
Biomarker Discovery | Identifying protein biomarkers for diseases | Discovering peptide biomarkers for diagnostic purposes | Potential to discover novel biomarkers for various diseases | Validation of biomarkers is resource-intensive and time-consuming |
Protein-Protein Interactions | Analyzing interaction networks | Studying peptide-mediated interactions | Insight into protein interaction networks and cellular processes | Difficult to detect transient or weak interactions |
Structural Proteomics | Investigating protein structures and conformations | Analyzing peptide structures and dynamics | Understanding protein folding, stability and interactions | Requires advanced techniques like X-ray crystallography or NMR |
Clinical Proteomics | Profiling proteins in clinical samples (e.g., blood, tissue) | Profiling peptides in clinical samples | Direct application to clinical diagnostics and personalized medicine | Variability in clinical samples can affect reproducibility |
Proteome Mapping | Mapping the entire proteome of organisms or cells | Mapping peptidomes for specific conditions | Comprehensive overview of proteome composition and changes | Requires extensive data analysis and integration |
Drug Development | Identifying drug targets and mechanisms | Screening peptide-based therapeutics | Identification of novel drug targets and understanding mechanisms of action | High cost and time investment in drug discovery pipeline |
Pathway Analysis | Studying protein roles in biological pathways | Analyzing peptides involved in signaling pathways | Insight into biological pathways and their regulation | Complexity of pathway interactions and need for high-throughput analysis |
Immune Monitoring | Profiling immune-related proteins | Characterizing antigenic peptides | Identification of immune responses and potential vaccine targets | High variability and need for extensive validation |
De Novo Sequencing | Sequencing unknown proteins | Sequencing unknown peptides | Determining the sequence of novel proteins and peptides | Requires advanced algorithms and high-quality mass spectrometry data |
The statements, opinions and data contained in all publications are solely those of the individual author(s) and contributor(s) and not of MDPI and/or the editor(s). MDPI and/or the editor(s) disclaim responsibility for any injury to people or property resulting from any ideas, methods, instructions or products referred to in the content. |
Share and Cite
Vitorino, R. Transforming Clinical Research: The Power of High-Throughput Omics Integration. Proteomes 2024 , 12 , 25. https://doi.org/10.3390/proteomes12030025
Vitorino R. Transforming Clinical Research: The Power of High-Throughput Omics Integration. Proteomes . 2024; 12(3):25. https://doi.org/10.3390/proteomes12030025
Vitorino, Rui. 2024. "Transforming Clinical Research: The Power of High-Throughput Omics Integration" Proteomes 12, no. 3: 25. https://doi.org/10.3390/proteomes12030025
Article Metrics
Article access statistics, further information, mdpi initiatives, follow mdpi.
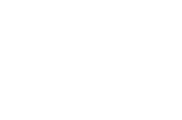
Subscribe to receive issue release notifications and newsletters from MDPI journals
Modelling a storage system of a wind farm with a ramp-rate limitation: a semi-Markov modulated Brownian bridge approach
- Original Research
- Open access
- Published: 06 September 2024
Cite this article
You have full access to this open access article
- Abel Azze ORCID: orcid.org/0000-0002-3124-6420 1 na1 ,
- Guglielmo D’Amico ORCID: orcid.org/0000-0002-6948-2912 2 na1 ,
- Bernardo D’Auria ORCID: orcid.org/0000-0002-1272-8352 3 na1 &
- Salvatore Vergine ORCID: orcid.org/0000-0002-3926-3081 4 na1
We propose a new methodology to simulate the discounted penalty applied to a wind-farm operator by violating ramp-rate limitation policies. It is assumed that the operator manages a wind turbine plugged into a battery, which either provides or stores energy on demand to avoid ramp-up and ramp-down events. The battery stages, namely charging, discharging, or neutral, are modeled as a semi-Markov process. During each charging/discharging period, the energy stored/supplied is assumed to follow a modified Brownian bridge that depends on three parameters. We prove the validity of our methodology by testing the model on 10 years of real wind-power data and comparing real versus simulated results.
Similar content being viewed by others
Markov Processes for the Management of a Microgrid
Balancing Wind and Batteries: Towards Predictive Verification of Smart Grids
Stochastic coordinated operation of wind and battery energy storage system considering battery degradation.
Avoid common mistakes on your manuscript.
1 Introduction
In the last decades, we have assisted in the increase of renewable energy penetration in the electricity market, in particular from wind and solar sources. This is caused by the increasing concern about environmental pollution and global warming, and the awareness of having to exploit sources of clean energy to decrease the use of fossil fuels (Biancardi et al., 2023 ; Janzen et al., 2020 ; Razmjoo et al., 2021 ).
One of the main problems that hinder the use of wind power is its intermittent nature caused by rapid and unpredictable fluctuations in wind speed. This conflicts with the stability required by the energy market to guarantee a systemic balance and security (Frate et al., 2020 ).
Among the control strategies used to decrease the high variability of wind power, the ramp-rate limitation has seen increasing use in recent years (Bossavy et al., 2015 ; D’Amico et al., 2021 , 2022b ; Hittinger et al., 2014 ). Limiting the ramp rate means limiting the rate at which the power production varies between two consecutive time steps. The ramp-rate limits might be violated in two ways: up-ramping events, meaning that the variation is positive, and down-ramping events, when the change is negative (Gallego-Castillo et al., 2015 ). Predicting these two types of events has been in the spotlight of wind-farm managing literature for a while (Cui et al., 2021 ; Zheng et al., 2022 ).
The ramp-rate event is considered a critical event because its delayed and inadequate control can cause serious damage to the power grid and consequent economic losses. Several of the largest system operators, such as the Electric Reliability Council of Texas (ERCOT) and the state-owned electric power transmission operator in Ireland, EirGrid, require ramp-rate control to wind generators. According to the grid characteristics, it can be requested to control the ramp rate within 1- and 10-min limits (Cui et al., 2023 ; D’Amico et al., 2022b ; Hittinger et al., 2014 ).
Wind farms subjected to ramp-rate limitations usually use a storage system for two main purposes: providing power when a ramp-down occurs, and storing power in the presence of a ramp-up event (Abdullah et al., 2014 ; Hittinger et al., 2010 ; Khalid & Savkin, 2010 ; Lone & Mufti, 2008 ; Teleke et al., 2009 ). Batteries are the most used energy storage systems due to their quick response time and easy installation, and, in this context, its main variables of interest are the size and the state of charge, but in principle also pumped-storage generating system can be used (Li et al., 2019 ).
In the literature, particular attention is given to the ramp rate detection and prediction (Cui et al., 2021 ; Zheng et al., 2022 ), and, more in general, to the application of stochastic processes that make the operator able to know in advance the future behaviour of the system in terms of wind power variability (An et al., 2012 ; Chen et al., 2009 ; D’Amico et al., 2013a ; Grassi & Vecchio, 2010 ; Lee & Baldick, 2012 ). These aspects are relevant from an economic perspective due to the possibility of forecasting the economic losses of a wind farm under a ramp-rate policy (Cui et al., 2021 , 2017 ). The amount of penalty can be significant if we consider that, for example, that in ERCOT the penalty is computed by multiplying the energy (in MW-h) not meeting the ramp-rate up or down limitations by the regulation up or down prices, which were \(16\$\) /MW-h and \(13\$\) /MW-h in 2008–2009, respectively (Hittinger et al., 2014 ). According to Wan ( 2011 ), in Texas, between 2004 and 2009, the number of large ramp events with magnitude \(>25\%\) of the highest annual wind generation is 235 per year, on average. If we want to have a rough estimate of the penalization, we can assume that the average installed capacity in Texas is 2500 MW in such a period, and the average amount of ramp events with magnitude \(>25\%\) of the highest annual wind generation is 625 MW. By considering an average penalty of \(14.5\$\) /MW-h, we obtain a total average penalty of about \(9000,00\$\) per year. These economic losses refer to the ramp events with magnitude \(>25\%\) , which is very high. If we lower the percentage the losses increase.
Ramp-rate limitations are usually coupled with a penalty policy if the wind farm does not meet the imposed limits (Hittinger et al., 2014 ). Among the existing penalty systems applied by the system operators, one largely used method consists of multiplying a fixed monetary amount and the number of MW above or below the ramp-rate limits. The work of D’Amico et al. ( 2021 ) considers a system comprising a wind farm with a ramp-rate limitation policy and a battery, with the aim of forecasting the penalties received by the operator over a given time period. This kind of system shows a nonlinear behaviour, which is due to the interaction between the charge and discharge processes and the storage capacity of the battery. Indeed, as ramp events occur throughout time, the battery’s state of charge shifts accordingly. This affects the ability to dispatch or store energy as needed to avoid penalties. In summary, the variations of energy are subject to a time-varying nonlinear random constraint, which is the result of the wind speed fluctuations, state of charge, and ramp-rate policy. A discrete-time homogeneous Markov chain is used to model the battery operations, which are divided into three states: the charging event, the discharging event, and the neutral event or absence of operations. During each charging/discharging period, the random power stored/supplied by the battery is assumed to be a discrete collection of independent, not identically distributed, random variables. The penalty is then calculated by multiplying the random charges/discharges by the regulation fees. However, the Markovian assumption was proved to be not completely satisfactory in this context by D’Amico et al. ( 2022a ), where a semi-Markov process was instead considered to model the battery operations and, consequently, the penalty dynamics were set to evolve as a semi-Markov modulated reward process. This kind of stochastic process has been largely used in the literature (D’Amico et al., 2013b ; Feinberg, 1994 ; Papadopoulou et al., 2012 ).
This paper builds on the semi-Markov approach used in D’Amico et al. ( 2022a ) by using a modification of a Brownian Bridge (BB) to model the charging/discharging processes during the battery’s operation periods. Hence, we take off the independence assumption, considered by D’Amico et al. ( 2021 ) and ( 2022a ), between the random charges/discharges at different times, which does not seem to be supported by real data and can be considered only as an approximation of the behaviour of the system. Besides, a BB model accounts for the convenient Markovian and Gaussian properties along the waiting time of the underlying semi-Markov process, and it is one of the most exhaustively studied diffusion bridges, making it an appealing model from theoretical and applicable perspectives. We use 10 years of wind speed real data to compute the power production of a hypothetical wind turbine located in Sardinia, which we use to obtain the penalty associated to three different ramp-rate limitations: \(1\%\) , \(5\%\) and \(7\%\) of the wind turbine rated capacity. An estimation of the penalty process is then produced via a Monte Carlo simulation algorithm. The results suggest that our semi-Markov-modulated model succeeds in simulating the accumulated penalty process over a given time period.
The rest of the paper is structured as follows. Section 2 introduces the ramp-rate policy and sets the semi-Markov model that governs the battery operation process. When the battery is either charging or discharging, a discrete-time model based on a BB is proposed in Sect. 3 to model the dynamics of the random charges. We then introduce, in Sect. 4 , two key processes: the one associated with the state of the charge of the battery, and the penalty process. The last one is then generated via Monte Carlo simulations in Sect. 5 , where we show the competitiveness of our method by comparing its results against the penalty obtained from real data. Final thoughts are relegated to Sect. 6 .
2 The semi-Markov model of sequential ramp-rate events
Ramp-rate limitations can be used to smooth the power produced from the wind turbine and obtain a more stable output. We limit both up-ramping and down-ramping events, as done in Hittinger et al. ( 2014 ). This limitation decreases the slope by which the power profile changes between two consecutive time steps, it is indicated as a percentage of the rated capacity of the wind farm, and its unit of measure can be MW/h. Lower percentages represent stricter limitations. For example, if we consider a rated capacity of 2 MW, a ramp-rate limitation of \(1\%\) penalizes changes faster than 0.02 MW/h.
For \(\delta > 0\) and \(k\in \mathbb {N}\) let e ( k ) be the power generated at time \(\delta k\) , and define the modified power at the same time, denoted by \(\bar{e}(k)\) , as
where \(\ell > 0\) is the ramp-rate limit. For the sake of simplicity and because it does not sacrifice generality, we set \(\delta = 1\) for the rest of the paper. Working with an arbitrary time-step length follows identical steps. The following is an interpretation of the earlier quantities: the power generated by the wind turbine at time k is denoted by e ( k ), while the power that is effectively injected into the electrical grid at time k is denoted by \(\overline{e}(k)\) . The ramp-rate policy is employed to stabilize the grid because \(\overline{e}(k)\) exhibits milder variations and less steep slopes than e ( k ).
We connect a battery to the wind turbine to either store or supply energy in case of an up-ramping or down-ramping event, respectively. A penalization is applied every time the battery cannot store the total energy surplus or provide the required energy.
We consider the battery operations over time as a Markov chain \(\left\{ J_n\right\} _{n\in \mathbb {N}}\) with state space \(E=\{-1,0,+1\}\) . States \(-1\) and \(+1\) indicate discharging and charging operations, associated, respectively, with a down-ramping and up-ramping event. The state 0 represents the unchanged condition, that is, the battery is neither charging nor discharging, which occurs when the power production meets the ramp-rate limits. The process \(\left\{ K_n\right\} _{n\in \mathbb {N}}\) stands for the n -th time in which the battery changes state. We assume that \(K_0 = 0\) and \(K_n < K_{n+1}\) for all \(n \in \mathbb {N}\) . The (sojourn) time the battery remains in the state \(J_{n}\) , before the \((n+1)\) th jump, is denoted by \(X_{n+1}\) . Formal definitions of \(J_n\) , \(K_n\) , and \(X_n\) , are given below:
We assume that \(\left\{ (J_n, K_n)\right\} _{n\in \mathbb {N}}\) is a Markov Renewal process, and define its kernel as
According to the previous relation, regardless of what the values of the past variables were, knowing the last battery operation, \(J_n\) , is sufficient to provide the conditional joint distribution of the pair, \(J_{n+1}\) , and \(X_{n+1}\) .
For later reference, we introduce the conditional sojourn-time distribution
as well as the transition probabilities of the embedded Markov chain
and the conditional (to the sojourn time) transition probabilities
Finally, consider the process \(N(k) = \max \{{n > 0:K_n \le k\}}\) , which counts the number of transitions up to time k , as well as the Semi-Markov Chain (SMC) associated with the Markov Renewal chain \((J_{n}, K_{n})\) , indicated by \(J_{N(k)}\) , for \(k\in \mathbb {N}\) .
3 A Gauss–Markov model for battery operations between ramp-rate events
The purpose of this section is to model the battery storing operations using a BB, which is a well-studied process and accounts for two desirable properties, namely Gaussianity and Markovianity.
3.1 Setting the model
Consider the semi-Markov model of battery operations introduced in the previous section and call a random segment to any triplet \((J_n, J_{n+1}, X_{n+1})\) , for \(n\in \mathbb {N}\) . Hence, a random segment comprises an initial state \(J_n\) denoting the current operation, its time length \(X_{n+1}\) , and the next operation \(J_{n+1}\) . Thereby, the triplet \((J_n = +1, J_{n+1} = -1, X_{n+1} = 5)\) , for instance, denotes a segment where the battery after charging for 5 units of time starts discharging afterwards.
Let C ( k ) represents the theoretical random energy charged/discharged into/from the battery at time \(k\in \mathbb {N}\) . Define \(C_{J_n, J_{n+1}, X_{n+1}}:= \left\{ C_{J_n, J_{n+1}, X_{n+1}}(k)\right\} _{k = 1}^{X_{n+1}}\) be the process representing the charge ( \(J_n = +1\) ) or discharge ( \(J_n = -1\) ) during the n th stage of the SMC. Note that this notation presumes that the charging/discharging process depends on \(J_n\) , \(J_{n+1}\) , and \(X_{n+1}\) , and is independent of \(K_n\) . We assume that it is also independent of \(\left\{ C(m)\right\} _{m = 0}^{K_n - 1}\) . That is, for \(k = 1, \ldots , x\) ,
Consider the discrete-time processes \(\mathcal {C}_{i,j,x}:= \left\{ \mathcal {C}_{i, j, x}(k)\right\} _{k = 0}^{x+1}\) , for \(i\in \{-1, +1\}\) , \(j \in \{-1, 0, +1\}\backslash \{i\}\) , \(x \in \mathbb {N}\) . Moreover, set
\(\left\{ \mathcal {C}_{i,j,x}(k)\right\} _{k = 1}^x = \left\{ |C_{i,j,x}(k)|\right\} _{k = 1}^x\) ,
\(\mathcal {C}_{i,j,x}(0) = \mathcal {C}_{i,j,x}(x+1) = 0\) .
In this way, we are embedding the charging process \(C_{i,j,x}\) into the bridge process \(\mathcal {C}_{i,j,x}\) . Indeed, (P.1) sets the embedding while (P.2) ensures that \(\mathcal {C}_{i,j,x}\) can be regarded as a bridge that vanishes at its initial and terminal times. Note that the absolute-value transformation in condition (P.1) does not introduce identifiability issues as \(C_{i,j,x}\) has constant sign. Figure 1 shows some linearly-interpolated sample paths of \(\mathcal {C}_{i,j,x}\) .
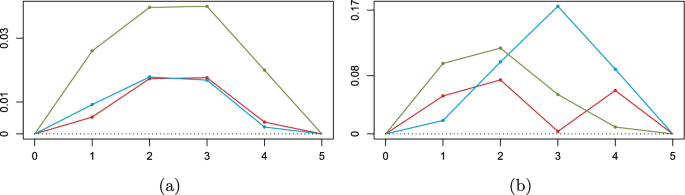
Battery charges/discharges \(\mathcal {C}_{i, j, x}\) . The coloured dots represent the actual values of the charges/discharges, which are linearly interpolated. (a) accounts for \((i = -1, j = 0, x = 4)\) , while in (b) \((i = +1, j = 0, x = 4)\) . (Color figure online)
Essentially, properties (P.1) and (P.2) define the size of the charging/discharging values for each segment of the SMC that is enlarged by introducing two boundary conditions at the times 0 and \(X_{n+1}+ 1\) , where the size of the charging/discharging is set to zero.
For a neater notation, we will define the shorthands \(C_n:= C_{J_n, J_{n+1}, X_{n+1}}\) and \(\mathcal {C}_n:= \mathcal {C}_{J_n, J_{n+1}, X_{n+1}}\) .
We now introduce a parsimonious model for \(\mathcal {C}_{i,j,x}\) . Define the parameters
and the error process
where, for \(0< \tau < x+1\) and \(h > 0\) , \(g_{i, j, x}\) is given by
Thus, \(C_n\) can be split into the sum of the (triangle-shaped) function \(g_{i,j,x}\) plus the stochastic process \(E_{i,j,x}\) . Figure 2 shows a few paths of these three processes.
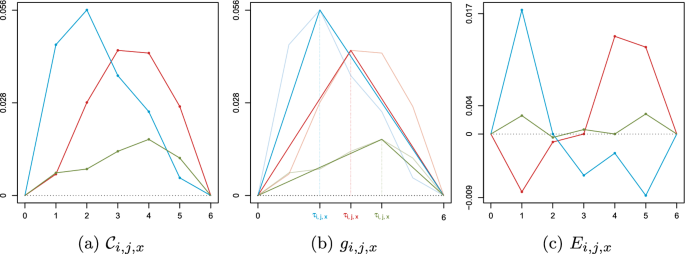
(a) Shows three paths of the bridge process Ci,j,x. (b) Displays these same charges (transparent lines), alongside the triangle functions \(g_{i, j, x}\) derived from them. The associated error process \(E_{i, j, x}\) is shown in ( c ). All images account for \(i = -1\) , \(j = 0\) , \(x = 5\) , and the ramp-rate coefficient \(\ell = 0.02\) . The values of \(\{\mathcal {C}_{i, j, x}(k)\}_{k = 1}^x\) are remarked using bullet points in the curves that result after linearly interpolating them
Let \(\bar{e}_{i, j, x}\) be the corrected power at the time when the battery changes to the state \(i\in E\) and remains there for a sojourn time x , after which it changes to the state \(j \in E\backslash \{i\}\) . Hence, \(\bar{e}_{i, j, x}\) has the same distribution as \(\bar{e}(K_n)\) for all n such that \((J_n = i, J_{n+1} = j, X_{n+1} = x)\) . We are implicitly assuming that \(\bar{e}(K_n)\) depends entirely on \(J_n\) , \(J_{n+1}\) , and \(X_{n+1}\) .
Consider now the variable
where \(P_r\) is the rated capacity of the wind turbine. The variable \(\rho _{i, j, x}\) can be regarded as the initial corrected power along the random segment \((J_n = i, J_{n+1} = j, X_{n+1} = x)\) .
In ( 8 ), the adjustments \(\bar{e}_{i,j,x} - \ell \) and \(Pr -(\bar{e}_{i,j,x}) - \ell \) are justified by the assumption that the battery has been charging/discharging for exactly one unit of time before the first observed battery operation of the random segment. The lower bounds \(\ell (x+1)\) and \(Pr - \ell (x+1)\) are needed because the battery remains in the state i exactly one unit of time after the last observed operation, making a total charging/discharging time length of \(x+1\) . Finally, the formula takes into account the fact that the initial power cannot exceed the rated capacity \(P_r\) neither be negative.
Inequality ( 9 ) alongside the non-negativity of \(\mathcal {C}_n\) yield the following restrictions to the error process \(E_{i, j, x}\) :
for some process \(Y_{i, j, x}:= \left\{ Y_{i, j, x}(k)\right\} _{k = 1}^x\) . We choose to model \(Y_{i, j, x}\) as a BB going from \((0, 0)\in \mathbb {R}^2\) to \((T, 0)\in \mathbb {R}^2\) and forced to stop by \((\tau _{i, j, x}, 0)\) . Hence, \(Y_{i, j, x}\) admits the representation
where \(Y_{i, j, x}^{(1)}\) and \(Y_{i, j, x}^{(2)}\) are two independent BBs satisfying the representation
where \(W^{(1)}\) and \(W^{(2)}\) are independent standard Brownian motions, and \(\sigma _{i, j, x} > 0\) is the common volatility term.
3.2 Estimation of the parameters
We provide here a mechanism to generate the parameters \(\rho _{i, j, x}\) , \(\tau _{i, j, x}\) , \(h_{i, j, x}\) , and \(\sigma _{i, j, x}\) . Define the shorthand notations \(\rho _n:= \rho _{J_n, J_{n+1}, X_{n+1}}\) , \(\tau _n:= \tau _{J_n, J_{n+1}, X_{n+1}}\) , and \(h_n:= h_{J_n, J_{n+1}, X_{n+1}}\) . For \(\rho _n\) , \(\tau _n\) , and \(h_n\) , in alignment to D’Amico et al. ( 2022a ), we assume that all their values belong to the same population as soon as they share the same sojourn time as well as initial and next charging stages. That is, if we consider a generic segment identified by the triplet \((J_n = i, J_{n+1} = j, X_{n+1} = x)\) , then the set of values
are simulations of the joint distribution of \((\rho _{i, j, x}, \tau _{i, j, x}, h_{i, j, x})\) , which we denote by \(f_{i, j, x}:{\mathcal {D}}_{n} \rightarrow \mathbb {R}_{+}\) , and has the support
with, in alignment to ( 8 ),
The upper bound \(\overline{h}\) comes after ( 8 ) and the definition of \(h_n\) in ( 5 ).
We estimate \(f_{i, j, x}\) in a non-parametric fashion by relying on kernel estimations of their vine copulas. This method is well-documented in Nagler ( 2014 ). Besides the flexibility of its non-parametric nature, the main drive for choosing this technique was its robustness in high-dimensional frameworks.
This method simulates \(\tau _{i, j, x}\) as a continuous variable within the interval [0, x ], and then we replace that original simulation with the nearest value within the support \(\left\{ 1, \ldots , x\right\} \) .
We leaned on the approach suggested by Geenens et al. ( 2017 ) for the copulas density estimation. They build on a larger body of works that transform observations in the unit square \([0, 1]^2\) into \(\mathbb {R}^2\) , where standard kernel density estimation techniques can be used, and a back-transformation recovers the copula density estimation. Specifically, Geenens et al. ( 2017 ) propose a local likelihood estimator by means of quadratic polynomials approximations.
We performed the kernel density estimation with these specifications via the R package kdevine (Nagler, 2022 ).
To avoid running into small data issues for the bandwidth matrix estimation, we bootstrapped the sample of any random segment with less than 10 observations and introduced small perturbations to guarantee differences among the new data values.
We illustrate in Fig. 3 how this method of estimating \(\rho _{i, j, x}\) , \(\tau _{i, j, x}\) , and \(h_{i, j, x}\) captures the distribution of the real data.
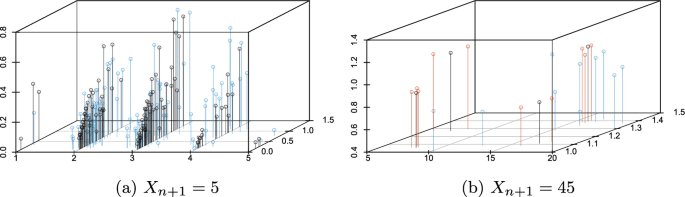
Simulation of the parameter \(\tau _{i, j, x}\) ( x -axis), \(h_{i, j, x}\) ( y -axis), and \(\rho _{i, j, x}\) ( z -axis). Black dots represent real data, while blue dots are randomly generated points from the kernel density estimation of \(f_{i, j, x}\) . Red points in ( b ) indicate the augmented bootstrapped data. For all images, \(i = -1\) , \(j = 0\) , and \(\ell = 0.02\) . (Color figure online)
The remaining parameter to be estimated is the volatility term \(\sigma _{i, j, x}\) . Recall that the BBs in ( 12 ) and ( 13 ) share the same volatility. The Gaussian and Markovian properties of the BB make it easy to come up with the following formula for the maximum likelihood estimator of \(\sigma _{i, j, x}\) :
with \(M_{i, j, x}:= \sum _{k=1}^{x} \mathbb {1}(Y_{i, j, x}(k) = E_{i, j, x}(t_k))\) , and
The definition of \(u_m\) in ( 14 ) is necessary to pick up only the times that truly represent the jumps in the BBs’ paths, and do not account for spurious values due to the representation of the error process \(E_{i, j, x}\) in ( 10 ). In ( 15 ), we set \(\tau _{i, j, x}(m)\) as a single term to denote the different terminal points of \(B_{i, j, x}^{(1)}\) and \(B_{i, j, x}^{(2)}\) .
One might be tempted to assume that \(\sigma _{i, j, x}\) is homogeneous with respect to other parameters like \(\rho _{i, j, x}\) , \(\tau _{i, j, x}\) , \(h_{i, j, x}\) , and x , or the battery stages \(J_n = i\) and \(J_{n+1} = j\) . However, empirical evidence suggests otherwise. For instance, higher heights \(h_{i, j, x}\) tend to produce higher volatilities, as Fig. 4 shows. It also illustrates that a convenient transformation of the volatility might result in a linear relationship between these two parameters.
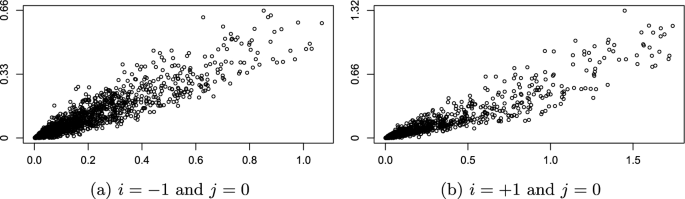
Images of the relation between the volatility and the height. The y axis marks the values of \(\widehat{\sigma }_{i, j, x}\) for any value \(x\in \mathbb {N}\) , and with \(i = -1\) and \(j = 0\) for ( a ), and \(i = -1\) and \(j = 0\) for ( b ), while values of \(h_{i, j, x}\) are in the x -axis. For both figures, \(\ell = 0.02\)
In light of this numerical evidence, we take \(\rho _{i, j, x}\) , \(\tau _{i, j, x}\) , \(h_{i, j, x}\) , x , and all their first-order interactions, as regressors in a linear model where a transformation of \(\widehat{\sigma }_{i, j, x}\) is the response. The transformation is chosen from a catalog of several parameterized functions, such that it better helps the linear model to meet its assumptions, namely normality, homoscedasticity, and linearity. We used the R package trafo (Medina et al., 2018 ) to perform this transformation selection. All cases pointed out to Box-Cox-type transformations, having the following form
The final estimation of the volatility \(\sigma _{i, j, x}\) is taken to be the anti-transformed mean of the linear model response, whose parameters are chosen to better fit the data
Actually, we fit the linear model twice. A first fitting was used to ditch out observations with outlier Cook’s distances, according to Tukey’s method of tagging an outlier as anything farther than 3 times the interquartile range from the median. The second and final fitting was done with the remaining observations. Figure 5 illustrates the final fit for \(i = -1\) and \(j = 0\) . Table 1 shows the values of the adjusted \(R^2\) as a metric of the goodness of the different linear models.
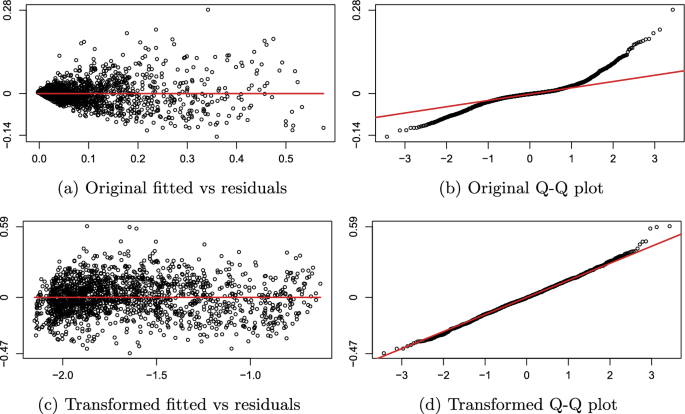
Check for the linear-model assumptions before and after taking the transformation \(q_\lambda \) , with \(i = -1\) , \(j = 0\) , and \(\ell = 0.02\)
The GitHub repository https://github.com/aguazz/WindPower-BatteryCharge provides all the R code and data necessary to implement the estimations and numerical algorithms introduced in this section.
Section 5 provides an algorithm to simulate paths of \(\mathcal {C}_{i, j, x}\) . The algorithm’s performance is validated by comparing the mean and covariance matrices of real and simulated data of \(\mathcal {C}_{i, j, x}\) , for different values of i , j , and x .
4 Techno-economical analysis
The study of the ramp-rate policy requires an analysis of the battery’s State Of Charge (SOC) along with the mechanism of the penalty cost.
Consider the backward-recurrence-time process \(B(k) = k - K_{N(k)}\) , and let S ( k ) represents the SOC of the battery at time \(k \ge 1\) , defined by
where \(\overline{c}\) and \(\underline{c}\) are the maximum and minimum SOC levels, respectively. Note that \(S(k)\in [\underline{c},\overline{c}]\) for all \(k \ge 1\) . We remind that, although it is not explicitly stated in the equation above, \(\mathcal {C}_n\) depends on \(J_n\) , \(J_{n+1}\) , and \(X_{n+1}\) . The previous relation is illustrative of the nonlinear nature of the considered stochastic system. The state of charge process is the result of a nonlinear transformation applied to the charging/discharging process which involves the random charge/discharge and the limit of the battery’s capacity. It is obvious that, in contrast to linear reward structures, the penalty process inhales the nonlinearity of the SOC process and makes it challenging to evaluate the accumulated discounted penalty process.
Likewise, consider the penalty process
where the constants \(x_{+1}\) and \(x_{-1}\) are the penalties per unit of time associated with up-ramping and down-ramping events, respectively.
Finally, consider the cumulative discounted penalty up until time \(k \in \mathbb {N}\) , defined as
where \(r \ge 0\) is the discount rate.
5 System simulation
The two simulation Algorithms 1 and 2 can be used to generate random paths of the charging/discharging process \(C_{i, j, x}\) discussed in Sect. 3 , as well as the SOC and the penalty processes, S and M , introduced in Sect. 4 .
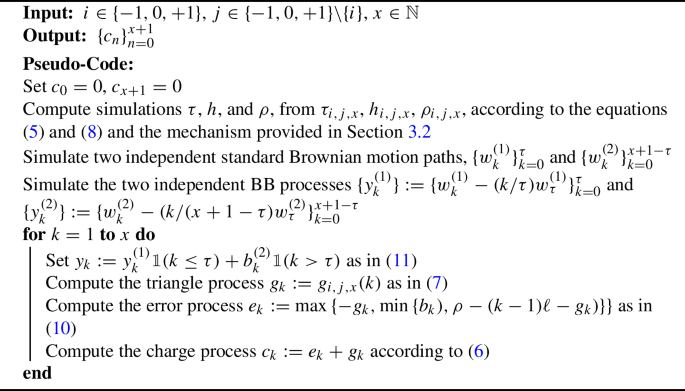
Battery charge/discharge simulator
Figure 6 shows simulated paths of \(\mathcal {C}_{i, j, x}\) , for different values of i , j , and x , produced by implementing Algorithm 1. Note that they visually resemble the real-data paths.
Besides the visual validation in Fig. 6 , we provide the relative mean \(L^2\) -error between the real and the estimated sample means and sample covariance matrices, displayed in Figs. 7 and 8 , respectively. We only considered those random segments with at least 30 observations. For each random segment, we simulated as many paths as the maximum between 3 times the real-data sample size and 100 trajectories.
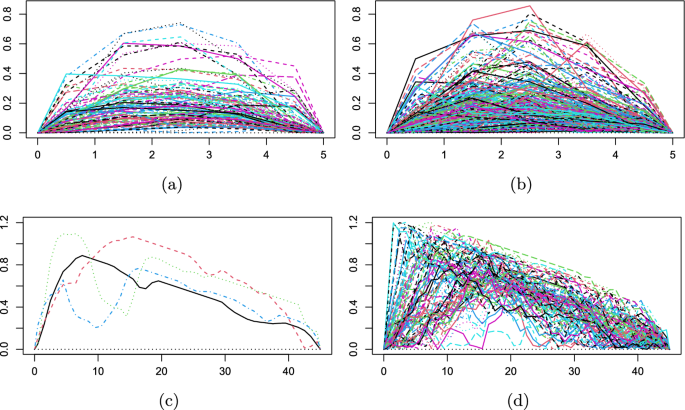
Real versus simulated charges. The first column of images accounts for real-data charges, that is, paths of \(\mathcal {C}_{i, j, x}\) pinned to (0, 0) and \((x + 1, 0)\) . The second column displays simulated paths of \(\mathcal {C}_{i, j, x}\) . The images on the first raw account for \(x = 5\) , while the second row uses \(x = 45\) . For all images, \(i = -1\) , \(j = 0\) , and \(\ell = 0.02\)
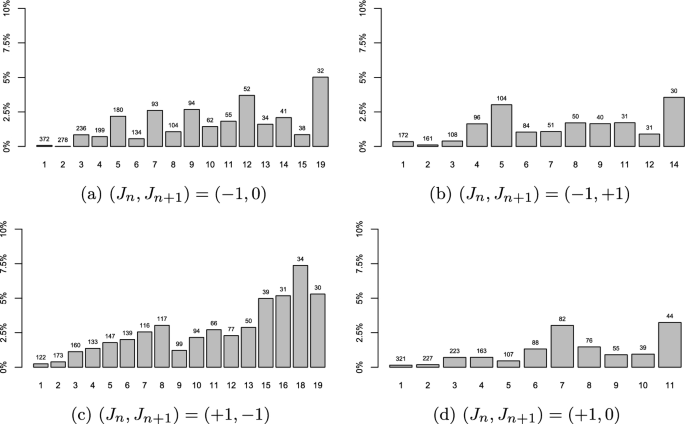
Relative \(L^2\) -error, expressed in percentage terms, between the real-data and simulated sample mean. The numbers in the x -axis represent the values of \(X_{n+1}\) , while the numbers on top of the bars are the real-data sample size. For all the images, \(\ell = 0.02\)
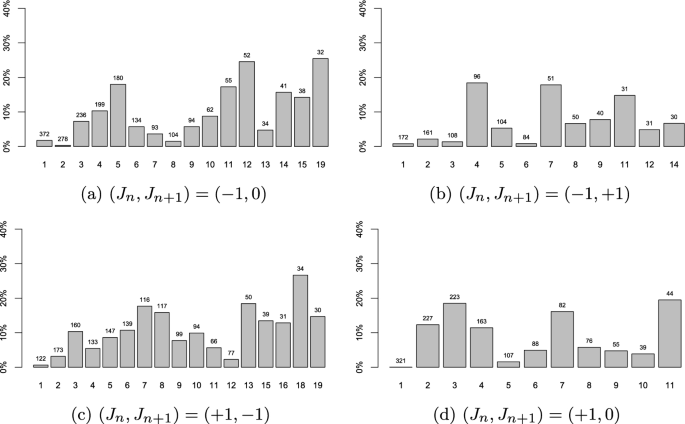
Relative \(L^2\) -error, expressed in percentage terms, between the real-data and simulated sample covariance matrices. The numbers in the x -axis represent the values of \(X_{n+1}\) , while the numbers on top of the bars are the real-data sample size. For all the images, \(\ell = 0.02\)
Algorithm 2 simulates trayectories of the Markov Renewal chain \(\{(J_{n}, K_{n})\}_{n\in \mathbb {N}}\) , as well as the SOC process \(\{S(k)\}_{k\in \mathbb {N}}\) and the penalty process \(\{M(k)\}_{k\in \mathbb {N}}\) .
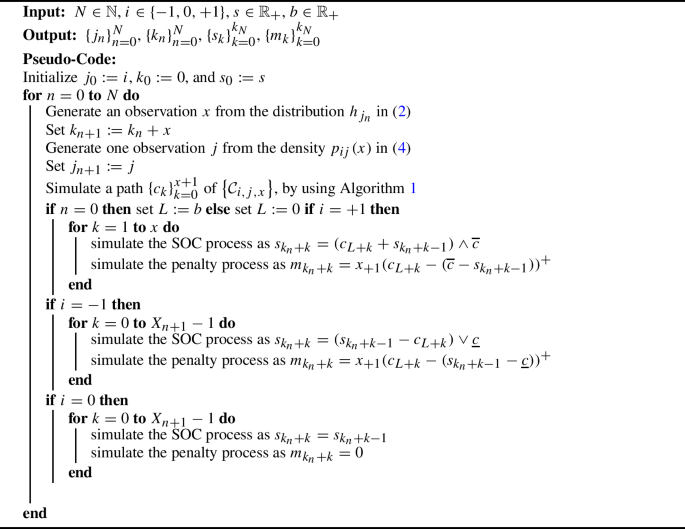
Semi-Markov reward, SOC, and penalty processes simulator
Next we estimate the first and second moments of \(W = \{W(k)\}_{k = 1}^K\) , for \(K = 24\) . That is, the hourly average and standard deviation of the cumulative penalty process within a day. To do so, we used Algorithms 1 and 2 to simulate N different trayectories of W , \(W^n = \{W(k)^{(n)}\}_{k = 1}^K\) , \(n = 1, \ldots , N\) . Once the N trajectories have been simulated, it is possible to estimate the moments of the accumulated penalty process for any time \(t \in \mathbb {N}\) by computing the corresponding sampling moments
For the wind-farm layout, we consider the battery described in (Hittinger et al. 2014 , Table 4), that is, a NaS battery with a module energy capacity equal to 0.36 MWh. These batteries are remarkably cost-efficient compared to super-capacitors and flywheels (Hittinger et al., 2010 ), and their fast response is fundamental to smooth wind-power changes. We consider 10 years of real-data hourly wind speed to obtain the power production of a hypothetical wind turbine located in Sardinia. As done in D’Amico et al. ( 2022a ), we transform the wind speed data into wind power production by means of the function
where \(\underline{v}\) is the cut-in wind speed, \(\overline{v}\) is the cut-out wind speed, \(v_r\) is the rated velocity, and \(P_r\) is the rated capacity of the wind turbine. We set \(\underline{v} = 4\,m/s\) , \(\overline{v} = 25\,m/s\) , \(v_r = 13\,m/s\) , and \(P_r =2MW\) (D’Amico et al., 2022a ; Vergine et al., 2022 ).
The penalty fees are set to \(x_{+1} = 21.52\) €/MWh and \(x_{-1} = 26.50\) €/MWh. These values are the ones used in Hittinger et al. ( 2014 ) after being made proportional to the average electricity price in Italy.
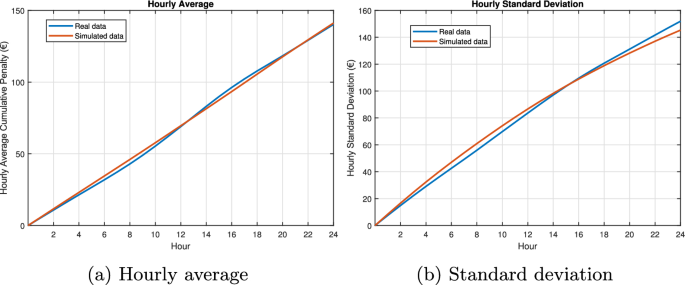
Hourly average cumulative penalty and standard deviation of real and simulated data with ramp-rate limitation percentage equal to \(1\%\) ( \(\ell = 0.02\) )
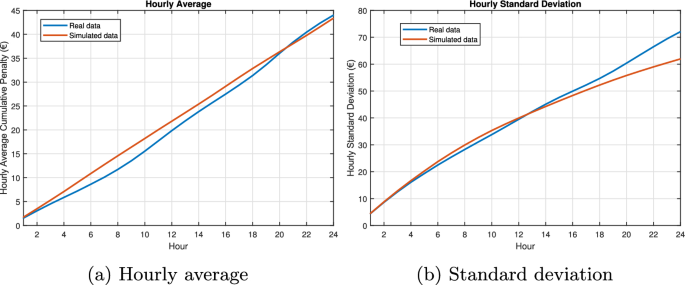
Hourly average cumulative penalty and standard deviation of real and simulated data with ramp-rate limitation percentage equal to \(5\%\) ( \(\ell = 0.1\) )
Looking at Figs. 9 , 10 and 11 , we can notice that the simulations are less accurate for the ramp-rate limitation of 7%. This is due to the fact that a higher percentage corresponds to a less strict limitation and, consecutively, to a smaller number of times that the system does not comply with it, which leads to a smaller data-set and, consequently to more biased estimations.
This fact is supported by the Mean Absolute Percentage Error (MAPE) calculated for the hourly average between real and simulated data. It is a metric that defines how accurate the forecasted quantities are in comparison with the actual quantities and represents the average of the absolute percentage errors. We obtain the values of 2.54, 11.34, and 20.18 for the ramp-rate limitations of \(1\%\) , \(5\%\) , and \(7\%\) , respectively. This confirms the behavior described above with the value of 2.54, which is very close to the value of 1.77 obtained in D’Amico et al. ( 2022a ) with the same ramp-rate limitation, where the proposed model needs a larger number of parameters, being the charge/discharge values independent and not identically distributed at each time within a sojourn time length. The model proposed in this work gives similar results but captures better the correlation structure in the sample charge/discharge paths. The second-order moment is characterized by greater but contained values of MAPE, 5.56, 5.92, and 8.35 for the three studied limitations.
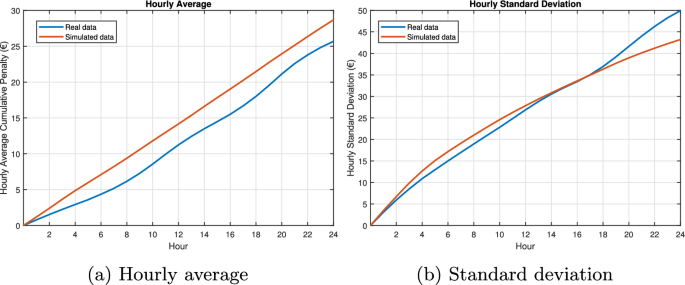
Hourly average cumulative penalty and standard deviation of real and simulated data with ramp rate limitation percentage equal to \(7\%\) ( \(\ell = 0.14\) )
6 Concluding remarks
We applied a discrete-time semi-Markov process to model the operations of charge and discharge of a battery storage system connected to a wind farm under a ramp-rate limitation strategy. Within each charging/discharging period, we model the charge/discharge process as a modified Brownian bridge with three parameters. The resulting semi-Markov-modulated modified Brownian bridge model was used, via Monte Carlo simulation, to estimate the first and second-order moments of the cumulative discounted penalty coming from violating up-ramp and down-ramp limitations. Not only the estimations are accurate when compared to real data, but they resemble the results obtained by D’Amico et al. ( 2022a ), where the authors used a model with a large number of parameters. In particular, the results show average daily losses ranging from almost 30€ for a ramp rate limitation of \(7\%\) up to almost 150€ for the more strict limitation of \(1\%\) .
Our results can be used to improve the management of the wind farm, since they allow us to obtain detailed information about the state of charge of the battery energy system, as well as the penalty dynamics associated to a ramp-rate limitation policy. The two algorithms we propose provide an accurate calculation of these variables over time.
Potential extensions of this work include exploring different limitation strategies and storage system technologies and estimating higher moments of the cumulative penalty process. The theoretical calculation of the cumulative penalty process moments is also a worthy path to explore. Finally, using the continuous-time version of the Brownian bridge process in ( 12 ) and ( 13 ), one might be able to come up with a continuous-time model for the battery charges/discharges.
This work represents the first step for alluring wind-power producers into accepting ramp-rate policies, by designing effective incentive systems to compensate the potential associated penalties. These systems have the complementary objective of ensuring the stability of the network by charging costs not only to wind-energy producers.
Abdullah, M. A., Muttaqi, K. M., Sutanto, D., & Agalgaonkar, A. P. (2014). An effective power dispatch control strategy to improve generation schedulability and supply reliability of a wind farm using a battery energy storage system. IEEE Transactions on Sustainable Energy, 6 (3), 1093–1102.
Article Google Scholar
An, X., Jiang, D., Zhao, M., & Liu, C. (2012). Short-term prediction of wind power using EMD and chaotic theory. Communications in Nonlinear Science and Numerical Simulation, 17 (2), 1036–1042.
Biancardi, M., Bufalo, M., Di Bari, A., & Villani, G. (2023). Flexibility to switch project size: A real option application for photovoltaic investment valuation. Communications in Nonlinear Science and Numerical Simulation, 116 , 106869.
Bossavy, A., Girard, R., & Kariniotakis, G. (2015). An edge model for the evaluation of wind power ramps characterization approaches. Wind Energy, 18 (7), 1169–1184.
Chen, P., Pedersen, T., Bak-Jensen, B., & Chen, Z. (2009). Arima-based time series model of stochastic wind power generation. IEEE Transactions on Power Systems, 25 (2), 667–676.
Cui, Y., Chen, Z., He, Y., Xiong, X., & Li, F. (2023). An algorithm for forecasting day-ahead wind power via novel long short-term memory and wind power ramp events. Energy, 263 , 125888.
Cui, Y., He, Y., Xiong, X., Chen, Z., Li, F., Xu, T., & Zhang, F. (2021). Algorithm for identifying wind power ramp events via novel improved dynamic swinging door. Renewable Energy, 171 , 542–556.
Cui, M., Zhang, J., Wang, Q., Krishnan, V., & Hodge, B.-M. (2017). A data-driven methodology for probabilistic wind power ramp forecasting. IEEE Transactions on Smart Grid, 10 (2), 1326–1338.
D’Amico, G., Petroni, F., & Prattico, F. (2013a). First and second order semi-Markov chains for wind speed modeling. Physica A: Statistical Mechanics and its Applications, 392 (5), 1194–1201.
D’Amico, G., Petroni, F., & Prattico, F. (2013b). Wind speed modeled as an indexed semi-Markov process. Environmetrics, 24 (6), 367–376.
D’Amico, G., Petroni, F., & Vergine, S. (2021). An analysis of a storage system for a wind farm with ramp-rate limitation. Energies, 14 (13), 4066.
D’Amico, G., Petroni, F., & Vergine, S. (2022a). Modelling and simulation of a storage system connected to a wind farm under ramp-rate limitation. International Journal of Modelling and Simulation, 43 (6), 1021–1040.
D’Amico, G., Petroni, F., & Vergine, S. (2022b). Ramp rate limitation of wind power: An overview. Energies, 15 (16), 5850.
Feinberg, E. A. (1994). Constrained semi-Markov decision processes with average rewards. Zeitschrift für Operations Research, 39 (3), 257–288.
Google Scholar
Frate, G. F., Ferrari, L., & Desideri, U. (2020). Impact of forecast uncertainty on wind farm profitability. Journal of Engineering for Gas Turbines and Power, 142 (4), 041018.
Gallego-Castillo, C., Cuerva-Tejero, A., & Lopez-Garcia, O. (2015). A review on the recent history of wind power ramp forecasting. Renewable and Sustainable Energy Reviews, 52 , 1148–1157.
Geenens, G., Charpentier, A., & Paindaveine, D. (2017). Probit transformation for nonparametric kernel estimation of the copula density. Bernoulli, 23 (3), 1848–1873. https://doi.org/10.3150/15-BEJ798
Grassi, G., & Vecchio, P. (2010). Wind energy prediction using a two-hidden layer neural network. Communications in Nonlinear Science and Numerical Simulation, 15 (9), 2262–2266.
Hittinger, E., Apt, J., & Whitacre, J. (2014). The effect of variability-mitigating market rules on the operation of wind power plants. Energy Systems, 5 (4), 737–766.
Hittinger, E., Whitacre, J., & Apt, J. (2010). Compensating for wind variability using co-located natural gas generation and energy storage. Energy Systems, 1 (4), 417–439.
Janzen, R., Davis, M., & Kumar, A. (2020). Greenhouse gas emission abatement potential and associated costs of integrating renewable and low carbon energy technologies into the Canadian oil sands. Journal of Cleaner Production, 272 , 122820.
Khalid, M., & Savkin, A. V. (2010). A model predictive control approach to the problem of wind power smoothing with controlled battery storage. Renewable Energy, 35 (7), 1520–1526.
Lee, D., & Baldick, R. (2012). Limiting ramp rate of wind power output using a battery based on the variance gamma process. In: Conf. Renew. Energies Power (pp. 1–6). Citeseer.
Li, H., Chen, D., Arzaghi, E., Abbassi, R., Kilicman, A., Caraballo, T., Patelli, E., Gao, X., & Xu, B. (2019). Dynamic safety assessment of a nonlinear pumped-storage generating system in a transient process. Communications in Nonlinear Science and Numerical Simulation, 67 , 192–202.
Lone, S. A., & Mufti, M. (2008). Modelling and simulation of a stand-alone hybrid power generation system incorporating redox flow battery storage system. International Journal of Modelling and Simulation, 28 (3), 337–346.
Medina, L., Castro, P., Kreutzmann, A.-K., & Rojas-Perilla, N. (2018). Trafo: Estimation, Comparison and Selection of Transformations. R package version 1.0.1. https://CRAN.R-project.org/package=trafo
Nagler, T. (2014). Kernel methods for vine copula estimation. PhD thesis, Department of Mathematics. Technische Universität München.
Nagler, T. (2022). Kdevine: Multivariate Kernel Density Estimation with Vine Copulas. R package version 0.4.4. https://CRAN.R-project.org/package=kdevine
Papadopoulou, A. A., Tsaklidis, G., McClean, S., & Garg, L. (2012). On the moments and the distribution of the cost of a semi Markov model for healthcare systems. Methodology and Computing in Applied Probability, 14 (3), 717–737.
Razmjoo, A., Kaigutha, L. G., Rad, M. V., Marzband, M., Davarpanah, A., & Denai, M. (2021). A technical analysis investigating energy sustainability utilizing reliable renewable energy sources to reduce co2 emissions in a high potential area. Renewable Energy, 164 , 46–57.
Teleke, S., Baran, M. E., Huang, A. Q., Bhattacharya, S., & Anderson, L. (2009). Control strategies for battery energy storage for wind farm dispatching. IEEE Transactions on Energy Conversion, 24 (3), 725–732.
Vergine, S., Álvarez-Arroyo, C., D’Amico, G., Escaño, J. M., & Alvarado-Barrios, L. (2022). Optimal management of a hybrid and isolated microgrid in a random setting. Energy Reports, 8 , 9402–9419.
Wan, Y. (2011). Analysis of wind power ramping behavior in ercot. Technical report, National Renewable Energy Lab. (NREL).
Zheng, X., Yang, S., Ye, Y., & Wang, J. (2022). Offshore wind power ramp prediction based on optimal combination model. Energy Sources, Part A: Recovery, Utilization, and Environmental Effects, 44 (2), 4334–4348.
Download references
Acknowledgements
Guglielmo D’Amico and Bernardo D’Auria are members of the Gruppo Nazionale Calcolo Scientifico-Istituto Nazionale di Alta Matematica (GNCS-INdAM). The authors thank the anonymous referees for their comments, which helped in improving the quality of the manuscript.
Open access funding provided by Università Politecnica delle Marche within the CRUI-CARE Agreement. This research was partially supported by the Spanish Ministerio de Asuntos Económicos y Transformación Digital grant PID2020-116694GB-I00. The first author acknowledges the financial support of the Universidad Carlos III de Madrid, by the grant “Ayuda para la movilidad de investigadores/as de la UC3M en centros de investigación nacionales y extranjeros”, from the program “Programa Propio de Investigación”. The last three authors acknowledge the financial support from the European Union - NextGenerationEU program MUR PRIN 2022 n. 2022ETEHRM “Stochastic models and techniques for the management of wind farms and power systems” by the Italian Ministero dell’Universitá e della Ricerca. The third author also acknowledges the partial financial support from the program MUR PRIN 2022 PNRR n. P20224TM7Z "Probabilistic methods for energy transition" by the Italian Ministero dell’Universitá e della Ricerca.
Author information
Abel Azze, Guglielmo D’Amico, Bernardo D’Auria and Salvatore Vergine have contributed equally to this work.
Authors and Affiliations
Department of Quantitative Methods, CUNEF Universidad, Calle Pirineos 55, 28040, Madrid, Spain
Department of Economics, University G. d’Annunzio of Chieti–Pescara, Viale Pindaro, 42, 65127, Pescara, Italy
Guglielmo D’Amico
Department of Mathematics “Tullio Levi Civita”, University of Padova, Via Trieste 63, 35121, Padova, Italy
Bernardo D’Auria
Department of Management, Marche Polytechnic University, Piazzale R. Martelli 8, 60121, Ancona, Italy
Salvatore Vergine
You can also search for this author in PubMed Google Scholar
Corresponding author
Correspondence to Salvatore Vergine .
Ethics declarations
Conflict of interest.
The authors declare that they have no conflict of interest.
Ethics approval
This article does not contain any studies with human participants or animals performed by any of the authors.
Consent to participate
Not applicable.
Additional information
Publisher's note.
Springer Nature remains neutral with regard to jurisdictional claims in published maps and institutional affiliations.
Rights and permissions
Open Access This article is licensed under a Creative Commons Attribution 4.0 International License, which permits use, sharing, adaptation, distribution and reproduction in any medium or format, as long as you give appropriate credit to the original author(s) and the source, provide a link to the Creative Commons licence, and indicate if changes were made. The images or other third party material in this article are included in the article's Creative Commons licence, unless indicated otherwise in a credit line to the material. If material is not included in the article's Creative Commons licence and your intended use is not permitted by statutory regulation or exceeds the permitted use, you will need to obtain permission directly from the copyright holder. To view a copy of this licence, visit http://creativecommons.org/licenses/by/4.0/ .
Reprints and permissions
About this article
Azze, A., D’Amico, G., D’Auria, B. et al. Modelling a storage system of a wind farm with a ramp-rate limitation: a semi-Markov modulated Brownian bridge approach. Ann Oper Res (2024). https://doi.org/10.1007/s10479-024-06236-6
Download citation
Received : 09 March 2024
Accepted : 16 August 2024
Published : 06 September 2024
DOI : https://doi.org/10.1007/s10479-024-06236-6
Share this article
Anyone you share the following link with will be able to read this content:
Sorry, a shareable link is not currently available for this article.
Provided by the Springer Nature SharedIt content-sharing initiative
- Brownian bridge
- Monte Carlo simulation
- Power ramping
- Semi-Markov process
- Find a journal
- Publish with us
- Track your research
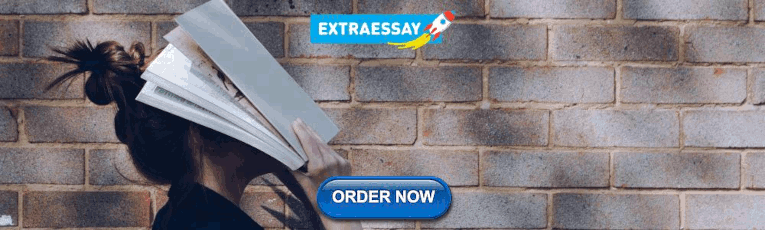
IMAGES
VIDEO
COMMENTS
How to Write Limitations of the Study (with examples)
Organizing Your Social Sciences Research Paper
Limitations in Research. Limitations in research refer to the factors that may affect the results, conclusions, and generalizability of a study. These limitations can arise from various sources, such as the design of the study, the sampling methods used, the measurement tools employed, and the limitations of the data analysis techniques.
Research limitations in a typical dissertation may relate to the following points: 1. Formulation of research aims and objectives. You might have formulated research aims and objectives too broadly. You can specify in which ways the formulation of research aims and objectives could be narrowed so that the level of focus of the study could be ...
Methodological Limitations Dr Andrew Booth (a) and Professor Jane Noyes (b) (a) Reader, School of Health and Related Research (ScHARR), University of Sheffield, UK. [email protected] (b) Professor in Health and Social Services Research and Child Health. School of Medical and Health Sciences. Bangor University, Wales. [email protected]
Research methodology formats can vary depending on the specific requirements of the research project, but the following is a basic example of a structure for a research methodology section: ... Identify any potential limitations of the research methodology and how they may impact the results and conclusions; VII. Conclusion.
Whether you're working on a dissertation, thesis or any other type of formal academic research, remember the five most common research limitations and interpret your data while keeping them in mind. Access to Information (literature and data) Time and money. Sample size and composition. Research design and methodology.
Understanding Limitations in Research
Literature review as a research methodology: An overview ...
The methods used in many methodological studies have been borrowed from systematic and scoping reviews. This practice has influenced the direction of the field, with many methodological studies including searches of electronic databases, screening of records, duplicate data extraction and assessments of risk of bias in the included studies.
What Is a Research Methodology? | Steps & Tips
Generic Qualitative Approaches: Pitfalls and Benefits of ...
What are the limitations in research and how to write them?
sentence tha. signals what you're about to discu. s. For example:"Our study had some limitations."Then, provide a concise sentence or two identifying each limitation and explaining how the limitation may have affected the quality. of the study. s findings and/or their applicability. For example:"First, owing to the rarity of the ...
The limitations of the study are those characteristics of design or methodology that impacted or influenced the interpretation of the findings from your research. They are the constraints on generalizability, applications to practice, and/or utility of findings that are the result of the ways in which you initially chose to design the study and ...
What is Research Methodology? Definition, Types, and ...
21 Research Limitations Examples (2024)
Strengths and Limitations of Qualitative and Quantitative ...
This chapter provides an overview of the three research questions used by the authors to guide the research. Moreover, it provides insight into the nature of qualitative research and the use of the two models to guide the analysis, namely, the Federal Qualitative Secondary Data Case Study Triangulation Model, and the York Intelligence Red Team ...
Limitations of the Study - Organizing Academic Research ...
Systematic reviews: Brief overview of methods, limitations, ...
A quick look through the articles in this issue offers a handy instant view of the focus of current research into learning with technologies. Educational researchers are overwhelmingly keen on using technology to flip learning, to bring context into the classroom through Virtual and Augmented Reality, and to use enquiry or problem-based scenarios and game-based activities for collaborative and ...
Writing the limitations of the research papers is often assumed to require lots of effort. However, identifying the limitations of the study can help structure the research better. Therefore, do not underestimate the importance of research study limitations. 3. Opportunity to make suggestions for further research.
In this section we are going to present the various types of methods and tools dedicated to the analysis of a single trait. These include tools for meta-analysis, tools for the estimation of heritability, tools for implementing gene-based tests, gene set methods and fine mapping methods.. Meta-analysis. One of the most obvious uses of GWAS summary data is to combine them and perform a meta ...
There are some limitations in this study that could provide promising areas for future research. First, we used the self-rated performance scale. This can provide information about how employees assess their own performance, and thus, we may not conclude that the performance ratings are the reflection of supervisor's evaluation of job ...
High-throughput omics technologies have dramatically changed biological research, providing unprecedented insights into the complexity of living systems. This review presents a comprehensive examination of the current landscape of high-throughput omics pipelines, covering key technologies, data integration techniques and their diverse applications. It looks at advances in next-generation ...
We propose a new methodology to simulate the discounted penalty applied to a wind-farm operator by violating ramp-rate limitation policies. It is assumed that the operator manages a wind turbine plugged into a battery, which either provides or stores energy on demand to avoid ramp-up and ramp-down events. The battery stages, namely charging, discharging, or neutral, are modeled as a semi ...