Ph.D. in Statistics
Our doctoral program in statistics gives future researchers preparation to teach and lead in academic and industry careers.
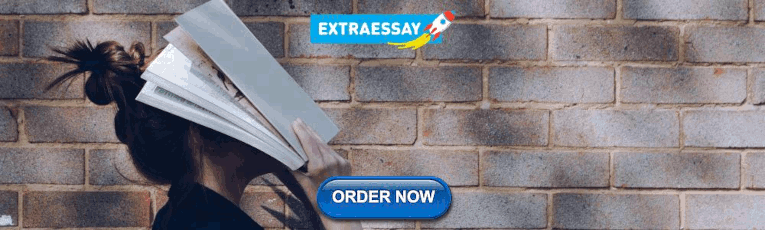
Program Description
Degree type.
approximately 5 years
The relatively new Ph.D. in Statistics strives to be an exemplar of graduate training in statistics. Students are exposed to cutting edge statistical methodology through the modern curriculum and have the opportunity to work with multiple faculty members to take a deeper dive into special topics, gain experience in working in interdisciplinary teams and learn research skills through flexible research electives. Graduates of our program are prepared to be leaders in statistics and machine learning in both academia and industry.
The Ph.D. in Statistics is expected to take approximately five years to complete, and students participate as full-time graduate students. Some students are able to finish the program in four years, but all admitted students are guaranteed five years of financial support.
Within our program, students learn from global leaders in statistics and data sciences and have:
20 credits of required courses in statistical theory and methods, computation, and applications
18 credits of research electives working with two or more faculty members, elective coursework (optional), and a guided reading course
Dissertation research
Coursework Timeline
Year 1: focus on core learning.
The first year consists of the core courses:
- SDS 384.2 Mathematical Statistics I
- SDS 383C Statistical Modeling I
- SDS 387 Linear Models
- SDS 384.11 Theoretical Statistics
- SDS 383D Statistical Modeling II
- SDS 386D Monte Carlo Methods
In addition to the core courses, students of the first year are expected to participate in SDS 190 Readings in Statistics. This class focuses on learning how to read scientific papers and how to grasp the main ideas, as well as on practicing presentations and getting familiar with important statistics literature.
At the end of the first year, students are expected to take a written preliminary exam. The examination has two purposes: to assess the student’s strengths and weaknesses and to determine whether the student should continue in the Ph.D. program. The exam covers the core material covered in the core courses and it consists of two parts: a 3-hour closed book in-class portion and a take-home applied statistics component. The in-class portion is scheduled at the end of the Spring Semester after final exams (usually late May). The take-home problem is distributed at the end of the in-class exam, with a due-time 24 hours later.
Year 2: Transitioning from Student to Researcher
In the second year of the program, students take the following courses totaling 9 credit hours each semester:
- Required: SDS 190 Readings in Statistics (1 credit hour)
- Required: SDS 389/489 Research Elective* (3 or 4 credit hours) in which the student engages in independent research under the guidance of a member of the Statistics Graduate Studies Committee
- One or more elective courses selected from approved electives ; and/or
- One or more sections of SDS 289/389/489 Research Elective* (2 to 4 credit hours) in which the student engages in independent research with a member(s) of the Statistics Graduate Studies Committee OR guided readings/self-study in an area of statistics or machine learning.
- Internship course (0 or 1 credit hour; for international students to obtain Curricular Practical Training; contact Graduate Coordinator for appropriate course options)
- GRS 097 Teaching Assistant Fundamentals or NSC 088L Introduction to Evidence-Based Teaching (0 credit hours; for TA and AI preparation)
* Research electives allow students to explore different advising possibilities by working for a semester with a particular professor. These projects can also serve as the beginning of a dissertation research path. No more than six credit hours of research electives can be taken with a single faculty member in a semester.
Year 3: Advance to Candidacy
Students are encouraged to attend conferences, give presentations, as well as to develop their dissertation research. At the end of the second year or during their third year, students are expected to present their plan of study for the dissertation in an Oral candidacy exam. During this exam, students should demonstrate their research proficiency to their Ph.D. committee members. Students who successfully complete the candidacy exam can apply for admission to candidacy for the Ph.D. once they have completed their required coursework and satisfied departmental requirements. The steps to advance to candidacy are:
- Discuss potential candidacy exam topics with advisor
- Propose Ph.D. committee: the proposed committee must follow the Graduate School and departmental regulations on committee membership for what will become the Ph.D. Dissertation Committee
- Application for candidacy
Year 4+: Dissertation Completion and Defense
Students are encouraged to attend conferences, give presentations, as well as to develop their dissertation research. Moreover, they are expected to present part of their work in the framework of the department's Ph.D. poster session.
Students who are admitted to candidacy will be expected to complete and defend their Ph.D. thesis before their Ph.D. committee to be awarded the degree. The final examination, which is oral, is administered only after all coursework, research and dissertation requirements have been fulfilled. It is expected that students will be prepared to defend by the end of their fifth year in the doctoral program.
General Information and Expectations for All Ph.D. students
- 2023-24 Student Handbook
- Annual Review At the end of every spring semester, students in their second year and beyond are expected to fill out an annual review form distributed by the Graduate Program Administrator.
- Seminar Series All students are expected to attend the SDS Seminar Series
- SDS 189R Course Description (when taken for internship)
- Internship Course Registration form
- Intel Corporation
- Berry Consultants
Attending Conferences
Students are encouraged to attend conferences to share their work. All research-related travel while in student status require prior authorization.
- Request for Travel Authorization (both domestic and international travel)
- Request for Authorization for International Travel
- Graduate Studies
Ph.D. Program
The PhD program prepares students for research careers in theory and application of probability and statistics in academic and non-academic (e.g., industry, government) settings. Students might elect to pursue either the general Statistics track of the program (the default), or one of the four specialized tracks that take advantage of UW’s interdisciplinary environment: Statistical Genetics (StatGen), Statistics in the Social Sciences (CSSS), Machine Learning and Big Data (MLBD), and Advanced Data Science (ADS).
Admission Requirements
For application requirements and procedures, please see the graduate programs applications page .
Recommended Preparation
The Department of Statistics at the University of Washington is committed to providing a world-class education in statistics. As such, having some mathematical background is necessary to complete our core courses. This background includes linear algebra at the level of UW’s MATH 318 or 340, advanced calculus at the level of MATH 327 and 328, and introductory probability at the level of MATH 394 and 395. Real analysis at the level of UW’s MATH 424, 425, and 426 is also helpful, though not required. Descriptions of these courses can be found in the UW Course Catalog . We also recognize that some exceptional candidates will lack the needed mathematical background but succeed in our program. Admission for such applicants will involve a collaborative curriculum design process with the Graduate Program Coordinator to allow them to make up the necessary courses.
While not a requirement, prior background in computing and data analysis is advantageous for admission to our program. In particular, programming experience at the level of UW’s CSE 142 is expected. Additionally, our coursework assumes familiarity with a high-level programming language such as R or Python.
Graduation Requirements
This is a summary of the department-specific graduation requirements. For additional details on the department-specific requirements, please consult the Ph.D. Student Handbook . For previous versions of the Handbook, please contact the Graduate Student Advisor . In addition, please see also the University-wide requirements at Instructions, Policies & Procedures for Graduate Students and UW Doctoral Degrees .
General Statistics Track
- Core courses: Advanced statistical theory (STAT 581, STAT 582 and STAT 583), statistical methodology (STAT 570 and STAT 571), statistical computing (STAT 534), and measure theory (either STAT 559 or MATH 574-575-576).
- Elective courses: A minimum of four approved 500-level classes that form a coherent set, as approved in writing by the Graduate Program Coordinator. A list of elective courses that have already been pre-approved or pre-denied can be found here .
- M.S. Theory Exam: The syllabus of the exam is available here .
- Research Prelim Exam. Requires enrollment in STAT 572.
- Consulting. Requires enrollment in STAT 599.
- Applied Data Analysis Project. Requires enrollment in 3 credits of STAT 597.
- Statistics seminar participation: Students must attend the Statistics Department seminar and enroll in STAT 590 for at least 8 quarters.
- Teaching requirement: All Ph.D. students must satisfactorily serve as a Teaching Assistant for at least one quarter.
- General Exam.
- Dissertation Credits. A minimum of 27 credits of STAT 800, spread over at least three quarters.
- Passage of the Dissertation Defense.
Statistical Genetics (StatGen) Track
Students pursuing the Statistical Genetics (StatGen) Ph.D. track are required to take BIOST/STAT 550 and BIOST/STAT 551, GENOME 562 and GENOME 540 or GENOME 541. These courses may be counted as the four required Ph.D.-level electives. Additionally, students are expected to participate in the Statistical Genetics Seminar (BIOST581) in addition to participating in the statistics seminar (STAT 590). Finally, students in the Statistics Statistical Genetics Ph.D. pathway may take STAT 516-517 instead of STAT 570-571 for their Statistical Methodology core requirement. This is a transcriptable program option, i.e., the fact that the student completed the requirements will be noted in their transcript.
Statistics in the Social Sciences (CSSS) Track
Students in the Statistics in the Social Sciences (CSSS) Ph.D. track are required to take four numerically graded 500-level courses, including at least two CSSS courses or STAT courses cross-listed with CSSS, and at most two discipline-specific social science courses that together form a coherent program of study. Additionally, students must complete at least three quarters of participation (one credit per quarter) in the CS&SS seminar (CSSS 590). This is not a transcriptable option, i.e., the fact that the student completed the requirements will not be noted in their transcript.
Machine Learning and Big Data Track
Students in the Machine Learning and Big Data (MLBD) Ph.D. track are required to take the following courses: one foundational machine learning course (STAT 535), one advanced machine learning course (either STAT 538 or STAT 548 / CSE 547), one breadth course (either on databases, CSE 544, or data visualization, CSE 512), and one additional elective course (STAT 538, STAT 548, CSE 515, CSE 512, CSE 544 or EE 578). At most two of these four courses may be counted as part of the four required PhD-level electives. Students pursuing this track are not required to take STAT 583 and can use STAT 571 to satisfy the Applied Data Analysis Project requirement. This is not a transcriptable option, i.e., the fact that the student completed the requirements will not be noted in their transcript.
Advanced Data Science (ADS) Track
Students in the Advanced Data Science (ADS) Ph.D. track are required to take the same coursework as students in the Machine Learning and Big Data track. They are also not required to take STAT 583 and can use STAT 571 to satisfy the Applied Data Analysis Project requirement. The only difference in terms of requirements between the MLBD and the ADS tracks is that students in the ADS track must also register for at least 4 quarters of the weekly eScience Community Seminar (CHEM E 599). Also, unlike the MLBD track, the ADS is a transcriptable program option, i.e., the fact that the student completed the requirements will be noted in their transcript.
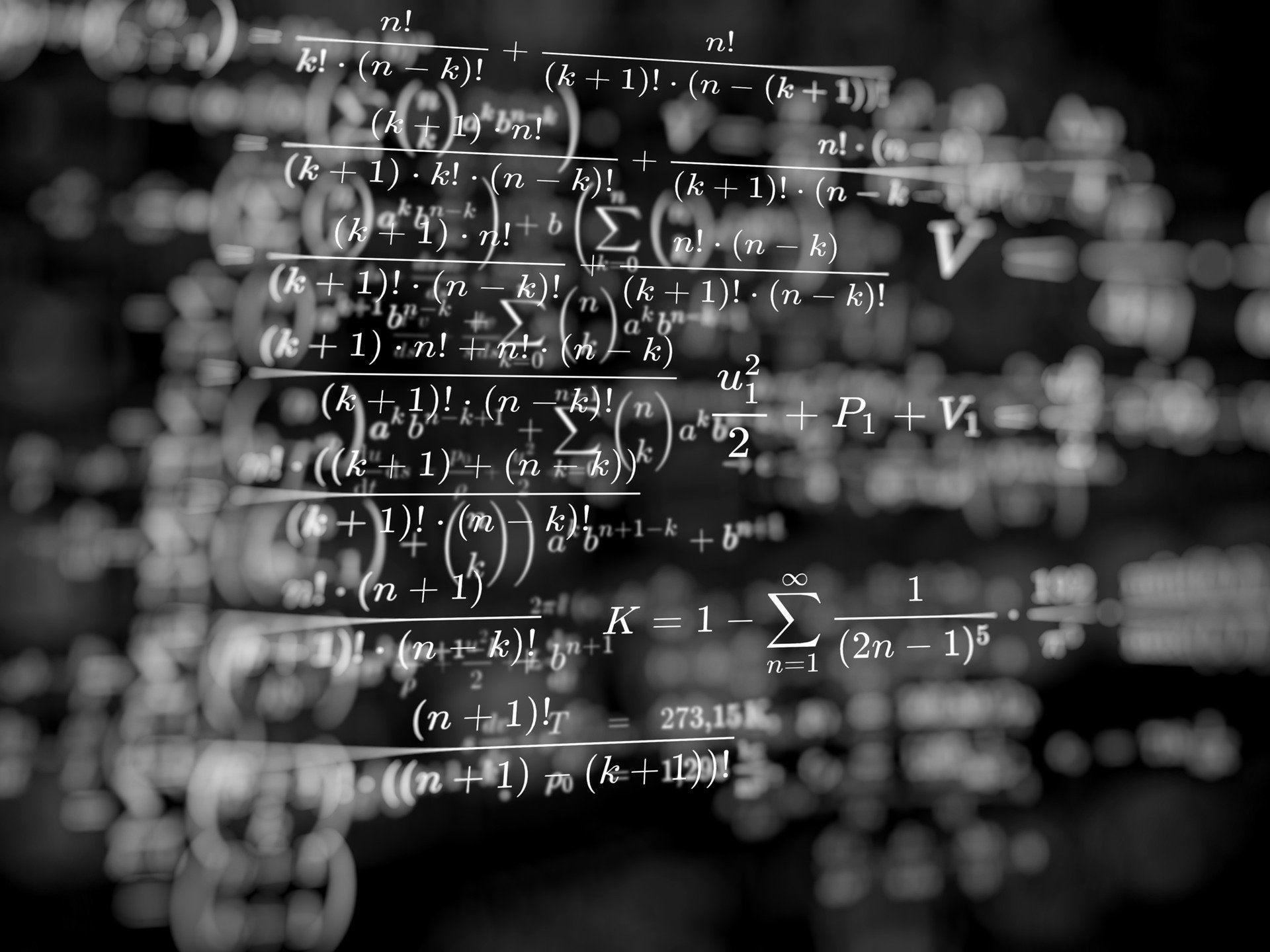
Is a PhD In Statistics Worth It?
At face value, a statistics PhD seems like a sound career investment, the ticket to higher paying jobs and career growth.
It’s no surprise, then, that one of the most common questions we hear is: Are statistics PhD programs worth it for data science jobs?
If we’re just looking at PhD in statistics salaries, sure, from a purely financial perspective, a PhD might be a good investment in your data science career. There’s a strong financial case you can make for earning one.
But beyond the great statistics PhD salary data, there are many other variables that make the answer a little less clear. When you think about the time commitment - almost 8 years - and the average salaries between master’s and PhD students in statistics, you’ll see that there are a number of trade-offs and that the bump in earnings isn’t so significant as to be a no-brainer.
That’s not to say there aren’t tons of great benefits of a PhD, because there are. For one, a PhD provides much more specialized knowledge, which can help you land competitive, more senior-level jobs. (It’s a preferred qualification for many Google jobs, in fact.) And of course, the average starting salaries for statistics PhDs are very enticing.
To help answer the question, “Is a PhD worth it?” we took a closer look at salaries for data scientists and statistics PhDs, as well as some of the pros and cons of pursuing a PhD for your data science career.
PhD In Stats: Salary Comparison
It’s probably not all that surprising that a PhD can increase your earnings, often by 2X or 3X. That’s really across the board, in all industries. For example, according to the Bureau of Labor Statistics, median weekly pay for a PhD ($1,885) was 45% higher than bachelor’s ($1,305) in 2020.
When you take a closer look at PhDs by field, though, PhDs in math and statistics have some of the best starting salaries in any industry. According to 2019 Survey of Doctorate Recipients data , recipients of a PhD in statistics have an average median starting salary of $140,000 (when pursuing a job in industry). That’s better than business administration, economics, and engineering:
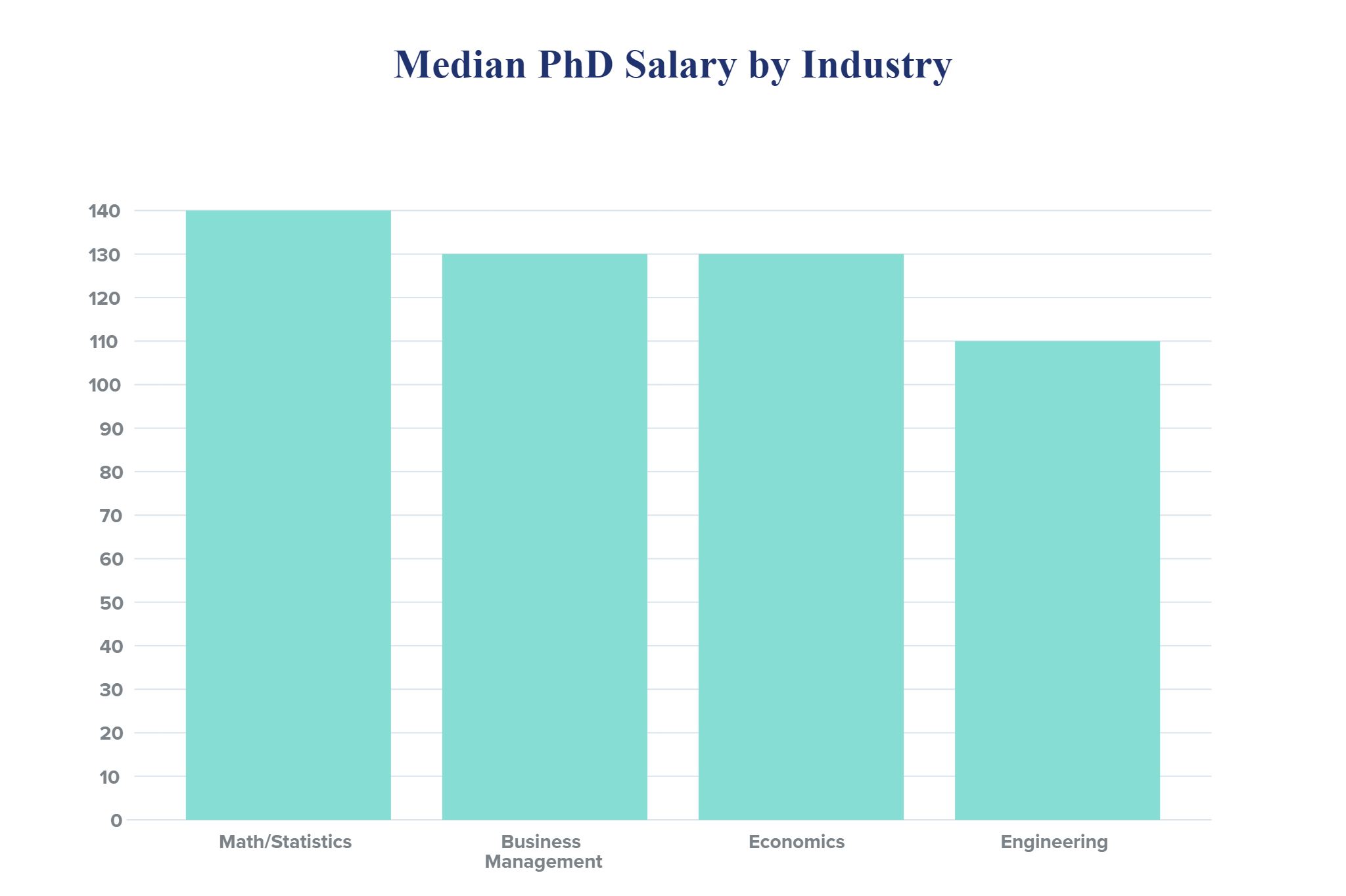
A PhD also results in a pretty big bump in salary compared to just earning a bachelor’s or master’s degree. For instance, median salaries for statistics PhD are two times that of bachelor’s recipients and 1.5 times that of master’s of statistics recipients:
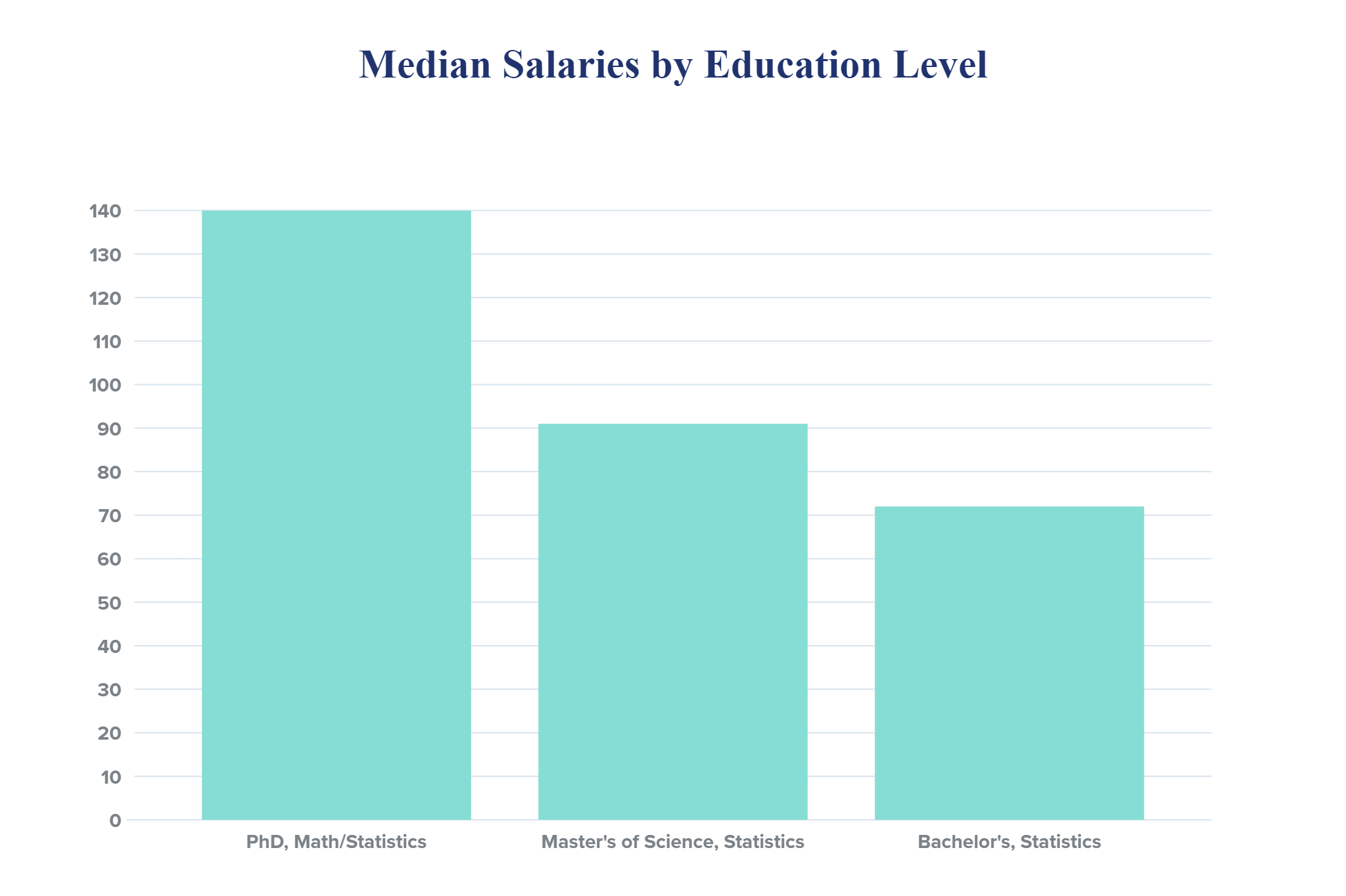
In other words, if you’re looking at the question through a purely financial lens, yes, a PhD in statistics is worth it.
But there’s one caveat. The lifetime earnings of a PhD vs a master’s recipient in statistics isn’t all too significant (on average about $3.6 million vs $3.45 million).
PhD in Stats: The Skills Bump
A big reason why starting salaries are so good for statistics PhDs is that your knowledge will be much more specialized.
Master’s in statistics programs tend to provide broad knowledge in the field. You’ll get a strong foundation of the fundamentals, and become well-versed in many different statistical concepts and methodologies. But you likely won’t get the depth of knowledge that you would from a PhD program.
A PhD differs quite a bit, and these programs are built around research. Here’s how it usually works: After completing initial coursework (usually 2 years), you’ll choose an area to focus your research. And then, you’ll spend 3-5 years researching that topic and preparing a dissertation on it.
The difference in focus, therefore, provides you with very specialized knowledge, and that’s a big reason why starting PhD salaries tend to be so high.
Is It Worth It? Delayed Earnings and Career Goals
Of course, the biggest trade-off in getting all this knowledge is the time commitment. PhD candidates in statistics spend nearly a decade – 7.75 years on average – earning the credential.
And that commitment is something you have to consider to really know if it’s worth it to you. Do you want to make this time commitment and spend the next 8 years researching a topic?
As a master’s recipient, you’ll gain a lot of useful professional skills and can jump right into a career. Sure, you might fully understand advanced statistical methodologies, but you will have a strong grasp of the fundamentals. And you can learn a lot to advance your career with professional development and on-the-job training.
Although they spend a lot of time researching a topic, PhDs do have one advantage: They’re often qualified for more senior-level data science jobs. At Google, for example, a PhD is a preferred qualification for many of their data science jobs, and that’s increasingly true for many FAANG companies.
A PhD Is a Good Investment, But With One Caveat
There’s a lot of reasons why you might consider a PhD in statistics. Salaries, for one, are some of the highest in data science , and job growth for statisticians is about 30% year-over-year. You’ll also have a lot of specialized knowledge that will increase your worth and prepare you for senior-level positions.
But here’s the caveat:
Even if you earn a PhD, you’ll still have a skills gaps that you need to fill, especially if you’re interested in a career in data science. There will skills - like coding or machine learning - that you might need to brush up on.
So if you’re expecting that a PhD is a ticket to a FAANG job, it’s not. But the specialized knowledge that it brings is, increasingly, a preferred qualification.
If you want to read more topics that are similar to this one, consider reading more through our blog where we dive into topics such as our PostgreSQL Interview Questions Guide , Machine Learning Case Studies , and even this article on ‘ Is Data Science a Good Career? ’
Learn and grow more by using resources here at Interview Query !
Stack Exchange Network
Stack Exchange network consists of 183 Q&A communities including Stack Overflow , the largest, most trusted online community for developers to learn, share their knowledge, and build their careers.
Q&A for work
Connect and share knowledge within a single location that is structured and easy to search.
Things to consider about masters programs in statistics
It is admission season for graduate schools. I (and many students like me) am now trying to decide which statistics program to pick.
- What are some things those of you who work with statistics suggest we consider about masters programs in statistics?
- Are there common pitfalls or mistakes students make (perhaps with regard to school reputation)?
- For employment, should we look to focus on applied statistics or a mix of applied and theoretical statistics?
Edit: Here is some additional information about my personal situation: All of the programs I am now considering are in the United States. Some focus on the more applied side and give masters degrees in "applied statistics" while others have more theoretical coursework and grant degrees in "statistics". I'm personally not that intent on working in one industry over another. I have some programming background and know the tech industry a little better than, say, the genomics or bioinformatics industry. However, I'm primarily looking for a career with interesting problems.
Edit : Tried to make the question more generally applicable.
- machine-learning
- mathematical-statistics
- 8 $\begingroup$ This depends very much on a lot of personal factors, making it hard to give good advice. We don't know what part of the world your programs are from, how focused your interests already are or what they are. The question is stated too broadly to be answered authoritatively, but would be at risk of being closed as too localized if it was geared solely toward giving advice to just one person. I suggest providing some more context, but not making it specific only to your particular case. $\endgroup$ – cardinal Commented Apr 2, 2012 at 17:42
- 1 $\begingroup$ Fair enough. All the programs I am now considering are in the United States. Some focus on the more applied side and give masters degrees in "applied statistics" while others have more theoretical coursework and grant degrees in "statistics". I'm personally not that intent on working in one industry over another. I have some programming background and know the tech industry a little better than say the genomics or bioinformatics industry. However, I'm primarily looking for a career with interesting problems. $\endgroup$ – AttemptedStudent Commented Apr 2, 2012 at 17:53
- $\begingroup$ Thank you. That is very helpful. I still think community wiki would be best, but this makes it possible for there to be a more productive conversation here. (deleting my previous comment.. ) $\endgroup$ – gung - Reinstate Monica Commented Apr 2, 2012 at 22:25
3 Answers 3
Here is a somewhat blunt set of general thoughts and recommendations on masters programs in statistics. I don't intend for them to be polemic, though some of them may sound like that.
I am going to assume that you are interested in a terminal masters degree to later go into industry and are not interested in potentially pursuing a doctorate. Please do not take this reply as authoritative, though.
Below are several points of advice from my own experiences. I've ordered them very roughly from what I think is most important to least. As you choose a program, you might weigh each of them against one another taking some of the points below into account.
Try to make the best choice for you personally . There are very many factors involved in such a decision: geography, personal relationships, job and networking opportunities, coursework, costs of education and living, etc. The most important thing is to weigh each of these yourself and try to use your own best judgment. You are the one that ultimately lives with the consequences of your choice, both positive and negative, and you are the only one in a position to appraise your whole situation. Act accordingly.
Learn to collaborate and manage your time . You may not believe me, but an employer will very likely care more about your personality, ability to collaborate with others and ability to work efficiently than they will care about your raw technical skills. Effective communication is crucial in statistics, especially when communicating with nonstatisticians. Knowing how to manage a complex project and make steady progress is very important. Take advantage of structured statistical-consulting opportunities, if they exist, at your chosen institution.
Learn a cognate area . The greatest weakness I see in many masters and PhD graduates in statistics, both in industry and in academia, is that they often have very little subject-matter knowledge. The upshot is that sometimes "standard" statistical analyses get used due to a lack of understanding of the underlying mechanisms of the problem they are trying to analyze. Developing some expertise in a cognate area can, therefore, be very enriching both statistically and professionally. But, the most important aspect of this is the learning itself: Realizing that incorporating subject matter knowledge can be vital to correctly analyzing a problem. Being competent in the vocabulary and basic knowledge can also aid greatly in communication and will improve the perception that your nonstatistician colleagues have of you.
Learn to work with (big) data . Data sets in virtually every field that uses statistics have been growing tremendously in size over the last 20 years. In an industrial setting, you will likely spend more time manipulating data than you will analyzing them. Learning good data-management procedures, sanity checking, etc. is crucial to valid analysis. The more efficient you become at it, the more time you'll spend doing the "fun" stuff. This is something that is very heavily underemphasized and underappreciated in academic programs. Luckily, there are now some bigger data sets available to the academic community that one can play with. If you can't do this within the program itself, spend some time doing so outside of it.
Learn linear regression and the associated applied linear algebra very, very well . It is surprising how many masters and PhD graduates obtain their degrees (from "top" programs!), but can't answer basic questions on linear regression or how it works. Having this material down cold will serve you incredibly well. It is important in its own right and is the gateway to many, many more advanced statistical and machine-learning techniques.
If possible, do a masters report or thesis . The masters programs associated with some of the top U.S. statistics departments (usually gauged more on their doctorate programs) seem to have moved away from incorporating a report or a thesis. The fact of the matter is that a purely course-based program usually deprives the student of developing any real depth of knowledge in a particular area. The area itself is not so important, in my view, but the experience is. The persistence, time-management, collaboration with faculty, etc. required to produce a masters report or thesis can pay off greatly when transitioning to industry. Even if a program doesn't advertise one, if you're otherwise interested in it, send an email to the admissions chair and ask about the possibility of a customized program that allows for it.
Take the most challenging coursework you can manage . While the most important thing is to understand the core material very, very well, you should also use your time and money wisely by challenging yourself as much as possible. The particular topic matter you choose to learn may appear to be fairly "useless", but getting some contact with the literature and challenging yourself to learn something new and difficult will make it easier when you have to do so later in industry. For example, learning some of the theory behind classical statistics turns out to be fairly useless in and of itself for the daily work of many industrial statisticians, but the concepts conveyed are extremely useful and provide continual guidance. It also will make all the other statistical methods you come into contact with seem less mysterious.
A program's reputation only matters for your first job . Way too much emphasis is put on a school's or program's reputation. Unfortunately, this is a time- and energy-saving heuristic for human-resource managers. Be aware that programs are judged much more by their research and doctoral programs than their masters ones. In many such top departments, the M.S. students often end up feeling a bit like second-class citizens since most of the resources are expended on the doctoral programs.
One of the brightest young statistical collaborators I've worked with has a doctorate from a small foreign university you've probably never heard of. People can get a wonderful education (sometimes a much better one, especially at the undergraduate and masters level!) at "no-name" institutions than at "top" programs. They're almost guaranteed to get more interaction with core faculty at the former.
The name of the school at the top of your resume is likely to have a role in getting you in the door for your first job and people will care more about where your most advanced degree came from than where any others did. After that first job, people will care substantially more about what experience you bring to the table. Finding a school where lots of interesting job opportunities come to you through career fairs, circulated emails, etc., can have a big payoff and this happens more at top programs.
A personal remark : I personally have a preference for somewhat more theoretical programs that still allow some contact with data and a smattering of applied courses. The fact of the matter is that you're simply not going to become a good applied statistician by obtaining a masters degree. That comes only with (much more) time and experience in struggling with challenging problems and analyses on a daily basis.
- 14 $\begingroup$ +1. Sometimes, as here, a good answer makes a question worth keeping. $\endgroup$ – whuber ♦ Commented Apr 2, 2012 at 20:31
- 4 $\begingroup$ I know this is a very individual decision. However, your thoughtful reply helps a lot. It is particularly interesting to see how highly you ranked learning a cognate area. Some programs allow me to take courses in other departments. I'm now starting to think that breadth is a particularly valuable characteristic of the program. $\endgroup$ – AttemptedStudent Commented Apr 2, 2012 at 20:51
- $\begingroup$ (+1) Very nice response. I particularly liked Point 3. $\endgroup$ – chl Commented Apr 2, 2012 at 21:55
- 2 $\begingroup$ @AttemptedStudent: Traditionally, I think most graduate students (PhD, in particular) in statistics have undergraduate math backgrounds and have had little contact with actual applied problems that require statistical concepts and thinking. That may be part of the reason learning a cognate area ended up so high on my list. But, as I mentioned in the body, the ordering is a bit rough. :) $\endgroup$ – cardinal Commented Apr 4, 2012 at 14:01
- 1 $\begingroup$ +1, nice answer. I liked points 3-5. Observation on data manipulation is spot on. $\endgroup$ – mpiktas Commented Apr 4, 2012 at 14:03
I would advise to either get in the best school possible with a brand name (like MIT), or the best overall deal (e.g. a decent public school with in-state tuition). I would not waste money on second rate private schools.
The brand name schools payoff. The price difference between a school like MIT and second tier schools like GWU is not big enough to justify the difference in the brand power.
On the other hand, some public schools, e.g. William and Mary, while being dirt cheap offer decent education. Some of them even have comparable brand power, e.g. Berkeley vs. Stanford. Thus due to the significant cost difference, they're an alternative to best private schools.
Take a look at Pharmacoepidemiology. In particular as it relates to Drug safety. This is a very new area of research with a lots of very interested questions.
- 1 $\begingroup$ Was this accidentally posted in the wrong place? $\endgroup$ – Macro Commented May 14, 2012 at 22:28
Your Answer
Sign up or log in, post as a guest.
Required, but never shown
By clicking “Post Your Answer”, you agree to our terms of service and acknowledge you have read our privacy policy .
Not the answer you're looking for? Browse other questions tagged machine-learning mathematical-statistics careers or ask your own question .
- Featured on Meta
- Announcing a change to the data-dump process
- Bringing clarity to status tag usage on meta sites
Hot Network Questions
- Is 3 ohm resistance value of a PCB fuse reasonable?
- 70s-80s animation with an island of robots
- Can you give me an example of an implicit use of Godel's Completeness Theorem, say for example in group theory?
- How important is philosophy: does anyone delimit its importance?
- 2 in 1: Twin Puzzle
- Word for a collection of awards, such as an Olympic athlete’s earned medals
- How much new mathematics should we learn during the PhD? Are the courses we take during the PhD really important?
- What counts as the Earth's mass? At which point would it increase or decrease?
- Which version of Netscape, on which OS, appears in the movie “Cut” (2000)?
- Journal keeps messing with my proof
- How can one be honest with oneself if one IS oneself?
- What is happening when a TV satellite stops broadcasting during an "eclipse"?
- How did Oswald Mosley escape treason charges?
- Where to donate foreign-language academic books?
- What does it mean to say that a statistical model is a location family?
- Do passengers transiting in YVR (Vancouver) from international to US go through Canadian immigration?
- Seinfeldisms in O.R
- How to remove obligation to run as administrator in Windows?
- Has the US said why electing judges is bad in Mexico but good in the US?
- Generate vectors with a certain property
- Why is the tortoise associated with 人情?
- Cannot open and HTML file stored on RAM-disk with a browser
- How to frame certain cells with tabluar?
- What is the highest apogee of a satellite in Earth orbit?
PhD Program information
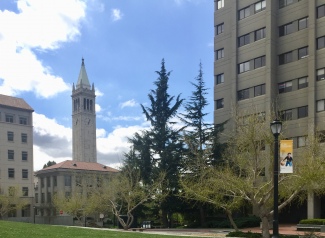
The Statistics PhD program is rigorous, yet welcoming to students with interdisciplinary interests and different levels of preparation. Students in the PhD program take core courses on the theory and application of probability and statistics during their first year. The second year typically includes additional course work and a transition to research leading to a dissertation. PhD thesis topics are diverse and varied, reflecting the scope of faculty research interests. Many students are involved in interdisciplinary research. Students may also have the option to pursue a designated emphasis (DE) which is an interdisciplinary specialization: Designated Emphasis in Computational and Genomic Biology , Designated Emphasis in Computational Precision Health , Designated Emphasis in Computational and Data Science and Engineering . The program requires four semesters of residence.
Normal progress entails:
Year 1 . Perform satisfactorily in preliminary coursework. In the summer, students are required to embark on a short-term research project, internship, graduate student instructorship, reading course, or on another research activity. Years 2-3 . Continue coursework. Find a thesis advisor and an area for the oral qualifying exam. Formally choose a chair for qualifying exam committee, who will also serve as faculty mentor separate from the thesis advisor. Pass the oral qualifying exam and advance to candidacy by the end of Year 3. Present research at BSTARS each year. Years 4-5 . Finish the thesis and give a lecture based on it in a department seminar.
Program Requirements
- Qualifying Exam
Course work and evaluation
Preliminary stage: the first year.
Effective Fall 2019, students are expected to take four semester-long courses for a letter grade during their first year which should be selected from the core first-year PhD courses offered in the department: Probability (204/205A, 205B,), Theoretical Statistics (210A, 210B), and Applied Statistics (215A, 215B). These requirements can be altered by a member of the PhD Program Committee (in consultation with the faculty mentor and by submitting a graduate student petition ) in the following cases:
- Students primarily focused on probability will be allowed to substitute one semester of the four required semester-long courses with an appropriate course from outside the department.
- Students may request to postpone one semester of the core PhD courses and complete it in the second year, in which case they must take a relevant graduate course in their first year in its place. In all cases, students must complete the first year requirements in their second year as well as maintain the overall expectations of second year coursework, described below. Some examples in which such a request might be approved are described in the course guidance below.
- Students arriving with advanced standing, having completed equivalent coursework at another institution prior to joining the program, may be allowed to take other relevant graduate courses at UC Berkeley to satisfy some or all of the first year requirements
Requirements on course work beyond the first year
Students entering the program before 2022 are required to take five additional graduate courses beyond the four required in the first year, resulting in a total of nine graduate courses required for completion of their PhD. In their second year, students are required to take three graduate courses, at least two of them from the department offerings, and in their third year, they are required to take at least two graduate courses. Students are allowed to change the timing of these five courses with approval of their faculty mentor. Of the nine required graduate courses, students are required to take for credit a total of 24 semester hours of courses offered by the Statistics department numbered 204-272 inclusive. The Head Graduate Advisor (in consultation with the faculty mentor and after submission of a graduate student petition) may consent to substitute courses at a comparable level in other disciplines for some of these departmental graduate courses. In addition, the HGA may waive part of this unit requirement.
Starting with the cohort entering in the 2022-23 academic year , students are required to take at least three additional graduate courses beyond the four required in the first year, resulting in a total of seven graduate courses required for completion of their PhD. Of the seven required graduate courses, five of these courses must be from courses offered by the Statistics department and numbered 204-272, inclusive. With these reduced requirements, there is an expectation of very few waivers from the HGA. We emphasize that these are minimum requirements, and we expect that students will take additional classes of interest, for example on a S/U basis, to further their breadth of knowledge.
For courses to count toward the coursework requirements students must receive at least a B+ in the course (courses taken S/U do not count, except for STAT 272 which is only offered S/U). Courses that are research credits, directed study, reading groups, or departmental seminars do not satisfy coursework requirements (for courses offered by the Statistics department the course should be numbered 204-272 to satisfy the requirements). Upper-division undergraduate courses in other departments can be counted toward course requirements with the permission of the Head Graduate Advisor. This will normally only be approved if the courses provide necessary breadth in an application area relevant to the student’s thesis research.
First year course work: For the purposes of satisfactory progression in the first year, grades in the core PhD courses are evaluated as: A+: Excellent performance in PhD program A: Good performance in PhD program A-: Satisfactory performance B+: Performance marginal, needs improvement B: Unsatisfactory performance First year and beyond: At the end of each year, students must meet with his or her faculty mentor to review their progress and assess whether the student is meeting expected milestones. The result of this meeting should be the completion of the student’s annual review form, signed by the mentor ( available here ). If the student has a thesis advisor, the thesis advisor must also sign the annual review form.
Guidance on choosing course work
Choice of courses in the first year: Students enrolling in the fall of 2019 or later are required to take four semesters of the core PhD courses, at least three of which must be taken in their first year. Students have two options for how to schedule their four core courses:
- Option 1 -- Complete Four Core Courses in 1st year: In this option, students would take four core courses in the first year, usually finishing the complete sequence of two of the three sequences. Students following this option who are primarily interested in statistics would normally take the 210A,B sequence (Theoretical Statistics) and then one of the 205A,B sequence (Probability) or the 215A,B sequence (Applied Statistics), based on their interests, though students are allowed to mix and match, where feasible. Students who opt for taking the full 210AB sequence in the first year should be aware that 210B requires some graduate-level probability concepts that are normally introduced in 205A (or 204).
- Option 2 -- Postponement of one semester of a core course to the second year: In this option, students would take three of the core courses in the first year plus another graduate course, and take the remaining core course in their second year. An example would be a student who wanted to take courses in each of the three sequences. Such a student could take the full year of one sequence and the first semester of another sequence in the first year, and the first semester of the last sequence in the second year (e.g. 210A, 215AB in the first year, and then 204 or 205A in the second year). This would also be a good option for students who would prefer to take 210A and 215A in their first semester but are concerned about their preparation for 210B in the spring semester. Similarly, a student with strong interests in another discipline, might postpone one of the spring core PhD courses to the second year in order to take a course in that discipline in the first year. Students who are less mathematically prepared might also be allowed to take the upper division (under-graduate) courses Math 104 and/or 105 in their first year in preparation for 205A and/or 210B in their second year. Students who wish to take this option should consult with their faculty mentor, and then must submit a graduate student petition to the PhD Committee to request permission for postponement. Such postponement requests will be generally approved for only one course. At all times, students must take four approved graduate courses for a letter grade in their first year.
After the first year: Students with interests primarily in statistics are expected to take at least one semester of each of the core PhD sequences during their studies. Therefore at least one semester (if not both semesters) of the remaining core sequence would normally be completed during the second year. The remaining curriculum for the second and third years would be filled out with further graduate courses in Statistics and with courses from other departments. Students are expected to acquire some experience and proficiency in computing. Students are also expected to attend at least one departmental seminar per week. The precise program of study will be decided in consultation with the student’s faculty mentor.
Remark. Stat 204 is a graduate level probability course that is an alternative to 205AB series that covers probability concepts most commonly found in the applications of probability. It is not taught all years, but does fulfill the requirements of the first year core PhD courses. Students taking Stat 204, who wish to continue in Stat 205B, can do so (after obtaining the approval of the 205B instructor), by taking an intensive one month reading course over winter break.
Designated Emphasis: Students with a Designated Emphasis in Computational and Genomic Biology or Designated Emphasis in Computational and Data Science and Engineering should, like other statistics students, acquire a firm foundation in statistics and probability, with a program of study similar to those above. These programs have additional requirements as well. Interested students should consult with the graduate advisor of these programs.
Starting in the Fall of 2019, PhD students are required in their first year to take four semesters of the core PhD courses. Students intending to specialize in Probability, however, have the option to substitute an advanced mathematics class for one of these four courses. Such students will thus be required to take Stat 205A/B in the first year, at least one of Stat 210A/B or Stat 215A/B in the first year, in addition to an advanced mathematics course. This substitute course will be selected in consultation with their faculty mentor, with some possible courses suggested below. Students arriving with advanced coursework equivalent to that of 205AB can obtain permission to substitute in other advanced probability and mathematics coursework during their first year, and should consult with the PhD committee for such a waiver.
During their second and third years, students with a probability focus are expected to take advanced probability courses (e.g., Stat 206 and Stat 260) to fulfill the coursework requirements that follow the first year. Students are also expected to attend at least one departmental seminar per week, usually the probability seminar. If they are not sufficiently familiar with measure theory and functional analysis, then they should take one or both of Math 202A and Math 202B. Other recommended courses from the department of Mathematics or EECS include:
Math 204, 222 (ODE, PDE) Math 205 (Complex Analysis) Math 258 (Classical harmonic analysis) EE 229 (Information Theory and Coding) CS 271 (Randomness and computation)
The Qualifying Examination
The oral qualifying examination is meant to determine whether the student is ready to enter the research phase of graduate studies. It consists of a 50-minute lecture by the student on a topic selected jointly by the student and the thesis advisor. The examination committee consists of at least four faculty members to be approved by the department. At least two members of the committee must consist of faculty from the Statistics and must be members of the Academic Senate. The chair must be a member of the student’s degree-granting program.
Qualifying Exam Chair. For qualifying exam committees formed in the Fall of 2019 or later, the qualifying exam chair will also serve as the student’s departmental mentor, unless a student already has two thesis advisors. The student must select a qualifying exam chair and obtain their agreement to serve as their qualifying exam chair and faculty mentor. The student's prospective thesis advisor cannot chair the examination committee. Selection of the chair can be done well in advance of the qualifying exam and the rest of the qualifying committee, and because the qualifying exam chair also serves as the student’s departmental mentor (unless the student has co-advisors), the chair is expected to be selected by the beginning of the third year or at the beginning of the semester of the qualifying exam, whichever comes earlier. For more details regarding the selection of the Qualifying Exam Chair, see the "Mentoring" tab.
Paperwork and Application. Students at the point of taking a qualifying exam are assumed to have already found a thesis advisor and to should have already submitted the internal departmental form to the Graduate Student Services Advisor ( found here ). Selection of a qualifying exam chair requires that the faculty member formally agree by signing the internal department form ( found here ) and the student must submit this form to the Graduate Student Services Advisor. In order to apply to take the exam, the student must submit the Application for the Qualifying Exam via CalCentral at least three weeks prior to the exam. If the student passes the exam, they can then officially advance to candidacy for the Ph.D. If the student fails the exam, the committee may vote to allow a second attempt. Regulations of the Graduate Division permit at most two attempts to pass the oral qualifying exam. After passing the exam, the student must submit the Application for Candidacy via CalCentral .
The Doctoral Thesis
The Ph.D. degree is granted upon completion of an original thesis acceptable to a committee of at least three faculty members. The majority or at least half of the committee must consist of faculty from Statistics and must be members of the Academic Senate. The thesis should be presented at an appropriate seminar in the department prior to filing with the Dean of the Graduate Division. See Alumni if you would like to view thesis titles of former PhD Students.
Graduate Division offers various resources, including a workshop, on how to write a thesis, from beginning to end. Requirements for the format of the thesis are rather strict. For workshop dates and guidelines for submitting a dissertation, visit the Graduate Division website.
Students who have advanced from candidacy (i.e. have taken their qualifying exam and submitted the advancement to candidacy application) must have a joint meeting with their QE chair and their PhD advisor to discuss their thesis progression; if students are co-advised, this should be a joint meeting with their co-advisors. This annual review is required by Graduate Division. For more information regarding this requirement, please see https://grad.berkeley.edu/ policy/degrees-policy/#f35- annual-review-of-doctoral- candidates .
Teaching Requirement
For students enrolled in the graduate program before Fall 2016, students are required to serve as a Graduate Student Instructor (GSI) for a minimum of 20 hours (equivalent to a 50% GSI appointment) during a regular academic semester by the end of their third year in the program.
Effective with the Fall 2016 entering class, students are required to serve as a GSI for a minimum of two 50% GSI appointment during the regular academic semesters prior to graduation (20 hours a week is equivalent to a 50% GSI appointment for a semester) for Statistics courses numbered 150 and above. Exceptions to this policy are routinely made by the department.
Each spring, the department hosts an annual conference called BSTARS . Both students and industry alliance partners present research in the form of posters and lightning talks. All students in their second year and beyond are required to present a poster at BSTARS each year. This requirement is intended to acclimate students to presenting their research and allow the department generally to see the fruits of their research. It is also an opportunity for less advanced students to see examples of research of more senior students. However, any students who do not yet have research to present can be exempted at the request of their thesis advisor (or their faculty mentors if an advisor has not yet been determined).
Mentoring for PhD Students
Initial Mentoring: PhD students will be assigned a faculty mentor in the summer before their first year. This faculty mentor at this stage is not expected to be the student’s PhD advisor nor even have research interests that closely align with the student. The job of this faculty mentor is primarily to advise the student on how to find a thesis advisor and in selecting appropriate courses, as well as other degree-related topics such as applying for fellowships. Students should meet with their faculty mentors twice a semester. This faculty member will be the designated faculty mentor for the student during roughly their first two years, at which point students will find a qualifying exam chair who will take over the role of mentoring the student.
Research-focused mentoring : Once students have found a thesis advisor, that person will naturally be the faculty member most directly overseeing the student’s progression. However, students will also choose an additional faculty member to serve as a the chair of their qualifying exam and who will also serve as a faculty mentor for the student and as a member of his/her thesis committee. (For students who have two thesis advisors, however, there is not an additional faculty mentor, and the quals chair does NOT serve as the faculty mentor).
The student will be responsible for identifying and asking a faculty member to be the chair of his/her quals committee. Students should determine their qualifying exam chair either at the beginning of the semester of the qualifying exam or in the fall semester of the third year, whichever is earlier. Students are expected to have narrowed in on a thesis advisor and research topic by the fall semester of their third year (and may have already taken qualifying exams), but in the case where this has not happened, such students should find a quals chair as soon as feasible afterward to serve as faculty mentor.
Students are required to meet with their QE chair once a semester during the academic year. In the fall, this meeting will generally be just a meeting with the student and the QE chair, but in the spring it must be a joint meeting with the student, the QE chair, and the PhD advisor. If students are co-advised, this should be a joint meeting with their co-advisors.
If there is a need for a substitute faculty mentor (e.g. existing faculty mentor is on sabbatical or there has been a significant shift in research direction), the student should bring this to the attention of the PhD Committee for assistance.
PhD Student Forms:
Important milestones: .
Each of these milestones is not complete until you have filled out the requisite form and submitted it to the GSAO. If you are not meeting these milestones by the below deadline, you need to meet with the Head Graduate Advisor to ask for an extension. Otherwise, you will be in danger of not being in good academic standing and being ineligible for continued funding (including GSI or GSR appointments, and many fellowships).
Identify PhD Advisor† | End of 2nd year |
Identify Research Mentor (QE Chair) | Fall semester of 3rd year |
Pass Qualifying Exam and Advance to Candidacy | End of 3rd year |
Thesis Submission | End of 4th or 5th year |
†Students who are considering a co-advisor, should have at least one advisor formally identified by the end of the second year; the co-advisor should be identified by the end of the fall semester of the 3rd year in lieu of finding a Research Mentor/QE Chair.
Expected Progress Reviews:
Spring 1st year | Annual Progress Review | Faculty Mentor |
Review of 1st year progress | Head Graduate Advisor | |
Spring 2nd year | Annual Progress Review | Faculty Mentor or Thesis Advisor(s) (if identified) |
Fall 3+ year | Research progress report* | Research mentor** |
Spring 3+ year | Annual Progress Review* | Jointly with PhD advisor(s) and Research mentor |
* These meetings do not need to be held in the semester that you take your Qualifying Exam, since the relevant people should be members of your exam committee and will discuss your research progress during your qualifying exam
** If you are being co-advised by someone who is not your primary advisor because your primary advisor cannot be your sole advisor, you should be meeting with that person like a research mentor, if not more frequently, to keep them apprised of your progress. However, if both of your co-advisors are leading your research (perhaps independently) and meeting with you frequently throughout the semester, you do not need to give a fall research progress report.
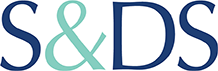
Department of Statistics and Data Science
Ph.d. program.
Fields of study include the main areas of statistical theory (with emphasis on foundations, Bayes theory, decision theory, nonparametric statistics), probability theory (stochastic processes, asymptotics, weak convergence), information theory, bioinformatics and genetics, classification, data mining and machine learning, neural nets, network science, optimization, statistical computing, and graphical models and methods.
With this background, graduates of the program have found excellent positions in universities, industry, and government. See the list of alumni for examples.
FellowshipBard
Phd in statistics: requirements, salary, jobs, & career growth, what is phd in statistics.
A PhD in Statistics is a doctoral-level program focusing on advanced statistical theory, methods, and applications.
Individuals with a good background in mathematics, statistics, or a similar discipline who want to build skill in statistical research, analysis, and data modeling generally pursue it.
Advanced statistical theory, probability theory, mathematical statistics, statistical computing, and data analysis are common courses in a PhD in Statistics program.
Depending on the individual curriculum and research interests of the student, it may also need study in adjacent subjects such as mathematics, computer science, or economics.
A PhD in Statistics program often requires students to undertake original statistics research and write a dissertation, which is a major and original piece of scholarly work that contributes to the subject of statistics.
This research frequently entails developing and executing statistical methodologies, evaluating data, and interpreting findings.
How much money do people make with a PhD in Statistics?
Individuals with a PhD in Statistics typically earn better salaries than others with less schooling in statistics or similar subjects.
According to data from the US Bureau of Labor Statistics (BLS) and other sources, the median annual pay for statisticians was $92,270 in May 2020. It is crucial to remember, however, that this figure indicates the median wage, which means that half of statisticians make more and half earn less.
Salaries for statisticians with a PhD in academia can vary based on the level of the academic employment.
Salaries for statisticians in academia can range from $60,000 to more than $150,000 per year, or even higher for highly talented and experienced statisticians working in famous institutions.
In the workplace, salaries for statisticians with a PhD might vary greatly based on the industry, employer, and job duties.
For example, statisticians working in banking, pharmaceuticals, technology, and consulting may earn more than those working in other areas.
In the business, salaries for statisticians can range from roughly $80,000 to well over $150,000 or more per year, depending on criteria such as employment level, region, and employer.
What is expected job growth with PhD in Statistics?
Individuals with a PhD in Statistics have a generally good career growth outlook, as demand for statistical skills continues to rise in a variety of industries due to a rising reliance on data-driven decision making and the increasing availability of massive datasets.
While particular job growth estimates differ depending on factors such as geography, sector, and economic conditions, there are a number of indicators that point to a positive work future for statisticians with a PhD.
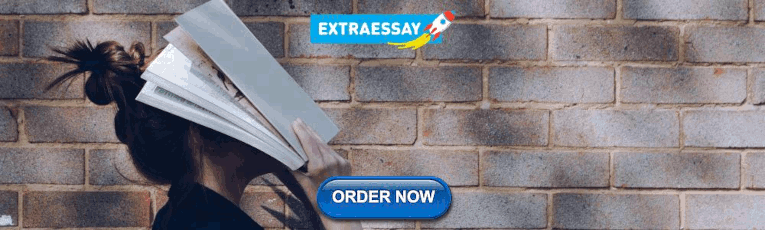
What can you do with a PhD in Statistics?
A PhD in Statistics opens the door to a variety of careers in academia, research institutes, government organizations, and the commercial sector. With their extensive statistical knowledge, research abilities, and data analysis experience, statisticians with a PhD can pursue a variety of careers, including:
1. Careers in academia and research: Many PhD statisticians choose to work in academia as professors, researchers, or postdoctoral associates in universities or research institutions. They can conduct cutting-edge statistical methods research, create new statistical models, publish research articles, and contribute to statistical theory and practice advancement. They may also teach statistics courses and guide students interested in careers in statistics or similar subjects.
2. Statistical Consulting: PhD-level statisticians can work as statistical consultants, providing knowledge to firms in a variety of industries including healthcare, finance, technology, marketing, and others. They can work with multidisciplinary teams to develop experiments, analyze data, interpret results, and provide statistical insights to help decision-makers.
3. Data Science and Analytics: Statisticians with a PhD can work as data scientists or analysts, analyzing complicated data sets, developing and evaluating models, and deriving meaningful insights from data. They could work in fields such as finance, healthcare, technology, or marketing, where data-driven decision making is essential.
4. Government and Public Policy: PhD statisticians can work in government agencies such as the National Institutes of Health (NIH), the National Institute of Standards and Technology (NIST), or the Bureau of Labor Statistics (BLS), where they can contribute to policy development, design and conduct statistical surveys, analyze data, and provide statistical expertise in fields such as public health, economics, and social sciences.
5. Research and Development: Statistics PhD holders can work in research and development roles in industries such as pharmaceuticals, biotechnology, and technology, where they can contribute to the design and analysis of experiments, develop statistical models, and provide statistical support for research projects.
6. Entrepreneurship and consulting: PhD statisticians can start their own statistical consulting firms or data analytics firms, delivering specialized statistical services to clients in a variety of industries. They can give experience in experimental design, data analysis, statistical modeling, and tailored solutions to meet the demands of their clients.
7. Teaching and training: Statisticians with a PhD can work in educational institutions, training programs, or professional development settings, where they can teach statistics courses, develop curricula, and train students, professionals, or organizations on statistical methods, software, and data analysis techniques.
What are the requirements for a PhD in Statistics?
The specific requirements for obtaining a PhD in Statistics can vary depending on the institution and program, but generally, the following are common requirements:
1. Bachelor’s or Master’s Degree: Applicants to most PhD programs in Statistics must have a Bachelor’s degree from a recognized university. Although it is not usually required, certain schools may accept applicants with a Master’s degree in a related discipline.
2. Academic Transcripts: Applicants are usually expected to present certified transcripts of their undergraduate and graduate education, which demonstrate their academic performance and achievement.
3. Statement of Purpose: Applicants are typically expected to provide a personal statement or statement of purpose detailing their research interests, academic ambitions, and reason for pursuing a PhD in Statistics.
4. Standardized Test Scores: Applicants to many PhD programs may be required to submit scores from standardized tests such as the Graduate Record Examination (GRE) or other related assessments.
5. Letters of Recommendation: Applicants to PhD programs in Statistics are frequently required to provide letters of recommendation from academic or professional sources who may speak to the applicant’s academic talents, research potential, and eligibility for a PhD program.
Looking For Scholarship Programs? Click here
How long does it take to get a phd in statistics.
The time it takes to complete a PhD in Statistics depends on a number of factors, including the specific program, the individual’s rate of advancement, and any additional prerequisites or obligations. A PhD in Statistics normally requires 4 to 6 years of full-time study beyond a bachelor’s degree.
Looking For Fully Funded PhD Programs? Click Here
Do you need a masters in statistics to get a phd in statistics.
In many circumstances, a master’s degree in statistics is not required to pursue a PhD in statistics. While some statistics PhD programs accept students with only a bachelor’s degree, others may need a master’s degree or equivalent coursework in statistics or a related discipline as a prerequisite for entrance.
A master’s degree in Statistics or a similar discipline, on the other hand, can provide considerable benefits when pursuing a PhD in Statistics.
It can provide a good foundation in statistical theory, technique, and data analysis, as well as indicate a person’s potential to perform at the graduate level.
It can also potentially allow students to transfer credits from their master’s program to their PhD program, which can reduce the overall time and coursework required to complete the PhD.
What are the Best PhD in Statistics Degree programs?
1. stanford university – phd in statistics 2. university of california, berkeley – phd in statistics 3. harvard university – phd in statistics 4. carnegie mellon university – phd in statistics 5. university of chicago – phd in statistics 6. columbia university – phd in statistics 7. duke university – phd in statistical science 8. university of washington – phd in statistics 9. massachusetts institute of technology (mit) – phd in statistics 10. university of michigan – phd in statistics, leave a comment cancel reply.
Save my name, email, and website in this browser for the next time I comment.
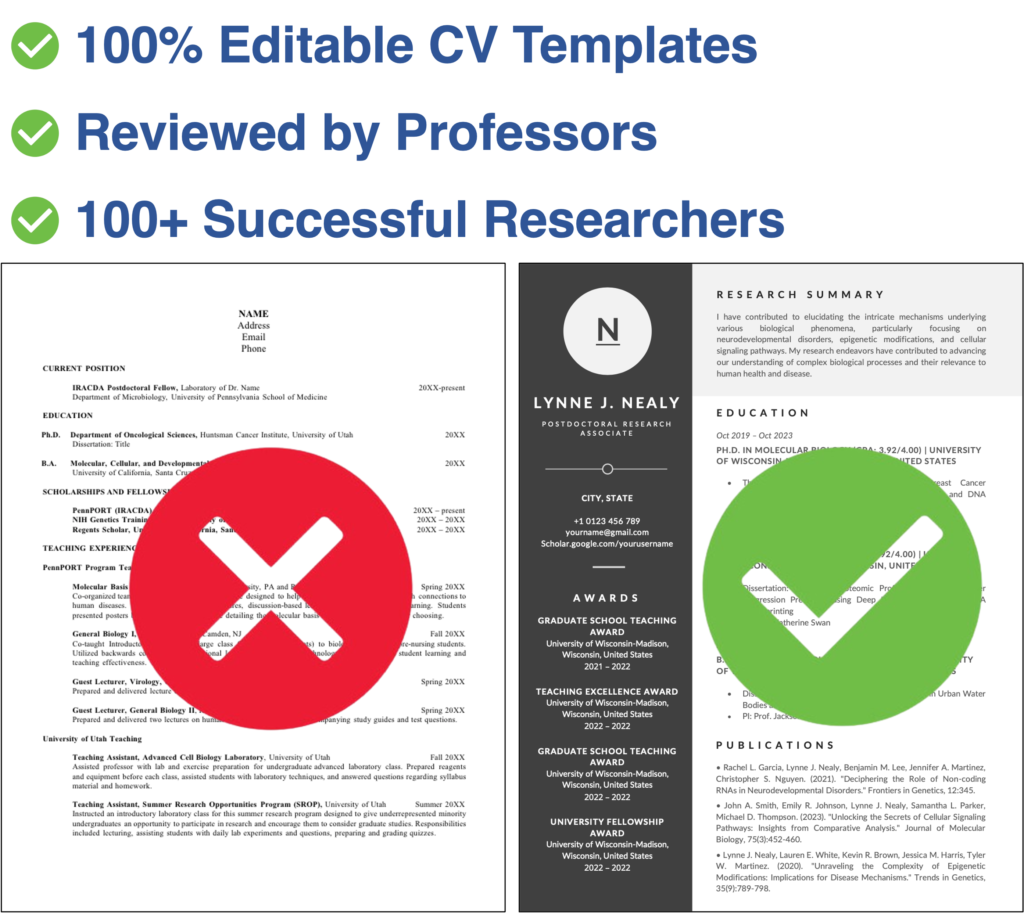
Professors Not Responding? Your CV May Be the Reason.
Try Our Ready-to-Use CV Templates Land You in Harvard, MIT, Oxford, and Beyond!
Stack Exchange Network
Stack Exchange network consists of 183 Q&A communities including Stack Overflow , the largest, most trusted online community for developers to learn, share their knowledge, and build their careers.
Q&A for work
Connect and share knowledge within a single location that is structured and easy to search.
Any risks down the line choosing maths vs. stats PhD programme?
It seems funding for a PhD (at least in the UK) is a lot easier to get for a PhD in statistics rather then maths. Provided one can find a supervisor who has the background and research interestes that would allow one to keep extending ones pure mathematical toolbox are there any major risks in going for a PhD in stats ?
It seems to me that at any given stage there is a lot of flexibility with a degree in mathematics. (For instance people seem very open minded to let a maths MSc do a stats PhD). Is it justified to be worried about losing this flexibility if one opts for the PhD in stats rather then maths?
In particular I am worried about whether it is possible to transition back to the maths departement for a postdoctoral position or something equivalent. So essentially I want to know whether one will get branded to an extend that would make it difficult to go on and work in pure mathematics after the PhD.
- graduate-school
- career-path
- Looks much better. There are a few mathematicians on this site who can hopefully provide a good answer now. – eykanal Commented Oct 24, 2012 at 13:11
2 Answers 2
Provided one can find a supervisor who has the background and research interestes that would allow one to keep extending ones pure mathematical toolbox are there any major risks in going for a PhD in stats?
There's a small risk, but it can be managed. The first issue is that you need to make the mathematical content of your work very clear, for example by publishing in journals that could be considered both math journals and statistics journals (e.g., IMS journals). However, if you're interested in math departments I assume you'd be doing that anyway.
The slightly more subtle issue is how mathematicians view statisticians. There's sometimes a mild prejudice that people in mathematical statistics are overly specialized and outside of the mainstream of mathematics. For example, it's possible to get a Ph.D. in mathematical statistics while having considerably less breadth of mathematical background than would be considered acceptable for a math grad student. (Of course, the flip side is that you are expected to know other things instead.) There's sometimes a fear that a statistician would be unlikely to talk much with other math department members, or might be unwilling or unable to teach anything but statistics.
Plenty of statisticians have found jobs in math departments, so I don't want to be discouraging. However, I'd recommend focusing on mathematical breadth. For example, if you work with people in combinatorics or algebra, then it will be clear to everyone why a math department is a natural fit. If you talk only to statisticians, it will be less clear. It can still work out even then, but generally when the department either has a thriving statistics group or has decided they really need a statistician (and either way this cuts down on the flexibility of your job search).
I cannot answer about stats/maths directly, but in general the department you get your PhD in matters less than who it is with. This is especially true in places like the UK were there is no course work component. In my current department, psychology, about 50% of the faculty, including myself, did not get our PhD from Psychology departments. That said, if you are only willing to teach in a Maths department, then you should probably go to a Maths department. If you are willing to teach in either Maths or Stats, then it doesn't matter too much.

You must log in to answer this question.
Not the answer you're looking for browse other questions tagged phd graduate-school career-path advisor ..
- Featured on Meta
- Bringing clarity to status tag usage on meta sites
- Announcing a change to the data-dump process
Hot Network Questions
- What is opinion?
- Fast algorithm to obtain an orthogonal vector to a set of vectors
- How can one be honest with oneself if one IS oneself?
- Should you refactor when there are no tests?
- Unable to update ubuntu 24.04 after incorrectly installing debian key
- How is it possible to know a proposed perpetual motion machine won't work without even looking at it?
- Encode a VarInt
- How did Oswald Mosley escape treason charges?
- WII U Single disc not working
- Who is the referent of "him" in Genesis 18:2?
- Duties when bringing Canadian goods to US in luggage
- Is this screw inside a 2-prong receptacle a possible ground?
- Coding exercise to represent an integer as words using python
- Whatever happened to Chessmaster?
- Why is simpler prose more popular these days?
- Rings demanding identity in the categorical context
- How much easier/harder would it be to colonize space if humans found a method of giving ourselves bodies that could survive in almost anything?
- How specific does the GDPR require you to be when providing personal information to the police?
- Whence “uniform distribution”?
- magnetic boots inverted
- What happens when touching a piece which cannot make a legal move?
- Overstayed Schengen but can I switch to US passport?
- How do eradicated diseases make a comeback?
- Reconstruction of Riemann surface from a germ of holomorphic function
- Communities Pre-Med Medical Resident Audiology Dental Optometry Pharmacy Physical Therapy Podiatry Psychology Rehab Sci Veterinary
- What's new Trending New posts Latest activity
- Support Account Help Confidential Advising
- Vision, Values and Policies
- Research Communities
- Physician Scientists
Anyone with MD/PhD with PhD in biostatistics / statistics / mathematics?
- Thread starter cdpiano27
- Start date Feb 18, 2008

Senior Member
- Feb 18, 2008
I would agree with you. http://en.wikipedia.org/wiki/National_Institute_of_Biomedical_Imaging_and_Bioengineering Signed into law by Bill Clinton. How could you not like it ?

Full Member
- Sep 21, 2011
pantouka said: I am a college pre-med sophomore interested in MAYBE going the MD/PHD route with an emphasis in biostatistics, but I'm having difficulty imagining exactly what the job would be like with the intersection of the two degrees. Click to expand...
pantouka said: 1) Would an MD/PHD-stats person have a huge role in running clinical trials? Would they ever be involved in analysis? Click to expand...
pantouka said: I2) How would the PHD portion of the joint degree be different from an MPH joint degree in practice? Click to expand...
pantouka said: I3) Are there any fields of medicine better suited for a stats PHD? I can honestly see relevance in disciplines ranging from psychiatry to infectious diseases. Click to expand...
I'm planning to pursue mathematics/statistics for my PhD and future career. There aren't many of us--maybe one or two graduate with it a year in the US... 1) Would an MD/PHD-stats person have a huge role in running clinical trials? Would they ever be involved in analysis? Yes, I'm planning to be involved in analyses and study design in clinical trials, as well as basic science research (hopefully genomics and population health). 2) How would the PHD portion of the joint degree be different from an MPH joint degree in practice? The MPH will not teach you enough statistics to do your own analyses very easily. Typically, MPH students only learn analysis up to multiple regression. The emphasis is public health, not statistics. In statistics/mathematics, you'll learn how to create new ways to analyse data that you can test in clinical trials and basic science research (probability theory, generalized linear models, network-based methodology...). An MS in statistics would probably give you a good overview, though. 3) Are there any fields of medicine better suited for a stats PHD? I can honestly see relevance in disciplines ranging from psychiatry to infectious diseases. Genetics, public health, and neuroscience are big areas in stats these days, so specialties relating to these areas of research might be a good starting point. I'm planning on a career in academics and government with medical service work abroad. A few things to consider in undergrad: -Most stats PhD programs require 3 semesters of calculus, linear algebra, several statistics courses (preferably with calculus), and a probability course. -You'll probably need the GRE in addition to the MCAT (not GRE math subject exam, though). GRE math should be substantially over 700. -There aren't a lot of schools offering this. U Minnesota, MUSC, U Miami, U Florida, UIC MSP, Stanford, and U of Chicago were the ones I found offering math/stats options. Bioinformatics is also a good option that's offered at more schools...
what exactly is the difference between bioinformatics and biostatistics?
- Sep 22, 2011
tortuga87 said: what exactly is the difference between bioinformatics and biostatistics? Click to expand...
Bioinformatics uses computer science to gather data in biology (genomics technology, algorithms to compute things for statisticians). Biostatistics is the application and development of new methods of data analysis from the field of mathematics (usually have someone in computer science to help you write the programs you need to analyze data by the new method).
It seems like: biostatician + learning some programming > bioinformatician because you can develop more elegant methods with biostatistics?
- Sep 23, 2011
tortuga87 said: It seems like: biostatician + learning some programming > bioinformatician because you can develop more elegant methods with biostatistics? Click to expand...
Biostaticians develop tools for analyzing data. Bioinformaticians develop tools for gathering data. Generally people in one will have some grounding in the other, but it's silly to say one is better than the other...
I'm currently a 2nd year MSPH in Biostatistics who deferred medical school to complete my masters degree. So here are the things that I've learned that I think would be most helpful: 1. Courses The main difference between MSPH/MPH and PhD courses is that the masters degree will ultimately be in public health, and biostats is just a concentration. Therefore, as a masters student you will be required to take public health courses such as epidemiology, behavioral science and health education, health policy management, and environmental sciences. For PhD students, these courses are optional although most choose to take these anyways. In terms of biostat courses, masters and PhD students have the same coursework the first year, with the PhD student also having to take an additional class to prepare them to be teaching assistants in the following years. The second year, PhD students go on to take more advanced statistical and probability theory classes. They may also be required as part of their stipend package to TA introductory biostat classes. 2. Thesis The masters thesis takes about a semester and a half to complete, and is significantly less involved than a PhD dissertation. The masters thesis is mainly explanation and application of a statistical model to a data set, whereas a PhD dissertation is expected to introduce new statistical theory and/or methods to a very specific area of research. Starting from about the third year of the PhD program, the students start to devote the majority of their time to working on their research and holding outside jobs. 3. Jobs Overall, masters programs have more of an emphasis on application and practical experience, and PhD programs have more of an emphasis on theory. Jobs that prefer masters degrees to PhD degrees are the ones that need someone who is more involved in the entire research process and study design. This often requires the biostatistician to physically go into, for example, a hospital and monitor the accuracy of which data is being collected. PhD-level biostatisticians would be over-qualified to work in this type of role, so they serve mostly as the lead data analyst. Outside of academia, PhD degrees are more often hired by biostat consulting firms and pharmaceutical companies to handle complex data analysis. All in all, if you want to be involved in the actual study and not just the data analysis, masters degrees are probably the way to go. 4. MD/MPH or MD/PhD When you're a biostatistician analyzing data, its always helpful, if not necessary, to understand the context of the problem you're working with. If you start working on a study about degenerative lumbar spine diseases, it would be really helpful if you already have working knowledge about the spine going into the project. However, if you don't have that knowledge, you can always do the background research yourself, although it takes a lot more energy and work. In this case, having an MD would definitely be a leg up. Also, if you hold an additional MD degree, you are most likely the one that is asking the research questions rather than working on other people's trials. This is a HUGE advantage because understanding your research backwards and forwards means that you are able to look at your data from multiple perspectives. For example, as a clinician you may know that certain biological mechanisms can affect your outcome and you actually know the statistical methods that allow you to identify and analyze those variables. On the other hand, a stastician without such an in-depth background in medicine and/or biology would not be able to reach the same conclusions. 5. Future Many studies have pointed out that PhD programs don't place enough of an emphasis on application of theory. Case in point: I am currently working on a study that has a lot of missing data due to patients not coming in for follow-up. To perform an accurate analysis, I need to know how to account for this missing data. I go a PhD-level professor who gives me literature to read on some theories on how the missing data might affect my conclusions. I go to a masters-level professor who tells me exactly what to do with my data to get a more accurate conclusion. As the field evolves, biostatisticians need to have both a firm grasp of theory as well as how to apply it in real-life situations. I hope all this helps. As you can tell, I'm very passionate about biostats and I really do wish there were more people that are interested in this field. Whatever path you decide, even if you decide to just pursue a masters degree, there is such a demand for biostats that your future will be bright now matter what.
- Sep 26, 2011
I personally am not getting a PhD in stats, but I did work for an MD/PhD when I was an undergrad who was a dermatologist whose research was skin cancer epidemiology. He was one of the archetypal 75/20/5 guys who spent most of his time doing research, two half days a week in general derm clinic, and taught a few lectures a semester. He was heavily involved in clinical trials and evaluating public health programs for skin cancer.
prolixity29
- Feb 20, 2012
Bump. I have a few questions regarding pursuing an MD/PhD in biostatistics... Really look forward to hearing your responses. I am currently pursuing my MSPH in Biostatistics in the Philippines (though I am from the US). After finishing my degree, I will attend medical school in the US. Originally, I had decided to study biostatistics out-of-interest and because I received a scholarship for my glide year, but I am truly enjoying the theoretical coursework and am now considering studying biostatistics when I enroll in medical school. The applied problem areas also interest me deeply, particularly genomics and environmental health. Since I will already have a master's degree by the time I matriculate into med school, it seems that the next logical step would be to shoot for a PhD. The problem is though that I am a non-traditional student in my late 20's. I want to have a family and need to start making an income and this would prolong my non-income-generating years... My eventual career goal in getting an MD/PhD would be either working/researching biostatistics while practicing in an academic hospital or consulting for industry (e.g. pharma or biotech) while practicing. Questions: 1) Is pursuing an MD/PhD at my age impractical? 2) Can you do a fellowship in biostatistics that would get you to the same place as an MD/PhD? I have tried searching for "biostatistics fellowships" and the ones I have seen are for post-docs. 3) Assuming I did pursue a PhD, would I be able to bypass the master's level courses given my MSPH? If so and assuming that I work diligently and do not burn out, what is a reasonable estimate for the number of years I could shave off the dual degree? Could it be done in 6 years? Thanks so much for your responses.
- Feb 21, 2012
prolixity29 said: Bump. I have a few questions regarding pursuing an MD/PhD in biostatistics... Really look forward to hearing your responses. I am currently pursuing my MSPH in Biostatistics in the Philippines (though I am from the US). After finishing my degree, I will attend medical school in the US. Originally, I had decided to study biostatistics out-of-interest and because I received a scholarship for my glide year, but I am truly enjoying the theoretical coursework and am now considering studying biostatistics when I enroll in medical school. The applied problem areas also interest me deeply, particularly genomics and environmental health. Since I will already have a master's degree by the time I matriculate into med school, it seems that the next logical step would be to shoot for a PhD. The problem is though that I am a non-traditional student in my late 20's. I want to have a family and need to start making an income and this would prolong my non-income-generating years... My eventual career goal in getting an MD/PhD would be either working/researching biostatistics while practicing in an academic hospital or consulting for industry (e.g. pharma or biotech) while practicing. Questions: 1) Is pursuing an MD/PhD at my age impractical? 2) Can you do a fellowship in biostatistics that would get you to the same place as an MD/PhD? I have tried searching for "biostatistics fellowships" and the ones I have seen are for post-docs. 3) Assuming I did pursue a PhD, would I be able to bypass the master's level courses given my MSPH? If so and assuming that I work diligently and do not burn out, what is a reasonable estimate for the number of years I could shave off the dual degree? Could it be done in 6 years? Thanks so much for your responses. Click to expand...
Similar threads
- heartman1998
- Jul 20, 2024

- Dec 31, 2023

- Jul 24, 2024

- trilobites10294814
- May 23, 2024
- This site uses cookies to help personalize content, tailor your experience and to keep you logged in if you register. By continuing to use this site, you are consenting to our use of cookies and terms of service . Accept Learn more…
2024 Best Statistics Doctor's Degree Schools
Choosing a great statistics school for your doctor's degree, overall quality is a must, average early-career salaries, other factors we consider, one size does not fit all, featured statistics programs, best schools for doctorate students to study statistics in the united states, 10 top schools for a doctorate in stats, additional noteworthy schools.
Rank | College | Location |
---|---|---|
11 | West Lafayette, IN | |
12 | College Station, TX | |
13 | Ann Arbor, MI | |
14 | Ames, IA | |
15 | New York, NY |
Statistics by Region
Region |
---|
Other Rankings
Best associate degrees in statistics, best master's degrees in statistics, best value in statistics, best for non-traditional students in statistics, best online in statistics, most popular online in statistics, best bachelor's degrees in statistics, best overall in statistics, highest paid grads in statistics, best for veterans in statistics, most popular in statistics, most focused in statistics, statistics related rankings by major, stats focus areas.
Major | Annual Graduates |
---|---|
433 | |
18 | |
5 |
Majors Similar to Stats
Related Major | Annual Graduates |
---|---|
1,149 | |
315 | |
30 | |
11 |
Notes and References
Popular reports, compare your school options.
Doctoral Program
Program summary.
Students are required to
- master the material in the prerequisite courses
- pass the first-year core program
- attempt all three parts of the qualifying examinations and show acceptable performance in at least two of them (end of 1st year)
- confirm a principal dissertation research advisor and file for candidacy (early spring quarter of 2nd year)
- satisfy the depth and breadth requirements (2nd/3rd/4th year)
- successfully complete the thesis proposal meeting and submit the Dissertation Reading Committee form (winter quarter of the 3rd year)
- present a draft of their dissertation and pass the university oral examination (4th/5th year)
The PhD requires a minimum of 135 units. Students are required to take a minimum of nine units of advanced topics courses (for depth) offered by the department (not including literature, research, consulting or Year 1 coursework), and a minimum of nine units outside of the Statistics Department (for breadth). Courses for the depth and breadth requirements must equal a combined minimum of 24 units. In addition, students must enroll in STATS 390 Statistical Consulting, taking it at least twice.
All students who have passed the qualifying exams but have not yet passed the Thesis Proposal Meeting must take STATS 319 at least once each year. For example, a student taking the qualifying exams in the summer after Year 1 and having the dissertation proposal meeting in Year 3, would take 319 in Years 2 and 3. Students in their second year are strongly encouraged to take STATS 399 with at least one faculty member. All details of program requirements can be found in the Department of Statistics PhD Student Handbook (available to Stanford affiliates only, using Stanford authentication. Requests for access from non-affiliates will not be approved).
Statistics Department PhD Handbook
All students are expected to abide by the Honor Code and the Fundamental Standard .
Doctoral and Research Advisors
During the first two years of the program, students' academic progress is monitored by the department's Director of Graduate Studies (DGS). Each student should meet at least once a quarter with the DGS to discuss their academic plans and their progress towards choosing a thesis advisor (before the final study list deadline of spring of the second year). From the third year onward students are advised by their selected advisor.
Qualifying Examinations and Candidacy
Qualifying examinations are part of most PhD programs in the United States. At Stanford these exams are intended to test the student's level of knowledge when the first-year program, common to all students, has been completed. There are separate examinations in the three core subjects of statistical theory and methods, applied statistics, and probability theory, which are typically taken during the summer at the end of the student's first year. Students are expected to attempt all three examinations and show acceptable performance in at least two of them. Letter grades are not given. Qualifying exams may be taken only once. After passing the qualifying exams, students must file for PhD Candidacy, a university milestone, by early spring quarter of their second year.
While nearly all students pass the qualifying examinations, those who do not can arrange to have their financial support continued for up to three quarters while alternative plans are made. Usually students are able to complete the requirements for the M.S. degree in Statistics in two years or less, whether or not they have passed the PhD qualifying exams.
Thesis Proposal Meeting and Dissertation Reading Committee
The thesis proposal meeting is intended to demonstrate a student's depth in some areas of statistics, and to examine the general plan for their research. In the meeting the student gives a 60-minute presentation involving ideas developed to date and plans for completing a PhD dissertation, and for another 60 minutes answers questions posed by the committee. which consists of their advisor and two other members. The meeting must be successfully completed by the end of winter quarter of the third year. If a student does not pass, the exam must be repeated. Repeated failure can lead to a loss of financial support.
The Dissertation Reading Committee consists of the student’s advisor plus two faculty readers, all of whom are responsible for reading the full dissertation. Of these three, at least two must be members of the Statistics Department (faculty with a full or joint appointment in Statistics but excluding for this purpose those with only a courtesy or adjunct appointment). Normally, all committee members are members of the Stanford University Academic Council or are emeritus Academic Council members; the principal dissertation advisor must be an Academic Council member.
The Doctoral Dissertation Reading Committee form should be completed and signed at the Dissertation Proposal Meeting. The form must be submitted before approval of TGR status or before scheduling a University Oral Examination.
For further information on the Dissertation Reading Committee, please see the Graduate Academic Policies and Procedures (GAP) Handbook section 4.8.
University Oral Examinations
The oral examination consists of a public, approximately 60-minute, presentation on the thesis topic, followed by a 60 minute question and answer period attended only by members of the examining committee. The questions relate to the student's presentation and also explore the student's familiarity with broader statistical topics related to the thesis research. The oral examination is normally completed during the last few months of the student's PhD period. The examining committee typically consists of four faculty members from the Statistics Department and a fifth faculty member from outside the department serving as the committee chair. Four out of five passing votes are required and no grades are given. Nearly all students can expect to pass this examination, although it is common for specific recommendations to be made regarding completion of the thesis.
The Dissertation Reading Committee must also read and approve the thesis.
For further information on university oral examinations and committees, please see the Graduate Academic Policies and Procedures (GAP) Handbook section 4.7 .
Dissertation
The dissertation is the capstone of the PhD degree. It is expected to be an original piece of work of publishable quality. The research advisor and two additional faculty members constitute the student's Dissertation Reading Committee. Normally, all committee members are members of the Stanford University Academic Council or are emeritus Academic Council members.
Department of Statistics
Last update: 11/10/23
PhD Degree in Statistics
The Department of Statistics offers an exciting and recently revamped PhD program that involves students in cutting-edge interdisciplinary research in a wide variety of fields. Statistics has become a core component of research in the biological, physical, and social sciences, as well as in traditional computer science domains such as artificial intelligence and machine learning. The massive increase in the data acquired, through scientific measurement on one hand and through web-based collection on the other, makes the development of statistical analysis and prediction methodologies more relevant than ever.
Our graduate program prepares students to address these issues through rigorous training in scientific computation, and in the theory, methodology, and applications of statistics. The course work includes four core sequences:
- Probability (STAT 30400, 38100, 38300)
- Mathematical statistics (STAT 30400, 30100, 30210)
- Applied statistics (STAT 34300, 34700, 34800)
- Computational mathematics and machine learning (STAT 30900, 31015/31020, 37710).
All students must take the Applied Statistics and Theoretical Statistics sequence. In addition it is highly recommended that students take a third core sequence based on their interests and in consultation with the Department Graduate Advisor (DGA). At the start of their second year, the students take two preliminary examinations. All students must take the Applied Statistics Prelim. For the second the students can choose to take either the Theoretical Statistics or the Probability prelim. Students planning to take the Probability prelim should take the Probability sequence as their third sequence.
Incoming first-year students have the option of taking any or all of these exams; if an incoming student passes one or more of these, then he/she will be excused from the requirement of taking the first-year courses in that subject. During the second and subsequent years, students can take more advanced courses, and perform research, with world-class faculty in a wide variety of research areas .
In recent years, a large majority of our students complete the PhD within four or five years of entering the program. Students who have significant graduate training before entering the program can (and do) obtain their doctor's degree in three years.
Most students receiving a doctorate proceed to faculty or postdoctoral appointments in research universities. A substantial number take positions in government or industry, such as in research groups in the government labs, in communications, in commercial pharmaceutical companies, and in banking/financial institutions. The department has an excellent track record in placing new PhDs.
Prerequisites for the Program
A student applying to the PhD program normally should have taken courses in advanced calculus, linear algebra, probability, and statistics. Additional courses in mathematics, especially a course in real analysis, will be helpful. Some facility with computer programming is expected. Students without background in all of these areas, however, should not be discouraged from applying, especially if they have a substantial background, through study or experience, in some area of science or other discipline involving quantitative reasoning and empirical investigation. Statistics is an empirical and interdisciplinary field, and a strong background in some area of potential application of statistics is a considerable asset. Indeed, a student's background in mathematics and in science or another quantitative discipline is more important than his or her background in statistics.
To obtain more information about applying, see the Guide For Applicants .
Students with questions may contact Yali Amit for PhD Studies, Mei Wang for Masters Studies, and Keisha Prowoznik for all other questions, Bahareh Lampert (Dean of Students in the Physical Sciences Division), or Amanda Young (Associate Director, Graduate Student Affairs) in UChicagoGRAD.
Handbook for PhD Students in Statistics
Information for first and second year phd students in statistics.
Meet Our New PhD Students!
We’ll be featuring mini-profiles of our new PhD students over the next few weeks. We look forward to welcoming them into our community!

Hi, my name is Hannah Jin, and I was born and raised in the Greater Philadelphia area. I just finished my undergraduate degree at the University of Pennsylvania (Penn). There, I was a Math major and Neuroscience minor.
As an undergraduate, I applied statistics to evaluate how social and environmental factors relate to clinical outcomes in frontotemporal degeneration (FTD), a neurodegenerative disease.
At the Penn FTD Center, I performed statistical analysis to explore how occupation, job-related environmental exposures, and genetics relate to cognitive reserve, disease progression, and survival in patients with FTD. Additionally, I applied statistics to study racial disparities in clinical presentation among individuals diagnosed with FTD, with the hope that this research will promote health equity by sparking further investigation into the social determinants underlying these disparities. I hope to continue contributing to public health through researching social and environmental determinants of health.
I also did causal inference research at the Penn Statistics and Data Science department in my junior and senior years. In one project, I researched how various types of childhood sports participation affect cognitive and emotional health in adolescents. In a methodological project, I worked on developing a novel statistical test for assessing whether covariate balance among matched quadruples has been achieved, which allows for a matched quadruple design for studying interaction effects.
I hope to contribute to biostatistical methods and public health research in causal inference, environmental health, and neurology at HSPH. Additionally, I am excited to explore other subfields of biostatistics.
Outside of school and research, I have been singing in choirs and a cappella groups for the past fourteen years, so I would love to join a choir at Harvard. I like painting and drawing, so I am excited to wander around art museums in the Boston area. I also like exercising, dancing, and singing karaoke. I am very excited to meet everyone!

Hi, my name is Ethan Lee. I’m from the Philadelphia area, but went to boarding school in New England, so I am excited to be back. I graduated from NYU in 2024 with a major in Mathematics and a minor in Computer Science.
My experience with health sciences began in high school, when I spent two summers working at a microbiology lab at the University of Pennsylvania School of Medicine. During my undergrad, I collaborated closely with a group from the Department of Population Health at NYU medical school. We primarily focused on the topic of domain generalization/domain adaptation, which arises during a prediction task when the training set is not an unbiased sample, that is it may only include data from certain prevalent domains in a population. I felt that this issue itself is highly relevant in today’s society, where minority groups are often underrepresented during sampling.
Our approach utilized a selection-guided approach, meaning we leveraged the fact each individual’s domain selection probability depended on their individual covariates. We eventually expanded this approach to more general contexts, including a semi- supervised approach which utilized unlabeled training data.
During my PhD studies at Harvard, I am keen on exploring more of the potential methods to analyze large datasets, such as EHR. Moreover, I am also interested in the aspects of developing statistics/machine learning methods, such as reinforcement learning, to analyze complex, high-dimensional data collected from mobile and wearable devices.
In my free time, I enjoy baseball statistics and am interested in utilizing statistical methods for areas such as in-season prediction and player evaluation metrics. I also enjoy tennis and squash.
News from the School

Red meat and diabetes

How for-profit medicine is harming health care

A tradition of mentoring

Promising HIV treatment
Get the Reddit app
/r/Statistics is going dark from June 12-14th as an act of protest against Reddit's treatment of 3rd party app developers. _This community will not grant access requests during the protest. Please do not message asking to be added to the subreddit._
[Q] Do any of you actually regret not doing a PhD in Statistics/Data Science
Hello, my goals are to work as a data scientist in industry. At this point I’m just kind of unsure if I should pursue a PhD or not. My goals are to just be a data scientist in the industry. For any of you in industry, and I’m sure this is also based on the specific industry type, do you guys regret not getting a PhD in statistics for your job? My plan is to get an MS in stats and work, but for any of you, did you regret not finishing through after your MS? If you do have regrets what are they, and how had the MS limited you if any?
By continuing, you agree to our User Agreement and acknowledge that you understand the Privacy Policy .
Enter the 6-digit code from your authenticator app
You’ve set up two-factor authentication for this account.
Enter a 6-digit backup code
Create your username and password.
Reddit is anonymous, so your username is what you’ll go by here. Choose wisely—because once you get a name, you can’t change it.
Reset your password
Enter your email address or username and we’ll send you a link to reset your password
Check your inbox
An email with a link to reset your password was sent to the email address associated with your account
Choose a Reddit account to continue
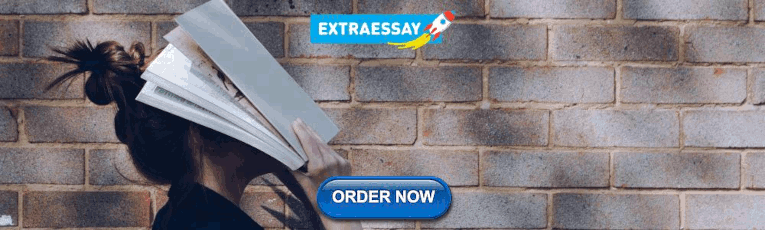
COMMENTS
Users share their experiences and opinions on pursuing a PhD in statistics, both for academic and non-academic careers. They highlight the challenges, benefits, and alternatives of a PhD in statistics.
A PhD is a research degree, but it is also a requirement for most stats teaching positions. You can teach high school with a BS. You can teach at a university with a MS, but most of those positions are adjunct. You almost always need a PhD to teach classes above the most basic level or to have any job security.
/r/Statistics is going dark from June 12-14th as an act of protest against Reddit's treatment of 3rd party app developers. _This community will not grant access requests during the protest. ... I'm applying to PhD programs in statistics this fall, with a very average academic profile (3.4/3.5 gpa). I'm a stats major and math minor, and my ...
Even with an irrelevant project, a PhD in statistics is going to give you some training that is useful in a general sense (better theory knowledge, better maths, etc.). Although there is value in this program, there is also a big opportunity cost. If you spend a standard full-time period of four years doing a PhD, that is going to be at the ...
A PhD in statistics is more flexible and useful that PhDs in some other areas. The usual issue with PhDs one hears about is that one becomes over-qualified for non-academic work once one has a PhD. Additionally, there is a lot of time spent getting it. However, statistics is intrinsically an applied science, and one that is in big demand across ...
I personally have a math Ph.D., then started to do applied statistics in a "classical" academic environment (analyzing psychology studies), finally ended up in a Data Science-type job. I fully agree that postgraduate work in statistics would be the best preparation for a career in Data Science.
The relatively new Ph.D. in Statistics strives to be an exemplar of graduate training in statistics. Students are exposed to cutting edge statistical methodology through the modern curriculum and have the opportunity to work with multiple faculty members to take a deeper dive into special topics, gain experience in working in interdisciplinary teams and learn research skills through flexible ...
So, if you have good record (not just grades but grasp), you may be able to get to the same level of salary as a PhD would. This is because statistics is an applied branch and is in demand. Share. Improve this answer. Follow answered Aug 22, 2012 at 22:22. Stat-R Stat-R. 2,635 ...
The PhD program prepares students for research careers in theory and application of probability and statistics in academic and non-academic (e.g., industry, government) settings. Students might elect to pursue either the general Statistics track of the program (the default), or one of the four specialized tracks that take advantage of UW's interdisciplinary environment: Statistical Genetics ...
Finishing my PhD next semester in Statistics and moving into industry at a big tech company. Absolutely worth it. ... /r/Statistics is going dark from June 12-14th as an act of protest against Reddit's treatment of 3rd party app developers. _This community will not grant access requests during the protest. Please do not message asking to be ...
In other words, if you're looking at the question through a purely financial lens, yes, a PhD in statistics is worth it. But there's one caveat. The lifetime earnings of a PhD vs a master's recipient in statistics isn't all too significant (on average about $3.6 million vs $3.45 million).
1. Fair enough. All the programs I am now considering are in the United States. Some focus on the more applied side and give masters degrees in "applied statistics" while others have more theoretical coursework and grant degrees in "statistics". I'm personally not that intent on working in one industry over another.
The Statistics PhD program is rigorous, yet welcoming to students with interdisciplinary interests and different levels of preparation. Students in the PhD program take core courses on the theory and application of probability and statistics during their first year. The second year typically includes additional course work and a transition to ...
See the list of alumni for examples. Department of Statistics and Data Science. Yale University. Kline Tower. 219 Prospect Street. New Haven, CT 06511. Mailing Address: PO Box 208290, New Haven, CT 06520-8290. Shipping Address (packages and Federal Express): 266 Whitney Avenue, New Haven, CT 06511.
Salaries for statisticians in academia can range from $60,000 to more than $150,000 per year, or even higher for highly talented and experienced statisticians working in famous institutions. In the workplace, salaries for statisticians with a PhD might vary greatly based on the industry, employer, and job duties.
There's sometimes a mild prejudice that people in mathematical statistics are overly specialized and outside of the mainstream of mathematics. For example, it's possible to get a Ph.D. in mathematical statistics while having considerably less breadth of mathematical background than would be considered acceptable for a math grad student.
Outside of academia, PhD degrees are more often hired by biostat consulting firms and pharmaceutical companies to handle complex data analysis. All in all, if you want to be involved in the actual study and not just the data analysis, masters degrees are probably the way to go. 4. MD/MPH or MD/PhD.
/r/Statistics is going dark from June 12-14th as an act of protest against Reddit's treatment of 3rd party app developers. _This community will not grant access requests during the protest. ... In a statistics PhD your first year will mostly be courses anyway, and I think coming right from undergrad you are best prepared for these courses ...
10 Top Schools for a Doctorate in Stats. 1. University of Chicago. Chicago, IL. 6 Annual Graduates. It is difficult to beat University of Chicago if you wish to pursue a doctor's degree in statistics. Located in the large city of Chicago, UChicago is a private not-for-profit university with a large student population.
Students are required to. The PhD requires a minimum of 135 units. Students are required to take a minimum of nine units of advanced topics courses (for depth) offered by the department (not including literature, research, consulting or Year 1 coursework), and a minimum of nine units outside of the Statistics Department (for breadth).
PhD Degree in Statistics. The Department of Statistics offers an exciting and recently revamped PhD program that involves students in cutting-edge interdisciplinary research in a wide variety of fields. Statistics has become a core component of research in the biological, physical, and social sciences, as well as in traditional computer science ...
Users share their opinions and experiences on whether to pursue a PhD in statistics after working in industry for several years. Some argue that it is not worth it for career advancement, while others suggest alternative paths or fields.
During my PhD studies at Harvard, I am keen on exploring more of the potential methods to analyze large datasets, such as EHR. Moreover, I am also interested in the aspects of developing statistics/machine learning methods, such as reinforcement learning, to analyze complex, high-dimensional data collected from mobile and wearable devices.
The ones looking for quantitative PhDs are looking for that training for a reason, e.g. taking statistics research and implementing it for their experimentation platform or similar take-academic-research-and-apply-it work in the case of CompSci PhDs. You definitely don't need a PhD to secure a product DS role.
A year of college in the U.S. costs $38,270 a year, on average, according to the Education Data Initiative, a research nonprofit that collects education statistics. College costs have doubled ...