Regression Analysis
- Reference work entry
- First Online: 03 December 2021
- Cite this reference work entry
- Bernd Skiera 4 ,
- Jochen Reiner 4 &
- Sönke Albers 5
8375 Accesses
5 Citations
Linear regression analysis is one of the most important statistical methods. It examines the linear relationship between a metric-scaled dependent variable (also called endogenous, explained, response, or predicted variable) and one or more metric-scaled independent variables (also called exogenous, explanatory, control, or predictor variable). We illustrate how regression analysis work and how it supports marketing decisions, e.g., the derivation of an optimal marketing mix. We also outline how to use linear regression analysis to estimate nonlinear functions such as a multiplicative sales response function. Furthermore, we show how to use the results of a regression to calculate elasticities and to identify outliers and discuss in details the problems that occur in case of autocorrelation, multicollinearity and heteroscedasticity. We use a numerical example to illustrate in detail all calculations and use this numerical example to outline the problems that occur in case of endogeneity.
This is a preview of subscription content, log in via an institution to check access.
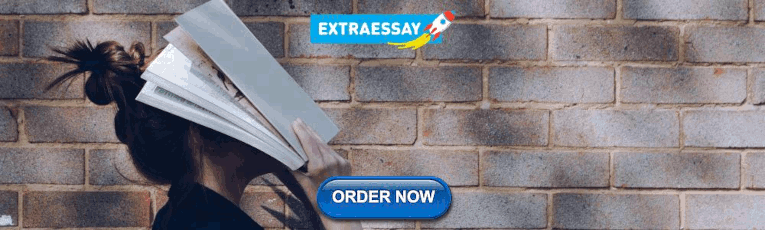
Access this chapter
Subscribe and save.
- Get 10 units per month
- Download Article/Chapter or eBook
- 1 Unit = 1 Article or 1 Chapter
- Cancel anytime
- Available as PDF
- Read on any device
- Instant download
- Own it forever
- Available as EPUB and PDF
- Durable hardcover edition
- Dispatched in 3 to 5 business days
- Free shipping worldwide - see info
Tax calculation will be finalised at checkout
Purchases are for personal use only
Institutional subscriptions
Similar content being viewed by others
Estimation and Testing
Albers, S. (2012). Optimizable and implementable aggregate response modeling for marketing decision support. International Journal of Research in Marketing, 29 (2), 111–122.
Article Google Scholar
Albers, S., Mantrala, M. K., & Sridhar, S. (2010). Personal selling elasticities: A meta-analysis. Journal of Marketing Research, 47 (5), 840–853.
Assmus, G., Farley, J. W., & Lehmann, D. R. (1984). How advertising affects sales: A meta-analysis of econometric results. Journal of Marketing Research, 21 (1), 65–74.
Bijmolt, T. H. A., van Heerde, H., & Pieters, R. G. M. (2005). New empirical generalizations on the determinants of price elasticity. Journal of Marketing Research, 42 (2), 141–156.
Chatterjee, S., & Hadi, A. S. (1986). Influential observations, high leverage points, and outliers in linear regressions. Statistical Science, 1 (3), 379–416.
Google Scholar
Greene, W. H. (2008). Econometric analysis (6th ed.). Upper Saddle River: Pearson.
Gujarati, D. N. (2003). Basic econometrics (4th ed.). New York: McGraw Hill.
Hair, J. F., Black, W. C., Babin, J. B., & Anderson, R. E. (2014). Multivariate data analysis (7th ed.). Upper Saddle River: Pearson.
Hair, J. F., Hult, G. T. M., Ringle, C. M., & Sarstedt, M. (2017). A primer on partial least squares structural equation modeling (PLS-SEM) (2nd ed.). Thousand Oaks: Sage.
Hanssens, D. M., Parsons, L. J., & Schultz, R. L. (1990). Market response models: Econometric and time series analysis . Boston: Springer.
Hsiao, C. (2014). Analysis of panel data (3rd ed.). Cambridge: Cambridge University Press.
Book Google Scholar
Irwin, J. R., & McClelland, G. H. (2001). Misleading heuristics and moderated multiple regression models. Journal of Marketing Research, 38 (1), 100–109.
Koutsoyiannis, A. (1977). Theory of econometrics (2nd ed.). Houndmills: MacMillan.
Laurent, G. (2013). EMAC distinguished marketing scholar 2012: Respect the data! International Journal of Research in Marketing, 30 (4), 323–334.
Leeflang, P. S. H., Wittink, D. R., Wedel, M., & Neart, P. A. (2000). Building models for marketing decisions . Berlin: Kluwer.
Lodish, L. L., Abraham, M. M., Kalmenson, S., Livelsberger, J., Lubetkin, B., Richardson, B., & Stevens, M. E. (1995). How TV advertising works: A meta-analysis of 389 real world split cable T. V. advertising experiments. Journal of Marketing Research, 32 (2), 125–139.
Pindyck, R. S., & Rubenfeld, D. (1998). Econometric models and econometric forecasts (4th ed.). New York: McGraw-Hill.
Sethuraman, R., Tellis, G. J., & Briesch, R. A. (2011). How well does advertising work? Generalizations from meta-analysis of brand advertising elasticities. Journal of Marketing Research, 48 (3), 457–471.
Snijders, T. A. B., & Bosker, R. J. (2012). Multilevel analysis: An introduction to basic and advanced multilevel modeling (2nd ed.). London: Sage.
Stock, J., & Watson, M. (2015). Introduction to econometrics (3rd ed.). Upper Saddle River: Pearson.
Tellis, G. J. (1988). The price sensitivity of selective demand: A meta-analysis of econometric models of sales. Journal of Marketing Research, 25 (4), 391–404.
White, H. (1980). A heteroskedasticity-consistent covariance matrix estimator and a direct test for heteroskedasticity. Econometrica, 48 (4), 817–838.
Wooldridge, J. M. (2009). Introductory econometrics: A modern approach (4th ed.). Mason: South-Western Cengage.
Download references
Author information
Authors and affiliations.
Goethe University Frankfurt, Frankfurt, Germany
Bernd Skiera & Jochen Reiner
Kuehne Logistics University, Hamburg, Germany
Sönke Albers
You can also search for this author in PubMed Google Scholar
Corresponding author
Correspondence to Bernd Skiera .
Editor information
Editors and affiliations.
Department of Business-to-Business Marketing, Sales, and Pricing, University of Mannheim, Mannheim, Germany
Christian Homburg
Department of Marketing & Sales Research Group, Karlsruhe Institute of Technology (KIT), Karlsruhe, Germany
Martin Klarmann
Marketing & Sales Department, University of Mannheim, Mannheim, Germany
Arnd Vomberg
Rights and permissions
Reprints and permissions
Copyright information
© 2022 Springer Nature Switzerland AG
About this entry
Cite this entry.
Skiera, B., Reiner, J., Albers, S. (2022). Regression Analysis. In: Homburg, C., Klarmann, M., Vomberg, A. (eds) Handbook of Market Research. Springer, Cham. https://doi.org/10.1007/978-3-319-57413-4_17
Download citation
DOI : https://doi.org/10.1007/978-3-319-57413-4_17
Published : 03 December 2021
Publisher Name : Springer, Cham
Print ISBN : 978-3-319-57411-0
Online ISBN : 978-3-319-57413-4
eBook Packages : Business and Management Reference Module Humanities and Social Sciences Reference Module Business, Economics and Social Sciences
Share this entry
Anyone you share the following link with will be able to read this content:
Sorry, a shareable link is not currently available for this article.
Provided by the Springer Nature SharedIt content-sharing initiative
- Publish with us
Policies and ethics
- Find a journal
- Track your research
Multiple Regression Analysis Example with Conceptual Framework
Data analysis using multiple regression analysis is a fairly common tool used in statistics. Many graduate students find this too complicated to understand. However, this is not that difficult to do, especially with computers as everyday household items nowadays. You can now quickly analyze more than just two sets of variables in your research using multiple regression analysis.
How is multiple regression analysis done? This article explains this handy statistical test when dealing with many variables, then provides an example of a research using multiple regression analysis to show how it works. It explains how research using multiple regression analysis is conducted.
Multiple regression is often confused with multivariate regression. Multivariate regression, while also using several variables, deals with more than one dependent variable . Karen Grace-Martin clearly explains the difference in her post on the difference between the Multiple Regression Model and Multivariate Regression Model .
Table of Contents
Statistical software applications used in computing multiple regression analysis.
Using multiple regression analysis requires a dedicated statistical software like the popular Statistical Package for the Social Sciences (SPSS) , Statistica, Microstat, and open-source statistical software applications like SOFA statistics and Jasp, among other sophisticated statistical packages.
However, a standard spreadsheet application like Microsoft Excel can help you compute and model the relationship between the dependent variable and a set of predictor or independent variables. But you cannot do this without activating first the setting of statistical tools that ship with MS Excel.
Activating MS Excel
Multiple Regression Analysis Example
The study pertains to identifying the factors predicting a current problem among high school students, the long hours they spend online for a variety of reasons. The purpose is to address many parents’ concerns about their difficulty of weaning their children away from the lures of online gaming, social networking, and other engaging virtual activities.
Review of Literature on Internet Use and Its Effect on Children
Given that there is a need to use a computer to analyze multiple variable data, a principal who is nearing retirement was “forced” to buy a laptop, as she had none. Anyhow, she is very much open-minded and performed the class activities that require data analysis with much enthusiasm.
The Research on High School Students’ Use of the Internet
“Is there a significant relationship between the total number of hours spent online and the students’ age, gender, relationship with their mother, and relationship with their father?”
Although many studies have identified factors that influence the use of the internet, it is standard practice to include the respondents’ profile among the set of predictor or independent variables. Hence, the standard variables age and gender are included in the multiple regression analysis.
Findings of the Research Using Multiple Regression Analysis
The number of hours spent online relates significantly to the number of hours spent by a parent, specifically the mother, with her child. These two factors are inversely or negatively correlated.
The number of hours spent by the children online relates significantly to the mother’s number of hours interacting with their children.
But establishing a close bond between mother and child is a good start. Undertaking more investigations along this research concern will help strengthen the findings of this study.
Thus, this example of a research using multiple regression analysis streamlines solutions and focuses on those influential factors that must be given attention.
Related Posts
5 time management strategies for researchers, effective strategies for engineers writing technical reports in english, using a matrix to write your research proposal, about the author, patrick regoniel.
the example is good but lacks the table of regression results. With the tables, a student could learn more on how to interpret regression results
SimplyEducate.Me Privacy Policy
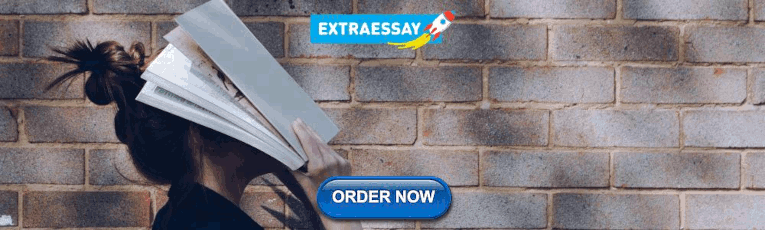
Log in using your username and password
- Search More Search for this keyword Advanced search
- Latest content
- Current issue
- Write for Us
- BMJ Journals
You are here
- Volume 24, Issue 4
- Understanding and interpreting regression analysis
- Article Text
- Article info
- Citation Tools
- Rapid Responses
- Article metrics

- http://orcid.org/0000-0002-7839-8130 Parveen Ali 1 , 2 ,
- http://orcid.org/0000-0003-0157-5319 Ahtisham Younas 3 , 4
- 1 School of Nursing and Midwifery , University of Sheffield , Sheffield , South Yorkshire , UK
- 2 Sheffiled University Interpersonal Violence Research Group , The University of Sheffiled SEAS , Sheffield , UK
- 3 Faculty of Nursing , Memorial University of Newfoundland , St. John's , Newfoundland and Labrador , Canada
- 4 Swat College of Nursing , Mingora, Swat , Pakistan
- Correspondence to Ahtisham Younas, Memorial University of Newfoundland, St. John's, NL A1C 5S7, Canada; ay6133{at}mun.ca
https://doi.org/10.1136/ebnurs-2021-103425
Statistics from Altmetric.com
Request permissions.
If you wish to reuse any or all of this article please use the link below which will take you to the Copyright Clearance Center’s RightsLink service. You will be able to get a quick price and instant permission to reuse the content in many different ways.
- statistics & research methods
Introduction
A nurse educator is interested in finding out the academic and non-academic predictors of success in nursing students. Given the complexity of educational and clinical learning environments, demographic, clinical and academic factors (age, gender, previous educational training, personal stressors, learning demands, motivation, assignment workload, etc) influencing nursing students’ success, she was able to list various potential factors contributing towards success relatively easily. Nevertheless, not all of the identified factors will be plausible predictors of increased success. Therefore, she could use a powerful statistical procedure called regression analysis to identify whether the likelihood of increased success is influenced by factors such as age, stressors, learning demands, motivation and education.
What is regression?
Purposes of regression analysis.
Regression analysis has four primary purposes: description, estimation, prediction and control. 1 , 2 By description, regression can explain the relationship between dependent and independent variables. Estimation means that by using the observed values of independent variables, the value of dependent variable can be estimated. 2 Regression analysis can be useful for predicting the outcomes and changes in dependent variables based on the relationships of dependent and independent variables. Finally, regression enables in controlling the effect of one or more independent variables while investigating the relationship of one independent variable with the dependent variable. 1
Types of regression analyses
There are commonly three types of regression analyses, namely, linear, logistic and multiple regression. The differences among these types are outlined in table 1 in terms of their purpose, nature of dependent and independent variables, underlying assumptions, and nature of curve. 1 , 3 However, more detailed discussion for linear regression is presented as follows.
- View inline
Comparison of linear, logistic and multiple regression
Linear regression and interpretation
Linear regression analysis involves examining the relationship between one independent and dependent variable. Statistically, the relationship between one independent variable (x) and a dependent variable (y) is expressed as: y= β 0 + β 1 x+ε. In this equation, β 0 is the y intercept and refers to the estimated value of y when x is equal to 0. The coefficient β 1 is the regression coefficient and denotes that the estimated increase in the dependent variable for every unit increase in the independent variable. The symbol ε is a random error component and signifies imprecision of regression indicating that, in actual practice, the independent variables are cannot perfectly predict the change in any dependent variable. 1 Multiple linear regression follows the same logic as univariate linear regression except (a) multiple regression, there are more than one independent variable and (b) there should be non-collinearity among the independent variables.
Factors affecting regression
Linear and multiple regression analyses are affected by factors, namely, sample size, missing data and the nature of sample. 2
Small sample size may only demonstrate connections among variables with strong relationship. Therefore, sample size must be chosen based on the number of independent variables and expect strength of relationship.
Many missing values in the data set may affect the sample size. Therefore, all the missing values should be adequately dealt with before conducting regression analyses.
The subsamples within the larger sample may mask the actual effect of independent and dependent variables. Therefore, if subsamples are predefined, a regression within the sample could be used to detect true relationships. Otherwise, the analysis should be undertaken on the whole sample.
Building on her research interest mentioned in the beginning, let us consider a study by Ali and Naylor. 4 They were interested in identifying the academic and non-academic factors which predict the academic success of nursing diploma students. This purpose is consistent with one of the above-mentioned purposes of regression analysis (ie, prediction). Ali and Naylor’s chosen academic independent variables were preadmission qualification, previous academic performance and school type and the non-academic variables were age, gender, marital status and time gap. To achieve their purpose, they collected data from 628 nursing students between the age range of 15–34 years. They used both linear and multiple regression analyses to identify the predictors of student success. For analysis, they examined the relationship of academic and non-academic variables across different years of study and noted that academic factors accounted for 36.6%, 44.3% and 50.4% variability in academic success of students in year 1, year 2 and year 3, respectively. 4
Ali and Naylor presented the relationship among these variables using scatter plots, which are commonly used graphs for data display in regression analysis—see examples of various scatter plots in figure 1 . 4 In a scatter plot, the clustering of the dots denoted the strength of relationship, whereas the direction indicates the nature of relationships among variables as positive (ie, increase in one variable results in an increase in the other) and negative (ie, increase in one variable results in decrease in the other).
- Download figure
- Open in new tab
- Download powerpoint
An Example of Scatter Plot for Regression.
Table 2 presents the results of regression analysis for academic and non-academic variables for year 4 students’ success. The significant predictors of student success are denoted with a significant p value. For every, significant predictor, the beta value indicates the percentage increase in students’ academic success with one unit increase in the variable.
Regression model for the final year students (N=343)
Conclusions
Regression analysis is a powerful and useful statistical procedure with many implications for nursing research. It enables researchers to describe, predict and estimate the relationships and draw plausible conclusions about the interrelated variables in relation to any studied phenomena. Regression also allows for controlling one or more variables when researchers are interested in examining the relationship among specific variables. Some of the key considerations are presented that may be useful for researchers undertaking regression analysis. While planning and conducting regression analysis, researchers should consider the type and number of dependent and independent variables as well as the nature and size of sample. Choosing a wrong type of regression analysis with small sample may result in erroneous conclusions about the studied phenomenon.
Ethics statements
Patient consent for publication.
Not required.
- Montgomery DC ,
- Schneider A ,
Twitter @parveenazamali, @@Ahtisham04
Funding The authors have not declared a specific grant for this research from any funding agency in the public, commercial or not-for-profit sectors.
Competing interests None declared.
Provenance and peer review Commissioned; internally peer reviewed.
Read the full text or download the PDF:

An official website of the United States government
The .gov means it’s official. Federal government websites often end in .gov or .mil. Before sharing sensitive information, make sure you’re on a federal government site.
The site is secure. The https:// ensures that you are connecting to the official website and that any information you provide is encrypted and transmitted securely.
- Publications
- Account settings
Preview improvements coming to the PMC website in October 2024. Learn More or Try it out now .
- Advanced Search
- Journal List
- Cardiopulm Phys Ther J
- v.20(3); 2009 Sep
Regression Analysis for Prediction: Understanding the Process
Phillip b palmer.
1 Hardin-Simmons University, Department of Physical Therapy, Abilene, TX
Dennis G O'Connell
2 Hardin-Simmons University, Department of Physical Therapy, Abilene, TX
Research related to cardiorespiratory fitness often uses regression analysis in order to predict cardiorespiratory status or future outcomes. Reading these studies can be tedious and difficult unless the reader has a thorough understanding of the processes used in the analysis. This feature seeks to “simplify” the process of regression analysis for prediction in order to help readers understand this type of study more easily. Examples of the use of this statistical technique are provided in order to facilitate better understanding.
INTRODUCTION
Graded, maximal exercise tests that directly measure maximum oxygen consumption (VO 2 max) are impractical in most physical therapy clinics because they require expensive equipment and personnel trained to administer the tests. Performing these tests in the clinic may also require medical supervision; as a result researchers have sought to develop exercise and non-exercise models that would allow clinicians to predict VO 2 max without having to perform direct measurement of oxygen uptake. In most cases, the investigators utilize regression analysis to develop their prediction models.
Regression analysis is a statistical technique for determining the relationship between a single dependent (criterion) variable and one or more independent (predictor) variables. The analysis yields a predicted value for the criterion resulting from a linear combination of the predictors. According to Pedhazur, 15 regression analysis has 2 uses in scientific literature: prediction, including classification, and explanation. The following provides a brief review of the use of regression analysis for prediction. Specific emphasis is given to the selection of the predictor variables (assessing model efficiency and accuracy) and cross-validation (assessing model stability). The discussion is not intended to be exhaustive. For a more thorough explanation of regression analysis, the reader is encouraged to consult one of many books written about this statistical technique (eg, Fox; 5 Kleinbaum, Kupper, & Muller; 12 Pedhazur; 15 and Weisberg 16 ). Examples of the use of regression analysis for prediction are drawn from a study by Bradshaw et al. 3 In this study, the researchers' stated purpose was to develop an equation for prediction of cardiorespiratory fitness (CRF) based on non-exercise (N-EX) data.
SELECTING THE CRITERION (OUTCOME MEASURE)
The first step in regression analysis is to determine the criterion variable. Pedhazur 15 suggests that the criterion have acceptable measurement qualities (ie, reliability and validity). Bradshaw et al 3 used VO 2 max as the criterion of choice for their model and measured it using a maximum graded exercise test (GXT) developed by George. 6 George 6 indicated that his protocol for testing compared favorably with the Bruce protocol in terms of predictive ability and had good test-retest reliability ( ICC = .98 –.99). The American College of Sports Medicine indicates that measurement of VO 2 max is the “gold standard” for measuring cardiorespiratory fitness. 1 These facts support that the criterion selected by Bradshaw et al 3 was appropriate and meets the requirements for acceptable reliability and validity.
SELECTING THE PREDICTORS: MODEL EFFICIENCY
Once the criterion has been selected, predictor variables should be identified (model selection). The aim of model selection is to minimize the number of predictors which account for the maximum variance in the criterion. 15 In other words, the most efficient model maximizes the value of the coefficient of determination ( R 2 ). This coefficient estimates the amount of variance in the criterion score accounted for by a linear combination of the predictor variables. The higher the value is for R 2 , the less error or unexplained variance and, therefore, the better prediction. R 2 is dependent on the multiple correlation coefficient ( R ), which describes the relationship between the observed and predicted criterion scores. If there is no difference between the predicted and observed scores, R equals 1.00. This represents a perfect prediction with no error and no unexplained variance ( R 2 = 1.00). When R equals 0.00, there is no relationship between the predictor(s) and the criterion and no variance in scores has been explained ( R 2 = 0.00). The chosen variables cannot predict the criterion. The goal of model selection is, as stated previously, to develop a model that results in the highest estimated value for R 2 .
According to Pedhazur, 15 the value of R is often overestimated. The reasons for this are beyond the scope of this discussion; however, the degree of overestimation is affected by sample size. The larger the ratio is between the number of predictors and subjects, the larger the overestimation. To account for this, sample sizes should be large and there should be 15 to 30 subjects per predictor. 11 , 15 Of course, the most effective way to determine optimal sample size is through statistical power analysis. 11 , 15
Another method of determining the best model for prediction is to test the significance of adding one or more variables to the model using the partial F-test . This process, which is further discussed by Kleinbaum, Kupper, and Muller, 12 allows for exclusion of predictors that do not contribute significantly to the prediction, allowing determination of the most efficient model of prediction. In general, the partial F-test is similar to the F-test used in analysis of variance. It assesses the statistical significance of the difference between values for R 2 derived from 2 or more prediction models using a subset of the variables from the original equation. For example, Bradshaw et al 3 indicated that all variables contributed significantly to their prediction. Though the researchers do not detail the procedure used, it is highly likely that different models were tested, excluding one or more variables, and the resulting values for R 2 assessed for statistical difference.
Although the techniques discussed above are useful in determining the most efficient model for prediction, theory must be considered in choosing the appropriate variables. Previous research should be examined and predictors selected for which a relationship between the criterion and predictors has been established. 12 , 15
It is clear that Bradshaw et al 3 relied on theory and previous research to determine the variables to use in their prediction equation. The 5 variables they chose for inclusion–gender, age, body mass index (BMI), perceived functional ability (PFA), and physical activity rating (PAR)–had been shown in previous studies to contribute to the prediction of VO 2 max (eg, Heil et al; 8 George, Stone, & Burkett 7 ). These 5 predictors accounted for 87% ( R = .93, R 2 = .87 ) of the variance in the predicted values for VO 2 max. Based on a ratio of 1:20 (predictor:sample size), this estimate of R , and thus R 2 , is not likely to be overestimated. The researchers used changes in the value of R 2 to determine whether to include or exclude these or other variables. They reported that removal of perceived functional ability (PFA) as a variable resulted in a decrease in R from .93 to .89. Without this variable, the remaining 4 predictors would account for only 79% of the variance in VO 2 max. The investigators did note that each predictor variable contributed significantly ( p < .05 ) to the prediction of VO 2 max (see above discussion related to the partial F-test).
ASSESSING ACCURACY OF THE PREDICTION
Assessing accuracy of the model is best accomplished by analyzing the standard error of estimate ( SEE ) and the percentage that the SEE represents of the predicted mean ( SEE % ). The SEE represents the degree to which the predicted scores vary from the observed scores on the criterion measure, similar to the standard deviation used in other statistical procedures. According to Jackson, 10 lower values of the SEE indicate greater accuracy in prediction. Comparison of the SEE for different models using the same sample allows for determination of the most accurate model to use for prediction. SEE % is calculated by dividing the SEE by the mean of the criterion ( SEE /mean criterion) and can be used to compare different models derived from different samples.
Bradshaw et al 3 report a SEE of 3.44 mL·kg −1 ·min −1 (approximately 1 MET) using all 5 variables in the equation (gender, age, BMI, PFA, PA-R). When the PFA variable is removed from the model, leaving only 4 variables for the prediction (gender, age, BMI, PA-R), the SEE increases to 4.20 mL·kg −1 ·min −1 . The increase in the error term indicates that the model excluding PFA is less accurate in predicting VO 2 max. This is confirmed by the decrease in the value for R (see discussion above). The researchers compare their model of prediction with that of George, Stone, and Burkett, 7 indicating that their model is as accurate. It is not advisable to compare models based on the SEE if the data were collected from different samples as they were in these 2 studies. That type of comparison should be made using SEE %. Bradshaw and colleagues 3 report SEE % for their model (8.62%), but do not report values from other models in making comparisons.
Some advocate the use of statistics derived from the predicted residual sum of squares ( PRESS ) as a means of selecting predictors. 2 , 4 , 16 These statistics are used more often in cross-validation of models and will be discussed in greater detail later.
ASSESSING STABILITY OF THE MODEL FOR PREDICTION
Once the most efficient and accurate model for prediction has been determined, it is prudent that the model be assessed for stability. A model, or equation, is said to be “stable” if it can be applied to different samples from the same population without losing the accuracy of the prediction. This is accomplished through cross-validation of the model. Cross-validation determines how well the prediction model developed using one sample performs in another sample from the same population. Several methods can be employed for cross-validation, including the use of 2 independent samples, split samples, and PRESS -related statistics developed from the same sample.
Using 2 independent samples involves random selection of 2 groups from the same population. One group becomes the “training” or “exploratory” group used for establishing the model of prediction. 5 The second group, the “confirmatory” or “validatory” group is used to assess the model for stability. The researcher compares R 2 values from the 2 groups and assessment of “shrinkage,” the difference between the two values for R 2 , is used as an indicator of model stability. There is no rule of thumb for interpreting the differences, but Kleinbaum, Kupper, and Muller 12 suggest that “shrinkage” values of less than 0.10 indicate a stable model. While preferable, the use of independent samples is rarely used due to cost considerations.
A similar technique of cross-validation uses split samples. Once the sample has been selected from the population, it is randomly divided into 2 subgroups. One subgroup becomes the “exploratory” group and the other is used as the “validatory” group. Again, values for R 2 are compared and model stability is assessed by calculating “shrinkage.”
Holiday, Ballard, and McKeown 9 advocate the use of PRESS-related statistics for cross-validation of regression models as a means of dealing with the problems of data-splitting. The PRESS method is a jackknife analysis that is used to address the issue of estimate bias associated with the use of small sample sizes. 13 In general, a jackknife analysis calculates the desired test statistic multiple times with individual cases omitted from the calculations. In the case of the PRESS method, residuals, or the differences between the actual values of the criterion for each individual and the predicted value using the formula derived with the individual's data removed from the prediction, are calculated. The PRESS statistic is the sum of the squares of the residuals derived from these calculations and is similar to the sum of squares for the error (SS error ) used in analysis of variance (ANOVA). Myers 14 discusses the use of the PRESS statistic and describes in detail how it is calculated. The reader is referred to this text and the article by Holiday, Ballard, and McKeown 9 for additional information.
Once determined, the PRESS statistic can be used to calculate a modified form of R 2 and the SEE . R 2 PRESS is calculated using the following formula: R 2 PRESS = 1 – [ PRESS / SS total ], where SS total equals the sum of squares for the original regression equation. 14 Standard error of the estimate for PRESS ( SEE PRESS ) is calculated as follows: SEE PRESS =, where n equals the number of individual cases. 14 The smaller the difference between the 2 values for R 2 and SEE , the more stable the model for prediction. Bradshaw et al 3 used this technique in their investigation. They reported a value for R 2 PRESS of .83, a decrease of .04 from R 2 for their prediction model. Using the standard set by Kleinbaum, Kupper, and Muller, 12 the model developed by these researchers would appear to have stability, meaning it could be used for prediction in samples from the same population. This is further supported by the small difference between the SEE and the SEE PRESS , 3.44 and 3.63 mL·kg −1 ·min −1 , respectively.
COMPARING TWO DIFFERENT PREDICTION MODELS
A comparison of 2 different models for prediction may help to clarify the use of regression analysis in prediction. Table Table1 1 presents data from 2 studies and will be used in the following discussion.
Comparison of Two Non-exercise Models for Predicting CRF
Variables | Heil et al = 374 | Bradshaw et al = 100 |
---|---|---|
Intercept | 36.580 | 48.073 |
Gender (male = 1, female = 0) | 3.706 | 6.178 |
Age (years) | 0.558 | −0.246 |
Age | −7.81 E-3 | |
Percent body fat | −0.541 | |
Body mass index (kg-m ) | −0.619 | |
Activity code (0-7) | 1.347 | |
Physical activity rating (0–10) | 0.671 | |
Perceived functional abilty | 0.712 | |
) | ||
.88 (.77) | .93 (.87) | |
4.90·mL–kg ·min | 3.44 mL·kg min | |
12.7% | 8.6% |
As noted above, the first step is to select an appropriate criterion, or outcome measure. Bradshaw et al 3 selected VO 2 max as their criterion for measuring cardiorespiratory fitness. Heil et al 8 used VO 2 peak. These 2 measures are often considered to be the same, however, VO 2 peak assumes that conditions for measuring maximum oxygen consumption were not met. 17 It would be optimal to compare models based on the same criterion, but that is not essential, especially since both criteria measure cardiorespiratory fitness in much the same way.
The second step involves selection of variables for prediction. As can be seen in Table Table1, 1 , both groups of investigators selected 5 variables to use in their model. The 5 variables selected by Bradshaw et al 3 provide a better prediction based on the values for R 2 (.87 and .77), indicating that their model accounts for more variance (87% versus 77%) in the prediction than the model of Heil et al. 8 It should also be noted that the SEE calculated in the Bradshaw 3 model (3.44 mL·kg −1 ·min −1 ) is less than that reported by Heil et al 8 (4.90 mL·kg −1 ·min −1 ). Remember, however, that comparison of the SEE should only be made when both models are developed using samples from the same population. Comparing predictions developed from different populations can be accomplished using the SEE% . Review of values for the SEE% in Table Table1 1 would seem to indicate that the model developed by Bradshaw et al 3 is more accurate because the percentage of the mean value for VO 2 max represented by error is less than that reported by Heil et al. 8 In summary, the Bradshaw 3 model would appear to be more efficient, accounting for more variance in the prediction using the same number of variables. It would also appear to be more accurate based on comparison of the SEE% .
The 2 models cannot be compared based on stability of the models. Each set of researchers used different methods for cross-validation. Both models, however, appear to be relatively stable based on the data presented. A clinician can assume that either model would perform fairly well when applied to samples from the same populations as those used by the investigators.
The purpose of this brief review has been to demystify regression analysis for prediction by explaining it in simple terms and to demonstrate its use. When reviewing research articles in which regression analysis has been used for prediction, physical therapists should ensure that the: (1) criterion chosen for the study is appropriate and meets the standards for reliability and validity, (2) processes used by the investigators to assess both model efficiency and accuracy are appropriate, 3) predictors selected for use in the model are reasonable based on theory or previous research, and 4) investigators assessed model stability through a process of cross-validation, providing the opportunity for others to utilize the prediction model in different samples drawn from the same population.
Correlation and Regression Analysis Using SPSS
- December 2019

- Tribhuvan University
Discover the world's research
- 25+ million members
- 160+ million publication pages
- 2.3+ billion citations
- Hongsong Zhang
- El Saiid, Mohamed Nabil Mohame
- Saleh, Ashraf

- Cosmin Dobrin

- Asnani Mappa
- Mattalatta Mattalatta

- Kurniawati Sandra

- Saud Alsaqer
- Ihab M. Katar
- Abdelhakim Abdelhadi
- Marcin Stanuch

- D. C. Montgomery
- INT STAT REV
- M. Eileen Magnello
- Nandini Dendukuri

- D N Gujarati
- P D Gunasekar
- Recruit researchers
- Join for free
- Login Email Tip: Most researchers use their institutional email address as their ResearchGate login Password Forgot password? Keep me logged in Log in or Continue with Google Welcome back! Please log in. Email · Hint Tip: Most researchers use their institutional email address as their ResearchGate login Password Forgot password? Keep me logged in Log in or Continue with Google No account? Sign up
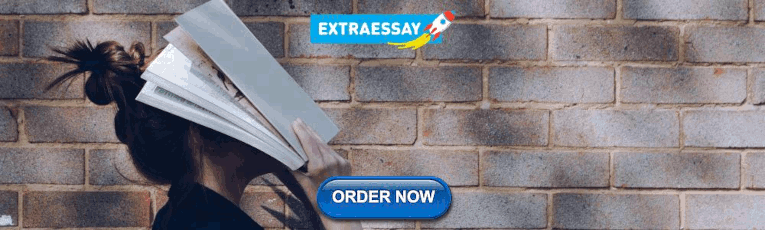
IMAGES
COMMENTS
How to interpret basic regression analysis results. What the issues with, and assumptions of regression analysis are. How to validate regression analysis results.
Recommended Citation Soule, Patrick. "Predicting Student Success: A Logistic Regression Analysis of Data From Multiple SIU-C Courses." (Fall 2017). This Article is brought to you for free and open access by the Graduate School at OpenSIUC. It has been accepted for inclusion in Research Papers by an authorized administrator of OpenSIUC.
Linear regression analysis is the most widely used of all statistical techniques. This article explains the basic concepts and explains how we can do linear regression calculations in SPSS and excel.
Regression models with one dependent variable and more than one independent variables are called multilinear regression. In this study, data for multilinear regression analysis is occur from Sakarya University Education Faculty student's lesson (measurement and evaluation, educational psychology, program development, counseling and ...
PDF | Regression analysis is a statistical technique for estimating the relationship among variables which have reason and result relation. Main focus... | Find, read and cite all the research you ...
The research methodology is based on statistical analysis, which in this paper includes the multiple regression analysis. This type of analysis is used for modeling and analyzing several variables.
There are various selection methods for linear regression modeling in order to specify how independent variables are entered into the analysis. By using different methods, a variety of regression models from the same set of variables could be constructed.
Abstract This paper is especially written for students and demonstrates the correct use of nominal and ordinal scaled variables in regression analysis by means of so-called 'dummy variables'. We start with examples of body mass index (BMI) differences between males and females, and between low, middle, and high educated people.
Regression analysis has become one of the most widely used statistical tools for analyzing multifactor data. It is appealing because it provides a conceptually simple method for investigating functional relationships among variables.
Conclusion The performance and interpretation of linear regression analysis are subject to a variety of pitfalls, which are discussed here in detail. The reader is made aware of common errors of interpretation through practical examples. Both the opportunities for applying linear regression analysis and its limitations are presented.
Linear regression analysis is one of the most important statistical methods. It examines the linear relationship between a metric-scaled dependent variable (also called endogenous, explained, response, or predicted variable) and one or more metric-scaled independent variables (also called exogenous, explanatory, control, or predictor variable).
A multiple linear regression analysis is carried out to predict the values of a dependent variable, Y, given a set of p explanatory variables (x1,x2,....,xp). In these notes, the necessary theory for multiple linear regression is presented and examples of regression analysis with census data are given to illustrate this theory.
Regression analysis is a statistical tool for the investigation of re-lationships between variables. Usually, the investigator seeks to ascertain the causal eVect of one variable upon another—the e Vect of a price increase upon demand, for example, or the e Vect of changes in the money supply upon the in Xation rate.
Abstract. Although regression models play a central role in the analysis of medical research projects, there still exist many misconceptions on various aspects of modeling leading to faulty analyses. Indeed, the rapidly developing statistical methodology and its recent advances in regression modeling do not seem to be adequately reflected in ...
Multiple regression is one of the most significant forms of regression and has a wide range of applications. The study of the implementation of multiple regression analysis in different settings ...
Later I shall show you how to use SAS to conduct a multiple regression analysis like this. Right now I simply want to give you an example of how to present the results of such an analysis. You can expect to receive from me a few assignments in which I ask you to conduct a multiple regression analysis and then present the results.
Linear Regression Analysis on Net Income of an Agrochemical Company in Thailand. ! 2! ! Abstract: The purpose of this research is to analyze the ABC Company's data and verify whether the regression analysis methods and models would work effectively in the ABC Company based in Bangkok, Thailand.
How is multiple regression analysis done? This article explains this handy statistical test when dealing with many variables, then provides an example of a research using multiple regression analysis to show how it works. It explains how research using multiple regression analysis is conducted.
Regression analysis is a powerful and useful statistical procedure with many implications for nursing research. It enables researchers to describe, predict and estimate the relationships and draw plausible conclusions about the interrelated variables in relation to any studied phenomena.
The data were submitted to linear regression analysis through structural equation modelling using AMOS 4.0. In our results, we showed that a proxy for SES was the strongest predictor of reading achievement.
Regression analysis is a statistical technique for determining the relationship between a single dependent (criterion) variable and one or more independent (predictor) variables. The analysis yields a predicted value for the criterion resulting from a linear combination of the predictors. According to Pedhazur, 15 regression analysis has 2 uses ...
Presentation of Regression Results I've put together some information on the "industry standards" on how to report regression results. Every paper uses a slightly different strategy, depending on author's focus. Please review the earlier handout on presenting data and tables, much of that discussion applies to regression tables as well
This research contributes to the literature by developing a quantitative corporate reputation index using principal component analysis (PCA), addressing gaps in CSRD research in developing regions, and highlighting the importance of national culture in influencing stakeholder perceptions of CSR activities.
Among various statistical tools, correlation and regression analysis are mostly used tools in many research works., e.g. the field of management, medicine, social science and education.