
An official website of the United States government
The .gov means it’s official. Federal government websites often end in .gov or .mil. Before sharing sensitive information, make sure you’re on a federal government site.
The site is secure. The https:// ensures that you are connecting to the official website and that any information you provide is encrypted and transmitted securely.
- Publications
- Account settings
Preview improvements coming to the PMC website in October 2024. Learn More or Try it out now .
- Advanced Search
- Journal List
- Comput Struct Biotechnol J
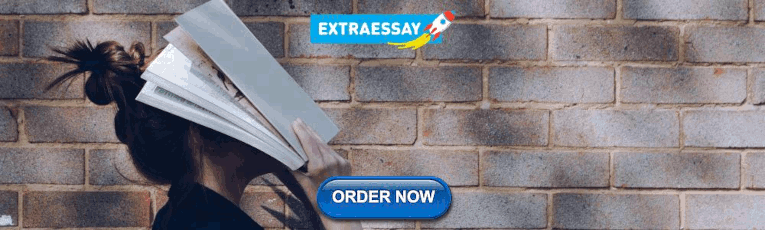
Association of risk factors with type 2 diabetes: A systematic review
Leila ismail.
a Intelligent Distributed Computing and Systems Research Laboratory, Department of Computer Science and Software Engineering, College of Information Technology, United Arab Emirates University, Al Ain, Abu Dhabi, 15551, United Arab Emirates
Huned Materwala
Juma al kaabi.
b College of Medicine and Health Sciences, Department of Internal Medicine, United Arab Emirates University, Al Ain, Abu Dhabi 15551, United Arab Emirates
c Mediclinic, Al Ain, Abu Dhabi, United Arab Emirates
Diabetes is the leading cause of severe health complications and one of the top 10 causes of death worldwide. To date, diabetes has no cure, and therefore, it is necessary to take precautionary measures to avoid its occurrence. The main aim of this systematic review is to identify the majority of the risk factors for the incidence/prevalence of type 2 diabetes mellitus on one hand, and to give a critical analysis of the cohort/cross-sectional studies which examine the impact of the association of risk factors on diabetes. Consequently, we provide insights on risk factors whose interactions are major players in developing diabetes. We conclude with recommendations to allied health professionals, individuals and government institutions to support better diagnosis and prognosis of the disease.
1. Introduction
Diabetes Mellitus (DM) commonly referred to as diabetes, is a chronic disease that affects how the body turns food into energy [1] . It is one of the top 10 causes of death worldwide causing 4 million deaths in 2017 [2] , [3] . According to a report by the International Diabetes Federation (IDF) [3] , the total number of adults (20–79 years) with diabetes in 2045 will be 629 million from 425 million in 2017 (48% increase). In 2017, diabetes caused at least 727 billion USD in health expenditure, which is 12% of the total spending on adults [3] . According to the National Diabetes Statistics Report [4] , 30.3 million (9.4% of the US population) people have diabetes, and 84.1 million (29.06% of the population) have pre-diabetes. 1 in 2 people (212 million) with diabetes was undiagnosed in 2017 according to IDF [5] . Diabetes if left untreated can cause serious medical issues, such as cardiovascular disease, stroke, chronic kidney disease, foot ulcers, damage to the eyes, and prolonged kidney ailment. To date, there is no permanent cure for diabetes and the patients have to rely on healthy lifestyle and timely medication [6] .
There are three main types of diabetes: type 1, type 2, and gestational diabetes (diabetes while pregnant) [1] . Type 1 diabetes mostly occurs in children and adolescents. 1,106,500 children were suffering from type 1 diabetes in 2017 [3] . The symptoms of type 1 diabetes include abnormal thirst and dry mouth, frequent urination, fatigue, constant hunger, sudden weight loss, bed-wetting, and blurred vision. Type 2 diabetes is mostly seen in adults, but it is increasing in children and adolescents due to the rising level of obesity, physical inactivity and unhealthy diet [5] . 372 million adults were at the risk of developing type 2 diabetes in 2019 [3] . In 2017, more than 21 million live births were affected by diabetes during pregnancy [3] . In this paper, we focus on type 2 diabetes due to the alarming numbers.
Type 2 Diabetes is thought to prevail in an individual from an interaction between several lifestyle, medical condition, hereditary, psychosocial and demographic risk factors such as high-level serum uric acid, sleep quality/quantity, smoking, depression, cardiovascular disease, dyslipidemia, hypertension, aging, ethnicity, family history of diabetes, physical inactivity, and obesity [6] . In this paper, we present a systematic review of the literature on the association of these risk factors with the incidence/prevalence of type 2 diabetes. We give insights on the contribution of independent risk factors in the development of type 2 diabetes along with possible solutions towards a preventive approach.
We conduct a systematic literature search using CINAHL, IEEE Xplore, Embase, MEDLINE, PubMed Central, ScienceDirect, Scopus, Springer, and Web of Science databases. Our search criteria does not include a time bound. Its main objective is to retrieve all the studies which examine the association between individual risk factors and the incidence/prevalence of type 2 diabetes. Table A1 shows the search string used for each risk factor. The relevant studies have to meet the following inclusion criteria: 1) published in the English language, 2) prospective cohort or cross-sectional study, 3) type 2 diabetes as a specified risk, 4) one of its risk factors, 5) findings in terms of Odds Ratio (OR), Risk Ratio/Relative Risk (RR), or Hazard Ratio (HR), and the corresponding 95% Confidence Intervals (CIs) for the association between the risk factor and type 2 diabetes. To assess the quality of the studies, we use the National Institutes of Health (NIH) quality assessment tool [7] . The tool consists of 14 questions to evaluate the validity and bias risk of a study. We answered each question by either yes, no, cannot be determined, not applicable, or not reported. The tool then classifies each study as high quality (Good), moderate quality (Fair) and low quality (Poor).
Fig. 1 shows the result of our systematic approach that is used to screen the relevant studies. Irrelevant studies that do not meet the inclusion criteria mentioned in the previous section were excluded after screening titles, abstracts and full texts. At last, 106 papers are considered for this review. These papers are divided into ten categories based on the risk factor under study ( Fig. 1 ). Our review reveals that there is no study that examines the association of age or physical inactivity as an independent risk factor with type 2 diabetes. Table A2 shows the quality assessment results for the studies included in this paper. For smoking, cardiovascular disease and hypertension risk factors, the majority of the studies are of high quality. For serum uric acid, sleep quantity/quality, depression, dyslipidemia, ethnicity, family history of diabetes and obesity, the majority of the studies are of moderate quality.

Flowchart of the selection of relevant studies.
3.1. Serum uric acid
Serum uric acid, a common component of urine generated by the metabolic breakdown of purines, have been associated with insulin resistance and type 2 diabetes [8] . High serum uric acid level in an individual leads to: 1) nitric-oxide mediated vasoconstriction (contraction of blood vessels) leading to impaired glucose uptake in the muscles [9] , 2) increase in oxidative stress [10] and 3) increase in inflammation leading to a decrease in adiponectin [11] , [12] . Consequently, the blood glucose level increases leading to dysfunctional and eventually dead beta-cells [13] . As a result, the individual develops type 2 diabetes. Table 1 shows the characteristics and findings of the work in the literature studying the association between high serum uric acid level and type 2 diabetes.
Characteristics and findings of the studies examining the association between high level serum uric acid and type 2 diabetes.
RS-Random Sample, MONICA-Multinational MONItoring of trends and determinants in CArdiovascular disease, ARIC-Atherosclerosis Risk in Communities, FDPS-Finnish Diabetes Prevention Study, CSCCS-Chin Shan Community Cardiovascular study, MRFIT-Multiple Risk Factor Intervention Trial, NHANES-National Health and Nutrition Examination Survey, QFS-Quebec Family Study, M-Men, W-Women, PCS-Prospective Cohort Study, CSS-Cross-Sectional Study.
Perry et al. [14] found that an individual having a uric acid level of more than 411 μ mol/l is at 1.5 times more risk of developing type 2 diabetes compared to an individual having uric acid level less than 302 μ mol/l. Niskanen et al. [15] also confirmed that change in uric acid levels is associated with a 2 times increase in the risk of incidence type 2 diabetes. Dehghan et al. [16] in their study showed that individuals having uric acid level > 370 μ mol/l are at high risk of incidence type 2 diabetes (HR 1.68, 95% CI 1.22–2.30) compared to those having uric acid level ⩽ 267 μ mol/l. The authors concluded that lowering uric acid level can be a novel approach for diabetes prevention. Xu et al. [17] found that the association between high serum uric acid level and diabetes is the same in both men and women (RR 1.131, 95% CI 1.084–1.179). The association (RR 1.17, 95% CI 1.09–1.25) is also examined by Kodama et al. [18] . Nakagawa et al. [19] showed that uric acid is a significant and independent risk factor in predicting hyperinsulinemia. The authors observed that serum uric acid level ⩾ 5.5 mg/dl is associated with the development of hyperinsulinemia after 6 months (OR 5.47, 90% CI 1.6–1.77) and 12 months (OR 3.4, 90% CI 1.1–10.4). However, the cohort was controlled for gender and age ( > 60 years). Consequently, it can not be concluded whether uric acid is an independent risk factor or there is an integrated effect of uric acid, gender and age.
Several studies argue that high-level uric acid is not an independent risk factor and it only emphasizes the association between independent risk factors such as age, obesity, hypertension, gender, and dyslipidemia, and type 2 diabetes [20] . Chou et al. show that uric acid has a significant association with type 2 diabetes in old and obese individuals [21] . Another study by Meisinger et al. [22] shows that high-level uric acid is associated with incidence of type 2 diabetes in women only with HR 2.5 per 1 mmol/L increase. Carnethon et al. [23] found that the risk of incidence type 2 diabetes increases (OR 1.3, (1.2–1.4)) with every 1.4 mg/dl increase in uric acid level. However, this is in combination with an increase in waist/hip ratio, smoking and obesity. Chien et al. [24] stated that individuals with a uric acid level of 0.486 mmol/L and having metabolic syndrome have a 3.3 times more risk of incidence type 2 diabetes compared to those with a uric acid level of 0.211 mmol/L and not having metabolic syndrome. Nan et al. [25] examined the impact of ethnicity and gender on the association between uric acid and incidence of type 2 diabetes. The authors found that the high serum uric acid is an independent risk factor for type 2 diabetes in Mauritian Indian men compared to Creole men, and there is a no-to-weak association in women of both ethnicity. Similarly, Choi et al. [26] studied the association between uric acid and type 2 diabetes in men having cardiovascular risk profile. The authors concluded that men with cardiovascular profile having high uric acid level are twice likely to develop type 2 diabetes. The authors also stated that this association between uric acid and diabetes is independent of other risk factors such as obesity, age, family history of diabetes, hypertension, and metabolic syndrome. Kramer et al. [27] analyzed the impact of age and impaired fasting glucose (IFG) on the association and found that high uric acid level can independently predict incidence of type 2 diabetes (OR 1.65, 95% CI 1.25–2.18) in older adults having IFG. Lv et al. [28] found that high serum uric acid level is associated to type 2 diabetes in middle-aged or older people (RR 1.56, 95% CI 1.39–1-76).
In summary, the association between high-level serum uric acid remains obscure. It is debatable whether serum uric acid is an independent risk factor for type 2 diabetes or it only emphasizes the association between other independent risk factors and type 2 diabetes. Some studies reported a positive association between high serum uric acid level and incidence of type 2 diabetes [14] , [15] , [16] , [19] , [24] , whereas others [25] , [29] reported no association. On the contrary, some studies reported an inverse association between uric acid and diabetes [30] , [31] , [32] . Furthermore, some studies argue that there is a reverse association, i.e., diabetes leads to high uric acid levels [33] , [34] .
3.2. Sleep quantity/quality
The quality and quantity of sleep are affected by several cultural, social, behavioral, psychological, and environmental factors. The working professionals often experience fatigue, tiredness and daytime napping due to irregular working hours and shifts. Evidence shows that the current average sleep of an individual, i.e., 6.8 h/night, is 1.5 h less than that a century ago [45] . The cause of sleep loss is multi-factorial. For instance 45% of adults report that they sleep fewer hours to get more work done, 43% reported that they watch television or use the Internet, and 22% reported to be suffering from insomnia. The unusual, disturbed and reduced sleep is associated with glucose intolerance [46] .
An individual suffering from sleep disorder, known as obstructive sleep apnea (OSA), experiences: 1) deficiency in the amount of oxygen reaching the tissues by total/partial collapse of upper airways while sleeping (hypoxia) and 2) inflammation. Frequent Hypoxia triggers an increase in sympathetic activity [47] . Increased sympathetic activity and inflammation lead to insulin resistance condition [48] , [49] and eventually to type 2 diabetes. Table 2 shows the characteristics and findings of the work in the literature studying the association between sleep quantity/quality and type 2 diabetes.
Characteristics and findings of the studies examining the association between sleep quantity/quality and type 2 diabetes.
DIS-Difficulty Initiating Sleep, DMS-Difficulty Maintaining Sleep, EPIC-European Prospective Investigation into Cancer and Nutrition, FIN D2D-Finnish type 2 Diabetes, HIPOP-OHP-High risk and Population Strategy for Occupational Health Promotion, IHHP-Isfahan Healthy Heart Program, IRAS-Insulin Resistance Atherosclerosis Study, M-Men, MC-Millennium Cohort, MMAS-Massachusetts Male Aging Study, MONICA-Multinational MONItoring of trends and determinants in CArdiovascular disease, MPP-Malmo Preventive Project, NHANES-National Health and Nutrition Examination Survey, NHIS-National Health Interview Survey, NHS-Nurse Health Study, NHW-Non Hispanic Whites, NIH AARP-National Institutes of Health American Association of Retired Persons Diet and Health Study, QFS-Quebec Family Study, RS-Random Sample, SHHS-Sleep Heart Health Study, W-Women, PCS-Prospective Cohort Study, CSS-Cross-Sectional Study.
The results in the literature show that compared to a reference sleep duration of 7-8 h, an individual having either short sleep duration ( < 6 h) or long sleep duration ( > 8 h) is at high risk of developing type 2 diabetes. However, [50] , [51] concluded that there is no significant association between sleep and incidence of type 2 diabetes. Mallon et al. [52] studied the impact of gender on the association between sleep and diabetes. The authors concluded that short sleep duration increases the risk of incidence diabetes in men, whereas, in women, long sleep duration dominates. The effect of ethnicity on the association is analyzed by [53] , [54] , [55] . Zizi et al. [53] and Jackson et al. [54] showed that the prevalence of type 2 diabetes is more in whites who sleep less than 5 h or more than 8 -9 h compared to blacks. Beihl [55] showed that the association is more in Hispanics/Non-Hispanic Whites compared to that in African-American. Xu et al. examined the association between day-time napping and type 2 diabetes and showed that an individual taking more than 1 h of day-time nap is at 1.5 times more risk to develop diabetes compared to an individual who does not take a nap during the day. In the context of sleep quality, the risk of incidence type 2 diabetes is more in an individual having difficulty initiating sleep (DIS), and the risk increases with increasing DIS frequency [56] , [57] , [58] . Furthermore, the association is more in women having DIS compared to men [59] .
In summary, there is a strong association between sleep quantity/quality and the incidence of type 2 diabetes. The association is stronger in women sleeping for more duration and in men with short sleep duration. Moreover, this association is affected by ethnicity.
3.3. Smoking
Smoking leads to more than 8 million deaths per year [60] . This is from both active and passive uses, i.e, non-smokers exposed to smokers. Smokers are 30–40% more likely to develop type 2 diabetes compared to non-smokers [61] . When an individual smokes, the level of nicotine increases in his/her body. This leads to a reduction in muscle glucose intake, developing insulin resistance and leading to type 2 diabetes [62] . The characteristics and findings of table:smokingtable:smoking/passive smoking and the incidence of type 2 diabetes are presented in Table 3 .
Characteristics and findings of the studies examining the association between smoking and type 2 diabetes.
ZS-Zutphen Study, NHS-Nurse Health Study, NHIS-National Health Interview Survey, HPFS-Health Professionals’ Follow-up Study, RS-Random Sample, SOF-Study of Osteoporotic Fractures, OHS-Osaka Health Survey, PHS-Physicians Health Survey, BRHS-British Regional Health Study, CPS-Cancer Prevention Study, NCDS-National Child Development Study, RIH-Regional Institute for Health, NTHS-Nord Trondelag Health Survey, IRAS-Insulin Resistance Atherosclerosis Study, ARIC-Atherosclerosis Risk in Communities, KCPS-Korean Cancer Prevention Study, JPHC-Japan Public Health Center, WHI-Women Health Initiative, KMIC-Korean Medical Insurance Corporation, M-Men, W-Women, PCS-Prospective Cohort Study, CSS-Cross-Sectional Study.
The results in the literature show that the association between smoking and diabetes increases with an increase in the number of cigarettes smoked/day. Will et al. [63] analyzed the impact of gender on this association and showed that the association between cigarette smoking and type 2 diabetes is more in men compared to women. Similar results are obtained by Jee et al. [64] . Wannamethee et al. [65] revealed that an individual smoking pipe/cigar is 2.15 times more likely to develop type 2 diabetes and an individual smoking cigarette is 1.6 times more likely compared to a non-smoker. Kowall et al. [66] showed that the risk of incidence type 2 diabetes is significantly high in active/passive prediabetic smokers compared to active/passive smokers without prediabetes.
The incidence and prevalence of type 2 diabetes in ex-smokers is examined by [67] , [68] , [69] , [70] , [71] , [72] . and [73] respectively. Results show that ex-smokers are associated with 17–60% increased risk of type 2 diabetes [67] , [68] , [70] , [71] , [72] . However, the results obtained by Simon et al. [73] and Manson et al. [69] showed no association between ex-smokers and type 2 diabetes. This discrepancy in the results can be due to the heterogeneous characteristics (sample size, age range, men/women ratio and ethnicity) of the cohorts used in these studies. Beziaud et al. [74] examined gender-based prevalence of type 2 diabetes in ex-smokers and showed that women are at higher risk compared to men. Furthermore, the duration of smoking cessation also impacts the association in ex-smokers [65] , [75] , [76] , [77] . An individual is at high risk of developing type 2 diabetes during first 5–10 years of smoking cessation. The risk then decreases with an increase in cessation duration. The association between smoking cessation and the incidence of type 2 diabetes is more in women than men [78] .
In summary, both active and passive smoking are strongly associated with the incidence of type 2 diabetes. The association is more in men compared to women. Moreover, the association remains significant in ex-smokers during first the 5–10 years of smoking. After 10 years of smoking cessation, the risk of incidence type 2 diabetes is the same as that in a non-smoker. Women ex-smokers are at a higher risk of developing diabetes compared to men ex-smokers.
3.4. Depression
Depression is a mood disorder that negatively affects the way a person feels, thinks and acts [130] . It can be due to a family history of depression, early childhood trauma, brain structure, medical conditions, drug use or surrounding environment. Depression is associated with multiple health conditions including diabetes [131] . It elevates the sympathetic nervous system activities and hypothalamic–pituitary–adrenal axis activities [132] . Elevated sympathetic nervous system activities lead to an increase in catecholamines and inflammation, and eventually causing insulin resistance [133] . On the other hand, elevated adrenal axis activities lead to an increase in cortisol and eventually blood sugar level [134] . Both insulin resistance and increased blood sugar levels develop type 2 diabetes. The characteristics and findings of the work in the literature examining the association between depression and the incidence of type 2 diabetes are presented in Table 4 .
Characteristics and findings of the studies examining the association between depression and type 2 diabetes.
RS-Random Sample, SDS-Self rating Depression Scale, ECAPS-Epidemiologic Catchment Area Program Survey, NHANES-National Health and Nutrition Examination Survey, ARIC-Atherosclerosis Risk in Communities, RNH-RegistratieNet Huisarts Praktijken, SWAN-Study of Womens’ Health Across the Nation, NTHS-Nord Trondelag Health Study, CHS-Cardiovascular Health Study, CESD-Center for Epidemiological Studies Depression Scale, RBHCDS-Rancho Bernardo Heart and Chronic Disease Study, BDI-Beck Depression Inventory, M-Men, W-Women, PCS-Prospective Cohort Study, CSS-Cross-Sectional Study.
The results show that depression is highly associated with the incidence of type 2 diabetes. In the context of gender, depressed men are at higher risk of incidence type 2 diabetes, whereas depression in women is not associated with type 2 diabetes [135] . Moreover, compared to Caucasian, Hispanic, Japanese-American and Chinese-American, depressed African-Americans are at 2.56 times higher risk of incidence type 2 diabetes [136] . Based on self rating depression scale (SDS) score, an individual having a score of 48–80 is at higher risk of developing diabetes compared to an individual having a score of 20–39 [137] . Similarly, an individual having a score ⩾ 11 using center for epidemiological studies depression scale (CES-D) or a score ⩾ 8 using beck depression inventory (BDI) is at higher risk of incidence type 2 diabetes [138] , [139] .
In summary, depression is associated with type 2 diabetes. However, the association is different in men and women. Moreover, the study by Yu et al. [140] show that depression itself is not a risk factor for diabetes, rather the activities related to depression such as physical inactivity, poor diet, and obesity lead to diabetes. In addition, the medical drugs used to treat depression also have an association with the incidence of type 2 diabetes. Consequently, similar to high-level serum uric acid, depression is not an independent risk factor but it emphasizes the impact of other independent risk factors such as gender, ethnicity, physical inactivity, and obesity.
3.5. Cardiovascular disease
Increased heart rate and cardiovascular disease can elevate the blood pressure in the arteries. As a result, the body’s glucose uptake decreases leading to insulin resistance condition. Consequently, a person suffering from heart disease is at a higher risk of developing type 2 diabetes. However, this association is still obscure. Few studies argue that a history of cardiovascular disease leads to the incidence of type 2 diabetes [141] , while others claim that type 2 diabetes increases the risk of cardiovascular disease [142] , [143] , [144] . Yeung et al. [141] examined the association between family history of coronary heart disease (CHD) and type 2 diabetes ( Table 5 ). The authors concluded that a high family CHD score is associated to the incidence of type 2 diabetes in individuals who have a positive history of family diabetes. For the individuals having a negative family history of diabetes, this association was non-significant. In summary, it is debatable whether cardiovascular disease is a risk factor for type 2 diabetes or not.
Characteristics and findings of the studies examining the association between cardiovascular disease and type 2 diabetes.
ARIC-Atherosclerosis Risk in Communities, CDH-Coronary Heart Disease, M-Men, W-Women, PCS-Prospective Cohort Study.
3.6. Dyslipidemia
Dyslipidemia refers to an abnormal level of lipids, such as triglycerides and cholesterol. It is characterized by high triglyceride levels, increased low-density lipoproteins (LDL) levels and decreased high-density lipoproteins (HDL) levels [145] . Elevated LDL and lowered HDL levels lead to beta-cell dysfunction inhibiting insulin secretion and consequently type 2 diabetes [146] , [147] . Table 6 shows the characteristics and findings of the work in the literature studying the association between dyslipidemia and type 2 diabetes.
Characteristics and findings of the studies examining the association between dyslipidemia and type 2 diabetes.
LWHS-Lowa Women’s Health Study, CCHS-Copenhagen City heart Study, CGPS-Copenhagen General Population Study, MA-Meta Analysis, REACTION-Risk Evaluation of cAncers in Chinese diabeTic Individuals: a lONgitudinal study, RS-Random Sample, M-Men, W-Women, PCS-Prospective Cohort Study.
Dietary fats, that raise the total cholesterol and LDL levels, are considered significant in the development of type 2 diabetes [148] . Substituting saturated fatty acid with polyunsaturated fatty acid and animal fat with vegetable fat can help lower blood cholesterol and eventually type 2 diabetes. This is because both polyunsaturated fatty acid and vegetable fat are inversely related to the risk of incidence type 2 diabetes with RR 0.84 (95% CI 0.71–0.98) and RR 0.78 (95% CI 0.67–0.91) respectively for the highest quintile of intake [148] . Tajima et al. [149] also confirmed the association between high cholesterol diet intake ( > 273 mg/day) and type 2 diabetes (RR 1.25, 95% CI 1.16–1.36) compared to low cholesterol intake ( < 185 mg/day).
In order to reduce elevated LDL level, LDL lowering therapy and drugs are suggested. However, these drugs and therapy are found to be associated with a higher risk of type 2 diabetes [150] . Individuals having familial hypercholesterolemia, a genetic disorder that results in high LDL levels, are less likely to have type 2 diabetes compared to individuals having high LDL levels due to dietary patterns [151] . Zhang et al. [152] in their analysis found that the ratio of non-HDL and HDL levels is an independent risk factor for incidence diabetes. They show that an individual having a ratio of 3.1 is at 40% increased risk of incidence diabetes (OR 1.4, 95% CI 1.1–1.8) compared to an individual having a ratio of 1.4. Elevated non-HDL and lowered HDL levels are significantly associated with incidence diabetes [153] .
On the contrary to studies confirming the association between low-HDL levels and the incidence of type 2 diabetes, Haase et al. [154] in their study concluded that a life-long reduction in HDL levels are not associated with an increased risk of type 2 diabetes. They found that the association is most likely reverse causation, i.e., type 2 diabetes leads to low HDL levels.
3.7. Hypertension
Hypertension, also known as high blood pressure, is a medical condition in which the blood pressure in the arteries is persistently elevated. Hypertension elevates the sympathetic nervous system activity leading to a decrease in the body’s glucose uptake. This causes the condition of insulin resistance and eventually type 2 diabetes. Hypertension elevates sympathetic nervous system activities leading to impaired vasodilation of skeletal muscles. Consequently, muscle glucose uptake decreases with the eventual development of type 2 diabetes. Table 7 shows the characteristics and findings of the work in the literature studying the association between hypertension and type 2 diabetes.
Characteristics and findings of the studies examining the association between hypertension and type 2 diabetes.
RS-Random Sample, ARIC-Atherosclerosis Risk in Communities, WHS, Women’s Health Study, CARDIA-Coronary Artery Risk Development in Young Adults, FHS-Framingham Heart Study, GPPS-Gothenburg Primary Prevention Study, KGES-Korean Genome and Epidemiology Study, M-Men, W-Women, PCS-Prospective Cohort Study.
Hayashi et al. [166] examined the association between high normal blood pressure ( ⩾ 130 and < 140 mmHg/ ⩾ 85 and < 90) and hypertension ( ⩾ 140 mmHg/ ⩾ 90 mmHg), and the incidence of type 2 diabetes in men. The authors concluded that both high normal blood pressure (RR 1.39, 95%1.14–1.69) and hypertension (RR 1.75, 95% CI 1.43–2.16) are associated with an increased risk of type 2 diabetes. This association is dependent on obesity and hypertension medications. Hypertension medications are considered to increase the risk of diabetes depending on the type of medication [167] . For instance, hypertensive individuals taking thiazide diuretics and angiotensin-converting-enzyme medications are at lower risk of diabetes compared to the hypertensive individuals not taking any medication. However, those taking beta-blockers medication are at 28% higher risk of incidence type 2 diabetes (HR 1.28, 95% CI 1.04–1.57) [167] . The association between hypertension and the incidence of type 2 diabetes is significant in women as well [168] . Women having hypertension are at 2 times increased risk of developing diabetes (HR 2.03, 95% CI 1.77–2.32) compared to women having normal blood pressure ( < 120/75) [168] . The association is more in overweight and obese women. Irrespective of gender, prehypertension (HR 1.27, 95%CI 1.09–1.48) and hypertension (HR 1.51, 95% CI 1.29–1.76) are associated with increased risk of incidence type 2 diabetes [169] . In the context of ethnicity, whites individuals having hypertension are at higher risk of developing diabetes (HR 1.25, 95% CI 1.03–1.53), but no such association is seen in African American hypertensive individuals (HR 0.92, 95% CI 0.70–1.21) [170] .
In summary, hypertension is associated with the development of type 2 diabetes in both men and women. However, the association is ethnicity-dependent. The selection of hypertensive medications should be made properly as the medication impacts the strength of the association. Furthermore, an obese individual with hypertension is at higher risk compared to a non-obese.
The number of elderly people (above 60 years) is increasing worldwide. The 900 million global elderly population in 2015 is expected to rise to 2 billion by 2050 [171] . Aging increases the risk of metabolic syndrome and chronic diseases including type 2 diabetes. Aging increases chronic inflammation in an elderly individual leading to insulin resistance [172] . In addition, lipid metabolism disorder due to aging increases the accumulation of body fat leading to elevated free fatty acids concentration in the blood/plasma and eventually insulin resistance [173] . Consequently, an aged individual is at higher risk of developing type 2 diabetes. However, there is not much work concluding that aging is an independent risk factor for type 2 diabetes. Choi et al. [174] concluded that the risk of diabetes increases with aging only in overweight individuals, and the risk decreases with a moderate level of physical activity. Aging can be considered as triggering the association between independent risk factors and risk of diabetes, but more evidence and studies are required to examine the association between aging as an independent factor and diabetes.
3.9. Ethnicity
Ethnicity is associated with a range of health complications including diabetes because of the heterogeneity in the demographic environmental conditions and lifestyle. It is an independent risk factor which tends to be exacerbated by the social disadvantage and the affluent way of living. Table 8 shows the characteristics and findings of the work in the literature studying the association between ethnicity and type 2 diabetes. Compared to white individuals, type 2 diabetes is more prevalent in Pacific Islanders (OR 3.1, 95% CI 1.4–6.8), followed by Blacks (OR 2.3, 95% CI 2.1–2.6), Native Americans (OR 2.2, 95% CI 1.6–2.9), Hispanics (OR 2.0, 95% CI 1.8–2.3), and Multiracial (OR 1.8, 95% CI 1.5–2.9) [175] . In another study by Shai et al. [176] , it was found that compared to whites, Asians (RR 1.94, 95% CI 1.46–2.58), Hispanics (RR 1.70, 95% CI 1.28–2.26), and Blacks (RR 1.36, 95% CI 1.14–1.63) are at higher risk of incidence type 2 diabetes.
Characteristics and findings of the studies examining the association between ethnicity and type 2 diabetes.
RS-Random Sample, BRFSS-Behavioral Risk Factor Surveillance System, NHS-Nurses’ Health Study, PCS-Prospective Cohort Study.
A study by Zimmet et al. [177] showed that type 2 diabetes is 10 times more prevalent in rural Indians compared to rural Melanesians, and 2 times more prevalent in urban Indians compared to urban Melanesians. They also revealed that the prevalence is 5 times more in urban Melanesians compared to rural Melanesians. One of the reason could be that the rural residents have an increased amount of physical activity compared to the urban ones, leading to decreased risk of diabetes [178] . It should thus important to have a moderate amount of physical activity as a therapy for diabetes prevention. Compared to Europeans, type 2 diabetes is 3.8 times more prevalent in Indians, and the prevalence increases to 5 times for 40–64 years old individuals [179] . In another comparison between Asian and non-Asian ethnicity, it is found that the prevalence of type 2 diabetes in Bangladeshis (Asians) is more [180] . Furthermore, the prevalence is high in women (5.75 times) compared to that in men (2.2 times). However, ethnicity can not be considered as an independent risk factor for this association as Bangladeshis had higher smoking rates and a lower ratio of polyunsaturated fatty acids to saturated fatty acids. Consequently, ethnicity, smoking and dyslipidemia all contributed to the risk of incidence type 2 diabetes. Simmons et al. [181] also confirmed in their study that the prevalence is more in Asians compared to Whites. However, in contrast to the results obtained by [180] , Simmons et al. [181] found that the prevalence is more in men compared to women. This inconsistency should be examined further.
In summary, ethnicity is associated with the incidence of type 2 diabetes. However, there is no definite explanation of why individuals of a particular ethnicity are at higher risk of type 2 diabetes compared to the others. One possible explanation can be the ethnicity-dependent relation between BMI and body fat. For instance, Asians have around 3–4 kg/ m 2 lower BMI compared to Caucasians for a given percentage of body fat [182] . Another reason could be ethnicity-based insulin sensitivity. Studies show that Asians, Blacks and Mexican Americans are less insulin sensitive compared to non-Hispanic Whites [183] , [184] .
3.10. Family history of diabetes
Family history information can serve as a useful tool for prognosis/diagnosis and public health. Family history of diabetes reflects both genetic as well as environmental factors and can lead to better prediction of incidence type 2 diabetes than only genetic factors and environmental factors alone [192] . Table 9 shows the characteristics and findings of the work in the literature studying the association between family history of diabetes and type 2 diabetes.
Characteristics and findings of the studies examining the association between family history of diabetes and type 2 diabetes.
RS-Random Sample, SAHS-San Antonio Heart Study, MRFIT-Multiple Risk Factor Intervention Trial, MA-Meta Analysis, THHP-The Honolulu Heart Program, EPIC-European Prospective Investigation into Cancer, FHS-Framingham Heart Study, MONICA-Multinational MONItoring of trends and determinants in CArdiovascular disease, NHANES-National Health and Nutrition Examination Survey, PD-Prediabetes, IFG-Impaired Fasting Glucose, IGT-Impaired Glucose Tolerance, M-Men, W-Women, PCS-Prospective Cohort Study, CSS-Cross-Sectional Study.
A study by Tsenkova et al. [193] revealed that a family history of diabetes is strongly associated with incidence diabetes (OR 2.77, 95% CI 2.03–3.78). Another study also shows that parental history of diabetes is an independent risk factor for diabetes (OR 1.73, 95% CI 1.29–2.33) [194] . However, the association becomes weaker in men free of cardiovascular disease (OR 1.63, 95% CI 1.18-.2.24). Moreover, the association is much higher in 45–54 years old men (OR 1.99, 95% CI 1.38–2.89) compared to 55–68 years old men (OR 1.33, 95% CI 0.70–2.52). Furthermore, the prevalence of type 2 diabetes is stronger in men compared to women [195] . This indicates that parental history of diabetes in combination with other risk factors such as aging, gender and cardiovascular diseases, increases the risk of incidence type 2 diabetes.
Rodríguez-Moran et al. [196] showed that a family history of diabetes in first degree of relative (parents, offspring and siblings) is a strong and independent risk factor for the prevalence of impaired fasting glucose (prediabetes) (OR 11.7, 95% 9.5–21.2) in children and adolescents. This is in the absence of obesity. The results reveal that is it important to consider the parental history of diabetes while screening for diabetes children and adolescents. This is because only obesity-based screening could lead to underestimation. Valdez et al. [197] also showed that the family history of diabetes in at least two first-degree relatives or one first-degree and at least two second-degree relatives is significant for prevalence of type 2 diabetes. However, it can not be denied that the presence of a family history of diabetes can make the association between obesity and diabetes stronger [198] . Given a BMI ⩾ 35, an individual with a family history of diabetes is at a higher risk of incidence diabetes (OR 26.7, 95% CI 14.4–49.4) compared to the one without a family history of diabetes (OR 6.1, 95% CI3.4–11.2). Furthermore, ethnicity is also considered an important factor in an obese individual with a family history of diabetes [199] , [200] .
An individual having a family history of diabetes can have an early onset of diabetes compared to the ones without a family history. However, it is hard to conclude that which among the maternal, paternal and both maternal and paternal family history of diabetes is more significant for incidence/prevalence of type 2 diabetes as the results in the literature are inconsistent [195] , [201] , [202] , [203] , [204] , [205] .
3.11. Obesity
Obesity is a complex health condition that involves an excessive amount of body fat. It is defined by the BMI and further evaluated in terms of fat distribution via the waist-hip ratio. Abdominal fat in the body increases inflammation which decreases insulin sensitivity by disrupting the function of beta-cells. The insulin resistance condition then leads to the prevalence of type 2 diabetes. Table 10 shows the characteristics and findings of the work in the literature studying the association between obesity and type 2 diabetes.
Characteristics and findings of the studies examining the association between obesity and type 2 diabetes.
SWHS-Shanghai Women’s Health Study, BWHS- Black Women’s Health Study, RS-Random Sample, WC-Waist Circumference, TLGS-Tehran Lipid and Glucose Study, MAHES-Massachusetts Hispanic Elderly Study, H-Hispanics, NH-Non Hispanics, M-Men, W-Women, PCS-Prospective Cohort Study, CSS-Cross-Sectional Study.
Ishikawa-Takata et al. [206] found that the risk of diabetes increases significantly for an individual having a BMI greater than 29 kg/ m 2 . The relative risk of diabetes increases up to 38.8 (95% CI 31.9–47.2) for an individual having a BMI greater than 34.9 kg/ m 2 [119] . Furthermore, study shows that the association between obesity and incidence diabetes is gender-dependent [207] . For each 2 kg/ m 2 lower BMI, men are at 23% (15–30%) lower risk of diabetes, whereas women are at 27% (23–32%) lower risk. Further, the association between obesity and diabetes is also dependent on ethnicity [207] . For each 2 kg/ m 2 lower BMI, Asians are at 37% (26–46%) lower risk of diabetes, whereas Australians are at 25% (21–29%) lower risk.
Ohnishi et al. [208] found that compared to overall obesity, central obesity is highly associated with the risk of type 2 diabetes (RR 2.07, 95% CI 1.03–4.16). This association is more in elderly people ( ⩾ 60 years) (OR 3.8, 95% CI 1.8–7.7) [209] . The association between central obesity and the incidence of type 2 diabetes is found significant in both men and women. However, centrally obese women are at higher risk (OR 2.875, 95% CI 1.987–4.160) compared to centrally obese men (OR 2.308, 95% CI 1.473–3.615) [210] . The prevalence of type 2 diabetes in obese individual is ethnicity dependent [211] . Non-Hispanics centrally obese women are at higher risk of developing type 2 diabetes (OR 15.1, 95% CI 1.9–117.6) compared to centrally obese Hispanic women (OR 1.6, 95% CI 1.0–2.8). The centrally Hispanic men are also at risk of developing type 2 diabetes (OR 2.1, 95% CI 1.2–3.9). No such association is found in centrally obese Non-Hispanic men. However, all these studies examining the association between central obesity and the incidence of type 2 diabetes consider different definitions of central obesity. For instance, [208] defines central obesity as waist circumference (WC) ⩾ 85 cm in men and ⩾ 90 cm in women, whereas [211] defines it as WC > 102 cm in men and > 88 cm in women. Consequently, it is difficult to conclude the association between central obesity and the incidence of type 2 diabetes.
In summary, although obesity is a significant predictor, the association between obesity and diabetes is a factor of gender and ethnicity. Women with high BMI are at greater risk of diabetes compared to men. Moreover, the association is stronger in Asians compared to Australians. The association between central obesity is also found to be significant for the prevalence of type 2 diabetes. This association is the strongest in Non-Hispanics women. However, more studies are required to examine the association between central obesity and type 2 diabetes following one standard criterion defining central obesity.
3.12. Physical inactivity
An individual is considered physically inactive if he/she does not get the recommended 30–60 min of exercise three to four times a week. Physical inactivity decreases insulin sensitivity with progressive loss of beta-cells. This leads to impaired glucose tolerance and eventually type 2 diabetes. However, no work examines the association between physical inactivity as an independent factor and the prevalence of diabetes. One of the reasons that physical inactivity leads to type 2 diabetes can be that physical inactivity can cause obesity which in turn is a significant risk factor for type 2 diabetes.
4. Conclusion
Diabetes is a global crisis that is primarily driven by rapid urbanization, changing lifestyles, and uneven dietary patterns [215] , [216] . It is crucial to predict the prevalence of diabetes in an individual to reduce the risk of diabetes development and save lives. Diabetes is thought to prevail due to several risk factors such as high-level serum uric acid, sleep quality/quantity, smoking, depression, cardiovascular disease, dyslipidemia, hypertension, aging, ethnicity, family history of diabetes, physical inactivity, and obesity. Studies in the literature have examined the association between each of these risk factors and the risk of developing type 2 diabetes. In this review, we provide an analysis of the studies in the literature to deduce inferences on the relationship between the risk factors and incidence/prevalence of type 2 diabetes.
In conclusion, it can be observed that sleep quantity/quality, smoking, dyslipidemia, hypertension, ethnicity, family history of diabetes, obesity and physical inactivity are strongly associated with the development of type 2 diabetes. Both sleep quantity and quality are found to be strongly associated with the development of type 2 diabetes. The association is stronger in women sleeping for more hours and in men sleeping for fewer hours. However, the sleeping quantity and quality data in these studies are self-reported by the participants, and therefore, prone to errors. More studies are required that use measurement techniques for data collection to validate the association between sleep quantity/quality and type 2 diabetes. Smoking is also found to be a significant risk factor for type 2 diabetes. Both active and passive smokers are at higher risk of developing type 2 diabetes. Moreover, the risk for developing type 2 diabetes remains high in ex-smokers for the first 5–10 years of smoking cessation. Dyslipidemia is associated with the development of type 2 diabetes. Increased non-HDL and decreased HDL levels are strongly associated with type 2 diabetes. However, in the majority of these studies, the incidence or prevalence of type 2 diabetes is self-reported. Consequently, further studies are needed to validate this association between dyslipidemia and type 2 diabetes using standardized measurement techniques, such as A1C test [217] . Hypertension is a significant risk factor for type 2 diabetes and this is further elevated in obese individuals. Ethnicity strongly associates with the development of type 2 diabetes. This could be due to the fact that insulin sensitivity varies among individuals of different ethnicity. Family history of diabetes in first degree of relatives is strongly associated with the development of type 2 diabetes. In addition, family history of diabetes also signifies the association between obesity and type 2 diabetes. Obesity is found to a significant risk factor for incidence of type 2 diabetes and the association is stronger in women compared to men.
The association between serum uric acid and type 2 diabetes remains obscure. It can not be concluded that serum uric acid is an independent risk factor for type 2 diabetes or it only elevates the association between other independent risk factors such as obesity, hypertension, and dyslipidemia, and type 2 diabetes. Moreover, our analysis shows that there might be no association between serum uric acid and the development of type 2 diabetes, but rather there might be a reverse association, i.e., diabetes leads to elevated serum uric acid level. Similarly, based on the evidence in the literature, aging can not be considered as an independent risk factor for type 2 diabetes. Aging only emphasizes the association between obesity and type 2 diabetes. Depression as well is not found to an independent risk factor contributing to the development of type 2 diabetes. Rather, the activities related to depression such as physical inactivity, poor diet, and obesity leads to diabetes. There is no sufficient evidence to conclude the association between cardiovascular disease and type 2 diabetes. It is debatable whether cardiovascular disease leads to the development of type 2 diabetes. Consequently, more studies are required to study the direct association between these risk factors, i.e., serum uric acid, aging, depression, and cardiovascular disease, and incidence of type 2 diabetes.
Based on this study, we devise recommendations to different stakeholders leading to better patient care. In particular, we provide recommendations for allied healthcare professionals, individuals, and government institutions as follows:
- • Allied healthcare professionals: The hypertensive medications and the LDL lowering therapy and drugs should be carefully prescribed as they are associated with increased risk of type 2 diabetes. In addition, overweight and obese adults should be screened for diabetes.
- • Individuals: A healthy lifestyle, which involves intake of polyunsaturated fatty acids and vegetable fats, regular exercise, a healthy diet and proper sleep, is crucial. Individuals should avoid both active and passive smoking.
- • Government: Physical activity in the nation should be promoted for a healthy nation. Law policies should be implemented to restrict public smoking as passive smoking significantly increases the risk of type 2 diabetes. For instance, designated smoking areas can be established to eliminate the risk of developing passive smokers. It would be beneficial to have periodic surveys that include the demographic and lifestyle features of the citizens and the surveys’ results can be then used to develop a nation-wide diabetes prevention plan, in coordination with the allied health professionals.
CRediT authorship contribution statement
Leila Ismail: Conceptualization, Methodology, Investigation, Writing - original draft, Writing - review & editing. Huned Materwala: Investigation, Writing - original draft. Juma Al Kaabi: Validation, Writing - review & editing.
Declaration of Competing Interest
The authors declare that they have no known competing financial interests or personal relationships that could have appeared to influence the work reported in this paper.
Acknowledgments
This work is funded by the National Water and Energy Center of the United Arab Emirates University (Grant No. 31R215). We thank the anonymous reviewers for their valuable comments which helped us improve the paper.
Appendix A.
Search string used to retrieve the studies on the association between risk factor and type 2 diabetes.
Quality assessment of the included studies according to the Quality assessment tool for observational cohort and cross-sectional studies.
Q1. Was the research question or objective in this paper clearly stated?.
Q2. Was the study population clearly specified and defined?.
Q3. Was the participation rate of eligible persons at least 50%?
Q4. Were all the subjects selected or recruited from the same or similar populations (including the same time period)? Were inclusion and exclusion criteria for being in the study prespecified and applied uniformly to all participants?.
Q5. Was a sample size justification, power description, or variance and effect estimates provided?.
Q6. For the analyses in this paper, were the exposure(s) of interest measured prior to the outcome(s) being measured?.
Q7. Was the timeframe sufficient so that one could reasonably expect to see an association between exposure and outcome if it existed?.
Q8. For exposures that can vary in amount or level, did the study examine different levels of the exposure as related to the outcome (e.g., categories of exposure, or exposure measured as continuous variable)?.
Q9. Were the exposure measures (independent variables) clearly defined, valid, reliable, and implemented consistently across all study participants?.
Q10. Was the exposure(s) assessed more than once over time?.
Q11. Were the outcome measures (dependent variables) clearly defined, valid, reliable, and implemented consistently across all study participants?.
Q12. Were the outcome assessors blinded to the exposure status of participants?.
Q13. Was loss to follow-up after baseline 20% or less?.
Q14. Were key potential confounding variables measured and adjusted statistically for their impact on the relationship between exposure(s) and outcome(s)?.
CD-Cannot be Determined; NA-Not Applicable; NR-Not Reported.
- - Google Chrome
Intended for healthcare professionals
- Access provided by Google Indexer
- My email alerts
- BMA member login
- Username * Password * Forgot your log in details? Need to activate BMA Member Log In Log in via OpenAthens Log in via your institution

Search form
- Advanced search
- Search responses
- Search blogs
- Trends in incidence of...
Trends in incidence of total or type 2 diabetes: systematic review
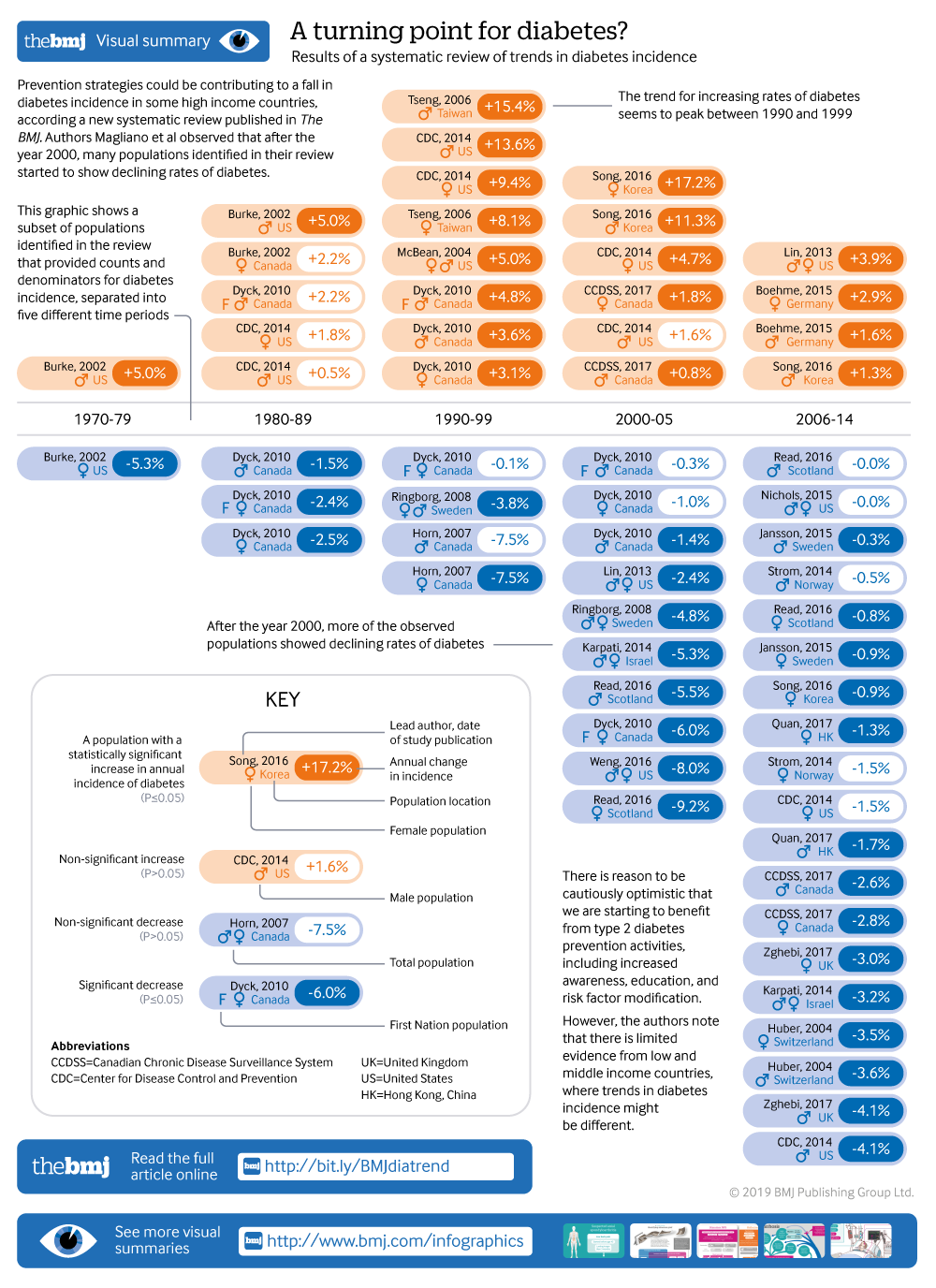
Visual summary available
Showing the turning point in diabetes incidence in 61 populations
Linked editorial
Trends in type 2 diabetes
- Related content
- Peer review
- Dianna J Magliano , laboratory head of diabetes and population health 1 2 ,
- Rakibul M Islam , postdoctoral research fellow 1 2 ,
- Elizabeth L M Barr , postdoctoral research fellow 1 ,
- Edward W Gregg , chair in diabetes and cardiovascular disease epidemiology 3 4 ,
- Meda E Pavkov , physician scientist 3 ,
- Jessica L Harding , research fellow 3 ,
- Maryam Tabesh , research study coordinator 1 2 ,
- Digsu N Koye , postdoctoral research fellow 1 2 ,
- Jonathan E Shaw , deputy director of Baker Heart and Diabetes Institute 1 2
- 1 Baker Heart and Diabetes Institute, Melbourne, VIC 3004, Australia
- 2 School of Public Health and Preventive Medicine, Monash University, Melbourne, VIC 3004, Australia
- 3 Centres for Diseases Control and Prevention, Division of Diabetes Translation, Atlanta, GA, USA
- 4 School of Public Health, Epidemiology and Biostatistics, Imperial College London, London, UK
- Correspondence to: D J Magliano dianna.magliano{at}baker.edu.au
- Accepted 16 July 2019
Objective To assess what proportions of studies reported increasing, stable, or declining trends in the incidence of diagnosed diabetes.
Design Systematic review of studies reporting trends of diabetes incidence in adults from 1980 to 2017 according to PRISMA guidelines.
Data sources Medline, Embase, CINAHL, and reference lists of relevant publications.
Eligibility criteria Studies of open population based cohorts, diabetes registries, and administrative and health insurance databases on secular trends in the incidence of total diabetes or type 2 diabetes in adults were included. Poisson regression was used to model data by age group and year.
Results Among the 22 833 screened abstracts, 47 studies were included, providing data on 121 separate sex specific or ethnicity specific populations; 42 (89%) of the included studies reported on diagnosed diabetes. In 1960-89, 36% (8/22) of the populations studied had increasing trends in incidence of diabetes, 55% (12/22) had stable trends, and 9% (2/22) had decreasing trends. In 1990-2005, diabetes incidence increased in 66% (33/50) of populations, was stable in 32% (16/50), and decreased in 2% (1/50). In 2006-14, increasing trends were reported in only 33% (11/33) of populations, whereas 30% (10/33) and 36% (12/33) had stable or declining incidence, respectively.
Conclusions The incidence of clinically diagnosed diabetes has continued to rise in only a minority of populations studied since 2006, with over a third of populations having a fall in incidence in this time period. Preventive strategies could have contributed to the fall in diabetes incidence in recent years. Data are limited in low and middle income countries, where trends in diabetes incidence could be different.
Systematic review registration Prospero CRD42018092287.
Introduction
Over the past few decades, the prevalence of diabetes in developed and developing countries has risen substantially, making diabetes a key health priority globally. 1 Examination of trends in total burden of diabetes is an essential part of the monitoring of this health priority area, but, to date, it has consisted primarily of studies looking at diabetes prevalence. 1 2 3 4 5 Prevalence estimates suggest that the diabetes burden is still rising in most countries, and this is often interpreted as evidence of increasing risk in the population. However, selective incidence studies 6 7 and some accompanying risk factor data 8 suggest otherwise. Prevalence can be a crude and misleading metric of the trajectory of an epidemic, because increasing prevalence of a disease might be due to either increasing incidence or to improved survival. Furthermore, prevalence cannot be reliably used to study the effects of changes in population risk factors, because their effects are detected earlier with incidence trends than with prevalence trends, and incidence is not affected by changes in survival.
Incidence measures the proportion of people who develop diabetes over a period of time among the population at risk. It is the appropriate measure of population risk, and a valuable way of assessing whether public health campaigns for diabetes prevention are succeeding. While prevalence can rise simply because mortality falls, incidence of diagnosed diabetes is affected only by the risk of the population and the amount of screening undertaken. Changes in prevalence might be an inadequate guide to the effects of prevention activities, and could lead to the inappropriate rejection of effective interventions. It is only by measuring both incidence and prevalence that a better understanding of the extent of diabetes can be achieved.
Among existing diabetes incidence data, a few studies suggest that diabetes incidence could be falling despite rising or stable prevalence, 6 7 9 but not all data are consistently showing the same trends. For example, studies from England and Wales (1994-98), 10 Portugal (1992-2015), 11 and Canada (1995-2007) 12 are reporting increases in diabetes incidence. To understand what is happening at a global level over time, a systematic approach to review all incidence trend data should be undertaken to study patterns and distributions of incidence trends by time, age, and sex. So far, no systematic reviews have reported on trends in the incidence of diabetes. Therefore, we conducted a systematic review of the literature reporting diabetes incidence trends.
Data sources and searches
We conducted a systematic review in accordance with PRISMA guidelines. 13 We searched Medline, Embase, and CINAHL from January 1980 to December 2017 without language restrictions. The full search strategy is available in supplementary table 1.
Study selection
Inclusion and exclusion criteria.
Eligible studies needed to report diabetes incidence in two or more time periods. Study populations derived from open, population based cohort studies (that is, with ongoing recruitment over time), diabetes registries, or administrative or health insurance databases based mainly or wholly in primary care (electronic medical records, health insurance databases, or health maintenance organisations). We also included serial, cross sectional, population based studies where incidence was defined as a person reporting the development of diabetes in the 12 months before the survey. Studies were required to report on the incidence of either total diabetes or type 2 diabetes. We excluded studies reporting incidence restricted to select groups (eg, people with heart failure) and studies reporting only on children or youth.
Each title and abstract was screened by at least two authors (DJM, JES, DNK, JLH, and MT) and discrepancies were resolved by discussion. We aimed to avoid overlap of populations between studies. Therefore, if national data and regional data were available from the same country over the same time period, we only included the national data. If multiple publications used the same data source, over the same time period, we chose the publication that covered the longest time period.
Outcome measure
Our outcome was diabetes incidence using various methods of diabetes ascertainment including: blood glucose, glycated haemoglobin (HbA1c), linkage to drug treatment or reimbursement registries, clinical diagnosis by physicians, administrative data (ICD codes (international classification of diseases)), or self report. Several studies developed algorithms based on several of these elements to define diabetes. We categorised the definition of diabetes into one of five groups: clinical diagnosis, diabetes treatment, algorithm derived, glycaemia defined (blood glucose or HbA1c, with or without treatment), and self report.
Data extraction and quality of studies
We extracted crude and standardised incidence by year (including counts and denominators) and the reported pattern of the trends (increasing, decreasing, or stable, (that is, no statistically significant change)) in each time period as well as study and population characteristics. Age specific data were also extracted if available. Data reported only in graphs were extracted by DigitizeIt software (European Organisation for Nuclear Research, Germany). We assessed study quality using a modified Newcastle-Ottawa scale for assessing the risk of bias of cohort studies 14 (supplementary material).
Statistical methods
Data were reported as incidence density (per person year) or yearly rates (percentage per year). From every study, we extracted data from every subpopulation reported, such that a study reporting incidence in men and women separately contributed two populations to this analysis. If studies reported two different trends over different time periods, we considered these as two populations. Further, if the study was over 10 years in duration, we treated these as two separate time periods. To avoid double counting, when the data were reported in the total population as well as by sex and ethnic groups, we only included data once and prioritised ethnicity specific data over sex specific data.
We extracted the age specific incidence data reported for every individual calendar year. These data were then categorised into four age bands (<40, 40-54, 55-69, and ≥70), and were plotted against calendar year. In studies where counts and denominators were reported by smaller age groups than we used, we recalculated incidence across our specified larger age groups. If we found multiple age groups within any of our broader age groups, but with insufficient information to combine the data into a new category, only data from one age group were used. To limit overcrowding on plots, if data were available for men, women, and the total population, only total population data were plotted. Data from populations with high diabetes incidence such as Mauritians 15 and First Nation populations from Canada 16 were plotted separately to allow the examination of most of the data more easily on a common scale (supplementary material). Furthermore, studies reporting data before 1991 or populations with fewer than three data points were not plotted. We also categorised studies into European and non-European populations on the basis of the predominant ethnicity of the population in which they were conducted. Studies conducted in Israel, Canada, and the United States were assigned to the European category.
We took two approaches to analyse trends of diabetes incidence over time. Firstly, we allocated the reported trend (increasing, decreasing, or stable (that is, no statistically significant change)) of each population to the mid-point of each study’s observational period, and then assigned this trend into one of five time periods (1960-79, 1980-89, 1990-99, 2000-05, and 2006-14). Where a test of significance of trends was not reported or when a time period was longer than 10 years, we performed Joinpoint trend analyses 17 18 to observe any significant trends in the data (assuming a constant standard deviation). Joinpoint Trend Analysis Software (version 4.5.0.1) uses permutation tests to identify points where linear trends change significantly in direction or in magnitude, and calculates an annual percentage change for each time period identified. In sensitivity analyses we also tested different cut points in the last two time periods.
The second approach was used to more accurately allocate trends to the prespecified time periods. Among the studies that reported raw counts of diabetes cases and denominators, we examined the association between calendar year and incidence, using Poisson models with the log person years as offset. The midpoints of age and calendar period were used as continuous covariates, and the effects of these were taken as linear functions. We analysed each study separately by prespecified time periods, and reported annual percentage change when the number of data points in the time period was at least four. For studies that did not provide raw data but did report a sufficient number of points, we analysed the relation between year and incidence using Joinpoint regression across the time periods specified above and reported annual percentage change. Analyses were conducted with Stata software version 14.0 (Stata Corporation, College Station, TX, USA), and Joinpoint (Joinpoint Desktop Software Version 4.5.0.1). 17 18
Patient and public involvement
No patients or members of the public were involved in setting the research question or the outcome measures for this study. No patients were asked to advise on interpretation or writing up of results. We intend to disseminate this research through press releases and at research meetings.
We found 22 833 unique abstracts from 1 January 1980 to the end of 2017. Among these, 80 described trends of diabetes incidence, of which 47 met all inclusion criteria. Articles describing trends were excluded for the following reasons: duplicated data (n=21), closed cohorts (n=5), populations included youth only (n=1), occupational cohorts (n=2), or no usable data presented (n=4; fig 1 ).

Flowchart of study selection
- Download figure
- Open in new tab
- Download powerpoint
Table 1 and supplementary material table 2 describe the characteristics of the included studies. Only 19% (9/47) of studies were from predominantly non-Europid populations and 4% (2/47) of studies were from low or middle income countries (China 25 and Mauritius 15 ). Administrative datasets, health insurance data, registry data, survey data, and cohort studies accounted for 38% (n=18), 21% (n=10), 19% (n=9), 11% (n=5), and 11% (n=5) of the 47 data sources, respectively. Among the 47 studies, diabetes was defined by a clinical diagnosis, diabetes treatment (via linkage to drug treatment registers), an algorithm, blood glucose, and self report in 28% (n=13), 9% (n=4), 47% (n=22), 11% (n=5), and 6% (n=3) of studies, respectively. Sample sizes of the populations were greater than 10 000 in every year in 85% (n=40) of the studies, and greater than 130 000 per year in 70% (n=33) of the studies. A total of 62% (n=29) of the 47 included studies exclusively reported on type 2 diabetes, and 38% (n=18) reported on total diabetes.
Characteristics of 47 included studies reporting on diabetes incidence trends, by country
- View inline
Summary of patterns of diabetes incidence trends based on analyses reported in publications in 1960-99
Trends of diabetes incidence
Among the 47 studies, 16 provided information on incidence by age group. Of these 16 studies, 14 were plotted in figure 2 , with those from high incidence countries plotted in supplementary figure 1. In these figures, incidence in most studies increased progressively until the mid-2000s in all age groups. Thereafter, most studies showed a stable or decreasing trend, apart from studies in Denmark 26 27 and Germany 31 and in a US health insurance population 9 where the incidence inflected upwards in the later years for some age groups.

Incidence of diabetes over time for populations aged under 40, 40-54, 55-69, and 70 or more, among studies reporting age specific data. Only populations with at least three points were plotted. NHIS=National Health Interview Survey
Using the first approach to analyse trends of diabetes incidence over time, we separated the data into populations based on sex and ethnicity, and allocated a time period to each population, generating 105 populations for analysis. Seventy four and 31 populations were predominantly Europid and non-Europid, respectively. Table 2 and table 3 show the reported trend for each population. Table 4 summarises the findings in table 2 and table 3 , and shows that the proportion of populations reporting increasing trends peaked in 1990-99 and fell progressively in the two later time periods. Between 1960 and 1989, 36% (8/22) of the populations studied had increasing trends in incidence of diabetes, 55% (12/22) had stable trends, and 9% (2/22) had decreasing trends. In 1990-2005, diabetes incidence increased in 66% (33/50) of populations, was stable in 32% (16/50), and decreased in 2% (1/50). In 2006-14, increasing trends were reported in 33% (11/33) of populations, whereas 30% (10/33) and 36% (12/33) had stable or declining incidence, respectively.
Summary of patterns of diabetes incidence trends based on analyses reported in publications in 2000-14
Summary of incidence trends over time of total or type 2 diabetes
Populations that reported a decrease in incidence after 2005 came from the US, 6 9 Israel, 34 Switzerland, 46 Hong Kong, 32 Sweden, 43 and Korea. 36 Populations reporting increasing incidence after 2005 included Portugal, 11 Denmark, 26 27 and Germany, 31 while populations from Canada, 19 Italy, 35 Scotland, 40 Norway, 39 US (non-Hispanic white), 56 and the United Kingdom 50 showed stable incidence. For two studies (16 populations), 16 29 we could not determine a direction of a trend (increasing, decreasing, or stable), because they showed three phases of change with the trend of the middle phase differing from the trend of the first and last phase. Across the total time period, we observed a higher proportion of populations reporting stable or decreasing trends in predominantly Europid than in non-Europid populations (52% v 41%).
Using the second approach to analyse trends of diabetes incidence over time, we modelled 21 studies (62 populations) that reported diabetes counts and denominators specifically within each time period ( table 5 ). The percentage of populations with a decreased or stable incidence was highest in 1980-89 (88%; 7/8), but this proportion was based on only eight populations in three studies. From 1990 onwards, the percentage with decreasing or stable incidence increased progressively, reaching 83% (19/23) of populations in 2006-14. Eight studies (21 populations) that were analysed by Joinpoint had no data on counts or denominators (supplementary table 3). When these data were considered with the data in table 5 , the percentage of populations in 2006-14 with decreasing or stable incidence fell to 70% (19/27), but this proportion was still the highest of all the time periods, whereas the percentage for 1990-99 remained the lowest at 31% (5/16).
Annual percentage change in diabetes incidence in men (M), women (W), or total population (T) among studies that provided counts and denominators, by time period
In a sensitivity analysis, we tested whether our selection of time periods was driving our results. When we defined the final time periods to be 2000-07 and 2008-14, our results were not altered, with 66% (21/32) of the populations in the last time period showing decreasing or stable trends. We also repeated the analysis in table 4 and excluded cohort studies and surveys, and found that the results were not materially altered, with 65% (20/31) of populations in the last time period (from 2006 onwards) showing decreasing or stable incidence of diabetes.
Quality of studies
The median score for study quality was 10 (interquartile range 8-11; supplementary table 4). We repeated the analyses reported in table 4 after excluding studies that had quality scores in the lowest quarter, and observed similar results to the main findings. For example, in 1960-89, 67% (10/15) of populations reported stable or decreasing incidence, while in the final time period, 67% (18/27) of populations reported stable or decreasing incidence of diagnosed diabetes.
Principal findings
In this systematic review of population based studies on diabetes incidence, we show evidence that the incidence of diagnosed diabetes increased in most populations from the 1960s to the early 2000s, after which a pattern emerged of levelling trends in 30% and declining trends in 36% of the reported populations. Although the lack of data for non-Europid populations leaves global trends in incidence unclear, these findings suggest that trends in the diabetes epidemic in some high income countries have turned in a more encouraging direction compared with previous decades. It is important to note that these results apply predominantly to type 2 diabetes, as even though many studies did not accurately define diabetes type, the incidence of type 2 diabetes in adults is an order of magnitude greater than that of type 1 diabetes.
The countries that showed stable or decreasing trends in the last time period were from Europe and east Asia, with no obvious clustering or commonalities. For the countries showing decreasing or stable diabetes trends, if the prevalence data were used to understand the diabetes epidemic in that country, a different message would be obtained. For example, national data from Korea showed that the prevalence of diabetes increased from 2000 to 2010. 59 Similarly in Sweden, the prevalence of pharmacologically treated diabetes increased moderately from 2006 to 2014. 43 In the US, the prevalence of diabetes reached a plateau when incidence began to decrease. However, we lacked incidence data from many areas of the world where the most steady and substantial increases in prevalence have been reported, including the Pacific Islands, Middle East, and south Asia. Large increases in incidence could still be occurring in these areas. The lack of incidence data for much of the world, combined with the common observation of discordance between incidence and prevalence rates where such data exist, both underscore the importance of using incidence data to understand the direction of the diabetes epidemic.
Incidence could be starting to fall for several reasons. Firstly, we might be starting to benefit from prevention activities of type 2 diabetes, including increased awareness, education, and risk factor modification. These activities have involved both targeted prevention among high risk individuals, similar to that conducted in the Diabetes Prevention study 60 and Diabetes Prevention Programme 61 62 in many countries, 63 and less intensive interventions with broader reach such as telephone counselling in the general community. 64 65 67 Secondly, health awareness and education programmes have also been implemented in schools and work places, and many changes to the physical environment, such as the introduction of bike tracks and exercise parks, have occurred. 68 Thirdly, favourable trends in selected risk factors of type 2 diabetes in some countries provide indirect evidence of positive changes to reduce diabetes incidence. Finally, in the US, there is some evidence in recent years of improved diets and related behaviours, which include reductions in intake of sugar sweetened beverages 69 and fat, 70 small declines in overall energy intake, and declines in some food purchases. 8 71
Similar reduction in consumptions of sugar sweetened beverages have occurred in Norway 72 and Australia 73 and fast food intake has decreased in Korea. 74 Some of these changes could be linked to a fall in diabetes incidence. Some places such as Scotland 75 have also had a plateauing of obesity prevalence, but this is not universal. In the US, despite earlier studies suggesting that the rate of increase in obesity might be slowing down, 76 77 more recent data show a small increase. 78 79 While some evidence supports the hypothesis that these prevention activities for type 2 diabetes and an improved environment could trigger sufficient behaviour change to have an effect on diabetes incidence, other data, such as the continuing rising obesity prevalence in the US, 79 casts some doubt over the explanations underpinning our findings on diabetes incidence trends.
Other factors might have also influenced reported diabetes incidence. Only 11% (n=5) of the studies reported here screened for undiagnosed diabetes, and therefore trends could have been influenced by secular changes in diagnostic behaviour. In 1997, the threshold for fasting plasma glucose for diagnosis of diabetes was reduced from 7.8 to 7.0 mmol/L, which could increase diagnosis of new cases of type 2 diabetes. In 2009-10, HbA1c was then introduced as an alternative way to diagnose diabetes. 80 Evidence from some studies suggests that the HbA1c diagnostic threshold detects fewer people with diabetes than do the thresholds for fasting plasma blood glucose, 80 81 potentially leading to a lowering of incidence estimates. However, across multiple studies, prevalence estimates based on fasting plasma glucose only versus HbA1c definitions are similar. 82 Furthermore, because HbA1c can be measured in the non-fasting state (unlike the fasting blood glucose or oral glucose tolerance test), the number of people who actually undergo diagnostic testing could be higher with HbA1c. Nichols and colleagues 56 reported that among seven million insured US adults, despite a shift towards HbA1c as the diagnostic test in 2010, the incidence of diabetes did not change from 2010 to 2011.
Another potential explanation for declining or stable diabetes incidence after the mid-2000s is a reduction in the pool of undiagnosed diabetes 83 through the intensification of diagnostic and screening activities 83 84 and changing diagnostic criteria during the previous decade. 80 Data from Read and colleagues provide some evidence to support this notion. 41
Among the included studies, two studies specifically examined clinical screening patterns in parallel with incidence trends. These studies reported that the proportion of the population screened for diabetes increased over time, and the incidence of diabetes remained stable 56 or fell. 34 While the Karpati study 34 combined data for glucose testing with HbA1c testing, the study by Nichols and colleagues 56 separated the two, and showed that both glucose testing and HbA1c testing increased over time. A third study, in Korea, 36 also noted that the incidence of diabetes decreased in the setting of an increase in the uptake of the national health screening programme. Despite the introduction of HbA1c for diagnosis of diabetes by the World Health Organization, this practice has not been adopted everywhere. For example, neither Scotland nor Hong Kong have introduced the use of HbA1c for screening or diagnosis of diabetes, and studies in these areas showed a levelling of diabetes incidence trends and decreasing trends, respectively.
Our findings appear to contrast with data showing increasing global prevalence of diabetes. 1 3 However, increasing prevalence could be influenced by improved survival of people with diabetes, because this increases the length of time that each individual remains within the diabetes population. As is shown in several studies in this review, 23 41 mortality from diabetes and incidence of diabetes might both be falling but as long as mortality is lower than incidence, prevalence will rise. Therefore, we argue that prevalence alone is an insufficient measure to track the epidemic of diabetes and other non-communicable diseases.
Strengths and weaknesses of this study
A key strength of this work was the systematic approach and robust methodology to describe trends in diagnosed diabetes incidence. We also presented the reported trends allocated to approximate time periods, as well as conducting our own regression within exact time periods. The following limitations should also be considered. Firstly, we did not formally search the grey literature, because a preliminary grey literature search revealed only low quality studies, with inadequate methodological detail to provide confidence in any observed incidence trends, and thus review could be subject to publication bias. Secondly, we were not able to source age or sex specific data on all populations. Thirdly, it was not possible to adjust for different methods of diabetes diagnosis or ascertain trends by different definitions of diabetes. Fourthly, most data sources reported only on clinically diagnosed diabetes and so were subject to influence from diagnostic behaviour and coding practices. Fifthly, study type changed over time, with large administrative datasets becoming more common and cohort studies becoming less common over time. Nevertheless, the size and absence of volunteer bias in administrative datasets likely make them less biased. Finally, data were limited in low and middle income countries.
Conclusions and unanswered questions
This systematic review shows that in most countries for which data are available, the incidence of diagnosed diabetes was rising from the 1990s to the mid-2000s, but has been stable or falling since. Preventive strategies and public health education and awareness campaigns could have contributed to this recent trend. Data are limited in low and middle income countries where trends in diabetes incidence might be different. Improvement of the collection, availability, and analysis of incidence data will be important to effectively monitor the epidemic and guide prevention efforts into the future.
What is already known on this topic
Monitoring of the diabetes epidemic has mainly focused on reporting diabetes prevalence, which continues to rise; however, increasing prevalence is partly driven by improved medical treatment and declining mortality
Studies on diabetes incidence are scarce, but among those that exist, some report a fall or stabilisation of diabetes incidence;
Whether the proportion of studies reporting falling incidence has changed over time is not known
What this study adds
This systematic review of published data reporting diabetes incidence trends over time shows that in most countries with available data, incidence of diabetes (mainly diagnosed diabetes) increased from the 1990s to the mid-2000s, and has been stable or falling since
Preventive strategies and public health education and awareness campaigns could have contributed to this flattening of rates, suggesting that worldwide efforts to curb the diabetes epidemic over the past decade might have been effective
Published data were very limited in low and middle income countries, where trends in diabetes incidence might be different
Acknowledgments
The findings and conclusions in this report are those of the authors and do not necessarily represent the official position of the US Centers for Disease Control and Prevention (CDC).
Contributors: MT, DNK, JLH, and RMI are postdoctoral fellows who screened abstracts for selection into the systematic review. JES and DJM also screened abstracts. ELMB applied the quality criteria to the selected articles. RMI extracted data, applied quality criteria to selected articles, and contributed to preparing the manuscript. DJM conceived the project, screened abstracts, extracted the data, analysed the data, and wrote the manuscript. JES, MEP, and EWG conceived the project, edited the manuscript, and provided intellectual input throughout the process. The funder of the study (CDC) was part of the study group and contributed to data collection, data analysis, data interpretation, and writing of the report. The corresponding author had full access to all the data in the study and had final responsibility for the decision to submit for publication. DJM is guarantor. The corresponding author attests that all listed authors meet authorship criteria and that no others meeting the criteria have been omitted.
Funding: Funded by the CDC. The researchers were independent from the funders.
Competing interests: All authors have completed the ICMJE uniform disclosure form at www.icmje.org/coi_disclosure.pdf and declare: support from the CDC for the submitted work; no financial relationships with any organisations that might have an interest in the submitted work in the previous three years; no other relationships or activities that could appear to have influenced the submitted work.
Ethical approval: Not required because this work was a systematic review.
Data sharing: Data are available from the corresponding author ([email protected]).
The lead author affirms that the manuscript is an honest, accurate, and transparent account of the study being reported; that no important aspects of the study have been omitted; and that any discrepancies from the study as planned (and, if relevant, registered) have been explained.
This is an Open Access article distributed in accordance with the Creative Commons Attribution Non Commercial (CC BY-NC 4.0) license, which permits others to distribute, remix, adapt, build upon this work non-commercially, and license their derivative works on different terms, provided the original work is properly cited and the use is non-commercial. See: http://creativecommons.org/licenses/by-nc/4.0/ .
- Finucane MM ,
- Global Burden of Metabolic Risk Factors of Chronic Diseases Collaborating Group (Blood Glucose)
- Whiting DR ,
- Guariguata L ,
- International Diabetes Federation
- NCD Risk Factor Collaboration (NCD-RisC)
- Karuranga S ,
- Abraham TM ,
- Pencina KM ,
- Pencina MJ ,
- Slining MM ,
- Kimball ES ,
- Newnham A ,
- de Sousa-Uva M ,
- Antunes L ,
- Johnson JA ,
- Hemmelgarn BR ,
- Liberati A ,
- Tetzlaff J ,
- Altman DG ,
- PRISMA Group
- ↵ Wells G, Shea B, O’connell D, et al. The Newcastle-Ottawa Scale (NOS) for assessing the quality of nonrandomised studies in meta-analyses. Ottawa Hospital Research Institute, 2014. www.ohri.ca/programs/clinical_epidemiology/oxford.asp Last accessed 14 December 2018.
- Söderberg S ,
- Tuomilehto J ,
- ↵ Joinpoint Regression Program. 4.6.0.0 version. Statistical Methodology and Applications Branch, Surveillance Research Program: National Cancer Institute, 2018. https://surveillance.cancer.gov/joinpoint/ .
- Midthune DN
- ↵ Canadian Chronic Disease Surveillance System. Canadian Chronic Disease Surveillance System 2017. https://www.canada.ca/en/public-health.html
- Blanchard JF ,
- Lipscombe LL ,
- Jacobs-Whyte H ,
- Paradis G ,
- Macaulay AC
- Carstensen B ,
- Kristensen JK ,
- Ottosen P ,
- Borch-Johnsen K ,
- Steering Group of the National Diabetes Register
- Jensen PB ,
- Abouzeid M ,
- Wikström K ,
- Peltonen M ,
- Reunanen A ,
- Klaukka T ,
- Maatela J ,
- Michaelis D ,
- Boehme MW ,
- Buechele G ,
- Frankenhauser-Mannuss J ,
- Vilbergsson S ,
- Sigurdsson G ,
- Sigvaldason H ,
- Hreidarsson AB ,
- Sigfusson N
- Karpati T ,
- Cohen-Stavi CJ ,
- Leibowitz M ,
- Feldman BS ,
- Baviera M ,
- Marzona I ,
- Zimmet PZ ,
- Ruwaard D ,
- Bartelds AI ,
- Hirasing RA ,
- Verkleij H ,
- Birkeland KI ,
- Barnett KN ,
- Ogston SA ,
- Kerssens JJ ,
- McAllister DA ,
- Scottish Diabetes Research Network Epidemiology Group
- Stenström G ,
- Sundkvist G
- Jansson SP ,
- Andersson DK ,
- Svärdsudd K
- Ringborg A ,
- Lindgren P ,
- Martinell M ,
- Stålhammar J
- Schwenkglenks M ,
- Holden SH ,
- Barnett AH ,
- Peters JR ,
- Zghebi SS ,
- Steinke DT ,
- Rutter MK ,
- Emsley RA ,
- Ashcroft DM
- Akushevich I ,
- Kravchenko J ,
- Ukraintseva S ,
- O’Brien P ,
- Centers for Disease Control and Prevention (CDC)
- McBean AM ,
- Gilbertson DT ,
- Narayanan ML ,
- Schraer CD ,
- Bulkow LR ,
- Nichols GA ,
- Schroeder EB ,
- Karter AJ ,
- SUPREME-DM Study Group
- Tabaei BP ,
- Chamany S ,
- Driver CR ,
- Pavkov ME ,
- Hanson RL ,
- Knowler WC ,
- Bennett PH ,
- Krakoff J ,
- Lindström J ,
- Eriksson JG ,
- Finnish Diabetes Prevention Study Group
- Barrett-Connor E ,
- Fowler SE ,
- Diabetes Prevention Program Research Group
- Saaristo T ,
- Moilanen L ,
- Korpi-Hyövälti E ,
- Troughton J ,
- Chatterjee S ,
- Schmittdiel JA ,
- Neugebauer R ,
- Solomon LS ,
- Giles-Corti B ,
- Vernez-Moudon A ,
- Bolt-Evensen K ,
- Brand-Miller JC ,
- ↵ Bromley C, Dowling S, L G. The Scottish Health Survey. Scotland: A National Statistics Publication for Scotland, 2013.
- Carroll MD ,
- Flegal KM ,
- Kruszon-Moran D ,
- Freedman DS ,
- American Diabetes Association
- Lorenzo C ,
- Rasmussen SS ,
- Johansen NB ,
Advertisement
Decision models in type 2 diabetes mellitus: A systematic review
- Original Article
- Open access
- Published: 03 June 2021
- Volume 58 , pages 1451–1469, ( 2021 )
Cite this article
You have full access to this open access article
- Jiayu Li 1 , 2 , 3 na1 ,
- Yun Bao 2 na1 ,
- Xuedi Chen 1 , 2 &
- Limin Tian ORCID: orcid.org/0000-0002-4769-4375 1 , 2
3780 Accesses
11 Citations
Explore all metrics
To reduce the burden of type 2 diabetes (T2DM), the disease decision model plays a vital role in supporting decision-making. Currently, there is no comprehensive summary and assessment of the existing decision models for T2DM. The objective of this review is to provide an overview of the characteristics and capabilities of published decision models for T2DM. We also discuss which models are suitable for different study demands.
Materials and methods
Four databases (PubMed, Web of Science, Embase, and the Cochrane Library) were electronically searched for papers published from inception to August 2020. Search terms were: “Diabetes-Mellitus, Type 2”, “cost-utility”, “quality-of-life”, and “decision model”. Reference lists of the included studies were manually searched. Two reviewers independently screened the titles and abstracts following the inclusion and exclusion criteria. If there was insufficient information to include or exclude a study, then a full-text version was sought. The extracted information included basic information, study details, population characteristics, basic modeling methodologies, model structure, and data inputs for the included applications, model outcomes, model validation, and uncertainty.
Fourteen unique decision models for T2DM were identified. Markov chains and risk equations were utilized by four and three models, respectively. Three models utilized both. Except for the Archimedes model, all other models ( n = 13) implemented an annual cycle length. The time horizon of most models was flexible. Fourteen models had differences in the division of health states. Ten models emphasized macrovascular and microvascular complications. Six models included adverse events. Majority of the models ( n = 11) were patient-level simulation models. Eleven models simulated annual changes in risk factors (body mass index, glycemia, HbA1c, blood pressure (systolic and/or diastolic), and lipids (total cholesterol and/or high-density lipoprotein)). All models reported the main data sources used to develop health states of complications. Most models ( n = 11) could deal with the uncertainty of models, which were described in varying levels of detail in the primary studies. Eleven studies reported that one or more validation checks were performed.
Conclusions
The existing decision models for T2DM are heterogeneous in terms of the level of detail in the classification of health states. Thus, more attention should be focused on balancing the desired level of complexity against the required level of transparency in the development of T2DM decision models.
Similar content being viewed by others
A systematic review of cost-effectiveness models in type 1 diabetes mellitus.
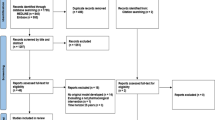
A Systematic Review of Methodologies Used in Models of the Treatment of Diabetes Mellitus
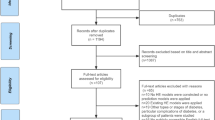
Prediction of complications in health economic models of type 2 diabetes: a review of methods used
Avoid common mistakes on your manuscript.
Introduction
Diabetes is a major health issue that has reached alarming levels. Today, nearly half a billion people are living with diabetes worldwide. In 2017, it was estimated that 425 million people had diabetes (types 1 and 2 combined), increasing to 463 million in 2019, and this number is projected to reach 578 million by 2030 [ 1 ]. Due to population growth and aging, the Global Burden of Disease Study showed that all-age disability-adjusted life-years (DALYs) of people with diabetes in 2016 were 57,233.7, which increased by 24.4% from 1990 to 2016 [ 2 ]. To decrease the high disease burden [ 3 , 4 , 5 ], efficient prevention and treatment of diabetes and its complications are major tasks for health policy. In these situations, disease decision models play a vital role in supporting decision-making for evaluating the long-term health and economic outcomes of interventions in the public and private health sectors [ 6 ].
Disease decision models are logical mathematical frameworks that synthesize the available data (e.g., short-run clinical trial outcomes, risk equations, and progression rates) and known physiologic relationships into a coherent internally consistent framework that can be extrapolated over time [ 7 , 8 ]. Many models have been developed and validated for type 2 diabetes mellitus (T2DM) populations and used in a variety of ways, such as estimating long-term clinical outcomes and costs of a clinical trial and aiding decision makers in choosing between available interventions in these populations [ 9 , 10 , 11 , 12 ]. For instance, the Centers for Disease Control (CDC) Diabetes Cost-effectiveness Group used the Diabetes Cost-Effectiveness Model (DCEM) to estimate the incremental cost-effectiveness of intensive glycemic control (relative to conventional control), intensified hypertension control, and reduction in serum cholesterol levels in patients with T2DM [ 12 ]. From a modeling standpoint, T2DM ranks among the most challenging disease areas because of its impact on multiple interrelated organ systems and multiple treatment goals (including blood glucose, blood pressure, and blood lipids) [ 13 ]. However, unlike models in type 1 diabetes mellitus (T1DM) and prediabetes [ 14 , 15 ], there are few comprehensive summaries and assessments of the existing decision models for T2DM.
Our research provides an overview of the characteristics and capabilities of published decision models in T2DM. We also discuss which models are more suitable for different study demands.
Search strategy and selection criteria
This systematic review was conducted and reported in accordance with the Preferred Reporting Items for Systematic Reviews and Meta-Analyses (PRISMA) guidelines [ 16 ].
Four databases (PubMed, Web of Science, Embase, and the Cochrane Library) were electronically searched for papers that were published from inception to August 2020. The following search terms/MeSH terms were used: “Diabetes Mellitus”, “Type 2”, “cost-utility”, “quality of life”, and “decision model”. The integral search strategy is provided in Appendix 1. We also manually searched the reference lists of the included studies. References were managed using ENDNOTE X9 (Clarivate, Philadelphia, PA). Studies were eligible for inclusion if they met the following predefined criteria:
Population: Patients with T2DM; modeling studies conducted in a mixed population (T1DM and T2DM) were included only if the model adaptation for T2DM patients was reported separately in the full-text publication;
Intervention and comparators: No restrictions;
Outcomes: Studies with decision models in T2DM that reported health economics outcomes such as costs, (quality-adjusted) life expectancy, and diabetes-related complications;
Study design: All modeling studies capable of performing a full economic evaluation were included.
The exclusion criteria were as follows:
Population: T1DM only, or gestational diabetes or maturity-onset diabetes of the young (MODY);
Outcomes: Modeling studies with a limited focus on particular sub-components of T2DM (e.g., only one complication of T2DM), or modeling application studies with a time horizon of ≤ 5 years;
Study design: Abstracts or full-text unavailable.
Two reviewers (L.J. and C.X) independently screened the titles and abstracts according to the inclusion criteria. If there was insufficient information to include or exclude a study, then a full-text version was sought. A consensus between both reviewers was required. Full-text versions of all the relevant studies were also obtained and read by two independent reviewers (L.J. and B.Y.) to ensure that the inclusion criteria were met. Any disagreement between the two reviewers was resolved by a third reviewer for assessment. If there was insufficient information to include a study, then the authors were contacted when possible.
Quality assessment
Two reviewers (L.J. and B.Y.) independently assessed the quality of all the included studies by using the Philips et al. [ 17 ] checklist, which assesses the quality of reporting of the decision models and model-based economic evaluations, as recommended in the Cochrane Handbook for Systematic Reviews of Interventions [ 18 ]. Any disagreement between the two reviewers was resolved by a third reviewer for the assessment. The checklist by Philips et al. evaluates three domains of a model: (1) structure, (2) data, and (3) consistency.
Data extraction and analysis
If a decision model was found to be associated with multiple studies, these studies were assessed as sharing the same parent model: Only the primary study (the study that described the model in greater detail) for each model was considered for the review, while supplementary and subsequent studies were documented as secondary studies. Data from secondary studies were not extracted. Data from the identified studies included in the review were extracted into data extraction grids (supplementary material Appendix 2) by two independent reviewers (L.J. and B.Y.). The extracted information included basic information, study details, population characteristics, basic modeling methodologies, model structure, data inputs for the included applications, model outcomes, model validation, and uncertainty.
A total of 25,995 related studies were searched in this systematic review; 10,102 duplicates were removed, and 15,893 studies were excluded based on first-pass screening using the title and abstract. Following the full-text review, 140 identified studies involving 14 decision models in T2DM were identified. Figure 1 shows the flow of studies throughout the review. Among the 140 identified studies, 79 used the CORE Diabetes Model (CDM), 17 used the Cardiff model, 13 used the United Kingdom Prospective Diabetes Study Outcomes Model 1 (UKPDS-OM1), 5 used the Archimedes model, 4 used the UKPDS-OM2, 4 used the Swedish Institute of Health Economics Cohort Model of Type 2 Diabetes (IHE), 3 used the Economic and Health Outcomes Model for T2DM (ECHO), 3 used the Michigan model, 3 used the Diabetes Cost-Effectiveness Model (DCEM), 2 used the Chinese Outcomes Model for T2DM (COMT), 2 used the Non-Insulin-Dependent Diabetes Mellitus model (NIDDM), 2 used the Sheffield model, 2 used the Ontario Diabetes Economic Model (ODEM), and 1 used the Cornerstone Diabetes Simulation model (CDS). For each model, only the primary studies that described the model in greater detail were considered for review, and supplementary and subsequent studies were documented as secondary studies. The list of secondary studies is summarized in supplementary material Appendix 3. Models were set in the USA ( n = 3) [ 9 , 19 , 20 ], UK ( n = 3) [ 10 , 21 , 22 ], Sweden ( n = 2) [ 23 , 24 ], Canada ( n = 2) [ 11 , 25 ], China ( n = 1) [ 26 ], Switzerland (n = 1) [ 27 ], Australia ( n = 1) [ 28 ], and in multiple countries ( n = 1) [ 12 ]. Four models [ 9 , 12 , 20 , 27 ] solely utilized Markov chains, seven models [ 11 , 19 , 21 , 22 , 25 , 26 , 28 ] solely utilized risk equations, and three models [ 10 , 23 , 24 ] utilized both of them. Except for the Archimedes model, all other models ( n = 13) implemented an annual cycle length. The time horizon of most models is flexible, up to the course of a lifetime. Almost all models involved cost-utility or cost-effectiveness analysis. An overview of each model is outlined in Tables 1 and 2 sorted by year of publication.
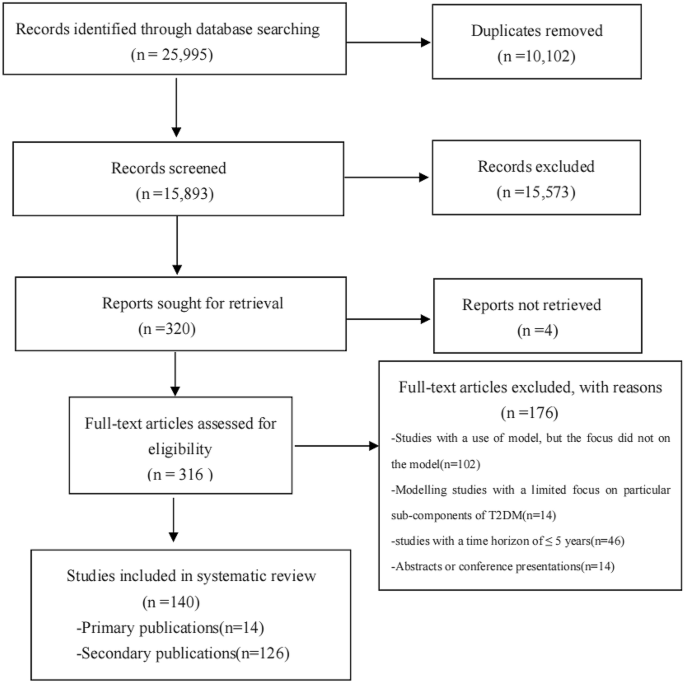
Flow diagram of literature search
Model structure
Tables 1 and 2 show aspects of model structures. Eight model structures [ 10 , 11 , 12 , 22 , 23 , 25 , 26 , 28 ] were constructed in reference to pre-existing models. Models had certain differences in how health states were divided (Tables 3 and 4 ). The DCEM model placed greater emphasis on macrovascular complications, whereas the NIDDM and Michigan models placed greater emphasis on microvascular complications. Other models, apart from the Archimedes model, emphasized both macrovascular and microvascular complications (CDM, UKPDS OM1/2, IHE, ODEM, Cardiff, Sheffield, CDS, COMT, ECHO). The Archimedes model has no clear-cut health states, as it is continuous in time, with no discrete time steps, and any event could occur at any time. The IHE model included numerous health states for complications and used two parallel Markov chains. The first chain consisted of 120 different microvascular health states, and the second chain was made up of 100 different macrovascular health states. Six models [ 19 , 22 , 23 , 24 , 26 , 27 ] included adverse events. Almost all these models classified them as treatment outcomes, not as independent health states. However, the CDM model incorporated adverse events into the model as independent health states. All models included death as a health state, while each model had different levels of detail in this state.
Eleven identified models were patient-level simulation models, while cohorts were used in the DCEM and IHE models. Either the patient -or cohort-level simulation method can be used in the CDM model. Except for the Archimedes model and the ECHO model, others illustrated the model perspective in the primary citations. Ten models considered a healthcare-related perspective in the base case (7 models [ 9 , 10 , 11 , 12 , 21 , 26 , 28 ] used a healthcare-system perspective, 2 models [ 23 , 25 ] used a healthcare decision-maker perspective, and 1 model [ 27 ] used a healthcare-payer perspective), while the NIDDM and Sheffield models considered a patient perspective and a social perspective, respectively.
Thirteen models used an annual cycle length, while the Archimedes model was continuous in time. Three models [ 21 , 26 , 27 ] did not use an annual cycle length for specific health states. The time horizon of 9 models [ 9 , 10 , 11 , 19 , 20 , 23 , 24 , 25 , 27 ] was defined by users, up to one’s lifetime, while the time horizon of 5 models [ 12 , 21 , 22 , 26 , 28 ] was set to one’s lifetime. The transition probabilities between models varied in complexity. Risk equations were applied in most models to handle transition probabilities depending on the epidemiology of T2DM, the risk factors, the incidence and prevalence of diabetic complications, and comorbidities.
Incorporation of risk factors
Eleven models [ 10 , 11 , 20 , 21 , 22 , 23 , 24 , 25 , 26 , 27 , 28 ] simulated annual changes in risk factors such as body mass index (BMI), glycemia, HbA1c, blood pressure (systolic and/or diastolic), and lipids (total cholesterol and/or high-density lipoprotein) (Table 2 ). The simulated trajectory of risk factors could affect the subsequent occurrence or development of diabetes and its complications. The DCEM and COMT models precisely controlled risk factors to reduce the onset and development of diabetes and its complications.
Model outcomes
The major model outcomes are summarized as follows (Table 5 ):
Twelve models [ 11 , 12 , 19 , 20 , 21 , 22 , 23 , 24 , 25 , 26 , 27 , 28 ] reported life-years (LYs), ten model [ 11 , 12 , 19 , 20 , 22 , 23 , 24 , 25 , 26 , 27 ] reported incremental cost-effectiveness ratios (ICERs), and thirteen models [ 10 , 11 , 12 , 19 , 20 , 21 , 22 , 23 , 24 , 25 , 26 , 27 , 28 ] reported quality-adjusted life years (QALYs). The ECHO and IHE models also reported net monetary benefits (NMBs). Some models [ 9 , 10 , 12 , 19 , 22 , 24 , 26 , 27 ] also reported other outcomes.
All models reported costs, albeit at different levels of detail. Eleven models [ 9 , 11 , 12 , 19 , 20 , 22 , 23 , 24 , 25 , 26 , 27 ] reported direct costs, whereas the CDM and IHE models reported both direct and indirect costs. Three models (UKPDS OM1/2 and the Michigan model) did not describe cost in detail. The outcomes of three models (UKPDS OM1/2 model and the Michigan model) included costs, but none of the included studies classified costs into direct and indirect costs.
Health utility
All models reported utility values as outcomes. Thus, subsequent cost-utility analyses (CUA) could be performed. Each health state in a model had a corresponding utility value. Utility values for complications were obtained with the EQ-5D health status questionnaire [ 10 , 21 , 28 ] and the Quality of Well Being–Self-Administered questionnaire (QWB-SA) [ 9 ]. Most CUA were made by calculating QALYs. Some models [ 11 , 12 , 19 , 20 , 22 , 23 , 24 , 25 , 26 , 27 ] also took ICERs into account and thus could perform incremental analyses.
Main data sources for complications
All models reported some main data sources used to develop the health states of complications. The data commonly used to develop macrovascular complications included the Framingham datasets [ 20 , 27 ] and the UKPDS [ 9 , 10 , 12 , 19 , 21 , 22 , 23 , 27 , 28 ]. For microvascular complications, the data sources were more complicated, and the commonly used sources were the Wisconsin Epidemiological Study of Diabetic Retinopathy (WESDR) [ 20 , 27 ] and the UKPDS [ 27 ]. More than half of the models applied multiple data sources for each complication, while the remaining models only contained one or two data resources (Table 6 ).
Model validation
Eleven of fourteen primary studies reported that one or more validation checks had been performed. Four studies [ 10 , 24 , 26 , 28 ] presented model face validation, eleven studies [ 9 , 10 , 19 , 20 , 21 , 23 , 24 , 25 , 26 , 27 , 28 ] presented internal validation, ten studies [ 10 , 19 , 20 , 21 , 23 , 24 , 25 , 26 , 27 , 28 ] presented external validation, while cross-validation was conducted by three studies [ 24 , 25 , 28 ]. However, none of the 14 studies demonstrated predictive validation. Primary studies using the DCEM, ODEM, and Sheffield models did not report aspects of model validation (Table 7 ).
Model uncertainty
Eleven models [ 9 , 10 , 11 , 12 , 20 , 21 , 22 , 23 , 25 , 27 , 28 ] were able to deal with model uncertainty, which was described in varying levels of detail in the primary studies. One-way sensitivity analysis was run in the Cardiff, DCEM, ODEM, and UKPDS-OM2 models. Based on 14 primary studies, none of the models reported a multi-way sensitivity analysis. Probabilistic sensitivity analysis (PSA) capabilities were reported by 9 models (NIDDM, DCEM, CDM, UKPDS-OM1/2, Michigan, Sheffield, IHE, COMT). Five models [ 9 , 20 , 25 , 27 , 28 ] used the Monte Carlo technique for PSA, while three models [ 12 , 21 , 27 ] used the nonparametric bootstrap method. Only 3 model [ 23 , 27 , 28 ] clearly indicated whether first-order or second-order uncertainty was performed (Table 8 ).
Model quality
In accordance with the checklist from Philips et al. [ 17 ], the percentage of fulfilled criteria was unequally distributed across studies and dimensions of quality (model structure, data, and consistency). Overall, 45% of the criteria were met, 26% were not met, and 29% were not applicable in the 14 primary studies. Figure 2 shows that on average across all included studies, model structure ranked the highest, with 65% of criteria for quality being met, followed by model consistency (43%) and model data (32%) (Tables 9 , 10 , and 11 ).
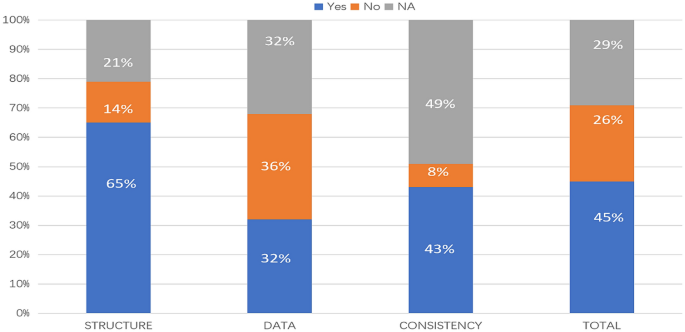
Quality of modeling studies according to the Phillips checklist. Legend: A “yes” answer was assigned if a criterion was fulfilled. A “No” answer was assigned to criteria that were not fulfilled. NA indicates not applicable
Our systematic review included 140 studies describing 14 decision models in T2DM. We extracted data from the primary studies for each model, and the remaining 126 studies were identified as secondary studies (Supplementary material Appendix 2). We found that there were fairly mature modeling technologies and relatively fixed model structures for existing decision models for T2DM. Overall, the 13 identified models (except for the Archimedes model) divided the disease into discrete health states, followed by establishing Markov chains or risk equations to simulate the lifelong course of the disease. However, the review of these studies showed that the existing T2DM models still had certain limitations in terms of quality and extrapolation.
Previous systematic reviews of T2DM models [ 29 , 30 , 31 , 32 ] have focused more on model outputs than on their capabilities. However, the primary focus of this systematic review was the capabilities of these models. Based on the characteristics of each model, we briefly summarized the more suitable models for different study demands as follows:
If a study focused on simulating the trajectory of T2DM and/or diabetic macrovascular complications (e.g., cardiovascular disease, angina, myocardial infarction, or cardiac arrest), the best choice is the DCEM model.
If the study focused on simulating the trajectory of T2DM and/or diabetic microvascular complications (e.g., retinopathy and/or nephropathy), the best choices are the NIDDM model or the Michigan model. It is worth noting that the NIDDM model was the first diabetes model and it is rarely used now, but it is still of great value in the development of diabetes models. Many current models were constructed based on the NIDDM model.
If the objective is to conduct a comprehensive study of the trajectory of T2DM and its various complications, the best choices are the CDM model, the UKPDS OM1/2 model, the IHE model, the ODEM model, the Cardiff model, the Sheffield model, CDS model, COMT model, or the ECHO model.
If the objective is to simulate a continuous trajectory of diabetes and its complications, the Archimedes model is the best choice.
If the study is aimed at Chinese and Asian populations, it is recommended to use the COMT model.
If the study focuses on risk factors, the UKDPS-OM1 or UKDPS-OM2 models can be considered for simulation.
To evaluate T2DM interventions where hundreds of simulations are routinely required (e.g., given multiple indications and treatment comparators and the need for extensive sensitivity analysis), the IHE model can be considered first, because the run times for the IHE model were short when compared to most T2DM microsimulation models.
In this systematic review, the 14 identified models were rather heterogeneous in terms of model structures, the main data sources used by models, and model uncertainty.
We observed that most model structures were composed of discrete health states, and each discrete state was simulated annually through transition probabilities. However, the Archimedes model applied a comprehensive approach to model structure by simulating the disease at the organ level; it has no clear-cut health states. The level of detail in the classification of health states was different between models, and not all models had a clear definition of each health state it contained. However, the desired level of complexity must be balanced with the required transparency. Despite variations in model structure and scope, there should be a reasonably clear consensus of what broad categories of health states should be considered in the same type of T2DM models.
Many of the data sources used in model development are older data sets, such as the UKPDS and Framingham datasets; this limitation also exists in T1DM models. Although this limitation is well known, these data sources are currently recognized as the best available sources for modeling. This review also found that most of the data inputted to models were based on European populations; only 1 of the 14 models was developed based on Asian population data (the COMT model). However, in the era of real-world evidence, with an increasing availability of registry data from clinical practice settings, model validation incorporating modern T2DM epidemiological data into disease progression equations for simulation will be important. The development of this technology may resolve the impacts of limitations on model simulation.
The level of description of model uncertainty varied among the included studies, and there is a lack of standardized terminology regarding model uncertainty in these studies. This may hinder the understanding of what has actually been carried out. For example, in studies conducting Monte Carlo simulation or PSA, it was not always clear whether the report considered first- or second-order uncertainty. This should be noted because many health technology assessment (HTA) agencies demand that second-order uncertainty be captured in PSA. However, it does require multiple and complex computer calculations to solve second-order uncertainty through the PSA of the microsimulation models. This may be why some studies have not clearly stated their uncertainty.
Although a rigorous systematic review was undertaken to identify all relevant studies of decision models in T2DM, some limitations of this review should be acknowledged. First, the data were extracted mainly through the primary study for each model, rather than the latest study, which may cause some of the latest views on models to be ignored. In general, ICERs were also obtained when calculating QALYs to perform CUA. However, in model outcomes, 13 models reported QALYs, and only 10 of these models reported ICERs. This may be due to the lack of data from secondary studies. A similar review should be conducted on secondary studies of each model to provide a more comprehensive evaluation of the included models. Second, models with a limited focus on particular sub-components of T2DM were excluded. Models focused on particular sub-components of T2DM may provide a more meticulous and complex simulation method. However, these models only involved specific components of T2DM, which may lead to failure to consider the connection of the various components of diabetes in modeling. Finally, the assessment of study quality may be biased, as some studies were not described in full detail because of word limits for publications.
We conducted a comprehensive systematic review focusing on capabilities of the existing decision models for T2DM, and briefly summarized the more suitable models for different study demands. It is necessary to use decision models to simulate the lifelong course of diseases, especially for chronic diseases, to evaluate whether new technologies or interventions have values. A general conclusion from the review is that the existing decision models for T2DM were rather heterogeneous on the level of detail in the classification of health states. Thus, more attention should be focused on balancing the desired level of complexity against the required level of transparency in the development of T2DM decision models. Furthermore, we should consider including secondary studies for a more comprehensive systematic review.
Registration
This systematic review was registered in the PROSPERO database (CRD42020171838). https://www.crd.york.ac.uk/prospero/display_record.php?ID=CRD42020171838
Availability of data and material
Evaluated studies are publicly available peer-reviewed scientific publications.
International Diabetes Federation (2019) IDF Diabetes Atlas. 9th ed. Brussels: International Diabetes Federation; 2019. Available at http://www.diabetesatlas.org . Accessed Feb 2020
Global, regional, and national disability-adjusted life-years (DALYs) for 333 diseases and injuries and healthy life expectancy (HALE) for 195 countries and territories, 1990–2016: a systematic analysis for the Global Burden of Disease Study 2016 (2017). Lancet 390(10100):1260–1344. https://doi.org/10.1016/s0140-6736(17)32130-x
Wang L, Gao P, Zhang M et al (2017) Prevalence and ethnic pattern of diabetes and prediabetes in China in 2013. JAMA 317(24):2515–2523. https://doi.org/10.1001/jama.2017.7596
Article PubMed PubMed Central Google Scholar
Chan JC, Zhang Y, Ning G (2014) Diabetes in China: a societal solution for a personal challenge. Lancet Diabetes Endocrinol 2(12):969–979. https://doi.org/10.1016/s2213-8587(14)70144-5
Article PubMed Google Scholar
Xu Y, Wang L, He J et al (2013) Prevalence and control of diabetes in Chinese adults. JAMA 310(9):948–959. https://doi.org/10.1001/jama.2013.168118
Article CAS PubMed Google Scholar
Dakin HA, Devlin NJ, Odeyemi IA (2006) “Yes”, “No” or “Yes, but”? Multinomial modelling of NICE decision-making. Health Policy 77(3):352–367. https://doi.org/10.1016/j.healthpol.2005.08.008
Caro JJ, Briggs AH, Siebert U, Kuntz KM (2012) Modeling good research practices–overview: a report of the ISPOR-SMDM Modeling Good Research Practices Task Force–1. Value Health 15(6):796–803. https://doi.org/10.1016/j.jval.2012.06.012
Weinstein MC, O’Brien B, Hornberger J et al (2003) Principles of good practice for decision analytic modeling in health-care evaluation: report of the ISPOR Task Force on Good Research Practices-Modeling Studies. Value Health 6(1):9–17. https://doi.org/10.1046/j.1524-4733.2003.00234.x
Zhou H, Isaman DJM, Messinger S et al (2005) A computer simulation model of diabetes progression, quality of life, and cost. Diabetes Care 28(12):2856–2863. https://doi.org/10.2337/diacare.28.12.2856
McEwan P, Peters JR, Bergenheim K, Currie CJ (2006) Evaluation of the costs and outcomes from changes in risk factors in type 2 diabetes using the Cardiff stochastic simulation cost-utility model (DiabForecaster). Curr Med Res Opin 22(1):121–129. https://doi.org/10.1185/030079906x80350
O’Reilly D, Hopkins R, Blackhouse G et al (2007) Long-term cost-utility analysis of a multidisciplinary primary care diabetes management program in Ontario. Can J Diabetes 31(3):205–214. https://doi.org/10.1016/S1499-2671(07)13007-0
Article Google Scholar
Hoerger TJ, Bethke AD, Richter A et al (2002) Cost-effectiveness of intensive glycemic control, intensified hypertension control, and serum cholesterol level reduction for type 2 diabetes. J Am Med Assoc 287(19):2542–2551
Kahn R (2004) Guidelines for computer modeling of diabetes and its complications. Diabetes Care 27(9):2262–2265. https://doi.org/10.2337/diacare.27.9.2262
Leal J, Morrow LM, Khurshid W, Pagano E, Feenstra T (2019) Decision models of prediabetes populations: a systematic review. Diabetes Obes Metab 21(7):1558–1569. https://doi.org/10.1111/dom.13684
Henriksson M, Jindal R, Sternhufvud C et al (2016) A systematic review of cost-effectiveness models in type 1 diabetes mellitus. Pharmacoeconomics 34(6):569–585. https://doi.org/10.1007/s40273-015-0374-8
Page MJ, McKenzie JE, Bossuyt PM et al (2021) The PRISMA 2020 statement: An updated guideline for reporting systematic reviews. PLoS Med 18(3):e1003583. https://doi.org/10.1371/journal.pmed.1003583
Philips Z, Bojke L, Sculpher M, Claxton K, Golder S (2006) Good practice guidelines for decision-analytic modelling in health technology assessment: a review and consolidation of quality assessment. Pharmacoeconomics 24(4):355–371. https://doi.org/10.2165/00019053-200624040-00006
Wright D, Little R, Turner D, Thornley T (2019) Diabetes screening through community pharmacies in England: A cost-effectiveness study. Pharm (Basel). https://doi.org/10.3390/pharmacy7010030
Eddy DM, Schlessinger L (2003) Archimedes: a trial-validated model of diabetes. Diabetes Care 26(11):3093–3101. https://doi.org/10.2337/diacare.26.11.3093
Eastman RC, Javitt JC, Herman WH et al (1997) Model of complications of NIDDM I Model construction and assumptions. Diabetes Care 20(5):725–734
Article CAS Google Scholar
Clarke PM, Gray AM, Briggs A et al (2004) A model to estimate the lifetime health outcomes of patients with type 2 diabetes: the United Kingdom prospective diabetes study (UKPDS) outcomes model (UKPDS No. 68). Diabetologia 47(10):1747–1759. https://doi.org/10.1007/s00125-004-1527-z
Gillett M, Dallosso HM, Dixon S et al (2010) Delivering the diabetes education and self management for ongoing and newly diagnosed (DESMOND) programme for people with newly diagnosed type 2 diabetes: Cost effectiveness analysis. BMJ. https://doi.org/10.1136/bmj.c4093
Lundqvist A, Carlsson KS, Johansen P, Andersson E, Willis M (2014) Validation of the IHE cohort model of type 2 diabetes and the impact of choice of macrovascular risk equations. PLoS ONE. https://doi.org/10.1371/journal.pone.0110235
Willis M, Asseburg C, He J (2013) Validation of economic and health outcomes simulation model of type 2 diabetes mellitus (ECHO-T2DM). J Med Econ 16(8):1007–1021. https://doi.org/10.3111/13696998.2013.809352
Su ZT, Bartelt-Hofer J, Brown S et al (2019) The use of computer simulation modeling to estimate complications in patients with type 2 diabetes mellitus: comparative validation of the cornerstone diabetes simulation model. Pharmacoecon Open. https://doi.org/10.1007/s41669-019-0156-x
Article PubMed Central Google Scholar
Wu B, Ma J, Zhang S, Zhou L, Wu H (2018) Development and validation of a health policy model of type 2 diabetes in Chinese setting. J Comparative Effect Res. https://doi.org/10.2217/cer-2018-0001
Palmer AJ, Roze S, Valentine WJ et al (2004) The CORE Diabetes model: Projecting long-term clinical outcomes, costs and cost-effectiveness of interventions in diabetes mellitus (types 1 and 2) to support clinical and reimbursement decision-making. Curr Med Res Opin 20(Suppl 1):S5-26. https://doi.org/10.1185/030079904x1980
Hayes AJ, Leal J, Gray AM, Holman RR, Clarke PM (2013) UKPDS Outcomes Model 2: a new version of a model to simulate lifetime health outcomes of patients with type 2 diabetes mellitus using data from the 30 year United Kingdom prospective diabetes study: UKPDS 82. Diabetologia 56(9):1925–1933. https://doi.org/10.1007/s00125-013-2940-y
Asche CV, Hippler SE, Eurich DT (2014) Review of models used in economic analyses of new oral treatments for type 2 diabetes mellitus. Pharmacoeconomics 32(1):15–27. https://doi.org/10.1007/s40273-013-0117-7
Becker C, Langer A, Leidl R (2011) The quality of three decision-analytic diabetes models: a systematic health economic assessment. Expert Rev Pharmacoecon Outcomes Res 11(6):751–762. https://doi.org/10.1586/erp.11.68
Yi Y, Philips Z, Bergman G, Burslem K (2010) Economic models in type 2 diabetes. Curr Med Res Opin 26(9):2105–2118. https://doi.org/10.1185/03007995.2010.494451
Tarride JE, Hopkins R, Blackhouse G et al (2010) A review of methods used in long-term cost-effectiveness models of diabetes mellitus treatment. Pharmacoeconomics 28(4):255–277. https://doi.org/10.2165/11531590-000000000-00000
Lerner DJ, Kannel WB (1986) Patterns of coronary heart disease morbidity and mortality in the sexes: a 26-year follow-up of the Framingham population. Am Heart J 111(2):383–390. https://doi.org/10.1016/0002-8703(86)90155-9
Klein R, Klein BE, Moss SE (1993) Prevalence of microalbuminuria in older-onset diabetes. Diabetes Care 16(10):1325–1330. https://doi.org/10.2337/diacare.16.10.1325
Ballard DJ, Humphrey LL, Melton LJ 3rd et al (1988) Epidemiology of persistent proteinuria in type II diabetes mellitus Population-based study in Rochester Minnesota. Diabetes 37(4):405–412. https://doi.org/10.2337/diab.37.4.405
Javitt JC, Aiello LP, Chiang Y et al (1994) Preventive eye care in people with diabetes is cost-saving to the federal government. Implications for health-care reform. Diabetes Care 17(8):909–917. https://doi.org/10.2337/diacare.17.8.909
Klein R, Klein BE, Moss SE, Davis MD, DeMets DL (1989) The Wisconsin Epidemiologic Study of Diabetic Retinopathy. X. Four-year incidence and progression of diabetic retinopathy when age at diagnosis is 30 years or more. Arch Ophthalmol 107(2):244–249. https://doi.org/10.1001/archopht.1989.01070010250031
Park JY, Kim HK, Chung YE, Kim SW, Hong SK, Lee KU (1998) Incidence and determinants of microalbuminuria in Koreans with type 2 diabetes. Diabetes Care 21(4):530–534. https://doi.org/10.2337/diacare.21.4.530
Dyck PJ, Kratz KM, Karnes JL et al (1993) The prevalence by staged severity of various types of diabetic neuropathy, retinopathy, and nephropathy in a population-based cohort: the Rochester Diabetic Neuropathy Study. Neurology 43(4):817–824. https://doi.org/10.1212/wnl.43.4.817
Weinstein MC, Coxson PG, Williams LW et al (1987) Forecasting coronary heart disease incidence, mortality, and cost: the Coronary Heart Disease Policy Model. Am J Public Health 77(11):1417–1426. https://doi.org/10.2105/ajph.77.11.1417
Article CAS PubMed PubMed Central Google Scholar
Anderson KM, Odell PM, Wilson PW, Kannel WB (1991) Cardiovascular disease risk profiles. Am Heart J 121(1 Pt 2):293–298. https://doi.org/10.1016/0002-8703(91)90861-b
Hunink MG, Goldman L, Tosteson AN et al (1997) The recent decline in mortality from coronary heart disease, 1980–1990. The effect of secular trends in risk factors and treatment. JAMA 277(7):535–542
Eastman RC, Javitt JC, Herman WH et al (1997) Model of complications of NIDDM. II. Analysis of the health benefits and cost-effectiveness of treating NIDDM with the goal of normoglycemia. Diabetes Care 20(5):735–744
D’Agostino RB, Russell MW, Huse DM et al (2000) Primary and subsequent coronary risk appraisal: new results from the Framingham study. Am Heart J 139(2 Pt 1):272–281. https://doi.org/10.1067/mhj.2000.96469
Herlitz J, Bång A, Karlson BW (1996) Mortality, place and mode of death and reinfarction during a period of 5 years after acute myocardial infarction in diabetic and non-diabetic patients. Cardiology 87(5):423–428. https://doi.org/10.1159/000177131
Almbrand B, Johannesson M, Sjöstrand B, Malmberg K, Rydén L (2000) Cost-effectiveness of intense insulin treatment after acute myocardial infarction in patients with diabetes mellitus; results from the DIGAMI study. Eur Heart J 21(9):733–739. https://doi.org/10.1053/euhj.1999.1859
Wolfe RA, Ashby VB, Milford EL et al (1999) Comparison of mortality in all patients on dialysis, patients on dialysis awaiting transplantation, and recipients of a first cadaveric transplant. N Engl J Med 341(23):1725–1730. https://doi.org/10.1056/nejm199912023412303
Klein R, Klein BE, Moss SE, Davis MD, DeMets DL (1989) The wisconsin epidemiologic study of diabetic retinopathy. IX. Four-year incidence and progression of diabetic retinopathy when age at diagnosis is less than 30 years. Arch Ophthalmol 107(2):237–243. https://doi.org/10.1001/archopht.1989.01070010243030
Chaturvedi N, Sjolie AK, Stephenson JM et al (1998) Effect of lisinopril on progression of retinopathy in normotensive people with type 1 diabetes. The EUCLID Study Group. EURODIAB Controlled Trial of Lisinopril in Insulin-Dependent Diabetes Mellitus. Lancet 351(9095):28–31. https://doi.org/10.1016/s0140-6736(97)06209-0
Stratton IM, Kohner EM, Aldington SJ et al (2001) UKPDS 50: risk factors for incidence and progression of retinopathy in Type II diabetes over 6 years from diagnosis. Diabetologia 44(2):156–163. https://doi.org/10.1007/s001250051594
Partanen J, Niskanen L, Lehtinen J et al (1995) Natural history of peripheral neuropathy in patients with non-insulin-dependent diabetes mellitus. N Engl J Med 333(2):89–94. https://doi.org/10.1056/nejm199507133330203
The effect of intensive diabetes therapy on the development and progression of neuropathy The Diabetes Control and Complications Trial Research Group (1995). Ann Intern Med 122(8):561–568. https://doi.org/10.7326/0003-4819-122-8-199504150-00001
Petty GW, Brown RD Jr, Whisnant JP et al (1998) Survival and recurrence after first cerebral infarction: a population-based study in Rochester, Minnesota, 1975 through 1989. Neurology 50(1):208–216. https://doi.org/10.1212/wnl.50.1.208
Sprafka JM, Virnig BA, Shahar E, McGovern PG (1994) Trends in diabetes prevalence among stroke patients and the effect of diabetes on stroke survival: the Minnesota Heart Survey. Diabet Med 11(7):678–684. https://doi.org/10.1111/j.1464-5491.1994.tb00332.x
Ragnarson Tennvall G, Apelqvist J (2001) Prevention of diabetes-related foot ulcers and amputations: a cost-utility analysis based on Markov model simulations. Diabetologia 44(11):2077–2087. https://doi.org/10.1007/s001250100013
Ben-Ami H, Nagachandran P, Mendelson A, Edoute Y (1999) Drug-induced hypoglycemic coma in 102 diabetic patients. Arch Intern Med 159(3):281–284. https://doi.org/10.1001/archinte.159.3.281
Stepka M, Rogala H, Czyzyk A (1993) Hypoglycemia: a major problem in the management of diabetes in the elderly. Aging (Milano) 5(2):117–121. https://doi.org/10.1007/bf03324137
Intensive blood-glucose control with sulphonylureas or insulin compared with conventional treatment and risk of complications in patients with type 2 diabetes (UKPDS 33). UK Prospective Diabetes Study (UKPDS) Group (1998). Lancet 352(9131):837–853
Malmberg K, Yusuf S, Gerstein HC et al (2000) Impact of diabetes on long-term prognosis in patients with unstable angina and non-Q-wave myocardial infarction: results of the OASIS (Organization to Assess Strategies for Ischemic Syndromes) Registry. Circulation 102(9):1014–1019. https://doi.org/10.1161/01.cir.102.9.1014
Ulvenstam G, Aberg A, Bergstrand R et al (1985) Long-term prognosis after myocardial infarction in men with diabetes. Diabetes 34(8):787–792. https://doi.org/10.2337/diab.34.8.787
Löwel H, Koenig W, Engel S, Hörmann A, Keil U (2000) The impact of diabetes mellitus on survival after myocardial infarction: can it be modified by drug treatment? Results of a population-based myocardial infarction register follow-up study. Diabetologia 43(2):218–226. https://doi.org/10.1007/s001250050032
Stevens RJ, Kothari V, Adler AI, Stratton IM (2001) The UKPDS risk engine: a model for the risk of coronary heart disease in type II diabetes (UKPDS 56). Clin Sci (Lond) 101(6):671–679
Gall MA, Hougaard P, Borch-Johnsen K, Parving HH (1997) Risk factors for development of incipient and overt diabetic nephropathy in patients with non-insulin dependent diabetes mellitus: prospective, observational study. BMJ 314(7083):783–788. https://doi.org/10.1136/bmj.314.7083.783
Ravid M, Savin H, Jutrin I et al (1993) Long-term stabilizing effect of angiotensin-converting enzyme inhibition on plasma creatinine and on proteinuria in normotensive type II diabetic patients. Ann Intern Med 118(8):577–581. https://doi.org/10.7326/0003-4819-118-8-199304150-00001
Klein R, Klein BE, Moss SE, Cruickshanks KJ (1994) The Wisconsin Epidemiologic Study of diabetic retinopathy XIV Ten-year incidence and progression of diabetic retinopathy. Arch Ophthalmol 112(9):1217–1228. https://doi.org/10.1001/archopht.1994.01090210105023
Klein R, Klein BE, Moss SE, Cruickshanks KJ (1995) The wisconsin epidemiologic study of diabetic retinopathy. XV. The long-term incidence of macular edema. Ophthalmology 102(1):7–16. https://doi.org/10.1016/s0161-6420(95)31052-4
Moss SE, Klein R, Klein BE (1988) The incidence of vision loss in a diabetic population. Ophthalmology 95(10):1340–1348. https://doi.org/10.1016/s0161-6420(88)32991-x
Moss SE, Klein R, Klein BE (1994) Ten-year incidence of visual loss in a diabetic population. Ophthalmology 101(6):1061–1070. https://doi.org/10.1016/s0161-6420(94)31217-6
Sands ML, Shetterly SM, Franklin GM, Hamman RF (1997) Incidence of distal symmetric (sensory) neuropathy in NIDDM. The San Luis valley diabetes study. Diabetes Care 20(3):322–329. https://doi.org/10.2337/diacare.20.3.322
Adler AI, Boyko EJ, Ahroni JH, Smith DG (1999) Lower-extremity amputation in diabetes. The independent effects of peripheral vascular disease, sensory neuropathy, and foot ulcers. Diabetes Care 22(7):1029–1035. https://doi.org/10.2337/diacare.22.7.1029
Hier DB, Foulkes MA, Swiontoniowski M et al (1991) Stroke recurrence within 2 years after ischemic infarction. Stroke 22(2):155–161. https://doi.org/10.1161/01.str.22.2.155
Sacco RL, Shi T, Zamanillo MC, Kargman DE (1994) Predictors of mortality and recurrence after hospitalized cerebral infarction in an urban community: the Northern Manhattan Stroke Study. Neurology 44(4):626–634. https://doi.org/10.1212/wnl.44.4.626
Kothari V, Stevens RJ, Adler AI et al (2002) UKPDS 60: risk of stroke in type 2 diabetes estimated by the UK prospective diabetes study risk engine. Stroke 33(7):1776–1781. https://doi.org/10.1161/01.str.0000020091.07144.c7
Currie CJ, Morgan CL, Peters JR (1998) The epidemiology and cost of inpatient care for peripheral vascular disease, infection, neuropathy, and ulceration in diabetes. Diabetes Care 21(1):42–48. https://doi.org/10.2337/diacare.21.1.42
Clarke P, Gray A, Legood R, Briggs A, Holman R (2003) The impact of diabetes-related complications on healthcare costs: results from the United Kingdom Prospective Diabetes Study (UKPDS Study No. 65). Diabet Med 20(6):442–450. https://doi.org/10.1046/j.1464-5491.2003.00972.x
Effect of intensive therapy on the development and progression of diabetic nephropathy in the Diabetes Control and Complications Trial. The Diabetes Control and Complications (DCCT) Research Group (1995). Kidney Int 47(6):1703–1720. https://doi.org/10.1038/ki.1995.236
Bagust A, Hopkinson PK, Maier W, Currie CJ (2001) An economic model of the long-term health care burden of type II diabetes. Diabetologia 44(12):2140–2155. https://doi.org/10.1007/s001250100023
Ahmad Kiadaliri A, Gerdtham UG, Nilsson P et al (2013) Towards renewed health economic simulation of type 2 diabetes: risk equations for first and second cardiovascular events from Swedish register data. PLoS ONE 8(5):e62650. https://doi.org/10.1371/journal.pone.0062650
Brown JB, Russell A, Chan W, Pedula K, Aickin M (2000) The global diabetes model: user friendly version 3.0. Diabetes Res Clin Pract 50(Suppl 3):S15-46. https://doi.org/10.1016/s0168-8227(00)00215-1
Gerstein HC, Miller ME, Genuth S et al (2011) Long-term effects of intensive glucose lowering on cardiovascular outcomes. N Engl J Med 364(9):818–828. https://doi.org/10.1056/NEJMoa1006524
Wing RR, Bolin P, Brancati FL et al (2013) Cardiovascular effects of intensive lifestyle intervention in type 2 diabetes. N Engl J Med 369(2):145–154. https://doi.org/10.1056/NEJMoa1212914
Perreault L, Pan Q, Mather KJ et al (2012) Effect of regression from prediabetes to normal glucose regulation on long-term reduction in diabetes risk: results from the diabetes prevention program outcomes study. Lancet 379(9833):2243–2251. https://doi.org/10.1016/s0140-6736(12)60525-x
Patel A, MacMahon S, Chalmers J et al (2008) Intensive blood glucose control and vascular outcomes in patients with type 2 diabetes. N Engl J Med 358(24):2560–2572. https://doi.org/10.1056/NEJMoa0802987
Clarke PM, Simon J, Cull CA, Holman RR (2006) Assessing the impact of visual acuity on quality of life in individuals with type 2 diabetes using the short form-36. Diabetes Care 29(7):1506–1511. https://doi.org/10.2337/dc05-2150
McEwan P, Bennett H, Ward T, Bergenheim K (2015) Refitting of the UKPDS 68 risk equations to contemporary routine clinical practice data in the UK. Pharmacoeconomics 33(2):149–161. https://doi.org/10.1007/s40273-014-0225-z
Diabetes Control and Complications Trial Research Group (1995) The effect of intensive diabetes treatment on the progression of diabetic retinopathy in insulin-dependent diabetes mellitus. The Diabetes Control and Complications Trial. Arch Ophthalmol 113(1):36–51. https://doi.org/10.1001/archopht.1995.01100010038019
Download references
Acknowledgements
This work was sponsored by National Key R&D Program of China (No. 2018YFC1311502), and Gansu Province Health Industry Research Project (No. GSWSKY2020-08). The funders had no role in the study design, data collection and analysis, decision to publish, or manuscript preparation.
Author information
Jiayu Li and Yun Bao contributed equally to this work.
Authors and Affiliations
Department of Endocrinology, Gansu Provincial Hospital, Lanzhou, 730000, Gansu Province, China
Jiayu Li, Xuedi Chen & Limin Tian
Clinical Research Center for Metabolic Diseases, No. 204 Donggang west road, Lanzhou, 730000, Gansu Province, China
Jiayu Li, Yun Bao, Xuedi Chen & Limin Tian
School of Clinical Medicine, Ningxia Medical University, Yinchuan, 750004, Ningxia Province, China
You can also search for this author in PubMed Google Scholar
Contributions
All authors contributed to the study conception and design. Material preparation, data collection and analysis were performed by Jiayu Li, Yun Bao and Xuedi Chen. Jiayu Li and Yun Bao contributed equally to this work. All authors commented on previous versions of the manuscript. All authors read and approved the final manuscript.
Corresponding author
Correspondence to Limin Tian .
Ethics declarations
Conflict of interest.
The authors declare that they have no conflicts of interest.
Human and Animal Rights disclosure
This article does not contain any studies with human or animal subjects performed by the any of the authors.
Informed consent
No identifying information from individual patients was retrieved or published at any stage by any of the authors.
Additional information
Managed By Massimo Porta.
Publisher's Note
Springer Nature remains neutral with regard to jurisdictional claims in published maps and institutional affiliations.
Supplementary Information
Below is the link to the electronic supplementary material.
Supplementary file1 (DOCX 170 kb)
Rights and permissions.
Open Access This article is licensed under a Creative Commons Attribution 4.0 International License, which permits use, sharing, adaptation, distribution and reproduction in any medium or format, as long as you give appropriate credit to the original author(s) and the source, provide a link to the Creative Commons licence, and indicate if changes were made. The images or other third party material in this article are included in the article's Creative Commons licence, unless indicated otherwise in a credit line to the material. If material is not included in the article's Creative Commons licence and your intended use is not permitted by statutory regulation or exceeds the permitted use, you will need to obtain permission directly from the copyright holder. To view a copy of this licence, visit http://creativecommons.org/licenses/by/4.0/ .
Reprints and permissions
About this article
Li, J., Bao, Y., Chen, X. et al. Decision models in type 2 diabetes mellitus: A systematic review. Acta Diabetol 58 , 1451–1469 (2021). https://doi.org/10.1007/s00592-021-01742-6
Download citation
Received : 27 February 2021
Accepted : 07 May 2021
Published : 03 June 2021
Issue Date : November 2021
DOI : https://doi.org/10.1007/s00592-021-01742-6
Share this article
Anyone you share the following link with will be able to read this content:
Sorry, a shareable link is not currently available for this article.
Provided by the Springer Nature SharedIt content-sharing initiative
- Type 2 diabetes mellitus
- Decision model
- Cost-utility
- Find a journal
- Publish with us
- Track your research
- Open access
- Published: 13 July 2020
Screening strategies for adults with type 2 diabetes mellitus: a systematic review protocol
- Helen Mearns ORCID: orcid.org/0000-0002-7971-1256 1 , 2 ,
- Paul Kuodi Otiku 1 , 2 ,
- Mary Shelton 3 ,
- Tamara Kredo 4 , 5 ,
- Benjamin M. Kagina 1 , 2 &
- Bey-Marrié Schmidt 4
Systematic Reviews volume 9 , Article number: 156 ( 2020 ) Cite this article
6509 Accesses
4 Citations
9 Altmetric
Metrics details
There is limited evidence on whether screening for type 2 diabetes mellitus affects health outcomes. A recent systematic review of randomised clinical trials found only one trial that met their inclusion criteria; therefore, current guidelines for screening interventions for type 2 diabetes mellitus are based on expert opinions and best practice rather than synthesised evidence. This systematic review seeks to collate evidence from non-randomised studies to investigate the effect of screening for adults with type 2 diabetes on outcomes including diabetes-related morbidity, mortality (all-cause and diabetes-related) and harms.
This systematic review will follow Effective Practice and Organisation of Care (EPOC) guidelines for the synthesis of non-randomised studies. We will search PubMed/MEDLINE, Scopus, Web of Science, CINAHL, Academic Search Premier and Health Source Nursing Academic (from inception onwards). We will include non-randomised trials, controlled before-after studies, interrupted time-series studies, repeated measures studies and concurrently controlled prospective cohort studies. The primary outcome will be diabetes-related morbidity (microvascular complications of diabetic retinopathy, nephropathy or neuropathy or macrovascular complications of non-fatal myocardial infarction, peripheral arterial disease or non-fatal stroke). The secondary outcomes will be mortality (all-cause and diabetes-related) and harms of screening strategies to patients (including psychological harms or adverse events following treatments) or to health care system (including resource allocation for false-positives or overdiagnosis). Two reviewers will independently screen all citations and full-text articles. Data will be abstracted by one reviewer and checked by a second. The risk of bias of individual studies will be appraised using the ROBINS-I tool. GRADE will be used to determine the quality of the scientific evidence. If feasible, we will conduct random effects meta-analysis where appropriate. If necessary, analyses will be conducted to explore the potential sources of heterogeneity (e.g. age, sex, socio-economic status, rural versus urban or low-middle income versus high-income country). We will disseminate the findings via publications and through relevant networks.
The protocol outlines the methods for systematically reviewing and synthesising evidence of screening strategies for type 2 diabetes mellitus and their effect on health outcomes associated with the disease. The potential impact of this systematic review is improved evidence-informed decision-making for policies and practice for screening of type-2 diabetes.
Systematic review registration
PROSPERO CRD42020147439
Peer Review reports
Description of the condition
Diabetes mellitus is a disease of increasing global concern. The global prevalence of diabetes was approximately 425 million people in 2017, approximately 8.5% of the adult population, and is expected to double by 2045 [ 1 ]. In high-income countries, type 2 diabetes mellitus accounts for approximately 90% of diabetes cases; there is insufficient data to estimate the ratio of type 2 diabetes mellitus in low- and middle- income countries, but it is assumed to be similar [ 1 , 2 ]. Clinical diabetes is diagnosed through the detection of elevated levels of glucose in the blood (hyperglycemia) [ 3 ]; however, it is estimated that half of the people who have diabetes are not diagnosed [ 1 ].
In addition to those individuals who have clinical diabetes, another 352 million, approximately 7.3% of the adult population, have intermediate blood glucose levels that are considered in between normal and clinically diagnosed diabetes [ 1 , 3 ]. These intermediate blood glucose levels perform as a risk score, where increasing values are associated with an increasing likelihood of progression to diabetes, cardiovascular disease and all-cause mortality [ 2 , 4 ]. Patients who present with intermediate levels of blood glucose are described using a number of terminologies including mild glucose intolerance, non-diabetic hyperglycaemia and prediabetes. The terminology promoted by the World Health Organization (WHO) is impaired glucose tolerance (IGT), impaired fasting glucose (IFG) and intermediate hyperglycaemia [ 3 , 5 ]. The term prediabetes is gaining in popularity even though the WHO has warned its use may lead to disease stigma and detract from the significant cardiovascular risk of this population [ 5 ]. About a third of people with IGT and IFG are young, aged between 20–39 years, meaning they will spend many years at risk of developing diabetes [ 1 ]. Other risk factors, apart from intermediate glucose levels, for the development of diabetes are increasing age of more than 45 years and obesity [ 2 ].
Type 2 diabetes mellitus arises due to defective insulin activity in body tissues, defective insulin secretion from pancreas or a combination of the two [ 2 ]. Type 2 diabetes mellitus usually occurs in older adults, but with a change in lifestyle factors, such as inactivity and obesity, the condition is increasingly being detected in children, adolescents and young adults [ 1 , 2 ]. Current management of type 2 diabetes mellitus involves lifestyle modification: increasing physical activity, improving diet, reaching a healthy body weight and stopping smoking, all monitored by regular screening [ 2 ]. If lifestyle modification does not result in sufficiently decreased blood glucose levels, medication may be prescribed, of which there are a range of treatment options available [ 2 ]. The complication with type 2 diabetes mellitus is the long latency period, often lasting several years, during which time the individual is often asymptomatic and unaware of their condition [ 1 , 2 ]. This prolonged asymptomatic state results in long-term damage to the body’s organs that leads to negative health outcomes including pregnancy complications, oral health problems, disabilities such as blindness, reduced wound healing, foot disease that may require amputation, stroke, heart and kidney disease and death [ 1 , 2 , 3 ].
Description of the intervention
There are many types of screening interventions and strategies that may be used to detect disease in a population often classified as mass, opportunistic and targeted strategies—as presented in Table 1 [ 2 , 6 ]. This systematic review will use these classifications, but if additional strategies are noted, these too will be included.
The biochemical tests commonly used are fasting plasma glucose (FPG), oral glucose tolerance test (OGTT) and detection of glycated haemoglobin A1C (HbA1c) although there are also urine glucose tests available or random blood glucose tests [ 2 , 6 ]. In addition, there are a number of risk scores [ 7 , 8 ], including the Finnish Diabetes Risk Score (FINDRISC) [ 9 ] and the American Diabetes Association’s risk test [ 10 ]; however, these are not commonly used as stand-alone screening tools. Classification of patients post testing can be termed as in the normal range or as having diabetes, impaired glucose tolerance (IGT) or impaired fasting glucose (IFG) (as presented in Table 2 ) [ 1 , 3 ].
How the intervention might work
The theory behind screening for type 2 diabetes mellitus is to identify either disease or associated risk factors to initiate preventative measures that can halt, slow or improve the course of disease [ 11 ]. Therefore, the earlier the disease is detected, especially where there is high risk of disease, theoretically, the better the expected outcomes. The logic model in Fig. 1 describes a complex system in which the intervention interacts with participants, context, implementation and how these affect the outcomes and the impact of this research [ 12 ].
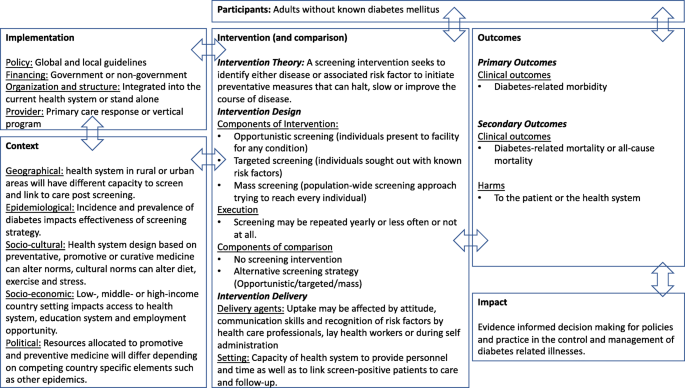
Logic model describing the interactions between screening for diabetes, implementation, context, participants, outcomes and impact
Why it is important to do this review
Guidelines for screening interventions for type 2 diabetes mellitus, such as those released by the UK National Screening Committee [ 13 ], the American Diabetes Association [ 2 ] or the Society for Endocrinology, Metabolism and Diabetes of South Africa [ 14 ], are based on expert opinion and local practice rather than synthesised evidence. This is because there is limited information to provide evidence about best practice for screening interventions for type 2 diabetes mellitus and even less evidence in low- and middle-income countries [ 15 ]. A recently published Cochrane review assessed the effects of any type of screening compared with no screening for type 2 diabetes [ 16 ] and found only one trial, the ADDITION-Cambridge trial [ 17 ], that met their inclusion criteria. The ADDITION-Cambridge trial consisted of 20,184 participants aged 40–69 years from general practices in England who were at risk for diabetes but had no known diabetes. These participants were randomised to screening versus no screening arms, and followed up for a median of 9.6 years (November 2001 to November 2011). The review found moderate certainty evidence that screening for diabetes probably makes little or no difference to all-cause mortality and low certainty evidence that it may make little or no difference to diabetes-related mortality. However, because the review only included one trial, firm conclusions about early diabetes screening on health outcomes cannot be drawn. In consultation with the authors of the unpublished Cochrane review and considering the public health importance of screening and the potential impact on large populations, we propose to assess evidence from non-randomised intervention study designs. The questions for the systematic review will include the following: Does screening for type 2 diabetes mellitus reduce morbidity and/or mortality? Does a particular screening strategy result in a greater reduction of morbidity and/or mortality as compared to another screening strategy? Does screening for type 2 diabetes mellitus result in harms to participants or the health system?
Primary objective
To assess the effectiveness of targeted, opportunistic or mass screening for type 2 diabetes mellitus on reduction of diabetes-associated morbidity in adults
Secondary objectives
To assess the effectiveness of targeted, opportunistic or mass screening for type 2 diabetes mellitus on reduction of mortality (all cause as well as diabetes-associated) in adults
To assess the harms of targeted, opportunistic or mass screening for type 2 diabetes mellitus in adults
The present protocol has been registered within the PROSPERO database (CRD42020147439). This manuscript is being reported in accordance with the reporting guidance provided in the Preferred Reporting Items for Systematic Reviews and Meta-Analyses Protocols (PRISMA-P) statement [ 18 ] (see checklist in Additional file 1 ).
Study and source eligibility
Types of studies.
As existing reviews have found limited randomised evidence addressing this question [ 15 , 19 , 20 ], we will focus on non-randomised intervention studies (NRIS). We will employ the Cochrane EPOC criteria [ 21 ], and NRIS of interest will include non-randomised trials, controlled before-after studies, interrupted time-series study, repeated measures study and concurrently controlled prospective cohort study. The difficulty associated with labelling NRIS is well-documented in the literature; several of these designs, for example, have been used interchangeably; we will make use of the EPOC definitions and flow diagram to assist in study design identification ( Appendix 1 ):
Non-randomised trial (NRT) is a study design in which individual participants, or clusters of participants, are allocated to intervention or comparator in a quasi-random or non-random manner. If there is an allocation rule, it is often by, for example, alternation, day of the week, odd/even hospital, or identification number.
Controlled before-after (CBA) is a study design that estimates intervention effectiveness by comparing pre- and post-intervention outcomes in individuals or clusters that receive the intervention and those that do not.
Interrupted time series (ITS) studies design uses multiple observations from individuals or clusters pre-intervention to establish the pre-existing outcome trend; intervention effectiveness is then estimated by measuring post-intervention changes in the expected outcome trend associated with the introduction of an intervention (the ‘interruption’). An ITS study can identify both immediate and long-term changes associated with the intervention. The interrupted time-series studies will be required to have a clearly defined point in time when the intervention occurred and a minimum of 3 time points before and 3 time points after the intervention [ 21 ].
A repeated measures (RM) study is an interrupted time-series study but where the outcomes of interest are measured in the same participants at each point in time.
Concurrently controlled prospective cohort study (PCS) is where subjects are identified prospectively as having received an intervention or comparator and are then followed over time. The allocation rule is often in relation to organizational factors such as ward, clinic, doctor or provider organisation. Control arms should be contemporaneous, we will not include retrospective control arms.
‘PICO’ eligibility
Types of participants.
We will include adults aged 18 years and older without documented diabetes mellitus or pregnancy.
Types of interventions
We will include studies comparing one of the screening strategies, targeted, opportunistic or mass screening interventions for the detection of type 2 diabetes mellitus, against no screening or another of the screening strategies (Table 1 ). There will be a 6-month minimum follow-up time required for the primary clinical outcome of morbidity.
Types of outcome measures
Primary outcomes.
Clinical outcomes
Diabetes-related morbidity defined as study-reported microvascular complications (diabetic retinopathy, diabetic nephropathy, diabetic neuropathy) or macrovascular complications (non-fatal myocardial infarction, peripheral arterial disease, non-fatal stroke) and measured from 6 months after screening
Secondary outcomes
Mortality (all-cause and diabetes-related) defined as death due to any-cause including diabetes or other cardiovascular causes (including acute myocardial infarction, ischemic heart disease, stroke or any cardiovascular disorder that lead to death) and measured at any time after screening
Harms of diabetes screening
Harms to patients is defined as event/s reported in the study at any time after screening.
Psychological harms such as anxiety or stigma that impacts on quality of life due to a false-positive test
Number of days of work lost
Side-effects from treatment
Loss of health insurance benefits
Harms to health care system is defined as event/s reported in the study at any time after screening.
False-positive test resulting in human, physical and financial resource allocation to patients who are not in need
Overdiagnosis may lead to over-extension of human, physical and financial resources for patients who end up in prolonged treatment and engagement with the health system even if they never develop disease
The rationale for prioritisation of outcomes: Primary outcome serves to inform whether screening alters the course of disease as assumed per screening theory [ 11 ] and depicted in Fig. 1 . Secondary outcome of mortality contributes to the current data outlining no reduction in mortality following screening intervention [ 19 ] while also assessing harms that may arise from screening intervention [ 3 ] and therefore contribute to evidence to substantiate policy and practice recommendations.
Search methods for identification of studies
Electronic searches.
The University of Cape Town Health Sciences Reference Librarian (MS) assisted the first author (HM) in developing the search strategy and will provide advice and guidance in conducting the searches for the review.
Electronic Database Search (from inception onwards)
PubMed (MEDLINE)
Scopus (includes majority of EMBASE contents)
Web of Science Platform (Web of Science Core Collection, Biological Abstracts, SciELO Citation Index)
Academic Search Premier (on the EBSCOhost platform)
CINAHL (on the EBSCOhost platform)
Health Source Nursing Academic (on the EBSCOhost platform)
A draft search strategy for PubMed/MEDLINE, based on the original search strategy utilised by the Cochrane Review team and revised by an information specialist, is provided in Appendix 2 (see Appendix 2 ). We will include all studies regardless of publication status; however, we will only include English language studies. We are aware that this decision may lead to language bias [ 22 ], but due to capacity and resource limitation of the systematic review team, we are restricted to English only. We will search all databases from inception to the date of search. The search syntax will first be tested and optimised in PubMed. We will thereafter replicate the searches in the other databases adapting search syntax as necessary for those databases.
Grey literature search
We will conduct a grey literature search to identify studies not indexed in the databases listed above.
OpenGrey (multidisciplinary European database, covering science, technology, biomedical science, economics, social science and humanities)
Conference abstracts from The American Diabetes Association (ADA), the European Association for the Study of Diabetes (EASD) meeting and Diabetologia will be used to track down full text articles.
National Institute for Health Research Economic Evaluation Database (NHS EED)
Cost-Effectiveness Analysis Registry (CEA) (www.healtheconomics)
We will search key references, such as systematic reviews, by cross-checking reference lists for additional potentially eligible primary studies [ 23 ]. We will also contact experts in the field to check if we have missed any relevant studies. We may contact authors of included studies to clarify reported published information and to seek unpublished data.
Methods for screening search results
Screening methods.
We will collate and transfer search results to the Rayyan screening software [ 24 ] and remove duplicate records. At least two review authors will independently screen titles and abstracts of every record retrieved. Outcome measures will not be used to exclude studies during title and abstract screening. The potentially eligible records will be retrieved for full text screening. The two review authors will independently review full text records for compliance of studies with eligibility criteria of the review. A decision tree based on the eligibility criteria will be used to assist in decision making for exclusion of studies (see Appendix 3 ). Two review authors will resolve any disagreements through discussion or, if required, will consult a third review author. A study must meet all inclusion criteria to be included. We will list excluded studies at the full text screening stage in the ‘Characteristics of excluded studies’ table. We will collate multiple reports of the same study so that each study rather than each report is the unit of interest in the review. We will provide any information we can obtain about ongoing studies. We will record the selection process in sufficient detail to complete a PRISMA flow diagram [ 25 ].
Data collection and analysis
Data extraction.
We will use a standard data extraction form in Microsoft Excel to capture study characteristics and outcome data [ 22 , 26 ]; we will pilot the form on at least one eligible study. One review author will extract the following study characteristics from the included studies, and an independent review author will check the extraction:
Source: study ID (created by review author), review author ID (created by review author), citation and contact details
Eligibility: confirm eligibility for review, reason for exclusion
Methods: study design, number of study centres and location, study setting, withdrawals, date of study, follow-up, confounding factors considered, and the methods used to control for confounding, aspects of risk of bias specific for NRIS (see “Assessment of risk of bias in included studies” below), how missing data was handled
Participants: number, mean/median age, age range, gender, severity of condition, diagnostic criteria, inclusion criteria, exclusion criteria, screening criteria, diagnostic criteria, presence of known risk factors for type 2 diabetes mellitus (obesity, family history), co-morbidity (hypertension, dyslipidaemia), socio-demographics
Interventions: intervention components, comparison, fidelity assessment using the Template for Intervention Description and Replication (TIDieR) as a guide [ 27 ]
Outcomes: primary and secondary outcomes specified above in the section “Types of outcome measures”.
Miscellaneous: funding source, notable conflicts of interest of study authors, ethical approval, key conclusions of the study authors, miscellaneous comments from the study authors, references to other relevant studies, correspondence required, miscellaneous comments by the review authors.
One review author will extract outcome data from included studies, and an independent review author will check extracted data. We will note in the ‘Characteristics of included studies’ table if outcome data were reported in an unusable way. We will resolve disagreements by consensus or by involving a third review author.
Assessment of risk of bias in included studies
Two review authors will independently assess risk of bias for each study using the ROBINS-I tool [ 28 ]. Any disagreement will be resolved by discussion or by involving a third review author.
We will assess the risk of bias according to the following domains:
Pre-intervention: bias due to confounding
Pre-intervention: bias in selection of participants into the study
At intervention: bias in classification of interventions
Post-intervention: bias due to deviations from intended interventions
Post-intervention: bias due to missing data
Post-intervention: bias in measurement of outcomes
Post-intervention: bias in selection of the reported result
We will judge each potential source of bias as low risk, moderate risk, serious risk, critical risk of bias or no information. We will summarise the ‘Risk of bias’ judgements across different studies for each of the domains listed. We will consider blinding separately for different key outcomes where necessary (e.g. for unblinded outcome assessment, risk of bias for all-cause mortality may be very different than for a patient reported pain scale). Where information on risk of bias relates to unpublished data or correspondence with a trialist, we will note this in the ‘Risk of bias’ table. We will not exclude studies on the grounds of their risk of bias but will clearly report the risk of bias when presenting the results of the studies. When considering treatment effects, we will take into account the risk of bias for the studies that contribute to that outcome. We will conduct the review according to this published protocol and report any deviations from it in the ‘Differences between protocol and review’ section of the systematic review.
Dealing with missing data
Authors will be contacted, and missing data will be requested. If only returned in part and data can be logically imputed, such as standard errors, this will occur. All missing data will be clearly reported in the data extraction forms and risk of bias table and as such be assessed in the sensitivity analysis.
Data management
EndNote X9 and Microsoft Excel will be used for data management. If there is a conflict between data reported across multiple sources for a single study (e.g. between a published article and a trial registry record), we will report the data from the first peer-reviewed published article.
Data synthesis
Preparation for data synthesis.
In preparation for synthesis (either meta-analyses or synthesis without meta-analysis), we will assess how much data are available for each of our objectives by creating a table to compare the PICO elements and the study design features as well as the extracted numerical data for the compilation of a meta-analysis.
Measures of treatment effect
We will estimate the effect of the intervention using risk ratio for dichotomous data, and mean difference or standardised mean difference for continuous data. Time to event outcomes will be reported as hazard ratios. If other effect estimates are provided, we will convert between estimates where possible. Measures of precision will be 95% confidence intervals. We will ensure that an increase in scores for continuous outcomes can be interpreted in the same way for each outcome, explain the direction to the reader, and report where the directions were reversed if this was necessary. Interrupted time series data will be analysed and, if required, a statistical comparison of time trends before and after the intervention will be performed. For ITS studies, the guideline as outlined in Analysis in EPOC reviews will be followed with assistance of a statistician to ensure integrity of analysis [ 29 ].
Unit of analysis issues
To avoid unit of analysis errors we will consider the unit used to cluster the intervention (such as a ward, clinic, doctor or provider organisation) or in the case of repeated measures that there will be multiple observations for the same outcome. For instance, multiple screening intervention events per participant may occur over time that may cause a unit-of-analysis error. In order to calculate the confidence intervals, the participants per treatment group rather than the number of intervention attempts will be used [ 22 ]. Multiple intervention groups could create unit-of-analysis issues especially if different screening interventions are compared against no screening intervention and use the same participants with no screening intervention in both comparisons [ 22 ]. If there is more than one comparison in the study design, we will combine groups into a single pairwise comparison. If there is a unit of analysis error in the reported analysis for a study and there is insufficient information to reanalyse the results, the study authors will be contacted to obtain necessary data. If these data are not available, we will not report confidence intervals or p values for which there is a unit of analysis error [ 30 ].
Quantitative synthesis
We will undertake meta-analyses only where this is meaningful, i.e. if the interventions, participants and the underlying clinical question are similar enough for pooling to make sense. If feasible and appropriate, outcome data from primary studies will be used to perform random effects meta-analyses. Since heterogeneity is expected a priori, we will estimate the pooled treatment effect estimates and its 95% confidence interval using the random effects model. The random effects model assumes that the effect estimates follow a normal distribution, considering both within-study and between-study variation.
Assessment of heterogeneity
Forest plots will be used to visualise the extent of heterogeneity among studies. We will quantify statistical heterogeneity by estimating the variance between studies using I 2 statistic. The I 2 is the proportion of variation in effect estimates that is due to genuine variation rather than sampling (random) error. I 2 ranges between 0 and 100% (with values of 0–25% and 75–100% taken to indicate low and considerable heterogeneity, respectively) [ 22 ]. We will also calculate the chi-squared test where a p value < 0.1 indicates statistically significant heterogeneity.
Assessment of publication bias
If we include more than 10 studies investigating a particular outcome, we will use a funnel plot to explore possible publication bias, interpreting the results with caution [ 31 ].
Subgroup analysis and investigation of heterogeneity
We expect the following population characteristics may introduce clinical heterogeneity: age, sex, socio-economic status [ 6 ].
We expect the following contexts may introduce health system heterogeneity: study setting of rural or urban or in a low-middle income country or a high-income country (as defined by the World Bank) [ 6 ].
We will use the following outcomes in subgroup analysis:
Diabetes-associated morbidity
Mortality (all-cause and diabetes-associated)
Sensitivity analysis
We may conduct a sensitivity analysis to explore the influence of various factors on the effect size of the primary outcomes of the review only. We will stratify studies according to:
Restricting the analysis to published studies.
Restricting the analysis to studies with a low risk of bias, as specified in “Assessment of risk of bias in included studies”
Imputing missing data.
Any post hoc sensitivity analyses that may arise during the review process will be justified in the final report.
Assessment of certainty of evidence using the GRADE approach
Two review authors will independently assess the certainty of the evidence (high, moderate, low and very low) for each outcome using the five GRADE considerations for downgrading the certainty of evidence (risk of bias, consistency of effect, imprecision, indirectness, and publication bias) and the three criteria for upgrading the certainty of evidence (large effect, dose response and residual confounding opposing the observed effect) [ 32 ]. We will use the GRADEpro software GDT [ 33 ] to create the ‘Summary of findings’ tables for the main intervention comparisons and include the following outcomes: diabetes-associated morbidity, mortality (all-cause and diabetes-associated), harms (see Appendix 4 for SoF). We will resolve disagreements on certainty ratings by discussion and provide justification for decisions to down- or upgrade the ratings using footnotes in the SoF table and make comments to aid readers’ understanding of the review where necessary. We will use plain language statements to report these findings in the review [ 34 ]. The SoF tables will be used to draw conclusions about the certainty of the evidence within the text of the review. If during the review process, we become aware of an important outcome that we failed to list in our planned ‘SoF’ tables, we will include the relevant outcome and explain the reasons for this is the section ‘Differences between protocol and review’.
Systematic reviews of screening for type 2 diabetes have found no evidence that this intervention saves lives [ 15 , 19 , 20 ]; therefore, this review will primarily focus on the impact of screening on the reduction of diabetes-associated morbidities. The impact of this review is synthesised data for the provision of evidence-based decision-making for informing policy and practice around screening strategies for type 2 diabetes mellitus. Important protocol amendments will be documented and noted in the discussion.
Limitations
The potential limitations of this review at a study (outcome) level include the following: the potential finding of insufficient studies of similar study design and clinical question to synthesise abstracted study data; the overall completeness and applicability of evidence and quality of evidence especially due to the limitation to non-randomised studies due to the lack of randomised studies and therefore the lower quality of evidence; the limitation to English studies only and therefore the potential to miss published research; the limitation of not being able to discern between all-cause mortality and diabetes-related mortality and therefore combining this outcome under one mortality outcome. The potential limitation of this review at a systematic review process level includes the potential biases in the review process such as post hoc analysis and focus of outcome objectives.
Availability of data and materials
Not applicable.
Abbreviations
American Diabetes Association
Controlled before-after
Cost-Effectiveness Analysis Registry
Collaboration for Evidence-Based Healthcare and Public Health in Africa
European Association for the Study of Diabetes
Effective Practice and Organisation of Care
Fasting plasma glucose
Grading of Recommendations Assessment, Development and Evaluation
Detection of glycated haemoglobin A1C
Interrupted time series
NHS Economic Evaluation Database
Non-randomised trial
Non-randomised intervention studies
Oral glucose tolerance test
Prospective cohort study
Preferred Reporting Items for Systematic Reviews and Meta-Analyses
Repeated measures
Summary of findings
Template for Intervention Description and Replication
Ogurtsova K, da Rocha Fernandes JD, Huang Y, Linnenkamp U, Guariguata L, Cho NH, et al. IDF Diabetes Atlas: global estimates for the prevalence of diabetes for 2015 and 2040. Diabetes Res Clin Pract. 2017;128:40–50.
Article CAS Google Scholar
American Diabetes Association. 2. Classification and diagnosis of diabetes: standards of medical care in diabetes-2018. Diabetes Care. 2018;41(Suppl 1):S13–27.
Article Google Scholar
World Health Organization. Global report on diabetes. 2016.
Sorkin JD, Muller DC, Fleg JL, Andres R. The relation of fasting and 2-h postchallenge plasma glucose concentrations to mortality: data from the Baltimore Longitudinal Study of Aging with a critical review of the literature. Diabetes Care. 2005;28(11):2626–32.
World Health Organization, International Diabetes Federation. Definition and diagnosis of diabetes mellitus and intermediate hyperglycaemia : report of a WHO/IDF consultation. World Health Organization. 2006.
World Health Organization. Screening for type 2 diabetes. Geneva, Switzerland: Department of Noncommunicable Disease Management; 2003.
Google Scholar
Schulze MB, Hoffmann K, Boeing H, Linseisen J, Rohrmann S, Mohlig M, et al. An accurate risk score based on anthropometric, dietary, and lifestyle factors to predict the development of type 2 diabetes. Diabetes Care. 2007;30(3):510–5.
Muhlenbruch K, Ludwig T, Jeppesen C, Joost HG, Rathmann W, Meisinger C, et al. Update of the German Diabetes Risk Score and external validation in the German MONICA/KORA study. Diabetes Res Clin Pract. 2014;104(3):459–66.
Jolle A, Midthjell K, Holmen J, Carlsen SM, Tuomilehto J, Bjorngaard JH, et al. Validity of the FINDRISC as a prediction tool for diabetes in a contemporary Norwegian population: a 10-year follow-up of the HUNT study. BMJ Open Diabetes Res Care. 2019;7(1):e000769.
American Diabetes Association. Screening for diabetes. Diabetes Care. 2002;25(suppl 1):s21–s4.
Speechley M, Kunnilathu A, Aluckal E, Balakrishna MS, Mathew B, George EK. Screening in public health and clinical care: similarities and differences in definitions, types, and aims - a systematic review. J Clin Diagn Res. 2017;11(3):LE01–LE4.
PubMed PubMed Central Google Scholar
Rohwer A, Pfadenhauer L, Burns J, Brereton L, Gerhardus A, Booth A, et al. Series: clinical epidemiology in South Africa. Paper 3: logic models help make sense of complexity in systematic reviews and health technology assessments. J Clin Epidemiol. 2017;83:37–47.
Waugh NR, Shyangdan D, Taylor-Phillips S, Suri G, Hall B. Screening for type 2 diabetes: a short report for the National Screening Committee. Health Technol Assess. 2013;17(35):1–90.
Amod A. The 2017 SEMDSA guideline for the management of type 2 diabetes. JEMDSA. 2017;22(1):S1–S196.
Selph S, Dana T, Blazina I, Bougatsos C, Patel H, Chou R. Screening for type 2 diabetes mellitus: a systematic review for the U.S. Preventive Services Task Force. Ann Intern Med. 2015;162(11):765–76.
Peer N, Balakrishna Y, Durao S. Screening for type 2 diabetes mellitus. Cochrane Database Syst Rev. 2020;5:CD005266.
PubMed Google Scholar
Simmons RK, Echouffo-Tcheugui JB, Sharp SJ, Sargeant LA, Williams KM, Prevost AT, et al. Screening for type 2 diabetes and population mortality over 10 years (ADDITION-Cambridge): a cluster-randomised controlled trial. Lancet. 2012;380(9855):1741–8.
Moher D, Shamseer L, Clarke M, Ghersi D, Liberati A, Petticrew M, et al. Preferred reporting items for systematic review and meta-analysis protocols (PRISMA-P) 2015 statement. Syst Rev. 2015;4:1.
Saquib N, Saquib J, Ioannidis JP. Does screening for disease save lives in asymptomatic adults? Systematic review of meta-analyses and randomized trials. Int J Epidemiol. 2015;44(1):264–77.
Durao S, Ajumobi O, Kredo T, Naude C, Levitt NS, Steyn K, et al. Evidence insufficient to confirm the value of population screening for diabetes and hypertension in low- and-middle-income settings. S Afr Med J. 2015;105(2):98–102.
Cochrane Effective Practice and Organisation of Care (EPOC). EPOC review and what should they be called? EPOC resources for review authors. 2017.
Higgins JPT, Thomas J, Chandler J, Cumpston M, Li T, Page MJ, et al. Cochrane handbook for systematic reviews of interventions 2019 July 2019.
Horsley T, Dingwall O, Sampson M. Checking reference lists to find additional studies for systematic reviews. Cochrane Database Syst Rev. 2011;8:MR000026.
Ouzzani M, Hammady H, Fedorowicz Z, Elmagarmid A. Rayyan-a web and mobile app for systematic reviews. Syst Rev. 2016;5(1):210.
Liberati A, Altman DG, Tetzlaff J, Mulrow C, Gotzsche PC, Ioannidis JP, et al. The PRISMA statement for reporting systematic reviews and meta-analyses of studies that evaluate health care interventions: explanation and elaboration. PLoS Med. 2009;6(7):e1000100.
Cochrane Effective Practice and Organisation of Care (EPOC). Data collection form. 2013.
Hoffmann TC, Glasziou PP, Boutron I, Milne R, Perera R, Moher D, et al. Better reporting of interventions: template for intervention description and replication (TIDieR) checklist and guide. BMJ : British Medical Journal. 2014;348:g1687.
Sterne JA, Hernan MA, Reeves BC, Savovic J, Berkman ND, Viswanathan M, et al. ROBINS-I: a tool for assessing risk of bias in non-randomised studies of interventions. BMJ. 2016;355:i4919.
Cochrane Effective Practice and Organisation of Care (EPOC). Interrupted time series (ITS) analyses. 2017.
Cochrane Effective Practice and Organisation of Care (EPOC). Analysis in EPOC reviews. 2017.
Sterne JA, Sutton AJ, Ioannidis JP, Terrin N, Jones DR, Lau J, et al. Recommendations for examining and interpreting funnel plot asymmetry in meta-analyses of randomised controlled trials. BMJ. 2011;343:d4002.
Guyatt GH, Oxman AD, Vist GE, Kunz R, Falck-Ytter Y, Alonso-Coello P, et al. GRADE: an emerging consensus on rating quality of evidence and strength of recommendations. BMJ. 2008;336(7650):924–6.
McMaster University. GRADEpro GDT. In: Group HOGW, editor. 2014.
Cochrane Effective Practice and Organisation of Care (EPOC). EPOC worksheets for preparing a 'Summary of findings' table using GRADE. 2013.
Download references
Acknowledgements
The authors would like to thank the following people who have supported this protocol:
• The authors of an unpublished Cochrane systematic review titled “Screening for type 2 diabetes mellitus”: Nasheeta Peer and Solange Durao, South African Medical Research Council, for providing their protocol to form the basis of this protocol, as well as a summary of their findings to form the rationale of the protocol and a quote to summarise the findings of their unpublished review.
• Information specialist Maria-Inti Metzendorf, Cochrane Metabolic and Endocrine Disorders Group, provided search strategy for the unpublished Cochrane review by Peer and Durao upon which the current search strategy is based.
• CEBHA+ Methodological Support Group Jake Burns and Peter Philipsborn, Ludwig-Maximillians Universitat Munchen, are internal reviewers for CEBHA+ which funds this review and assisted by providing critical review of the draft protocol.
• Prof Naomi Levitt, Chronic Disease for Africa, UCT, is a clinical Endocrinologist/Diabetologist who contributed to the PICO of this review when conceptualising the project and gave critical feedback to assist with responding to reviewers comments on the protocol before resubmission.
The work reported herein was made possible through Cochrane South Africa, South African Medical Research Council under the Collaboration for Evidence-Based Healthcare and Public Health in Africa (CEBHA+) Scholarship Programme. CEBHA+ receives funding from the Federal Ministry for Education and Research (Bundesministerium fur Bildung und Forschung, BMBF), Germany, through the BMBF funding of Research Networks for Health Innovation in Sub-Saharan Africa. The content hereof is the sole responsibility of the authors and does not necessarily represent the official views of SAMRC or the funders. The funding body, the Federal Ministry for Education and Research (Bundesministerium fur Bildung und Forschung, BMBF), Germany, played no role in the design and writing of the protocol; however, members of the Collaboration for Evidence-Based Healthcare and Public Health in Africa (CEBHA+) provided critical review and expert advice on the penultimate version of the protocol.
Author information
Authors and affiliations.
Vaccines for Africa Initiative, University of Cape Town, Cape Town, South Africa
Helen Mearns, Paul Kuodi Otiku & Benjamin M. Kagina
School of Public Health & Family Medicine, University of Cape Town, Cape Town, South Africa
Health Sciences Library, University of Cape Town, Cape Town, South Africa
Mary Shelton
Cochrane South Africa, South African Medical Research Council, Cape Town, South Africa
Tamara Kredo & Bey-Marrié Schmidt
Division of Clinical Pharmacology, Department of Medicine, Faculty of Medicine and Health Sciences, University of Stellenbosch, Cape Town, South Africa
Tamara Kredo
You can also search for this author in PubMed Google Scholar
Contributions
Conceiving the protocol: BS, TK. Designing the protocol: HM, BS. Co-ordinating the protocol: HM. Designing search strategies: MS, HM. Writing the protocol: HM. Providing general advice on the protocol and approving the final version: PKO, MS, BK, TK, BS. Securing funding for the protocol: BS, TK. Performing previous work that was the foundation of the current study: NP, SD. Guarantor of the review: BS. The authors read and approved the final manuscript.
Corresponding author
Correspondence to Helen Mearns .
Ethics declarations
Ethics approval and consent to participate.
Ethics approval is not required for a systematic review of secondary data.
Consent for publication
Competing interests.
The authors declare that they have no competing interests.
HM: None known
PKO: None known
MS: None known
BMK: None known
TK: None known
BS: None known
Additional information
Publisher’s note.
Springer Nature remains neutral with regard to jurisdictional claims in published maps and institutional affiliations.
Supplementary information
Additional file 1..
PRISMA Checklist.
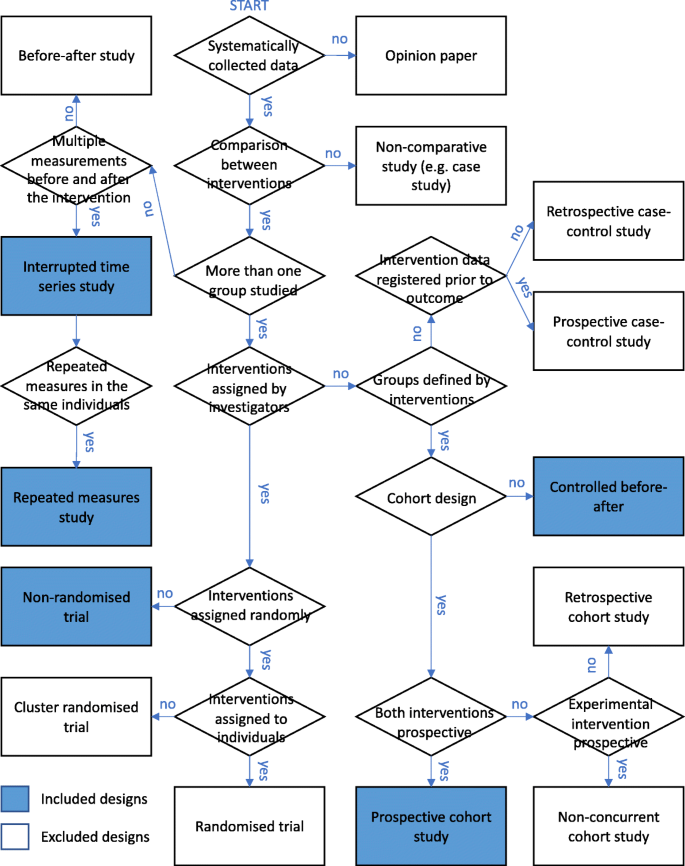
Flow diagram to assist with identifying the type of study (modified from [ 34 ])
Search Strategy for PubMed:
Set 1: Diabetes Mellitus, Type 2 [MeSH] OR [Text Word field:] Adult onset diabetes OR late onset diabetes OR latent diabetes OR mature onset diabetes OR MODY OR NIDDM OR noninsulin-dependent diabetes OR slow onset diabetes OR stable onset diabetes OR type 2 diabetes OR type II diabetes OR T2DM OR T2D
Set 2: Diabetes Insipidus [MeSH] OR [Text Word field:] diabetes insipidus
Set 3 : 1 NOT 2
Set 4: Mass screening [MeSH] OR [Text Word field:] screening
Set 5: 3 AND 4
Set 6: Animals [MeSH] NOT Humans [MeSH]
Set 7: 5 NOT 6
Set 8: [All fields:] Trial OR trials OR before-and-after study OR before-and-after studies OR cohort OR comparative study OR comparative studies OR Controlled OR evaluation study OR evaluation studies OR follow-up study OR follow-up studies OR interrupted time series OR longitudinal study OR longitudinal studies OR non-randomised OR non-randomized OR nonrandomised OR nonrandomized OR non randomised OR non randomized OR program evaluation OR programme evaluation OR prospective study OR prospective studies OR quantitative study OR quantitative studies OR quasi experimental OR repeated measures
Set 9: 7 AND 8
Rights and permissions
Open Access This article is licensed under a Creative Commons Attribution 4.0 International License, which permits use, sharing, adaptation, distribution and reproduction in any medium or format, as long as you give appropriate credit to the original author(s) and the source, provide a link to the Creative Commons licence, and indicate if changes were made. The images or other third party material in this article are included in the article's Creative Commons licence, unless indicated otherwise in a credit line to the material. If material is not included in the article's Creative Commons licence and your intended use is not permitted by statutory regulation or exceeds the permitted use, you will need to obtain permission directly from the copyright holder. To view a copy of this licence, visit http://creativecommons.org/licenses/by/4.0/ . The Creative Commons Public Domain Dedication waiver ( http://creativecommons.org/publicdomain/zero/1.0/ ) applies to the data made available in this article, unless otherwise stated in a credit line to the data.
Reprints and permissions
About this article
Cite this article.
Mearns, H., Otiku, P.K., Shelton, M. et al. Screening strategies for adults with type 2 diabetes mellitus: a systematic review protocol. Syst Rev 9 , 156 (2020). https://doi.org/10.1186/s13643-020-01417-3
Download citation
Received : 21 December 2019
Accepted : 30 June 2020
Published : 13 July 2020
DOI : https://doi.org/10.1186/s13643-020-01417-3
Share this article
Anyone you share the following link with will be able to read this content:
Sorry, a shareable link is not currently available for this article.
Provided by the Springer Nature SharedIt content-sharing initiative
- Mass screening
- Opportunistic
- Type 2 diabetes mellitus
Systematic Reviews
ISSN: 2046-4053
- Submission enquiries: Access here and click Contact Us
- General enquiries: [email protected]
- Open access
- Published: 08 June 2018
Prevalence of cardiovascular disease in type 2 diabetes: a systematic literature review of scientific evidence from across the world in 2007–2017
- Thomas R. Einarson 1 ,
- Annabel Acs 2 ,
- Craig Ludwig ORCID: orcid.org/0000-0002-4901-576X 2 &
- Ulrik H. Panton 3
Cardiovascular Diabetology volume 17 , Article number: 83 ( 2018 ) Cite this article
99k Accesses
1144 Citations
80 Altmetric
Metrics details
Cardiovascular disease (CVD) is a common comorbidity in type 2 diabetes (T2DM). CVD’s prevalence has been growing over time.
To estimate the current prevalence of CVD among adults with T2DM by reviewing literature published within the last 10 years (2007–March 2017).
We searched Medline, Embase, and proceedings of major scientific meetings for original research documenting the prevalence of CVD in T2DM. CVD included stroke, myocardial infarction, angina pectoris, heart failure, ischemic heart disease, cardiovascular disease, coronary heart disease, atherosclerosis, and cardiovascular death. No restrictions were placed on country of origin or publication language. Two reviewers independently searched for articles and extracted data, adjudicating results through consensus. Data were summarized descriptively. Risk of bias was examined by applying the STROBE checklist.
We analyzed data from 57 articles with 4,549,481 persons having T2DM. Europe produced the most articles (46%), followed by the Western Pacific/China (21%), and North America (13%). Overall in 4,549,481 persons with T2DM, 52.0% were male, 47.0% were obese, aged 63.6 ± 6.9 years old, with T2DM duration of 10.4 ± 3.7 years. CVD affected 32.2% overall (53 studies, N = 4,289,140); 29.1% had atherosclerosis (4 studies, N = 1153), 21.2% had coronary heart disease (42 articles, N = 3,833,200), 14.9% heart failure (14 studies, N = 601,154), 14.6% angina (4 studies, N = 354,743), 10.0% myocardial infarction (13 studies, N = 3,518,833) and 7.6% stroke (39 studies, N = 3,901,505). CVD was the cause of death in 9.9% of T2DM patients (representing 50.3% of all deaths). Risk of bias was low; 80 ± 12% of STROBE checklist items were adequately addressed.
Conclusions
Globally, overall CVD affects approximately 32.2% of all persons with T2DM. CVD is a major cause of mortality among people with T2DM, accounting for approximately half of all deaths over the study period. Coronary artery disease and stroke were the major contributors.
The International Diabetes Federation (IDF) estimates that worldwide, 415 million people have diabetes, 91% of whom have type 2 diabetes mellitus (T2DM) [ 1 ]. People with diabetes comprise 8.8% of the world’s population, and IDF predicts that the number of cases of diabetes will rise to 642 million by 2040 [ 1 ]. The prevalence of T2DM has been steadily increasing over time. Using data from the Framingham Heart Study, Abraham et al. [ 2 ] noted that the overall annualized incidence rates of the disease per 1000 persons increased from 3.0 in the 1970s to 5.5 in the first decade of the 2000s. That change represented an increase in the incidence of T2DM of 83.3% and was higher in males than females by a factor of 1.61.
Cardiovascular disease (CVD) is a major cause of death and disability among people with diabetes [ 1 , 3 ]. Adults with diabetes historically have a higher prevalence rate of CVD than adults without diabetes [ 4 ], and the risk of CVD increases continuously with rising fasting plasma glucose levels, even before reaching levels sufficient for a diabetes diagnosis [ 5 ].
T2DM reduces life expectancy by as much as 10 years, and the main cause of death for patients with T2DM is CVD [ 1 , 3 ]. Furthermore, people with T2DM are disproportionately affected by CVD compared with non-diabetic subjects [ 6 ]. Haffner et al. [ 6 ] reported death rates due to cardiovascular causes over a 7-year period in patients with and without T2DM. In persons with T2DM, the death rates were 15.4% for those with no prior myocardial infarction (MI) and 42.0% in patients having a history of MI. In contrast, patients who did not have T2DM, the death rates due to cardiovascular causes were 2.1 and 15.9%, respectively.
In the Framingham Heart Study, Fox [ 7 ] reported that, along with the increasing T2DM prevalence, the attributable risk of CVD due to T2DM increased from 5.4% in the period 1952–1974 to 8.7% in the period 1975 and 1998. In a longitudinal study of 881 patients with T2DM over 10 years, van Hateren et al. [ 8 ] indicated that the hazard ratio for death due to CVD was constantly increasing each year. Thus, an increasing burden of diabetes will likely be followed by an increasing burden of CVD.
Given the clinical burden that CVD complications have on T2DM patients, there has been an increased focus on the joint management of T2DM and CVD. Good glycemic control remains the main foundation for managing T2DM. Although the importance of intensive glycemic control for protection against microvascular complications and CVD in people with T1DM is well established [ 9 , 10 ], its role for reducing cardiovascular risk has not been established as clearly in people with T2DM [ 11 , 12 , 13 ]. Hence, the most effective approach for prevention of macrovascular complications appears to be multifactorial risk factor reduction (glycemic control, smoking cessation, diet, exercise, aggressive blood pressure control, treatment of dyslipidemia).
As a result, diabetes treatment guidelines have been updated to provide guidance on how to prevent and manage the onset of CVD [ 14 ]. Furthermore, there is increasing pressure from regulatory agencies that antidiabetic treatments demonstrate cardiovascular safety and benefits, especially for major cardiovascular events such as cardiovascular mortality, non-fatal MI, and stroke [ 15 , 16 ]. Following these regulatory requirements, several cardiovascular outcomes trials (CVOT) have been completed, which demonstrate that certain anti-diabetic treatments are associated with a lower risk of CVD [ 17 , 18 , 19 , 20 ].
The increased focus on adequately treating patients with both CVD and T2DM requires that we have updated prevalence rates of CVD among patients with T2DM. This is especially needed to inform clinical and policy level decision-making by healthcare providers, healthcare policy decision-makers, and health economic analysts. Reviews have been published on the epidemiology of type 1 diabetes (T1DM), and CVD [ 21 ], pre-diabetes and the risk of CVD [ 22 ], or reviews have focused on specific countries [ 23 ]. However, there is no recent global review on the prevalence of CVD among adults with T2DM. Therefore, the objective of this systematic literature review was to quantitatively summarize rates of prevalence of CVD in adults with T2DM in studies published during the past 10 years.
Although CVD is an umbrella term that includes coronary artery disease (CAD), cerebrovascular disease (CBV), and peripheral vascular disease, the focus of this review was on CVD outcomes that are relevant to major cardiovascular events. Therefore, the review specifically focused on the prevalence of CAD and CBV. CAD has many synonyms, including ischemic heart disease, coronary heart disease (CHD), atherosclerotic heart disease, and atherosclerotic CVD. Conditions within this category are stable angina pectoris, unstable angina pectoris, MI (also known as heart attack), and sudden cardiac death (SCD). CBV comprises mainly stroke (intracerebral hemorrhage, cerebral infarction, cerebral arterial disease), but also may include transient ischemic attacks.
This review was undertaken in adherence to the PRISMA Statement for systematic reviews [ 24 ].
Eligibility criteria
Criteria for eligibility were guided by the PICO reporting system (which describes the participants, interventions, comparisons, and outcome[s] of the systematic review), together with the specification of the type of study design (PICOS), from the Preferred Reporting Items for Systematic Reviews and Meta-Analyses (PRISMA) [ 24 ].
Participants
Included in this research were adult patients ≥ 18 years old who had been diagnosed with T2DM.
Interventions
Not applicable in this research.
Comparisons
Prevalence rates of CVD between males and females, and between obese and non-obese patients were compared. It was acknowledged that, in the literature, authors often used different terms or combinations of terms to describe their patients. The aim was to be all-inclusive in order to capture all relevant patient populations. Broad definitions of acceptable diseases were CVD, CAD, CHD, ischemic heart disease (IHD), congestive heart failure (CHF), or CBV. Specific conditions of interest included stroke, MI/heart attack, angina pectoris, heart failure, and atherosclerosis as well as cardiovascular or cardiac death.
Excluded were other forms of CVD including peripheral artery disease (PAD), rheumatic heart disease, cardiac dysrhythmias (e.g., atrial or ventricular fibrillation), or requirement for surgery such as coronary artery bypass grafting (CABG)/coronary revascularization. Also excluded were intermediate states such as hypertension or metabolic syndrome or studies of carotid intima-media thickness (CIMT).
The outcome of interest was the prevalence of each of these diseases/outcomes, then aggregated by continent/IDF Region, by country, and by the country’s economic status.
Study design
The primary focus was on prevalence studies and cross-sectional surveys, including database studies or patient chart reviews. Incidence studies were accepted only if they provided population-based baseline and follow-up data. Included were peer-reviewed studies published in any language. Both published articles and abstracts from scientific meetings were eligible. However, any published studies from clinical trial programs or individual pharmaceutical products were excluded.
Information sources and search strategy
The search was undertaken between February 15 and March 6, 2017. Databases searched included Medline and Embase between January 2007 and March 2017. In addition, PubMed was searched from 2014 to identify articles that were “ahead of print” yet fully available. Evidence presented at selected conferences during the last 5 years were accessed, including the Annual Meetings of the International Society Pharmacoeconomic Outcomes and Research (ISPOR), American Diabetes Association (ADA), European Association for the Study of Diabetes (EASD) and American Association of Clinical Endocrinologists (AACE). Keywords linked to MeSH terms specific to each database were used in the search including prevalence, OR epidemiology, AND acute coronary syndrome, OR cardiovascular disease, OR cardiovascular death, OR non-fatal myocardial infarction, OR non-fatal stroke, OR obesity AND type 2 diabetes mellitus. Other keywords were cerebrovascular disease, cerebral arterial disease, intracerebral hemorrhage, cerebral infarction, coronary artery disease, ischemic heart disease, atherosclerotic heart disease, coronary heart disease, angina pectoris. Identified articles and previous reviews were hand searched for articles that may have included data useful to this search.
Article identification and selection
Two reviewers independently searched Medline, Embase and the proceedings of major scientific meetings for suitable papers. Results were compared and adjudicated through consensus discussion. A third reviewer checked all results for quality assurance.
Data collection
Data extracted from articles included information concerning the publication, the patients involved, and outcomes of interest. Publication items included the first author, year of publication, the country in which the data were collected, and date of data collection. Patient data collected included the number of patients screened, percentages of males and females, average age, duration of T2DM, the proportion with obesity (or average body mass index (BMI) ± SD). Outcome data consisted of the numbers and percentages of patients having each cardiovascular outcome, overall and separately for males and females, where available. The same procedure (two independent reviewers plus a third judge) was followed for data collection as for article selection.
Data analysis
Data were analyzed descriptively, with sums, averages, and medians, and ranges reported. The primary outcome was the estimate of prevalence rates of CVD in patients with T2DM. No overall quantitative synthesis was undertaken. Weighted averages were calculated for individual countries and IDF regions. For patient characteristics, we calculated simple averages and medians across studies. Due to a single study with a sample size of more than three million people, which skewed the data, we calculated weighted averages for patient characteristics with and without that study. It should be noted that averages were based on the studies that reported the outcome, which may then represent a subgroup of the entire pool of studies.
The risk of bias was explored by applying the checklist from the STROBE initiative [ 25 ]. They have produced a validated checklist of items that should be addressed in reports of observational studies. There are 22 main items, each of which addresses an issue of research design and is presented in a list of recommendations. Items are scored as dichotomously as acceptable or not acceptable.
Included studies
The flowchart in Fig. 1 depicts the article selection process. We initially identified 1539 papers that appeared to be suitable, but after examining them systematically, 57 studies were accepted. Three articles each presented two different sets of results [ 26 , 27 , 28 ]; therefore, there are 60 sets of analyses within these 57 articles. Table 1 lists these studies along with their descriptive variables. There were 51 full articles and six abstracts presented at scientific meetings. Data collectively represent more than 4.5 million people with T2DM from around the globe.
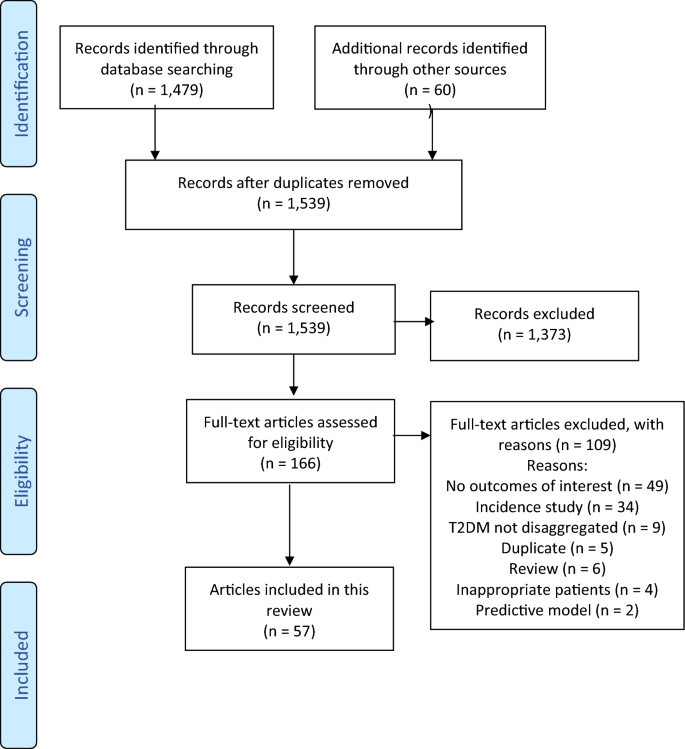
Flowchart of article selection. The flowchart depicts the article selection process
In Table 2 , results are presented geographically according to the classification system used by the IDF [ 1 ]. Studies from 25 countries were represented in this review: Australia, Belgium, Brazil, Cameroon, China, France, India, Indonesia, Iraq, Ireland, Italy, Korea, Mexico, Netherlands, Portugal, Qatar, Russian Federation, Saudi Arabia, Scotland, Spain, Sweden, Switzerland, Thailand, UK and USA. Details are provided in Table 3 . Three areas were responsible for generating 80% of the studies. Europe produced most articles (46%), followed by the Western Pacific/China (21%), and North America (13%). The other 20% were from the rest of the world. There were no discernible patterns differentiating prevalence rates between countries, based on income status. Part of the problem is that there are few studies in low- and middle-income countries and none from those in the lowest income level.
Patient characteristics
In the 57 individual studies, data from 4.5 million people with T2DM were presented with nearly 3.1 million people coming from a single Russian study by Shestakova [ 29 ]. As presented in Table 1 , using a simple average across studies, the average age was 63.6 ± 6.9 (median = 64.3 years; weighted average = 66.3 ± 6.9 years). The weighted average proportion of persons with obesity was 46.3 ± 15.0%, with a simple average of 47.0 ± 14.7% (median = 45.4%), defined as a BMI ≥ 30 kg/m 2 . The mean percentage of males across the studies was 50.5 ± 10.3% (median = 52.0%); the weighted average of the proportion of males was 36.0 ± 10.0%, including the study by Shestakova [ 29 ], and 54.1 ± 9.9% excluding that study. The patients had T2DM for an average duration of 10.4 ± 3.7 years (median = 10.0 years; weighted average = 6.6 ± 3.7 years). Among the 23 studies that reported duration of follow-up, the average was 7.3% ± 4.5 years (median = 6.0 years; weighted average = 5.2 ± 4.3 years).
Prevalence rates of cardiovascular comorbidities are summarized in Table 4 for all patients as well as separately for males and females. In studies reporting gender-specific prevalence rates, males had higher prevalence rates than females for all outcomes except overall CVD, where both sexes had an overall prevalence rate of approximately 27%. Overall, in studies that presented prevalence rates for males and females combined, the prevalence of CVD among persons with T2DM was 32.2%. CAD and atherosclerosis were the most prevalent CVD comorbidities, with prevalence rates of 21.2 and 29.1%, respectively, whereas stroke was the least prevalent with a prevalence rate of 7.6%. It is unclear why people with T2DM have different susceptibilities to these diseases. An explanation for the high prevalence rate for atherosclerosis could be that it is an artifact of patient selection. In the studies that examined atherosclerosis, most patients were enrolled if they had high-risk scores for atherosclerosis, resulting in a very high rate of disease detection.
CVD mortality among patients with T2DM
Table 5 presents the data regarding the rates of mortality associated with CVD in persons with T2DM. The weighted average of death rates from the eight studies with 3,208,557 patients with T2DM was 9.9% (95% CI 8.6–11.3%) [ 29 , 30 , 31 , 32 , 33 , 34 , 35 , 36 ]. There were 6.3% who died due to CAD and another 1.5% from CBV. Comparing patients with both T2DM and CVD with patients having neither T2DM nor CVD, the odds ratio for death was 4.56 (95% CI 3.53–5.89) [ 32 ]. Using a weighted average from seven studies (N = 86,557) [ 29 , 30 , 31 , 32 , 33 , 34 , 35 ], the calculated deaths due to CVD comprised 50.3% (95% CI 37.0–63.7%) of all deaths in patients with T2DM. The major contributors were CAD, which was responsible for 29.7% (95% CI 25.1–34.4%) and stroke/CBV for 11.0% (95% CI 8.8–13.3%).
CVD among obese vs. non-obese people with T2DM
About half of the patients included in this analysis had obesity. Three-quarters of the included studies reported on patients’ BMI or the percent of patients with obesity. While the definitions and BMI cut-off points of obesity varied across studies, the most commonly used definition of obesity was a BMI ≥ 30 kg/m 2 , which was employed by 16 studies (43% of those providing a definition).
Five papers reported prevalence rates of CVD according to obesity status, and all of them found a positive relationship between obesity and increased prevalence rates of CVD [ 26 , 37 , 38 , 39 , 40 ]. Using logistic regression to control for multiple factors, Bhatti et al. [ 26 ] found a positive correlation between obesity and CAD (P = 0.021). Tamba et al. [ 37 ] reported positive correlations between obesity and both CAD (r = 0.3, P < 0.001) and stroke (r = 0.5, P < 0.001). Boonman-de Winter et al. [ 38 ] quantified the relationship between BMI and heart failure. The prevalence rate of heart failure was 38.7% (95% CI 31.2–46.1%) in patients with a BMI ≥ 30 kg/m 2 and 23.4% (95% CI 19.4–27.5%) in those with a BMI < 30 kg/m 2 , which represents a 65% increase due to obesity.
Two studies explored the relationship between increasing BMI and risk of CVD [ 39 , 40 ]. According to Wentworth et al. [ 39 ], for CAD in both males and females, the prevalence rate of CAD increased with each successive increase in BMI, with a five-fold increase between the lowest and highest categories [< 25 (normal), 25–30 kg/m 2 (overweight), 30–35 kg/m 2 (mild obesity), 35–40 kg/m 2 (moderate obesity) and > 40 kg/m 2 (severe obesity)]. The difference was that prevalence rates in males were about double those for females in every BMI category. For the outcome stroke/transient ischemic attack (TIA) in males, only the highest category (BMI > 40) had elevated prevalence rates, which were about double those for the lowest category (BMI < 25). For females, prevalence rates of stroke/TIA increased in those who were overweight and had mild or moderate obesity but decreased for those with severe obesity. Finally, Glogner et al. [ 40 ] had quite different results. They reported a steady increase in prevalence rates of MI from 6.86% in those with a BMI < 20–9.33% in patients who were overweight (BMI 25–30), a 36% increase. However, MI prevalence rates declined thereafter with each increasing category of obesity. The highest category (BMI ≥ 40) had a prevalence rate of 5.01%, which was 27% lower than those in the lowest category (BMI < 20). Thus, patterns vary quite widely, and studies often examined different outcomes.
Risk of bias in included studies
In the assessment of risk for bias, the studies addressed 80% of the STROBE checklist items (i.e., research design and data presentation), on average. The mean was 80 ± 12%, and the median was 81%, with a range of 54–100%. The two items that were addressed by 100% of the articles were reporting of outcome data and reporting of outcomes. The two items addressed the least were the statement of funding (56%) and indicating the study design with a commonly used term in the title or abstract (60%).
In this systematic review of 4,549,481 persons with T2DM, we estimated the overall prevalence of CVD at 32.2%. The most frequent type of CVD reported was CAD (21.2%) and lowest was stroke (7.6%). Males had higher rates of prevalent disease than females. CVD was responsible for 50.3% of all deaths in T2DM patients over the period of the review. Along with diabetes, cardiovascular disease is associated with several risk factors, obesity, and age. We, therefore, evaluated the association between age and obesity among patients with CVD and T2DM in the selected articles.
Age as a risk factor for CVD
Age is a well-known risk factor for CVD. Out of the 57 articles, thirteen (25%) reported on the relationship between age and CVD and the results were quite mixed. Nine studies identified a significant relationship between age and CVD [ 30 , 38 , 41 , 42 , 43 , 44 , 45 , 46 , 47 ], but only two presented results across multiple age categories [ 38 , 42 ]. Alonso-Moran [ 42 ] found that the odds ratio for IHD, stroke, heart failure and MI all increased sequentially with each increase in 5-year age category as compared with the age group 35–39 used as a reference. All of these individual outcomes achieved statistical significance (P < 0.001). Boonman-de Winter et al. [ 38 ] similarly reported a sequential increase in prevalence rates of heart failure for all patients in 5-year age categories from 60 to 64 to > 80 years of age. Other authors reported that older patients had higher prevalence rates than younger patients, but provided few details on age categories [ 30 , 41 , 43 , 45 , 46 ]. On the other hand, four studies reported no differences between age categories [ 26 , 34 , 48 , 49 ]. Three other studies used age as a covariate in a logistic regression with no further details [ 28 , 50 , 51 ]. Therefore, few studies have quantified the effect of age on CVD prevalence rates among people with T2DM.
Obesity as a risk factor for CVD
Obesity has long been established as an independent risk factor for CVD [ 7 , 52 ], and is associated with CAD [ 53 , 54 ], atherosclerosis [ 51 ], and cardiac death [ 55 , 56 ]. Furthermore, it has been shown that overweight and obesity are highly prevalent in T2DM patients with high CV risk and that BMI and waist circumference are related to major cardiometabolic risk factors such as hypertension and elevated low-density lipoprotein cholesterol (LDL-C) [ 57 ].
Obesity is usually defined by body mass index (BMI, calculated as body weight in kg divided by the square of height in meters), with the World Health Organization (WHO) classifying adults with a BMI 30 kg/m 2 as obese [ 58 ]. However, BMI as a measure to stratify patients with obesity has limitations and does not account for the wide variation in body fat distribution nor the quality of fat, and may not account for associated health risk in different individuals and populations [ 58 ]. This has been shown to be true for South Asian populations [ 59 ]. In a study from Raji et al. [ 60 ] noted that compared with Caucasians, Asian Indians had significantly greater total abdominal and visceral fat matched with Caucasians of the same age, gender, and BMI, meaning that this population has an increased CVD risk. Besides, there is a weaker association between increasing BMI and T2DM in Asian populations compared with Caucasians due to the risk for T2DM begins increasing at comparatively normal BMI in Asian populations [ 61 ].
Seven of the included studies evaluated the relationship between obesity and/or BMI and CVD risk. Five of the studies included in this review identified a positive relationship between obesity and increased prevalence rates of CVD [ 26 , 37 , 38 , 39 , 40 ]. One of these studies [ 26 ] used lower BMI cut-off points to account for Asian populations in accordance with WHO recommendations on BMI for Asian populations [ 62 ] and evaluated abdominal adiposity with waist circumference measurements to determine the prevalence of obesity. Overall, the studies found a positive relationship between increasing BMI and CVD; except in one study [ 39 ], where women with severe obesity had a reduced prevalence of stroke. While the authors do not explain the reduced prevalence of stroke/TIA, it may be explained by differences in vascular risk markers in men, such as pre-existing ischemic heart disease, age, and smoking [ 63 ]. Furthermore, the presence of gonadal steroids, most notably estrogen, may lend a protective effect against stroke/TIA in women and it has been shown that adiposity is associated with increased levels of estrogen [ 64 ].
Although obesity is identified as a risk factor for CVD, it is associated with a paradox in that mortality is lower in patients who are overweight or obese than in those whose BMI is normal or underweight [ 65 ]. Lee et al. [ 66 ] reported that obesity provided a survival benefit to patients with heart failure who did not have comorbid diabetes, but not in patients who did have concomitant diabetes. In contrast, a group led by Abi Khalil [ 67 ] examined a cohort of 2492 T2DM patients in seven countries in the Middle East, Gulf region, with acute heart failure. They reported that BMI was inversely correlated with the risk of mortality, with severe obesity associated with less mortality risk.
It is clear that the relationship between obesity and the risk of CVD and CVD-related deaths requires further exploration to identify these mechanisms and relationships.
CVD-related mortality in T2DM
In persons with T2DM, CVD is responsible for at least half of the mortality, as previously mentioned. Among the specific diseases within that term, CAD was most lethal, followed by stroke. Similar results have been demonstrated with other models. In an incidence-based study, Straka et al. [ 68 ] followed 29,863 patients (5501 with T2DM and 24,362 without T2DM) over a 1-year period. Four of the incident cardiovascular outcomes they reported were significantly higher in those with T2DM. Patients with T2DM had 10% greater risk of CAD, 53% of MI, 58% of stroke, and 112% increased risk of heart failure. Therefore, T2DM is a substantial risk factor for CVD and its consequences.
CVD prevalence rates across regions and countries
As this was a global review, studies from across the world were included. Given the variation in which diabetes and its macrovascular complications are treated and managed across countries and income levels, it is relevant to look at prevalence rates across regions and countries. However, almost half (46.0%) of the research was produced in Europe, and very little information was obtained from the less developed regions of the world such as Africa, Latin America, and the Asian subcontinent.
As shown in Table 2 , the regions with the highest prevalence of overall CVD were North America and Caribbean (46.0%; N = 4,327,503), Southeast Asia (42.5%, N = 537) and Western Pacific (including China) (33.6%; N = 44,062). Southeast Asia stands out with a higher prevalence of CAD (29.4%) compared with other regions. The prevalence of CAD in this region is driven by one study from India [ 69 ], which specifically investigated the pattern of CAD in 134 symptomatic T2DM patients in India. However, epidemiological studies on people of South Asian origin have shown an increased likelihood of developing CAD that is up to two times higher than in Caucasians [ 70 ]. The higher risk is due to both pathophysiological and life course-related risk factors [ 70 ].
The summaries across countries and regions provide an overview of the geographic spread of research but should be interpreted with caution given the limited number of studies for some of the regions and countries. Figure 2 illustrates the distribution of studies across regions and countries and clearly shows that few studies exist for several regions. For example, only one study from one country in the African region was identified and therefore should not be seen to represent findings for the region’s entire T2DM population.
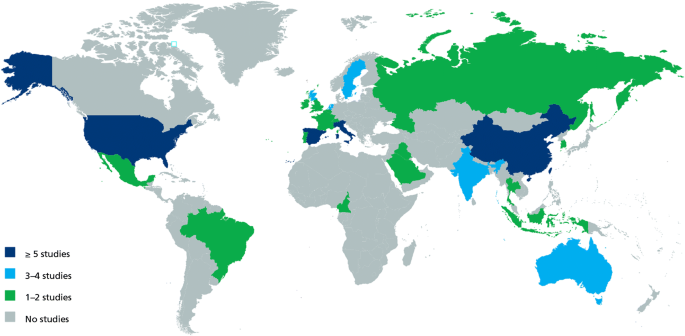
Distribution of studies across countries and regions. The figure illustrates the global distribution of studies across countries and regions
Treatment of both T2DM and CVD vary greatly between and within countries, and although much of the CVD risk in T2DM can be associated with the long-term complications of T2DM, there has been growing interest to determine whether certain antidiabetic drugs influence this risk. For example, sulfonylureas which are the second most commonly used antidiabetic drug after metformin, have been shown to be associated with an increased risk of cardiovascular events and mortality [ 71 ]. Newer antidiabetic drugs have been shown to lower the risk of CVD in T2DM patients [ 17 , 18 , 19 , 20 ]; however, these drugs are often intended to be used as second- to third-line treatments and many years may pass before patients can benefit.
Temporal trends in CVD risk assessment and management in T2DM
Encouragingly, CVD mortality is declining in high-income countries among the general population due to reductions in cardiovascular risk factors as well as to recent advances in prevention, treatment, and management [ 72 ]. This trend has also been observed in people with T2DM in some countries. Jung et al. [ 73 ] estimated trends in CVD in people with and without T2DM in South Korea using data from the national health insurance system. The results show a significant reduction in CVD risk among people with T2DM brought on by improvements in the care and management of patients. However, in many developing countries where the burden of T2DM is rapidly rising and lifestyle patterns changing an increase in CV risk factors among people with T2DM can be expected [ 3 ]. A study from China analyzed the relationship between lifestyle behaviors and multiple CV risk factors in 25,454 people with T2DM [ 74 ]. The researchers found that unhealthy lifestyles were common, especially among those who are non-elderly, and above-college educated. Furthermore, it was found that an unhealthy lifestyle was associated with poor blood, blood lipid, and blood pressure control. Decreasing the impact of T2DM and CVD in developing countries will require interventions aimed at changing risky lifestyle behaviors.
Screening people with T2DM for CV risk is an important strategy for reducing mortality and CVD events. A study from Denmark [ 75 ] found that a single round of diabetes screening and cardiovascular risk assessment in middle-aged adults in general practice was associated with a significant reduction in risk of all-cause mortality and CVD events in people with T2DM. The same researchers found that population-based stepwise screening for T2DM and CVD among all middle-aged adults was not associated with a reduction in mortality or CV events. Therefore, the benefits of population-based screening are limited in this context [ 76 ]. Kesall et al. [ 77 ] found that targeting specific occupational and industry groups with health checks could help identify individuals at high risk of both T2DM and CVD. In a study of 500,000 members of the Australian working population, they found that high T2DM and CVD risk was increased significantly in many occupational groups and industries.
Recent research points to an increasingly better understanding of the markers for identifying high CVD risk in people with T2DM. Li et al. [ 78 ] found that the combined application of carotid and lower extremity ultrasonography may be helpful to identify patients with T2DM who have a higher CVD risk. In a study of 2830 hospitalized patients with T2DM, they found that the concomitant presence of carotid and lower extremity atherosclerosis further increases the risk of CVD in patients with T2DM, compared with those who had either carotid or lower limb atherosclerosis and those without atherosclerosis. A study by Mohammedi et al. [ 79 ] found that major peripheral arterial disease (PAD) presenting as lower-extremity ulceration or amputation and peripheral revascularization is associated with increased risk of death and CV events in people with T2DM. The researchers conclude that screening for PAD along with active management are crucial for prevention of CVD in people with T2DM. In addition, coronary artery calcium (CAC) assessments have been found to significantly improve the risk classification for CHD and atherosclerotic CVD events in people with T2DM—regardless of the duration of diabetes [ 80 ]. Thus, a CAC assessment can be a useful tool for classifying people with T2DM into lower- or higher-risk groups for long-term CVD risk.
Lipid profile has long been considered among the most important risk factors for CVD in T2DM, and several trials have confirmed that lowering low-density lipoprotein cholesterol (LDL-C) via statins in T2DM was effective in reducing the risk of CVD [ 81 , 82 ]. It is also well known that statins also have a triglyceride-lowering effect [ 83 ]. In a cross-sectional study of 223,612 patients with T2DM in China, researchers found that although lower triglyceride was associated with reduced CVD risk in the short-term, it was associated with increased risk in the long-term [ 84 ]. This paradox could mean that low triglyceride is not necessarily associated with good clinical outcomes in all people with T2DM and that there are subgroup associations with CVD in patients with different durations of T2DM. Furthermore, Clua-Espuny et al. [ 85 ] suggest that the relative importance of risk factors wanes in complex chronic patients with T2DM with advancing age. In a cohort study of almost 3500 complex chronic patients above the age of 80 of whom 53% had diabetes and a high prevalence of associated classical risk factors, the researchers found that all-cause mortality was more affected by aging factors than by specific complications of diabetes. The authors make the recommendation that, for these patients, the care strategy may need to be redefined and adapted to comorbidities and functional autonomy rather than being focused on treatment outcomes.
Limitations
As with all literature reviews, we were limited by the availability of the literature and the validity and quality of the articles. Some of the results appeared only in abstract form, and many were not subsequently published as full articles within the time horizon of this review. Abstracts had space limitations, restricting the amount of information they could present. As well, we noted that there was often incomplete reporting or selective reporting of specific outcomes of interest.
Furthermore, the findings of this literature review are limited to a select patient population. Specifically, in this research, we accepted only data from adults aged 18 or older. Therefore, our results may not apply to children or adolescents. As well, we dealt only with T2DM; therefore, outcomes may not apply to T1DM or secondary diabetes such as that associated with hemochromatosis or pancreatitis.
This study was also challenged by the fact that CVD and its associated conditions are described differently across the literature. For example, CHD was used interchangeably with CAD or ischemic heart disease. We made every effort to standardize definitions and to group like with like. Furthermore, the types of CVD conditions evaluated varied across articles. Some articles focused on a single outcome, whereas others focused on several outcomes. As a result, the calculated prevalence rates may represent underestimates, as not all studies reported all outcomes.
The types of studies included would have also impacted the overall results of this study. First, we analyzed only prevalence studies; incidence studies would have different results due to their different perspective. Second, the studies varied both in the method of data collection (e.g., national databases versus clinic records) and the length of time over which they collected data. It is plausible that time-period over which studies were conducted could have impacted the observed prevalence rate of CVD. For example, health status, lifestyle, and treatments have varied over time, which could impact the prevalence rates in the studies using older data.
Overall, it is possible that the prevalence estimates for CVD presented in this article overestimate the prevalence of CVD among patients with T2DM. First, studies in the medical literature tend to include a sicker population compared to the general T2DM population; therefore, due to self-selection bias, the sample may not be representative of the broader T2DM population and thus lead to an overestimate of the prevalence of CVD. Second, some of the studies included T2DM patients with an existing CVD diagnosis; therefore, the overall estimate of CVD within these studies could be higher compared to the broader T2DM population.
Finally, only 25 countries were represented in this analysis. Noticeably absent were such countries as Germany, Canada, and Denmark, which all have excellent electronic health data, yet no research studies have been published from them. Very little has appeared from Africa, the Asian subcontinent or Latin America. More studies from these areas would be welcome. While the scope of this study was to evaluate evidence from peer-reviewed literature, an alternative approach to estimating the prevalence of CVD among patients with T2DM could be to analyze data within existing registries.
This is the first systematic review to synthesize global prevalence rates of CVD, including stroke, MI, angina, heart failure, atherosclerosis and CAD among people with T2DM. The results show that CVD is a major cause of comorbidity and death among patients with T2DM with CAD having the highest prevalence. There is a paucity of research studies investigating both the prevalence of CVD and risk factors such as obesity among people with T2DM. Given the large burden that CVD exerts on healthcare systems, patients and families around the world, more evidence is needed, ideally in the form of registry studies, to more accurately quantify the global prevalence of CVD among people with T2DM.
International Diabetes Federation. idf diabetes atlas. 7th ed. Brussels: International Diabetes Federation; 2015.
Google Scholar
Abraham TM, Pencina KM, Pencina MJ, Fox CS. Trends in diabetes incidence: the Framingham heart study. Diab Care. 2015;38:482–7.
Article Google Scholar
International Diabetes Federation. Diabetes and cardiovascular disease. Brussels: International Diabetes Federation; 2016. p. 1–144.
Sarwar N, Gao P, Seshasai SR, Gobin R, Kaptoge S, Angelantonio D, et al. Diabetes mellitus, fasting blood glucose concentration, and risk of vascular disease: a collaborative meta-analysis of 102 prospective studies. emerging risk factors collaboration. Lancet. 2010;375:2215–22.
Article PubMed CAS Google Scholar
Singh GM, Danaei G, Farzadfar F, Stevens GA, Woodward M, Wormser D, Kaptoge S, Whitlock G, Qiao Q, Lewington S. The age-specific quantitative effects of metabolic risk factors on cardiovascular diseases and diabetes: a pooled analysis. PLoS ONE. 2013;8:e65174.
Article PubMed PubMed Central CAS Google Scholar
Haffner SM, Lehto S, Rönnemaa T, Pyörälä K, Laakso M. Mortality from coronary heart disease in subjects with type 2 diabetes and in nondiabetic subjects with and without prior myocardial infarction. N Engl J Med. 1998;339:229–34.
Fox CS, Pencina MJ, Wilson PW, Paynter NP, Vasan RS, D’Agostino RB. Lifetime risk of cardiovascular disease among individuals with and without diabetes stratified by obesity status in the Framingham heart study. Diab Care. 2008;31:1582–4.
Van Hateren KJ, Landman GW, Kleefstra N, Logtenberg SJ, Groenier KH, Kamper AM, Houweling ST, Bilo HJ. The lipid profile and mortality risk in elderly type 2 diabetic patients: a 10-year follow-up study (ZODIAC-13). PLoS ONE. 2009;4:e8464.
The Diabetes Control and Complications Trial Research Group. The effect of intensive treatment of diabetes on the development and progression of long-term complications in insulin-dependent diabetes mellitus. N Engl J Med. 1993;329:977–86.
Nathan DM, Cleary PA, Backlund JY, Genuth SM, Lachin JM, Orchard TJ, Raskin P, Zinman B. Intensive diabetes treatment and cardiovascular disease in patients with type 1 diabetes. N Engl J Med. 2005;353:2643–53.
Article PubMed Google Scholar
ADVANCE Collaborative Group. Intensive blood glucose control and vascular outcomes in patients with type 2 diabetes. N Engl J Med. 2008;358:2560–72.
UK Prospective Diabetes Study. (UKPDS) Group: intensive blood-glucose control with sulphonylureas or insulin compared with conventional treatment and risk of complications in patients with type 2 diabetes (UKPDS 33). UK prospective diabetes study (UKPDS) group. Lancet. 1998;352:837–53.
Action to Control Cardiovascular Risk in Diabetes Study. Group: effects of intensive glucose lowering in type 2 diabetes. N Engl J Med. 2008;358:2545–59.
Rydén L, Grant PJ, Anker SD, Berne C, Cosentino F, Danchin N, Deaton C, Escaned J, Hammes H-P, Huikuri H. ESC guidelines on diabetes, pre-diabetes, and cardiovascular diseases developed in collaboration with the EASD-summary. Diab Vasc Dis Res. 2014;11:133–73.
Schnell O, Rydén L, Standl E, Ceriello A. Updates on cardiovascular outcome trials in diabetes. Cardiovasc Diabetol. 2017;16:128.
Article PubMed PubMed Central Google Scholar
Food and Drug Administration: Guidance for industry: diabetes mellitus—evaluating cardiovascular risk in new antidiabetic therapies to treat type 2 diabetes. US Department of Health and Human Services. 2008.
Marso SP, Bain SC, Consoli A, Eliaschewitz FG, Jódar E, Leiter LA, Lingvay I, Rosenstock J, Seufert J, Warren ML. Semaglutide and cardiovascular outcomes in patients with type 2 diabetes. N Engl J Med. 2016;375:1834–44.
Marso SP, Daniels GH, Brown-Frandsen K, Kristensen P, Mann JF, Nauck MA, Nissen SE, Pocock S, Poulter NR, Ravn LS. Liraglutide and cardiovascular outcomes in type 2 diabetes. N Engl J Med. 2016;2016:311–22.
Article CAS Google Scholar
Sanon VP, Patel S, Sanon S, Rodriguez R, Pham SV, Chilton R. Differential cardiovascular profiles of sodium-glucose cotransporter 2 inhibitors: critical evaluation of empagliflozin. Ther Clin Risk Manag. 2017;13:603.
Trujillo JM, Nuffer WA. Impact of sodium–glucose cotransporter 2 inhibitors on nonglycemic outcomes in patients with type 2 diabetes. Pharmacotherapy. 2017;37:481–91.
Schnell O, Cappuccio F, Genovese S, Standl E, Valensi P, Ceriello A. Type 1 diabetes and cardiovascular disease. Cardiovasc Diabetol. 2013;12:156.
Ford ES, Zhao G, Li C. Pre-diabetes and the risk for cardiovascular disease. J Am Coll Cardiol. 2010;55:1310–7.
Saquib N, Saquib J, Ahmed T, Khanam MA, Cullen MR. Cardiovascular diseases and type 2 diabetes in Bangladesh: a systematic review and meta-analysis of studies between 1995 and 2010. BMC Public Health. 2012;12:434.
Moher D, Liberati A, Tetzlaff J, Altman DG. Group. P: preferred reporting items for systematic reviews and meta-analyses: the PRISMA statement. Ann Intern Med. 2009;151:264–9.
von Elm E, Altman DG, Egger M, Pocock SJ, Gotzsche PC, Vandenbroucke JP, Initiative S. The strengthening the reporting of observational studies in epidemiology (STROBE) statement: guidelines for reporting observational studies. Int J Surg. 2014;12:1495–9.
Bhatti GK, Bhadada SK, Vijayvergiya R, Mastana SS, Bhatti JS. Metabolic syndrome and risk of major coronary events among the urban diabetic patients: North Indian Diabetes and cardiovascular disease study—NIDCVD-2. J Diab Complications. 2016;30:72–8.
Collier A, Ghosh S, Hair M, Waugh N. Impact of socioeconomic status and gender on glycaemic control, cardiovascular risk factors and diabetes complications in type 1 and 2 diabetes: a population based analysis from a Scottish region. Diab Metab. 2015;41:145–51.
Tan ED, Davis WA, Davis TM. Changes in characteristics and management of Asian and Anglo-Celts with type 2 diabetes over a 15-year period in an urban Australian community: the fremantle diabetes study. J Diab. 2016;8:139–47.
Shestakova M: Dynamics in prevalence of diabetes, diabetic complications and quality of diabetes care in Russian Federation in 2014–2015 by data of national diabetes register. In: Conference: 52nd annual meeting of the european association for the study of diabetes, EASD. 2016. p. 309.
Alwakeel JS, Sulimani R, Al-Asaad H, Al-Harbi A, Tarif N, Al-Suwaida A, Al-Mohaya S, Isnani AC, Alam A, Hammad D. Diabetes complications in 1952 type 2 diabetes mellitus patients managed in a single institution in Saudi Arabia. Ann Saudi Med. 2008;28:260–6.
Cardoso C, Salles G. Gross proteinuria is a strong risk predictor for cardiovascular mortality in Brazilian type 2 diabetic patients. Braz J Med Biol Res. 2008;41:674–80.
Carnethon MR, Biggs ML, Barzilay J, Kuller LH, Mozaffarian D, Mukamal K, Smith NL, Siscovick D. Diabetes and coronary heart disease as risk factors for mortality in older adults. Am J Med. 2010;123:556.
Eeg-Olofsson K, Cederholm J, Nilsson PM, Zethelius B, Svensson AM, Gudbjörnsdóttir S, Eliasson B. New aspects of HbA1c as a risk factor for cardiovascular diseases in type 2 diabetes: an observational study from the Swedish National Diabetes Register (NDR). J Intern Med. 2010;268:471–82.
Malik MO, Govan L, Petrie JR, Ghouri N, Leese G, Fischbacher C, Colhoun H, Philip S, Wild S, McCrimmon R, et al. Ethnicity and risk of cardiovascular disease (CVD): 4.8 year follow-up of patients with type 2 diabetes living in Scotland. Diabetologia. 2015;58:716–25.
Salinero-Fort MA, Andres-Rebollo FJ, Burgos-Lunar C, Abanades-Herranz JC, Carrillo-de-Santa-Pau E, Chico-Moraleja RM, Jimenez-Garcia R, Lopez-de-Andres A, Gomez-Campelo P. Cardiovascular and all-cause mortality in patients with type 2 diabetes mellitus in the MADIABETES Cohort Study: association with chronic kidney disease. J Diab Complications. 2016;30:227–36.
Menzaghi C, Xu M, Salvemini L, De Bonis C, Palladino G, Huang T, Copetti M, Zheng Y, Li Y, Fini G. Circulating adiponectin and cardiovascular mortality in patients with type 2 diabetes mellitus: evidence of sexual dimorphism. Cardiovasc Diabetol. 2014;13:130.
Tamba SM, Ewane ME, Bonny A, Muisi CN, Nana E, Ellong A, Mvogo CE, Mandengue SH. Micro and macrovascular complications of diabetes mellitus in Cameroon: risk factors and effect of diabetic check-up-a monocentric observational study. Pan African Med J. 2013;15:141.
Boonman-de Winter LJ, Rutten FH, Cramer MJ, Landman MJ, Liem AH, Rutten GE, Hoes AW. High prevalence of previously unknown heart failure and left ventricular dysfunction in patients with type 2 diabetes. Diabetologia. 2012;55:2154–62.
Wentworth JM, Fourlanos S, Colman PG. Body mass index correlates with ischemic heart disease and albuminuria in long-standing type 2 diabetes. Diab Res Clin Pract. 2012;97:57–62.
Glogner S, Rosengren A, Olsson M, Gudbjörnsdottir S, Svensson AM, Lind M. The association between BMI and hospitalization for heart failure in 83,021 persons with Type 2 diabetes: a population-based study from the Swedish National Diabetes Registry. Diab Med. 2014;31:586–94.
Alaboud AF, Tourkmani AM, Alharbi TJ, Alobikan AH, Abdelhay O, Al Batal SM, Alkashan HI, Mohammed UY. Microvascular and macrovascular complications of type 2 diabetic mellitus in Central, Kingdom of Saudi Arabia. Saudi Med J. 2016;37:1408–11.
Alonso-Moran E, Orueta JF, Fraile Esteban JI, Arteagoitia Axpe JM, Marques Gonzalez ML, Toro Polanco N, Ezkurra Loiola P, Gaztambide S, Nuno-Solinis R. The prevalence of diabetes-related complications and multimorbidity in the population with type 2 diabetes mellitus in the Basque Country. BMC Public Health. 1059;2014:14.
Carrasco-Sánchez FJ, Gomez-Huelgas R, Formiga F, Conde-Martel A, Trullàs JC, Bettencourt P, Arévalo-Lorido JC, Pérez-Barquero MM. Association between type-2 diabetes mellitus and post-discharge outcomes in heart failure patients: findings from the RICA registry. Diabetes Res Clin Pract. 2014;104:410–9.
Gregg EW, Cheng YJ, Saydah S, Cowie C, Garfield S, Geiss L, Barker L. Trends in death rates among US adults with and without diabetes between 1997 and 2006. Diab Care. 2012;35:1252–7.
Lin PJ, Cohen JT, Kent WA, Neumann PJ. Patterns of comorbidity clusters among adults with diabetes. Value Health. 2013;16:A155–6.
Song S, Hardisty C. Early onset type 2 diabetes mellitus: a harbinger for complications in later years—clinical observation from a secondary care cohort. QJM. 2009;102:799–806.
Yang HK, Kang B, Lee S-H, Yoon K-H, Hwang B-H, Chang K, Han K, Kang G, Cho JH. Association between hemoglobin A1c variability and subclinical coronary atherosclerosis in subjects with type 2 diabetes. J Diab Complications. 2015;29:776–82.
Mansour AA, Ajeel NA. Atherosclerotic cardiovascular disease among patients with type 2 diabetes in Basrah. World J Diab. 2013;4:82.
Menghua Z. GW25-e1447 clinical significance of multislice coronary CT angiography in asymptomatic patients with type 2 diabetes mellitus. J Am Coll Cardiol. 2014;64:C227.
Norhammar A, Bodegard J, Nystrom T, Thuresson M, Eriksson JW, Nathanson D. Incidence, prevalence and mortality of type 2 diabetes requiring glucose-lowering treatment, and associated risks of cardiovascular complications: a nationwide study in Sweden, 2006–2013. Diabetologia. 2016;59:1692–701.
Rossi MC, Lucisano G, Comaschi M, Coscelli C, Cucinotta D, Di Blasi P, Bader G, Pellegrini F, Valentini U, Vespasiani G. Quality of diabetes care predicts the development of cardiovascular events: results of the AMD-QUASAR study. Diab Care. 2011;34:347–52.
Hubert HB, Feinleib M, McNamara PM, Castelli WP. Obesity as an independent risk factor for cardiovascular disease: a 26-year follow-up of participants in the Framingham Heart study. Circulation. 1983;67:968–77.
Rabkin SW, Mathewson FA, Hsu PH. Relation of body weight to development of ischemic heart disease in a cohort of young North American men after a 26 year observation period: the Manitoba study. Am J Cardiol. 1977;39:452–8.
Garcia-Labbe D, Ruka E, Bertrand OF, Voisine P, Costerousse O, Poirier P. Obesity and coronary artery disease: evaluation and treatment. Can J Cardiol. 2015;31:184–94.
Plourde B, Sarrazin JF, Nault I, Poirier P. Sudden cardiac death and obesity. Exp Rev Cardiovasc Ther. 2014;12:1099–110.
Engeland A, Bjorge T, Sogaard AJ, Tverdal A. Body mass index in adolescence in relation to total mortality: 32-year follow-up of 227,000 Norwegian boys and girls. Am J Epidemiol. 2003;157:517–23.
Masmiquel L, Leiter L, Vidal J, Bain S, Petrie J, Franek E, Raz I, Comlekci A, Jacob S. Gaal Lv: LEADER 5: prevalence and cardiometabolic impact of obesity in cardiovascular high-risk patients with type 2 diabetes mellitus: baseline global data from the LEADER trial. Cardiovasc Diabetol. 2016;15:29.
World Health Organization. Obesity and Overweight. Factsheet No 311. Geneva: World Health Organization; 2017.
Gujral UP, Pradeepa R, Weber MB, Narayan KM, Mohan V. Type 2 diabetes in South Asians: similarities and differences with white Caucasian and other populations. Ann N Y Acad Sci. 2013;1281:51–63.
Raji A, Seely EW, Arky RA, Simonson DC. Body fat distribution and insulin resistance in healthy Asian Indians and Caucasians. J Clin Endocrinol Metab. 2001;86:5366–71.
Huxley R, James W, Barzi F, Patel J, Lear S, Suriyawongpaisal P, Janus E, Caterson I, Zimmet P, Prabhakaran D. Ethnic comparisons of the cross-sectional relationships between measures of body size with diabetes and hypertension. Obes Rev. 2008;9:53–61.
World Health Organization Expert Consultation. Appropriate body-mass index for Asian populations and its implications for policy and intervention strategies. Lancet. 2004;363:157.
Koellhoffer EC, McCullough LD. The effects of estrogen in ischemic stroke. Transl Stroke Res. 2013;4:390–401.
Meseguer A, Puche C, Cabero A. Sex steroid biosynthesis in white adipose tissue. Horm Metab Res. 2002;34:731–6.
Hartrumpf M, Kuehnel RU, Albes JM. The obesity paradox is still there: a risk analysis of over 15,000 cardiosurgical patients based on body mass index. Interact Cardiovasc Thorac Surg. 2017;25:18–24.
Lee KS, Moser DK, Lennie TA, Pelter MM, Nesbitt T, Southard JA, Dracup K. Obesity paradox: comparison of heart failure patients with and without comorbid diabetes. Am J Crit Care. 2017;26:140–8.
Abi Khalil C, Sulaiman K, Singh R, Jayyousi A, Asaad N, AlHabib KF, Alsheikh-Ali A, Al-Jarallah M, Bulbanat B, Al Mahmeed W, et al. BMI is inversely correlated to the risk of mortality in patients with type 2 diabetes hospitalized for acute heart failure: findings from the Gulf aCute heArt failuRE (Gulf-CARE) registry. Int J Cardiol. 2017;5273(0116):34386–8.
Straka RJ, Liu LZ, Girase PS, DeLorenzo A, Chapman RH. Incremental cardiovascular costs and resource use associated with diabetes: an assessment of 29,863 patients in the US managed-care setting. Cardiovasc Diabetol. 2009;8:53.
Senthil AN, Ravishankar G, Ravi MS, Meenakshi K, Muthu Kumar D, Swaminathan N, Paul J, Venkatesan S. Pattern of coronary artery disease in symptomatic Type 2 diabetic subjects in the contemporary era and the difference from past studies. Indian Heart J. 2014;66:S46.
Nair M, Prabhakaran D. Why do South Asians have high risk for CAD? Global Heart. 2012;7:307–14.
Azoulay L, Suissa S. Sulfonylureas and the risks of cardiovascular events and death: a methodological meta-regression analysis of the observational studies. Diab Care. 2017;40:706–14.
World Health Organization. Global status report on noncommunicable diseases. Geneva: World Health Organization; 2014. p. 1–302.
Jung CH, Chung JO, Han K, Ko S-H, Ko KS, Park J-Y. Improved trends in cardiovascular complications among subjects with type 2 diabetes in Korea: a nationwide study (2006–2013). Cardiovasc Diabetol. 2017;16:1.
Huang Y, Li J, Zhu X, Sun J, Ji L, Hu D, Pan C, Tan W, Jiang S, Tao X. Relationship between healthy lifestyle behaviors and cardiovascular risk factors in Chinese patients with type 2 diabetes mellitus: a subanalysis of the CCMR-3B STUDY. Acta Diabetol. 2017;54:569–79.
Simmons RK, Griffin SJ, Lauritzen T, Sandbæk A. Effect of screening for type 2 diabetes on risk of cardiovascular disease and mortality: a controlled trial among 139,075 individuals diagnosed with diabetes in Denmark between 2001 and 2009. Diabetologia. 2017;60:2192–9.
Simmons RK, Griffin SJ, Witte DR, Borch-Johnsen K, Lauritzen T, Sandbæk A. Effect of population screening for type 2 diabetes and cardiovascular risk factors on mortality rate and cardiovascular events: a controlled trial among 1,912,392 Danish adults. Diabetologia. 2017;60:2183–91.
Kelsall HL, Fernando PHS, Gwini SM, Sim MR. Cardiovascular disease and type 2 diabetes risk across occupational groups and industry in a statewide study of an Australian working population. J Occup Environ Med. 2018;60:286–94.
Li M-F, Zhao C-C, Li T-T, Tu Y-F, Lu J-X, Zhang R, Chen M-Y, Bao Y-Q, Li L-X, Jia W-P. The coexistence of carotid and lower extremity atherosclerosis further increases cardio-cerebrovascular risk in type 2 diabetes. Cardiovasc Diabetol. 2016;15:43.
Mohammedi K, Woodward M, Marre M, Colagiuri S, Cooper M, Harrap S, Mancia G, Poulter N, Williams B, Zoungas S. Comparative effects of microvascular and macrovascular disease on the risk of major outcomes in patients with type 2 diabetes. Cardiovasc Diabetol. 2017;16:95.
Malik S, Zhao Y, Budoff M, Nasir K, Blumenthal RS, Bertoni AG, Wong ND. Coronary artery calcium score for long-term risk classification in individuals with type 2 diabetes and metabolic syndrome from the multi-ethnic study of atherosclerosis. JAMA Cardiol. 2017;2:1332–40.
Cannon CP, Braunwald E, McCabe CH, Rader DJ, Rouleau JL, Belder R, Joyal SV, Hill KA, Pfeffer MA, Skene AM. Intensive versus moderate lipid lowering with statins after acute coronary syndromes. N Engl J Med. 2004;350:1495–504.
Shepherd J, Barter P, Carmena R, Deedwania P, Fruchart J-C, Haffner S, Hsia J, Breazna A, LaRosa J, Grundy S. Effect of lowering LDL cholesterol substantially below currently recommended levels in patients with coronary heart disease and diabetes: the treating to new targets (TNT) study. Diab Care. 2006;29:1220–6.
Stein EA, Lane M, Laskarzewski P. Comparison of statins in hypertriglyceridemia. Am J Cardiol. 1998;81:66B–9B.
Ren Y, Ren Q, Lu J, Guo X, Huo X, Ji L, Yang X. Low triglyceride as a marker for increased risk of cardiovascular diseases in patients with long-term type 2 diabetes: a cross-sectional survey in China. Diab Metab Res Rev. 2018;34:e2960.
Clua-Espuny JL, González-Henares MA, Queralt-Tomas MLL, et al. Mortality and cardiovascular complications in older complex chronic patients with type 2 diabetes. BioMed Res Int. 2017;2017:6078498.
Cheng Y, Zhang H, Chen R, Yang F, Li W, Chen L, Lin S, Liang G, Cai D, Chen H. Cardiometabolic risk profiles associated with chronic complications in overweight and obese type 2 diabetes patients in South China. PLoS ONE. 2014;9:e101289.
Cortez-Dias N, Martins S, Belo A, Fiuza M. em nome dos Investigadores do Estudio VALSIM: prevalência, tratamento e controlo da diabetes mellitus e dos factores de risco associados nos cuidados de saúde primários em Portugal. Rev Port Cardiol. 2010;29:509–37.
PubMed Google Scholar
Daghash MH, Bener A, Zirie M, Dabdoob W, Al-Hamaq AO, Al-Arabi ZA. Lipoprotein profile in Arabian type 2 diabetic patients. relationship to coronary artery diseases. Int J Cardiol. 2007;121:91–2.
Doucet JA, Bauduceau B, Le Floch JP, Verny C. Medical treatments of elderly, French patients with type 2 diabetes: results at inclusion in the GERODIAB Cohort. Fundam Clin Pharmacol. 2016;30:76–81.
Farrell C, Moran J. Comparison of comorbidities in patients with pre-diabetes to those with diabetes mellitus type 2. IMJ. 2014;3:107.
Fu AZ, Qiu Y, Radican L, Yin DD, Mavros P. Impact of concurrent macrovascular co-morbidities on healthcare utilization in patients with type 2 diabetes in Europe: a matched study. Diab Obes Metab. 2010;12:631–7.
Giallauria F, Fattirolli F, Tramarin R, Ambrosetti M, Griffo R, Riccio C, De Feo S, Piepoli MF, Vigorito C. Clinical characteristics and course of patients with diabetes entering cardiac rehabilitation. Diab Res Clin Pract. 2015;107:267–72.
Gobardhan SN, Dimitriu-Leen AC, van Rosendael AR, van Zwet EW, Roos CJ, Oemrawsingh PV, Kharagjitsingh AV, Jukema JW, Delgado V, Schalij MJ. Prevalence by computed tomographic angiography of coronary plaques in South Asian and white patients with type 2 diabetes mellitus at low and high risk using four cardiovascular risk scores (UKPDS, FRS, ASCVD, and JBS3). Am J Cardiol. 2017;119:705–11.
Gondim F, Caribé A, Vasconcelos KF, Segundo AD, Bandeira F. Vitamin D deficiency is associated with severity of acute coronary syndrome in patients with type 2 diabetes and high rates of sun exposure. Clin Med Insights. 2016;9:37.
CAS Google Scholar
Hermans MP, Bouenizabila E, Ahn SA, Rousseau MF. How to transform a metabolic syndrome score into an insulin sensitivity value? Diab Metab Res Rev. 2016;32:87–94.
Hunt KJ, Kistner-Griffin E, Spruill I, Teklehaimanot AA, Garvey WT, Sale M, Fernandes J. Cardiovascular risk in Gullah African Americans with high familial risk of type 2 diabetes mellitus: project SuGAR. South Med J. 2014;107:607–14.
Jackson CA, Jones NR, Walker JJ, Fischbacher CM, Colhoun HM, Leese GP, Lindsay RS, McKnight JA, Morris AD, Petrie JR, et al. Area-based socioeconomic status, type 2 diabetes and cardiovascular mortality in Scotland. Diabetologia. 2012;55:2938–45.
Jurado J, Ybarra J, Solanas P, Caula J, Gich I, Pou JM, Romeo JH. Prevalence of cardiovascular disease and risk factors in a type 2 diabetic population of the North Catalonia diabetes study. J Am Acad Nurse Pract. 2009;21:140–8.
Kucharska-Newton AM, Couper DJ, Pankow JS, Prineas RJ, Rea TD, Sotoodehnia N, Chakravarti A, Folsom AR, Siscovick DS, Rosamond WD. Diabetes and the risk of sudden cardiac death, the atherosclerosis risk in communities study. Acta Diabetol. 2010;47(Suppl 1):161–8.
Kwon H, Lim J, Shin J, Son J, Lee S, Kim S, Yoo S. A relationship of asymptomatic coronary artery disease and type 2 diabetes in acute ischaemic stroke patients; cerebral angiography and coronary angiography study. Diabetoligia. 2014;57:S26.
Liu X, Liu Y, Lv Y, Li C, Cui Z, Ma J. Prevalence and temporal pattern of hospital readmissions for patients with type I and type II diabetes. BMJ Open. 2015;5:e007362.
Luo Y, Wang X, Wang Y, Wang C, Wang H, Wang D, Liu L, Jia Q, Liu G, Zhao X, et al. Association of glomerular filtration rate with outcomes of acute stroke in type 2 diabetic patients: results from the China National Stroke Registry. Diab Care. 2014;37:173–9.
MacDonald MR, Petrie MC, Home PD, Komajda M, Jones NP, Beck-Nielsen H, Gomis R, Hanefeld M, Pocock SJ, Curtis PS, McMurray JJ. Incidence and prevalence of unrecognized myocardial infarction in people with diabetes: a substudy of the rosiglitazone evaluated for cardiac outcomes and regulation of glycemia in diabetes (RECORD) study. Diab Care. 2011;34:1394–6.
Mazza A, Zamboni S, Rizzato E, Pessina AC, Tikhonoff V, Schiavon L, Casiglia E. Serum uric acid shows a J-shaped trend with coronary mortality in non-insulin-dependent diabetic elderly people. The CArdiovascular STudy in the ELderly (CASTEL). Acta Diabetol. 2007;44:99–105.
Mody R, Kalsekar I, Kavookjian J, Iyer S, Rajagopalan R, Pawar V. Economic impact of cardiovascular co-morbidity in patients with type 2 diabetes. J Diab Complications. 2007;21:75–83.
Mundet X, Cano F, Mata-Cases M, Roura P, Franch J, Birules M, Gimbert R, Llusa J, Cos X. Trends in chronic complications of type 2 diabetic patients from Spanish primary health care centres (GEDAPS study): 10 year-implementation of St. Vincent recommendations. Prim Care Diab. 2012;6:11–8.
Narksawat K, Sujirarat D, Panket P. Combined effects of hypertension and diabetes mellitus with stroke among Thais in the central region of Thailand: a cross-sectional study. J Med Assoc Thai. 2013;5:S1–7.
Penno G, Solini A, Zoppini G, Fondelli C, Trevisan R, Vedovato M, Cavalot F, Gruden G, Lamacchia O, Laviola L. Independent correlates of urinary albumin excretion within the normoalbuminuric range in patients with type 2 diabetes: the renal insufficiency and cardiovascular events (RIACE) Italian multicentre study. Acta Diabetol. 2015;52:971–81.
Rodriguez-Poncelas A, Coll-De Tuero G, Turro-Garriga O, Barrot-de la Puente J, Franch-Nadal J, Mundet-Tuduri X, Red GSG. Impact of chronic kidney disease on the prevalence of cardiovascular disease in patients with type 2 diabetes in Spain: PERCEDIME2 study. BMC Nephrol. 2014;15:150.
Soetedjo N, Permana H, Ruslami R, Livia R, Panduru N, Critchley J, Hulscher M, Tack C, Alisjahbana B, van Crevel R. PO035 High prevalence of macrovascular complications and insufficient cardiovascular management in indonesian diabetes patients; a hospital survey. Diab Res Clin Pract. 2014;106:S63.
Suh DC, Kim CM, Choi IS, Plauschinat CA. Comorbid conditions and glycemic control in elderly patients with type 2 diabetes mellitus, 1988 to 1994 to 1999 to 2004. J Am Geriatr Soc. 2008;56:484–92.
Utrera-Lagunas M, Orea-Tejeda A, Castillo-Martinez L, Balderas-Munoz K, Keirns-Davis C, Espinoza-Rosas S, Sanchez-Ortiz NA, Olvera-Mayorga G. Abnormal myocardial perfusion and risk of heart failure in patients with type 2 diabetes mellitus. Exp Clin Cardiol. 2013;18:e44–6.
PubMed PubMed Central Google Scholar
Vinagre I, Mata-Cases M, Hermosilla E, Morros R, Fina F, Rosell M, Castell C, Franch-Nadal J, Bolibar B, Mauricio D. Control of glycemia and cardiovascular risk factors in patients with type 2 diabetes in primary care in Catalonia (Spain). Diab Care. 2012;35:774–9.
Wong K, Glovaci D, Malik S, Franklin SS, Wygant G, Iloeje U, Kan H, Wong ND. Comparison of demographic factors and cardiovascular risk factor control among US adults with type 2 diabetes by insulin treatment classification. J Diab Complications. 2012;26:169–74.
Yan BP, Zhang Y, Kong AP, Luk AO, Ozaki R, Yeung R, Tong PC, Chan WB, Tsang C-C, Lau K-P. Borderline ankle–brachial index is associated with increased prevalence of micro-and macrovascular complications in type 2 diabetes: a cross-sectional analysis of 12,772 patients from the Joint Asia Diabetes Evaluation Program. Diab Vasc Dis Res. 2015;12:334–41.
Zekry D, Frangos E, Graf C, Michel JP, Gold G, Krause KH, Herrmann FR, Vischer UM. Diabetes, comorbidities and increased long-term mortality in older patients admitted for geriatric inpatient care. Diab Metab. 2012;38:149–55.
World Bank. GNI ranking, atlas method. http://data.worldbank.org/data-catalog/GNI-per-capita-Atlas-and-PPP-table . Accessed 26 Apr 2017.
World Bank. The data blog: New country classifications by income level. https://blogs.worldbank.org/opendata/new-countryclassifications-2016 . Accessed 26 Apr 2017.
Download references
Authors’ contributions
TRE, AA, CL and UHP made substantial contributions to conception and design, or acquisition of data, or analysis and interpretation of data; been involved in drafting the manuscript or revising it critically for important intellectual content. All authors read and approved the final manuscript.
Acknowledgements
This research was funded by Novo Nordisk A/S, Bagsværd, Denmark.
TRE, AA, and CL all received consulting fees for undertaking this project.
Competing interests
TRE, AA, and CL all received consulting fees for undertaking this project. UHP is an employee of Novo Nordisk A/S.
Availability of data and materials
Data sharing is not applicable to this article as no datasets were generated or analyzed during the current study.
Consent for publication
Not applicable.
Ethics approval and consent to participate
Publisher’s note.
Springer Nature remains neutral with regard to jurisdictional claims in published maps and institutional affiliations.
Author information
Authors and affiliations.
Leslie Dan Faculty of Pharmacy, University of Toronto, Barrie, Canada
Thomas R. Einarson
Last Mile, Holte, Denmark
Annabel Acs & Craig Ludwig
Novo Nordisk A/S, Søborg, Denmark
Ulrik H. Panton
You can also search for this author in PubMed Google Scholar
Corresponding author
Correspondence to Craig Ludwig .
Rights and permissions
Open Access This article is distributed under the terms of the Creative Commons Attribution 4.0 International License ( http://creativecommons.org/licenses/by/4.0/ ), which permits unrestricted use, distribution, and reproduction in any medium, provided you give appropriate credit to the original author(s) and the source, provide a link to the Creative Commons license, and indicate if changes were made. The Creative Commons Public Domain Dedication waiver ( http://creativecommons.org/publicdomain/zero/1.0/ ) applies to the data made available in this article, unless otherwise stated.
Reprints and permissions
About this article
Cite this article.
Einarson, T.R., Acs, A., Ludwig, C. et al. Prevalence of cardiovascular disease in type 2 diabetes: a systematic literature review of scientific evidence from across the world in 2007–2017. Cardiovasc Diabetol 17 , 83 (2018). https://doi.org/10.1186/s12933-018-0728-6
Download citation
Received : 20 November 2017
Accepted : 28 March 2018
Published : 08 June 2018
DOI : https://doi.org/10.1186/s12933-018-0728-6
Share this article
Anyone you share the following link with will be able to read this content:
Sorry, a shareable link is not currently available for this article.
Provided by the Springer Nature SharedIt content-sharing initiative
- Cardiovascular disease
- Type 2 diabetes
- Ischemic heart disease
- Myocardial infarction
Cardiovascular Diabetology
ISSN: 1475-2840
- Submission enquiries: [email protected]
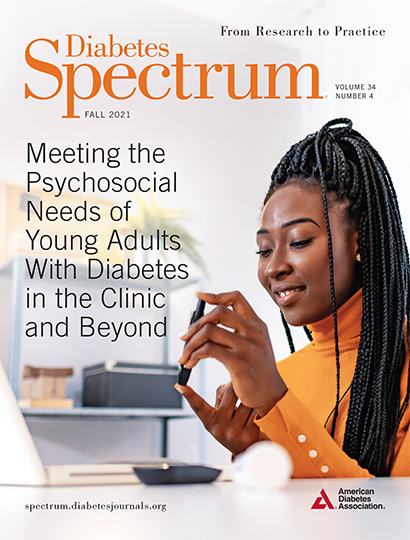
- Previous Article
- Next Article
Research Design and Methods
Article information, literature review of type 2 diabetes management and health literacy.

- Split-Screen
- Article contents
- Figures & tables
- Supplementary Data
- Peer Review
- Open the PDF for in another window
- Cite Icon Cite
- Get Permissions
Rulla Alsaedi , Kimberly McKeirnan; Literature Review of Type 2 Diabetes Management and Health Literacy. Diabetes Spectr 1 November 2021; 34 (4): 399–406. https://doi.org/10.2337/ds21-0014
Download citation file:
- Ris (Zotero)
- Reference Manager
The purpose of this literature review was to identify educational approaches addressing low health literacy for people with type 2 diabetes. Low health literacy can lead to poor management of diabetes, low engagement with health care providers, increased hospitalization rates, and higher health care costs. These challenges can be even more profound among minority populations and non-English speakers in the United States.
A literature search and standard data extraction were performed using PubMed, Medline, and EMBASE databases. A total of 1,914 articles were identified, of which 1,858 were excluded based on the inclusion criteria, and 46 were excluded because of a lack of relevance to both diabetes management and health literacy. The remaining 10 articles were reviewed in detail.
Patients, including ethnic minorities and non-English speakers, who are engaged in diabetes education and health literacy improvement initiatives and ongoing follow-up showed significant improvement in A1C, medication adherence, medication knowledge, and treatment satisfaction. Clinicians considering implementing new interventions to address diabetes care for patients with low health literacy can use culturally tailored approaches, consider ways to create materials for different learning styles and in different languages, engage community health workers and pharmacists to help with patient education, use patient-centered medication labels, and engage instructors who share cultural and linguistic similarities with patients to provide educational sessions.
This literature review identified a variety of interventions that had a positive impact on provider-patient communication, medication adherence, and glycemic control by promoting diabetes self-management through educational efforts to address low health literacy.
Diabetes is the seventh leading cause of death in the United States, and 30.3 million Americans, or 9.4% of the U.S. population, are living with diabetes ( 1 , 2 ). For successful management of a complicated condition such as diabetes, health literacy may play an important role. Low health literacy is a well-documented barrier to diabetes management and can lead to poor management of medical conditions, low engagement with health care providers (HCPs), increased hospitalizations, and, consequently, higher health care costs ( 3 – 5 ).
The Healthy People 2010 report ( 6 ) defined health literacy as the “degree to which individuals have the capacity to obtain, process, and understand basic health information and services needed to make appropriate health decisions.” Diabetes health literacy also encompasses a wide range of skills, including basic knowledge of the disease state, self-efficacy, glycemic control, and self-care behaviors, which are all important components of diabetes management ( 3 – 5 , 7 ). According to the Institute of Medicine’s Committee on Health Literacy, patients with poor health literacy are twice as likely to have poor glycemic control and were found to be twice as likely to be hospitalized as those with adequate health literacy ( 8 ). Associations between health literacy and health outcomes have been reported in many studies, the first of which was conducted in 1995 in two public hospitals and found that many patients had inadequate health literacy and could not perform the basic reading tasks necessary to understand their treatments and diagnoses ( 9 ).
Evaluation of health literacy is vital to the management and understanding of diabetes. Several tools for assessing health literacy have been evaluated, and the choice of which to use depends on the length of the patient encounter and the desired depth of the assessment. One widely used literacy assessment tool, the Test of Functional Health Literacy in Adults (TOFHLA), consists of 36 comprehension questions and four numeric calculations ( 10 ). Additional tools that assess patients’ reading ability include the Rapid Estimate of Adult Literacy in Medicine (REALM) and the Literacy Assessment for Diabetes. Tests that assess diabetes numeracy skills include the Diabetes Numeracy Test, the Newest Vital Sign (NVS), and the Single-Item Literacy Screener (SILS) ( 11 ).
Rates of both diabetes and low health literacy are higher in populations from low socioeconomic backgrounds ( 5 , 7 , 12 ). People living in disadvantaged communities face many barriers when seeking health care, including inconsistent housing, lack of transportation, financial difficulties, differing cultural beliefs about health care, and mistrust of the medical professions ( 13 , 14 ). People with high rates of medical mistrust tend to be less engaged in their care and to have poor communication with HCPs, which is another factor HCPs need to address when working with their patients with diabetes ( 15 ).
The cost of medical care for people with diabetes was $327 billion in 2017, a 26% increase since 2012 ( 1 , 16 ). Many of these medical expenditures are related to hospitalization and inpatient care, which accounts for 30% of total medical costs for people with diabetes ( 16 ).
People with diabetes also may neglect self-management tasks for various reasons, including low health literacy, lack of diabetes knowledge, and mistrust between patients and HCPs ( 7 , 15 ).
These challenges can be even more pronounced in vulnerable populations because of language barriers and patient-provider mistrust ( 17 – 19 ). Rates of diabetes are higher among racial and ethnic minority groups; 15.1% of American Indians and Alaskan Natives, 12.7% of Non-Hispanic Blacks, 12.1% of Hispanics, and 8% of Asian Americans have diagnosed diabetes, compared with 7.4% of non-Hispanic Whites ( 1 ). Additionally, patient-provider relationship deficits can be attributed to challenges with communication, including HCPs’ lack of attention to speaking slowly and clearly and checking for patients’ understanding when providing education or gathering information from people who speak English as a second language ( 15 ). White et al. ( 15 ) demonstrated that patients with higher provider mistrust felt that their provider’s communication style was less interpersonal and did not feel welcome as part of the decision-making process.
To the authors’ knowledge, there is no current literature review evaluating interventions focused on health literacy and diabetes management. There is a pressing need for such a comprehensive review to provide a framework for future intervention design. The objective of this literature review was to gather and summarize studies of health literacy–based diabetes management interventions and their effects on overall diabetes management. Medication adherence and glycemic control were considered secondary outcomes.
Search Strategy
A literature review was conducted using the PubMed, Medline, and EMBASE databases. Search criteria included articles published between 2015 and 2020 to identify the most recent studies on this topic. The search included the phrases “diabetes” and “health literacy” to specifically focus on health literacy and diabetes management interventions and was limited to original research conducted in humans and published in English within the defined 5-year period. Search results were exported to Microsoft Excel for evaluation.
Study Selection
Initial screening of the articles’ abstracts was conducted using the selection criteria to determine which articles to include or exclude ( Figure 1 ). The initial search results were reviewed for the following inclusion criteria: original research (clinical trials, cohort studies, and cross-sectional studies) conducted in human subjects with type 2 diabetes in the United States, and published in English between 2015 and 2020. Articles were considered to be relevant if diabetes was included as a medical condition in the study and an intervention was made to assess or improve health literacy. Studies involving type 1 diabetes or gestational diabetes and articles that were viewpoints, population surveys, commentaries, case reports, reviews, or reports of interventions conducted outside of the United States were excluded from further review. The criteria requiring articles to be from the past 5 years and from the United States were used because of the unique and quickly evolving nature of the U.S. health care system. Articles published more than 5 years ago or from other health care systems may have contributed information that was not applicable to or no longer relevant for HCPs in the United States. Articles were screened and reviewed independently by both authors. Disagreements were resolved through discussion to create the final list of articles for inclusion.
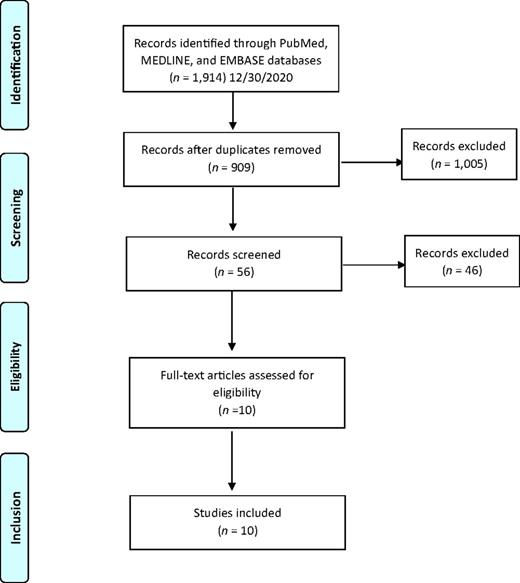
PRISMA diagram of the article selection process.
Data Extraction
A standard data extraction was performed for each included article to obtain information including author names, year of publication, journal, study design, type of intervention, primary outcome, tools used to assess health literacy or type 2 diabetes knowledge, and effects of intervention on overall diabetes management, glycemic control, and medication adherence.
A total of 1,914 articles were collected from a search of the PubMed, MEDLINE, and EMBASE databases, of which 1,858 were excluded based on the inclusion and exclusion criteria. Of the 56 articles that met criteria for abstract review, 46 were excluded because of a lack of relevance to both diabetes management and health literacy. The remaining 10 studies identified various diabetes management interventions, including diabetes education tools such as electronic medication instructions and text message–based interventions, technology-based education videos, enhanced prescription labels, learner-based education materials, and culturally tailored interventions ( 15 , 20 – 28 ). Figure 1 shows the PRISMA diagram of the article selection process, and Table 1 summarizes the findings of the article reviews ( 15 , 20 – 28 ).
Findings of the Article Reviews (15,20–28)
SAHLSA, Short Assessment of Health Literacy for Spanish Adults.
Medical mistrust and poor communication are challenging variables in diabetes education. White et al. ( 15 ) examined the association between communication quality and medical mistrust in patients with type 2 diabetes. HCPs at five health department clinics received training in effective health communication and use of the PRIDE (Partnership to Improve Diabetes Education) toolkit in both English and Spanish, whereas control sites were only exposed to National Diabetes Education Program materials without training in effective communication. The study evaluated participant communication using several tools, including the Communication Assessment Tool (CAT), Interpersonal Processes of Care (IPC-18), and the Short Test of Functional Health Literacy in Adults (s-TOFHLA). The authors found that higher levels of mistrust were associated with lower CAT and IPC-18 scores.
Patients with type 2 diabetes are also likely to benefit from personalized education delivery tools such as patient-centered labeling (PCL) of prescription drugs, learning style–based education materials, and tailored text messages ( 24 , 25 , 27 ). Wolf et al. ( 27 ) investigated the use of PCL in patients with type 2 diabetes and found that patients with low health literacy who take medication two or more times per day have higher rates of proper medication use when using PCL (85.9 vs. 77.4%, P = 0.03). The objective of the PCL intervention was to make medication instructions and other information on the labels easier to read to improve medication use and adherence rates. The labels incorporated best-practice strategies introduced by the Institute of Medicine for the Universal Medication Schedule. These strategies prioritize medication information, use of larger font sizes, and increased white space. Of note, the benefits of PCL were largely seen with English speakers. Spanish speakers did not have substantial improvement in medication use or adherence, which could be attributed to language barriers ( 27 ).
Nelson et al. ( 25 ) analyzed patients’ engagement with an automated text message approach to supporting diabetes self-care activities in a 12-month randomized controlled trial (RCT) called REACH (Rapid Education/Encouragement and Communications for Health) ( 25 ). Messages were tailored based on patients’ medication adherence, the Information-Motivation-Behavioral Skills model of health behavior change, and self-care behaviors such as diet, exercise, and self-monitoring of blood glucose. Patients in this trial were native English speakers, so further research to evaluate the impact of the text message intervention in patients with limited English language skills is still needed. However, participants in the intervention group reported higher engagement with the text messages over the 12-month period ( 25 ).
Patients who receive educational materials based on their learning style also show significant improvement in their diabetes knowledge and health literacy. Koonce et al. ( 24 ) developed and evaluated educational materials based on patients’ learning style to improve health literacy in both English and Spanish languages. The materials were made available in multiple formats to target four different learning styles, including materials for visual learners, read/write learners, auditory learners, and kinesthetic learners. Spanish-language versions were also available. Researchers were primarily interested in measuring patients’ health literacy and knowledge of diabetes. The intervention group received materials in their preferred learning style and language, whereas the control group received standard of care education materials. The intervention group showed significant improvement in diabetes knowledge and health literacy, as indicated by Diabetes Knowledge Test (DKT) scores. More participants in the intervention group reported looking up information about their condition during week 2 of the intervention and showed an overall improvement in understanding symptoms of nerve damage and types of food used to treat hypoglycemic events. However, the study had limited enrollment of Spanish speakers, making the applicability of the results to Spanish-speaking patients highly variable.
Additionally, findings by Hofer et al. ( 22 ) suggest that patients with high A1C levels may benefit from interventions led by community health workers (CHWs) to bridge gaps in health literacy and equip patients with the tools to make health decisions. In this study, Hispanic and African American patients with low health literacy and diabetes not controlled by oral therapy benefited from education sessions led by CHWs. The CHWs led culturally tailored support groups to compare the effects of educational materials provided in an electronic format (via iDecide) and printed format on medication adherence and self-efficacy. The study found increased adherence with both formats, and women, specifically, had a significant increase in medication adherence and self-efficacy. One of the important aspects of this study was that the CHWs shared cultural and linguistic characteristics with the patients and HCPs, leading to increased trust and satisfaction with the information presented ( 22 ).
Kim et al. ( 23 ) found that Korean-American participants benefited greatly from group education sessions that provided integrated counseling led by a team of nurses and CHW educators. The intervention also had a health literacy component that focused on enhancing skills such as reading food package labels, understanding medical terminology, and accessing health care services. This intervention led to a significant reduction of 1–1.3% in A1C levels in the intervention group. The intervention established the value of collaboration between CHW educators and nurses to improve health information delivery and disease management.
A collaboration between CHW educators and pharmacists was also shown to reinforce diabetes knowledge and improve health literacy. Sharp et al. ( 26 ) conducted a cross-over study in four primary care ambulatory clinics that provided care for low-income patients. The study found that patients with low health literacy had more visits with pharmacists and CHWs than those with high health literacy. The CHWs provided individualized support to reinforce diabetes self-management education and referrals to resources such as food, shelter, and translation services. The translation services in this study were especially important for building trust with non-English speakers and helping patients understand their therapy. Similar to other studies, the CHWs shared cultural and linguistic characteristics with their populations, which helped to overcome communication-related and cultural barriers ( 23 , 26 ).
The use of electronic tools or educational videos yielded inconclusive results with regard to medication adherence. Graumlich et al. ( 20 ) implemented a new medication planning tool called Medtable within an electronic medical record system in several outpatient clinics serving patients with type 2 diabetes. The tool was designed to organize medication review and patient education. Providers can use this tool to search for medication instructions and actionable language that are appropriate for each patient’s health literacy level. The authors found no changes in medication knowledge or adherence, but the intervention group reported higher satisfaction. On the other hand, Yeung et al. ( 28 ) showed that pharmacist-led online education videos accessed using QR codes affixed to the patients’ medication bottles and health literacy flashcards increased patients’ medication adherence in an academic medical hospital.
Goessl et al. ( 21 ) found that patients with low health literacy had significantly higher retention of information when receiving evidence-based diabetes education through a DVD recording than through an in-person group class. This 18-month RCT randomized participants to either the DVD or in-person group education and assessed their information retention through a teach-back strategy. The curriculum consisted of diabetes prevention topics such as physical exercise, food portions, and food choices. Participants in the DVD group had significantly higher retention of information than those in the control (in-person) group. The authors suggested this may have been because participants in the DVD group have multiple opportunities to review the education material.
Management of type 2 diabetes remains a challenge for HCPs and patients, in part because of the challenges discussed in this review, including communication barriers between patients and HCPs and knowledge deficits about medications and disease states ( 29 ). HCPs can have a positive impact on the health outcomes of their patients with diabetes by improving patients’ disease state and medication knowledge.
One of the common themes identified in this literature review was the prevalence of culturally tailored diabetes education interventions. This is an important strategy that could improve diabetes outcomes and provide an alternative approach to diabetes self-management education when working with patients from culturally diverse backgrounds. HCPs might benefit from using culturally tailored educational approaches to improve communication with patients and overcome the medical mistrust many patients feel. Although such mistrust was not directly correlated with diabetes management, it was noted that patients who feel mistrustful tend to have poor communication with HCPs ( 20 ). Additionally, Latino/Hispanic patients who have language barriers tend to have poor glycemic control ( 19 ). Having CHWs work with HCPs might mitigate some patient-provider communication barriers. As noted earlier, CHWs who share cultural and linguistic characteristics with their patient populations have ongoing interactions and more frequent one-on-one encounters ( 12 ).
Medication adherence and glycemic control are important components of diabetes self-management, and we noted that the integration of CHWs into the diabetes health care team and the use of simplified medication label interventions were both successful in improving medication adherence ( 23 , 24 ). The use of culturally tailored education sessions and the integration of pharmacists and CHWs into the management of diabetes appear to be successful in reducing A1C levels ( 12 , 26 ). Electronic education tools and educational videos alone did not have an impact on medication knowledge or information retention in patients with low health literacy, but a combination of education tools and individualized sessions has the potential to improve diabetes medication knowledge and overall self-management ( 20 , 22 , 30 ).
There were several limitations to our literature review. We restricted our search criteria to articles published in English and studies conducted within the United States to ensure that the results would be relevant to U.S. HCPs. However, these limitations may have excluded important work on this topic. Additional research expanding this search beyond the United States and including articles published in other languages may demonstrate different outcomes. Additionally, this literature review did not focus on A1C as the primary outcome, although A1C is an important indicator of diabetes self-management. A1C was chosen as the method of evaluating the impact of health literacy interventions in patients with diabetes, but other considerations such as medication adherence, impact on comorbid conditions, and quality of life are also important factors.
The results of this work show that implementing health literacy interventions to help patients manage type 2 diabetes can have beneficial results. However, such interventions can have significant time and monetary costs. The potential financial and time costs of diabetes education interventions were not evaluated in this review and should be taken into account when designing interventions. The American Diabetes Association estimated the cost of medical care for people with diabetes to be $327 billion in 2017, with the majority of the expenditure related to hospitalizations and nursing home facilities ( 16 ). Another substantial cost of diabetes that can be difficult to measure is treatment for comorbid conditions and complications such as cardiovascular and renal diseases.
Interventions designed to address low health literacy and provide education about type 2 diabetes could be a valuable asset in preventing complications and reducing medical expenditures. Results of this work show that clinicians who are considering implementing new interventions may benefit from the following strategies: using culturally tailored approaches, creating materials for different learning styles and in patients’ languages, engaging CHWs and pharmacists to help with patient education, using PCLs for medications, and engaging education session instructors who share patients’ cultural and linguistic characteristics.
Diabetes self-management is crucial to improving health outcomes and reducing medical costs. This literature review identified interventions that had a positive impact on provider-patient communication, medication adherence, and glycemic control by promoting diabetes self-management through educational efforts to address low health literacy. Clinicians seeking to implement diabetes care and education interventions for patients with low health literacy may want to consider drawing on the strategies described in this article. Providing culturally sensitive education that is tailored to patients’ individual learning styles, spoken language, and individual needs can improve patient outcomes and build patients’ trust.
Duality of Interest
No potential conflicts of interest relevant to this article were reported.
Author Contributions
Both authors conceptualized the literature review, developed the methodology, analyzed the data, and wrote, reviewed, and edited the manuscript. R.A. collected the data. K.M. supervised the review. K.M. is the guarantor of this work and, as such, has full access to all the data in the study and takes responsibility for the integrity of the data and the accuracy of the data analysis.
Prior Presentation
Portions of this research were presented at the Washington State University College of Pharmacy and Pharmaceutical Sciences Honors Research Day in April 2019.
Email alerts
- Online ISSN 1944-7353
- Print ISSN 1040-9165
- Diabetes Care
- Clinical Diabetes
- Diabetes Spectrum
- Standards of Medical Care in Diabetes
- Scientific Sessions Abstracts
- BMJ Open Diabetes Research & Care
- ShopDiabetes.org
- ADA Professional Books
Clinical Compendia
- Clinical Compendia Home
- Latest News
- DiabetesPro SmartBrief
- Special Collections
- DiabetesPro®
- Diabetes Food Hub™
- Insulin Affordability
- Know Diabetes By Heart™
- About the ADA
- Journal Policies
- For Reviewers
- Advertising in ADA Journals
- Reprints and Permission for Reuse
- Copyright Notice/Public Access Policy
- ADA Professional Membership
- ADA Member Directory
- Diabetes.org
- X (Twitter)
- Cookie Policy
- Accessibility
- Terms & Conditions
- Get Adobe Acrobat Reader
- © Copyright American Diabetes Association
This Feature Is Available To Subscribers Only
Sign In or Create an Account
- Reference Manager
- Simple TEXT file
People also looked at
Review article, a systematic review of type 2 diabetes mellitus and hypertension in imaging studies of cognitive aging: time to establish new norms.
- 1 Baycrest Centre, Rotman Research Institute, Toronto, ON, Canada
- 2 Sunnybrook Research Institute, Heart and Stroke Foundation Canadian Partnership for Stroke Recovery, Toronto, ON, Canada
- 3 Department of Medical Biophysics, Faculty of Medicine, University of Toronto, Toronto, ON, Canada
- 4 Department of Nutritional Sciences, Faculty of Medicine, University of Toronto, Toronto, ON, Canada
- 5 Departments of Psychology and Psychiatry, University of Toronto, Toronto, ON, Canada
The rising prevalence of type 2 diabetes (T2DM) and hypertension in older adults, and the deleterious effect of these conditions on cerebrovascular and brain health, is creating a growing discrepancy between the “typical” cognitive aging trajectory and a “healthy” cognitive aging trajectory. These changing health demographics make T2DM and hypertension important topics of study in their own right, and warrant attention from the perspective of cognitive aging neuroimaging research. Specifically, interpretation of individual or group differences in blood oxygenation level dependent magnetic resonance imaging (BOLD MRI) or positron emission tomography (PET H 2 O 15 ) signals as reflective of differences in neural activation underlying a cognitive operation of interest requires assumptions of intact vascular health amongst the study participants. Without adequate screening, inclusion of individuals with T2DM or hypertension in “healthy” samples may introduce unwanted variability and bias to brain and/or cognitive measures, and increase potential for error. We conducted a systematic review of the cognitive aging neuroimaging literature to document the extent to which researchers account for these conditions. Of the 232 studies selected for review, few explicitly excluded individuals with T2DM (9%) or hypertension (13%). A large portion had exclusion criteria that made it difficult to determine whether T2DM or hypertension were excluded (44 and 37%), and many did not mention any selection criteria related to T2DM or hypertension (34 and 22%). Of all the surveyed studies, only 29% acknowledged or addressed the potential influence of intersubject vascular variability on the measured BOLD or PET signals. To reinforce the notion that individuals with T2DM and hypertension should not be overlooked as a potential source of bias, we also provide an overview of metabolic and vascular changes associated with T2DM and hypertension, as they relate to cerebrovascular and brain health.
Introduction
Amongst middle-aged and older adults, the rising prevalence of T2DM, hypertension, and other conditions that comprise the metabolic syndrome is a global health epidemic, attributed largely to sedentary lifestyles, poor diet, and lack of exercise. In 2008, it was estimated that 347 million adults worldwide had T2DM, up from 153 million in 1980 ( Danaei et al., 2011 ). Over the next two decades, it is expected that these numbers will continue to rise, by as much as 38% by 2030 ( Shaw et al., 2010 ). Prevalence rates of hypertension are even higher. In 2000, the global prevalence of hypertension was 26.4%, affecting an estimated 972 million people worldwide. Again, these numbers are expected to increase by approximately 60% by 2025, to a total of 1.56 billion people ( Kearney et al., 2005 ). Critically, hypertension is present in up to 75% of individuals with T2DM ( Colosia et al., 2013 ). The growing number of middle-aged and older adults living with T2DM and/or hypertension makes these conditions important topics of study in their own right.
Better long-term health care and disease management allow middle-aged and older adults to live with T2DM and hypertension for many years; however, both of these conditions have long-term deleterious effects on cerebrovascular and brain health, and contribute to cognitive impairment and decline ( Gorelick et al., 2011 ). T2DM and midlife hypertension confer a high risk for development of mild cognitive impairment (MCI) and dementia ( Launer et al., 2000 ; Kloppenborg et al., 2008 ; Creavin et al., 2012 ; Crane et al., 2013 ; Roberts et al., 2014 ), and older individuals with T2DM progress to dementia at faster rates ( Xu et al., 2010 ; Morris et al., 2014 ). These changing health demographics have created a discrepancy: what we define as “normal” or “typical” cognitive aging is becoming farther and farther removed from what would be considered optimal, or “healthy” cognitive aging.
This trend warrants attention from the perspective of cognitive aging research. Without adequate screening procedures in place, inclusion of individuals with T2DM and hypertension in otherwise healthy study samples may introduce unwanted variability and bias to brain and/or cognitive measures, and increase the potential for type 1 and type 2 errors. Functional neuroimaging studies may be particularly vulnerable in this regard. Blood oxygenation level dependent magnetic resonance imaging (BOLD MRI) and positron emission tomography (PET H 2 O 15 ) measure hemodynamic changes associated with neural activity, and thus provide an indirect measure of neural function ( Logothetis et al., 2001 ). To interpret individual or group differences in BOLD or PET signaling as reflective of individual or group differences in neural activation underlying a cognitive operation of interest, we rely on assumptions of intact neurovascular signaling, cerebrovascular reactivity, and vascular health amongst the study participants. These assumptions may be true in young and healthy individuals, but do not hold in older adults with conditions that affect vascular health ( D'Esposito et al., 2003 ). Even normal, age-related changes in the integrity of the cerebrovascular system can undermine these assumptions ( D'Esposito et al., 1999 ).
Yet, it was our impression that relatively few studies in the cognitive aging neuroimaging literature consider T2DM or hypertension during recruitment, or control for potential confounds associated with these conditions during analysis. To clarify the extent to which current research practices consider T2DM and hypertension in study design, we present the results of a systematic review of the cognitive aging neuroimaging literature, looking at study inclusion/exclusion criteria and methodology related to T2DM and hypertension. Then, to reinforce the notion that individuals with T2DM and hypertension should not be overlooked as a potential source of bias, we provide an overview of metabolic and vascular changes associated with T2DM and hypertension, as they relate to vascular health, structural brain atrophy, and functional integrity. The final section discusses best practices moving forward.
Systematic Review
This review focuses on the cognitive aging neuroimaging literature, however the issues associated with inclusion of individuals with T2DM and hypertension in study samples are by no means limited to this area of research. Any research study whose population of interest has high prevalence rates of T2DM or hypertension should be cognizant of these issues. For example, psychiatric populations have a higher incidence of metabolic disruption and T2DM that is mediated, at least partially, by the use of mood stabilizers, anticonvulsants, and antipsychotic medications ( Regenold et al., 2002 ; Newcomer and Haupt, 2006 ).
It should also be noted that the purpose of this review is not to quantitatively compare the results of studies that have excluded T2DM and/or hypertension with those that have not. This type of comparison is not feasible for numerous reasons, the primary one being that the extent to which individuals with T2DM or hypertension were present in study samples that did not screen for either condition is unknown. Rather, the aim of this review is to highlight the proportion of studies in the cognitive aging neuroimaging literature that consider T2DM and/or hypertension in their inclusion/exclusion criteria, or attempt to account for the potential bias introduced by inclusion of these individuals in their study groups.
We searched PsychInfo, MedLine, and PubMed between 1995 and February, 2013 using the search terms [“functional magnetic resonance imaging” or “positron emission tomography”], [“geriatrics” or “aging” or “age differences”], and [“cognit*” or “neuropsych*” or “memory” or “attention”]. Across the three databases, these search terms produced 704 unique empirical studies. From these results, we excluded studies that did not include a “healthy” or “normal” older adult sample ( n = 125), included a clinical sample other than MCI or Alzheimer disease (AD)/dementia (e.g., psychiatric; n = 46), did not use BOLD or PET H 2 O 15 imaging ( n = 227), and did not scan during a cognitive or resting state task ( n = 74; Figure 1 ).
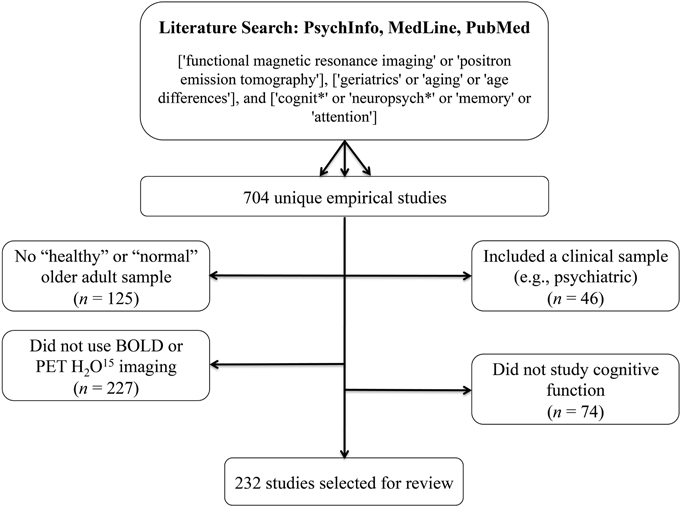
Figure 1. Literature search terms and exclusion criteria . Based on these criteria, 232 studies were selected for review.
Based on these criteria, 232 studies were selected for review. These studies are identified with an asterisk (*) in the reference section. Two hundred and nineteen of these used BOLD imaging, one used both BOLD and PET H 2 O 15 , and 12 used PET H 2 O 15 only. One hundred and sixty five of these studies compared a “healthy” older group with a group of young participants, 34 studies compared a “healthy” older sample to an MCI and/or AD group (two of which also included a young adult comparison group), and the remaining 33 studies looked only at a “healthy” older sample. The majority of surveyed studies employed a memory paradigm during imaging (e.g., encoding/recognition of words, pictures, scenes, faces, autobiographical memory, spatial memory, associative memory, implicit learning). Working memory and executive processes were also well-studied (e.g., cognitive control, inhibition, decision making, mental rotation, task-switching, attention, judgment, processing speed, naming, imagery, verb generation, fluency). We also included resting-state studies in the sample.
Our primary concern was how sample selection was reported to have occurred. In particular, we were interested to learn how many studies specifically screened for T2DM and/or hypertension in their healthy older adult samples. For each of the 232 identified studies, the inclusion/exclusion criteria were examined according to the following criteria: (i) explicit exclusion of T2DM and/or hypertension, or exclusion of medical disorders/physical illnesses/systemic illnesses (implying that all medical conditions, including T2DM and hypertension, were excluded); (ii) exclusion of “significant,” “major,” or “severe” medical/physical/systemic disorders; or (iii) no screening criteria related to T2DM and/or hypertension provided. We also surveyed each of the 232 studies to determine how subjects were screened (e.g., self-report questionnaire, clinical assessment with a medical doctor, laboratory testing), and how—if at all—the potential influence of intersubject vascular variability on the measured BOLD or PET signals was addressed.
In each section below, superscript numbers, letters, and symbols are used to represent the extent to which studies screened for T2DM and hypertension, the screening method, and the degree to which studies attempted to account for intersubject vascular variability, respectively. The identified studies are denoted in the reference section according to these superscript classifiers.
Inclusion/exclusion of T2DM and hypertension
Of the 232 studies surveyed, only 22 (9.5%) explicitly excluded individuals with T2DM( 1 ), and only 29 (12.5%) explicitly excluded individuals with hypertension( 2 ). Thirteen studies—approximately 6%—excluded both T2DM and hypertension. Fourteen studies (6.0%) excluded individuals on antihypertensive medication( 3 ), however few of these studies also clarified whether individuals were assessed for untreated hypertension and excluded, if present. Nineteen studies (8.2%) excluded medical illnesses, systemic illnesses, medical disorders or physical illnesses( 4 ). This criterion implies that all medical conditions, including T2DM and hypertension, were excluded.
In contrast, almost half of the included studies (102; 44.0%) had exclusion criteria that made it difficult to determine whether T2DM was excluded( 5 ), and 85 studies (36.6%) had exclusion criteria that made it difficult to determine whether hypertension and/or antihypertensive medications were excluded( 6 ). These studies listed “major medical illnesses,” “significant medical conditions,” “serious systemic illnesses,” “conditions/medications interfering with cognitive and/or brain function,” “vascular disease,” “cardiovascular disease,” and/or “conditions/medications interfering with the fMRI signal” as exclusion criteria, or simply described their sample as “healthy.” There were also many studies that did not mention any selection criteria related to T2DM (80; 34.5%)( 7 ) or hypertension (51; 22.0%)( 8 ).
In addition, 26 studies (11.2%) included individuals with controlled hypertension( 9 ), 8 studies (3.5%) included controlled and uncontrolled hypertension( 10 ), 3 studies (1.3%) included individuals with controlled T2DM( 11 ), and 6 studies (2.5%) included individuals with controlled and uncontrolled T2DM in their healthy cohort( 12 ). Figure 2 provides a visual depiction of these results.
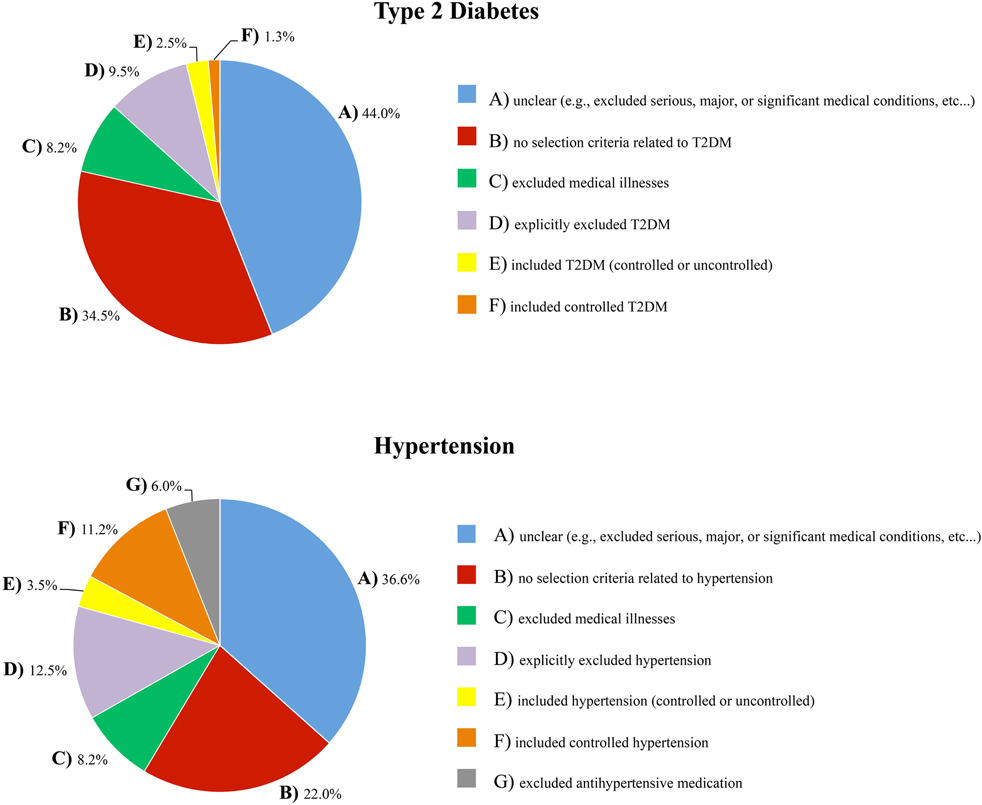
Figure 2. The extent to which T2DM and hypertension were accounted for in the inclusion/exclusion criteria of the healthy samples that were surveyed .
Screening method
The majority of studies (173; 75%) did not report how they conducted their medical screening( a ). Only 28 studies (12%) reported having screened subjects with physician-conducted medical examinations and/or laboratory testing( b ). Sixteen studies (7%) screened participants with telephone interviews, in-person clinical interviews, medical history, chart reviews, or a combination of these methods( c ). The remaining 15 studies (6%) used a self-report questionnaire to assess medical status( d ).
Accounting for intersubject vascular variability
A survey of the 232 included studies found that just under one third (29%) acknowledged and/or addressed the potential influence of intersubject vascular variability on the reported results. Many excluded subjects with a high vascular burden by screening for white matter hyperintensities in the imaging data( ■ ). Others compared groups on vascular risk factors( + ), compared outcome measures on hypertension status or antihypertensive treatment status( ♦ ), or attempted to control for health, blood pressure, and/or white matter hyperintensities in the reported associations ( ❖ ). Several studies noted in their discussion the possibility that the reported results were influenced by vascular factors, or explained why they did not think this was an issue( • ). A few studies used the measured BOLD or PET signals to examine and account for individual differences in vascular health( □ ); for example, by ensuring that groups were equated on BOLD signal variability, by comparing the temporal characteristics of the hemodynamic response curve across groups, with proportional scaling of the BOLD or PET signal, or by focusing on group by task interactions (instead of group main effects) or comparing within-subject task contrasts across individuals or groups to minimize any individual or group differences in vascular integrity.
There are rigorous ways to account for intersubject vascular variability, such as additional task data or an additional imaging contrast. Several studies included in the present review used arterial spin labeling (ASL) MRI ( ▴ ) or PET ( ▾ ) to measure resting cerebral blood flow and control for individual differences in perfusion. Three studies used a breath-hold task to index individual differences in cerebrovascular reactivity ( ❍ ), and two studies included a low-level motor or baseline task to ensure that participants demonstrated an adequate hemodynamic response ( × ).
Our results found that fewer than 10% of the selected functional imaging studies on cognitive aging explicitly excluded individuals with T2DM from their normative samples, and fewer than 15% explicitly excluded individuals with hypertension. A number of studies reported selection criteria that were insufficient to determine whether T2DM or hypertension were screened. Critically, one third of included studies had no reported inclusion or exclusion criteria related to T2DM, while almost a quarter had no reported inclusion or exclusion criteria related to hypertension. Only 67 of the 232 selected studies (29%) acknowledged or addressed the potential influence of intersubject vascular variability on the measured BOLD or PET signals.
Moreover, the large majority of studies did not include information about the medical screening process itself (e.g., laboratory testing vs. clinical interview vs. self-report questionnaire). This is not ideal when established tests for T2DM and hypertension are available (for example, 24-h ambulatory blood pressure monitoring would be the gold-standard for determining hypertension status, and an oral glucose tolerance test for determining T2DM status). Furthermore, we posit that participants may be less likely to volunteer T2DM or hypertension status as a “significant” medical illness without specific probing (i.e., compared to cancer, HIV, multiple sclerosis, or heart disease), because when these conditions are well-controlled they can have a minimal impact on day-to-day functioning, and, in the case of T2DM, can be controlled by diet alone. Collectively, these observations point to a lack of awareness that T2DM and hypertension are major medical illnesses that interfere significantly with cognitive and brain function in older adults.
Overview: Metabolic and Vascular Complications of Type 2 Diabetes Mellitus and Hypertension
To reinforce the position that T2DM and hypertension are conditions that can have a major effect on brain health and cognitive aging, this next section reviews evidence on the cognitive deficits, structural changes, and functional consequences associated with T2DM and hypertension, and describes some of the mechanisms that mediate these changes.
Type 2 Diabetes Mellitus
T2DM is the result of peripheral insulin resistance, which leads to insulin dysregulation and hyperglycemia. These metabolic changes affect cerebrovascular health, structural integrity, and brain function, and underlie the associations between T2DM, cognitive decline, and dementia risk.
Insulin dysregulation
Insulin is a peptide hormone that is critical for regulation of blood glucose levels. Binding of insulin to its receptors, found on nearly all cells throughout the body, facilitates the cellular uptake of glucose from the blood. When bound, insulin and insulin-like growth factor also activate complex intracellular signaling pathways that promote cell growth and survival, regulate glucose metabolism, and inhibit oxidative stress and apoptosis (for a review, see Nakae et al., 2001 ).
The defining characteristic of T2DM is peripheral insulin resistance, which occurs when cells in the body decrease their response to insulin stimulation. In the developing stage of this disease, the pancreas is able to produce enough insulin to overcome this resistance. This results in peripheral hyperinsulinemia, and blood glucose levels remain within the normal range. As the disease progresses, however, the pancreas can no longer keep up, and blood glucose levels begin to rise. When blood glucose levels are high even in the fasting state, T2DM is diagnosed.
Peripheral insulin resistance and hyperinsulinemia have a counterintuitive impact on insulin levels within the central nervous system. In the face of peripheral hyperinsulinemia, insulin transport across the blood brain barrier is effectively reduced, resulting in a brain hypo -insulinemic state (e.g., Heni et al., 2013 ). Low brain insulin levels and disrupted insulin signaling contribute to cognitive impairments directly, particularly in medial temporal lobe regions where insulin receptors are abundant ( Convit, 2005 ; Craft, 2006 ). Indirectly, low brain insulin levels exacerbate amyloid beta (Aβ) and tau pathology, hallmarks of Alzheimer disease (AD). It is here that we see the link between T2DM and Alzheimer disease pathology: brain insulin deficiency results in the down-regulation of insulin degrading enzyme (IDE; Luchsinger, 2008 ), which also has a role in degrading Aβ ( Carlsson, 2010 ). As a result, Aβ degradation is effectively reduced, contributing to its aggregation and amyloid plaque formation. Decreased brain insulin levels also suppress the enzymes involved in tau phosphorylation, contributing to the formation of neurofibrillary tangles ( Akter et al., 2011 ). While the downstream impact of T2DM-mediated brain insulin deficiency and insulin resistance is more moderate than that associated with AD, the underlying pathogenic mechanisms are similar ( Steen et al., 2005 ), and it has been proposed that AD is a form of diabetes mellitus that selectively affects the brain (T3DM; for discussion, see de la Monte and Wands, 2008 ). Given this, is not surprising that individuals with T2DM show a pattern of memory impairment, medial temporal lobe atrophy, and reduced hippocampal connectivity that is similar to the classic pattern of memory deficits, neurodegeneration, and network disruption in AD (e.g., Gold et al., 2007 ; Zhou et al., 2010 ; Baker et al., 2011 ; Cui et al., 2014 ).
Hyperglycemia
When cells in the body become resistant to the effects of insulin, blood glucose levels rise, resulting in hyperglycemia. Endothelial cells are particularly vulnerable to the effects of hyperglycemia, because they are less efficient at reducing glucose uptake in the face of high blood glucose levels ( Kaiser et al., 1993 ). Under such conditions, the resultant intracellular hyperglycemia induces an overproduction of reactive oxygen species in the mitochondria, which increases oxidative stress within the cell. This initiates a cascade of biochemical events that mediate much of the microvascular and macrovascular damage associated with T2DM including, but not limited to, increased intracellular formation of advanced glycation end-products (AGEs) and protein kinase C activation ( Du et al., 2000 ; Nishikawa et al., 2000 ; Brownlee, 2005 ; Giacco and Brownlee, 2010 ; Johnson, 2012 ).
AGEs are formed during normal metabolism on proteins with slower rates of turnover, in almost all cells throughout the body. AGE accumulation over time is a major factor in normal aging; however, under hyperglycemic conditions, AGE production is exacerbated beyond normal levels. AGEs cause intracellular damage and induce apoptosis through a process called cross-linking ( Shaikh and Nicholson, 2008 ). AGEs also contribute to oxidative stress, and themselves activate inflammatory signaling cascades (for a review, see Yan et al., 2008 ). Critically, under hyperglycemic conditions, the Aβ protein itself can act as an AGE ( Granic et al., 2009 ), which enhances its own aggregation and further increases amyloid plaque formation.
Protein kinase C activation, on the other hand, affects a variety of changes in gene expression that culminate in vascular dysfunction. Production of nitric oxide (NO), a vasodilator, is decreased, and production of endothelin-1, a vasoconstrictor, is increased. As a result, blood vessels are less able to dilate to accommodate increased blood flow demand. Over time, chronic exposure to high concentrations of endothelin-1 and decreased concentrations of NO contribute to diminished vessel elasticity, and structural changes in the vessel wall that result in atherosclerotic plaque formation ( Kalani, 2008 ).
In the brain, hyperglycemia-mediated macro- and microvascular damage reduces the delivery of nutrients and oxygen required to meet metabolic demands. Altered cerebral autoregulation has been observed in middle-aged adults with T2DM ( Brown et al., 2008 ), and may be an early manifestation of microvascular disease ( Kim et al., 2008 ). Older adults with T2DM show decreased blood flow velocity, increased cerebrovascular resistance, and impaired vasoreactivity ( Novak et al., 2006 ). Over time, declines in cerebrovascular health and reduced perfusion of brain tissue lead to structural atrophy and altered brain function.
Cognitive effects
The cognitive profile of individuals with T2DM includes deficits in attention, processing speed, learning and memory, and executive function (e.g., Reaven et al., 1990 ; Brands et al., 2007 ; Yeung et al., 2009 ; Whitehead et al., 2011 ). Moreover, these individuals, and individuals with pre-diabetes (impaired glucose tolerance), show an accelerated trajectory of cognitive decline relative to that associated with healthy aging ( Gregg et al., 2000 ; Fontbonne et al., 2001 ; Arvanitakis et al., 2004 ; Yaffe et al., 2004 ; Fischer et al., 2009 ; Nooyens et al., 2010 ; Espeland et al., 2011 ; for conflicting results, see van den Berg et al., 2010 ).
Cognitive deficits in T2DM have been linked to multiple disease-related processes, including: (i) poor glucose control (i.e., hemoglobin A1c [HbA1c]; Ryan and Geckle, 2000 ; Kanaya et al., 2004 ; Cukierman-Yaffe et al., 2009 ; Maggi et al., 2009 ; Luchsinger et al., 2011 ; Tuligenga et al., 2014 ; for conflicting results, see Christman et al., 2011 ), (ii) glucose intolerance ( Rizzo et al., 2010 ; Zhong et al., 2012b ), (iii) high peripheral AGE levels ( Yaffe et al., 2011 ), (iv) high levels of inflammatory cytokines ( Marioni et al., 2010 ), and (v) peripheral hyperinsulinemia and insulin resistance ( Bruehl et al., 2010 ; Zhong et al., 2012a ). Even in non-diabetic adults, poorer glucoregulation has been associated with deficits and/or declines in verbal memory, working memory, processing speed, and executive function ( Dahle et al., 2009 ; Bruehl et al., 2010 ; Messier et al., 2010 , 2011 ; Ravona-Springer et al., 2012 ).
The link between cognitive impairment and poor metabolic control may be largely mediated by the structural and functional brain changes that occur in the presence of chronic insulin dysregulation and hyperglycemia. Associations between glucoregulation, hypoperfusion in temporal regions, hippocampal atrophy, and memory impairment have been observed in T2DM ( Gold et al., 2007 ; Last et al., 2007 ), and in non-diabetic adults with decreased peripheral glucose regulation ( Convit et al., 2003 ), or high fasting plasma glucose levels within the normal range ( Cherbuin et al., 2012 ; Kerti et al., 2013 ). In other studies of T2DM, cognitive deficits and structural brain atrophy were linked to cerebral hypoperfusion and altered vascular reactivity ( Last et al., 2007 ; Brundel et al., 2012 ), and disrupted default-mode network connectivity was associated with peripheral hyperinsulinemia, insulin resistance, and white matter integrity ( Musen et al., 2012 ; Hoogenboom et al., 2014 ). Regardless of the underlying cause, brain atrophy in T2DM is associated with poor cognition ( Moran et al., 2013 ), and cognitive declines have been associated with progression of brain atrophy over time ( van Elderen et al., 2010 ; Reijmer et al., 2011 ). Some studies suggest that structural changes may occur early in the course of T2DM; enlarged lateral ventricles, particularly within the frontal horns, have been observed less than a year after diagnosis ( Lee et al., 2013 ), and middle-aged, as well as older adults with T2DM, show reduced prefrontal volumes ( Bruehl et al., 2009 ) and generalized global atrophy ( de Bresser et al., 2010 ; Kamiyama et al., 2010 ; Espeland et al., 2013 ).
Hypertension
The brain is one of the most highly perfused organs. The cerebral hemispheres are supplied by capillary beds connected to the pial vasculature by penetrating arterioles, and the pial vasculature stems from a system of arteries branching off the anterior, middle, and posterior cerebral arteries. Maintenance of brain function depends on a constant blood supply through this network. Hypertension causes changes to the structure and function of these blood vessels, which impacts perfusion in affected areas. Hypoperfusion, for example, can interfere with the delivery of oxygen and nutrients required to meet metabolic demands, and makes hypertension a major risk factor for vascular cognitive impairment, stroke, and dementia.
Cerebrovascular changes
Hypertension places enormous stress on the cerebral circulation (for a comprehensive review, see Pires et al., 2013 ). A hallmark of chronic hypertension is increased vascular resistance, particularly in the small blood vessels that perfuse the brain. Vascular resistance increases as vessel walls thicken. This remodeling is an adaptive response required to maintain chronically increased blood pressure, but it decreases the interior space of the blood vessels (the lumen). Vascular resistance also increases as the number of blood vessels decrease. Rat models of hypertension have shown both of these effects: reductions in lumen diameter and in the number of capillaries making up capillary beds in the cerebral vasculature ( Sokolova et al., 1985 ).
Blood flow is reduced when vascular resistance is high, and chronic hypertension-mediated hypoperfusion has been linked to white matter degradation, gray matter atrophy, and cognitive deficits. Studies of older adults with hypertension show reduced blood flow, particularly in occipito-temporal, prefrontal, and medial temporal lobe regions ( Beason-Held et al., 2007 ), positive correlations between blood pressure and white matter burden ( White et al., 2011 ; Raji et al., 2012 ), and negative correlations between blood pressure and total brain volume ( Nagai et al., 2008 ). Blood vessel function is also impacted by hypertension. Cerebral autoregulation (i.e., the ability to maintain a constant perfusion rate over a range of arterial pressures) is impaired, as is cerebrovascular reactivity, the ability of blood vessels to dilate to accommodate increased blood flow demand ( Last et al., 2007 ; Hajjar et al., 2010 ).
The cognitive profile of older adults with hypertension includes poorer performance on tests of executive function, including verbal fluency, Trails B-A switching score, Stroop interference scores ( Bucur and Madden, 2010 ), slowed processing speed ( Dahle et al., 2009 ), and deficits in attention and memory (see Gifford et al., 2013 for a meta-analysis). Prospective cohort studies show that midlife cardiovascular risk factors like hypertension predict cognitive impairment in later life (e.g., Virta et al., 2013 ), and, similarly, cross-sectional studies show a relation between higher systolic blood pressure and poorer cognitive performance, even within the normotensive range, a relation that is particularly strong in midlife (e.g., Knecht et al., 2008 , 2009 ). Hypertension is associated with decreases in cognitive reserve ( Giordano et al., 2012 ), and older adults with MCI and cardiovascular risk factors like hypertension are twice as likely to develop dementia compared to those without such risk factors ( Johnson et al., 2010 ; Ettorre et al., 2012 ). Moreover, cognitive declines may be faster in those with MCI and hypertension, compared to those without hypertension ( Li et al., 2011 ; Goldstein et al., 2013 ).
The association between hypertension and cognitive decline appears to be strongest in executive and processing speed domains, and weakest in memory and language domains. Hypertension increased the risk of non-amnestic MCI, but not amnestic MCI, regardless of APOEε 4 genotype or hypertensive medication status ( Reitz et al., 2007 ), and predicted progression to dementia in non-amnestic MCI, but not amnestic or multi-domain MCI ( Oveisgharan and Hachinski, 2010 ). The impact of hypertension on executive and processing speed domains is consistent with studies that show a positive relation between hypertension and white matter changes ( Kennedy and Raz, 2009 ; Raz et al., 2012 ), and between white matter changes and deficits in processing speed, executive function, and attention, but not memory (e.g., Debette et al., 2011 ).
Cognitive deficits in hypertensive adults are linked to various indicators of vascular and brain health. There are correlations between white matter integrity and performance on tests of executive function and attention ( Hannesdottir et al., 2009 ), and between decreased flow-mediated dilation and poorer executive function ( Smith et al., 2011 ). Deficits in attention and psychomotor speed in late middle-aged adults with hypertension are associated with reductions in global brain perfusion, reductions that were not fully ameliorated following 6-months of antihypertensive treatment ( Efimova et al., 2008 ). Global cognitive decline has been linked to reduced cerebral blood flow in the face of white matter lesions and lacunar infarcts ( Kitagawa et al., 2009 ), to higher pulse pressure and arterial stiffness ( Scuteri et al., 2007 ; Waldstein et al., 2008 ; Triantafyllidi et al., 2009 ), and to hypertension-mediated deep-brain vascular pathology ( Yakushiji et al., 2012 ). In another large study of patients with MCI, those with hypertension and deep white matter lesions were at higher risk of dementia ( Clerici et al., 2012 ).
Conclusions
Taken together, these studies provide abundant evidence that middle-aged and older adults with T2DM and hypertension, relative to healthy older adults, are more likely to show signs of cognitive dysfunction, widespread structural atrophy, vascular damage, and functional changes. In light of their rising prevalence amongst older adults, there is an increasing likelihood that, without adequate screening at recruitment, individuals with T2DM and/or hypertension will be included in healthy older adult samples. This may introduce unwanted variability and bias to brain and/or cognitive measures, and increase the potential for type 1 and type 2 errors. Given the state of the neuroimaging literature on this topic and the need to advance our understanding, we view T2DM and hypertension as important new frontiers in cognitive neuroscience.
Moving forward, there is an opportunity to develop best practices when it comes to cognitive neuroscience research in older adult populations. Reconciling the vascular risk component in T2DM and hypertension may be the most tractable option since there are myriad approaches one can take to do this. The most rigorous approach in this respect may be inclusion of a breath-hold task, or a measure of cerebral blood flow (e.g., ASL) in the functional imaging protocol, as this allows for a direct estimate of each subject's vascular health. Breath-hold tasks can be used to index cerebrovascular reactivity in response to non-neuronal signals. The breath-hold period induces hypercapnia, which stimulates vasodilation and increases blood flow and blood volume in the brain, a signal change that occurs independently of neuronal activation. ASL or resting-state PET scans provide a direct measure of blood flow, and can be used to account for individual differences in perfusion. As noted above, these methods have already been used in some studies of cognitive aging to account for individual differences in cerebrovascular health. Whether other means of equating vascular risk across participants or across groups (e.g., screening participants for excessive white matter hyperintensities, post-hoc comparison of outcome measures or study groups on vascular risk factors, or statistical analyses aimed at controlling for the effects of vascular variability in the reported results) are similarly effective requires further study.
It may also be important for investigators to acknowledge a distinction between “healthy” and “typical” brain aging. Studies characterizing healthy aging should adopt T2DM and hypertension as exclusion criteria. Conversely, given the high prevalence of T2DM and hypertension in older adults, community- or population-based studies characterizing the typical trajectory of cognitive aging would benefit by including these participants to maximize the generalizability of results, and reconciling the heterogeneity through study design groups (e.g., stratifying based on diagnosis of T2DM and hypertension) or covariates in their analysis.
As the proportion of older adults living with T2DM and hypertension increase, it is imperative that functional imaging studies are designed to account for these population trends. The current state of the cognitive aging neuroimaging literature suggests that there is limited appreciation and/or awareness that T2DM and hypertension are significant medical illnesses that disrupt brain vasculature, brain structure, and brain function. By adopting best practices that take T2DM and hypertension into account, we can advance our understanding of these conditions, and of cognitive aging in general.
Author Contributions
Liesel-Ann C. Meusel selecting, indexing, and reviewing articles, writing of drafts; Nisha Kansal selecting articles, editing of drafts; Ekaterina Tchistiakova contributing to the first draft, editing of drafts; William Yuen selecting articles, contributing to the first draft, editing of drafts; Bradley J. MacIntosh provided conceptual foundation for paper, editing of drafts; Carol E. Greenwood provided conceptual foundation for paper, editing of drafts; Nicole D. Anderson provided conceptual foundation for paper, editing of drafts.
Conflict of Interest Statement
The authors declare that the research was conducted in the absence of any commercial or financial relationships that could be construed as a potential conflict of interest.
Acknowledgments
This research was supported in part by postdoctoral fellowships from the Centre for Stroke Recovery and the Alzheimer Society of Canada awarded to Liesel-Ann C. Meusel, and grant funds from CIHR (MOP111244).
Akter, K., Lanza, E. A., Martin, S. A., Myronyuk, N., Rua, M., and Raffa, R. B. (2011). Diabetes mellitus and Alzheimer's disease: shared pathology and treatment? Br. J. Clin. Pharmacol . 71, 365–376. doi: 10.1111/j.1365-2125.2010.03830.x
Pubmed Abstract | Pubmed Full Text | CrossRef Full Text
* 5,6,a Anderson, K. E., Lynch, K., Zarahn, E., Scarmeas, N., Van Heertum, R., Sackeim, H. et al. (2005). H215O PET study of impairment of nonverbal recognition with normal aging. J. Neuropsychiatry Clin. Neurosci . 17, 192–200. doi: 10.1176/appi.neuropsych.17.2.192
* 7,8,d Anguera, J. A., Reuter-Lorenz, P. A., Willingham, D. T., and Seidler, R. D. (2011). Failure to engage spatial working memory contributes to age-related declines in visuomotor learning. J. Cogn. Neurosci . 23, 11–25. doi: 10.1162/jocn.2010.21451
* 5,6,a Ansado, J., Monchi, O., Ennabil, N., Faure, S., and Joanette, Y. (2012). Load-dependent posterior-anterior shift in aging in complex visual selective attention situations. Brain Res . 1454, 14–22. doi: 10.1016/j.brainres.2012.02.061
* 5,6,a,□ Antonova, E., Parslow, D., Brammer, M., Dawson, G. R., Jackson, S. H. D., and Morris, R. G. (2009). Age-related neural activity during allocentric spatial memory. Memory 17, 125–143. doi: 10.1080/09658210802077348
Arvanitakis, Z., Wilson, R. S., Bienias, J. L., Evans, D. A., and Bennett, D. A. (2004). Diabetes mellitus and risk of Alzheimer disease and decline in cognitive function. Arch. Neurol . 61, 661–666. doi: 10.1001/archneur.61.5.661
* 5,6,b Bäckman, L., Karlsson, S., Fischer, H., Karlsson, P., Brehmer, Y., Rieckmann, A. et al. (2011). Dopamine D(1) receptors and age differences in brain activation during working memory. Neurobiol. Aging 32, 1849–1856. doi: 10.1016/j.neurobiolaging.2009.10.018
* 5,6,a Bagurdes, L. A., Mesulam, M. M., Gitelman, D. R., Weintraub, S., and Small, D. M. (2008). Modulation of the spatial attention network by incentives in healthy aging and mild cognitive impairment. Neuropsychologia 46, 2943–2948. doi: 10.1016/j.neuropsychologia.2008.06.005
* 5,6,c Bai, F., Liao, W., Watson, D. R., Shi, Y., Wang, Y., Yue, C. et al. (2011). Abnormal whole-brain functional connection in amnestic mild cognitive impairment patients. Behav. Brain Res . 216, 666–672. doi: 10.1016/j.bbr.2010.09.010
* 5,6,a Bai, F., Zhang, Z., Yu, H., Shi, Y., Yuan, Y., Zhu, W. et al. (2008). Default-mode network activity distinguishes amnestic type mild cognitive impairment from healthy aging: a combined structural and resting-state functional MRI study. Neurosci. Lett . 438, 111–115. doi: 10.1016/j.neulet.2008.04.021
Baker, L. D., Cross, D. J., Minoshima, S., Belongia, D., Watson, G. S., and Craft, S. (2011). Insulin resistance and Alzheimer-like reductions in regional cerebral glucose metabolism for cognitively normal adults with prediabetes or early type 2 diabetes. Arch. Neurol . 68, 51–57. doi: 10.1001/archneurol.2010.225
* 5,6,c,▴ Bangen, K. J., Kaup, A. R., Mirzakhanian, H., Wierenga, C. E., Jeste, D. V., and Eyler, L. T. (2012). Compensatory brain activity during encoding among older adults with better recognition memory for face-name pairs: an integrative functional, structural, and perfusion imaging study. J. Int. Neuropsychol. Soc . 18, 402–413. doi: 10.1017/S1355617712000197
* 9,12,b,▴ Bangen, K. J., Restom, K., Liu, T. T., Jak, A. J., Wierenga, C. E., Salmon, D. P. et al. (2009). Differential age effects on cerebral blood flow and BOLD response to encoding: associations with cognition and stroke risk. Neurobiol. Aging 30, 1276–1287. doi: 10.1016/j.neurobiolaging.2007.11.012
* 7,8,a,▾ Beason-Held, L. L., Kraut, M. A., and Resnick, S. M. (2008). I. Longitudinal changes in aging brain function. Neurobiol. Aging 29, 483–496. doi: 10.1016/j.neurobiolaging.2006.10.031
Beason-Held, L. L., Moghekar, A., Zonderman, A. B., Kraut, M. A., and Resnick, S. M. (2007). Longitudinal changes in cerebral blood flow in the older hypertensive brain. Stroke 38, 1766–1773. doi: 10.1161/STROKEAHA.106.477109
* 9,12,c,+ Beeri, M. S., Lee, H., Cheng, H., Wollman, D., Silverman, J. M., and Prohovnik, I. (2011). Memory activation in healthy nonagenarians. Neurobiol. Aging 32, 515–523. doi: 10.1016/j.neurobiolaging.2009.02.022
* 1,2,a Berlingeri, M., Bottini, G., Danelli, L., Ferri, F., Traficante, D., Sacheli, L. et al. (2010). With time on our side? Task-dependent compensatory processes in graceful aging. Exp. Brain Res . 205, 307–324. doi: 10.1007/s00221-010-2363-7
* 4,a Bernard, F. A., Desgranges, B., Eustache, F., and Baron, J.-C. (2007). Neural correlates of age-related verbal episodic memory decline: a PET study with combined subtraction/correlation analysis. Neurobiol. Aging 28, 1568–1576. doi: 10.1016/j.neurobiolaging.2006.07.004
* 5,6,a Bollinger, J., Rubens, M. T., Masangkay, E., Kalkstein, J., and Gazzaley, A. (2011). An expectation-based memory deficit in aging. Neuropsychologia 49, 1466–1475. doi: 10.1016/j.neuropsychologia.2010.12.021
Brands, A. M. A., Van den Berg, E., Manschot, S. M., Biessels, G. J., Kappelle, L. J., De Haan, E. H. F. et al. (2007). A detailed profile of cognitive dysfunction and its relation to psychological distress in patients with type 2 diabetes mellitus. J. Int. Neuropsychol. Soc . 13, 288–297. doi: 10.1017/S1355617707070312
* 2,5,a Braskie, M. N., Landau, S. M., Wilcox, C. E., Taylor, S. D., O'Neil, J. P., Baker, S. L. et al. (2011). Correlations of striatal dopamine synthesis with default network deactivations during working memory in younger adults. Hum. Brain Mapp . 32, 947–961. doi: 10.1002/hbm.21081
* 5,6,a Braskie, M. N., Small, G. W., and Bookheimer, S. Y. (2009). Entorhinal cortex structure and functional MRI response during an associative verbal memory task. Hum. Brain Mapp . 30, 3981–3992. doi: 10.1002/hbm.20823
* 1,10,b,❖ Braskie, M. N., Small, G. W., and Bookheimer, S. Y. (2010). Vascular health risks and fMRI activation during a memory task in older adults. Neurobiol. Aging 31, 1532–1542. doi: 10.1016/j.neurobiolaging.2008.08.016
Brown, C. M., Marthol, H., Zikeli, U., Ziegler, D., and Hilz, M. J. (2008). A simple deep breathing test reveals altered cerebral autoregulation in type 2 diabetic patients. Diabetologia 51, 756–761. doi: 10.1007/s00125-008-0958-3
Brownlee, M. (2005). The pathobiology of diabetic complications: a unifying mechanism. Diabetes 54, 1615–1625. doi: 10.2337/diabetes.54.6.1615
Bruehl, H., Sweat, V., Hassenstab, J., Polyakov, V., and Convit, A. (2010). Cognitive impairment in nondiabetic middle-aged and older adults is associated with insulin resistance. J. Clin. Exp. Neuropsychol . 32, 487–493. doi: 10.1080/13803390903224928
Bruehl, H., Wolf, O. T., Sweat, V., Tirsi, A., Richardson, S., and Convit, A. (2009). Modifiers of cognitive function and brain structure in middle-aged and elderly individuals with type 2 diabetes mellitus. Brain Res . 1280, 186–194. doi: 10.1016/j.brainres.2009.05.032
Brundel, M., van den Berg, E., Reijmer, Y. D., de Bresser, J., Kappelle, L. J., and Biessels, G. J. (2012). Cerebral haemodynamics, cognition and brain volumes in patients with type 2 diabetes. J. Diabetes Complicat . 26, 205–209. doi: 10.1016/j.jdiacomp.2012.03.021
Bucur, B., and Madden, D. J. (2010). Effects of adult age and blood pressure on executive function and speed of processing. Exp. Aging Res . 36, 153–168. doi: 10.1080/03610731003613482
* 7,9,a Burgmans, S., van Boxtel, M. P. J., Vuurman, E. F. P. M., Evers, E. A. T., and Jolles, J. (2010). Increased neural activation during picture encoding and retrieval in 60-year-olds compared to 20-year-olds. Neuropsychologia 48, 2188–2197. doi: 10.1016/j.neuropsychologia.2010.04.011
* 2,5,a Cabeza, R., Anderson, N. D., Locantore, J. K., and McIntosh, A. R. (2002). Aging gracefully: compensatory brain activity in high-performing older adults. Neuroimage 17, 1394–1402. doi: 10.1006/nimg.2002.1280
* 2,5,d Cabeza, R., Daselaar, S. M., Dolcos, F., Prince, S. E., Budde, M., and Nyberg, L. (2004). Task-independent and task-specific age effects on brain activity during working memory, visual attention and episodic retrieval. Cereb. Cortex 14, 364–375. doi: 10.1093/cercor/bhg133
* 2,5,a Cabeza, R., Grady, C. L., Nyberg, L., McIntosh, A. R., Tulving, E., Kapur, S. et al. (1997). Age-related differences in neural activity during memory encoding and retrieval: a positron emission tomography study. J. Neurosci . 17, 391–400.
Pubmed Abstract | Pubmed Full Text
* 7,8,a Campbell, K. L., Grady, C. L., Ng, C., and Hasher, L. (2012). Age differences in the frontoparietal cognitive control network: implications for distractibility. Neuropsychologia 50, 2212–2223. doi: 10.1016/j.neuropsychologia.2012.05.025
* 7,8,a Cappell, K. A., Gmeindl, L., and Reuter-Lorenz, P. A. (2010). Age differences in prefontal recruitment during verbal working memory maintenance depend on memory load. Cortex 46, 462–473. doi: 10.1016/j.cortex.2009.11.009
* 7,8,a Carlson, M. C., Erickson, K. I., Kramer, A. F., Voss, M. W., Bolea, N., Mielke, M. et al. (2009). Evidence for neurocognitive plasticity in at-risk older adults: the experience corps program. J. Gerontol. A Biol. Sci. Med. Sci . 64, 1275–1282. doi: 10.1093/gerona/glp117
Carlsson, C. M. (2010). Type 2 diabetes mellitus, dyslipidemia, and Alzheimer's disease. J. Alzheimers. Dis . 20, 711–722. doi: 10.3233/JAD-2010-100012
* 7,8,a Celone, K. A., Calhoun, V. D., Dickerson, B. C., Atri, A., Chua, E. F., Miller, S. L. et al. (2006). Alterations in memory networks in mild cognitive impairment and Alzheimer's disease: an independent component analysis. J. Neurosci . 26, 10222–10231. doi: 10.1523/JNEUROSCI.2250-06.2006
* 2,5,a Chen, N., Chou, Y., Song, A. W., and Madden, D. J. (2009). Measurement of spontaneous signal fluctuations in fMRI: adult age differences in intrinsic functional connectivity. Brain Struct. Funct . 213, 571–585. doi: 10.1007/s00429-009-0218-4
Cherbuin, N., Sachdev, P., and Anstey, K. J. (2012). Higher normal fasting plasma glucose is associated with hippocampal atrophy: the PATH Study. Neurology 79, 1019–1026. doi: 10.1212/WNL.0b013e31826846de
Christman, A. L., Matsushita, K., Gottesman, R. F., Mosley, T., Alonso, A., Coresh, J. et al. (2011). Glycated haemoglobin and cognitive decline: the Atherosclerosis Risk in Communities (ARIC) study. Diabetologia 54, 1645–1652. doi: 10.1007/s00125-011-2095-7
* 5,6,a Chua, E. F., Schacter, D. L., and Sperling, R. A. (2009). Neural basis for recognition confidence in younger and older adults. Psychol. Aging 24, 139–153. doi: 10.1037/a0014029
* 5,6,b Clément, F., and Belleville, S. (2009). Test-retest reliability of fMRI verbal episodic memory paradigms in healthy older adults and in persons with mild cognitive impairment. Hum. Brain Mapp . 30, 4033–4047. doi: 10.1002/hbm.20827
Clerici, F., Caracciolo, B., Cova, I., Fusari Imperatori, S., Maggiore, L., Galimberti, D. et al. (2012). Does vascular burden contribute to the progression of mild cognitive impairment to dementia? Dement. Geriatr. Cogn. Disord . 34, 235–243. doi: 10.1159/000343776
Colosia, A. D., Palencia, R., and Khan, S. (2013). Prevalence of hypertension and obesity in patients with type 2 diabetes mellitus in observational studies: a systematic literature review. Diabetes Metab. Syndr. Obes . 6, 327–338. doi: 10.2147/DMSO.S51325
Convit, A. (2005). Links between cognitive impairment in insulin resistance: an explanatory model. Neurobiol. Aging 26(Suppl. 1), 31–35. doi: 10.1016/j.neurobiolaging.2005.09.018
Convit, A., Wolf, O. T., Tarshish, C., and de Leon, M. J. (2003). Reduced glucose tolerance is associated with poor memory performance and hippocampal atrophy among normal elderly. Proc. Natl. Acad. Sci. U.S.A . 100, 2019–2022. doi: 10.1073/pnas.0336073100
* 7,8,b Cook, I. A., Bookheimer, S. Y., Mickes, L., Leuchter, A. F., and Kumar, A. (2007). Aging and brain activation with working memory tasks: an fMRI study of connectivity. Int. J. Geriatr. Psychiatry 22, 332–342. doi: 10.1002/gps.1678
Craft, S. (2006). Insulin resistance syndrome and Alzheimer disease: pathophysiologic mechanisms and therapeutic implications. Alzheimer Dis. Assoc. Disord . 20, 298–301. doi: 10.1097/01.wad.0000213866.86934.7e
Crane, P. K., Walker, R., Hubbard, R. A., Li, G., Nathan, D. M., Zheng, H. et al. (2013). Glucose levels and risk of dementia. N. Engl. J. Med . 369, 540–548. doi: 10.1056/NEJMoa1215740
Creavin, S. T., Gallacher, J., Bayer, A., Fish, M., Ebrahim, S., and Ben-Shlomo, Y. (2012). Metabolic syndrome, diabetes, poor cognition, and dementia in the Caerphilly prospective study. J. Alzheimers Dis . 28, 931–939. doi: 10.3233/JAD-2011-111550
Cui, Y., Jiao, Y., Chen, Y.-C., Wang, K., Gao, B., Wen, S. et al. (2014). Altered spontaneous brain activity in type 2 diabetes: a resting-state functional MRI study. Diabetes 63, 749–760. doi: 10.2337/db13-0519
Cukierman-Yaffe, T., Gerstein, H. C., Williamson, J. D., Lazar, R. M., Lovato, L., Miller, M. E. et al. (2009). Relationship between baseline glycemic control and cognitive function in individuals with type 2 diabetes and other cardiovascular risk factors: the action to control cardiovascular risk in diabetes-memory in diabetes (ACCORD-MIND) trial. Diabetes Care 32, 221–226. doi: 10.2337/dc08-1153
Dahle, C. L., Jacobs, B. S., and Raz, N. (2009). Aging, vascular risk, and cognition: blood glucose, pulse pressure, and cognitive performance in healthy adults. Psychol. Aging 24, 154–162. doi: 10.1037/a0014283
Danaei, G., Finucane, M. M., Lu, Y., Singh, G. M., Cowan, M. J., Paciorek, C. J. et al. (2011). National, regional, and global trends in fasting plasma glucose and diabetes prevalence since 1980: systematic analysis of health examination surveys and epidemiological studies with 370 country-years and 2·7 million participants. Lancet 378, 31–40. doi: 10.1016/S0140-6736(11)60679-X
* 2,5,d Daselaar, S. M., Fleck, M. S., Dobbins, I. G., Madden, D. J., and Cabeza, R. (2006). Effects of healthy aging on hippocampal and rhinal memory functions: an event-related fMRI study. Cereb. Cortex 16, 1771–1782. doi: 10.1093/cercor/bhj112
* 7,8,d Daselaar, S. M., Veltman, D. J., Rombouts, S. A. R. B., Raaijmakers, J. G. W., and Jonker, C. (2003). Neuroanatomical correlates of episodic encoding and retrieval in young and elderly subjects. Brain 126, 43–56. doi: 10.1093/brain/awg005
* 2,5,a Davis, S. W., Dennis, N. A., Daselaar, S. M., Fleck, M. S., and Cabeza, R. (2008). Que PASA? The posterior-anterior shift in aging. Cereb. Cortex 18, 1201–1209. doi: 10.1093/cercor/bhm155
Debette, S., Seshadri, S., Beiser, A., Au, R., Himali, J. J., Palumbo, C. et al. (2011). Midlife vascular risk factor exposure accelerates structural brain aging and cognitive decline. Neurology 77, 461–468. doi: 10.1212/WNL.0b013e318227b227
de Bresser, J., Tiehuis, A. M., van den Berg, E., Reijmer, Y. D., Jongen, C., Kappelle, L. J. et al. (2010). Progression of cerebral atrophy and white matter hyperintensities in patients with type 2 diabetes. Diabetes Care 33, 1309–1314. doi: 10.2337/dc09-1923
* 7,9,a de Chastelaine, M., Wang, T. H., Minton, B., Muftuler, L. T., and Rugg, M. D. (2011). The effects of age, memory performance, and callosal integrity on the neural correlates of successful associative encoding. Cereb. Cortex 21, 2166–2176. doi: 10.1093/cercor/bhq294
de la Monte, S. M., and Wands, J. R. (2008). Alzheimer's disease is type 3 diabetes-evidence reviewed. J. Diabetes Sci. Technol . 2, 1101–1113. doi: 10.1177/193229680800200619
* 5,6,a Dennis, N. A., and Cabeza, R. (2011). Age-related dedifferentiation of learning systems: an fMRI study of implicit and explicit learning. Neurobiol. Aging 32, 2318.e17–30. doi: 10.1016/j.neurobiolaging.2010.04.004
* 5,6,a Dennis, N. A., Daselaar, S., and Cabeza, R. (2007a). Effects of aging on transient and sustained successful memory encoding activity. Neurobiol. Aging 28, 1749–1758. doi: 10.1016/j.neurobiolaging.2006.07.006
* 5,6,a Dennis, N. A., Hayes, S. M., Prince, S. E., Madden, D. J., Huettel, S. A., and Cabeza, R. (2008a). Effects of aging on the neural correlates of successful item and source memory encoding. J. Exp. Psychol. Learn. Mem. Cogn . 34, 791–808. doi: 10.1037/0278-7393.34.4.791
* 5,6,a Dennis, N. A., Kim, H., and Cabeza, R. (2008b). Age-related differences in brain activity during true and false memory retrieval. J. Cogn. Neurosci . 20, 1390–1402. doi: 10.1162/jocn.2008.20096
* 5,6,a Dennis, N. A., Kim, H., and Cabeza, R. (2007b). Effects of aging on true and false memory formation: an fMRI study. Neuropsychologia 45, 3157–3166. doi: 10.1016/j.neuropsychologia.2007.07.003.
D'Esposito, M., Deouell, L. Y., and Gazzaley, A. (2003). Alterations in the BOLD fMRI signal with ageing and disease: a challenge for neuroimaging. Nat. Rev. Neurosci . 4, 863–872. doi: 10.1038/nrn1246
D'Esposito, M., Zarahn, E., Aguirre, G. K., and Rypma, B. (1999). The effect of normal aging on the coupling of neural activity to the bold hemodynamic response. Neuroimage 10, 6–14. doi: 10.1006/nimg.1999.0444
* 5,6,b,• Dickerson, B. C., Salat, D. H., Greve, D. N., Chua, E. F., Rand-Giovannetti, E., Rentz, D. M. et al. (2005). Increased hippocampal activation in mild cognitive impairment compared to normal aging and AD. Neurology 65, 404–411. doi: 10.1212/01.wnl.0000171450.97464.49
* 5,6,a,× DiGirolamo, G. J., Kramer, A. F., Barad, V., Cepeda, N. J., Weissman, D. H., Milham, M. P. et al. (2001). General and task-specific frontal lobe recruitment in older adults during executive processes: a fMRI investigation of task-switching. Neuroreport 12, 2065–2071. doi: 10.1097/00001756-200107030-00054
* 1,6,b,■ Donix, M., Poettrich, K., Weiss, P. H., Werner, A., von Kummer, R., Fink, G. R. et al. (2010). Age-dependent differences in the neural mechanisms supporting long-term declarative memories. Arch. Clin. Neuropsychol . 25, 383–395. doi: 10.1093/arclin/acq037
* 5,6,a Drobyshevsky, A., Baumann, S. B., and Schneider, W. (2006). A rapid fMRI task battery for mapping of visual, motor, cognitive, and emotional function. Neuroimage 31, 732–744. doi: 10.1016/j.neuroimage.2005.12.016
Du, X. L., Edelstein, D., Rossetti, L., Fantus, I. G., Goldberg, H., Ziyadeh, F. et al. (2000). Hyperglycemia-induced mitochondrial superoxide overproduction activates the hexosamine pathway and induces plasminogen activator inhibitor-1 expression by increasing Sp1 glycosylation. Proc. Natl. Acad. Sci. U.S.A . 97, 12222–12226. doi: 10.1073/pnas.97.22.12222
* 1,2,a,■ Duarte, A., Graham, K. S., and Henson, R. N. (2010). Age-related changes in neural activity associated with familiarity, recollection and false recognition. Neurobiol. Aging 31, 1814–1830. doi: 10.1016/j.neurobiolaging.2008.09.014
* 1,2,a,■ Duarte, A., Henson, R. N., and Graham, K. S. (2008). The effects of aging on the neural correlates of subjective and objective recollection. Cereb. Cortex 18, 2169–2180. doi: 10.1093/cercor/bhm243
* 3,5,a Dulas, M. R., and Duarte, A. (2011). The effects of aging on material-independent and material-dependent neural correlates of contextual binding. Neuroimage 57, 1192–1204. doi: 10.1016/j.neuroimage.2011.05.036
* 3,5,a Dulas, M. R., and Duarte, A. (2012). The effects of aging on material-independent and material-dependent neural correlates of source memory retrieval. Cereb. Cortex 22, 37–50. doi: 10.1093/cercor/bhr056
* 7,9,a Duverne, S., Motamedinia, S., and Rugg, M. D. (2009). The relationship between aging, performance, and the neural correlates of successful memory encoding. Cereb. Cortex 19, 733–744. doi: 10.1093/cercor/bhn122
Efimova, I. Y., Efimova, N. Y., Triss, S. V., and Lishmanov, Y. B. (2008). Brain perfusion and cognitive function changes in hypertensive patients. Hypertens. Res . 31, 673–678. doi: 10.1291/hypres.31.673
* 1,2,a Emery, L., Heaven, T. J., Paxton, J. L., and Braver, T. S. (2008). Age-related changes in neural activity during performance matched working memory manipulation. Neuroimage 42, 1577–1586. doi: 10.1016/j.neuroimage.2008.06.021
* 7,8,a Erickson, K. I., Colcombe, S. J., Wadhwa, R., Bherer, L., Peterson, M. S., Scalf, P. E. et al. (2007). Training-induced plasticity in older adults: effects of training on hemispheric asymmetry. Neurobiol. Aging 28, 272–283. doi: 10.1016/j.neurobiolaging.2005.12.012
Espeland, M. A., Bryan, R. N., Goveas, J. S., Robinson, J. G., Siddiqui, M. S., Liu, S. et al. (2013). Influence of type 2 diabetes on brain volumes and changes in brain volumes: results from the Women's Health Initiative Magnetic Resonance Imaging studies. Diabetes Care 36, 90–97. doi: 10.2337/dc12-0555
Espeland, M. A., Miller, M. E., Goveas, J. S., Hogan, P. E., Coker, L. H., Williamson, J. et al. (2011). Cognitive function and fine motor speed in older women with diabetes mellitus: results from the women's health initiative study of cognitive aging. J. Womens. Health (Larchmt) . 20, 1435–1443. doi: 10.1089/jwh.2011.2812
Ettorre, E., Cerra, E., Marigliano, B., Vigliotta, M., Vulcano, A., Fossati, C. et al. (2012). Role of cardiovascular risk factors (CRF) in the patients with mild cognitive impairment (MCI). Arch. Gerontol. Geriatr . 54, 330–332. doi: 10.1016/j.archger.2011.04.025
* 5,6,a Fakhri, M., Sikaroodi, H., Maleki, F., Ali Oghabian, M., and Ghanaati, H. (2012). Age-related frontal hyperactivation observed across different working memory tasks: an fMRI study. Behav. Neurol . 25, 351–361. doi: 10.3233/BEN-2012-120280
* 4,a,• Fera, F., Weickert, T. W., Goldberg, T. E., Tessitore, A., Hariri, A., Das, S. et al. (2005). Neural mechanisms underlying probabilistic category learning in normal aging. J. Neurosci . 25, 11340–11348. doi: 10.1523/JNEUROSCI.2736-05.2005
* 7,9,a Fernandes, M. A., Pacurar, A., Moscovitch, M., and Grady, C. (2006). Neural correlates of auditory recognition under full and divided attention in younger and older adults. Neuropsychologia 44, 2452–2464. doi: 10.1016/j.neuropsychologia.2006.04.020
* 2,11,a,■,▴ Filippini, N., Ebmeier, K. P., MacIntosh, B. J., Trachtenberg, A. J., Frisoni, G. B., Wilcock, G. K. et al. (2011). Differential effects of the APOE genotype on brain function across the lifespan. Neuroimage 54, 602–610. doi: 10.1016/j.neuroimage.2010.08.009
* 2,12,a,■,• Filippini, N., Nickerson, L. D., Beckmann, C. F., Ebmeier, K. P., Frisoni, G. B., Matthews, P. M. et al. (2012). Age-related adaptations of brain function during a memory task are also present at rest. Neuroimage 59, 3821–3828. doi: 10.1016/j.neuroimage.2011.11.063
Fischer, A. L., de Frias, C. M., Yeung, S. E., and Dixon, R. A. (2009). Short-term longitudinal trends in cognitive performance in older adults with type 2 diabetes. J. Clin. Exp. Neuropsychol . 31, 809–822. doi: 10.1080/13803390802537636
Fontbonne, A., Berr, C., Ducimetière, P., and Alpérovitch, A. (2001). Changes in cognitive abilities over a 4-year period are unfavorably affected in elderly diabetic subjects: results of the Epidemiology of Vascular Aging Study. Diabetes Care 24, 366–370. doi: 10.2337/diacare.24.2.366
* 3,5,a Gandini, D., Lemaire, P., Anton, J.-L., and Nazarian, B. (2008). Neural correlates of approximate quantification strategies in young and older adults: an fMRI study. Brain Res . 1246, 144–157. doi: 10.1016/j.brainres.2008.09.096
* 5,6,d,■ Garrett, D. D., Kovacevic, N., McIntosh, A. R., and Grady, C. L. (2011). The importance of being variable. J. Neurosci . 31, 4496–4503. doi: 10.1523/JNEUROSCI.5641-10.2011
* 3,5,a,□ Gazzaley, A., Cooney, J. W., Rissman, J., and D'Esposito, M. (2005). Top-down suppression deficit underlies working memory impairment in normal aging. Nat. Neurosci . 8, 1298–1300. doi: 10.1038/nn1543
Giacco, F., and Brownlee, M. (2010). Oxidative stress and diabetic complications. Circ. Res . 107, 1058–1070. doi: 10.1161/CIRCRESAHA.110.223545
Gifford, K. A., Badaracco, M., Liu, D., Tripodis, Y., Gentile, A., Lu, Z. et al. (2013). Blood pressure and cognition among older adults: a meta-analysis. Arch. Clin. Neuropsychol . 28, 649–664. doi: 10.1093/arclin/act046
* 7,8,a,■ Gigi, A., Babai, R., Penker, A., Hendler, T., and Korczyn, A. D. (2010). Prefrontal compensatory mechanism may enable normal semantic memory performance in mild cognitive impairment (MCI). J. Neuroimaging 20, 163–168. doi: 10.1111/j.1552-6569.2009.00386.x
Giordano, N., Tikhonoff, V., Palatini, P., Bascelli, A., Boschetti, G., De Lazzari, F. et al. (2012). Cognitive functions and cognitive reserve in relation to blood pressure components in a population-based cohort aged 53 to 94 years. Int. J. Hypertens . 2012:274851. doi: 10.1155/2012/274851
* 5,6,a,+ Giovanello, K. S., De Brigard, F., Hennessey Ford, J., Kaufer, D. I., Burke, J. R., Browndyke, J. N. et al. (2012). Event-related functional magnetic resonance imaging changes during relational retrieval in normal aging and amnestic mild cognitive impairment. J. Int. Neuropsychol. Soc . 18, 886–897. doi: 10.1017/S1355617712000689
* 5,6,a Giovanello, K. S., Kensinger, E. A., Wong, A. T., and Schacter, D. L. (2010). Age-related neural changes during memory conjunction errors. J. Cogn. Neurosci . 22, 1348–1361. doi: 10.1162/jocn.2009.21274
Gold, S. M., Dziobek, I., Sweat, V., Tirsi, A., Rogers, K., Bruehl, H. et al. (2007). Hippocampal damage and memory impairments as possible early brain complications of type 2 diabetes. Diabetologia 50, 711–719. doi: 10.1007/s00125-007-0602-7
* 5,6,b Gold, B. T., Jiang, Y., Jicha, G. A., and Smith, C. D. (2010a). Functional response in ventral temporal cortex differentiates mild cognitive impairment from normal aging. Hum. Brain Mapp . 31, 1249–1259. doi: 10.1002/hbm.20932
* 2,7,d,■ Gold, B. T., Powell, D. K., Xuan, L., Jicha, G. A., and Smith, C. D. (2010b). Age-related slowing of task switching is associated with decreased integrity of frontoparietal white matter. Neurobiol. Aging 31, 512–522. doi: 10.1016/j.neurobiolaging.2008.04.005
Goldstein, F. C., Levey, A. I., and Steenland, N. K. (2013). High blood pressure and cognitive decline in mild cognitive impairment. J. Am. Geriatr. Soc . 61, 67–73. doi: 10.1111/jgs.12067
Gorelick, P. B., Scuteri, A., Black, S. E., DeCarli, C., Greenberg, S. M., Iadecola, C. et al. (2011). Vascular contributions to cognitive impairment and dementia: a statement for healthcare professionals from the American Heart Association/American Stroke Association. Stroke 42, 2672–2713. doi: 10.1161/STR.0b013e3182299496
* 4, a,• Gould, R. L., Brown, R. G., Owen, A. M., Ffytche, D. H., and Howard, R. J. (2003). fMRI BOLD response to increasing task difficulty during successful paired associates learning. Neuroimage 20, 1006–1019. doi: 10.1016/S1053-8119(03)00365-3
* 1,9,a Grady, C. L., Grigg, O., and Ng, C. (2012). Age differences in default and reward networks during processing of personally relevant information. Neuropsychologia 50, 1682–1697. doi: 10.1016/j.neuropsychologia.2012.03.024
* 5,6,d,■,• Grady, C. L., Protzner, A. B., Kovacevic, N., Strother, S. C., Afshin-Pour, B., Wojtowicz, M. et al. (2010). A multivariate analysis of age-related differences in default mode and task-positive networks across multiple cognitive domains. Cereb. Cortex 20, 1432–1447. doi: 10.1093/cercor/bhp207
* 7,9,a,■,• Grady, C. L., Springer, M. V., Hongwanishkul, D., McIntosh, A. R., and Winocur, G. (2006). Age-related changes in brain activity across the adult lifespan. J. Cogn. Neurosci . 18, 227–241. doi: 10.1162/089892906775783705
Granic, I., Dolga, A. M., Nijholt, I. M., van Dijk, G., and Eisel, U. L. M. (2009). Inflammation and NF-kappaB in Alzheimer's disease and diabetes. J. Alzheimers Dis . 16, 809–821. doi: 10.3233/JAD-2009-0976
Gregg, E. W., Yaffe, K., Cauley, J. A., Rolka, D. B., Blackwell, T. L., Narayan, K. M. et al. (2000). Is diabetes associated with cognitive impairment and cognitive decline among older women? Study of Osteoporotic Fractures Research Group. Arch. Intern. Med . 160, 174–180. doi: 10.1001/archinte.160.2.174
* 5,6,c Grön, G., Bittner, D., Schmitz, B., Wunderlich, A. P., Tomczak, R., and Riepe, M. W. (2003). Variability in memory performance in aged healthy individuals: an fMRI study. Neurobiol. Aging 24, 453–462. doi: 10.1016/S0197-4580(02)00128-8
* 6,7,a,□ Grossman, M., Cooke, A., DeVita, C., Alsop, D., Detre, J., Chen, W. et al. (2002). Age-related changes in working memory during sentence comprehension: an fMRI study. Neuroimage 15, 302–317. doi: 10.1006/nimg.2001.0971
Hajjar, I., Zhao, P., Alsop, D., and Novak, V. (2010). Hypertension and cerebral vasoreactivity: a continuous arterial spin labeling magnetic resonance imaging study. Hypertension 56, 859–864. doi: 10.1161/HYPERTENSIONAHA.110.160002
* 5,6,a Hampstead, B. M., Stringer, A. Y., Stilla, R. F., Amaraneni, A., and Sathian, K. (2011). Where did I put that? Patients with amnestic mild cognitive impairment demonstrate widespread reductions in activity during the encoding of ecologically relevant object-location associations. Neuropsychologia 49, 2349–2361. doi: 10.1016/j.neuropsychologia.2011.04.008
* 5,6,a Hampstead, B. M., Stringer, A. Y., Stilla, R. F., Giddens, M., and Sathian, K. (2012). Mnemonic strategy training partially restores hippocampal activity in patients with mild cognitive impairment. Hippocampus 22, 1652–1658. doi: 10.1002/hipo.22006
* 7,8,a Han, S. D., Arfanakis, K., Fleischman, D. A., Leurgans, S. E., Tuminello, E. R., Edmonds, E. C. et al. (2012a). Functional connectivity variations in mild cognitive impairment: associations with cognitive function. J. Int. Neuropsychol. Soc . 18, 39–48. doi: 10.1017/S1355617711001299
* 4, b Han, Y., Lui, S., Kuang, W., Lang, Q., Zou, L., and Jia, J. (2012b). Anatomical and functional deficits in patients with amnestic mild cognitive impairment. PLoS ONE 7:e28664. doi: 10.1371/journal.pone.0028664
Hannesdottir, K., Nitkunan, A., Charlton, R. A., Barrick, T. R., MacGregor, G. A., and Markus, H. S. (2009). Cognitive impairment and white matter damage in hypertension: a pilot study. Acta Neurol. Scand . 119, 261–268. doi: 10.1111/j.1600-0404.2008.01098.x
* 7,8,a Hartley, A. A., Jonides, J., and Sylvester, C.-Y. C. (2011). Dual-task processing in younger and older adults: similarities and differences revealed by fMRI. Brain Cogn . 75, 281–291. doi: 10.1016/j.bandc.2011.01.004
* 3,7,a,• Hedden, T., Van Dijk, K. R. A., Shire, E. H., Sperling, R. A., Johnson, K. A., and Buckner, R. L. (2012). Failure to modulate attentional control in advanced aging linked to white matter pathology. Cereb. Cortex 22, 1038–1051. doi: 10.1093/cercor/bhr172
Heni, M., Schöpfer, P., Peter, A., Sartorius, T., Fritsche, A., Synofzik, M. et al. (2013). Evidence for altered transport of insulin across the blood–brain barrier in insulin-resistant humans. Acta Diabetol . doi: 10.1007/s00592-013-0546-y. [Epub ahead of print].
* 5,9,b Holtzer, R., Rakitin, B. C., Steffener, J., Flynn, J., Kumar, A., and Stern, Y. (2009). Age effects on load-dependent brain activations in working memory for novel material. Brain Res . 1249, 148–161. doi: 10.1016/j.brainres.2008.10.009
Hoogenboom, W. S., Marder, T. J., Flores, V. L., Huisman, S., Eaton, H. P., Schneiderman, J. S. et al. (2014). Cerebral white matter integrity and resting-state functional connectivity in middle-aged patients with type 2 diabetes. Diabetes 63, 728–738. doi: 10.2337/db13-1219
* 5,6,a Hosseini, S. M. H., Rostami, M., Yomogida, Y., Takahashi, M., Tsukiura, T., and Kawashima, R. (2010). Aging and decision making under uncertainty: behavioral and neural evidence for the preservation of decision making in the absence of learning in old age. Neuroimage 52, 1514–1520. doi: 10.1016/j.neuroimage.2010.05.008
* 5,6,a Huang, C.-M., Polk, T. A., Goh, J. O., and Park, D. C. (2012). Both left and right posterior parietal activations contribute to compensatory processes in normal aging. Neuropsychologia 50, 55–66. doi: 10.1016/j.neuropsychologia.2011.10.022
* 5,6,a Hubert, V., Beaunieux, H., Chételat, G., Platel, H., Landeau, B., Viader, F. et al. (2009). Age-related changes in the cerebral substrates of cognitive procedural learning. Hum. Brain Mapp . 30, 1374–1386. doi: 10.1002/hbm.20605
* 4, c, • Iidaka, T., Sadato, N., Yamada, H., Murata, T., Omori, M., and Yonekura, Y. (2001). An fMRI study of the functional neuroanatomy of picture encoding in younger and older adults. Brain Res. Cogn. Brain Res . 11, 1–11. doi: 10.1016/S0926-6410(00)00058-6
* 1,2,b,♦ Jennings, J. R., van der Veen, F. M., and Meltzer, C. C. (2006). Verbal and spatial working memory in older individuals: a positron emission tomography study. Brain Res . 1092, 177–189. doi: 10.1016/j.brainres.2006.03.077
* 1,2,a Jimura, K., and Braver, T. S. (2010). Age-related shifts in brain activity dynamics during task switching. Cereb. Cortex 20, 1420–1431. doi: 10.1093/cercor/bhp206
* 5,6,a Jin, M., Pelak, V. S., and Cordes, D. (2012). Aberrant default mode network in subjects with amnestic mild cognitive impairment using resting-state functional MRI. Magn. Reson. Imaging 30, 48–61. doi: 10.1016/j.mri.2011.07.007
Johnson, E. L. (2012). Glycemic variability in type 2 diabetes mellitus: oxidative stress and macrovascular complications. Adv. Exp. Med. Biol . 771, 139–154.
* 5,6,a Johnson, M. K., Mitchell, K. J., Raye, C. L., and Greene, E. J. (2004). An age-related deficit in prefrontal cortical function associated with refreshing information. Psychol. Sci . 15, 127–132. doi: 10.1111/j.0963-7214.2004.01502009.x
Johnson, J. K., Pa, J., Boxer, A. L., Kramer, J. H., Freeman, K., and Yaffe, K. (2010). Baseline predictors of clinical progression among patients with dysexecutive mild cognitive impairment. Dement. Geriatr. Cogn. Disord . 30, 344–351. doi: 10.1159/000318836
* 9,11,a,■ Johnson, S. C., Schmitz, T. W., Asthana, S., Gluck, M. A., and Myers, C. (2008). Associative learning over trials activates the hippocampus in healthy elderly but not mild cognitive impairment. Neuropsychol. Dev. Cogn. B Aging Neuropsychol. Cogn . 15, 129–145. doi: 10.1080/13825580601139444
* 10,12,c Jones, D. T., Machulda, M. M., Vemuri, P., McDade, E. M., Zeng, G., Senjem, M. L. et al. (2011). Age-related changes in the default mode network are more advanced in Alzheimer disease. Neurology 77, 1524–1531. doi: 10.1212/WNL.0b013e318233b33d
Kaiser, N., Sasson, S., Feener, E. P., Boukobza-Vardi, N., Higashi, S., Moller, D. E. et al. (1993). Differential regulation of glucose transport and transporters by glucose in vascular endothelial and smooth muscle cells. Diabetes 42, 80–89. doi: 10.2337/diab.42.1.80
Kalani, M. (2008). The importance of endothelin-1 for microvascular dysfunction in diabetes. Vasc. Health Risk Manag . 4, 1061–1068. doi: 10.2147/VHRM.S3920
* 5,6,a Kalkstein, J., Checksfield, K., Bollinger, J., and Gazzaley, A. (2011). Diminished top-down control underlies a visual imagery deficit in normal aging. J. Neurosci . 31, 15768–15774. doi: 10.1523/JNEUROSCI.3209-11.2011
Kamiyama, K., Wada, A., Sugihara, M., Kurioka, S., Hayashi, K., Hayashi, T. et al. (2010). Potential hippocampal region atrophy in diabetes mellitus type 2: a voxel-based morphometry VSRAD study. Jpn. J. Radiol . 28, 266–272. doi: 10.1007/s11604-009-0416-2
Kanaya, A. M., Barrett-Connor, E., Gildengorin, G., and Yaffe, K. (2004). Change in cognitive function by glucose tolerance status in older adults: a 4-year prospective study of the Rancho Bernardo study cohort. Arch. Intern. Med . 164, 1327–1333. doi: 10.1001/archinte.164.12.1327
* 5,6,a,° Kannurpatti, S. S., Motes, M. A., Rypma, B., and Biswal, B. B. (2010). Neural and vascular variability and the fMRI-BOLD response in normal aging. Magn. Reson. Imaging 28, 466–476. doi: 10.1016/j.mri.2009.12.007
* 5,6,a,° Kannurpatti, S. S., Motes, M. A., Rypma, B., and Biswal, B. B. (2011). Increasing measurement accuracy of age-related BOLD signal change: minimizing vascular contributions by resting-state-fluctuation-of-amplitude scaling. Hum. Brain Mapp . 32, 1125–1140. doi: 10.1002/hbm.21097
* 5,6,a Kaufmann, L., Ischebeck, A., Weiss, E., Koppelstaetter, F., Siedentopf, C., Vogel, S. E. et al. (2008). An fMRI study of the numerical Stroop task in individuals with and without minimal cognitive impairment. Cortex 44, 1248–1255. doi: 10.1016/j.cortex.2007.11.009
Kearney, P. M., Whelton, M., Reynolds, K., Muntner, P., Whelton, P. K., and He, J. (2005). Global burden of hypertension: analysis of worldwide data. Lancet 365, 217–223. doi: 10.1016/S0140-6736(05)17741-1
Kennedy, K. M., and Raz, N. (2009). Pattern of normal age-related regional differences in white matter microstructure is modified by vascular risk. Brain Res . 1297, 41–56. doi: 10.1016/j.brainres.2009.08.058
* 7,8,a Kennedy, K. M., Rodrigue, K. M., Devous, M. D. Sr., Hebrank, A. C., Bischof, G. N., and Park, D. C. (2012). Effects of beta-amyloid accumulation on neural function during encoding across the adult lifespan. Neuroimage 62, 1–8. doi: 10.1016/j.neuroimage.2012.03.077
Kerti, L., Witte, A. V., Winkler, A., Grittner, U., Rujescu, D., and Floel, A. (2013). Higher glucose levels associated with lower memory and reduced hippocampal microstructure. Neurology 81, 1746–1752. doi: 10.1212/01.wnl.0000435561.00234.ee
* 9,11,a,■,× Kikuchi, M., Hirosawa, T., Yokokura, M., Yagi, S., Mori, N., Yoshikawa, E. et al. (2011). Effects of brain amyloid deposition and reduced glucose metabolism on the default mode of brain function in normal aging. J. Neurosci . 31, 11193–11199. doi: 10.1523/JNEUROSCI.2535-11.2011
Kim, E., Cho, M. H., Cha, K. R., Park, J. S., Ahn, C.-W., Oh, B. H. et al. (2008). Interactive effect of central obesity and hypertension on cognitive function in older out-patients with Type 2 diabetes. Diabet. Med . 25, 1440–1446. doi: 10.1111/j.1464-5491.2008.02612.x
* 6,7,a Kim, S.-Y., and Giovanello, K. S. (2011). The effects of attention on age-related relational memory deficits: fMRI evidence from a novel attentional manipulation. J. Cogn. Neurosci . 23, 3637–3656. doi: 10.1162/jocn_a_00058
* 4,b Kircher, T., Weis, S., Leube, D., Freymann, K., Erb, M., Jessen, F. et al. (2008). Anterior hippocampus orchestrates successful encoding and retrieval of non-relational memory: an event-related fMRI study. Eur. Arch. Psychiatry Clin. Neurosci . 258, 363–372. doi: 10.1007/s00406-008-0805-z
* 1,9,a Kirchhoff, B. A., Anderson, B. A., Barch, D. M., and Jacoby, L. L. (2012). Cognitive and neural effects of semantic encoding strategy training in older adults. Cereb. Cortex 22, 788–799. doi: 10.1093/cercor/bhr129
Kitagawa, K., Oku, N., Kimura, Y., Yagita, Y., Sakaguchi, M., Hatazawa, J. et al. (2009). Relationship between cerebral blood flow and later cognitive decline in hypertensive patients with cerebral small vessel disease. Hypertens. Res . 32, 816–820. doi: 10.1038/hr.2009.100
Kloppenborg, R. P., van den Berg, E., Kappelle, L. J., and Biessels, G. J. (2008). Diabetes and other vascular risk factors for dementia: which factor matters most? A systematic review. Eur. J. Pharmacol . 585, 97–108. doi: 10.1016/j.ejphar.2008.02.049
* 2,5,a Klostermann, E. C., Braskie, M. N., Landau, S. M., O'Neil, J. P., and Jagust, W. J. (2012). Dopamine and frontostriatal networks in cognitive aging. Neurobiol. Aging 33, 623.e15–24. doi: 10.1016/j.neurobiolaging.2011.03.002
Knecht, S., Wersching, H., Lohmann, H., Berger, K., and Ringelstein, E. B. (2009). How much does hypertension affect cognition? Explained variance in cross-sectional analysis of non-demented community-dwelling individuals in the SEARCH study. J. Neurol. Sci . 283, 149–152. doi: 10.1016/j.jns.2009.02.362
Knecht, S., Wersching, H., Lohmann, H., Bruchmann, M., Duning, T., Dziewas, R. et al. (2008). High-normal blood pressure is associated with poor cognitive performance. Hypertension 51, 663–668. doi: 10.1161/HYPERTENSIONAHA.107.105577
* 7,8,b,• Koch, W., Teipel, S., Mueller, S., Buerger, K., Bokde, A. L. W., Hampel, H. et al. (2010). Effects of aging on default mode network activity in resting state fMRI: does the method of analysis matter? Neuroimage 51, 280–287. doi: 10.1016/j.neuroimage.2009.12.008
* 7,8,a Krause, J. B., Taylor, J. G., Schmidt, D., Hautzel, H., Mottaghy, F. M., and Müller-Gärtner, H. W. (2000). Imaging and neural modelling in episodic and working memory processes. Neural Netw . 13, 847–859
* 1,9,a Kühn, S., Schmiedek, F., Schott, B., Ratcliff, R., Heinze, H.-J., Düzel, E. et al. (2011). Brain areas consistently linked to individual differences in perceptual decision-making in younger as well as older adults before and after training. J. Cogn. Neurosci . 23, 2147–2158. doi: 10.1162/jocn.2010.21564
* 7,8,a Kukolja, J., Thiel, C. M., Wilms, M., Mirzazade, S., and Fink, G. R. (2009). Ageing-related changes of neural activity associated with spatial contextual memory. Neurobiol. Aging 30, 630–645. doi: 10.1016/j.neurobiolaging.2007.08.015
* 6,7,a Kukolja, J., Thiel, C. M., Wolf, O. T., and Fink, G. R. (2008). Increased cortisol levels in cognitively challenging situations are beneficial in young but not older subjects. Psychopharmacology (Berl.) 201, 293–304. doi: 10.1007/s00213-008-1275-8
* 1,2,a,• Lamar, M., Yousem, D. M., and Resnick, S. M. (2004). Age differences in orbitofrontal activation: an fMRI investigation of delayed match and nonmatch to sample. Neuroimage 21, 1368–1376. doi: 10.1016/j.neuroimage.2003.11.018
* 7,8,a Langenecker, S. A., Briceno, E. M., Hamid, N. M., and Nielson, K. A. (2007). An evaluation of distinct volumetric and functional MRI contributions toward understanding age and task performance: a study in the basal ganglia. Brain Res . 1135, 58–68. doi: 10.1016/j.brainres.2006.11.068
* 5,6,a Langenecker, S. A., and Nielson, K. A. (2003). Frontal recruitment during response inhibition in older adults replicated with fMRI. Neuroimage 20, 1384–1392. doi: 10.1016/S1053-8119(03)00372-0
* 5,6,a Langenecker, S. A., Nielson, K. A., and Rao, S. M. (2004). fMRI of healthy older adults during Stroop interference. Neuroimage 21, 192–200. doi: 10.1016/j.neuroimage.2003.08.027
Last, D., Alsop, D. C., Abduljalil, A. M., Marquis, R. P., de Bazelaire, C., Hu, K. et al. (2007). Global and regional effects of type 2 diabetes on brain tissue volumes and cerebral vasoreactivity. Diabetes Care 30, 1193–1199. doi: 10.2337/dc06-2052
Launer, L. J., Ross, G. W., Petrovitch, H., Masaki, K., Foley, D., White, L. R. et al. (2000). Midlife blood pressure and dementia: the Honolulu-Asia aging study. Neurobiol. Aging 21, 49–55. doi: 10.1016/S0197-4580(00)00096-8
Lee, J. H., Yoon, S., Renshaw, P. F., Kim, T.-S., Jung, J. J., Choi, Y. et al. (2013). Morphometric changes in lateral ventricles of patients with recent-onset type 2 diabetes mellitus. PLoS ONE 8:e60515. doi: 10.1371/journal.pone.0060515
* 5,6,a Leshikar, E. D., Gutchess, A. H., Hebrank, A. C., Sutton, B. P., and Park, D. C. (2010). The impact of increased relational encoding demands on frontal and hippocampal function in older adults. Cortex 46, 507–521. doi: 10.1016/j.cortex.2009.07.011
* 5,6,a Li, C., Zheng, J., Wang, J., Gui, L., and Li, C. (2009a). An fMRI stroop task study of prefrontal cortical function in normal aging, mild cognitive impairment, and Alzheimer's disease. Curr. Alzheimer Res . 6, 525–530. doi: 10.2174/156720509790147142
Li, J., Wang, Y. J., Zhang, M., Xu, Z. Q., Gao, C. Y., Fang, C. Q. et al. (2011). Vascular risk factors promote conversion from mild cognitive impairment to Alzheimer disease. Neurology 76, 1485–1491. doi: 10.1212/WNL.0b013e318217e7a4
* 7,8,a Li, Z., Moore, A. B., Tyner, C., and Hu, X. (2009b). Asymmetric connectivity reduction and its relationship to “HAROLD” in aging brain. Brain Res . 1295, 149–158. doi: 10.1016/j.brainres.2009.08.004
Logothetis, N. K., Pauls, J., Augath, M., Trinath, T., and Oeltermann, A. (2001). Neurophysiological investigation of the basis of the fMRI signal. Nature 412, 150–157. doi: 10.1038/35084005
Luchsinger, J. A. (2008). Adiposity, hyperinsulinemia, diabetes and Alzheimer's disease: an epidemiological perspective. Eur. J. Pharmacol . 585, 119–129. doi: 10.1016/j.ejphar.2008.02.048
Luchsinger, J. A., Palmas, W., Teresi, J. A., Silver, S., Kong, J., Eimicke, J. P. et al. (2011). Improved diabetes control in the elderly delays global cognitive decline. J. Nutr. Health Aging 15, 445–449. doi: 10.1007/s12603-011-0057-x
* 3,5,a MacDonald, S. W. S., Nyberg, L., Sandblom, J., Fischer, H., and Bäckman, L. (2008). Increased response-time variability is associated with reduced inferior parietal activation during episodic recognition in aging. J. Cogn. Neurosci . 20, 779–786. doi: 10.1162/jocn.2008.20502
* 2,5,a,■ Madden, D. J., Costello, M. C., Dennis, N. A., Davis, S. W., Shepler, A. M., Spaniol, J. et al. (2010). Adult age differences in functional connectivity during executive control. Neuroimage 52, 643–657. doi: 10.1016/j.neuroimage.2010.04.249
* 1,2,d,■ Madden, D. J., Langley, L. K., Denny, L. L., Turkington, T. G., Provenzale, J. M., Hawk, T. C. et al. (2002a). Adult age differences in visual word identification: functional neuroanatomy by positron emission tomography. Brain Cogn . 49, 297–321. doi: 10.1006/brcg.2001.1502
* 2,5,d,■ Madden, D. J., Spaniol, J., Whiting, W. L., Bucur, B., Provenzale, J. M., Cabeza, R. et al. (2007). Adult age differences in the functional neuroanatomy of visual attention: a combined fMRI and DTI study. Neurobiol. Aging 28, 459–476. doi: 10.1016/j.neurobiolaging.2006.01.005
* 1,2,d,■ Madden, D. J., Turkington, T. G., Provenzale, J. M., Denny, L. L., Langley, L. K., Hawk, T. C. et al. (2002b). Aging and attentional guidance during visual search: functional neuroanatomy by positron emission tomography. Psychol. Aging 17, 24–43. doi: 10.1037/0882-7974.17.1.24
Maggi, S., Limongi, F., Noale, M., Romanato, G., Tonin, P., Rozzini, R. et al. (2009). Diabetes as a risk factor for cognitive decline in older patients. Dement. Geriatr. Cogn. Disord . 27, 24–33. doi: 10.1159/000183842
* 1,9,a Maillet, D., and Rajah, M. N. (2011). Age-related changes in the three-way correlation between anterior hippocampus volume, whole-brain patterns of encoding activity and subsequent context retrieval. Brain Res . 1420, 68–79. doi: 10.1016/j.brainres.2011.08.071
Marioni, R. E., Strachan, M. W. J., Reynolds, R. M., Lowe, G. D. O., Mitchell, R. J., Fowkes, F. G. R. et al. (2010). Association between raised inflammatory markers and cognitive decline in elderly people with type 2 diabetes: the Edinburgh Type 2 Diabetes Study. Diabetes 59, 710–713. doi: 10.2337/db09-1163
* 3,5,b Mathis, A., Schunck, T., Erb, G., Namer, I. J., and Luthringer, R. (2009). The effect of aging on the inhibitory function in middle-aged subjects: a functional MRI study coupled with a color-matched Stroop task. Int. J. Geriatr. Psychiatry 24, 1062–1071. doi: 10.1002/gps.2222
* 4,a Mattay, V. S., Fera, F., Tessitore, A., Hariri, A. R., Berman, K. F., Das, S. et al. (2006). Neurophysiological correlates of age-related changes in working memory capacity. Neurosci. Lett . 392, 32–37. doi: 10.1016/j.neulet.2005.09.025
* 1,2,a,• Matthäus, F., Schmidt, J.-P., Banerjee, A., Schulze, T. G., Demirakca, T., and Diener, C. (2012). Effects of age on the structure of functional connectivity networks during episodic and working memory demand. Brain Connect . 2, 113–124. doi: 10.1089/brain.2012.0077
* 7,8,a,° Mayhew, S. D., Li, S., Storrar, J. K., Tsvetanov, K. A., and Kourtzi, Z. (2010). Learning shapes the representation of visual categories in the aging human brain. J. Cogn. Neurosci . 22, 2899–2912. doi: 10.1162/jocn.2010.21415
* 7,8,a,□ McGeown, W. J., Shanks, M. F., Forbes-McKay, K. E., and Venneri, A. (2009). Patterns of brain activity during a semantic task differentiate normal aging from early Alzheimer's disease. Psychiatry Res . 173, 218–227. doi: 10.1016/j.pscychresns.2008.10.005
* 5,6,a Meier, T. B., Desphande, A. S., Vergun, S., Nair, V. A., Song, J., Biswal, B. B. et al. (2012). Support vector machine classification and characterization of age-related reorganization of functional brain networks. Neuroimage 60, 601–613. doi: 10.1016/j.neuroimage.2011.12.052
* 7,9,c Meinzer, M., Flaisch, T., Seeds, L., Harnish, S., Antonenko, D., Witte, V. et al. (2012a). Same modulation but different starting points: performance modulates age differences in inferior frontal cortex activity during word-retrieval. PLoS ONE 7:e33631. doi: 10.1371/journal.pone.0033631
* 5,6,d Meinzer, M., Flaisch, T., Wilser, L., Eulitz, C., Rockstroh, B., Conway, T. et al. (2009). Neural signatures of semantic and phonemic fluency in young and old adults. J. Cogn. Neurosci . 21, 2007–2018. doi: 10.1162/jocn.2009.21219
* 7,9,c Meinzer, M., Seeds, L., Flaisch, T., Harnish, S., Cohen, M. L., McGregor, K. et al. (2012b). Impact of changed positive and negative task-related brain activity on word-retrieval in aging. Neurobiol. Aging 33, 656–669. doi: 10.1016/j.neurobiolaging.2010.06.020
Messier, C., Awad-Shimoon, N., Gagnon, M., Desrochers, A., and Tsiakas, M. (2011). Glucose regulation is associated with cognitive performance in young nondiabetic adults. Behav. Brain Res . 222, 81–88. doi: 10.1016/j.bbr.2011.03.023
Messier, C., Tsiakas, M., Gagnon, M., and Desrochers, A. (2010). Effect of age and glucoregulation on cognitive performance. J. Clin. Exp. Neuropsychol . 32, 809–821. doi: 10.1080/13803390903540323
* 7,9,a Meulenbroek, O., Kessels, R. P. C., de Rover, M., Petersson, K. M., Rikkert, M. G. M. O., Rijpkema, M. et al. (2010a). Age-effects on associative object-location memory. Brain Res . 1315, 100–110. doi: 10.1016/j.brainres.2009.12.011
* 1,2,a Meulenbroek, O., Petersson, K. M., Voermans, N., Weber, B., and Fernández, G. (2004). Age differences in neural correlates of route encoding and route recognition. Neuroimage 22, 1503–1514. doi: 10.1016/j.neuroimage.2004.04.007
* 7,8,a Meulenbroek, O., Rijpkema, M., Kessels, R. P. C., Rikkert, M. G. M. O., and Fernández, G. (2010b). Autobiographical memory retrieval in patients with Alzheimer's disease. Neuroimage 53, 331–340. doi: 10.1016/j.neuroimage.2010.05.082
* 7,8,b,■ Miettinen, P. S., Pihlajamäki, M., Jauhiainen, A. M., Niskanen, E., Hänninen, T., Vanninen, R. et al. (2011). Structure and function of medial temporal and posteromedial cortices in early Alzheimer's disease. Eur. J. Neurosci . 34, 320–330. doi: 10.1111/j.1460-9568.2011.07745.x
* 3,7,a,• Milham, M. P., Erickson, K. I., Banich, M. T., Kramer, A. F., Webb, A., Wszalek, T. et al. (2002). Attentional control in the aging brain: insights from an fMRI study of the stroop task. Brain Cogn . 49, 277–296. doi: 10.1006/brcg.2001.1501
* 5,6,a Mitchell, K. J., Johnson, M. K., Raye, C. L., and D'Esposito, M. (2000). fMRI evidence of age-related hippocampal dysfunction in feature binding in working memory. Brain Res. Cogn. Brain Res . 10, 197–206. doi: 10.1016/S0926-6410(00)00029-X
* 7,8,a Mitchell, K. J., Johnson, M. R., Higgins, J. A., and Johnson, M. K. (2010). Age differences in brain activity during perceptual versus reflective attention. Neuroreport 21, 293–297. doi: 10.1097/WNR.0b013e32833730d6
* 7,8,a Mitchell, K. J., Raye, C. L., Johnson, M. K., and Greene, E. J. (2006). An fMRI investigation of short-term source memory in young and older adults. Neuroimage 30, 627–633. doi: 10.1016/j.neuroimage.2005.09.039
* 1,2,a,▴ Mohtasib, R. S., Lumley, G., Goodwin, J. A., Emsley, H. C. A., Sluming, V., and Parkes, L. M. (2012). Calibrated fMRI during a cognitive Stroop task reveals reduced metabolic response with increasing age. Neuroimage 59, 1143–1151. doi: 10.1016/j.neuroimage.2011.07.092
Moran, C., Phan, T. G., Chen, J., Blizzard, L., Beare, R., Venn, A. et al. (2013). Brain atrophy in type 2 diabetes: regional distribution and influence on cognition. Diabetes Care 36, 4036–4042. doi: 10.2337/dc13-0143
* 3,5,a Morcom, A. M., and Friston, K. J. (2012). Decoding episodic memory in ageing: a Bayesian analysis of activity patterns predicting memory. Neuroimage 59, 1772–1782. doi: 10.1016/j.neuroimage.2011.08.071
* 3,5,a,□ Morcom, A. M., Good, C. D., Frackowiak, R. S. J., and Rugg, M. D. (2003). Age effects on the neural correlates of successful memory encoding. Brain 126, 213–229. doi: 10.1093/brain/awg020
* 5,6,a Mormino, E. C., Brandel, M. G., Madison, C. M., Marks, S., Baker, S. L., and Jagust, W. J. (2012). Aβ Deposition in aging is associated with increases in brain activation during successful memory encoding. Cereb. Cortex 22, 1813–1823. doi: 10.1093/cercor/bhr255
* 5,6,a Mormino, E. C., Smiljic, A., Hayenga, A. O., Onami, S. H., Greicius, M. D., Rabinovici, G. D. et al. (2011). Relationships between β-amyloid and functional connectivity in different components of the default mode network in aging. Cereb. Cortex 21, 2399–2407. doi: 10.1093/cercor/bhr025
Morris, J. K., Vidoni, E. D., Honea, R. A., and Burns, J. M. (2014). Impaired glycemia increases disease progression in mild cognitive impairment. Neurobiol. Aging 35, 585–589. doi: 10.1016/j.neurobiolaging.2013.09.033
* 5,6,a Mowinckel, A. M., Espeseth, T., and Westlye, L. T. (2012). Network-specific effects of age and in-scanner subject motion: a resting-state fMRI study of 238 healthy adults. Neuroimage 63, 1364–1373. doi: 10.1016/j.neuroimage.2012.08.004
* 5,6,a Murphy, K., and Garavan, H. (2004). Artifactual fMRI group and condition differences driven by performance confounds. Neuroimage 21, 219–228. doi: 10.1016/j.neuroimage.2003.09.016
* 3,7,b Murty, V. P., Sambataro, F., Das, S., Tan, H.-Y., Callicott, J. H., Goldberg, T. E. et al. (2009). Age-related alterations in simple declarative memory and the effect of negative stimulus valence. J. Cogn. Neurosci . 21, 1920–1933. doi: 10.1162/jocn.2009.21130
Musen, G., Jacobson, A. M., Bolo, N. R., Simonson, D. C., Shenton, M. E., McCartney, R. L. et al. (2012). Resting-state brain functional connectivity is altered in type 2 diabetes. Diabetes 61, 2375–2379. doi: 10.2337/db11-1669
Nagai, M., Hoshide, S., Ishikawa, J., Shimada, K., and Kario, K. (2008). Ambulatory blood pressure as an independent determinant of brain atrophy and cognitive function in elderly hypertension. J. Hypertens . 26, 1636–1641. doi: 10.1097/HJH.0b013e3283018333
* 7,8,a Nagel, I. E., Preuschhof, C., Li, S.-C., Nyberg, L., Bäckman, L., Lindenberger, U. et al. (2009). Performance level modulates adult age differences in brain activation during spatial working memory. Proc. Natl. Acad. Sci. U.S.A . 106, 22552–22557. doi: 10.1073/pnas.0908238106
* 10,12,a Nagel, I. E., Preuschhof, C., Li, S.-C., Nyberg, L., Bäckman, L., Lindenberger, U. et al. (2011). Load modulation of BOLD response and connectivity predicts working memory performance in younger and older adults. J. Cogn. Neurosci . 23, 2030–2045. doi: 10.1162/jocn.2010.21560
Nakae, J., Kido, Y., and Accili, D. (2001). Distinct and overlapping functions of insulin and IGF-I receptors. Endocr. Rev . 22, 818–835. doi: 10.1210/edrv.22.6.0452
Newcomer, J. W., and Haupt, D. W. (2006). The metabolic effects of antipsychotic medications. Can. J. Psychiatry 51, 480–491.
* 4, b, ■ Nichols, L. M., Masdeu, J. C., Mattay, V. S., Kohn, P., Emery, M., Sambataro, F. et al. (2012). Interactive effect of apolipoprotein e genotype and age on hippocampal activation during memory processing in healthy adults. Arch. Gen. Psychiatry 69, 804–813. doi: 10.1001/archgenpsychiatry.2011.1893
* 7,8,a,• Nielson, K. A., Douville, K. L., Seidenberg, M., Woodard, J. L., Miller, S. K., Franczak, M. et al. (2006). Age-related functional recruitment for famous name recognition: an event-related fMRI study. Neurobiol. Aging 27, 1494–1504. doi: 10.1016/j.neurobiolaging.2005.08.022
* 5,6,a Nielson, K. A., Langenecker, S. A., and Garavan, H. (2002). Differences in the functional neuroanatomy of inhibitory control across the adult life span. Psychol. Aging 17, 56–71. doi: 10.1037/0882-7974.17.1.56
* 3,5,a,□ Nielson, K. A., Langenecker, S. A., Ross, T. J., Garavan, H., Rao, S. M., and Stein, E. A. (2004). Comparability of functional MRI response in young and old during inhibition. Neuroreport 15, 129–133. doi: 10.1097/00001756-200401190-00025
Nishikawa, T., Edelstein, D., Du, X. L., Yamagishi, S., Matsumura, T., Kaneda, Y. et al. (2000). Normalizing mitochondrial superoxide production blocks three pathways of hyperglycaemic damage. Nature 404, 787–790. doi: 10.1038/35008121
Nooyens, A. C. J., Baan, C. A., Spijkerman, A. M. W., and Verschuren, W. M. M. (2010). Type 2 diabetes and cognitive decline in middle-aged men and women: the Doetinchem Cohort Study. Diabetes Care 33, 1964–1969. doi: 10.2337/dc09-2038
* 7,10,c,❖ Nordahl, C. W., Ranganath, C., Yonelinas, A. P., Decarli, C., Fletcher, E., and Jagust, W. J. (2006). White matter changes compromise prefrontal cortex function in healthy elderly individuals. J. Cogn. Neurosci . 18, 418–429. doi: 10.1162/089892906775990552
Novak, V., Last, D., Alsop, D. C., Abduljalil, A. M., Hu, K., Lepicovsky, L. et al. (2006). Cerebral blood flow velocity and periventricular white matter hyperintensities in type 2 diabetes. Diabetes Care 29, 1529–1534. doi: 10.2337/dc06-0261
* 5,6,a Nyberg, L., Dahlin, E., Stigsdotter Neely, A., and Bäckman, L. (2009). Neural correlates of variable working memory load across adult age and skill: dissociative patterns within the fronto-parietal network. Scand. J. Psychol . 50, 41–46. doi: 10.1111/j.1467-9450.2008.00678.x
* 7,8,a,• O'Brien, J. L., O'Keefe, K. M., LaViolette, P. S., DeLuca, A. N., Blacker, D., Dickerson, B. C. et al. (2010). Longitudinal fMRI in elderly reveals loss of hippocampal activation with clinical decline. Neurology 74, 1969–1976. doi: 10.1212/WNL.0b013e3181e3966e
* 7,8,a Osaka, M., Otsuka, Y., and Osaka, N. (2012a). Verbal to visual code switching improves working memory in older adults: an fMRI study. Front. Hum. Neurosci . 6:24. doi: 10.3389/fnhum.2012.00024
* 7,8,a Osaka, M., Yaoi, K., Otsuka, Y., Katsuhara, M., and Osaka, N. (2012b). Practice on conflict tasks promotes executive function of working memory in the elderly. Behav. Brain Res . 233, 90–98. doi: 10.1016/j.bbr.2012.04.044
* 7,8,a Otsuka, Y., Osaka, N., Morishita, M., Kondo, H., and Osaka, M. (2006). Decreased activation of anterior cingulate cortex in the working memory of the elderly. Neuroreport 17, 1479–1482. doi: 10.1097/01.wnr.0000236852.63092.9f
Oveisgharan, S., and Hachinski, V. (2010). Hypertension, executive dysfunction, and progression to dementia: the canadian study of health and aging. Arch. Neurol . 67, 187–192. doi: 10.1001/archneurol.2009.312
* 6,7,a Pacheco, J., Beevers, C. G., McGeary, J. E., and Schnyer, D. M. (2012). Memory monitoring performance and PFC activity are associated with 5-HTTLPR genotype in older adults. Neuropsychologia 50, 2257–2270. doi: 10.1016/j.neuropsychologia.2012.05.030
* 7,8,a Park, D. C., Welsh, R. C., Marshuetz, C., Gutchess, A. H., Mikels, J., Polk, T. A. et al. (2003). Working memory for complex scenes: age differences in frontal and hippocampal activations. J. Cogn. Neurosci . 15, 1122–1134. doi: 10.1162/089892903322598094
* 4, a, □ Park, J., Carp, J., Hebrank, A., Park, D. C., and Polk, T. A. (2010). Neural specificity predicts fluid processing ability in older adults. J. Neurosci . 30, 9253–9259. doi: 10.1523/JNEUROSCI.0853-10.2010
* 1,2,a Paxton, J. L., Barch, D. M., Racine, C. A., and Braver, T. S. (2008). Cognitive control, goal maintenance, and prefrontal function in healthy aging. Cereb. Cortex 18, 1010–1028. doi: 10.1093/cercor/bhm135
* 1,9,b Persson, J., Kalpouzos, G., Nilsson, L.-G., Ryberg, M., and Nyberg, L. (2011). Preserved hippocampus activation in normal aging as revealed by fMRI. Hippocampus 21, 753–766. doi: 10.1002/hipo.20794
* 7,10,a,+ Persson, J., Nyberg, L., Lind, J., Larsson, A., Nilsson, L.-G., Ingvar, M. et al. (2006). Structure-function correlates of cognitive decline in aging. Cereb. Cortex 16, 907–915. doi: 10.1093/cercor/bhj036
* 7,10,c,♦ Persson, J., Pudas, S., Lind, J., Kauppi, K., Nilsson, L.-G., and Nyberg, L. (2012). Longitudinal structure-function correlates in elderly reveal MTL dysfunction with cognitive decline. Cereb. Cortex 22, 2297–2304. doi: 10.1093/cercor/bhr306
* 2,5,a Persson, J., Sylvester, C.-Y. C., Nelson, J. K., Welsh, K. M., Jonides, J., and Reuter-Lorenz, P. A. (2004). Selection requirements during verb generation: differential recruitment in older and younger adults. Neuroimage 23, 1382–1390. doi: 10.1016/j.neuroimage.2004.08.004
* 7,8,a Petrella, J. R., Townsend, B. A., Jha, A. P., Ziajko, L. A., Slavin, M. J., Lustig, C. et al. (2005). Increasing memory load modulates regional brain activity in older adults as measured by fMRI. J. Neuropsychiatry Clin. Neurosci . 17, 75–83. doi: 10.1176/appi.neuropsych.17.1.75
* 5,6,a Piefke, M., Onur, Ö. A., and Fink, G. R. (2012). Aging-related changes of neural mechanisms underlying visual-spatial working memory. Neurobiol. Aging 33, 1284–1297. doi: 10.1016/j.neurobiolaging.2010.10.014
* 7,8,a Pihlajamäki, M., and Sperling, R. A. (2009). Functional MRI assessment of task-induced deactivation of the default mode network in Alzheimer's disease and at-risk older individuals. Behav. Neurol . 21, 77–91. doi: 10.3233/BEN-2009-0231
Pires, P. W., Dams Ramos, C. M., Matin, N., and Dorrance, A. M. (2013). The effects of hypertension on the cerebral circulation. Am. J. Physiol. Heart Circ. Physiol . 304, H1598–H1614. doi: 10.1152/ajpheart.00490.2012
* 4, c Podell, J. E., Sambataro, F., Murty, V. P., Emery, M. R., Tong, Y., Das, S. et al. (2012). Neurophysiological correlates of age-related changes in working memory updating. Neuroimage 62, 2151–2160. doi: 10.1016/j.neuroimage.2012.05.066
* 7,8,a Prakash, R. S., Erickson, K. I., Colcombe, S. J., Kim, J. S., Voss, M. W., and Kramer, A. F. (2009). Age-related differences in the involvement of the prefrontal cortex in attentional control. Brain Cogn . 71, 328–335. doi: 10.1016/j.bandc.2009.07.005
* 7,8,a Protzner, A. B., Mandzia, J. L., Black, S. E., and McAndrews, M. P. (2011). Network interactions explain effective encoding in the context of medial temporal damage in MCI. Hum. Brain Mapp . 32, 1277–1289. doi: 10.1002/hbm.21107
* 7,8,a,• Putcha, D., O'Keefe, K., LaViolette, P., O'Brien, J., Greve, D., Rentz, D. M. et al. (2011). Reliability of functional magnetic resonance imaging associative encoding memory paradigms in non-demented elderly adults. Hum. Brain Mapp . 32, 2027–2044. doi: 10.1002/hbm.21166
* 1,9,d Rajah, M. N., Languay, R., and Grady, C. L. (2011). Age-related changes in right middle frontal gyrus volume correlate with altered episodic retrieval activity. J. Neurosci . 31, 17941–17954. doi: 10.1523/JNEUROSCI.1690-11.2011
* 7,8,a,• Rajah, M. N., Languay, R., and Valiquette, L. (2010). Age-related changes in prefrontal cortex activity are associated with behavioural deficits in both temporal and spatial context memory retrieval in older adults. Cortex 46, 535–549. doi: 10.1016/j.cortex.2009.07.006
* 5,6,a,□ Rajah, M. N., and McIntosh, A. R. (2008). Age-related differences in brain activity during verbal recency memory. Brain Res . 1199, 111–125. doi: 10.1016/j.brainres.2007.12.051
Raji, C. A., Lopez, O. L., Kuller, L. H., Carmichael, O. T., Longstreth, W. T. Jr., Gach, H. M. et al. (2012). White matter lesions and brain gray matter volume in cognitively normal elders. Neurobiol. Aging 33, 834.e7–16. doi: 10.1016/j.neurobiolaging.2011.08.010
* 2,7,d,▴ Ramsøy, T. Z., Liptrot, M. G., Skimminge, A., Lund, T. E., Sidaros, K., Christensen, M. S. et al. (2012). Healthy aging attenuates task-related specialization in the human medial temporal lobe. Neurobiol. Aging 33, 1874–1889. doi: 10.1016/j.neurobiolaging.2011.09.032
* 6,7,a Rand-Giovannetti, E., Chua, E. F., Driscoll, A. E., Schacter, D. L., Albert, M. S., and Sperling, R. A. (2006). Hippocampal and neocortical activation during repetitive encoding in older persons. Neurobiol. Aging 27, 173–182. doi: 10.1016/j.neurobiolaging.2004.12.013
Ravona-Springer, R., Moshier, E., Schmeidler, J., Godbold, J., Akrivos, J., Rapp, M. et al. (2012). Changes in glycemic control are associated with changes in cognition in non-diabetic elderly. J. Alzheimers Dis . 30, 299–309. doi: 10.3233/JAD-2012-120106
* 5,6,a Raye, C. L., Mitchell, K. J., Reeder, J. A., Greene, E. J., and Johnson, M. K. (2008). Refreshing one of several active representations: behavioral and functional magnetic resonance imaging differences between young and older adults. J. Cogn. Neurosci . 20, 852–862. doi: 10.1162/jocn.2008.20508
Raz, N., Yang, Y., Dahle, C. L., and Land, S. (2012). Volume of white matter hyperintensities in healthy adults: contribution of age, vascular risk factors, and inflammation-related genetic variants. Biochim. Biophys. Acta 1822, 361–369. doi: 10.1016/j.bbadis.2011.08.007
Reaven, G. M., Thompson, L. W., Nahum, D., and Haskins, E. (1990). Relationship between hyperglycemia and cognitive function in older NIDDM patients. Diabetes Care 13, 16–21. doi: 10.2337/diacare.13.1.16
Regenold, W. T., Thapar, R. K., Marano, C., Gavirneni, S., and Kondapavuluru, P. V. (2002). Increased prevalence of type 2 diabetes mellitus among psychiatric inpatients with bipolar I affective and schizoaffective disorders independent of psychotropic drug use. J. Affect. Disord . 70, 19–26. doi: 10.1016/S0165-0327(01)00456-6
Reijmer, Y. D., van den Berg, E., de Bresser, J., Kessels, R. P. C., Kappelle, L. J., Algra, A. et al. (2011). Accelerated cognitive decline in patients with type 2 diabetes: MRI correlates and risk factors. Diabetes Metab. Res. Rev . 27, 195–202. doi: 10.1002/dmrr.1163
Reitz, C., Tang, M.-X., Manly, J., Mayeux, R., and Luchsinger, J. A. (2007). Hypertension and the risk of mild cognitive impairment. Arch. Neurol . 64, 1734–1740. doi: 10.1001/archneur.64.12.1734
* 5,6,a,▴ Restom, K., Bangen, K. J., Bondi, M. W., Perthen, J. E., and Liu, T. T. (2007). Cerebral blood flow and BOLD responses to a memory encoding task: a comparison between healthy young and elderly adults. Neuroimage 37, 430–439. doi: 10.1016/j.neuroimage.2007.05.024
* 7,8,a Rieckmann, A., Fischer, H., and Bäckman, L. (2010). Activation in striatum and medial temporal lobe during sequence learning in younger and older adults: relations to performance. Neuroimage 50, 1303–1312. doi: 10.1016/j.neuroimage.2010.01.015
* 7,8,a Rieckmann, A., Karlsson, S., Fischer, H., and Bäckman, L. (2011). Caudate dopamine D1 receptor density is associated with individual differences in frontoparietal connectivity during working memory. J. Neurosci . 31, 14284–14290. doi: 10.1523/JNEUROSCI.3114-11.2011
Rizzo, M. R., Marfella, R., Barbieri, M., Boccardi, V., Vestini, F., Lettieri, B. et al. (2010). Relationships between daily acute glucose fluctuations and cognitive performance among aged type 2 diabetic patients. Diabetes Care 33, 2169–2174. doi: 10.2337/dc10-0389
Roberts, R. O., Knopman, D. S., Geda, Y. E., Cha, R. H., Pankratz, V. S., Baertlein, L. et al. (2014). Association of diabetes with amnestic and nonamnestic mild cognitive impairment. Alzheimers. Dement . 10, 18–26. doi: 10.1016/j.jalz.2013.01.001
* 5,6,a Rombouts, S. A. R. B., Barkhof, F., Goekoop, R., Stam, C. J., and Scheltens, P. (2005a). Altered resting state networks in mild cognitive impairment and mild Alzheimer's disease: an fMRI study. Hum. Brain Mapp . 26, 231–239. doi: 10.1002/hbm.20160
* 5,6,a,• Rombouts, S. A. R. B., Goekoop, R., Stam, C. J., Barkhof, F., and Scheltens, P. (2005b). Delayed rather than decreased BOLD response as a marker for early Alzheimer's disease. Neuroimage 26, 1078–1085. doi: 10.1016/j.neuroimage.2005.03.022
* 7,10,b,❖ Rosano, C., Venkatraman, V. K., Guralnik, J., Newman, A. B., Glynn, N. W., Launer, L. et al. (2010). Psychomotor speed and functional brain MRI 2 years after completing a physical activity treatment. J. Gerontol. A Biol. Sci. Med. Sci . 65, 639–647. doi: 10.1093/gerona/glq038
* 3,5,a Rosen, A. C., Gabrieli, J. D. E., Stoub, T., Prull, M. W., O'Hara, R., Yesavage, J. et al. (2005). Relating medial temporal lobe volume to frontal fMRI activation for memory encoding in older adults. Cortex 41, 595–602. doi: 10.1016/S0010-9452(08)70199-0
Ryan, C. M., and Geckle, M. O. (2000). Circumscribed cognitive dysfunction in middle-aged adults with type 2 diabetes. Diabetes Care 23, 1486–1493. doi: 10.2337/diacare.23.10.1486
* 4, b Rypma, B., Berger, J. S., Genova, H. M., Rebbechi, D., and D'Esposito, M. (2005). Dissociating age-related changes in cognitive strategy and neural efficiency using event-related fMRI. Cortex 41, 582–594. doi: 10.1016/S0010-9452(08)70198-9
* 4, b Rypma, B., Eldreth, D. A., and Rebbechi, D. (2007). Age-related differences in activation-performance relations in delayed-response tasks: a multiple component analysis. Cortex 43, 65–76. doi: 10.1016/S0010-9452(08)70446-5
* 5,6,a Sala-Llonch, R., Arenaza-Urquijo, E. M., Valls-Pedret, C., Vidal-Piñeiro, D., Bargall,ó, N., Junqu,é, C. et al. (2012). Dynamic functional reorganizations and relationship with working memory performance in healthy aging. Front. Hum. Neurosci . 6, 152. doi: 10.3389/fnhum.2012.00152
* 5,6,b Salami, A., Eriksson, J., and Nyberg, L. (2012). Opposing effects of aging on large-scale brain systems for memory encoding and cognitive control. J. Neurosci . 32, 10749–10757. doi: 10.1523/JNEUROSCI.0278-12.2012
* 4, a, • Sambataro, F., Murty, V. P., Callicott, J. H., Tan, H.-Y., Das, S., Weinberger, D. R. et al. (2010). Age-related alterations in default mode network: impact on working memory performance. Neurobiol. Aging 31, 839–852. doi: 10.1016/j.neurobiolaging.2008.05.022
* 3,5,a Schneider-Garces, N. J., Gordon, B. A., Brumback-Peltz, C. R., Shin, E., Lee, Y., Sutton, B. P. et al. (2010). Span, CRUNCH, and beyond: working memory capacity and the aging brain. J. Cogn. Neurosci . 22, 655–669. doi: 10.1162/jocn.2009.21230
* 7,9,c Schulte, T., Müller-Oehring, E. M., Chanraud, S., Rosenbloom, M. J., Pfefferbaum, A., and Sullivan, E. V. (2011). Age-related reorganization of functional networks for successful conflict resolution: a combined functional and structural MRI study. Neurobiol. Aging 32, 2075–2090. doi: 10.1016/j.neurobiolaging.2009.12.002
Scuteri, A., Tesauro, M., Appolloni, S., Preziosi, F., Brancati, A. M., and Volpe, M. (2007). Arterial stiffness as an independent predictor of longitudinal changes in cognitive function in the older individual. J. Hypertens . 25, 1035–1040. doi: 10.1097/01.hjh.0000170384.38708.b7
* 7,8,a Shafto, M. A., Stamatakis, E. A., Tam, P. P., and Tyler, L. K. (2010). Word retrieval failures in old age: the relationship between structure and function. J. Cogn. Neurosci . 22, 1530–1540. doi: 10.1162/jocn.2009.21321
Shaikh, S., and Nicholson, L. F. B. (2008). Advanced glycation end products induce in vitro cross-linking of alpha-synuclein and accelerate the process of intracellular inclusion body formation. J. Neurosci. Res . 86, 2071–2082. doi: 10.1002/jnr.21644
Shaw, J. E., Sicree, R. A., and Zimmet, P. Z. (2010). Global estimates of the prevalence of diabetes for 2010 and 2030. Diabetes Res. Clin. Pract . 87, 4–14. doi: 10.1016/j.diabres.2009.10.007
* 5,6,b,• Siedlecki, K. L., Habeck, C. G., Brickman, A. M., Gazes, Y., and Stern, Y. (2009). Examining the multifactorial nature of cognitive aging with covariance analysis of positron emission tomography data. J. Int. Neuropsychol. Soc . 15, 973–981. doi: 10.1017/S1355617709990592
* 7,8,a Simon, J. R., Vaidya, C. J., Howard, J. H., and Howard, D. V. (2012). The effects of aging on the neural basis of implicit associative learning in a probabilistic triplets learning task. J. Cogn. Neurosci . 24, 451–463. doi: 10.1162/jocn_a_00116
Smith, P. J., Blumenthal, J. A., Babyak, M. A., Hinderliter, A., and Sherwood, A. (2011). Association of vascular health and neurocognitive performance in overweight adults with high blood pressure. J. Clin. Exp. Neuropsychol . 33, 559–566. doi: 10.1080/13803395.2010.537648
Sokolova, I. A., Manukhina, E. B., Blinkov, S. M., Koshelev, V. B., Pinelis, V. G., and Rodionov, I. M. (1985). Rarefication of the arterioles and capillary network in the brain of rats with different forms of hypertension. Microvasc. Res . 30, 1–9. doi: 10.1016/0026-2862(85)90032-9
* 2,5,d Solbakk, A.-K., Fuhrmann Alpert, G., Furst, A. J., Hale, L. A., Oga, T., Chetty, S. et al. (2008). Altered prefrontal function with aging: insights into age-associated performance decline. Brain Res . 1232, 30–47. doi: 10.1016/j.brainres.2008.07.060
* 5,6,a Solé-Padullés, C., Bartrés-Faz, D., Junqué, C., Vendrell, P., Rami, L., Clemente, I. C. et al. (2009). Brain structure and function related to cognitive reserve variables in normal aging, mild cognitive impairment and Alzheimer's disease. Neurobiol. Aging 30, 1114–1124. doi: 10.1016/j.neurobiolaging.2007.10.008
* 5,6,d,■ Spaniol, J., and Grady, C. (2012). Aging and the neural correlates of source memory: over-recruitment and functional reorganization. Neurobiol. Aging 33, 425.e3–18. doi: 10.1016/j.neurobiolaging.2010.10.005
* 6,7,a Sperling, R. A., Bates, J. F., Chua, E. F., Cocchiarella, A. J., Rentz, D. M., Rosen, B. R. et al. (2003). fMRI studies of associative encoding in young and elderly controls and mild Alzheimer's disease. J. Neurol. Neurosurg. Psychiatr . 74, 44–50. doi: 10.1136/jnnp.74.1.44
* 10,12,b Stebbins, G. T., Carrillo, M. C., Dorfman, J., Dirksen, C., Desmond, J. E., Turner, D. A. et al. (2002). Aging effects on memory encoding in the frontal lobes. Psychol. Aging 17, 44–55. doi: 10.1037/0882-7974.17.1.44
Steen, E., Terry, B. M., Rivera, E. J., Cannon, J. L., Neely, T. R., Tavares, R. et al. (2005). Impaired insulin and insulin-like growth factor expression and signaling mechanisms in Alzheimer's disease—is this type 3 diabetes? J. Alzheimers Dis . 7, 63–80.
* 5,6,b Steffener, J., Brickman, A. M., Rakitin, B. C., Gazes, Y., and Stern, Y. (2009). The impact of age-related changes on working memory functional activity. Brain Imaging Behav . 3, 142–153. doi: 10.1007/s11682-008-9056-x
* 5,6,a Stern, Y., Habeck, C., Moeller, J., Scarmeas, N., Anderson, K. E., Hilton, H. J. et al. (2005). Brain networks associated with cognitive reserve in healthy young and old adults. Cereb. Cortex 15, 394–402. doi: 10.1093/cercor/bhh142
* 4, a Stern, Y., Rakitin, B. C., Habeck, C., Gazes, Y., Steffener, J., Kumar, A. et al. (2012). Task difficulty modulates young-old differences in network expression. Brain Res . 1435, 130–145. doi: 10.1016/j.brainres.2011.11.061
* 7,8,a Stern, Y., Zarahn, E., Habeck, C., Holtzer, R., Rakitin, B. C., Kumar, A. et al. (2008). A common neural network for cognitive reserve in verbal and object working memory in young but not old. Cereb. Cortex 18, 959–967. doi: 10.1093/cercor/bhm134
* 4, a Stevens, W. D., Hasher, L., Chiew, K. S., and Grady, C. L. (2008). A neural mechanism underlying memory failure in older adults. J. Neurosci . 28, 12820–12824. doi: 10.1523/JNEUROSCI.2622-08.2008
* 7,9,a St Jacques, P. L., Rubin, D. C., and Cabeza, R. (2012). Age-related effects on the neural correlates of autobiographical memory retrieval. Neurobiol. Aging 33, 1298–1310. doi: 10.1016/j.neurobiolaging.2010.11.007
* 5,6,a Thiyagesh, S. N., Farrow, T. F. D., Parks, R. W., Accosta-Mesa, H., Young, C., Wilkinson, I. D. et al. (2009). The neural basis of visuospatial perception in Alzheimer's disease and healthy elderly comparison subjects: an fMRI study. Psychiatry Res . 172, 109–116. doi: 10.1016/j.pscychresns.2008.11.002
* 7,8,a Thomsen, T., Specht, K., Rimol, L. M., Hammar, A., Nyttingnes, J., Ersland, L. et al. (2004). Brain localization of attentional control in different age groups by combining functional and structural MRI. Neuroimage 22, 912–919. doi: 10.1016/j.neuroimage.2004.02.015
* 5,9,a Townsend, J., Adamo, M., and Haist, F. (2006). Changing channels: an fMRI study of aging and cross-modal attention shifts. Neuroimage 31, 1682–1692. doi: 10.1016/j.neuroimage.2006.01.045
Triantafyllidi, H., Arvaniti, C., Lekakis, J., Ikonomidis, I., Siafakas, N., Tzortzis, S. et al. (2009). Cognitive impairment is related to increased arterial stiffness and microvascular damage in patients with never-treated essential hypertension. Am. J. Hypertens . 22, 525–530. doi: 10.1038/ajh.2009.35
* 5,6,b Trivedi, M. A., Murphy, C. M., Goetz, C., Shah, R. C., Gabrieli, J. D. E., Whitfield-Gabrieli, S. et al. (2008a). fMRI activation changes during successful episodic memory encoding and recognition in amnestic mild cognitive impairment relative to cognitively healthy older adults. Dement. Geriatr. Cogn. Disord . 26, 123–137. doi: 10.1159/000148190
* 1,6,a Trivedi, M. A., Schmitz, T. W., Ries, M. L., Hess, T. M., Fitzgerald, M. E., Atwood, C. S. et al. (2008b). fMRI activation during episodic encoding and metacognitive appraisal across the lifespan: risk factors for Alzheimer's disease. Neuropsychologia 46, 1667–1678. doi: 10.1016/j.neuropsychologia.2007.11.035
* 4, b Trivedi, M. A., Stoub, T. R., Murphy, C. M., George, S., deToledo-Morrell, L., Shah, R. C. et al. (2011). Entorhinal cortex volume is associated with episodic memory related brain activation in normal aging and amnesic mild cognitive impairment. Brain Imaging Behav . 5, 126–136. doi: 10.1007/s11682-011-9117-4
* 5,6,a Tsukiura, T., Sekiguchi, A., Yomogida, Y., Nakagawa, S., Shigemune, Y., Kambara, T. et al. (2011). Effects of aging on hippocampal and anterior temporal activations during successful retrieval of memory for face-name associations. J. Cogn. Neurosci . 23, 200–213. doi: 10.1162/jocn.2010.21476
Tuligenga, R. H., Dugravot, A., Tabák, A. G., Elbaz, A., Brunner, E. J., Kivimäki, M. et al. (2014). Midlife type 2 diabetes and poor glycaemic control as risk factors for cognitive decline in early old age: a post-hoc analysis of the Whitehall II cohort study. Lancet Diabetes Endocrinol . 2, 228–235. doi: 10.1016/S2213-8587(13)70192-X.
* 7,8,a Tyler, L. K., Shafto, M. A., Randall, B., Wright, P., Marslen-Wilson, W. D., and Stamatakis, E. A. (2010). Preserving syntactic processing across the adult life span: the modulation of the frontotemporal language system in the context of age-related atrophy. Cereb. Cortex 20, 352–364. doi: 10.1093/cercor/bhp105
* 7,8,a Vallesi, A., McIntosh, A. R., and Stuss, D. T. (2009). Temporal preparation in aging: a functional MRI study. Neuropsychologia 47, 2876–2881. doi: 10.1016/j.neuropsychologia.2009.06.013
* 5,6,a,■ Vallesi, A., McIntosh, A. R., and Stuss, D. T. (2011). Overrecruitment in the aging brain as a function of task demands: evidence for a compensatory view. J. Cogn. Neurosci . 23, 801–815. doi: 10.1162/jocn.2010.21490
van den Berg, E., Reijmer, Y. D., de Bresser, J., Kessels, R. P. C., Kappelle, L. J., and Biessels, G. J. (2010). A 4 year follow-up study of cognitive functioning in patients with type 2 diabetes mellitus. Diabetologia 53, 58–65. doi: 10.1007/s00125-009-1571-9
* 4, a Vandenbroucke, M. W. G., Goekoop, R., Duschek, E. J. J., Netelenbos, J. C., Kuijer, J. P. A., Barkhof, F. et al. (2004). Interindividual differences of medial temporal lobe activation during encoding in an elderly population studied by fMRI. Neuroimage 21, 173–180. doi: 10.1016/j.neuroimage.2003.09.043
* 4, c van der Veen, F. M., Nijhuis, F. A. P., Tisserand, D. J., Backes, W. H., and Jolles, J. (2006). Effects of aging on recognition of intentionally and incidentally stored words: an fMRI study. Neuropsychologia 44, 2477–2486. doi: 10.1016/j.neuropsychologia.2006.04.023
van Elderen, S. G. C., de Roos, A., de Craen, A. J. M., Westendorp, R. G. J., Blauw, G. J., Jukema, J. W. et al. (2010). Progression of brain atrophy and cognitive decline in diabetes mellitus: a 3-year follow-up. Neurology 75, 997–1002. doi: 10.1212/WNL.0b013e3181f25f06
* 7,8,a van Impe, A., Coxon, J. P., Goble, D. J., Wenderoth, N., and Swinnen, S. P. (2011). Age-related changes in brain activation underlying single- and dual-task performance: visuomanual drawing and mental arithmetic. Neuropsychologia 49, 2400–2409. doi: 10.1016/j.neuropsychologia.2011.04.016
* 5,6,a,• Velanova, K., Lustig, C., Jacoby, L. L., and Buckner, R. L. (2007). Evidence for frontally mediated controlled processing differences in older adults. Cereb. Cortex 17, 1033–1046. doi: 10.1093/cercor/bhl013
* 7,8,a,❖ Venkatraman, V. K., Aizenstein, H., Guralnik, J., Newman, A. B., Glynn, N. W., Taylor, C. et al. (2010). Executive control function, brain activation and white matter hyperintensities in older adults. Neuroimage 49, 3436–3442. doi: 10.1016/j.neuroimage.2009.11.019
Virta, J. J., Heikkilä, K., Perola, M., Koskenvuo, M., Räihä, I., Rinne, J. O. et al. (2013). Midlife cardiovascular risk factors and late cognitive impairment. Eur. J. Epidemiol . 28, 405–416. doi: 10.1007/s10654-013-9794-y
* 7,8,a Voelcker-Rehage, C., Godde, B., and Staudinger, U. M. (2010). Physical and motor fitness are both related to cognition in old age. Eur. J. Neurosci . 31, 167–176. doi: 10.1111/j.1460-9568.2009.07014.x
* 7,8,a Waiter, G. D., Fox, H. C., Murray, A. D., Starr, J. M., Staff, R. T., Bourne, V. J. et al. (2008). Is retaining the youthful functional anatomy underlying speed of information processing a signature of successful cognitive ageing? An event-related fMRI study of inspection time performance. Neuroimage 41, 581–595. doi: 10.1016/j.neuroimage.2008.02.045
Waldstein, S. R., Carrington, S., Thayer, J. F., Najjar, S. S., Scuteri, A., and Zonderman, A. B. (2008). Pulse pressure and pulse wave velocity are related to cognitive decline in the Baltimore Longitudinal Study of Aging. Hypertension 51, 99–104. doi: 10.1161/HYPERTENSIONAHA.107.093674
* 5,6,a Wang, L., Laviolette, P., O'Keefe, K., Putcha, D., Bakkour, A., Van Dijk, K. R. A. et al. (2010a). Intrinsic connectivity between the hippocampus and posteromedial cortex predicts memory performance in cognitively intact older individuals. Neuroimage 51, 910–917. doi: 10.1016/j.neuroimage.2010.02.046
* 7,9,a Wang, L., Li, Y., Metzak, P., He, Y., and Woodward, T. S. (2010b). Age-related changes in topological patterns of large-scale brain functional networks during memory encoding and recognition. Neuroimage 50, 862–872. doi: 10.1016/j.neuroimage.2010.01.044
* 7,9,a Wang, T. H., Kruggel, F., and Rugg, M. D. (2009). Effects of advanced aging on the neural correlates of successful recognition memory. Neuropsychologia 47, 1352–1361. doi: 10.1016/j.neuropsychologia.2009.01.030
Weis, S., Leube, D., Erb, M., Heun, R., Grodd, W., and Kircher, T. (2011). Functional neuroanatomy of sustained memory encoding performance in healthy aging and in Alzheimer's disease. Int. J. Neurosci . 121, 384–392. doi: 10.3109/00207454.2011.565892
White, W. B., Wolfson, L., Wakefield, D. B., Hall, C. B., Campbell, P., Moscufo, N. et al. (2011). Average daily blood pressure, not office blood pressure, is associated with progression of cerebrovascular disease and cognitive decline in older people. Circulation 124, 2312–2319. doi: 10.1161/CIRCULATIONAHA.111.037036
Whitehead, B. P., Dixon, R. A., Hultsch, D. F., and MacDonald, S. W. S. (2011). Are neurocognitive speed and inconsistency similarly affected in type 2 diabetes? J. Clin. Exp. Neuropsychol . 33, 647–657. doi: 10.1080/13803395.2010.547845
* 7,9,a,× Wierenga, C. E., Benjamin, M., Gopinath, K., Perlstein, W. M., Leonard, C. M., Rothi, L. J. G. et al. (2008). Age-related changes in word retrieval: role of bilateral frontal and subcortical networks. Neurobiol. Aging 29, 436–451. doi: 10.1016/j.neurobiolaging.2006.10.024
* 7,9,b,■,□ Wierenga, C. E., Stricker, N. H., McCauley, A., Simmons, A., Jak, A. J., Chang, Y.-L. et al. (2010). Increased functional brain response during word retrieval in cognitively intact older adults at genetic risk for Alzheimer's disease. Neuroimage 51, 1222–1233. doi: 10.1016/j.neuroimage.2010.03.021
* 5,6,a Wood, G., Ischebeck, A., Koppelstaetter, F., Gotwald, T., and Kaufmann, L. (2009). Developmental trajectories of magnitude processing and interference control: an FMRI study. Cereb. Cortex 19, 2755–2765. doi: 10.1093/cercor/bhp056
* 7,8,a Woodard, J. L., Seidenberg, M., Nielson, K. A., Antuono, P., Guidotti, L., Durgerian, S. et al. (2009). Semantic memory activation in amnestic mild cognitive impairment. Brain 132, 2068–2078. doi: 10.1093/brain/awp157
* 5,6,a Woodard, J. L., Seidenberg, M., Nielson, K. A., Miller, S. K., Franczak, M., Antuono, P. et al. (2007). Temporally graded activation of neocortical regions in response to memories of different ages. J. Cogn. Neurosci . 19, 1113–1124. doi: 10.1162/jocn.2007.19.7.1113
* 5,6,c Woodard, J. L., Seidenberg, M., Nielson, K. A., Smith, J. C., Antuono, P., Durgerian, S. et al. (2010). Prediction of cognitive decline in healthy older adults using fMRI. J. Alzheimers Dis . 21, 871–885. doi: 10.3233/JAD-2010-091693
* 5,6,c Woodard, J. L., Sugarman, M. A., Nielson, K. A., Smith, J. C., Seidenberg, M., Durgerian, S. et al. (2012). Lifestyle and genetic contributions to cognitive decline and hippocampal structure and function in healthy aging. Curr. Alzheimer Res . 9, 436–446. doi: 10.2174/156720512800492477
* 5,6,a,• Wu, J.-T., Wu, H.-Z., Yan, C.-G., Chen, W.-X., Zhang, H.-Y., He, Y. et al. (2011). Aging-related changes in the default mode network and its anti-correlated networks: a resting-state fMRI study. Neurosci. Lett . 504, 62–67. doi: 10.1016/j.neulet.2011.08.059
Xu, W., Caracciolo, B., Wang, H.-X., Winblad, B., Bäckman, L., Qiu, C. et al. (2010). Accelerated progression from mild cognitive impairment to dementia in people with diabetes. Diabetes 59, 2928–2935. doi: 10.2337/db10-0539
Yaffe, K., Blackwell, T., Kanaya, A. M., Davidowitz, N., Barrett-Connor, E., and Krueger, K. (2004). Diabetes, impaired fasting glucose, and development of cognitive impairment in older women. Neurology 63, 658–663. doi: 10.1212/01.WNL.0000134666.64593.BA
Yaffe, K., Lindquist, K., Schwartz, A. V., Vitartas, C., Vittinghoff, E., Satterfield, S. et al. (2011). Advanced glycation end product level, diabetes, and accelerated cognitive aging. Neurology 77, 1351–1356. doi: 10.1212/WNL.0b013e3182315a56
Yakushiji, Y., Noguchi, T., Hara, M., Nishihara, M., Eriguchi, M., Nanri, Y. et al. (2012). Distributional impact of brain microbleeds on global cognitive function in adults without neurological disorder. Stroke 43, 1800–1805. doi: 10.1161/STROKEAHA.111.647065
Yan, S. F., Ramasamy, R., and Schmidt, A. M. (2008). Mechanisms of disease: advanced glycation end-products and their receptor in inflammation and diabetes complications. Nat. Clin. Pract. Endocrinol. Metab . 4, 285–293. doi: 10.1038/ncpendmet0786
Yeung, S. E., Fischer, A. L., and Dixon, R. A. (2009). Exploring effects of type 2 diabetes on cognitive functioning in older adults. Neuropsychology 23, 1–9. doi: 10.1037/a0013849
* 5,6,c Ystad, M., Eichele, T., Lundervold, A. J., and Lundervold, A. (2010). Subcortical functional connectivity and verbal episodic memory in healthy elderly—a resting state fMRI study. Neuroimage 52, 379–388. doi: 10.1016/j.neuroimage.2010.03.062
* 5,6,c Ystad, M., Hodneland, E., Adolfsdottir, S., Haász, J., Lundervold, A. J., Eichele, T. et al. (2011). Cortico-striatal connectivity and cognition in normal aging: a combined DTI and resting state fMRI study. Neuroimage 55, 24–31. doi: 10.1016/j.neuroimage.2010.11.016
Zhong, Y., Miao, Y., Jia, W. P., Yan, H., Wang, B. Y., and Jin, J. (2012a). Hyperinsulinemia, insulin resistance and cognitive decline in older cohort. Biomed. Environ. Sci . 25, 8–14. doi: 10.3967/0895-3988.2012.01.002
Zhong, Y., Zhang, X. Y., Miao, Y., Zhu, J. H., Yan, H., Wang, B. Y. et al. (2012b). The relationship between glucose excursion and cognitive function in aged type 2 diabetes patients. Biomed. Environ. Sci . 25, 1–7. doi: 10.3967/0895-3988.2012.01.001
Zhou, H., Lu, W., Shi, Y., Bai, F., Chang, J., Yuan, Y. et al. (2010). Impairments in cognition and resting-state connectivity of the hippocampus in elderly subjects with type 2 diabetes. Neurosci. Lett . 473, 5–10. doi: 10.1016/j.neulet.2009.12.057
* 7,8,a,• Zhu, D. C., Zacks, R. T., and Slade, J. M. (2010). Brain activation during interference resolution in young and older adults: an fMRI study. Neuroimage 50, 810–817. doi: 10.1016/j.neuroimage.2009.12.087
Keywords: type 2 diabetes mellitus, hypertension, cognition, aging, imaging
Citation: Meusel L-AC, Kansal N, Tchistiakova E, Yuen W, MacIntosh BJ, Greenwood CE and Anderson ND (2014) A systematic review of type 2 diabetes mellitus and hypertension in imaging studies of cognitive aging: time to establish new norms. Front. Aging Neurosci . 6 :148. doi: 10.3389/fnagi.2014.00148
Received: 25 January 2014; Accepted: 17 June 2014; Published online: 08 July 2014.
Reviewed by:
Copyright © 2014 Meusel, Kansal, Tchistiakova, Yuen, MacIntosh, Greenwood and Anderson. This is an open-access article distributed under the terms of the Creative Commons Attribution License (CC BY) . The use, distribution or reproduction in other forums is permitted, provided the original author(s) or licensor are credited and that the original publication in this journal is cited, in accordance with accepted academic practice. No use, distribution or reproduction is permitted which does not comply with these terms.
*Correspondence: Nicole D. Anderson, Rotman Research Institute, Baycrest, 3560 Bathurst Street, Toronto, ON M6A 2E1, Canada e-mail: [email protected]
- Open access
- Published: 16 May 2024
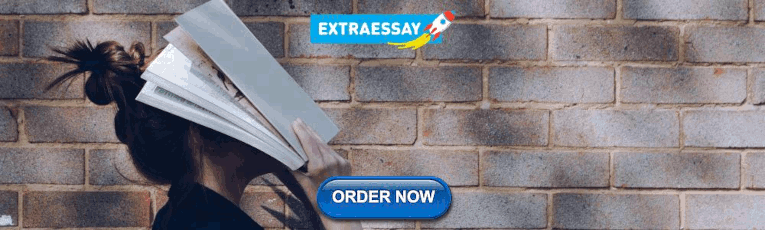
DNA methylation and type 2 diabetes: a systematic review
- Nikhil Nadiger 1 , 2 ,
- Jyothisha Kana Veed 2 ,
- Priyanka Chinya Nataraj 2 nAff3 &
- Arpita Mukhopadhyay 2
Clinical Epigenetics volume 16 , Article number: 67 ( 2024 ) Cite this article
266 Accesses
Metrics details
DNA methylation influences gene expression and function in the pathophysiology of type 2 diabetes mellitus (T2DM). Mapping of T2DM-associated DNA methylation could aid early detection and/or therapeutic treatment options for diabetics.
A systematic literature search for associations between T2DM and DNA methylation was performed. Prospero registration ID: CRD42020140436.
PubMed and ScienceDirect databases were searched (till October 19, 2023). Preferred Reporting Items for Systematic Reviews and Meta-Analyses (PRISMA) guidelines and New Castle Ottawa scale were used for reporting the selection and quality of the studies, respectively.
Thirty-two articles were selected. Four of 130 differentially methylated genes in blood, adipose, liver or pancreatic islets ( TXNIP , ABCG1 , PPARGC1A , PTPRN2 ) were reported in > 1 study. TXNIP was hypomethylated in diabetic blood across ethnicities. Gene enrichment analysis of the differentially methylated genes highlighted relevant disease pathways (T2DM, type 1 diabetes and adipocytokine signaling). Three prospective studies reported association of methylation in IGFBP2 , MSI2 , FTO , TXNIP , SREBF1 , PHOSPHO1 , SOCS3 and ABCG1 in blood at baseline with incident T2DM/hyperglycemia. Sex-specific differential methylation was reported only for HOOK2 in visceral adipose tissue (female diabetics: hypermethylated, male diabetics: hypomethylated). Gene expression was inversely associated with methylation status in 8 studies, in genes including ABCG1 (blood), S100A4 (adipose tissue), PER2 (pancreatic islets), PDGFA (liver) and PPARGC1A (skeletal muscle).
This review summarizes available evidence for using DNA methylation patterns to unravel T2DM pathophysiology. Further validation studies in diverse populations will set the stage for utilizing this knowledge for identifying early diagnostic markers and novel druggable pathways.
Introduction
Type 2 diabetes mellitus (T2DM) is a disorder of genetic and environmental factors. It is projected to affect 693 million people worldwide by 2045 [ 1 ]. DNA methylation had been proposed as one of the epigenetic phenomena for explaining the missing heritability of T2DM, as multiple, large genome-wide association studies have been able to account for only < 20% of the estimated T2DM heritability [ 2 ]. DNA methylation is an epigenetic phenomenon in which the C5 carbon of the cytosine residue is attached to a methyl group, predominantly in cytosine-phosphate-guanine (CpG) sites [ 3 , 4 , 5 ]. This epigenetic alteration influences gene expression, and thereby, gene function [ 6 , 7 ].
DNA methylation has been studied extensively in relation to T2DM, and 3 systematic reviews have summarized the findings a few years back [ 8 , 9 , 10 ]. From systematic literature done till August 2015, Muka et al. [ 10 ] could not find any consistent association between global DNA methylation with T2DM, glucose, insulin and insulin resistance and reported epigenetic regulation of few candidate genes in blood cells, muscle, adipose tissue and placenta without any overlap between them. Walaszczyk et al . [ 9 ] could replicate association of methylation with T2DM in blood samples from the Lifelines study at 5 CpGs (in ABCG1 , LOXL2 , TXNIP , SLC1A5 and SREBF1 ) out of the 52 CpGs they identified as reported to be differentially methylated in T2DM through a systematic review of the literature done till April 2017. Willmer et al . [ 8 ] also focused on differential methylation signatures in blood samples and reported TCF7L2 , KCNQ1 , ABCG1 , TXNIP , PHOSPHO1 , SREBF1 , SLC30A8 and FTO genes to be reproducibly associated with T2DM across multiple population groups in the literature reviewed between January 2002 and July 2018.
DNA methylation has been touted as a strong candidate biological process for identification of diagnostic and therapeutics for T2DM [ 11 ]. While the available systematic reviews have looked at DNA methylation associated with T2DM [ 8 , 9 , 10 ], they have not evaluated T2DM-associated DNA methylation comprehensively in all available human tissue and cell types. We set out to fill this research gap with the no time period cutoff until October 19, 2023, and including all available human tissue and cell types. We also report associated gene expression data, role of sex and ethnicity, in relation to DNA methylation in our review.
PubMed and Science Direct databases were independently searched by authors (NN, PN and JKV) using the key terms “type 2 diabetes mellitus” and “DNA methylation,” and their associated terms for all studies published up to October 19, 2023. All articles from the time of publication listing were considered, and as such no start date was set. No filters were applied during the search using the keywords, so as to not exclude any mislabeled/mis-annotated article type. The detailed search strategy is given in Additional file 1 : Table S1.
Study inclusion and exclusion criteria
The inclusion criteria were full-text English language articles on DNA methylation associated with T2DM in human subjects. Case–control and prospective studies investigating genome-wide methylation were included. Reviews, animal model studies, in vitro studies, irrelevant articles and articles published in other languages were excluded.
All participants, regardless of gender and ethnicity, classified as adults aged 18 years and above were included. All individuals who did not satisfy these criteria—children and adolescents under 18 years of age; as well as subjects with type 1 diabetes (T1DM) or gestational diabetes were excluded. As the association of DNA methylation with T2DM was the focus of this systematic review, intervention studies and clinical trials were excluded. Studies reporting association of DNA methylation with diabetes-related traits (hyperglycemia and insulin resistance) were retained.
All the articles were assessed for their eligibility based on their abstract or full text.
Disagreements between the authors, such as categorization and selection of articles, and data extraction, were resolved through discussion with AM. The Preferred Reporting Items for Systematic Reviews and Meta-Analyses (PRISMA) checklist was followed to represent the method used [ 12 ]. A total of 32 full-text articles are included in this systematic review.
The assessment of quality of the studies was done by adapting the New Castle Ottawa scale (NOS) [ 13 ]. The parameters used for the assessment are adequacy of case definition, representativeness of cases, selection of controls, definition of controls, comparability of cases and controls, ascertainment of exposure and method used for ascertainment of cases and controls. Scores were given to each of the included studies, and the total score was calculated according to the score sheet (NOS).
This review protocol was registered with the International Prospective Register of Systematic Reviews (PROSPERO) database ( https://www.crd.york.ac.uk/prospero/ ) [ 14 ] (accessed April 18, 2023) (registration ID: CRD42020140436).
Pathologically connected pathways with differentially methylated genes in T2DM were analyzed using Kyoto Encyclopedia of Genes and Genomes (KEGG) and Jensen Disease database via Enrichr-KG [ 15 ].
We identified a total of 5819 articles during the initial search. Duplicates, irrelevant articles based on the study design, publication language, article type, and other articles not within our scope of review were removed. Thirty-two full-text articles were finally selected (Fig. 1 ).
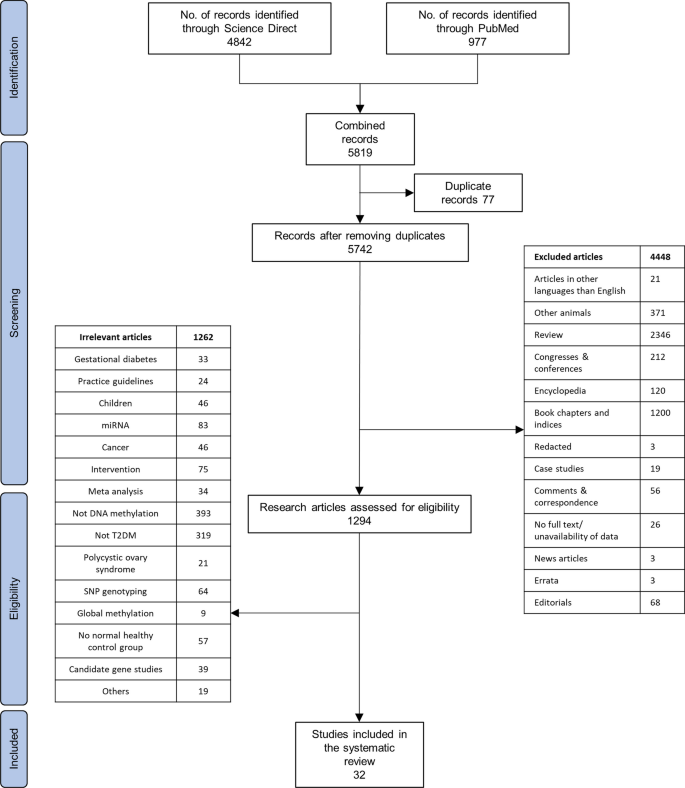
Preferred Reporting Items for Systematic Reviews and Meta-Analyses (PRISMA) [ 12 ] flowchart for the literature search process, performed up to October 19, 2023
NOS was used to access the quality of the articles. Of the 32 studies, 16 were assigned a score of more than 5, indicating high quality (Additional file 2 : Table S2). As all the studies have used the same method of ascertainment for cases and controls, and the authors are not blinded to case–control status, these redundant scores are not presented. As the nonresponse rate was not available for any of the studies, this also has been omitted from the quality assessment table.
Case–control studies that reported differential DNA methylation between T2DM (case) and normoglycemic (control) subjects or reported associations between DNA methylation and clinical parameters related to glycemic control of the subjects (HbA1c, fasting blood glucose) and prospective nested case–control studies that reported differential DNA methylation measured at baseline/recruitment between subjects who developed T2DM (incident cases) and those that remained normoglycemic (control) during the follow-up period were finally included.
Participant details such as number of cases and controls and location of the study are also included. Details of the study participants who do not explicitly belong to either case or control group are also presented. The tissue source of the gene/loci identified in; method used for determining methylation status; and the validation method used for confirming the methylation status are tabulated in Table 1 .
The loci/genes reported to be differentially methylated are tabulated in Table 2 , where their methylation status is represented as downward arrow (hypomethylation) or upward arrow (hypermethylation). Wherever reported, the statistical significance of methylation ( P value) is also mentioned. For studies reporting more than 10 differentially methylated genes, the top 5 hypo- and hypermethylated genes are listed.
Methods of DNA methylation analysis
Majority of the evaluated studies had employed array-based techniques to assess DNA methylation levels. Eighteen of 32 studies used Illumina 450 k array. Other array-based studies used Illumina 27 k array (2 studies), Illumina EPIC BeadChip array (4 studies; of which 2 studies specifically mentioned using the 850 k array—EPIC v1 array targeting 850 k probes), Affymetrix SNP6 microarray (1 study), Affymetrix GeneChip promoter 1.0R array (1 study) or Affymetrix axiom genome-wide Taiwan BioBank (TWB) array (1 studies). Rest of the studies used techniques such as methylated DNA immunoprecipitation (MEDIP) (2 studies), MEDIP-chromatin immune precipitation (1 study), reduced representation bisulfite sequencing (1 study) or next-generation sequencing (1 study) to measure DNA methylation levels.
Tissues used in DNA methylation analyses
Of the 32 articles retrieved, 17 (53%) studies used blood samples, 4 (13%) studies used pancreatic islet samples, 5 (16%) studies used adipose tissue samples, 2 (6%) studies used liver samples, 1 (3%) study used spermatozoa samples and 3 (9%) used skeletal muscle samples for their DNA methylation analyses. None of the 32 studies reviewed here utilized more than one tissue from the same subjects for DNA methylation analyses.
Genome-wide methylation analysis for T2DM
Of the 32 genome-wide methylation studies reviewed here, we identified a total of 130 loci that were differentially methylated between T2DM cases and controls across. In an instance where a study reports < 10 differentially methylated genes/loci, they are presented individually. However, in the case of a study which reports > 10 genes/loci, only the top 5 hypo- and 5 hypermethylated genes are highlighted for brevity and reported in Table 2 . The direction of methylation (hyper- or hypomethylated in T2DM, compared to controls) and the reported P values (both unadjusted, and after multiple testing correction) have been included.
We identified genes such as ABCG1, PPARGC1A , PTPRN2 and TXNIP with well-known T2DM genetic risk variants, which were consistently reported to be differentially methylated in more than one study (Fig. 2 ). Tissues used in identification of these gene were blood cells, liver, pancreatic islets and adipose tissue. TXNIP (cg19693031) was the most common gene identified consistently as hypomethylated in diabetic blood (9 studies). TXNIP also harbors established T2DM genetic risk variants [ 16 , 17 ].
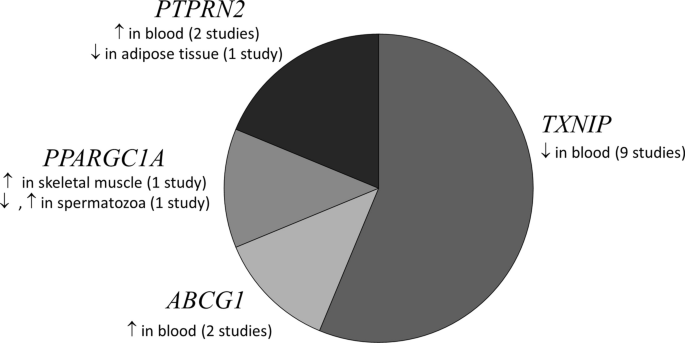
A pie chart depicting the genes that were consistently reported to be differentially methylated in ≥ 2 studies in various tissues from T2DM subjects. ↑: Hypomethylation, ↓: Hypermethylation in T2DM individuals compared to normoglycemics. PPARGC1A (chr4: 24,024,251–500) hypomethylated, (chr4: 24,111,501–750) hypermethylated in spermatozoa [ 57 ]
Although blood is not an insulin-responsive tissue, it is the prime minimally invasive tissue available for investigating T2DM-associated epigenetic markers. With the bulk (50%) of the studies coming from Europe, ABCG1 [ 18 , 19 ] and TXNIP [ 16 , 17 , 19 , 20 , 21 , 22 , 23 , 24 , 25 ] were some of the blood-based epigenetic markers which were reported to be associated with T2DM in more than one study. We were unable to find any study where differential methylation was investigated simultaneously in blood and other tissues from the same subjects.
Pancreatic islets
Insufficient secretion of insulin from pancreatic beta cells and increased secretion of glucagon from pancreatic alpha cells leads to development of T2DM and is known to be regulated by DNA methylation [ 26 ]. Three of the 32 studies, from Italy, South Korea and Sweden, included in this review have interrogated DNA methylation in pancreatic islets from T2DM individuals, donated after their death. Regions in SFRS2IP [ 3 ], MSI2 [ 27 ], which are known to be associated with critical roles in nucleic acid binding, and B3GNT7 [ 28 ] that is involved in synthesis of glycoprotein, were reported to be hypomethylated in pancreatic islets from T2DM individuals. Considering that DNA methylation can change based on the time of collection of tissue after death [ 29 , 30 ], findings from these studies need to be interpreted in cognizance of the lack of details available in these studies about the cause of death or collection and storage of pancreatic islet tissue after death.
Adipose tissue
Adipose tissue is known to play a critical role in regulating body metabolism and energy homeostasis [ 31 ]. Dysregulation in adipose biology imposes serious health complications such as obesity and development of T2DM [ 31 ]. DNA methylation is an important regulator factor in development [ 32 , 33 ] and dysfunction [ 34 , 35 ] of adipose tissue. Five studies—4 of these representing the European population—included in this review have dissected whether T2DM, and related risk factors are associated with epigenetic modifications in human adipose tissue [ 36 , 37 , 38 , 39 , 40 ]. It is possible that DNA methylation alterations in these reported genes including C1orf52 [ 36 ], HOOK2 [ 37 ], MFSD1 [ 38 ], HNF4A [ 39 ] and L1TD1 [ 40 ] contribute to or are caused by T2DM.
C1orf52 is involved in RNA binding in adipose tissue [ 41 ], and HOOK2 is responsible for cytoskeleton maintenance via regulation of microtubules [ 42 ], while MSFD1 regulates lysosome transport [ 43 ]. Epigenetic alterations in such genes involved in cell structure and function can cause dysfunction in adipose tissue, thereby leading to insulin resistance. While HNF4A mainly regulates transcription in hepatocytes and is associated with Fanconi renotubular syndrome 4 with maturity-onset diabetes of the young [ 44 ] and maturity-onset diabetes of the young, type 1 [ 45 ], it is also known to play a role in lipid and glucose metabolism [ 46 , 47 ]. L1TD1 is predicted to be involved in single-stranded RNA-binding activity [ 48 ].
Liver is known to be involved in regulating glucose level by storing and releasing glycogen in response to insulin and glucagon [ 49 ]. Impaired hepatic gluconeogenesis, glycogenolysis and insulin sensitivity are known to play an important role in T2DM development and other risk factors. Altered hepatic metabolism could be the cause or consequence of DNA methylation modification. Genes involved in intracellular tyrosine kinase activity— PDGFA [ 50 ], transferring phosphorus-containing groups and protein tyrosine kinase activity— RIPK4 [ 51 ], heme binding and oxidoreductase activity— CYB561D1 [ 51 ], were found to be hypomethylated in the diabetic groups. However, the gene involved in inflammation— IL23Ap19 [ 51 ] was identified to be hypermethylated in the diabetic group. Of the two studies reported here, one was from France and the other from Finland.
Gene expression studies
Out of the 32 studies reviewed, 8 had also examined differences in gene expression between T2DM and normoglycemic individuals. To examine if increase in methylation of a gene causes decrease in expression of that gene, we analyzed the studies that report both differentially methylated genes and gene expression, in the same population and study setting, using tissues from the same study participants (Table 3 ). For most of the loci with both DNA methylation and gene expression data available, we found that increase in methylation was associated with decrease in expression, concurrent to the current understanding [ 6 ]. Hypermethylation of PPARGC1A in skeletal muscles [ 52 ], ABCG1 in blood [ 18 ] and PER2 in pancreatic islets [ 3 ] was associated with lower expression of the corresponding genes.
Twin studies
Five of the 32 studies reviewed here have investigated DNA methylation in monozygotic twin cohorts [ 17 , 21 , 28 , 36 , 53 ] (Table 4 ). MALTI [ 53 ] which is known to be involved in energy and insulin signaling pathways [ 54 ], PTBP1 [ 36 ] that is involved in nucleic acid binding, and ANO8 [ 28 ] that is involved in calcium transport, were hypermethylated in diabetic twins in peripheral blood, adipose tissue and pancreatic islets, respectively. TXNIP [ 17 , 21 ], COL21A1 [ 36 ] and B3GNT7 [ 28 ] were hypomethylated in blood cells, adipose tissue and pancreatic islets, respectively, from the diabetic twins. Dayeh et al . reported differential methylation of ABCG1 (hypermethylated in blood and adipose tissue) and PHOSPHO1 (hypomethylated in skeletal muscle) in monozygotic twins discordant for T2DM [ 55 ].
Association between diabetes related traits and DNA methylation
Only 4 of the 32 studies reported association between diabetes-related traits (hyperglycemia and insulin resistance) and DNA methylation [ 17 , 18 , 19 , 22 ]. Kriebel et al . reported significant association between measures of glucose metabolism phenotypic traits and methylation levels of 31 CpG sites in PBMCs [ 18 ]. Five CpGs were found to be associated with fasting glucose, 1 CpG with 2-h glucose, 8 with fasting insulin and 26 with Homeostatic Model Assessment of Insulin Resistance (HOMA-IR) in model 1 (Table 2 ) [ 18 ]. There was no significant association between HbA1c and DNA methylation levels in model 1; in model 2, after adjustment for body mass index (BMI), the effect strength was reduced by 30% for DNA methylation associations with fasting glucose suggesting that the associations between DNA methylation and diabetes-related traits are partially mediated by BMI [ 18 ].
Kulkarni et al . investigated association between 446,356 autosomal CpG sites and phenotypic traits in PBMCs, of which a total of 51 CpG sites were significantly associated with T2DM, 19 with FBG and 24 with HOMA-IR (Table 2 ) [ 19 ].
Wang et al . report association between 63 differential methylated loci and fasting blood glucose and association between 6 differentially methylated loci with HbA1c in blood samples from twins discordant for diabetes [ 17 ]. Among these, hypomethylation of TXNIP [ 17 , 19 ] and hypermethylation of ABCG1 [ 18 , 19 ] were positively associated with fasting blood glucose (FBG), and hypermethylation of SAMD12 was negatively associated with FBG [ 19 ]. TXNIP hypomethylation in blood cells was found to be associated with hyperglycemia in individuals from Taiwan [ 23 ], France [ 24 ], the USA [ 21 ] and China [ 17 ].
Dawes et al . performed genome-wide DNA methylation on blood samples from normoglycemic (n = 142), pre-diabetic (n = 274) and diabetic (n = 90) individuals [ 22 ]. They identified HbA1c-associated DNA methylation loci by regressing the probes against HbA1c values, while controlling for age, sex and BMI [ 22 ]. They report cg19693031 ( TXNIP ) as the locus most highly associated with HbA1c [ 22 ].
Enrichment analysis of genes differentially methylated in T2DM
Enrichment analysis of signaling pathways relevant to the pathophysiology of T2DM using Enrichr-KG [ 15 ] was done in two steps. Initially, all 130 genes differentially methylated in T2DM in all 32 studies reviewed were included (Fig. 3 ). To take into account reproducibility of these findings, enrichment analysis was separately done specifically for the genes ( ABCG1 , TXNIP , PTPRN2 , PPARGC1A ) that were reported to be differentially methylated in T2DM in more than one study (Fig. 4 ). TXNIP hypomethylation in blood was linked to hyperglycemia. PPARGC1A hypermethylation in skeletal muscles, and two CpG sites that were hyper- and hypomethylated, respectively, in spermatozoa, was linked to hyperglycemia and adipocytokine signaling pathway. PTPRN2 that was reported to be hypermethylated in blood and hypomethylated in adipose tissue was associated with T2DM and T1DM.
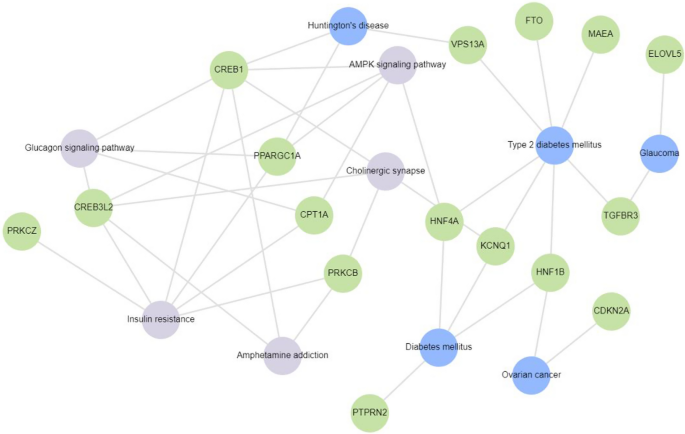
Gene enrichment analysis of 17 of the 130 genes reported to be differentially methylated in T2DM subjects in the 32 studies included for review using Enrichr-KG. These genes were mapped to diabetes and related disorders. Insulin resistance, glucagon signaling pathway, glaucoma, AMPK signaling pathway, cholinergic synapse, ovarian cancer, amphetamine addiction and Huntington’s disease were found to be associated with KCNQ1 , FTO , PPARGC1A , PTPRN2 , ELOVL5 , HNF1B , HNF4A , VPS13A , MAEA , CREB1 , CPT1A , PRKCZ , PRKCB , CREB3L2 , CDKN2A and TGFBR3
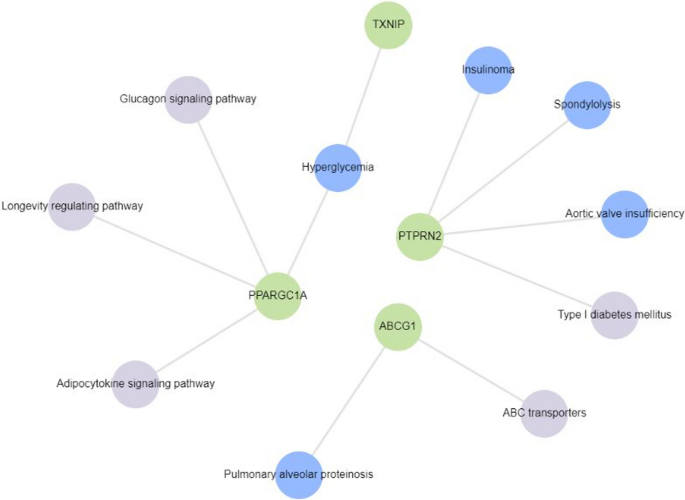
Gene enrichment analysis of 4 genes reported to be differentially methylated in T2DM subjects in > 1 study from among the 32 studies included for review using Enrichr-KG. Hyperglycemia, type 1 diabetes, adipocytokine signaling pathway, glucagon signaling pathway, longevity regulating pathway and ABC transporters were found to be associated with PPARGC1A , TXNIP , PTPRN2 and ABCG1
Subgroup analysis based on ethnicity
Out of the 32 studies, 16 (50%) were from Europe, 4 (13%) were from North America, 8 (25%) were from Asia and 1 (3%) from Africa. Three studies (9%) did not report their subjects’ ethnicity/demography.
TXNIP was the most commonly reported hypomethylated gene in blood cells of T2DM individuals from all the geographic locations [ 16 , 17 , 19 , 20 , 21 , 22 , 23 , 24 ]. ABCG1 was found be to hypermethylated in blood cells of type 2 diabetics in studies from Europe [ 18 ] and the USA [ 19 ]. PTPRN2 was reported to be hypermethylated in peripheral blood in studies from China [ 56 ] and France [ 24 ]. Conversely, PTPRN2 was reported to be hypomethylated in adipose tissue from a Spanish study [ 37 ].
Subgroup analysis based on sex
PPARGC1A was assessed for differential methylation in two studies which had only male participants [ 52 , 57 ]. PPARGC1A was hypermethylated in skeletal muscle of T2DM men [ 52 ]. Of the two differentially methylated regions in PPARGC1A identified in sperm, chr4: 24,111,501–750 was reported to be hypermethylated, and chr4: 24,024,251–500 was reported to be hypomethylated [ 57 ]. We did not find other epigenome-wide studies that report differential methylation of PPARGC1A in female-only or mixed-sex populations.
PDGFA was found to be hypomethylated in hepatocytes from liver biopsies of female T2DM participants of the discovery group and was later confirmed in both men and women by Abderrahmani et al . [ 50 ]. Similarly, hypomethylation of MSI2 in blood cells was first observed in a discovery group comprised of only men, and then in a replication group of both men and women by Jeon et al . [ 27 ].
In the cg 11,738,485-region (5 CpG nucleotides) of HOOK2 , female T2DM visceral adipose tissue samples were hypermethylated, while male T2DM samples were hypomethylated, compared to non-diabetic sex-matched control samples [ 37 ]. None of the other loci/genes were reported to be differentially methylated in a sex-specific manner.
Internal and/or external validation
Only 22% of the studies reviewed (7 out of 32) validated their findings in an independent set of subjects using the same DNA methylation measurement method that they had used for the discovery set of samples [ 17 , 25 , 27 , 36 , 37 , 50 , 53 ]. Others used either bisulfite pyrosequencing/sequencing (10 studies) [ 3 , 19 , 27 , 28 , 37 , 39 , 52 , 58 , 59 , 60 ], qPCR (1 study) [ 51 ], EpiTYPER (1 study) [ 16 ], Illumina 450 k (3 studies) [ 36 , 50 , 53 ] or MEDIP (1 study) [ 61 ] for their internal validation. Sixteen studies (50%) did not perform any validation for their findings.
Replication for case–control studies
We later looked for candidate-gene DNA methylation studies to see if the differentially methylated genes found in genome-wide studies have been confirmed in them. The following genes were reported to be differentially methylated in T2DM compared to normoglycemic controls in independent candidate-gene DNA methylation studies in the same tissue as the initial discovery group— ABCG1 [ 62 , 63 ], FTO [ 64 , 65 , 66 ], TXNIP [ 67 ] and KCNQ1 [ 64 , 68 ] in PBMCs, and PPARGC1A in pancreatic islets [ 69 ].
Prospective studies
As prospective studies observe the disease condition over a long period, they help in better understanding the role of a gene/set of genes toward pathogenesis. In our review, we came across three such studies that looked at incidence of T2DM and epigenetic modifications in genes associated with this incidence (Table 5 ).
In a 1:1 matched nested case–control study of 290 incident diabetics, who developed T2DM and 290 controls, who remained normoglycemic during the 4-year follow-up, baseline methylation at 7 CpG sites of IGFBP2 in blood cells (4 hypermethylated and 3 hypomethylated in cases) was associated with increased risk of incident T2DM [ 70 ].
Jeon et al . reported that differential methylation of three CpG sites in blood cells at baseline was associated with T2DM/hyperglycemia after a 10-year follow-up [ 27 ]. These CpG sites were cg23586172 (annotated to MSI2 , hypomethylated), cg22604213 (annotated to CXXC4, hypomethylated) and cg25290098 (hypomethylated) in T2DM [ 27 ]. They further reported MSI2 hypomethylation in a replication group of 220 normoglycemic and 220 T2DM individuals [ 27 ]. Furthermore, whole-genome bisulfite sequencing of pancreatic islets of 2 T2DM and 16 normoglycemic individuals revealed that chr17:55,484,635 in MSI2 was hypomethylated in T2DM [ 27 ]. While MSI2 hypomethylation was seen in both pancreatic islets and PBMCs, pancreatic islets showed increased difference of 16% methylation versus 3% in PBMCs of MSI2 in T2DM when compared to normoglycemics [ 27 ]. MSI2 differential methylation was not found to be replicated in locus-specific case–control studies.
From the Jerusalem LRC longitudinal study, Toperoff et al . selected 58 individuals who developed impaired glucose metabolism over a 13-year follow-up and reported hypomethylation of a single CpG site in the first intron of FTO in peripheral blood samples collected at baseline [ 58 ]. Chen et al . similarly reported hypomethylation of FTO in their case–control study [ 57 ].
In a longitudinal study of Indian Asians living in London, UK (1074 incident T2DM and 1590 normoglycemic controls), over 8 years of follow-up, Chambers et al . reported that DNA methylation levels of TXNIP , PROC , C7orf29 , SREBF1 , PHOSPHO1 , SOCS3 and ABCG1 in blood cells were positively associated with future T2DM incidence [ 71 ]. Of these, higher baseline methylation levels in TXNIP , SREBF1 , PHOSPHO1 , SOCS3 and ABCG1 were also associated with incident T2DM in an European cohort of 377 incident T2DM and 764 normoglycemic individuals [ 71 ].
Differential methylation in animal models
To check if animal model studies exist that have investigated or reported differential methylation in the genes identified as differentially methylated in the human case–control studies as playing causal or mechanistic role in the development of T2DM, a simple literature search was done using PubMed and bibliography search. A study in rat pancreatic islets reported Kcnq1 was hypomethylated in older rats (15 months of age) when compared to younger rats (3 months of age), but this difference was not statistically significant, while there was no comparison done with a rat T2DM model [ 72 ]. Though Toperoff et al . reported hypomethylation of KCNQ1 in blood cells [ 58 ], there are no human pancreatic islet studies reporting hypomethylation of KCNQ1 . Identification of multiple variants in genome-wide association studies [ 73 , 74 , 75 , 76 , 77 , 78 , 79 , 80 , 81 ] points toward the likely importance of KCNQ1 in T2DM pathophysiology.
High-fat diet was shown to induce hypermethylation of Tcf7l2, and subsequently, gene expression was decreased in mouse islets [ 82 ]. This is in contrast to the findings where TCF7L2 is hypomethylated in T2DM human blood cells [ 58 ] and pancreatic islets [ 59 ]. It is to be noted that the mice used were non-diabetic adult males aged 8 weeks (equivalent to middle-aged humans [ 83 ]) [ 82 ], while the human study group were a mix of men and women aged about 58–65 years, and for the human pancreatic islet study, the samples had been collected post-mortem [ 58 , 59 ]. Although there is an inverse differential methylation status among mice and humans, it is important to note that a high-fat diet caused suppression of Tcf7l2 gene expression and thus decreases pancreatic beta-cell survival (mediated via the transcription of Wnt/Beta-catenin signaling pathway [ 84 ]) [ 82 ].
From the 32 studies finally included for this systematic review, we identified 130 genes with T2DM-associated differential methylation across all tissues analyzed. These comprise of the top 5 hypo- and hypermethylated genes for studies reporting more than 10 differentially methylated genes/loci. Of these 130 genes, 4 (3%; ABCG1, PPARGC1A , PTPRN2 and TXNIP ) were reported in > 1 studies. The genes and associated pathways with altered DNA methylation in T2DM are conceptually summarized in Fig. 3 (for 16 of the 130 genes, for which pathway analysis could be conducted) and Fig. 4 (for the 4 genes reported to be differentially methylated in > 1 studies).
Previous systematic reviews [ 8 , 9 ] have reported differentially methylated loci in genes in T2DM blood cells including ABCG1 , TXNIP , KCNQ1 . While another such review by Muka et al . reported several epigenetically regulated genes from blood cells, adipose tissue, muscle and placenta, there was no overlap between them, and no association was found between global DNA methylation and T2DM/hyperglycemic markers [ 10 ].
We did not limit our search to a particular method used to identify DNA methylation, and several studies included have used Illumina’s 450 k array. The common method of validation/replication in the studies reviewed here was bisulfite pyrosequencing. We also looked at candidate-gene DNA methylation studies which aimed to replicate/validate the epigenome-wide studies reviewed here and found that in blood cells, ABCG1 [ 62 ], FTO [ 64 ] and KCNQ1 [ 64 ] were hypermethylated, while TXNIP was hypomethylated [ 67 ]. TXNIP codes for thioredoxin-interacting protein, and this protein plays a major role in pathways generating reactive oxygen species [ 85 ], regulating redox-dependent signaling pathways, mediating oxidative stress, suppressing cell growth and inducing pancreatic beta-cell apoptosis [ 86 ]. ABCG1 codes for the protein responsible for intracellular sterol transport [ 87 ], and it regulates cholesterol efflux from macrophages to high-density lipoprotein in diabetics [ 88 ], indicated by altered lipid levels [ 89 ]. While genetic variants and epigenetic modification of KCNQ1 have been linked with T2DM via whole body insulin sensitivity [ 90 ], there is no clear evidence for the mechanistic link. Likewise, there has been no clear evidence of FTO link with T2DM.
As gene expression is known to be regulated by DNA methylation, it is important to validate this claim in the epigenome-wide association studies. We were able to report the relation between DNA methylation in the promoter region and expression of the corresponding gene, as none of the studies had mentioned methylation status of other regions of the genes. Of the studies reviewed here, we found that DNA methylation of genes was inversely related to gene expression. For example, hypomethylation of S100A4 in adipose tissue [ 36 ] and PDGFA in hepatocytes [ 50 ] was associated with increased expression of these genes, and hypermethylation of PPARGC1A in skeletal muscles [ 52 ], ABCG1 in blood [ 18 ] and PER2 in pancreatic islets [ 3 ] was associated with lower expression of the corresponding genes. Even though we observed DNA methylation being related inversely with expression of the corresponding gene in the studies reviewed, this is not a rule as has been reported repeatedly [ 91 ]. It is also important to note that there have been reports of methylation levels differing between different regions of the gene that influence gene expression; for instance, Anastasiadi et al . recently reported that gene expression is dependent on methylation of the first exon, more than methylation of the promoter region [ 92 ]. Moreover, in other studies such as one by Ball and colleagues, highly expressed genes have been reported to have low methylation levels in the promoter region and high methylation levels in rest of the gene body [ 93 ]. We could not, however, evaluate the relations between DNA methylation in various regions of a gene and its corresponding expression in this study since the studies reviewed by us have reported DNA methylation specifically in the promoter region.
Epigenetic studies on twins discordant for disease status are crucial in understanding the genetic basis of epigenetic differences observed in cross-sectional studies. Of the 5 studies included in our search, 3 did not have any common differentially methylated genes among them, while the other two studies that used blood cells as the source tissue had TXNIP as the common differentially methylated gene between them, with hypomethylation of TXNIP in diabetic blood samples observed in both these studies [ 17 , 21 ]. TXNIP is the only gene reported to be hypomethylated in diabetic blood in both case–control studies [ 55 ] and in twin studies [ 17 , 21 ] where the influence of underlying genetic factors is not masked. TXNIP has also been reported to be hypomethylated in diabetic pancreatic islets [ 55 ] and skeletal muscle [ 55 ], making it a potentially important causal gene in the pathophysiology of T2DM.
T2DM is known to be associated with other comorbidities such as obesity and cardiovascular complication. These comorbidities share some common risk factors like age, BMI and cholesterol content in blood. These risk factors are influenced by genes such as KCNQ1 , TCF7L2 and FTO [ 94 ]. Other systematic reviews have looked at epigenetic changes in obesity [ 95 ], aging [ 96 , 97 ] and cardiovascular complications [ 98 ]. Andrade et al . aimed to identify epigenetic changes in human adipose tissue from obese/overweight individuals with and without metabolic disorders like T2DM [ 95 ]. They also report differentially methylated genes that we have been reported in this review, such as KCNQ1 , FASN , MFSD1 , TXNIP , PPARG , IRS1 and TCF7L2 , from the same studies [ 95 ]. Krolevets et al . report that in addition to about 75,000 CpG sites and 19,000 genes, PTPRN2 was among the most frequently reported gene that was associated with cardiac disorders, although the direction of methylation is not specified [ 98 ]. Of the two studies that investigated DNA methylation in aging [ 96 , 97 ], no genes/CpG sites/studies were common with the ones mentioned in our review.
One of the most important factors in looking at T2DM as an epidemic is the geographic location of the site of reported data. With a large amount of data coming in from Europe alone, it is important to perform similar studies in other parts of the world and including various other ethnic groups to validate these reports and also help in mapping the genetic diversity to be able to tackle T2DM. India being the most populous country [ 99 ] with about 11% of Indians suffering from T2DM (in 2020) [ 100 ], it is imperative to study this population to uncover T2DM susceptible loci/genes. Of note, Chambers et al . have followed up London resident Indian Asians, for 8 years, and found that DNA methylation levels of TXNIP , PROC , C7orf29 , SREBF1 , PHOSPHO1 , SOCS3 and ABCG1 were positively associated with future T2DM incidence [ 71 ], but similar studies are lacking in Indians living in India, where exposure to pollution and availability and consumption of healthy diet are vastly different.
As for sex-specific methylation signatures of T2DM, differences were not seen between men and women except in genes HOOK2 [ 37 ] and MSI2 [ 27 ], which were hypermethylated in adipose tissue, and hypomethylated in blood, respectively . Finally, we searched if the genes which we found to be highly reported to be differentially methylated in human were also reported to be differentially methylated in animal models. KCNQ1 was reported to be hypomethylated in both T2DM human [ 58 ], and older mice model compared with younger mice [ 72 ] suggesting age-related methylation changes across species. In both humans [ 58 ], and mice fed with a high-fat diet, TCF7L2 was hypomethylated, and this DNA methylation change in mice was induced because of their diet [ 82 ], suggesting that nutrient consumption plays a role in epigenetic modification of genes involved in beta-cell function, and a healthy diet can have a protective role in maintaining homeostasis.
Although we did not look at clinical trials and candidate-gene studies that report differential DNA methylation, our review is an up-to-date report of epigenome-wide studies that includes prospective studies. We also report gene expression data in comparison with DNA methylation. Furthermore, a systematic report of differentially methylated gene/loci in tissues including blood cells, adipose tissue, pancreatic islet, skeletal muscles, liver and spermatozoa is included. While sex and ethnicity play a major role in pathology, we have tried to highlight these effects.
As with previous reviews, we emphasize the need for more prospective studies and replication of genome-wide association studies in different tissue types and populations.
From the 32 studies that report differentially methylated genes/loci between T2DM and normoglycemic individuals, ABCG1 (hypermethylated in blood), FTO (hypermethylated in blood and spermatozoa), KCNQ1 (hypermethylated in blood and hypomethylated in spermatozoa), TXNIP (hypomethylated in blood), PPARGC1A loci at chr4: 24,111,501–750 (hypermethylated in skeletal muscle and spermatozoa) and loci at chr4: 24,024,251–500 (hypomethylated in spermatozoa), PTPRN2 (hypermethylated in blood, hypomethylated in adipose tissue) were reported in more than one study. We found reports of hypermethylation of these genes that were associated with decreased gene expression, and vice versa. We also report findings from studies done on monozygotic twins. Various traits that can affect T2DM such as sex, glucose levels, BMI and ethnicity were also taken into consideration.
As there were multiple methods that were used to measure DNA methylation, internal and external validation of these results is also reported. Finally, animal model studies that have reported differential DNA methylation of the genes that were found to be differentially methylated in human studies were looked at to get an understanding of the likely mechanisms linking epigenetic dysregulation of these genes in T2DM to its pathophysiology.
Although the majority of the top differentially methylated genes are well known, other more recent genes reported here should be investigated further to understand their role in pathogenesis of T2DM.
Data availability statement
All relevant data are presented as tables and/or figures.
Abbreviations
ATP-Binding Cassette Subfamily G Member 1
Anoctamin 8
Beta 1,3-N-Acetylglucosaminyltransferase 7
Chromosome 1 Open Reading Frame 52
Chromosome 7 Open Reading Frame 29
Collagen Type XXI Alpha 1
Cytochrome B561 Family Member D1
CXXC Finger Protein 4
Alpha-Ketoglutarate Dependent Dioxygenase
Glucagon Like Peptide 1 Receptor
Glutathione Peroxidase 6
Hepatocyte Nuclear Factor 4 Alpha
Hook Microtubule Tethering Protein 2
Insulin-Like Growth Factor-Binding Protein 2
Interleukin-23 Subunit Alpha
Potassium Voltage-Gated Channel Subfamily Q Member 1
LINE1 Type Transposase Domain Containing 1
Lysyl Oxidase Homolog 2
Mucosa-Associated Lymphoid Tissue Lymphoma Translocation Protein 1
Major Facilitator Superfamily Domain Containing 1
Musashi RNA-Binding Protein 2
Platelet Derived Growth Factor Subunit A
Pancreatic and Duodenal Homeobox 1
Period Circadian Regulator 2
Phosphoethanolamine/Phosphocholine Phosphatase 1
Peroxisome Proliferator-Activated Receptor Gamma Coactivator 1-Alpha
Protein C, Inactivator Of Coagulation Factors Va And VIIIa
Polypyrimidine Tract-Binding Protein 1
Protein Tyrosine Phosphatase Receptor Type N2
Receptor Interacting Serine/Threonine Kinase 4
S100 Calcium-Binding Protein A4
Sterile Alpha Motif Domain Containing 12
Solute Carrier Family 1 Member 5
Solute Carrier Family 22 Member 1
Solute Carrier Family 22 Member 3
Solute Carrier Family 30 Member 8
Sterol Regulatory Element-Binding Transcription Factor 1
Suppressor Of Cytokine Signaling 3
Transcription Factor 7-Like 2
Thioredoxin-Interacting Protein
Cho NH, Shaw JE, Karuranga S, Huang Y, da Rocha Fernandes JD, Ohlrogge AW, et al. IDF diabetes atlas: global estimates of diabetes prevalence for 2017 and projections for 2045. Diabetes Res Clin Pract. 2018;138:271–81.
Article CAS PubMed Google Scholar
Prasad R, Groop L. Genetics of type 2 diabetes—pitfalls and possibilities. Genes. 2015;6(1):87–123.
Article CAS PubMed PubMed Central Google Scholar
Volkmar M, Dedeurwaerder S, Cunha DA, Ndlovu MN, Defrance M, Deplus R, et al. DNA methylation profiling identifies epigenetic dysregulation in pancreatic islets from type 2 diabetic patients. EMBO J. 2012;31(6):1405–26.
Jin B, Li Y, Robertson KD. DNA methylation: superior or subordinate in the epigenetic hierarchy? Genes Cancer. 2011;2(6):607–17.
Robertson KD. DNA methylation and human disease. Nat Rev Genet. 2005;6(8):597–610.
Moore LD, Le T, Fan G. DNA methylation and its basic function. Neuropsychopharmacology. 2013;38(1):23–38.
Hall E, Dayeh T, Kirkpatrick CL, Wollheim CB, Dekker Nitert M, Ling C. DNA methylation of the glucagon-like peptide 1 receptor (GLP1R) in human pancreatic islets. BMC Med Genet. 2013;14:76–76.
Willmer T, Johnson R, Louw J, Pheiffer C. Blood-based DNA methylation biomarkers for type 2 diabetes: potential for clinical applications. Front Endocrinol. 2018;4(9):744.
Article Google Scholar
Walaszczyk E, Luijten M, Spijkerman AMW, Bonder MJ, Lutgers HL, Snieder H, et al. DNA methylation markers associated with type 2 diabetes, fasting glucose and HbA1c levels: a systematic review and replication in a case–control sample of the Lifelines study. Diabetologia. 2018;61(2):354–68.
Muka T, Nano J, Voortman T, Braun KVE, Ligthart S, Stranges S, et al. The role of global and regional DNA methylation and histone modifications in glycemic traits and type 2 diabetes: a systematic review. Nutr Metab Cardiovasc Dis. 2016;26(7):553–66.
Rönn T, Ling C. DNA methylation as a diagnostic and therapeutic target in the battle against Type 2 diabetes. Epigenomics. 2015;7(3):451–60.
Article PubMed Google Scholar
Moher D, Shamseer L, Clarke M, Ghersi D, Liberati A, Petticrew M, et al. Preferred reporting items for systematic review and meta-analysis protocols (PRISMA-P) 2015 statement. Syst Rev. 2015;1(4):1.
Wells G, Shea B, O’Connell D, Peterson J, Welch, Losos M, et al. The Newcastle-Ottawa Scale (NOS) for Assessing the Quality of Nonrandomised Studies in Meta-Analyses. 2014. http://www.ohri.ca/programs/clinical_epidemiology/oxford.asp
PROSPERO. [cited 2023 Apr 18]. https://www.crd.york.ac.uk/prospero/
Evangelista JE, Xie Z, Marino GB, Nguyen N, Clarke DJB, Ma’ayan A. Enrichr-KG: bridging enrichment analysis across multiple libraries. Nucl Acids Res. 2023;gkad393.
Soriano-Tárraga C, Jiménez-Conde J, Giralt-Steinhauer E, Mola-Caminal M, Vivanco-Hidalgo RM, Ois A, et al. Epigenome-wide association study identifies TXNIP gene associated with type 2 diabetes mellitus and sustained hyperglycemia. Hum Mol Genet. 2016;25(3):609–19.
Wang Z, Peng H, Gao W, Cao W, Lv J, Yu C, et al. Blood DNA methylation markers associated with type 2 diabetes, fasting glucose, and HbA1c levels: an epigenome-wide association study in 316 adult twin pairs. Genomics. 2021;113(6):4206–13.
Kriebel J, Herder C, Rathmann W, Wahl S, Kunze S, Molnos S, et al. Association between DNA methylation in whole blood and measures of glucose metabolism: KORA F4 study. PLoS ONE. 2016;11(3):e0152314–e0152314.
Article PubMed PubMed Central Google Scholar
Kulkarni H, Kos MZ, Neary J, Dyer TD, Kent JWJ, Göring HHH, et al. Novel epigenetic determinants of type 2 diabetes in Mexican-American families. Hum Mol Genet. 2015;24(18):5330–44.
Meeks KAC, Henneman P, Venema A, Addo J, Bahendeka S, Burr T, et al. Epigenome-wide association study in whole blood on type 2 diabetes among sub-Saharan African individuals: findings from the RODAM study. Int J Epidemiol. 2019;48(1):58–70.
Xiang Y, Wang Z, Hui Q, Gwinn M, Vaccarino V, Sun YV. DNA Methylation of TXNIP independently associated with inflammation and diabetes mellitus in twins. Twin Res Hum Genet. 2021;24(5):273–80.
Dawes K, Philibert W, Darbro B, Simons RL, Philibert R. Additive and interactive genetically contextual effects of HbA1c on cg19693031 methylation in type 2 diabetes. Genes (Basel). 2022;13(4):683.
Tsai HH, Shen CY, Ho CC, Hsu SY, Tantoh DM, Nfor ON, et al. Interaction between a diabetes-related methylation site (TXNIP cg19693031) and variant (GLUT1 rs841853) on fasting blood glucose levels among non-diabetics. J Transl Med. 2022;20(1):87.
Khamis A, Ning L, Balkau B, Bonnefond A, Canouil M, Roussel R, et al. Epigenetic changes associated with hyperglycaemia exposure in the longitudinal DESIR cohort. Diabetes Metab. 2022;48(4):101347.
Florath I, Butterbach K, Heiss J, Bewerunge-Hudler M, Zhang Y, Schöttker B, et al. Type 2 diabetes and leucocyte DNA methylation: an epigenome-wide association study in over 1,500 older adults. Diabetologia. 2016;59(1):130–8.
Kuroda A, Rauch TA, Todorov I, Ku HT, Al-Abdullah IH, Kandeel F, et al. Insulin gene expression is regulated by DNA methylation. PLoS ONE. 2009;4(9):6953.
Jeon JP, Koh IU, Choi NH, Kim BJ, Han BG, Lee S. Differential DNA methylation of MSI2 and its correlation with diabetic traits. PLoS ONE. 2017;12(5):e0177406–e0177406.
Dayeh T, Volkov P, Salö S, Hall E, Nilsson E, Olsson AH, et al. Genome-wide DNA methylation analysis of human pancreatic islets from type 2 diabetic and non-diabetic donors identifies candidate genes that influence insulin secretion. PLoS Genet. 2014;10(3):e1004160–e1004160.
Sjöholm LK, Ransome Y, Ekström TJ, Karlsson O. Evaluation of post-mortem effects on global brain DNA methylation and hydroxymethylation. Basic Clin Pharmacol Toxicol. 2018;122(2):208–13.
Vilahur N, Baccarelli AA, Bustamante M, Agramunt S, Byun HM, Fernandez MF, et al. Storage conditions and stability of global DNA methylation in placental tissue. Epigenomics. 2013;5(3):341–8.
Makki K, Froguel P, Wolowczuk I. Adipose tissue in obesity-related inflammation and insulin resistance: cells, cytokines, and chemokines. ISRN Inflammation. 2013;22(2013):1–12.
Dahlman I, Sinha I, Gao H, Brodin D, Thorell A, Rydén M, et al. The fat cell epigenetic signature in post-obese women is characterized by global hypomethylation and differential DNA methylation of adipogenesis genes. Int J Obes. 2015;39(6):910–9.
Article CAS Google Scholar
Fujiki K, Shinoda A, Kano F, Sato R, Shirahige K, Murata M. PPARγ-induced PARylation promotes local DNA demethylation by production of 5-hydroxymethylcytosine. Nat Commun. 2013;4(1):2262.
Pfeiffer S, Krüger J, Maierhofer A, Böttcher Y, Klöting N, El Hajj N, et al. Hypoxia-inducible factor 3A gene expression and methylation in adipose tissue is related to adipose tissue dysfunction. Sci Rep. 2016;6(1):27969.
Wang X, Cao Q, Yu L, Shi H, Xue B, Shi H. Epigenetic regulation of macrophage polarization and inflammation by DNA methylation in obesity. JCI Insight. 2016 Nov 17 [cited 2023 Jun 17]; 1(19). https://insight.jci.org/articles/view/87748
Nilsson E, Jansson PA, Perfilyev A, Volkov P, Pedersen M, Svensson MK, et al. Altered DNA methylation and differential expression of genes influencing metabolism and inflammation in adipose tissue from subjects with type 2 diabetes. Diabetes. 2014;63(9):2962–76.
Rodríguez-Rodero S, Menéndez-Torre E, Fernández-Bayón G, Morales-Sánchez P, Sanz L, Turienzo E, et al. Altered intragenic DNA methylation of HOOK2 gene in adipose tissue from individuals with obesity and type 2 diabetes. PLoS ONE. 2017;12(12):e0189153–e0189153.
Wang C, Ha X, Li W, Xu P, Zhang Z, Wang T, et al. Comparative gene expression profile and DNA methylation status in diabetic patients of Kazak and Han people. Medicine. 2018;97(36):e11982–e11982.
Ribel-Madsen R, Fraga MF, Jacobsen S, Bork-Jensen J, Lara E, Calvanese V, et al. Genome-wide analysis of DNA methylation differences in muscle and fat from monozygotic twins discordant for type 2 diabetes. PLoS ONE. 2012;7(12):e51302–e51302.
Andersen E, Ingerslev LR, Fabre O, Donkin I, Altıntaş A, Versteyhe S, et al. Preadipocytes from obese humans with type 2 diabetes are epigenetically reprogrammed at genes controlling adipose tissue function. Int J Obes. 2019;43(2):306–18.
Strausberg RL, Feingold EA, Grouse LH, Derge JG, Klausner RD, Collins FS, et al. Generation and initial analysis of more than 15,000 full-length human and mouse cDNA sequences. Proc Natl Acad Sci U S A. 2002;99(26):16899–903.
Walenta JH, Didier AJ, Liu X, Krämer H. The golgi-associated Hook3 protein is a member of a novel family of microtubule-binding proteins. J Cell Biol. 2001;152(5):923–34.
Massa López D, Thelen M, Stahl F, Thiel C, Linhorst A, Sylvester M, et al. The lysosomal transporter MFSD1 is essential for liver homeostasis and critically depends on its accessory subunit GLMP. Elife. 2019;8:e50025.
Kashoor I, Batlle D. Proximal renal tubular acidosis with and without Fanconi syndrome. Kidney Res Clin Pract. 2019;38(3):267–81.
Yamagata K. Roles of HNF1α and HNF4α in Pancreatic β-Cells. In: Vitamins & Hormones [Internet]. Elsevier; 2014 [cited 2023 Aug 5]. pp. 407–23. https://linkinghub.elsevier.com/retrieve/pii/B9780128001745000168
Hayhurst GP, Lee YH, Lambert G, Ward JM, Gonzalez FJ. Hepatocyte nuclear factor 4α (Nuclear Receptor 2A1) is essential for maintenance of hepatic gene expression and lipid homeostasis. Mol Cell Biol. 2001;21(4):1393–403.
Stoffel M, Duncan SA. The maturity-onset diabetes of the young (MODY1) transcription factor HNF4α regulates expression of genes required for glucose transport and metabolism. Proc Natl Acad Sci USA. 1997;94(24):13209–14.
Vedi M, Smith JR, Thomas Hayman G, Tutaj M, Brodie KC, De Pons JL, et al. 2022 updates to the rat genome database: a findable, accessible, interoperable, and reusable (FAIR) resource. Genetics. 2023;224(1):1042.
Han HS, Kang G, Kim JS, Choi BH, Koo SH. Regulation of glucose metabolism from a liver-centric perspective. Exp Mol Med. 2016;48(3):e218–e218.
Abderrahmani A, Yengo L, Caiazzo R, Canouil M, Cauchi S, Raverdy V, et al. Increased hepatic PDGF-AA signaling mediates liver insulin resistance in obesity-associated type 2 diabetes. Diabetes. 2018;67(7):1310–21.
Nilsson E, Matte A, Perfilyev A, de Mello VD, Käkelä P, Pihlajamäki J, et al. Epigenetic alterations in human liver from subjects with type 2 diabetes in parallel with reduced folate levels. J Clin Endocrinol Metab. 2015;100(11):E1491-1501.
Barres R, Osler ME, Yan J, Rune A, Fritz T, Caidahl K, et al. Non-CpG methylation of the PGC-1alpha promoter through DNMT3B controls mitochondrial density. Cell Metab. 2009;10(3):189–98.
Yuan W, Xia Y, Bell CG, Yet I, Ferreira T, Ward KJ, et al. An integrated epigenomic analysis for type 2 diabetes susceptibility loci in monozygotic twins. Nat Commun. 2014;5:5719–5719.
Kiechl S, Wittmann J, Giaccari A, Knoflach M, Willeit P, Bozec A, et al. Blockade of receptor activator of nuclear factor-κB (RANKL) signaling improves hepatic insulin resistance and prevents development of diabetes mellitus. Nat Med. 2013;19(3):358–63.
Dayeh T, Tuomi T, Almgren P, Perfilyev A, Jansson PA, De Mello VD, et al. DNA methylation of loci within ABCG1 and PHOSPHO1 in blood DNA is associated with future type 2 diabetes risk. Epigenetics. 2016;11(7):482–8.
Chen YT, Lin WD, Liao WL, Tsai YC, Liao JW, Tsai FJ. NT5C2 methylation regulatory interplay between DNMT1 and insulin receptor in type 2 diabetes. Sci Rep. 2020;10(1):16087.
Chen X, Lin Q, Wen J, Lin W, Liang J, Huang H, et al. Whole genome bisulfite sequencing of human spermatozoa reveals differentially methylated patterns from type 2 diabetic patients. Journal of diabetes investigation. 2019
Toperoff G, Aran D, Kark JD, Rosenberg M, Dubnikov T, Nissan B, et al. Genome-wide survey reveals predisposing diabetes type 2-related DNA methylation variations in human peripheral blood. Hum Mol Genet. 2012;21(2):371–83.
Volkov P, Bacos K, Ofori JK, Esguerra JLS, Eliasson L, Rönn T, et al. Whole-genome bisulfite sequencing of human pancreatic islets reveals novel differentially methylated regions in type 2 diabetes pathogenesis. Diabetes. 2017;66(4):1074–85.
García-Calzón S, Perfilyev A, Männistö V, de Mello VD, Nilsson E, Pihlajamäki J, et al. Diabetes medication associates with DNA methylation of metformin transporter genes in the human liver. Clin Epigenetics. 2017;9:102–102.
Zampieri M, Bacalini MG, Barchetta I, Scalea S, Cimini FA, Bertoccini L, et al. Increased PARylation impacts the DNA methylation process in type 2 diabetes mellitus. Clin Epigenet. 2021;13(1):114.
Qie R, Chen Q, Wang T, Chen X, Wang J, Cheng R, et al. Association of ABCG1 gene methylation and its dynamic change status with incident type 2 diabetes mellitus: the Rural Chinese Cohort Study. J Hum Genet. 2021;66(4):347–57.
Krause C, Sievert H, Geißler C, Grohs M, El Gammal AT, Wolter S, et al. Critical evaluation of the DNA-methylation markers ABCG1 and SREBF1 for Type 2 diabetes stratification. Epigenomics. 2019;11(8):885–97.
van Otterdijk SD, Binder AM, Szarc Vel Szic K, Schwald J, Michels KB. DNA methylation of candidate genes in peripheral blood from patients with type 2 diabetes or the metabolic syndrome. PLoS ONE. 2017;12(7):e0180955–e0180955.
Toperoff G, Kark JD, Aran D, Nassar H, Ahmad WA, Sinnreich R, et al. Premature aging of leukocyte DNA methylation is associated with type 2 diabetes prevalence. Clin Epigenetics. 2015;7(1):35–35.
Huang S, Qin P, Chen Q, Zhang D, Cheng C, Guo C, et al. Association of FTO gene methylation with incident type 2 diabetes mellitus: A nested case–control study. Gene. 2021;786: 145585.
Zhang D, Cheng C, Cao M, Wang T, Chen X, Zhao Y, et al. TXNIP hypomethylation and its interaction with obesity and hypertriglyceridemia increase type 2 diabetes mellitus risk: a nested case-control study. J Diabetes. 2020;12(7):512–20.
Hu F, Zhang Y, Qin P, Zhao Y, Liu D, Zhou Q, et al. Integrated analysis of probability of type 2 diabetes mellitus with polymorphisms and methylation of KCNQ1 gene: a nested case-control study. J Diabetes. 2021;13(12):975–86.
Ling C, Del Guerra S, Lupi R, Rönn T, Granhall C, Luthman H, et al. Epigenetic regulation of PPARGC1A in human type 2 diabetic islets and effect on insulin secretion. Diabetologia. 2008;51(4):615–22.
Wittenbecher C, Ouni M, Kuxhaus O, Jähnert M, Gottmann P, Teichmann A, et al. Insulin-like growth factor binding protein 2 (IGFBP-2) and the risk of developing type 2 diabetes. Diabetes. 2019;68(1):188–97.
Chambers JC, Loh M, Lehne B, Drong A, Kriebel J, Motta V, et al. Epigenome-wide association of DNA methylation markers in peripheral blood from Indian Asians and Europeans with incident type 2 diabetes: a nested case-control study. Lancet Diabetes Endocrinol. 2015;3(7):526–34.
Sandovici I, Hammerle CM, Cooper WN, Smith NH, Tarry-Adkins JL, Dunmore BJ, et al. Ageing is associated with molecular signatures of inflammation and type 2 diabetes in rat pancreatic islets. Diabetologia. 2016;59(3):502–11.
Lee YH, Kang ES, Kim SH, Han SJ, Kim CH, Kim HJ, et al. Association between polymorphisms in SLC30A8, HHEX, CDKN2A/B, IGF2BP2, FTO, WFS1, CDKAL1, KCNQ1 and type 2 diabetes in the Korean population. J Hum Genet. 2008;53(11–12):991–8.
Liu Y, Zhou DZ, Zhang D, Chen Z, Zhao T, Zhang Z, et al. Variants in KCNQ1 are associated with susceptibility to type 2 diabetes in the population of mainland China. Diabetologia. 2009;52(7):1315–21.
Hu C, Wang C, Zhang R, Ma X, Wang J, Lu J, et al. Variations in KCNQ1 are associated with type 2 diabetes and beta cell function in a Chinese population. Diabetologia. 2009;52(7):1322–5.
Jonsson A, Isomaa B, Tuomi T, Taneera J, Salehi A, Nilsson P, et al. A variant in the KCNQ1 gene predicts future type 2 diabetes and mediates impaired insulin secretion. Diabetes. 2009;58(10):2409–13.
Yasuda K, Miyake K, Horikawa Y, Hara K, Osawa H, Furuta H, et al. Variants in KCNQ1 are associated with susceptibility to type 2 diabetes mellitus. Nat Genet. 2008;40(9):1092–7.
Unoki H, Takahashi A, Kawaguchi T, Hara K, Horikoshi M, Andersen G, et al. SNPs in KCNQ1 are associated with susceptibility to type 2 diabetes in East Asian and European populations. Nat Genet. 2008;40(9):1098–102.
Müssig K, Staiger H, Machicao F, Kirchhoff K, Guthoff M, Schäfer SA, et al. Association of Type 2 diabetes candidate polymorphisms in KCNQ1 with incretin and insulin secretion. Diabetes. 2009;58(7):1715–20.
Qian Y, Dong M, Lu F, Li H, Jin G, Hu Z, et al. Joint effect of CENTD2 and KCNQ1 polymorphisms on the risk of type 2 diabetes mellitus among Chinese Han population. Mol Cell Endocrinol. 2015;407:46–51.
Been LF, Ralhan S, Wander GS, Mehra NK, Singh J, Mulvihill JJ, et al. Variants in KCNQ1 increase type II diabetes susceptibility in South Asians: a study of 3,310 subjects from India and the US. BMC Med Genet. 2011;12(1):18.
Hu Y, Shi P, He K, Zhu YQ, Yang F, Yang M, et al. Methylation of Tcf712 promoter by high-fat diet impairs β-cell function in mouse pancreatic islets. Diabetes Metab Res Rev. 2018;34(4): e2980.
Flurkey K, Currer JM, Harrison D. Mouse models in aging research. In: The mouse in biomedical research. Elsevier; 2007, pp. 637–72.
Papadopoulou S, Edlund H. Attenuated Wnt signaling perturbs pancreatic growth but not pancreatic function. Diabetes. 2005;54(10):2844–51.
Kumar A, Mittal R. Mapping Txnip: key connexions in progression of diabetic nephropathy. Pharmacol Rep. 2018;70(3):614–22.
Minn AH, Hafele C, Shalev A. Thioredoxin-interacting protein is stimulated by glucose through a carbohydrate response element and induces β-cell apoptosis. Endocrinology. 2005;146(5):2397–405.
Tarling EJ, Edwards PA. ATP binding cassette transporter G1 (ABCG1) is an intracellular sterol transporter. Proc Natl Acad Sci USA. 2011;108(49):19719–24.
Westerterp M, Bochem AE, Yvan-Charvet L, Murphy AJ, Wang N, Tall AR. ATP-binding cassette transporters, atherosclerosis, and inflammation. Circ Res. 2014;114(1):157–70.
Wilson PW, McGEE DL, Kannel WB. Obesity, very low density lipoproteins, and glucose intolerance over fourteen years: The Framingham Study. Am J Epidemiol. 1981;114(5):697–704.
Shah UJ, Xie W, Flyvbjerg A, Nolan JJ, Højlund K, Walker M, et al. Differential methylation of the type 2 diabetes susceptibility locus KCNQ1 is associated with insulin sensitivity and is predicted by CpG site specific genetic variation. Diabetes Res Clin Pract. 2019;148:189–99.
Mattei AL, Bailly N, Meissner A. DNA methylation: a historical perspective. Trends Genet. 2022;38(7):676–707.
Anastasiadi D, Esteve-Codina A, Piferrer F. Consistent inverse correlation between DNA methylation of the first intron and gene expression across tissues and species. Epigenetics Chromatin. 2018;11(1):37.
Ball MP, Li JB, Gao Y, Lee JH, LeProust EM, Park IH, et al. Targeted and genome-scale strategies reveal gene-body methylation signatures in human cells. Nat Biotechnol. 2009;27(4):361–8.
Berumen J, Orozco L, Betancourt-Cravioto M, Gallardo H, Zulueta M, Mendizabal L, et al. Influence of obesity, parental history of diabetes, and genes in type 2 diabetes: a case-control study. Sci Rep. 2019;9(1):2748.
Andrade S, Morais T, Sandovici I, Seabra AL, Constância M, Monteiro MP. Adipose tissue epigenetic profile in obesity-related dysglycemia: a systematic review. Front Endocrinol. 2021;29(12): 681649.
Ryan J, Wrigglesworth J, Loong J, Fransquet PD, Woods RL. A systematic review and meta-analysis of environmental, lifestyle, and health factors associated with DNA methylation age. J Gerontol Ser A. 2020;75(3):481–94.
Oblak L, Van Der Zaag J, Higgins-Chen AT, Levine ME, Boks MP. A systematic review of biological, social and environmental factors associated with epigenetic clock acceleration. Ageing Res Rev. 2021;69: 101348.
Krolevets M, Cate VT, Prochaska JH, Schulz A, Rapp S, Tenzer S, et al. DNA methylation and cardiovascular disease in humans: a systematic review and database of known CpG methylation sites. Clin Epigenet. 2023;15(1):56.
Department of Economic and Social Affairs UN. India to overtake China as world’s most populous country in April 2023, United Nations projects. [cited 2023 Jun 8]. https://www.un.org/en/desa/india-overtake-china-world-most-populous-country-april-2023-united-nations-projects
Anjana RM, Unnikrishnan R, Deepa M, Pradeepa R, Tandon N, Das AK, et al. Metabolic non-communicable disease health report of India: the ICMR-INDIAB national cross-sectional study (ICMR-INDIAB-17). Lancet Diabetes Endocrinol. 2023;11(7):474–89.
Hwang JY, Lee HJ, Go MJ, Jang HB, Choi NH, Bae JB, et al. Genome-wide methylation analysis identifies ELOVL5 as an epigenetic biomarker for the risk of type 2 diabetes mellitus. Sci Rep. 2018;8(1):14862–14862.
Zou L, Yan S, Guan X, Pan Y, Qu X. Hypermethylation of the PRKCZ Gene in Type 2 diabetes mellitus. J Diabetes Res. 2013;2013:721493–721493.
Chen YT, Liao JW, Tsai YC, Tsai FJ. Inhibition of DNA methyltransferase 1 increases nuclear receptor subfamily 4 group A member 1 expression and decreases blood glucose in type 2 diabetes. Oncotarget. 2016;7(26):39162–70.
Davegårdh C, Säll J, Benrick A, Broholm C, Volkov P, Perfilyev A, et al. VPS39-deficiency observed in type 2 diabetes impairs muscle stem cell differentiation via altered autophagy and epigenetics. Nat Commun. 2021;12(1):2431.
Whytock KL, Pino MF, Sun Y, Yu G, De Carvalho FG, Yeo RX, et al. Comprehensive interrogation of human skeletal muscle reveals a dissociation between insulin resistance and mitochondrial capacity. Am J Physiol-Endocrinol Metabol. 2023;325(4):E291-302.
Download references
Acknowledgements
AM is supported by the Wellcome Trust/DBT India Alliance Fellowship [Grant Number IA/CPHI/19/1/504593]. We thank Ms. Ramya for her insightful comments.
This research did not receive any specific grant from any funding agency in the public, commercial or not-for-profit sector.
Author information
Priyanka Chinya Nataraj
Present address: Vedantu, Bangalore, India
Authors and Affiliations
Research Scholar, Manipal Academy of Higher Education, Manipal, India
Nikhil Nadiger
Division of Nutrition, St. John’s Research Institute, St. John’s Medical College, St Johns National Academy of Health Sciences, Sarjapura Road, Koramangala, Bangalore, 560034, India
Nikhil Nadiger, Jyothisha Kana Veed, Priyanka Chinya Nataraj & Arpita Mukhopadhyay
You can also search for this author in PubMed Google Scholar
Contributions
AM was involved in conceptualization, review and editing of the manuscript. NN, JKV and PCN were involved in data extraction, formal analysis, investigation and writing the manuscript. All authors have read and approved the final manuscript.
Corresponding author
Correspondence to Arpita Mukhopadhyay .
Ethics declarations
Competing interests.
The authors declare no competing interests.
Ethics approval and consent to participate
Not applicable.
Consent for publication
Conflict of interest.
The authors declare no conflict of interest.
Additional information
Publisher's note.
Springer Nature remains neutral with regard to jurisdictional claims in published maps and institutional affiliations.
Supplementary Information
Additional file 1 search strategy for the systematic review of dna methylation association with t2dm, 13148_2024_1670_moesm2_esm.docx.
Additional file 2 Qualitative assessment of research articles included in the review based on the New Castle Ottawa Scale (NOS)
Rights and permissions
Open Access This article is licensed under a Creative Commons Attribution 4.0 International License, which permits use, sharing, adaptation, distribution and reproduction in any medium or format, as long as you give appropriate credit to the original author(s) and the source, provide a link to the Creative Commons licence, and indicate if changes were made. The images or other third party material in this article are included in the article's Creative Commons licence, unless indicated otherwise in a credit line to the material. If material is not included in the article's Creative Commons licence and your intended use is not permitted by statutory regulation or exceeds the permitted use, you will need to obtain permission directly from the copyright holder. To view a copy of this licence, visit http://creativecommons.org/licenses/by/4.0/ . The Creative Commons Public Domain Dedication waiver ( http://creativecommons.org/publicdomain/zero/1.0/ ) applies to the data made available in this article, unless otherwise stated in a credit line to the data.
Reprints and permissions
About this article
Cite this article.
Nadiger, N., Veed, J.K., Chinya Nataraj, P. et al. DNA methylation and type 2 diabetes: a systematic review. Clin Epigenet 16 , 67 (2024). https://doi.org/10.1186/s13148-024-01670-6
Download citation
Received : 20 January 2024
Accepted : 11 April 2024
Published : 16 May 2024
DOI : https://doi.org/10.1186/s13148-024-01670-6
Share this article
Anyone you share the following link with will be able to read this content:
Sorry, a shareable link is not currently available for this article.
Provided by the Springer Nature SharedIt content-sharing initiative
- Type 2 diabetes
- DNA methylation
- Epigenome-wide association studies
- Epigenetics
Clinical Epigenetics
ISSN: 1868-7083
- Submission enquiries: [email protected]
- General enquiries: [email protected]
Thank you for visiting nature.com. You are using a browser version with limited support for CSS. To obtain the best experience, we recommend you use a more up to date browser (or turn off compatibility mode in Internet Explorer). In the meantime, to ensure continued support, we are displaying the site without styles and JavaScript.
- View all journals
- My Account Login
- Explore content
- About the journal
- Publish with us
- Sign up for alerts
- Open access
- Published: 14 May 2024
Association of type 2 diabetes with family history of diabetes, diabetes biomarkers, mental and physical disorders in a Kenyan setting
- David M. Ndetei 1 , 2 , 3 ,
- Victoria Mutiso 2 , 3 ,
- Christine Musyimi 2 , 3 ,
- Pascalyne Nyamai 2 , 3 ,
- Cathy Lloyd 4 &
- Norman Sartorius 5
Scientific Reports volume 14 , Article number: 11037 ( 2024 ) Cite this article
125 Accesses
Metrics details
- Endocrinology
- Risk factors
This study aimed to determine the degree of family relations and associated socio-demographics characteristics, clinical/physical and mental disorders in type 2 diabetes mellitus in a Kenyan diabetes clinic. This study was part of a large multicentre study whose protocol and results had been published. It took place at the outpatient diabetes clinic at a County Teaching and Referral Hospital in South East Kenya involving 182 participants. We used a socio-demographic questionnaire, the Hamilton Depression (HAM-D) and PHQ-9 rating scales for depression, the MINI International Neuropsychiatric Interview (MINI; V5 or V6) for DSM-5 diagnoses, the WHO-5 Well-being scale and Problem Areas in Diabetes Scale (PAID). We extracted from the notes all physical conditions. We enquired about similar conditions in 1st and 2nd degree relatives. Descriptive, Chi-square test, Fisher’s exact test, one way ANOVA, and Multinomial logistic regression analysis were conducted to test achievements of our specific aims. Of the 182 patients who participated in the study, 45.1% (82/182) reported a family history of diabetes. Conditions significantly ( p < 0.05) associated with a degree of family history of diabetes were retinopathy, duration of diabetes (years), hypertension, and depressive disorder. On average 11.5% (21/182) scored severe depression (≥ 10) on PHQ-9 and 85.2% (115/182) scored good well-being (≥ 13 points). All DSM-5 psychiatric conditions were found in the 182 patients in varying prevalence regardless of relations. In addition, amongst the 182 patients, the highest prevalence was poor well-being on the WHO quality of life tool. This was followed by post-traumatic disorders (current), suicidality, and psychotic lifetime on DSM-5. The least prevalent on DSM-5 was eating disorders. Some type 2 diabetes mellitus physical disorders and depression have increased incidence in closely related patients. Overall, for all the patients, the prevalence of all DSM-5 diagnoses varied from 0.5 to 9.9%.
Similar content being viewed by others
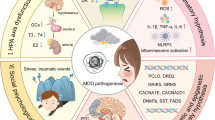
Major depressive disorder: hypothesis, mechanism, prevention and treatment
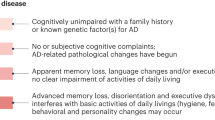
Alzheimer’s disease risk reduction in clinical practice: a priority in the emerging field of preventive neurology
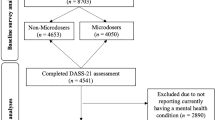
Adults who microdose psychedelics report health related motivations and lower levels of anxiety and depression compared to non-microdosers
Introduction.
Family history is a non-modifiable risk factor for diabetes 1 , 2 , 3 , 4 , 5 , 6 , 7 , 8 . The risk of developing type 2 diabetes mellitus (T2DM) increases approximately two to four times when either or both parents have T2DM 5 . Between 60 and 68.8% of diabetes patients have at least one family member with a history of diabetes 2 , 6 . Paternal history is significantly associated with higher chances of having T2DM 1 . An early age onset of T2DM is more likely if a family member had also an early onset of diabetes 2 , 8 , 9 , 10 .
A positive family history of diabetes is associated with increased levels of obesity, impaired glucose tolerance, fasting triglycerides, hemoglobin AIc (HbA1c), insulin dose per kilogram, lower levels of high-density lipoprotein cholesterol 3 , 8 , 11 , 12 , a greater waist to hip ratio as well as greater body mass index (BMI) 13 and a high prevalence of diabetes complications, particularly retinopathy and dyslipidemia compared to those without a relative with diabetes 9 . More specifically and in addition, there is an impact on leptin, (a hormone that regulates fat storage in the body) 14 , a high prevalence of hypertension, and lower low-density lipoprotein (LDL) cholesterol levels in those with fathers with T2DM as opposed to those with mothers with diabetes 15 . Various physical conditions are associated with diabetes. These include cardiovascular diseases 16 , 17 , hypertension 18 , thyroid abnormality, and diabetes complications such as retinopathy, neuropathy, and stroke 17 , 19 , 20 as well as high levels of biomarkers such as hemoglobin AIc (HbAIc) and cholesterol 21 .
Mental disorders such as schizophrenia, major depressive disorder, and bipolar disorder are associated with a family history of diabetes 22 , 23 , 24 . The risk of developing diabetes is three times higher in individuals with schizophrenia than in the normal population 23 . Siblings of schizophrenic parents are more likely to develop T2DM than those whose parents do not have schizophrenia 25 .
T2DM is also associated with anxiety, Post Traumatic Stress Disorder (PTSD), depression 26 , 27 , 28 , and eating disorders 29 , 30 , 31 . Research in this area is lacking in a Kenyan setting and is urgently required in order to inform clinical practice and potential community-based interventions.
Studies in African countries on the association between diabetes and family history have largely confirmed the global trends, showing an increased frequency of T2DM in persons with a family history of diabetes and an early onset of diabetes between 18 and 30 years 32 , 33 , 34 , 35 . Significantly higher blood glucose levels have been reported in those with a maternal family history of diabetes than in those without such a history 36 . Kenyan studies have found that people with T2DM are likely to have a positive family history specifically a first-degree relative and are also likely to develop diabetes early in life 37 , 38 . First-degree relations include an individual's biological parents, siblings, and children. Second-degree relatives include an individual's grandparents, grandchildren, uncles, aunts, nephews, nieces, and half-siblings. No study in Kenya has examined how physical conditions and mental disorders are comorbid in patients with T2DM or has examined the degree of family relations and how these vary with socio-demographics, measures of well-being, stress levels related to diabetes, and the prevalence of DSM-5 diagnoses in the Type 2 Diabetes. This information would inform an integrated approach to management. This study sought to fill these gaps.
The primary objective of this study was to determine the degree of family relations and associated socio-demographic characteristics, physical and mental disorders in people with T2DM. The secondary objective was to determine the overall prevalences of physical disorders and mental disorders in T2DM regardless of family relations.
The primary specific aims were:
To determine the relationships between social demographics in T2DM in different degrees of family relations
To determine the patterns of physical disorders and physical characteristics of T2DM in different degrees of family relations
To determine the mental health and disorders associated with T2DM in different degrees of family relations
To determine the independent predictors of T2DM in different degrees of family relations
The secondary specific aims were:
To determine the overall prevalence of physical disorders in T2DM
To determine the overall prevalence of mental disorders (stress, wellbeing, and psychiatric disorders) in T2DM
To determine the independent predictors of depression in T2DM
Study design and setting
This study was part of a larger multicentre study whose protocol has been published previously 39 . It took place between September 2013 and May 2015 at an outpatient diabetes clinic in one of the County Teaching and Referral Hospital in South East Kenya approximately 60 Kilometres from Nairobi. The clinic is run by a diabetologist and a team trained in diabetes management, offering psychoeducation, and counselling.
Study participants
Between September 2013 and May 2015, a sample of consecutive outpatient clinic attendees with T2DM were invited to participate in the study. Inclusion criteria were adults aged 18–65 with T2DM diagnosed at least 12 months earlier and able to give informed consent. Exclusion criteria included: communication and cognitive difficulties; life threatening or serious conditions in the previous 6 months and being an inpatient (as this may have indicated a serious condition); pregnant women or in the first 6 months post-partum clinic; substance use dependency or a current schizophrenic illness. All patients who met the inclusion criteria and did not have any exclusion criteria consented to the study and were included.
The trained research assistant completed a form that contained information from the medical records such as age, duration of diabetes, and presence/history of diabetes complications i.e. cardiovascular disease, retinopathy, peripheral neuropathy, peripheral vascular disease, and renal disease and associated disorders as well as the most recent measurement of blood pressure, HbA1c, height and weight.
For this study, we recorded the family history of T2DM in the following:
History of diabetes in 1st degree relatives (parent or sibling)
History of diabetes in 2nd degree relatives (grandparents, aunt, uncle, and cousin)
History of diabetes in both 1st and 2nd degree relatives
Study instruments
A standardised template for extracting information from the medical records on socio-demographic data and various medical complications known to be associated with T2DM, and laboratory indicators of T2DM was utilised. We also enquired about the history of smoking.
The following psychometric instruments were administered by a trained research assistant: (i) the Patient Health Questionnaire (PHQ-9), (ii) the Hamilton Depression (HAM-D) rating scale, (iii) the WHO-5 wellbeing scale, (iv) the Problem Areas in Diabetes Scale (PAID) and (v) the MINI International Neuropsychiatric Interview (MINI; V5 or V6). The psychometric properties of these instruments have been described in the protocol for this study 39 but also summarized here for quick reference. The PHQ-9 consists of 9 items on a 4-point Likert-type scale (0 = not at all; 1 = several days; 2 = more than half the days; 3 = nearly every day) with a total score ranging from 0–27. It has good psychometric properties and has been used extensively in many culturally diverse countries 40 . PHQ-9 scores with cut-off points of 1, 5, 10, 15, and 20 represent minimal, mild, moderate, moderately severe, and severe depression, respectively. Moderate to severe depressive symptomatology was defined as PHQ-9 scores > 10 , as this was a research study rather than clinical practice where a significant level of symptoms would usually be considered as PHQ-9 scores above 15 41 . The Hamilton Depression (HAM-D) Rating Scale has been considered a gold standard in depression studies and a preferred scale in the evaluation of depression treatment 42 .
It is the most widely employed depression scale on a global scale 43 and has been administered to various patient populations ranging from psychiatric, medical, and other research settings 44 . The HAM-D Rating Scale is a 17-item tool that takes 20–30 min to administer and scored between 0 and 4 points. Scores of 0–7 indicate normal, 8–16 indicate mild depression, 17–23 moderate depression, and counts over 24 are indicative of severe depression 42 . It has good psychometric properties with sufficient reliability (internal, inter-rater, and retest safety) and efficacy (convergent, discriminant, and predictive validity) 44 . The WHO-5 wellbeing scale is a 5-item questionnaire that measures a person’s overall psychological wellbeing 45 . The items are ‘I have felt cheerful and in good spirits’, ‘I have felt calm and relaxed’, ‘I have felt active and vigorous’, ‘I woke up feeling fresh and rested’, and ‘My daily life has been filled with things that interest me’. Poor wellbeing was defined as WHO-5 scores < 13 . The PAID is a 20-item questionnaire which measures the extent of diabetes-related emotional distress 46 . Items include ‘feeling overwhelmed with your diabetes’ and ‘feelings of guilt or anxiety when you get off track with your diabetes management’. Moderate-severe levels of diabetes-related distress are defined as scores (standardized to 100) > 40 46 . The MINI has been widely used in a range of different populations—including those with serious illnesses and in community surveys and is a reliable diagnostic tool according to DSM-V criteria 47 . It can be administrated by trained non-mental health specialists. Individuals diagnosed with depression (or other psychiatric disorders such as anxiety disorders) were advised to consult their physician for further assessment and treatment with a view to referral to the hospital psychiatric services. If any individual indicated suicidality (question 9 on the PHQ-9) immediate referral was made to the psychiatric service at the hospital.
Ethical consideration
Ethical approval was granted by the Kenyatta National Hospital—University of Nairobi (KNH-UoN) Ethics and Research Committee (ERC) (#P470/09/2013). All methods were performed in accordance with relevant guidelines and regulations as per the World Medical Association Declaration of Helsinki—ethical principles for medical research involving human subjects. Informed written consent was obtained from participants. For illiterate participants, informed written consent was obtained from their guardian/legally authorised representative.
Data analysis
This was performed using SPSS version 21 (IBM, Chicago, IL). All continuous variables were tested for normality using the Shapiro–Wilk test. Basic descriptive statistics in the form of frequency, percentage, mean, and standard deviation were carried out. The chi-square test or Fisher's exact test were used where appropriate to analyze the difference in the prevalence between family history of diabetes across different categories of socio-demographics, physical and mental disorder variables. Differences in levels of continuous variables were examined using the one way ANOVA for parametric data. Multinomial logistic regression was employed to identify the impact of a family history of diabetes on the risk factors of diabetes in the participants. Statistical significance was considered at p value < 0.05.
Socio-demographic characteristics
Table 1 summarizes the socio-demographic characteristics (frequencies and percentages) of the participants and the association between the degree of family history of diabetes and socio-demographic characteristics.
The mean age was 50.1 (± 11.1) years. The majority of respondents were female (74.2%), married/co-habiting (78.6%), had a regular income household (66.3%), were daily/weekly exercisers (74.6%) and non-smokers (90.7%), with the smallest proportion living in an urban area (18.1%) and the biggest proportion having access to health services (90.1%).
Of the 182 study participants, 45.1% (82/182) reported a family history of diabetes. The prevalence of diabetes in 1st degree relatives (parent, sibling) and 2nd degree relatives (grandparent, aunt, uncle, cousin) was 24.2% (44/182) and 12.1% (22/182) respectively; 8.8% (16/182) reported a family history of diabetes in both 1st degree and 2nd degree relatives.
The degree of family history of diabetes was not significantly ( p > 0.05) associated with any socio-demographic variable.
Physical conditions and clinical characteristics in family relations
Table 2 summarizes the associations between the degree of family history of diabetes and physical conditions/clinical characteristics while Fig. 1 summarizes various physical conditions in descending prevalence.
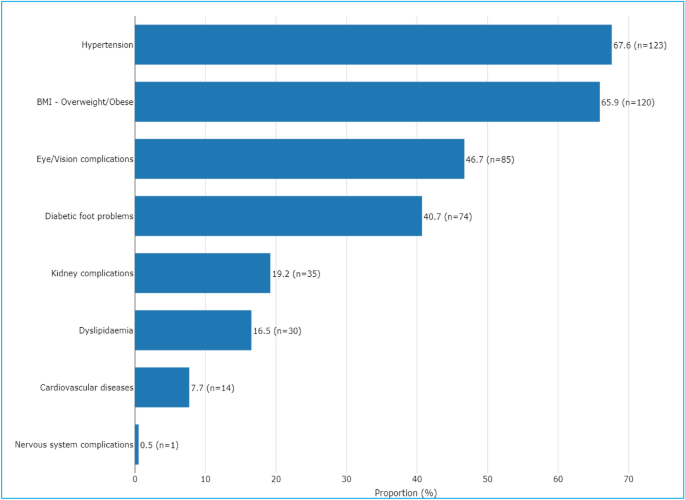
Prevalence of the various physical conditions in descending order (N = 182).
The physical conditions significantly ( p < 0.05) associated with the degree of family history of diabetes were retinopathy, duration of diabetes (years), and history of hypertension. The clinical characteristics significantly ( p < 0.05) associated with the degree of family history of diabetes were HbA1C (%) and hypertension.
Mental disorders
Table 3 summarizes the association between the degree of family history of diabetes and mental disorders, mean scores of HAM-D, WHO-5 Well-being, PAID, and PHQ-9. It also summarizes the various DSM-5 diagnoses.
Only depressive symptoms (as measured by the HAM-D) were significantly ( p = 0.030) associated with the degree of family history of diabetes. PHQ-9 unlike HAM-D did not reveal any significant trends ( p > 0.05). All other measures were not significantly associated with a family history of diabetes ( p > 0.05).
Independent predictors of T2DM in family relations
Table 4 summarizes the predictors of T2DM in different degrees of family relations.
Participants who had diabetes in both 1st and 2nd degree relatives had 6.28 increased odds of having retinopathy compared with participants who did not have a family history of diabetes. Diabetes in both 1st and 2nd degree relatives was associated with a higher duration of diabetes (years) and higher HbA1C (%).
Diabetes in 1st degree relatives was associated with higher HAM-D total scores.
PHQ-9 depression symptoms prevalence.
Figure 2 depicts the prevalence of various depression symptoms measured by PHQ-9.
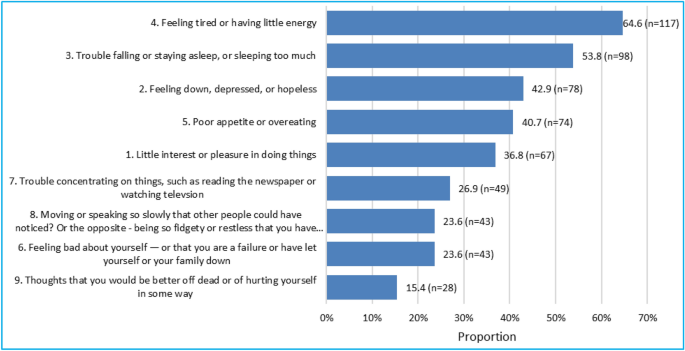
Prevalence of PHQ-9 aspects in descending order (N = 182).
Most respondents had experienced profound fatigue or low energy levels, with over half indicating trouble with sleep patterns. Notably, a significant portion, comprising 15.40% of respondents, reported thoughts of being better off dead or of hurting themselves in some way.
Diabetes type 2 regardless of family relation
Table 5 summarizes the prevalence of the various aspects of mental health disorders as measured by the various instruments used in all the 182 patients attending the clinic, regardless of family relations. The prevalence of these various conditions is summarized in Fig. 3 in descending order. HAM-D was by far the most common mental health disorder while eating disorders (bulimia and anorexia) were the least with suicidality occupying the third position in the descending order, while elevated PAID was among the least.
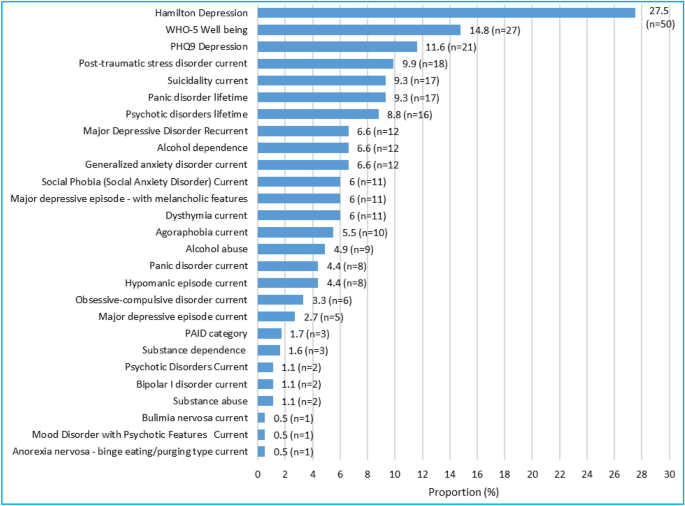
Prevalence of HAM-D, poor WHO wellbeing, PHQ-9, PAID and DSM-5 mental disorders in descending order (N = 182).
Table 6 summarizes the independent predictors of depression in Diabetes. These predictors are diabetic foot problems, poor WHO-5 Wellbeing, and suicidality.
This report serves two main purposes: to provide context-appropriate evidence for Kenya to support the holistic and liaison approach to the management of T2DM and secondly to contribute to the global data pool by offering recommendations that can be replicated in similar contexts.
To our knowledge, this is the first Kenyan cohort study that reports different genetic loading (family history in different degrees of relations) and the significant independent predictors of T2DM and the associations between T2DM and socio-demographic characteristics, physical conditions, and mental disorders. As far as we were able to establish, this is not just a first for Kenya but also in Africa.
Family history
The finding of 45.1% of family history is lower than the reported 60–68.9% in the literature. This discrepancy could be attributed to the selection of the research participants in various studies. Ours was an outpatient clinic that excluded those admitted and presumably with severe forms of T2DM and possibly higher genetic loading. However, the finding of 45% is still significant for the Kenyan context, given that it is a non-modifiable contributor, hence the need for concerted efforts to focus on modifiable factors that are feasible in the Kenyan situation with limited resources, besides genetic counseling.
Social-demographics
There were no significant differences between a family history of diabetes and all the socio-demographic variables studied, nor was any socio-demographic variable a predictor of T2DM. It is noteworthy that smoking status was not associated with any type of T2DM family history. This could be a reflection of no history of smoking in the cohort studied, a practice that should be encouraged and no doubt the policy in Kenya to put social pressure against smoking and also counseling at the clinic. Another unexpected finding though not reaching a significant level was that of only 25% males of the total clinic patients. This could be explained as a gender preference to attend this public facility or a reflection of the differential gender prevalence of diabetes in the communities served by this public facility. A further possible explanation is a trend though not significant that the overwhelming majority (84–87%) of females, as opposed to 12.5% -22.7% of males, had a family history of T2DM. Mixed methods studies are required to explain these findings.
Physical conditions and biomarkers
Our study has shown that the higher the genetic loading the higher the association of retinopathy with T2DM in 1st and 2nd degree relatives compared with other levels of family history. Additionally, the highest association with diabetes in both 1st and 2nd degree relatives was found for the duration of diabetes in years, hypertension, and two specific biomarkers—HbAIC (%) and blood pressure (BP). BP and by extension hypertension can be easily monitored in the community, with the support of a relative, using easily available and affordable but reliable and valid BP monitors at home or the nearest health facility. This is an efficient way of monitoring and preventing T2DM, especially in those with a high genetic loading of diabetes. There is a new policy for every Kenyan family and all the individuals in that family to be reached at their homes on a regular basis by the newly created cadre of Primary Health promoters. They will not only attend to health promotion through awareness and attend to minor ailments but also take blood pressure. This community approach to monitoring blood pressure if successful is likely to have a critical impact on diabetes. Routine screening for blood pressure achieves extra significance given that 16.5% of our study patients were aged 60 + on age group. It is at this age group where various dementing conditions increase and hypertension is a risk factor for dementia 48 , 49 . The same principle applies to a routine determination of HbAIC (%) in those with the highest family loading of genetic risk for diabetes. In the Kenyan situation, blood samples for these can be taken at the nearest facility, and analysis carried out in that or the nearest available facility. Routine liaison consultation with the easily available ophthalmic clinical officers, (with the option to refer) for ophthalmoscopy is required for all patients with T2DM and more mandatory for patients with the highest genetic family history of T2DM in all diabetes clinics everywhere. Good history taking on the duration of diabetes is a routine practice that is reemphasized.
Even where there is no significant association with a family history of T2DM, our findings suggest there is a need for liaison practice, especially with renal and cardiology expertise. This expertise is usually but not always, available at all the 47 County Referral and Teaching hospitals in Kenya including the hospital where this study took place. While all physical conditions associated with T2DM were found in this cohort, only diabetic foot problems predicted depression. The holistic approach in that clinic could have mitigated other physical conditions as predictors of depression.
Although there was co-morbidity of diabetes with various mental disorders including alcohol abuse and dependence, WHO-5 wellbeing and diabetic stress, only depression, as determined by HAM-D was significant but less common in those with the highest level of genetic loading i.e., in both 1st and 2nd degree relatives.
Unlike HAM-D, PHQ-9 did not show any significant trends, suggesting the HAM_D scale is probably more sensitive and also the possibility that it is more valid than PHQ-9 in the type of patients we studied. While we do not have a conclusive explanation for this finding, we note that our sample size was small so no strong inferences could be made. Nevertheless, we venture a plausible explanation.
Firstly, if there are other family members with diabetes you are less likely to be depressed or anxious because there is support around you to help with your diabetes, therefore, less diabetes distress and more knowledge and understanding of diabetes.
However, we do not know whether individuals were living alone, an unlikely possibility in the Kenyan social-cultural context, if not they could still have family contacts through the still operational extended family and family social support systems in Kenya, though, this is diminishing towards nuclear centered families. It is also possible—that if there was a more laissez-faire attitude towards diabetes in relatives, then that might also lead to lower levels of anxiety and stress. On the other side, this attitude could at the same time lead to poorer glycemic control and so increased risk for microvascular disease. Either way, there are important implications for practice—screening for diabetes as well as depression, and improved knowledge of the risks of diabetes. The depression could be secondary to the onset of T2DM and most likely related to the burden of care in patients with T2DM.
The prevalence of various mental disorders found in this study was less than has been reported previously in the wider non-diabetic general clinical population in Kenya during a past study 50 .
Although there were no significant associations of all other types of mental disorders with a family history of T2DM, the high co-morbidity, ranging up to 13.6% and with a particular note of suicidality, calls for liaison with mental health experts in the management of T2DM. Apart from the findings on family relations, there are other incidental but clinically important findings. Of note is that although the association with psychotic conditions did not achieve significance, these psychotic conditions could negatively affect the overall management of T2DM. It is likely that the patients with these symptoms were treatment naive or not yet diagnosed and had therefore not received appropriate treatment for their psychosis. We therefore recommend routine screening for mental disorders using easily self-administered tests for all patients attending diabetes clinics. This self-screen is recommended because diabetologists are not necessarily experts in mental health and may not have the time to take a full history or make a diagnosis using a clinician-administered tool. Secondly, more importantly, the patients themselves may not be aware of, or may not feel able to report their mental health problems. Thirdly, joint management of diabetes and any mental disorder may have a better outcome for both conditions. This is feasible at local health center facilities, which are widely accessible at the community level, using stepwise upward referrals to the higher levels where there is the necessary expertise. Recommendations for treatment can then be provided using a stepwise downward referral process so that the patients can be managed in their communities. This will enhance the availability and accessibility of services and benefit capacity building in skills at the grassroots level.
The low-level prevalence of emotional stress (2.7%) does not allow us to test significant associations. While being diagnosed with diabetes can cause anxiety and depression and lead to emotional distress, the cause-effect could also be bi-directional—i.e. diagnosis leading to emotional distress or conversely emotional distress from other unidentified factors such as physical conditions leading to anxiety and distress. This calls for a qualitative approach that explores at a clinical level any directional relationship in a particular patient.
This finding of 2.7% prevalence of diabetes-related emotional distress is one of the lowest as compared to 12.8–46% reported in the literature 51 , 52 , 53 . We speculate that this is a reflection of the type of engagement of the patients that goes beyond the prescription of drugs in that particular clinic. It is the integrated management of diabetes that we speculate reduces emotional distress within a setting where the patients are fully educated on their conditions and management. It is likely that the levels of emotional distress would be similar to those reported in the literature for other situations and clinics that do not incorporate such holistic practices. If indeed that is the case, then it is a reflection of good practice in that specific clinic which could be replicated elsewhere.
Only combined methods—quantitative and qualitative have the potential to delineate these associations. Overall, our findings suggest the need for screening for depression, WHO-5 wellbeing, and suicidality in routine clinical management of T2DM at least in all patients with T2DM. Any positive screening findings should be integrated into the management of the patient.
Conclusions
Family relationships are important in both physical disorders and depression, suggesting shared genetic predisposition, and/or modulation by shared environmental factors. Depression emerges as the common mental disorder in individuals with Type 2 Diabetes, irrespective of relational factors. Additionally, all examined patients exhibited various mental health concerns and DSM-5 disorders. This Kenyan study contributes to the global database on the topic of Types of diabetes and family relations and associated mental and physical conditions. We have achieved all our aims for this study.
Based on all the achieved general and specific aims, we have suggested some clinical and community health practices and policies.
Limitations and recommendations to overcome the limitation
This study was carried out in a cohort of patients attending a diabetes clinic and therefore does not reflect the wider population of people with T2DM. This study excluded those untreated patients in the community or where clinics do not provide psychoeducation as in this clinic. Conversely, this holistic approach could be replicated in other clinics and contexts.
Secondly, we could not establish any directional relationships using the quantitative methods, given that our data is cross-sectional. Only mixed qualitative and quantitative methods could address this.
Although we achieved our aims, we recommend more studies at the community level to include those who may have T2DM and go for other services or are not treated by a specialist. Such a study though necessary for better understanding would be expensive and would require more complicated logistics.
Data availability
The datasets used and/or analyzed during the current study are available from the corresponding author upon reasonable request.
Wang, C. et al. Association between parental history of diabetes and the incidence of type 2 diabetes mellitus differs according to the sex of the parent and offspring’s body weight: A finding from a Japanese worksite-based cohort study. Prev. Med. (Baltim.) 81 , 49–53 (2015).
Article Google Scholar
Gopalakrishnan, S. & Geetha, A. R. U. Study on the impact of family history of diabetes among type 2 diabetes mellitus patients in an urban area of Kancheepuram district, Tamil Nadu. Int. J. Community Med. Public Health 4 , 4151–4156 (2017).
Liu, Q., Yuan, J., Bakeyi, M., Li, J., Zhang, Z., Yang, X., et al. Development and validation of a nomogram to predict type 2 diabetes mellitus in overweight and obese adults: A prospective cohort study from 82938 adults in China. Int. J. Endocrinol. 2020 (2020).
Nagarathna, R., Bali, P., Anand, A., Srivastava, V., Patil, S., Sharma, G., et al. Prevalence of diabetes and its determinants in the young adults Indian population-call for yoga intervention. Front. Endocrinol. (Lausanne) . 846 (2020).
Papazafiropoulou, K. A., Papanas, N., Melidonis, A. & Maltezos, E. Family history of type 2 diabetes: Does having a diabetic parent increase the risk?. Curr. Diabet. Rev. 13 (1), 19–25 (2017).
Article CAS Google Scholar
Sheu, W. H. et al. Family aggregation and maternal inheritance of Chinese type 2 diabetes mellitus in Taiwan. Zhonghua yi xue za zh= Chin. Med. J. Free China ed. 62 (3), 146–151 (1999).
CAS Google Scholar
Hemminki, K., Li, X., Sundquist, K. & Sundquist, J. Familial risks for type 2 diabetes in Sweden. Diabet. Care 33 (2), 293–297. https://doi.org/10.2337/dc09-0947 (2009).
Alharithy, M. K., Alobaylan, M. M., Alsugair, Z. O. & Alswat, K. A. Impact of family history of diabetes on diabetes control and complications. Endocr. Pract. 24 (9), 773–779 (2018).
Article PubMed Google Scholar
Maghbooli, Z., Pasalar, P., Keshtkar, A., Farzadfar, F. & Larijani, B. Predictive factors of diabetic complications: A possible link between family history of diabetes and diabetic retinopathy. J. Diabet. Metab. Disord. 13 (1), 1–5 (2014).
Silverman-Retana, O. et al. Effect of familial diabetes status and age at diagnosis on type 2 diabetes risk: A nation-wide register-based study from Denmark. Diabetologia. 63 (5), 934–943 (2020).
Thorn, L. M. et al. Effect of parental type 2 diabetes on offspring with type 1 diabetes. Diabetes Care. 32 (1), 63–68 (2009).
Article PubMed PubMed Central Google Scholar
Tan, J. T., Tan, L. S. M., Chia, K. S., Chew, S. K. & Tai, E. S. A family history of type 2 diabetes is associated with glucose intolerance and obesity-related traits with evidence of excess maternal transmission for obesity-related traits in a South East Asian population. Diabet. Res. Clin. Pract. 82 (2), 268–275 (2008).
Parkkola, A., Turtinen, M., Härkönen, T., Ilonen, J. & Knip, M. Family history of type 2 diabetes and characteristics of children with newly diagnosed type 1 diabetes. Diabetologia. 64 (3), 581–590 (2021).
Article CAS PubMed Google Scholar
Koebnick, C. et al. Combined association of maternal and paternal family history of diabetes with plasma leptin and adiponectin in overweight Hispanic children. Diabet. Med. 25 (9), 1043–1048 (2008).
Article CAS PubMed PubMed Central Google Scholar
Papazafiropoulou, A. et al. Familial history of diabetes and clinical characteristics in Greek subjects with type 2 diabetes. BMC Endocr. Disord. 9 (1), 1–7 (2009).
Shirey, K. et al. Symptoms of depression among patients attending a diabetes care clinic in rural western Kenya. J. Clin. Transl. Endocrinol. 2 (2), 51–54 (2015).
PubMed PubMed Central Google Scholar
Atlas, D. International Diabetes Federation. IDF Diabetes Atlas 7th edn. (Belgium International Diabetes Federation, 2015).
Google Scholar
Amankwah-Poku, M., Amoah, A. G. B., Sefa-Dedeh, A. & Akpalu, J. Psychosocial distress, clinical variables and self-management activities associated with type 2 diabetes: A study in Ghana. Clin. Diabet. Endocrinol. 6 (1), 1–10 (2020).
Kêkê, L. M. et al. Body mass index and childhood obesity classification systems: A comparison of the French, International Obesity Task Force (IOTF) and World Health Organization (WHO) references. Rev. Epidemiol. Sante Publique. 63 (3), 173–182 (2015).
Gahlan, D., Rajput, R., Gehlawat, P. & Gupta, R. Prevalence and determinants of diabetes distress in patients of diabetes mellitus in a tertiary care centre. Diabet. Metab. Syndr. Clin. Res. Rev. 12 (3), 333–336 (2018).
Rehman, S. U., Shakaib, A., Rashid, S., Gary, T. L. & Brancati, F. L. Regarding depressive symptoms and metabolic control in African–Americans with type 2 diabetes/Response to Rehman et al.. Diabet. Care 23 (10), 1596 (2000).
Van Welie, H. et al. The prevalence of diabetes mellitus is increased in relatives of patients with a non-affective psychotic disorder. Schizophr. Res. 143 (2–3), 354–357 (2013).
Lamberti, J., Crilly, J., Maharaj, K., Olson, D. & Costea, O. Prevalence of adult-onset diabetes among outpatients receiving antipsychotic drugs. Schizophr. Res. 1 (60), 360 (2003).
Su, M.-H. et al. Familial aggregation and shared genetic loading for major psychiatric disorders and type 2 diabetes. Diabetologia. 65 , 800–810 (2022).
Huang, M.-H. et al. Increased risk of type 2 diabetes among the siblings of patients with schizophrenia. CNS Spectr. 24 (4), 453–459 (2019).
Wong, H., Singh, J., Go, R. M., Ahluwalia, N. & Guerrero-Go, M. A. The effects of mental stress on non-insulin-dependent diabetes: determining the relationship between catecholamine and adrenergic signals from stress, anxiety, and depression on the physiological changes in the pancreatic hormone secretion. Cureus. 11 (8), e5474 (2019).
Van der Feltz-Cornelis, C. M. et al. Effect of interventions for major depressive disorder and significant depressive symptoms in patients with diabetes mellitus: A systematic review and meta-analysis. Gen. Hosp. Psychiatry 32 (4), 380–395 (2010).
Chaturvedi, S. K. et al. More anxious than depressed: prevalence and correlates in a 15-nation study of anxiety disorders in people with type 2 diabetes mellitus. Gen. Psychiatry. 32 (4), e100076 (2019).
Winston, A. P. Eating disorders and diabetes. Curr. Diabet. Rep. 20 (8), 1–6 (2020).
Harris, S. R., Carrillo, M. & Fujioka, K. Binge-eating disorder and type 2 diabetes: A review. Endocr. Pract. 27 (2), 158–164 (2021).
Alessi, J. et al. Mental health in the era of COVID-19: Prevalence of psychiatric disorders in a cohort of patients with type 1 and type 2 diabetes during the social distancing. Diabetol. Metab. Syndr. 12 (1), 1–10 (2020).
Asiimwe, D., Mauti, G. O. & Kiconco, R. Prevalence and risk factors associated with type 2 diabetes in elderly patients aged 45–80 years at Kanungu District. J. Diabet. Res. 6 , 1–9 (2020).
Endris, T., Worede, A. & Asmelash, D. Prevalence of diabetes mellitus, prediabetes and its associated factors in Dessie Town, Northeast Ethiopia: A community-based study. Diabet. Metab. Syndr. Obes. Targets Ther. 12 , 2799 (2019).
Omar, S. M., Musa, I. R., ElSouli, A. & Adam, I. Prevalence, risk factors, and glycaemic control of type 2 diabetes mellitus in eastern Sudan: A community-based study. Ther. Adv. Endocrinol. Metab. 10 , 2042018819860071 (2019).
Erasmus, R. T. et al. Importance of family history in type 2 black South African diabetic patients. Postgrad. Med. J. 77 (907), 323–325 (2001).
Chetty, R. R. & Pillay, S. Glycaemic control and family history of diabetes mellitus: Is it all in the genes?. J. Endocrinol. Metab. Diabet. S. Afr. 26 (2), 66–71 (2021).
El-busaidy, H., Dawood, M., Kasay, A., Mwamlole, C., Koraya, N. & Parpia, H. How serious is the impact of type II diabetes in rural Kenya? (2014).
Kiraka, G. N., Kunyiha, N., Erasmus, R. & Ojwang, P. J. Family history as a risk for early-onset type 2 diabetes in Kenyan patients. Afr. J. Diabet. Med. 22 (2), 15–17 (2014).
Lloyd, C. E. et al. The INTERPRET–DD study of diabetes and depression: A protocol. Diabet. Med. 32 (7), 925–934 (2015).
Kroenke, K., Spitzer, R. L. & Williams, J. B. W. The PHQ-9: Validity of a brief depression severity measure. J. Gen. Intern. Med. 16 (9), 606–613 (2001).
Petrak, F., Baumeister, H., Skinner, T. C., Brown, A. & Holt, R. I. G. Depression and diabetes: Treatment and health-care delivery. Lancet Diabet. Endocrinol. 3 (6), 472–485 (2015).
Hamilton, M. A rating scale for depression. J. Neurol. Neurosurg. Psychiatry 23 (1), 56 (1960).
Vindbjerg, E., Makransky, G., Mortensen, E. L. & Carlsson, J. Cross-cultural psychometric properties of the Hamilton Depression Rating Scale. Can. J. Psychiatry 64 (1), 39–46 (2019).
Rohan, K. J. et al. A protocol for the Hamilton Rating Scale for Depression: Item scoring rules, Rater training, and outcome accuracy with data on its application in a clinical trial. J. Affect. Disord. 200 , 111–118 (2016).
Newnham, E. A., Hooke, G. R. & Page, A. C. Monitoring treatment response and outcomes using the World Health Organization’s Wellbeing Index in psychiatric care. J. Affect. Disord. 122 (1–2), 133–138 (2010).
Polonsky, W. H. et al. Assessment of diabetes-related distress. Diabet. Care. 18 (6), 754–760 (1995).
Sheehan, D. V. et al. The Mini-International Neuropsychiatric Interview (MINI): The development and validation of a structured diagnostic psychiatric interview for DSM-IV and ICD-10. J. Clin. Psychiatry 59 (20), 22–33 (1998).
PubMed Google Scholar
Musyimi, C. W., Muyela, L. A., Mutiso, V. N., Mutunga, E. & Ndetei, D. M. Understanding dementia care pathways for policy development and service planning in Kenya. Dementia. 22 , 1027–1037 (2023).
Comas-Herrera, A., Lorenz-Dant, K., Ferri, C., Govia, I., Sani, T., Jacobs, R., et al. Supporting people living with dementia and their carers in low-and middle-income countries during COVID-19. LTCcovid Org. 4 (2020).
Ndetei, D. M. et al. The prevalence of mental disorders in adults in different level general medical facilities in Kenya: A cross-sectional study. Ann. Gen. Psychiatry 8 (1), 1–8 (2009).
Lloyd, C. E. et al. Prevalence and correlates of depressive disorders in people with Type 2 diabetes: Results from the International Prevalence and Treatment of Diabetes and Depression (INTERPRET-DD) study, a collaborative study carried out in 14 countries. Diabet. Med. 35 (6), 760–769 (2018).
Perrin, N. E., Davies, M. J., Robertson, N., Snoek, F. J. & Khunti, K. The prevalence of diabetes-specific emotional distress in people with Type 2 diabetes: A systematic review and meta-analysis. Diabet. Med. 34 (11), 1508–1520 (2017).
Ramkisson, S., Pillay, B. J. & Sartorius, B. Diabetes distress and related factors in South African adults with type 2 diabetes. J. Endocrinol. Metab. Diabet. S. Afr. 21 (2), 35–39 (2016).
Download references
Acknowledgements
All the study participants and the staff of Machakos County Referral and Teaching Hospital Diabetes Clinic, the Association for the Improvement of Mental Health Programs, Switzerland, and the Africa Mental Health Research and Training Foundation.
Author information
Authors and affiliations.
Department of Psychiatry, University of Nairobi, Nairobi, Kenya
David M. Ndetei
Africa Mental Health Research and Training Foundation, Mawensi Road, Off Elgon Road, Mawensi Garden, P.O. Box 48423-00100, Nairobi, Kenya
David M. Ndetei, Victoria Mutiso, Christine Musyimi & Pascalyne Nyamai
World Psychiatric Association Collaborating Centre for Research and Training, Nairobi, Kenya
The Open University, Milton Keynes, UK
Cathy Lloyd
Association for the Improvement of Mental Health Programmes (AMH), Geneva, Switzerland
Norman Sartorius
You can also search for this author in PubMed Google Scholar
Contributions
D.M.N.—conceptualization, literature review, drafting; V.M.—study implementation; C.M.—ethical issues, data quality control; P.N.—literature review and drafting; C.L.—conceptualization and assisted with data analysis; N.S.—conceptualization. All authors read and approved the final manuscript.
Corresponding author
Correspondence to David M. Ndetei .
Ethics declarations
Competing interests.
The authors declare no competing interests.
Additional information
Publisher's note.
Springer Nature remains neutral with regard to jurisdictional claims in published maps and institutional affiliations.
Rights and permissions
Open Access This article is licensed under a Creative Commons Attribution 4.0 International License, which permits use, sharing, adaptation, distribution and reproduction in any medium or format, as long as you give appropriate credit to the original author(s) and the source, provide a link to the Creative Commons licence, and indicate if changes were made. The images or other third party material in this article are included in the article's Creative Commons licence, unless indicated otherwise in a credit line to the material. If material is not included in the article's Creative Commons licence and your intended use is not permitted by statutory regulation or exceeds the permitted use, you will need to obtain permission directly from the copyright holder. To view a copy of this licence, visit http://creativecommons.org/licenses/by/4.0/ .
Reprints and permissions
About this article
Cite this article.
Ndetei, D.M., Mutiso, V., Musyimi, C. et al. Association of type 2 diabetes with family history of diabetes, diabetes biomarkers, mental and physical disorders in a Kenyan setting. Sci Rep 14 , 11037 (2024). https://doi.org/10.1038/s41598-024-61984-6
Download citation
Received : 12 January 2024
Accepted : 13 May 2024
Published : 14 May 2024
DOI : https://doi.org/10.1038/s41598-024-61984-6
Share this article
Anyone you share the following link with will be able to read this content:
Sorry, a shareable link is not currently available for this article.
Provided by the Springer Nature SharedIt content-sharing initiative
- Family relations
- Type 2 diabetes mellitus
- Physical conditions
- Kenyan setting
By submitting a comment you agree to abide by our Terms and Community Guidelines . If you find something abusive or that does not comply with our terms or guidelines please flag it as inappropriate.
Quick links
- Explore articles by subject
- Guide to authors
- Editorial policies
Sign up for the Nature Briefing newsletter — what matters in science, free to your inbox daily.

- Research article
- Open access
- Published: 20 May 2024
ABO and Rhesus blood groups and multiple health outcomes: an umbrella review of systematic reviews with meta-analyses of observational studies
- Fang-Hua Liu 1 , 2 na1 ,
- Jia-Kai Guo 1 , 3 na1 ,
- Wei-Yi Xing 1 , 2 na1 ,
- Xue-Li Bai 1 , 4 na1 ,
- Yu-Jiao Chang 1 , 2 na1 ,
- Zhao Lu 1 , 5 ,
- Miao Yang 1 , 6 ,
- Ying Yang 1 , 7 ,
- Wen-Jing Li 1 , 8 ,
- Xian-Xian Jia 1 , 6 ,
- Tao Zhang 1 , 5 ,
- Jing Yang 1 , 9 ,
- Jun-Tong Chen 10 ,
- Song Gao 4 ,
- Lang Wu 11 ,
- De-Yu Zhang 4 ,
- Chuan Liu 4 ,
- Ting-Ting Gong 4 &
- Qi-Jun Wu ORCID: orcid.org/0000-0001-9421-5114 1 , 2 , 4 , 12
BMC Medicine volume 22 , Article number: 206 ( 2024 ) Cite this article
Metrics details
Numerous studies have been conducted to investigate the relationship between ABO and Rhesus (Rh) blood groups and various health outcomes. However, a comprehensive evaluation of the robustness of these associations is still lacking.
We searched PubMed, Web of Science, Embase, Scopus, Cochrane, and several regional databases from their inception until Feb 16, 2024, with the aim of identifying systematic reviews with meta-analyses of observational studies exploring associations between ABO and Rh blood groups and diverse health outcomes. For each association, we calculated the summary effect sizes, corresponding 95% confidence intervals, 95% prediction interval, heterogeneity, small-study effect, and evaluation of excess significance bias. The evidence was evaluated on a grading scale that ranged from convincing (Class I) to weak (Class IV). We assessed the certainty of evidence according to the Grading of Recommendations Assessment, Development, and Evaluation criteria (GRADE). We also evaluated the methodological quality of included studies using the A Measurement Tool to Assess Systematic Reviews (AMSTAR). AMSTAR contains 11 items, which were scored as high (8–11), moderate (4–7), and low (0–3) quality. We have gotten the registration for protocol on the PROSPERO database (CRD42023409547).
The current umbrella review included 51 systematic reviews with meta-analysis articles with 270 associations. We re-calculated each association and found only one convincing evidence (Class I) for an association between blood group B and type 2 diabetes mellitus risk compared with the non-B blood group. It had a summary odds ratio of 1.28 (95% confidence interval: 1.17, 1.40), was supported by 6870 cases with small heterogeneity ( I 2 = 13%) and 95% prediction intervals excluding the null value, and without hints of small-study effects ( P for Egger’s test > 0.10, but the largest study effect was not more conservative than the summary effect size) or excess of significance ( P < 0.10, but the value of observed less than expected). And the article was demonstrated with high methodological quality using AMSTAR (score = 9). According to AMSTAR, 18, 32, and 11 studies were categorized as high, moderate, and low quality, respectively. Nine statistically significant associations reached moderate quality based on GRADE.
Conclusions
Our findings suggest a potential relationship between ABO and Rh blood groups and adverse health outcomes. Particularly the association between blood group B and type 2 diabetes mellitus risk.
Peer Review reports
Blood groups can be categorized based on different systems, such as the ABO blood group system, the Rhesus (Rh) blood group system, and the MN blood group system [ 1 ]. ABO blood group system is the most frequently applied [ 2 ]. Each of the two alleles possesses antigen A, B, or neither. These alleles come together to be a combination, determining an individual’s blood type phenotype, thus perform as the type of O, A, B, or AB. The Rh blood group system is more polymorphic than others among human blood groups, which is composed of numerous antigens and next to ABO. The ABO and Rh blood group system are extensively utilized in clinical practice, affecting host susceptibility [ 3 , 4 ].
The previous study suggested that blood groups are involved in disease mechanisms at the molecular level mediated either through the blood group antigens or by the blood group reactive antibodies [ 5 ]. In addition, J. Höglund et al. found 39 plasma proteins were associated with variation at the ABO locus. For example, proteins with functions related to tumorigenesis (CA9, Gal-9, and KLK6) and pro-inflammatory or anti-inflammatory functions (IFN-gamma-R1, IL-18BP, and MARCO) [ 6 ]. Generally, the overexpression of these proteins leads to an abnormal cell proliferation or cell growth. Thus, blood group may influence disease development through protein expression levels.
Numerous systematic reviews with meta-analyses have been published, which explored correlations between ABO and Rh blood groups with various health outcomes [ 7 , 8 , 9 ]. However, to date, the association between these blood groups and human health outcomes remains controversial [ 10 , 11 , 12 ]. Most of them have primarily concentrated on one single disease end-point, lacking a comprehensive evaluation of the aforementioned relationships. In addition, the strength and reliability of the evidence remains unclear. To overcome the inherent limitations of systematic reviews with meta-analyses and provide a comprehensive overview of the claimed associations of ABO and Rh blood groups with health outcomes, in the form of an umbrella review (UR), is necessary.
UR synthesizes evidence from various systematic reviews with meta-analyses on a subject, appraising the certainty, precision, and potential bias of the correlations, thus facilitating evidence grading based on well-defined criteria [ 13 ]. We set out to conduct an UR to comprehensively evaluate systematic reviews with meta-analyses of observational studies, which examined associations of ABO and Rh blood groups with a range of health outcomes. This endeavor was aimed at presenting an overview of the breadth and validity for aforementioned associations. We thus hoped to provide both clinicians and policy makers with robust data to identify high-risk groups and inform clinical practice and guidelines.
Protocol registration
We have gotten the registration for the protocol of this UR with the International Prospective Register of Systematic Reviews (PROSPERO; registration number CRD42023409547). The study followed the Preferred Reporting Items for Systematic Reviews and Meta-analyses reporting guideline [ 14 ] (Additional file 1 : Table S1) and the Meta-analysis of Observational Studies in Epidemiology reporting guideline [ 15 ] (Additional file 1 : Table S2).
Search strategy
We systematically searched PubMed, Web of Science, Embase, Scopus, Cochrane Library, and several regional databases (Latin American and Caribbean Health Sciences Literature, Western Pacific Region Index Medicus, Index Medicus for South-East Asia Region, Index Medicus for the Eastern Mediterranean Region, and African Index Medicus) on the date from inception until Feb 16, 2024, to identify systematic reviews with meta-analyses of observational studies evaluating associations between ABO as well as Rh blood groups and diverse health outcomes. We used the keywords (“ABO” OR “blood group” OR “blood type” OR “Rh”) AND (“meta-analysis” OR “systematic review” OR “systematic overview”) (Additional file 1 : Table S3) to search. Besides, the literature search was reviewed by hand-checking the reference lists of all systematic reviews with meta-analyses.
Eligibility criteria
Articles were selected based on the following PECOS (Population, Exposure, Comparison, Outcome, Study design) strategy:
Population: population with ABO or Rh blood groups;
Exposure: ABO (blood types A, B, O, and AB) and Rh (Rh positive [Rh +] and Rh negative [Rh −]) blood groups (any method used to assess blood type, including genetic tests and forward/reverse agglutination tests, was accepted);
Comparison: different blood groups;
Outcome: any health outcome (e.g., cancer, coronavirus disease 2019 [COVID-19], coronary artery disease, etc.). Ascertained health outcomes using self-report, observed (e.g., clinical diagnoses) or objective [e.g., biomarkers, certified mortality] criteria); and
Study design: systematic reviews with meta-analyses of observational studies (cohort, case–control, or cross-sectional studies).
The exclusion criteria were established as follows: (1) systematic reviews without quantitative analysis, (2) systematic reviews with meta-analyses without study-level data (e.g., effect sizes, 95% confidence intervals [CIs], the number of cases, and participants/control), (3) studies on genetic polymorphisms, animal studies, laboratory studies, conference abstracts and randomized controlled trials, or (4) systematic reviews with meta-analyses conducted in languages other than English.
Given the requirement for a minimum of three original studies to calculate 95% prediction intervals (PIs), we incorporated meta-analyses comprising at least 3 original studies [ 16 ]. Associations were considered to overlap if they assessed the same research topic and were examined in more than one systematic review with meta-analysis [ 17 ]. The inclusion of primary studies once or more may be led by incorporating results of reviews with overlapping associations, and biased findings and estimates could be caused by incorporating results as well [ 18 , 19 ]. Therefore, the systematic review with meta-analysis which contained the largest number of primary studies was picked up if two or more systematic reviews with meta-analyses overlapped, while the one with the largest sample size of participants if more than one systematic review with meta-analysis kept the same numbered primary studies.
To ascertain the eligible articles, four experienced investigators (Y-JC, J-KG, J-TC, and YY) matched in pairs and screened titles, abstracts, and full texts independently. We also checked the references of relevant studies to confirm any other eligible articles by hand. If there were any discrepancies, they would be made out by a third reviewer (Q-JW).
Data extraction
Ten trained investigators (Y-JC, J-KG, YY, X-XJ, W-JL, T-Z, YY, MY, ZL, and X-LB) were paired to extract data independently, discrepancies were settled by a third reviewer (Q-JW) when it was needed. From every meta-analysis we identified, it was abstracted of the contents on the name of the first author, journal, publication year, exposures of interest, outcomes of interest, comparison, meta-analysis metrics (RR [risk ratio], OR [odds ratio], or HR [hazard ratio]), and the number of studies considered. From the individual studies included in every meta-analysis, it was extracted of the name of the first author, publication year, epidemiological study design, number of cases and controls in the observational case–control studies or total population in the observational cohort studies, maximally adjusted risk estimates, and 95% CIs.
Data analysis
Estimation of summary effect —We utilized a random-effects model for each meta-analysis to do a calculation for the summary effect size and corresponding 95% CI [ 20 ].
Estimation of prediction interval —We got the 95% prediction intervals (PIs) for the summary random effect sizes, because it can explain heterogeneity between varied studies and the uncertainty for the effect, with an expectation in another study concerning on the same relationship [ 21 ].
Assessment of heterogeneity —We evaluated heterogeneity with the I 2 metric. And I 2 value exceeding 50% is judged large heterogeneity, and 75% is judged very large heterogeneity similarly [ 22 ]. We also produced τ 2 statistic to assess the heterogeneity.
Assessment of small study effects —Through Egger’s regression asymmetry test [ 23 ], we evaluated small-study effects (i.e., whether larger studies are more likely to give indirectly smaller estimates of effect size when compared with smaller ones) [ 24 ]. Reasons for distinctions between small and large studies such as publication and other reporting biases, genuine heterogeneity, chance, or other conditions are revealed through small study effects [ 24 ]. They were considered to exist when the largest study effect was more conservative than the summary effect size in the meta-analysis and it was found that P value < 0.10 in the regression asymmetry test.
Evaluation of excess significance —We assessed excess significance bias by analyzing whether the number of observed studies ( O ) with nominally statistically significant results (“positive” studies, P < 0.05) was larger than the expected number of studies ( E ) with statistically significant results using the chi-square test [ 25 ]. The effect size of the largest study (that is, the smallest standard error) in a meta-analysis assessed the strength of the study which needed to use a noncentral t distribution [ 26 , 27 ]. The excess significance test was judged positive when it comes to both O > E and P < 0.10 [ 22 ].
Strength of evidence —According to the established criteria applied in previously published URs [ 13 , 28 , 29 , 30 ] and based on our calculation, significant associations ( P < 0.05) between ABO and Rh blood groups and health outcomes were divided into 4 levels of evidence strength (convincing [Class I], highly suggestive [Class II], suggestive [Class III], or weak [Class IV] evidence) to draw conclusions. This criterion was evaluated based on statistical significance, number of cases, heterogeneity, largest study, 95% PI, small-study effect, and excess significance bias. P value ≥ 0.05 demonstrated a statistically non-significant association (Additional file 1 : Table S4).
Certainty of the evidence —The credibility of the evidence was qualitatively assessed by two reviewers (W-YX and X-LB) using the GRADE (Grading of Recommendations, Assessment, Development, and Evaluations) method. As recommended by GRADE, the level of evidence was graded the high, moderate, low, and very low determined by risk of bias, inconsistency, indirectness, imprecision, and publication bias.
Sensitivity analyses —To verify the robustness of our findings, we conducted sensitivity analyses to assess the concordance of the summary associations, which were initially graded as convincing (Class I) or highly suggestive (Class II) evidence. The sensitivity analyses were realized by excluding small-sample studies (< 25th percentile) from meta-analyses with evidence of small-study effects and primary studies with low-quality evidence (Newcastle–Ottawa Scale < 6 [ 31 ], Agency for Healthcare Research and Quality < 8 [ 32 ], or effective public health practice project guideline rating moderate and low rather than strong quality [ 33 ]. Further sensitivity analysis was performed with the meta-analyses due to overlap in the main analysis. All statistical analyses were conducted in STATA version 17 and RStudio version 3.6.2.
Assessment of the methodological quality of meta-analyses
We used A Measurement Tool to Assess Systematic Reviews (AMSTAR) to evaluate the quality of systematic reviews and meta-analyses, which was considered as a valid and dependable measurement tool [ 34 ]. This instrument contains a total of 11 items. A “yes” scores one point, and the other answers score 0 points. The AMSTAR was graded as low (0–3 points), moderate (4–7 points), or high quality (8–11 points) [ 34 ]. Ten trained investigators (Y-CS, Z-PN, W-YX, YY, W-JL, ZL, JY, X-LB, MY, and J-NS) matched in pairs, and AMSTAR was used independently to assess the eligible systematic reviews with meta-analyses on methodological quality. Disagreements were made the final decision by the third author (Q-JW).
Literature identification and selection
We retrieved 6474 records from PubMed, Web of Science, Embase, Scopus, Cochrane Library, and several regional databases. According to the criterion, 159 full-text articles were retrieved and checked for inclusion after duplicate removal, title, and abstract screening. There were no additional eligible articles found by hand-checking the reference lists of all systematic reviews. Overall, 51 systematic reviews with meta-analyses corresponded to 270 unique associations were included [ 7 , 9 , 11 , 12 , 31 , 32 , 33 , 35 , 36 , 37 , 38 , 39 , 40 , 41 , 42 , 43 , 44 , 45 , 46 , 47 , 48 , 49 , 50 , 51 , 52 , 53 , 54 , 55 , 56 , 57 , 58 , 59 , 60 , 61 , 62 , 63 , 64 , 65 , 66 , 67 , 68 , 69 , 70 , 71 , 72 , 73 , 74 , 75 , 76 , 77 , 78 ] (Fig. 1 ). The two pairs of four investigators showed high consistency in terms of study selection, with kappa values of 0.893 and 0.926, respectively. The excluded articles and the reasons behind their removal are provided in Additional file 1 : Table S5. For meta-analyses excluded due to a lack of data relating to quantitative synthesis, we further summarized their findings in Additional file 1 : Table S6.
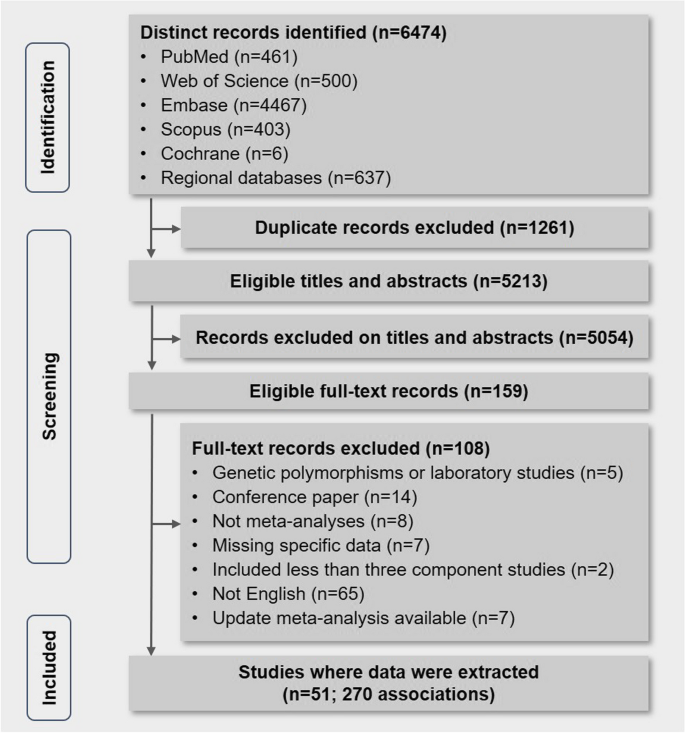
PRISMA flow chart. Flow chart of included and excluded systematic reviews and meta-analyses
Characteristics of included meta-analyses
The 51 systematic reviews with meta-analyses corresponded to 270 unique associations: 105 on cancer outcomes (39%), 91 on infectious outcomes (34%), 25 on cardiovascular outcomes (9%), 22 on oral-related outcomes (8%), 12 on pregnancy-related outcomes (4%), 5 on metabolic disease (2%), and 10 on other outcomes (4%) (Fig. 2 ). The systematic reviews with meta-analyses included in this UR were published from 2007 until 2023. The number of studies per association ranged from 3 to 49. One hundred and ninety-five meta-analyses included ≥ 1000 cases (Additional file 1 : Table S7).
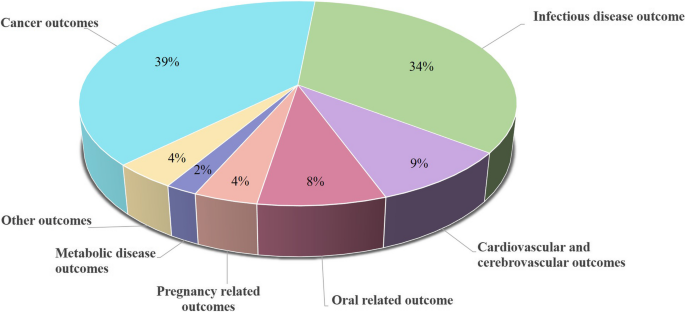
Map of 270 blood group related outcomes: percentage of outcomes per outcome category for all studies
Summary findings
Among 270 associations included in our UR. Eighty-nine associations (33%) presented a nominally statistically significant effect ( P < 0.05). Of these, 41 (46%) were in conformity with the principle of statistical significance at P < 10 −3 , and 24 (27%) reached P < 10 −6 . When calculating the effect size of the largest data study of the associations, 61 (69%) of the 89 associations showed statistical significance. After estimating the 95% PI, 66 (74%) contained null values. Twenty-three (26%) and 19 (21%) associations had significant (50% < I 2 ≤ 75%) and considerable ( I 2 > 75%) heterogeneity estimates, respectively. Twenty-one (24%) of the 89 associations presented evidence for small-study effects, and 29 (33%) associations presented evidence for excess significance bias.
Cancer outcomes
We summarized 105 associations between blood group and cancer outcomes. The magnitude of the observed summary random effects estimates ranged from 0.65 to 1.54 (Additional file 2 : Fig. S1). Thirty-one meta-analyses (30%) presented a nominally statistically significant effect ( P < 0.05). Of these, 14 associations were graded as suggestive or above evidence (Fig. 3 , Additional file 1 : Tables S7–8).
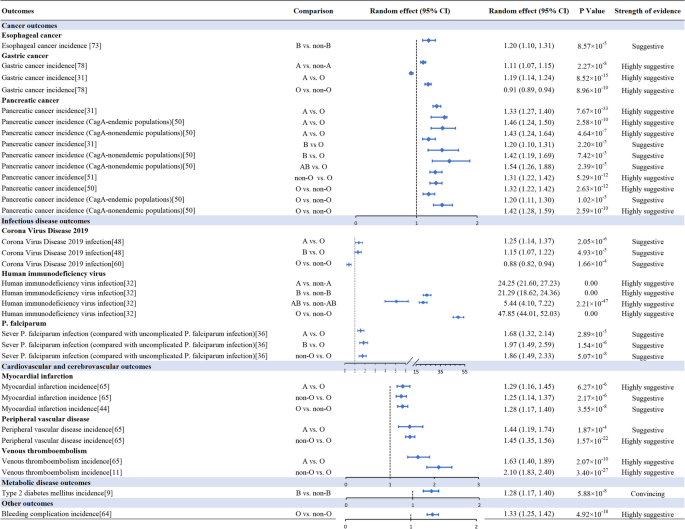
Forest plot showing studies investigating the association between blood group and health outcomes. CI, confidence interval; CagA, cytotoxin-associated gene A
Esophageal cancer
We found blood group B was associated with a higher risk of esophageal cancer (OR = 1.20; 95% CI: 1.10, 1.31), compared with blood group non-B. And the association was graded as suggestive evidence.
Gastric cancer
Blood group A was associated with a higher risk of gastric cancer, both compared with blood group non-A (OR = 1.11; 95% CI: 1.07, 1.15) and blood group O (OR = 1.19; 95% CI: 1.14, 1.24). However, blood group O was associated with a lower risk of gastric cancer (OR = 0.91; 95% CI: 0.89, 0.94), compared with blood group non-O. These above associations were graded as highly suggestive evidence.
Pancreatic cancer
Compared with blood group O, blood group A was associated with higher risk of pancreatic cancer (OR = 1.33; 95% CI: 1.27, 1.40), cytotoxin-associated gene A (CagA) endemic pancreatic cancer (OR = 1.46; 95% CI: 1.24, 1.50) and CagA-nonendemic pancreatic cancer (OR = 1.43; 95% CI: 1.24, 1.64); blood group B was associated with higher risk of pancreatic cancer (OR = 1.20; 95% CI: 1.10, 1.31) and CagA-nonendemic pancreatic cancer (OR = 1.42; 95% CI: 1.19, 1.69); blood group AB was associated with higher risk of CagA-nonendemic pancreatic cancer (OR = 1.54; 95% CI: 1.26, 1.88); Blood group non-O was also associated with higher risk of pancreatic cancer (OR = 1.31; 95% CI: 1.22, 1.42). Compared with blood group non-O, blood group O was associated with a higher risk of pancreatic cancer (OR = 1.32; 95% CI: 1.22, 1.42), CagA endemic pancreatic cancer (OR = 1.20; 95% CI: 1.11, 1.30), and CagA-nonendemic pancreatic cancer (OR = 1.42; 95% CI: 1.28, 1.59). According to the UR criteria, the associations between blood group A and pancreatic cancer, CagA endemic, and CagA-nonendemic pancreatic cancer risk, blood group O and pancreatic cancer and CagA-nonendemic pancreatic cancer, blood group non-O and pancreatic cancer were graded as highly suggestive evidence. The remaining associations were graded as suggestive evidence.
Infectious disease outcomes
Ninety-one associations between blood group and infectious disease outcomes were investigated. The magnitude of the observed summary random effects estimates ranged from 0.50 to 47.85 (Additional file 2 : Fig. S2). Overall, 27 (32%) of 85 associations reached a statistically significant value at P < 0.05. Ten associations were supported by suggestive or above evidence (Fig. 3 , Additional file 1 : Tables S7–8).
Coronavirus disease 2019 (COVID-19)
We found blood group A (OR = 1.25; 95% CI: 1.14, 1.37) and blood group B (OR = 1.15; 95% CI: 1.07, 1.22) were associated with an increased risk of COVID-19 infection, compared with blood group O. But blood group O was associated with a decreased risk of COVID-19 infection (OR = 0.88; 95% CI: 0.82, 0.94), compared with blood group O. The association between blood group O and COVID-19 infection was supported by highly suggestive evidence. The other two associations were supported by suggestive evidence.
Human immunodeficiency virus (HIV)
Four blood groups of ABO blood group system were associated with an increased risk of HIV infection (RR = 24.25; 95% CI: 21.60, 27.23; blood group A versus blood group non-A, RR = 21.29; 95% CI: 18.62, 24.36; blood group B versus blood group non-B, RR = 5.44; 95% CI: 4.10, 7.22; blood group AB versus blood group non-AB, and RR = 47.85; 95% CI: 44.01, 52.03; blood group O versus blood group non-O). And these four associations were supported by highly suggestive evidence.
P. falciparum
Blood group A (OR = 1.68; 95% CI:1.32, 2.14), blood group B (OR = 1.97; 95% CI:1.49, 2.59), and blood group non-O (OR = 1.86; 95% CI:1.49, 2.33) were associated with an increased risk of P. falciparum infection, compared with blood group O. All of these associations were supported by suggestive evidence.
Cardiovascular and cerebrovascular outcomes
Twenty-five associations between blood group and cardiovascular and cerebrovascular outcomes were summarized. The magnitude of the observed summary random effects estimates ranged from 0.58 to 2.55 (Additional file 2 : Fig. S3). Of which, 21 (84%) associations gave a show on statistically significant effect nominally ( P < 0.05), and 7 associations reached suggestive or above evidence (Fig. 3 , Additional file 1 : Tables S7–8).
Myocardial infarction (MI)
Blood group A (OR = 1.29; 95% CI: 1.16, 1.45) and blood group non-O (OR = 1.25; 95% CI:1.14, 1.37) had an increased risk of MI compared with blood group O. Another association showed blood group O had an increased risk of MI (OR = 1.28; 95% CI:1.17, 1.40) compared with blood group non-O. All three associations reached suggestive evidence.
Peripheral vascular disease (PVD)
Compared with blood group O, blood group A (OR = 1.44; 95% CI: 1.19, 1.74) and blood group non-O (OR = 1.45; 95% CI:1.35, 1.56) had an increased risk of PVD. The associations between blood group A and blood group non-O and PVD risk were reached suggestive and highly suggestive evidence, respectively.
Venous thromboembolism (VTE)
Blood group A (OR = 1.63; 95% CI: 1.40, 1.89) and blood group non-O (OR = 2.10; 95% CI:1.83, 2.40) had an increased risk of VTE compared with blood group O. And the two associations reached highly suggestive evidence.
Oral-related outcome
Twenty-two associations between blood group and oral-related outcomes were summarized. The magnitude of the observed summary random effects estimates ranged from 0.70 to 1.36 (Additional file 2 : Fig. S4). Only one association gave a show on statistically significant effect nominally ( P < 0.05). No association reached suggestive or above evidence (Additional file 1 : Tables S7–8).
Pregnancy-related outcomes
We summarized twelve associations between blood group and pregnancy-related outcomes. The summary random effects estimate magnitude ranged from 0.90 to 1.49 (Additional file 2 : Fig. S5). Only two associations were statistically significant at P < 0.05. And no association reached suggestive or above evidence (Additional file 1 : Tables S7–8).
Metabolic disease outcomes
We summarized 5 associations between blood group and metabolic disease outcomes. The magnitude of the observed summary random effects estimates ranged from 0.91 to 1.28 (Additional file 2 : Fig. S6). Only 2 (40%) of 5 associations were nominally statistically significant at a P < 0.05 level. One association was supported by suggestive or above evidence (Fig. 3 , Additional file 1 : Tables S7–8).
Type 2 diabetes mellitus incidence (T2DM)
Blood group B, compared with blood group non-B, had a greater risk of T2DM (OR = 1.28; 95% CI: 1.17, 1.40), and the association was supported by convincing evidence.
Other outcomes
Ten associations between blood group and other outcomes (such as bleeding complication, decreased ovarian reserve) were summarized. The summary random effects estimate magnitude ranged from 0.84 to 1.33 (Additional file 2 : Fig. S7). Only one association was statistically significant at P < 0.05. And which was supported by highly suggestive evidence (Fig. 3 , Additional file 1 : Tables S7–8).
Bleeding complication
Blood group O was associated with a higher risk of bleeding complication (OR = 1.33; 95% CI: 1.25, 1.42), compared with blood group non-O, which was supported by highly suggestive evidence.
In summary, we found an association between blood group B and an increased risk of T2DM incidence (OR = 1.28; 95% CI: 1.17, 1.40) was rated as convincing evidence when it was taken as comparison for blood group non-B, by owing over 1000 cases, random P value < 10 –6 , not large heterogeneity ( I 2 < 50%), 95% PI excluding the null value, no hints for small-study effects and excess significance bias (Fig. 3 ). Eighteen associations were rated as highly suggestive evidence, they reached a statistically significant value at P < 10 −6 , had more 1000 cases, and the P value of the largest study was less than 0.05, such as comparison with blood group O, both blood group A (OR = 1.63; 95% CI: 1.40, 1.89) and non-O blood group (OR = 2.10; 95% CI: 1.83, 2.40) increased the risk of VTE incidence. In addition, we found 14 associations were rated as suggestive evidence. Fifty-six associations were rated as weak evidence and the remaining 181 associations were not significant (Additional file 1 : Tables S7–8).
Methodological quality of the meta-analyses
With the measurement tool AMSTAR, 18 (35%) articles were categorized as high quality. Of the 51 articles, 32 (63%) articles and only 1 (2%) article were categorized as moderate and low quality, respectively (Additional file 1 : Table S9).
Certainty of the evidence
Based on the GRADE approach, no health outcomes reached high credibility criteria. Nine of 89 health outcomes met the moderate certainty criteria. Thirty-three and 47 of 89 health outcomes met the low and very low certainty criteria, respectively (Additional file 1 : Table S10).
Sensitivity analyses
Findings from sensitivity analyses are reported in Additional file 1 : Tables S11–13. Removal of small-sized studies from the meta-analyses with evidence of small-study effects, these evidence ratings were not modified. When excluding low-quality studies, the associations between blood group A and gastric cancer, pancreatic cancer, and VTE and blood group O and pancreatic cancer retained their highly suggestive evidence ratings. When we focused on the associations excluded due to overlap, twelve associations were downgraded because of random P value.
Main findings
This is the first UR to provide a comprehensive overview of the observational data assessing associations between the ABO and Rh blood groups and multiple health outcomes. And we found 89 statistically significant associations. Convincing (Class I) evidence was only presented for the association between blood group B and T2DM risk. Highly suggestive (Class II) evidence was presented for 18 associations, such as HIV and VTE.
Comparison with previous studies
The positive association between blood group B and the risk of T2DM detected in this UR was supported by a prospective cohort study. This study included 82,104 women and followed for 18 years in France, throughout which 3553 women had a validated diagnosis of T2DM. After adjustment for potential confounders, blood group B increased the risk of T2DM compared with blood group O (HR = 1.21; 95% CI: 1.07, 1.36) [ 79 ]. A comparative cross-sectional study, including 326 participants (163 T2DM patients and 163 age and sex-matched healthy individuals), confirmed the harmful association of blood group B with T2DM risks (OR = 1.96; 95% CI: 1.05, 3.65), compared with the non-B blood group [ 80 ]. A meta-analysis revealed blood group B was significantly associated with an increased risk of T2DM (RR = 1.05; 95% CI: 0.93, 1.18), compared with the non-B blood group [ 81 ]. Nevertheless, caution is warranted in interpreting the observed association between blood group B and T2DM risk. Despite our result being consistent with findings from a prospective cohort study conducted in France, it is important to note that they exclusively included women. Subgroup analysis stratified by gender is needed in the future. In addition, the above studies have different control groups, sample sizes, and study designs. Further well-designed, large-scale prospective studies are needed to clarify the association between blood group B and T2DM.
The association between blood groups and HIV infection wase debated. In our UR, we found all ABO blood group was associated with an increased risk of HIV infection, and all of them were supported by highly suggestive evidence. A cross-sectional study conducted in Nairobi, Kenya among 280 female sex workers showed blood group A (OR = 1.56; 95% CI: 1.06, 2.28) was associated with HIV infection, compared with blood group O. However, blood group B (OR = 1.63; 95% CI: 0.94, 2.80) and blood group AB (OR = 1.50; 95% CI: 0.57, 3.93) were not associated with HIV infection [ 82 ]. A previous cross-sectional study conducted in South Africa investigated the associations between ABO blood groups and HIV infection among blood donors. The results suggested that the ABO blood group was not related to HIV infection. However, the point estimate for OR assesses blood group AB and HIV infection is 1.03 [ 83 ]. Cross-sectional studies cannot be used to infer causality and potential biases should be considered in the observational studies. Further well-designed longitudinal studies and controlling for different sources of bias are warranted to assess causality.
The harmful association between blood group A and non-O blood group and VTE incidence observed in our UR was supported by previous studies. For example, a previous study that included 7830 patients found blood group A was associated with VTE incidence (OR = 2.16; 95% CI: 1.10, 4.24) [ 84 ] in comparison with the blood group.
Biological plausibility
Multifactorial mechanisms might explain the increased risk of T2DM associated with blood groups. The previous study showed ABO blood group is in association with the level of plasma soluble intercellular adhesion molecule-1 and tumor necrosis factor receptor-2 [ 85 ]. And the above markers are identified to contribute a higher risk of T2DM. Moreover, a study suggested that the ABO blood group, being a gene-determined host factor, modulated the composition of the intestinal microbiota [ 86 ], which played an important role in influencing metabolism including glucose metabolism, energy balance, and low-grade inflammation [ 87 ].
For potential mechanisms between blood group and HIV infection, some studies indicated that expression of glycosyltransferase could be induced due to HIV and further synthetization of antigens of blood type on lymphocyte surfaces [ 88 , 89 ]. Therefore, apart from releasing new virion particles from lymphocytes, HIV could also integrate antigens of the blood group into its envelope surface [ 89 ]. The presence of these antigens sensitizes the virus against neutralizing antibodies and complements specific blood groups, potentially influencing the virus’s transmission between individuals and different blood groups [ 88 ].
It has not been thoroughly clear of the exact mechanism revealing the ABO blood group and VTE. The most likely hypothesis is that ABO plays a role in dominating the glycosylation degree of von Willebrand factor via modifying GT expressions [ 90 ]. von Willebrand factor multimeric composition is regulated in plasma by ADAMTS13. Proteolysis is enhanced by von Willebrand factor deglycosylation by ADAMTS13 [ 91 ]. In addition, individuals with blood group A1 and blood group B are at the level of 20% higher circulating Willebrand factor on average, which factor VIII levels than for O or A2 [ 92 , 93 ], high plasma levels of Willebrand factor, and factor VIII having association with increased VTE risk [ 94 , 95 , 96 , 97 ].
Strengths and limitations
To our knowledge, this is the first UR that systematically and comprehensively appraises the hierarchy of evidence relating blood groups to various health outcomes. Beyond summarizing the findings for a series of health end-point, we further an inquiry into bias and heterogeneity in the observational blood group literature. Compared with an individual systematic review or meta-analysis. This UR helped to summarize the complicated and vast amounts of research by comparing and contrasting the results of individual reviews, which provided an efficient overview of the findings for a particular problem [ 98 ]. Moreover, we adhered to a systematic methodology involving a search strategy in electronic databases and study selection and extraction conducted by two separate researchers. We also used standard approaches to evaluate the methodological quality and epidemiological evidence strength of the included studies.
UR provides top-tier evidence and important insights, but several limitations should be considered. First, some systematic reviews and meta-analyses did not acquire the level of evidence because they did not provide the number of cases. Second, we used I 2 (an estimate of the proportion of variance reflecting true differences in effect size) and τ 2 (an estimate of true variation in the summary estimate) to evaluate statistical heterogeneity. According to UR criteria, I 2 < 50% was applied as one of the criteria for convincing evidence in our UR, assigning the best evidence grade to robust associations. Several systematic reviews with meta-analyses examined the clinical and methodological heterogeneity by performing subgroup analyses stratified by these characteristics. Of note, we also extracted this information and analyzed it in the present UR. For example, within the subgroup comprising pancreatic cancer patients classified as either CagA-nonendemic or CagA endemic and COVID-19 patients with hospitalization, we found the results from subgroup analyses were consistent with the main findings. Future studies should better explore clinical and methodological heterogeneity to verify the association between blood groups and various health outcomes. Third, for the same health outcome (e.g., COVID-19 infection), the comparison group is different (e.g., A vs B, A vs AB, A vs O, and A vs non-A). Therefore, the findings between the blood group and health outcome in our study should be interpreted with caution. Fourth, we identified studies from published systematic reviews with meta-analyses, which may have omitted some individual studies for not in the systematic reviews with meta-analyses above. However, the systematic reviews with meta-analyses included in the current study were those of included the largest number of primary studies, which was unlikely to affect our results. Fifth, the reliability of the UR relies directly on the incorporated systematic reviews with meta-analyses. However, some included systematic reviews with meta-analyses existed risk of bias, which might decrease the robustness of statistical analyses. The study did not adjust for confounding factors that could have mediated associations between blood group and outcomes, because adjustment for potential confounders was unavailable in published systematic reviews with meta-analyses. Sixth, as this UR only included observational data, limitations common to this approach may influence the results of this review, such as information bias and residual confounding. There was a limited number of systematic reviews with meta-analyses that exclusively included prospective study designs, where information bias was reduced. However, case–control and cross-sectional study designs were more common than prospective study designs and were associated with a higher potential for information bias and reverse causation.
This comprehensive UR will help investigators to judge the relative priority of health outcomes related to the ABO blood group and RH blood group for future research and clinical management of the disease. In summary, compared with the non-B blood group, we found the association between blood group B and increased risk of T2DM incidence (OR = 1.28; 95% CI: 1.17, 1.40) was supported by convincing evidence. We also found 18 associations, such as blood group A and the risk of VTE incidence (OR = 1.63; 95% CI: 1.40, 1.89) and non-O blood group and the risk of VTE incidence (OR = 2.10; 95% CI: 1.83, 2.40), were supported by highly suggestive evidence. To enhance the quality of evidence regarding these associations and be able to give strong recommendations, future studies should consider several aspects. For example, set the same control group to increase the comparability of results, use standard definition of exposure or outcome to reduce clinical heterogeneity, and match the characteristics between cases and controls to reduce the impact of potential confounding. In addition, future studies understanding mechanisms between blood groups and various health outcomes are needed.
Availability of data and materials
Not applicable.
Abbreviations
A Measurement Tool to Assess Systematic Reviews
Confidence intervals
Human immunodeficiency virus infection
Hazard ratio
Prediction intervals
Peripheral vascular disease
Type 2 diabetes mellitus
- Umbrella review
Venous thromboembolism
Daniels G. Human blood group systems. In: Practical transfusion medicine. 2017. p. 20–8.
Chapter Google Scholar
Landsteiner K, Wiener AS. An agglutinable factor in human blood recognized by immune sera for rhesus blood. Proc Soc Exp Biol Med. 1940;43(1):223.
Article CAS Google Scholar
Cooling L. Blood groups in infection and host susceptibility. Clin Microbiol Rev. 2015;28(3):801–70.
Article CAS PubMed PubMed Central Google Scholar
Avent ND, Reid ME. The Rh blood group system: a review. Blood. 2000;95(2):375–87.
Article CAS PubMed Google Scholar
Bruun-Rasmussen P, Hanefeld Dziegiel M, Banasik K, Johansson PI, Brunak S. Associations of ABO and Rhesus D blood groups with phenome-wide disease incidence: a 41-year retrospective cohort study of 482,914 patients. Elife. 2023;12:e83116.
Hoglund J, Karlsson T, Johansson T, Ek WE, Johansson A. Characterization of the human ABO genotypes and their association to common inflammatory and cardiovascular diseases in the UK Biobank. Am J Hematol. 2021;96(11):1350–62.
Clark P, Wu O. ABO(H) blood groups and pre-eclampsia. A systematic review and meta-analysis. Thromb Haemost. 2008;100(3):469–74.
CAS PubMed Google Scholar
Iodice S, Maisonneuve P, Botteri E, Sandri MT, Lowenfels AB. ABO blood group and cancer. Eur J Cancer. 2010;46(18):3345–50.
Getawa S, Bayleyegn B, Aynalem M, Worku YB, Adane T. Relationships of ABO and Rhesus blood groups with type 2 diabetes mellitus: a systematic review and meta-analysis. J Int Med Res. 2022;50(10):3000605221129547.
Bawazir WM. Systematic review and meta-analysis of the susceptibility of ABO blood groups to venous thromboembolism in individuals with Factor V Leiden. Diagnostics (Basel). 2022;12(8):1936.
Dentali F, Sironi AP, Ageno W, Turato S, Bonfanti C, Frattini F, et al. Non-O blood type is the commonest genetic risk factor for VTE: results from a meta-analysis of the literature. Semin Thromb Hemost. 2012;38(5):535–48.
Article PubMed Google Scholar
Zhang Q, Peng H, Hu L, Ren R, Peng X, Song J. Association between ABO blood group and venous thromboembolism risk in patients with peripherally inserted central catheters: a meta-analysis and systematic review. Front Oncol. 2022;12:906427.
Article PubMed PubMed Central Google Scholar
Ioannidis JP. Integration of evidence from multiple meta-analyses: a primer on umbrella reviews, treatment networks and multiple treatments meta-analyses. CMAJ. 2009;181(8):488–93.
Moher D, Liberati A, Tetzlaff J, Altman DG, Group P. Preferred reporting items for systematic reviews and meta-analyses: the PRISMA statement. PLoS Med. 2009;6(7):e1000097.
Stroup DF, Berlin JA, Morton SC, Olkin I, Williamson GD, Rennie D, et al. Meta-analysis of observational studies in epidemiology: a proposal for reporting. Meta-analysis Of Observational Studies in Epidemiology (MOOSE) group. JAMA. 2000;283(15):2008–12.
Bellou V, Belbasis L, Tzoulaki I, Evangelou E, Ioannidis JP. Environmental risk factors and Parkinson’s disease: an umbrella review of meta-analyses. Parkinsonism Relat Disord. 2016;23:1–9.
Pieper D, Antoine SL, Mathes T, Neugebauer EA, Eikermann M. Systematic review finds overlapping reviews were not mentioned in every other overview. J Clin Epidemiol. 2014;67(4):368–75.
Senn SJ. Overstating the evidence: double counting in meta-analysis and related problems. BMC Med Res Methodol. 2009;9:10.
Smith V, Devane D, Begley CM, Clarke M. Methodology in conducting a systematic review of systematic reviews of healthcare interventions. BMC Med Res Methodol. 2011;11(1):15.
Deeks JJ, Higgins JP, Altman DG. Chapter 10: Analysing data and undertaking meta-analyses. In: Cochrane handbook for systematic reviews of interventions. 2nd ed. 2019.
Google Scholar
Riley RD, Higgins JP, Deeks JJ. Interpretation of random effects meta-analyses. BMJ. 2011;342:d549.
Higgins JP, Thompson SG, Deeks JJ, Altman DG. Measuring inconsistency in meta-analyses. BMJ. 2003;327(7414):557–60.
Egger M, Davey Smith G, Schneider M, Minder C. Bias in meta-analysis detected by a simple, graphical test. BMJ. 1997;315(7109):629–34.
Sterne JA, Sutton AJ, Ioannidis JP, Terrin N, Jones DR, Lau J, et al. Recommendations for examining and interpreting funnel plot asymmetry in meta-analyses of randomised controlled trials. BMJ. 2011;343:d4002.
Ioannidis JP, Trikalinos TA. An exploratory test for an excess of significant findings. Clin Trials. 2007;4(3):245–53.
Hayhoe B, Kim D, Aylin PP, Majeed FA, Cowie MR, Bottle A. Adherence to guidelines in management of symptoms suggestive of heart failure in primary care. Heart (British Cardiac Society). 2019;105(9):678–85.
PubMed Google Scholar
Lubin JH, Gail MH. On power and sample size for studying features of the relative odds of disease. Am J Epidemiol. 1990;131(3):552–66.
Qin X, Chen J, Jia G, Yang Z. Dietary factors and pancreatic cancer risk: an umbrella review of meta-analyses of prospective observational studies. Adv Nutr. 2023;14(3):451–64.
Liu D, Meng X, Tian Q, Cao W, Fan X, Wu L, et al. Vitamin D and multiple health outcomes: an umbrella review of observational studies, randomized controlled trials, and Mendelian randomization studies. Adv Nutr. 2022;13(4):1044–62.
Aromataris E, Fernandez R, Godfrey CM, Holly C, Khalil H, Tungpunkom P. Summarizing systematic reviews: methodological development, conduct and reporting of an umbrella review approach. Int J Evid Based Healthc. 2015;13(3):132–40.
Cui H, Qu Y, Zhang L, Zhang W, Yan P, Yang C, et al. Epidemiological and genetic evidence for the relationship between ABO blood group and human cancer. Int J Cancer. 2023;153(2):320–30.
Noori M, Shokri P, Nejadghaderi SA, Golmohammadi S, Carson-Chahhoud K, Bragazzi NL, et al. ABO blood groups and risk of human immunodeficiency virus infection: a systematic review and meta-analysis. Rev Med Virol. 2022;32(3):e2298.
Degarege A, Gebrezgi MT, Beck-Sague CM, Wahlgren M, de Mattos LC, Madhivanan P. Effect of ABO blood group on asymptomatic, uncomplicated and placental Plasmodium falciparum infection: systematic review and meta-analysis. BMC Infect Dis. 2019;19(1):86.
Shea BJ, Hamel C, Wells GA, Bouter LM, Kristjansson E, Grimshaw J, et al. AMSTAR is a reliable and valid measurement tool to assess the methodological quality of systematic reviews. J Clin Epidemiol. 2009;62(10):1013–20.
Singh A, Purohit BM. ABO blood groups and its association with oral cancer, oral potentially malignant disorders and oral submucous fibrosis- a systematic review and meta-analysis. Asian Pac J Cancer Prev. 2021;22(6):1703–12.
Degarege A, Gebrezgi MT, Ibanez G, Wahlgren M, Madhivanan P. Effect of the ABO blood group on susceptibility to severe malaria: a systematic review and meta-analysis. Blood Rev. 2019;33:53–62.
Panda AK, Panda SK, Sahu AN, Tripathy R, Ravindran B, Das BK. Association of ABO blood group with severe falciparum malaria in adults: case control study and meta-analysis. Malar J. 2011;10:309.
Adegnika AA, Luty AJ, Grobusch MP, Ramharter M, Yazdanbakhsh M, Kremsner PG, et al. ABO blood group and the risk of placental malaria in sub-Saharan Africa. Malar J. 2011;10:101.
Bhattacharjee S, Banerjee M, Pal R. ABO blood groups and severe outcomes in COVID-19: a meta-analysis. Postgrad Med J. 2022;98(e2):e136–7.
Wu BB, Gu DZ, Yu JN, Yang J, Shen WQ. Association between ABO blood groups and COVID-19 infection, severity and demise: a systematic review and meta-analysis. Infect Genet Evol. 2020;84:104485.
Lubkin DT, Van Gent JM, Cotton BA, Brill JB. Mortality and outcomes by blood group in trauma patients: a systematic review and meta-analysis. Vox Sang. 2023;118(6):421–9.
Banchelli F, Negro P, Guido M, D’Amico R, Fittipaldo VA, Grima P, et al. The role of ABO blood type in patients with SARS-CoV-2 infection: a systematic review. J Clin Med. 2022;11(11):3029.
Dentali F, Sironi AP, Ageno W, Bonfanti C, Crestani S, Frattini F, et al. Relationship between ABO blood group and hemorrhage: a systematic literature review and meta-analysis. Semin Thromb Hemost. 2013;39(1):72–82.
Dentali F, Sironi AP, Ageno W, Crestani S, Franchini M. ABO blood group and vascular disease: an update. Semin Thromb Hemost. 2014;40(1):49–59.
Liu F, Li C, Zhu J, Ren L, Qi X. ABO blood type and risk of hepatocellular carcinoma: a meta-analysis. Expert Rev Gastroenterol Hepatol. 2018;12(9):927–33.
Urabe F, Kimura S, Iwatani K, Yasue K, Koike Y, Tashiro K, et al. The impact of ABO blood type on developing venous thromboembolism in cancer patients: systematic review and meta-analysis. J Clin Med. 2021;10(16):3692.
Balaouras G, Eusebi P, Kostoulas P. Systematic review and meta-analysis of the effect of ABO blood group on the risk of SARS-CoV-2 infection. PLoS ONE. 2022;17(7):e0271451.
Yang H, Tan Z, Zhang Y, Sun J, Huang P. ABO blood classification and the risk of lung cancer: a meta-analysis and trial sequential analysis. Oncol Lett. 2022;24(4):340.
Risch HA, Lu L, Wang J, Zhang W, Ni Q, Gao YT, et al. ABO blood group and risk of pancreatic cancer: a study in Shanghai and meta-analysis. Am J Epidemiol. 2012;177(12):1326–37.
Article Google Scholar
Risch HA, Lu L, Wang J, Zhang W, Ni Q, Gao YT, et al. ABO blood group and risk of pancreatic cancer: a study in Shanghai and meta-analysis. Am J Epidemiol. 2013;177(12):1326–37.
Takagi H, Umemoto T, All-Literature Investigation of Cardiovascular Evidence G. Meta-analysis of non-O blood group as an independent risk factor for coronary artery disease. Am J Cardiol. 2015;116(5):699–704.
Cheung JLS, Cheung VLS, Athale U. Impact of ABO Blood Group on the Development of Venous Thromboembolism in Children With Cancer: A Systematic Review and Meta-Analysis. J Pediatr Hematol Oncol. 2021;43(6):216–23.
Deng J, Jia M, Cheng X, Yan Z, Fan D, Tian X. ABO blood group and ovarian reserve: a meta-analysis and systematic review. Oncotarget. 2017;8(15):25628–36.
Zhao J, Yao Z, Hao J, Xu B, Wang Y, Li Y. Association of ABO blood groups with ovarian reserve, and outcomes after assisted reproductive technology: systematic review and meta-analyses. Reprod Biol Endocrinol. 2021;19(1):20.
Ai L, Li J, Wang W, Li Y. ABO blood group and risk of malaria during pregnancy: a systematic review and meta-analysis. Epidemiol Infect. 2022;150:e25.
Al-Askar M. Is there an association between periodontal diseases and ABO blood group? Systematic review and meta-analysis. Quintessence Int. 2022;53(5):404–12.
Bahardoust M, Barahman G, Baghaei A, Ghadimi P, Asadi Shahir MH, NajafiKandovan M, et al. The association between ABO blood group and the risk of colorectal cancer: a systematic literature review and meta-analysis. Asian Pac J Cancer Prev. 2023;24(8):2555–63.
Loscertales MP, Owens S, O’Donnell J, Bunn J, Bosch-Capblanch X, Brabin BJ. ABO blood group phenotypes and Plasmodium falciparum malaria: unlocking a pivotal mechanism. Adv Parasitol. 2007;65:1–50.
Gutierrez-Valencia M, Leache L, Librero J, Jerico C, Enguita German M, Garcia-Erce JA. ABO blood group and risk of COVID-19 infection and complications: a systematic review and meta-analysis. Transfusion. 2022;62(2):493–505.
Franchini M, Cruciani M, Mengoli C, Marano G, Candura F, Lopez N, et al. ABO blood group and COVID-19: an updated systematic literature review and meta-analysis. Blood Transfus. 2021;19(4):317–26.
PubMed PubMed Central Google Scholar
He M, Wolpin B, Rexrode K, Manson JE, Rimm E, Hu FB, et al. ABO blood group and risk of coronary heart disease in two prospective cohort studies. Arterioscler Thromb Vasc Biol. 2012;32(9):2314–20.
Liu N, Zhang T, Ma L, Zhang H, Wang H, Wei W, et al. The impact of ABO blood group on COVID-19 infection risk and mortality: a systematic review and meta-analysis. Blood Rev. 2021;48:100785.
Razzaghi N, Seraj H, Heydari K, Azadeh H, Salehi A, Behnamfar M, et al. ABO blood groups associations with ovarian cancer: a systematic review and meta-analysis. Ind J Gynecol Oncol. 2020;18(4). https://doi.org/10.1007/s40944-020-00463-y .
Wu O, Bayoumi N, Vickers MA, Clark P. ABO(H) blood groups and vascular disease: a systematic review and meta-analysis. J Thromb Haemost. 2008;6(1):62–9.
Ding P. ABO blood groups and prognosis of gastric cancer: a meta-analysis. 2019.
Tiongco RE, Paragas NA, Dominguez MJ, Lasta SL, Pandac JK, Pineda-Cortel MR. ABO blood group antigens may be associated with increased susceptibility to schistosomiasis: a systematic review and meta-analysis. J Helminthol. 2018;94:e21.
Jing SW, Xu Q, Zhang XY, Jing ZH, Zhao ZJ, Zhang RH, et al. Are people with blood group O more susceptible to nasopharyngeal carcinoma and have worse survival rates? A systematic review and meta-analysis. Front Oncol. 2021;11:698113.
Miao SY, Zhou W, Chen L, Wang S, Liu XA. Influence of ABO blood group and Rhesus factor on breast cancer risk: a meta-analysis of 9665 breast cancer patients and 244,768 controls. Asia Pac J Clin Oncol. 2014;10(2):101–8.
Itenov TS, Sessler DI, Khanna AK, Ostrowski SR, Johansson PI, Erikstrup C, et al. ABO blood types and sepsis mortality. Ann Intensive Care. 2021;11(1):61.
Li T, Wang Y, Wu L, Ling Z, Li C, Long W, et al. The association between ABO blood group and preeclampsia: a systematic review and meta-analysis. Front Cardiovasc Med. 2021;8:665069.
Nayeri T, Moosazadeh M, Dalimi Asl A, Ghaffarifar F, Sarvi S, Daryani A. Toxoplasma gondii infection and ABO blood groups: a systematic review and meta-analysis. Trans R Soc Trop Med Hyg. 2024;118(4):234–46.
Wang W, Liu L, Wang Z, Lu X, Wei M, Lin T, et al. ABO blood group and esophageal carcinoma risk: from a case-control study in Chinese population to meta-analysis. Cancer Causes Control. 2014;25(10):1369–77.
Jing W, Zhao S, Liu J, Liu M. ABO blood groups and hepatitis B virus infection: a systematic review and meta-analysis. BMJ Open. 2020;10(1):e034114.
Rattanapan Y, Duangchan T, Wangdi K, Mahittikorn A, Kotepui M. Association between Rhesus blood groups and malaria infection: a systematic review and meta-analysis. Trop Med Infect Dis. 2023;8(4):190.
Liao Y, Xue L, Gao J, Wu A, Kou X. ABO blood group-associated susceptibility to norovirus infection: a systematic review and meta-analysis. Infect Genet Evol. 2020;81:104245.
Chakrani Z, Robinson K, Taye B. Association between ABO blood groups and helicobacter pylori infection: a meta-analysis. Sci Rep. 2018;8(1):17604.
Wang Z, Liu L, Ji J, Zhang J, Yan M, Zhang J, et al. ABO blood group system and gastric cancer: a case-control study and meta-analysis. Int J Mol Sci. 2012;13(10):13308–21.
Chen Z, Yang SH, Xu H, Li JJ. ABO blood group system and the coronary artery disease: an updated systematic review and meta-analysis. Sci Rep. 2016;6:23250.
Fagherazzi G, Gusto G, Clavel-Chapelon F, Balkau B, Bonnet F. ABO and Rhesus blood groups and risk of type 2 diabetes: evidence from the large E3N cohort study. Diabetologia. 2015;58(3):519–22.
Walle M, Tesfaye A, Getu F. The association of ABO and Rhesus blood groups with the occurrence of type 2 diabetes mellitus: a comparative cross-sectional study. Medicine (Baltimore). 2023;102(35):e34803.
Cano EA, Esguerra MA, Batausa AM, Baluyut JR, Cadiz R, Docto HF, et al. Association between ABO blood groups and type 2 diabetes mellitus: a meta-analysis. Curr Diabetes Rev. 2023;19(6):e270422204139.
Chanzu NM, Mwanda W, Oyugi J, Anzala O. Mucosal blood group antigen expression profiles and HIV infections: a study among female sex workers in Kenya. PLoS ONE. 2015;10(7):e0133049.
Jacobs G, Van den Berg K, Vermeulen M, Swanevelder R, Custer B, Murphy EL. Association of ABO and RhD blood groups with the risk of HIV infection. PLoS ONE. 2023;18(4):e0284975.
Englisch C, Moik F, Nopp S, Raderer M, Pabinger I, Ay C. ABO blood group type and risk of venous thromboembolism in patients with cancer. Blood Adv. 2022;6(24):6274–81.
Barbalic M, Dupuis J, Dehghan A, Bis JC, Hoogeveen RC, Schnabel RB, et al. Large-scale genomic studies reveal central role of ABO in sP-selectin and sICAM-1 levels. Hum Mol Genet. 2010;19(9):1863–72.
Makivuokko H, Lahtinen SJ, Wacklin P, Tuovinen E, Tenkanen H, Nikkila J, et al. Association between the ABO blood group and the human intestinal microbiota composition. BMC Microbiol. 2012;12:94.
Cani PD, Osto M, Geurts L, Everard A. Involvement of gut microbiota in the development of low-grade inflammation and type 2 diabetes associated with obesity. Gut Microbes. 2012;3(4):279–88.
Arendrup M, Hansen JE, Clausen H, Nielsen C, Mathiesen LR, Nielsen JO. Antibody to histo-blood group A antigen neutralizes HIV produced by lymphocytes from blood group A donors but not from blood group B or O donors. AIDS. 1991;5(4):441–4.
Neil SJ, McKnight A, Gustafsson K, Weiss RA. HIV-1 incorporates ABO histo-blood group antigens that sensitize virions to complement-mediated inactivation. Blood. 2005;105(12):4693–9.
Jenkins PV, O’Donnell JS. ABO blood group determines plasma von Willebrand factor levels: a biologic function after all? Transfusion. 2006;46(10):1836–44.
Schulz KF, Altman DG, Moher D, Group C. CONSORT 2010 statement: updated guidelines for reporting parallel group randomised trials. BMC Med. 2010;8:18.
Morange PE, Tregouet DA, Frere C, Saut N, Pellegrina L, Alessi MC, et al. Biological and genetic factors influencing plasma factor VIII levels in a healthy family population: results from the Stanislas cohort. Br J Haematol. 2005;128(1):91–9.
O’Donnell J, Laffan MA. The relationship between ABO histo-blood group, factor VIII and von Willebrand factor. Transfus Med. 2001;11(4):343–51.
Koster T, Blann AD, Briet E, Vandenbroucke JP, Rosendaal FR. Role of clotting factor VIII in effect of von Willebrand factor on occurrence of deep-vein thrombosis. Lancet. 1995;345(8943):152–5.
Kraaijenhagen RA, in’t Anker PS, Koopman MM, Reitsma PH, Prins MH, van den Ende A, et al. High plasma concentration of factor VIIIc is a major risk factor for venous thromboembolism. Thromb Haemost. 2000;83(1):5–9.
Rietveld IM, Lijfering WM, le Cessie S, Bos MHA, Rosendaal FR, Reitsma PH, et al. High levels of coagulation factors and venous thrombosis risk: strongest association for factor VIII and von Willebrand factor. J Thromb Haemost. 2019;17(1):99–109.
Souto JC, Almasy L, Muniz-Diaz E, Soria JM, Borrell M, Bayen L, et al. Functional effects of the ABO locus polymorphism on plasma levels of von Willebrand factor, factor VIII, and activated partial thromboplastin time. Arterioscler Thromb Vasc Biol. 2000;20(8):2024–8.
Papatheodorou S. Umbrella reviews: what they are and why we need them. Eur J Epidemiol. 2019;34(6):543–6.
Download references
Acknowledgements
This work was supported by the National Key Research and Development Program of China (No. 2022YFC2704205 to Wu QJ), the Natural Science Foundation of China (No. 82073647 and No. 82373674 to Wu QJ and No.82103914 to Gong TT), Outstanding Scientific Fund of Shengjing Hospital (Wu QJ), and 345 Talent Project of Shengjing Hospital of China Medical University (Gong TT).
Author information
Fang-Hua Liu, Jia-Kai Guo, Wei-Yi Xing, Xue-Li Bai, and Yu-Jiao Chang contributed equally to this work.
Authors and Affiliations
Department of Clinical Epidemiology, Shengjing Hospital of China Medical University, Shenyang, China
Fang-Hua Liu, Jia-Kai Guo, Wei-Yi Xing, Xue-Li Bai, Yu-Jiao Chang, Zhao Lu, Miao Yang, Ying Yang, Wen-Jing Li, Xian-Xian Jia, Tao Zhang, Jing Yang & Qi-Jun Wu
Clinical Research Center, Shengjing Hospital of China Medical University, Shenyang, China
Fang-Hua Liu, Wei-Yi Xing, Yu-Jiao Chang & Qi-Jun Wu
Hospital Management Office, Shengjing Hospital of China Medical University, Shenyang, China
Jia-Kai Guo
Department of Obstetrics and Gynecology, The Fourth Affiliated Hospital of China Medical University, Shenyang, China
Xue-Li Bai, Song Gao, De-Yu Zhang, Chuan Liu, Ting-Ting Gong & Qi-Jun Wu
Department of Radiology, Shengjing Hospital of China Medical University, Shenyang, China
Zhao Lu & Tao Zhang
Department of Pediatrics, Shengjing Hospital of China Medical University, Shenyang, China
Miao Yang & Xian-Xian Jia
Department of Hematology, Shengjing Hospital of China Medical University, Shenyang, China
Department of Otolaryngology Head and Neck Surgery, Shengjing Hospital of China Medical University, Shenyang, China
Wen-Jing Li
Department of Endocrinology, Shengjing Hospital of China Medical University, Shenyang, China
School of Medicine, Zhejiang University, Hangzhou, China
Jun-Tong Chen
Cancer Epidemiology Division, Population Sciences in the Pacific Program, University of Hawaii Cancer Center, University of Hawaii at Manoa, Honolulu, HI, USA
NHC Key Laboratory of Advanced Reproductive Medicine and Fertility (China Medical University), National Health Commission, Shenyang, China
You can also search for this author in PubMed Google Scholar
Contributions
F-HL, J-KG, T-TG, and Q-JW contributed to the study design. J-KG, ZL, MY, X-LB, YY, W-JL, X-XJ, and TZ collection of data. F-HL and W-YX analysis of data. F-HL, J-KG, Y-JC, YJ, J-TC, SG, LW, D-YZ, CL, T-TG, and Q-JW wrote the first draft of the manuscript and edited the manuscript. All authors read and approved the final manuscript. F-HL, J-KG, W-YX, X-LB, and Y-JC contributed equally to this work.
Corresponding authors
Correspondence to De-Yu Zhang , Chuan Liu , Ting-Ting Gong or Qi-Jun Wu .
Ethics declarations
Ethics approval and consent to participate, consent for publication, competing interests.
The authors declare that they have no competing interests.
Additional information
Publisher’s note.
Springer Nature remains neutral with regard to jurisdictional claims in published maps and institutional affiliations.
Supplementary Information
12916_2024_3423_moesm1_esm.xlsx.
Additional file 1: Tables S1-13. Table S1-PRISMA checklist of items to include when reporting a systematic review or meta-analysis; Table S2-MOOSE checklist for meta-analyses of observational studies; Table S3-Search strategy; Table S4-Criteria for categorizing the credibility of evidence in the umbrella review; Table S5-The list of the excluded records during the process of full-text review; Table S6-The summary results of meta-analyses excluded due to lack of data for quantitative synthesis; Table S7-Description of 270 associations investigating the associations between ABO and Rhesus blood groups and multiple health outcomes; Table S8-Strength assessment of evidence from 270 associations examining associations between ABO and Rhesus blood groups and multiple health outcomes; Table S9-Methodological quality assessment of the included articles with AMSTAR; Table S10-The results of GRADE assessment of the evidence certainty on the associations between ABO and Rhesus blood groups and multiple health outcomes; Table S11-Sensitivity analysis results of omission of small-sized studies (< 25th percentile) from those meta-analyses with evidence of small-study effects; Table S12-Sensitivity analysis results of omission of primary studies with low-quality evidence; Table S13-Sensitivity analysis results of excluded meta-analyses due to overlap.
12916_2024_3423_MOESM2_ESM.docx
Additional file 2: Fig. S1-7. Fig. S1-Summary effects sizes with inverse of the variance of association between blood group and cancer outcomes; Fig. S2-Summary effects sizes with inverse of the variance of association between blood group and infectious disease outcomes; Fig. S3-Summary effects sizes with inverse of the variance of association between blood group and cardiovascular and cerebrovascular outcomes; Fig. S4-Summary effects sizes with inverse of the variance of association between blood group and oral related outcomes; Fig. S5-Summary effects sizes with inverse of the variance of association between blood group and pregnancy related outcomes; Fig. S6-Summary effects sizes with inverse of the variance of association between blood group and metabolic disease outcomes; Fig. S7-Summary effects sizes with inverse of the variance of association between blood group and other outcomes.
Rights and permissions
Open Access This article is licensed under a Creative Commons Attribution 4.0 International License, which permits use, sharing, adaptation, distribution and reproduction in any medium or format, as long as you give appropriate credit to the original author(s) and the source, provide a link to the Creative Commons licence, and indicate if changes were made. The images or other third party material in this article are included in the article's Creative Commons licence, unless indicated otherwise in a credit line to the material. If material is not included in the article's Creative Commons licence and your intended use is not permitted by statutory regulation or exceeds the permitted use, you will need to obtain permission directly from the copyright holder. To view a copy of this licence, visit http://creativecommons.org/licenses/by/4.0/ . The Creative Commons Public Domain Dedication waiver ( http://creativecommons.org/publicdomain/zero/1.0/ ) applies to the data made available in this article, unless otherwise stated in a credit line to the data.
Reprints and permissions
About this article
Cite this article.
Liu, FH., Guo, JK., Xing, WY. et al. ABO and Rhesus blood groups and multiple health outcomes: an umbrella review of systematic reviews with meta-analyses of observational studies. BMC Med 22 , 206 (2024). https://doi.org/10.1186/s12916-024-03423-x
Download citation
Received : 29 November 2023
Accepted : 09 May 2024
Published : 20 May 2024
DOI : https://doi.org/10.1186/s12916-024-03423-x
Share this article
Anyone you share the following link with will be able to read this content:
Sorry, a shareable link is not currently available for this article.
Provided by the Springer Nature SharedIt content-sharing initiative
- ABO blood group
- Meta-analysis
- Observational study
- Rhesus blood group
BMC Medicine
ISSN: 1741-7015
- Submission enquiries: [email protected]
- General enquiries: [email protected]
- Open access
- Published: 14 May 2024
Estimating effects of whole grain consumption on type 2 diabetes, colorectal cancer and cardiovascular disease: a burden of proof study
- Houpu Liu 1 ,
- Jiahao Zhu 1 ,
- Rui Gao 1 ,
- Lilu Ding 1 ,
- Ye Yang 1 ,
- Wenxia Zhao 1 ,
- Xiaonan Cui 2 ,
- Wenli Lu 3 ,
- Jing Wang 1 na1 &
- Yingjun Li 1 na1
Nutrition Journal volume 23 , Article number: 49 ( 2024 ) Cite this article
350 Accesses
Metrics details
Previous studies on whole grain consumption had inconsistent findings and lacked quantitative assessments of evidence quality. Therefore, we aimed to summarize updated findings using the Burden of Proof analysis (BPRF) to investigate the relationship of whole grain consumption on type 2 diabetes (T2D), colorectal cancer (CRC), stroke, and ischemic heart disease (IHD).
We conducted a literature search in the Medline and Web of Science up to June 12, 2023, to identify related cohort studies and systematic reviews. The mean RR (relative risk) curve and uncertainty intervals (UIs), BPRF function, risk-outcome score (ROS), and the theoretical minimum risk exposure level (TMREL) were estimated to evaluate the level of four risk-outcome pairs.
In total, 27 prospective cohorts were included in our analysis. Consuming whole grain at the range of TMREL (118.5–148.1 g per day) was associated with lower risks: T2D (declined by 37.3%, 95% UI: 5.8 to 59.5), CRC (declined by 17.3%, 6.5 to 27.7), stroke (declined by 21.8%, 7.3 to 35.1), and IHD (declined by 36.9%, 7.1 to 58.0). For all outcomes except stroke, we observed a non-linear, monotonic decrease as whole grain consumption increased; For stroke, it followed a J -shaped curve (the greatest decline in the risk of stroke at consuming 100 g whole grain for a day). The relationships between whole grain consumption and four diseases are all two-star pairs (ROS: 0.087, 0.068, 0.062, 0.095 for T2D, CRC, stroke, and IHD, respectively).
Consuming 100 g of whole grains per day offers broad protective benefits. However, exceeding this threshold may diminish the protective effects against stroke. Our findings endorse replacing refined grains with whole grains as the main source of daily carbohydrates.
Registry and registry number for systematic reviews or meta-analyses
We have registered our research in PROSPERO, and the identifier of our meta-analyses is CRD42023447345 .
Peer Review reports
Introduction
Whole grains have been widely endorsed as a superior substitute for primary energy and carbohydrate sources in daily dietary guidelines because of their high dietary fiber content and numerous bioactive compounds [ 1 ]. The Global Burden of Disease Study 2019 (GBD 2019) has reported that lower intake of whole grain accounted for 1,844,836 (95% uncertainty interval [UI]: 2,338,609–921,291) deaths and 42.5 million (53.2–17.5) disability-adjusted life years (DALYs) [ 2 ]. The large estimated burden demonstrated the importance of fully appreciating the relationship between whole grain consumption and potentially related health outcomes and of further improving the strength of evidence supporting the understanding of those relationships.
Increasing evidence has found that a high intake of whole grains is related to a reduction in the risk of type 2 diabetes (T2D), colorectal cancer (CRC), ischemic heart disease (IHD), and stroke [ 2 , 3 ]. However, regarding CRC, T2D and IHD, previous studies, including dose-response meta-analysis or cohort studies, exhibit variations in their consumption ranges. This complicates the comparability and consolidation of evidence [ 3 , 4 , 5 , 6 ]. Besides, in relation to stroke, recent meta-analyses have presented inconsistent findings [ 7 , 8 ]. Although there is an increasing body of evidence supporting the positive impact of consuming whole grains on health, the challenge lies in accurately estimating RR associated with varying levels of consumption. This limitation hinders the ability of decision-makers to fully comprehend the strength of the connection between consuming whole grains and various health outcomes.
Burden of proof risk function (BPRF) is a new meta-analysis method that can quantitatively estimate the level of risk closest to the null hypothesis [ 9 ]. Hitherto, most of meta-regression studies applied given fixed knots to fit the spline models or forced a log-linear assumption to simplify statistical analysis. However, such a method may limit their ability to capture the effects of whole grain consumption on health outcomes, as the relationship between increasing whole grain intake and its impact on health might not be straightforward: it could lead to slight decreases in positive effects, or it could even become harmful if the consumption of whole grains goes beyond a certain point [ 3 , 10 ]. Unlike existing methods, BPRF relaxed the conventional assumption of a log-linear shape in risk functions, and instead applied a data-driven approach to determine the relationship of risk-outcome pairs using a quadratic spline. Thus, BPRF can help to identify the ‘true’ shape of the risk function [ 11 ]. In addition, existing methods, such as Grading of Recommendations, Assessment, Development and Evaluations (GRADE) or NutriGRADE, are commonly applied to assess the quality of the underlying evidence [ 12 ]. However, such methods are unable to extend to quantify variation in true effect size caused by bias from covariates or other limitations of the evidence [ 11 ]. Nevertheless, BPRF can synthesize available evidence in algorithm to calculate uncertainty inclusive of between-study heterogeneity.
To precisely quantify the health effects of whole grain consumption, a meta-regression analysis was conducted on the evidence from prospective cohort studies. This study focused specifically on four health outcomes (T2D, CRC, IHD, and stroke) linked to whole grain consumption, as reported by the GBD study [ 13 ].
Our protocol has been registered in International Prospective Register of Systematic Reviews (PROSPERO, identifier: CRD42023447345 ). We followed a standard framework of Preferred Reporting Items for Systematic Reviews and Meta-Analyses (PRISMA) guideline to report our results [ 14 ].
Search strategy and selection criteria
We searched data published in English in the MEDLINE and Web of Science for systematic review, and cohort studies from 1 January, 2000 to 12 June, 2023, using standard search strings (Supplementary Table 1 ). A reference list of included publications was also manually screened to identify additional cohort studies. Titles and abstracts were screened by two reviewers (H Liu and J Wang), with discrepancies being reconciled through consulting a third author (Y Li).
Only prospective observational studies (for both incidence and mortality) published in English were included. Studies should report a relative risk ratio (RR), odds ratio (OR) or hazard ratio (HR) of the associations between whole grain consumption and at least one of the four outcomes. Additionally, they should specify the amount of whole grain consumption in both the reference group and the alternate group for comparison.
Retrospective studies, conference abstracts, ecological studies, case reports, case-series, letters to the editor, conference proceedings, umbrella reviews, systematic reviews or meta-analyses as well as studies conducted in animals, children, or adolescents were excluded. Besides, we excluded studies that failed to report whole grain consumption without grams or servings equivalent, such as studies that used aggregated “diet scores” as a measure of consumption, and those that only reported specific subtypes of grains were also excluded. And studies reporting outcomes outside the scope of interest, such as all-cause mortality, or lacking specificity such as cardiovascular disease or diabetes mellitus, have been excluded.
Data extraction
For each study, we collected the information of the eligible studies including the first author’s name, location, population characteristics (age, sex, race, and sample size), follow-up period, exposure definition, exposure assessment method, outcome definition, outcome ascertainment method, and covariates used in the study. Data were extracted by one author (H Liu) and checked by another author (J Wang) for accuracy. Besides, we also collected data on the range of exposure, sample size, person-years, number of events and risk estimate (RRs, HRs or ORs) and its corresponding uncertainty to conduct BPRF analysis. The uniform extraction procedures are shown in Supplementary Table 2 .
We used a framework of BPRF methodology developed by Zheng et al. to assess the risk of bias in included studies [ 11 , 15 , 16 ]. For each included study, we extracted information concerning aspects of study design that could potentially bias the reported effect size and coded this information into study-level covariates [ 11 ]. These study-level covariates are followed as: follow-up time (≤ 10 months and > 10 months), exposure definitions, outcome definitions, effect size measures (HRs, RRs or ORs), the endpoint of outcome events (incidence or mortality), frequency of exposure measurements (single or repeat), outcome ascertainment methods (administrative records or self-reports), and the level of adjustment for relevant confounders (creating cascading dummy variables standing for the number of confounders adjusted in risk regression model from selected studies, and the minimum threshold for confounder adjustment for age and sex) [ 11 ]. These covariates would be further adjusted in our BPRF analysis if they significantly biased our estimated risk functions.
In addition to these covariates, we selected four common study characteristics that are highly relevant and likely to introduce bias, in order to evaluate the study quality [ 9 , 17 , 18 ]. These characteristics include the representativeness of the study population (whether it represents the general population or specific sub-groups such as high-risk populations), outcome confirmation, exposure mesurement and assessment, and control for confounding factors [ 11 ]. The quality score for each selected study was calculated by summing the scores across these four domains.
Statistical methods
The estimates for our primary indicators of this work are mean RRs across a range of exposures, BRPFs, ROSs and star ratings for each risk-outcome pair. And the exposure unit was standardized to grams of consumption per day before synthesis. For each study that reported means or quantiles consumption rather than ranges of whole grain consumption, midpoint of defined quantile as the cutoff for intake intervals was used [ 10 , 19 , 20 , 21 , 22 , 23 , 24 , 25 , 26 , 27 , 28 , 29 ]. When the quantile dose range didn’t have a specific endpoint, and mean and standard deviation weren’t available, we assumed a consumption level of 0 g per day as the lowest amount [ 22 , 24 , 25 , 28 , 30 , 31 , 32 , 33 ]. For the upper limit of consumption, we used the range from the closest quartile or tertile within the cohort. In addition, we used 30 g to evaluate one serving of whole grain consumption if the value of a serving was not stated [ 34 ].
Estimating the shape of relationships between whole grain consumption and four health outcomes
We firstly modeled the mean log-RR (a measure of effect size) curve with MR-BRT (a Bayesian meta-regression tool) developed at the Institute for Health Metrics and Evaluation (IHME) [ 13 , 35 ], and followed a uniform analysis procedure to select model specifications for all dietary risks, which is described by Zheng et al. [ 15 ]. For protective risk factors with hypothesis of monotonic deceresing, the final models were run applying quadratic splines with two internal knots and a linearity prior on the right tail [ 11 , 15 ]. However, for the J-shaped risk curve, we employed quadratic splines with three internal knots and a linearity prior on both the right and left tails, without a monotonic prior [ 11 ]. Besides, to avoid the influence of extreme data and reduce publication bias, we trimmed 10% of data for each outcome as outliers [ 11 ].
Following the GRADE approach, we created binary covariates based on the the extracted information about specific study characteristics to identify potential sources of systematic bias within our included datasets. A step-wise Lasso approach were applied to assess the significance of these bias covariates at a threshold of 0.05. If the bias covariates were found to be significant, they were selected for adjustment in the final log-RR model.
To evaluate and adjust between-study heterogeneity, we quantified common sources of bias across the selected covariates that were likely to cause bias. And we calculated 95% UIs for each mean risk curve both with between-study heterogeneity incorporated (a ‘conservative’ UI) and without between-study heterogeneity incorporated (a ‘conventional’ UI) based on the selected biased covariates. Only the UIs that include between-study heterogeneity are presented in our main results unless specified.
Based on the aforementioned models, we then adjusted the selected bias covariates to decrease the variation in model residuals arising from differences in study quality and analysis. However, we primarily applied empirical evidence to choose bias covariate related to potential publication or reporting biases, which may ignore some confounders. Thus, Egger’s regression was conducted to detect publication bias, which estimated correlation between the study residuals and standard deviation of the corresponding data points. Funnel plots of the residuals of the risk function and standard deviations were generated to inspect reporting bias visually. And P value was used to assess the statistical significance of a risk for publication and/or reporting bias.
Estimating the TMREL/minimum risk exposure level
To draw robust conclusions about health benefits of whole grain consumption, we calculated the theoretical minimum risk exposure level (TMREL) of all potential outcomes linked to consuming whole grains. TMREL aligns with real-world consumption patterns supported by the included data, enabling an estimation of the average risk associated with whole grain intake. For protective risk factors, the lower bound of TMREL is defined as the 85th percentile of the lower limit within the highest consumption range across all studies. whereas the upper bound of TMREL is determined as the 85th percentile of the midpoint within the highest consumption range across all studies [ 15 ].
Estimating BPRF value, risk-outcome score (ROS) and star rating
Using the mean RR curves that incorporated between-study heterogeneity into uncertainty estimate, we estimated the BPRF from a conservative risk function. The BPRF was defined as the 5th (for harmful) or 95th percentile risk curve that is closest to the null. Afterwards, we calculated the ROS, which was equivalent to the mean log-BPRF averaged value over the 15th and 85th percentiles of the distribution of whole grain consumption. This value can give conservative interpretations regarding the association between whole grain consumption and four health outcomes [ 15 , 36 ]. Then, the ROSs of risk-outcome pairs were converted into a comparison across risk-outcome pairs and a star rating (from one to five) was assigned based on the quantitative assessment of the association, where a one-star rating indicating a non-significant relationship based on the conservative interpretation, two-star through five-star ratings implying a decrease in risk with average exposure (compared to no exposure). And the ranges of ROS in 0–0.1398 stands for two-star pairs, > 0.1398–0.4055 for three-star pairs, > 0.4055–0.6152 for four-star pairs and greater than 0.6152 for five-star pairs for protective risks [ 11 ].
Sensitivity analyses
To strengthen our estimates on the association between whole grain intake and four health outcomes and reduce the impact of outliers, we used trimming analysis with the Least Trimmed Squares (LTS) method. This method automatically identifies and removes outliers within the model’s likelihood. In our study, we trimmed the top 10% of data points that deviated the most from the expected dose-response curve as part of sensitivity analysis [ 11 , 35 ].
Dose-response analysis on whole grain consumption and four health outcomes was conducted by applying MR-BRT tool which included several Python packages (limetr 0.0.5, mrtool 0.0.1, IPOPT 1.2.0). And we executed BPRF analysis in Visual Studio Code with extensions of R version 4.2.1 and Python 3.9.0.
Study identification
A total of 3118 articles were found using search strings, and of those we identified 28 population-based prospective cohort studies, presenting a total of 184 estimates of effect sizes for associations between whole grain consumption and the four included health outcomes [ 10 , 25 , 26 , 28 , 29 , 32 , 33 ]. Details of the literature search are shown in Supplementary Fig. 1 . Eleven studies were from the United States, including data mainly from the HPFS, NHS, NHSII, the ATBC cohort, and the Cancer Prevention Study‑II Nutrition Cohort [ 10 , 20 , 21 , 27 , 28 , 29 , 32 , 38 , 39 , 41 , 42 ]. Fifteen of 28 studies were from the European population [ 19 , 22 , 23 , 24 , 25 , 26 , 30 , 33 , 25 , 43 , 44 , 45 , 46 , 47 ]. Besides, one study (the PURE cohort) collected information covering 21 countries (including the regions of North America and Europe, South America, Africa, the Middle East, South Asia, South East Asia, and China) [ 48 ] and one study reported the role of whole grain consumption on IHD in Chinese population [ 40 ]. Detailed information about the included cohorts is displayed in Supplementary Table 3 .
Characteristics of included studies
Of 28 included publications in the BPRF analysis, a total of eight studies investigated the association between whole grains and T2D [ 10 , 28 , 29 , 32 , 33 , 37 , 46 , 47 ], seven for CRC [ 19 , 20 , 21 , 30 , 42 , 43 , 44 ], six for both IHD and stroke [ 23 , 26 , 39 , 40 , 41 , 48 ], three for IHD [ 25 , 38 , 45 ], three for stroke [ 24 , 27 , 49 ], and one for IHD, stroke and CRC [ 22 ]. The median follow-up time of all included studies was 13.5 years (range: 6–25.8 years).
All included publications used dietary records or recalls, or food frequency questionnaires to collect data regarding whole grain intake. In total, seventeen publications used baseline data of whole grain intake in their analysis (single measurement) [ 19 , 23 , 24 , 25 , 26 , 30 , 33 , 39 , 25 , 43 , 44 , 45 , 48 ], whereas ten considered the average whole grain intake throughout the follow-up (i.e., based on multiple measurements) as the main exposure [ 10 , 20 , 21 , 22 , 27 , 37 , 38 , 40 , 41 , 42 ]. Three studies took self-report records to assess outcomes [ 28 , 29 , 32 ], and 25 studies used administrative medical records [ 10 , 19 , 21 , 22 , 25 , 26 , 27 , 30 , 33 , 37 , 41 , 43 , 44 , 49 ]. Three studies used mortality as the endpoint [ 26 , 39 , 43 ], and the rest studies considered incidence as the endpoint. 8 studies reported effect sizes with RRs [ 19 , 20 , 24 , 26 , 30 , 32 , 33 , 49 ], seventeen studies reported HRs [ 22 , 23 , 25 , 27 , 37 , 38 , 39 , 40 , 41 , 48 ], one study reported ORs [ 46 ], and one study reported incidence rate ratios (IRRs) [ 44 ]. The detailed information is presented in Supplementary Tables 4 – 7 .
Estimation of the shape of whole grains with T2D, CRC, IHD and stroke
Using BPRF methodology, our analyses revealed a correlation between higher whole grain intake and a reduced risk across all the outcomes considered. Figures 1 , 2 , 3 and 4 depict the BPRF curves for each risk-outcome pair, while Table 1 presents the results of the dose-response analysis.
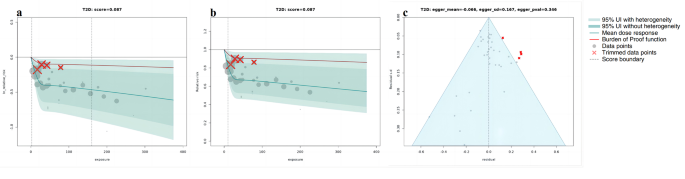
BPRF analysis on the association between whole grain consumption and T2D. a, log RR function. b, RR function. c, modified funnel plot showing the residuals (relative to zero) on the x-axis and the estimated s.d. that includes reported s.d. and between-study heterogeneity on the y-axis
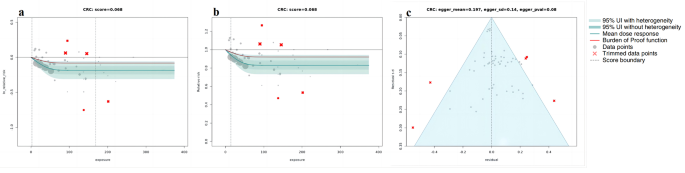
BPRF analysis on the association between whole grain consumption and CRC. a, log RR function. b, RR function. c, modified funnel plot showing the residuals (relative to zero) on the x-axis and the estimated s.d. that includes reported s.d. and between-study heterogeneity on the y-axis
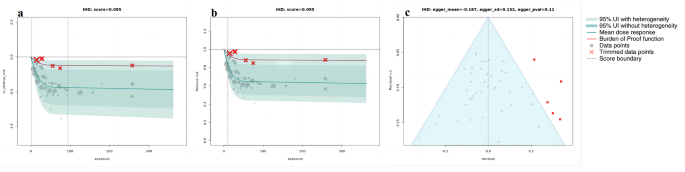
BPRF analysis on the association between whole grain consumption and IHD. a, log RR function. b, RR function. c, modified funnel plot showing the residuals (relative to zero) on the x-axis and the estimated s.d. that includes reported s.d. and between-study heterogeneity on the y-axis
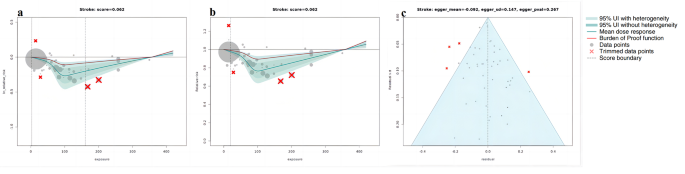
BPRF analysis on the association between whole grain consumption and stroke. a, log RR function. b, RR function. c, modified funnel plot showing the residuals (relative to zero) on the x-axis and the estimated s.d. that includes reported s.d. and between-study heterogeneity on the y-axis
Specifically, our analysis revealed that the associations between whole grain consumption and the risk of T2D, CRC and IHD all exhibited non-linear, monotonically decreasing trends (Figs. 1 , 2 and 3 ). In regard to T2D (Fig. 1 a and b), the sharpest decline in risk was noted at daily consumption of 50 g, with a reduction of 34.3% (95% UI including between-study heterogeneity: 5.3 to 55.7), compared to no whole grain consumption (at 0 g per day). Nonetheless, the reduction in risk tapered off to a mere 1.2% (0.2–1.6) when comparing a consumption level of 90 g per day to 50 g per day. With respect to CRC (Fig. 2 a and b), the largest reduction in CRC risk was identified when comparing the risk between an intake of 0 g per day and of 80 g per day, showcasing a noteworthy decline of 17.3%. we observed only marginal additional reductions in risk when consumption is beyond 80 g per day. As for IHD, the steepest decline of 32.1% (95% UI inclusive of between-study heterogeneity of 6.0 to 51.8) in IHD risk was observed when comparing risk between an intake of 0 g per day and of 30 g per day, with more modest marginal declines in IHD risk when consumption levels greater than 30 g per day (Fig. 3 a and b).
Different from the aforementioned results, a J -shape association was found between whole grain consumption and the risk of stroke (Fig. 4 a and b). The greatest reduction in stroke risk, observed at an intake of 100 g per day, was 24.6% (95% UI including between heterogeneity: 8.8 to 38.8). The mean risk of stroke at 60 g per day was 14.1% (7.0 to 21.3 including between-study heterogeneity) higher than at 100 g per day. And it was 1.5% (0.3 to 2.0) higher at 120 g per day compared to 100 g per day.
Additionally, the BPRF estimated ROSs for IHD, T2D, CRC and stroke of 0.095, 0.087, 0.068 and 0.062, respectively, which were applied to explore the average health benefits across the universe of whole grain consumption. Such estimates indicated that the consuming whole grains, on average, was related to a 9.9% decreased risk of IHD, a 9.1.% lower risk of T2D, a 7.0% lower risk of CRC and a 6.4% lower risk of stroke compared to a 0 g of whole grain intake. The star ratings of the four risk-outcome pairs all correspond to a two-star rating. After adjusting for between-study heterogeneity, the relationships still achieved statistical significance.
TMREL level of whole grain consumption
Based on observed exposure levels reported in the included studies, a TMREL of 118.5 g to 148.1 g per day, corresponding to approximately 4–5 servings per day, was estimated (detailed input information presented in Supplementary Table 8 ). Compared to TMREL (118.5–148.1 g), consuming no whole grains was associated with a 37.3% (5.8 to 59.5, inclusive of between-study heterogeneity) greater mean risk of T2D, a 17.3% (95% UI inclusive of between-study heterogeneity of 6.5 to 27.7) greater risk of CRC, a 36.9% (95% UI inclusive of between-study heterogeneity of 7.1 to 58.0) greater mean risk of IHD, and a 21.8% greater mean risk of stroke (95% UI inclusive of between-study heterogeneity of 7.3 to 35.1).
Sensitivity analysis and publication bias
The sensitivity analyses showed that trimming had significant effects on the ROS and reporting bias of the association between whole grain consumption and T2D and IHD. Without trimming, the ROS of T2D is -0.136, and a significant publication bias was detected using Egger’s regression ( P = 0.023, as shown in Supplementary Fig. 2 c). With respect to IHD, the results without trimming have reported a ROS of -0.273 and statistically significant evidence of small-study bias ( P for Egger’s regression = 0.028, Supplementary Fig. 3 c). However, both of the two health outcomes were found two significant study-level bias covariates (T2D: age of the population and outcome ascertainment methods; IHD: exposure measurement and outcome ascertainment methods). after removing outliers (T2D: 4 [ 31 , 50 ], IHD: 5 [ 24 , 50 ]) and adjusted for the selected bias covariates, no evidence of publication bias was observed (Figs. 1 c and 3 c). On the other hand, for CRC and stroke, trimming had a minor impact on the results. In both our analyses, with and without trimming, no significant evidence of publication bias was detected and no bias covariates were identified (Figs. 2 c and 4 c; and Supplementary Fig. 4 c, 5 c).
In this analysis, we applied a BPRF framework, which takes into account between-study heterogeneity, to quantify the association between whole grain consumption and four health outcomes. Our results suggested that increasing the intake of whole grains was significantly related to a reduction in the risk of CRC, T2D, IHD and stroke. When comparing TMREL (118.5–148.1 g per day) with a daily intake of 0 g of whole grains, the risk reductions for four diseases (T2D, CRC, IHD, and stroke) were 37.2%, 27.3%, 26.9%, and 21.8%, respectively. For all outcomes except stroke, we observed that mean risk exhibited a non-linear, monotonic decrease as whole grain consumption increased. However, the relationship between whole grains and stroke is like a J -shaped as the risk increased with exposure levels above or below a global minimum. Based on a conservative interpretation of available data (the averaged BPRF value), we found a slight decline in the risk of stroke, CRC, T2D, and IHD compared to no whole grain intake (by at least 6.4%, 7.0%, 9.1%, and 9.9%, respectively). The converted grade ratings of our evidence were all two-star ratings.
The protective role of whole grain consumption on CRC risk is well-documented. The World Cancer Research Fund/American Institute for Cancer Research (WCRF/AICR) has provided strong evidence on the protective role of whole grain consumption at 90 g/day on the risk of CRC (RR: 0.83; 95% CI: 0.79 to 0.89) [ 51 ]. Similarly, Schwingshackl et al. also reported an RR of 0.84 (95% CI: 0.78 to 0.90) of whole grain consumption at 90 g per day, and such evidence was rated as moderate according to the NutriGrade recommendation [ 5 ]. In consistent with previous studies, we found that consuming 90 g whole grains per day was also related with a similar reduction in the risk of CRC (RR: 0.83; 95% UI: 0.73 to 0.95), although the range of confidence interval was relatively wide (due to the consideration of between-study heterogeneity). Furthermore, as computing the mean across the universe of studies is appropriate to estimate the relationship between risk and outcome [ 52 ], we calculated the averaged BPRF value of 0.932, which is corresponding to a decline in the mean risk of CRC by 7.0%. Additionally, the star rating considering between-study heterogeneity of the whole grains-CRC pair is two-star. This estimate implied that the strength of the association evidence was relatively weak, in contrast to the WCRF/ARIC assessment, which categorized the evidence as “convincing” [ 11 ]. In fact, the extent of biases in nutritional epidemiological studies, including substantial residual confounding and selective reporting, can significantly impact the accuracy of health risks estimates related to the studied nutrients. Furthermore, the observational findings from prospective cohort studies exhibit considerable variation across different research endeavors [ 53 ]. Therefore, it is important to consider the strength of the association and employ a quantitative approach to assess consistency (that is, between-study heterogeneity) when evaluating evidence. Additionally, it is advisable to adopt a more conservative interpretation [ 54 ]. Our risk assessment indicated that increasing whole grain consumption can slightly reduce the risk of CRC, after correcting for biases due to factors such as study design, the representativeness of the study population, control for confounding, and so on.
With respect to IHD, the evidence stemming from previous meta-analyses has displayed a lack of consistency. For instance, Hu H et al. only found a linear association with 3 knots percentiles (25th, 50th, and 75th) selected [ 8 ]. However, Bechthold A, et al. provided evidence of a non-linear dose-response association ( P non-linearity <0.001) for IHD using three fixed knots at 10%, 50%, and 90% through the total distribution of the reported intake [ 7 ]. The disparities in their findings could be potentially due to variations in the selection of different knot placements along the estimated risk function curve, which might influence on the resulting accuracy of a spline approximation of a curve [ 55 ]. On the other hand, BPRF analysis, according to the given degree and number of knots, automatically sampled a set of knot placements for a feasible knot distribution, evaluated each resulting model by computing its fit and curvature, and then aggregated the final model as a weighted combination of the ensemble to mitigate the effect of spline parameter selection results and draw a robust conclusion. With this methodology, we found a non-linear, monotonic decline association between whole grain consumption and IHD.
In the case of stroke, previous meta-analyses generated mixed results. Bechthold et al. observed no association between whole grain intake and the risk of stroke in the non-linear dose-response analysis [ 7 ]. Conversely, Aune et al. observed a protective role of whole grain consumption on stroke risk, but this role was only significant in their non-linear dose-response analysis, and the risk curve exhibited a J -shaped pattern [ 6 ]. The difference between these studies might be partially attributable to different included studies [ 6 , 7 ]. Our analysis, including the results of newly published studies (the PURE study, China Kadoorie Biobank study and UK Biobank study) and applying BPRF methodology (free of log-linear hypothesis), found a J -shaped relationship between whole grain consumption and stroke, and we observed the greatest reduction in stroke risk observed at an intake of 100 g per day (RR: 0.75, 95%UI: 0.62 to 0.92). Unlike our analysis, both of the aforementioned studies assumed the association between whole grains and stroke to be log-linear [ 6 , 7 ], which might be inappropriate. A log-linear association implies that a fixed increment of health roles of whole grain consumption (for example, 30 g/day) remains constant across all levels of intake; however, an increase in consumption from 0 to 120 g/day would not have the same impact as an increase from 240 to 360 g/day, especially considering that excessive consumption may cause health issues such as overweight [ 56 ].
Our analyses support the need for stronger efforts and policies to encourage increased whole grain consumption as a means to reduce the risk of chronic diseases. Whole grains are well-known for their abundance of dietary fiber and nutrients. However, they can also be a notable source of food-borne contaminants. Nonetheless, current evidence suggests that increasing whole grain consumption could improve public health [ 57 ]. We estimated a TMREL of 118.1–148.5 g per day as the high consumption levels of whole grain intake in the real world, and such estimates are in line with the recommended intake of whole grains promoted by the GBD and the World Health Organization (WHO) [ 58 ], which is at least 125 g per day [ 59 ]. To address both individual and environmental health, the Lancet EAT Commission recommends a primarily plant-based diet, including 232 g of whole grains per day to reduce the carbon footprint of animal-based foods [ 60 ]. Nevertheless, our analysis solely took the individual-level health benefits into consideration, and the potential environmental benefits of increased whole grain consumption were not evaluated. Based on our analyses, particularly the notable protective roles observed with daily consumption of 100 g of whole grains against the risk of stroke, it seems that incorporating a minimum of three servings of whole grains per day has the potential to lower the risk of chronic diseases.
Our study employed BPRF methodology to estimate the association between whole grain consumption and four health outcomes. Compared to traditional meta-analysis methods, this method could quantify between-study heterogeneity, and infer flexible risk functions. It does so without imposing a log-linear hypothesis, which may exaggerate risks at higher exposure levels and overlook crucial details at lower exposure levels. With this methodology, we have found that the risk curves for whole grain consumption and IHD, CRC and T2D displayed decreasing marginal returns, indicating that as whole grain intake increases, the incremental health benefits of whole grains decrease. In addition, quantifications of between-study heterogeneity and corrections for biases due to study design in the methods can contribute to a conservative interpretation and a better understanding of the protective role of whole grain consumption in real-world settings. Thirdly, by estimating RRs associated with consuming whole grains at the TMREL (in correspondence to high real-world consumption levels), we were able to provide sufficient evidence to justify more robust efforts and policies promoting increased whole grain consumption to reduce chronic disease risk, especially with regard to CRC, T2D, IHD and stroke. In general, our analysis results indicate that improving whole grain consumption is beneficial toward enhancing public health.
Although the methodological framework addressed by Zheng et al. overcame many of the limitations in existing meta-analysis approaches, this study still has several limitations. Firstly, all studies included in our analysis were observational, and we were unable to definitively assess causality. Besides, this study mainly focused on total or whole grain consumption, and the impacts of different specific subtypes of whole grains on health outcomes may vary. For example, previous reviews have indicated that increasing whole-grain breakfast cereals, other than whole-grain bread, may decrease the risk of stroke [ 6 ]; furthermore, oats or oatmeal are linked to lower all-cause mortality but show no impact on T2D and CVD incidence [ 6 , 61 ]. Thus, further prospective cohort studies and randomized clinical trials focusing on different subtypes of whole grains and their associations with specific chronic diseases are required. Besides, most of the studies included were from the US and Europe, which limited the ability to make evidence-based recommendations, as dietary patterns can vary significantly between Asian and Western populations [ 62 ]. With respect to the Asian population, rather than whole grain consumption, most of studies investigated the role of refined grain consumption in the form of white rice and noodles [ 63 ], and further studies are needed to explore the association between whole grains and health outcomes on populations in Asia. Thirdly, the associations between whole grains and risks of different stroke types may be heterogeneous [ 24 ]. Unfortunately, we couldn’t investigate these associations separately due to a lack of reported data on stroke types in available studies.
In conclusion, the present study demonstrates that the consumption of whole grains plays a protective role in the risks of CRC, T2D, IHD and stroke, and the BPRF analysis, which did not rely on log-linear assumptions, revealed non-linear associations between whole grain intake and the four diseases of interests. The star ratings converted by ROSs for all four outcomes are all two stars, indicating that the associations between whole grain intake and CRC, T2D, IHD and stroke remain significant. The current body of evidence justifies the need for increased efforts and policies to promote higher whole grain consumption for the betterment of public health.
Data availability
No datasets were generated or analysed during the current study.
Cheng Z, Qiao D, Zhao S, Zhang B, Lin Q, Xie F. Whole grain rice: updated understanding of starch digestibility and the regulation of glucose and lipid metabolism. Compr Rev Food Sci Food Saf. 2022;21(4):3244–73.
Article CAS PubMed Google Scholar
Collaborators GBDRF. Global burden of 87 risk factors in 204 countries and territories, 1990–2019: a systematic analysis for the global burden of Disease Study 2019. Lancet. 2020;396(10258):1223–49.
Article Google Scholar
Reynolds A, Mann J, Cummings J, Winter N, Mete E, Te Morenga L. Carbohydrate quality and human health: a series of systematic reviews and meta-analyses. Lancet. 2019;393(10170):434–45.
Aune D, Norat T, Romundstad P, Vatten LJ. Whole grain and refined grain consumption and the risk of type 2 diabetes: a systematic review and dose-response meta-analysis of cohort studies. Eur J Epidemiol. 2013;28(11):845–58.
Schwingshackl L, Schwedhelm C, Hoffmann G, Knuppel S, Laure Preterre A, Iqbal K, et al. Food groups and risk of colorectal cancer. Int J Cancer. 2018;142(9):1748–58.
Aune D, Keum N, Giovannucci E, Fadnes LT, Boffetta P, Greenwood DC, et al. Whole grain consumption and risk of cardiovascular disease, cancer, and all cause and cause specific mortality: systematic review and dose-response meta-analysis of prospective studies. BMJ. 2016;353:i2716.
Article PubMed PubMed Central Google Scholar
Bechthold A, Boeing H, Schwedhelm C, Hoffmann G, Knuppel S, Iqbal K, et al. Food groups and risk of coronary heart disease, stroke and heart failure: a systematic review and dose-response meta-analysis of prospective studies. Crit Rev Food Sci Nutr. 2019;59(7):1071–90.
Hu H, Zhao Y, Feng Y, Yang X, Li Y, Wu Y, et al. Consumption of whole grains and refined grains and associated risk of cardiovascular disease events and all-cause mortality: a systematic review and dose-response meta-analysis of prospective cohort studies. Am J Clin Nutr. 2023;117(1):149–59.
Article PubMed Google Scholar
Dai X, Gil GF, Reitsma MB, Ahmad NS, Anderson JA, Bisignano C, et al. Health effects associated with smoking: a Burden of Proof study. Nat Med. 2022;28(10):2045–55.
Article CAS PubMed PubMed Central Google Scholar
Hu Y, Ding M, Sampson L, Willett WC, Manson JE, Wang M, et al. Intake of whole grain foods and risk of type 2 diabetes: results from three prospective cohort studies. BMJ. 2020;370:m2206.
Zheng P, Afshin A, Biryukov S, Bisignano C, Brauer M, Bryazka D, et al. The Burden of Proof studies: assessing the evidence of risk. Nat Med. 2022;28(10):2038–44.
Schwingshackl L, Knuppel S, Schwedhelm C, Hoffmann G, Missbach B, Stelmach-Mardas M, et al. Perspective: NutriGrade: a Scoring System to assess and judge the Meta-evidence of Randomized controlled trials and Cohort studies in Nutrition Research. Adv Nutr. 2016;7(6):994–1004.
Collaborators GBDCRF. The global burden of cancer attributable to risk factors, 2010-19: a systematic analysis for the global burden of Disease Study 2019. Lancet. 2022;400(10352):563–91.
Page MJ, McKenzie JE, Bossuyt PM, Boutron I, Hoffmann TC, Mulrow CD, et al. The PRISMA 2020 statement: an updated guideline for reporting systematic reviews. BMJ. 2021;372:n71.
Stanaway JD, Afshin A, Ashbaugh C, Bisignano C, Brauer M, Ferrara G, et al. Health effects associated with vegetable consumption: a Burden of Proof study. Nat Med. 2022;28(10):2066–74.
Lescinsky H, Afshin A, Ashbaugh C, Bisignano C, Brauer M, Ferrara G, et al. Health effects associated with consumption of unprocessed red meat: a Burden of Proof study. Nat Med. 2022;28(10):2075–82.
Siemieniuk R, Guyatt G. What is GRADE? BMJ Best Practice. 2021. https://bestpractice.bmj.com/info/us/toolkit/learn-ebm/what-is-grade/ .
The GRADE Working Group. GRADE handbook. 2013. https://gdt.gradepro.org/app/handbook/handbook.html .
Pietinen P, Malila N, Virtanen M, Hartman TJ, Tangrea JA, Albanes D, et al. Diet and risk of colorectal cancer in a cohort of Finnish men. Cancer Causes Control. 1999;10(5):387–96.
He X, Wu K, Zhang X, Nishihara R, Cao Y, Fuchs CS, et al. Dietary intake of fiber, whole grains and risk of colorectal cancer: an updated analysis according to food sources, tumor location and molecular subtypes in two large US cohorts. Int J Cancer. 2019;145(11):3040–51.
Hullings AG, Sinha R, Liao LM, Freedman ND, Graubard BI, Loftfield E. Whole grain and dietary fiber intake and risk of colorectal cancer in the NIH-AARP Diet and Health Study cohort. Am J Clin Nutr. 2020;112(3):603–12.
Thompson AS, Tresserra-Rimbau A, Karavasiloglou N, Jennings A, Cantwell M, Hill C, et al. Association of Healthful Plant-based Diet Adherence with Risk of Mortality and Major Chronic diseases among adults in the UK. JAMA Netw Open. 2023;6(3):e234714.
Sonestedt E, Hellstrand S, Schulz CA, Wallstrom P, Drake I, Ericson U, et al. The association between carbohydrate-rich foods and risk of cardiovascular disease is not modified by genetic susceptibility to dyslipidemia as determined by 80 validated variants. PLoS ONE. 2015;10(4):e0126104.
Tektonidis TG, Akesson A, Gigante B, Wolk A, Larsson SC. A Mediterranean diet and risk of myocardial infarction, heart failure and stroke: a population-based cohort study. Atherosclerosis. 2015;243(1):93–8.
Helnaes A, Kyro C, Andersen I, Lacoppidan S, Overvad K, Christensen J, et al. Intake of whole grains is associated with lower risk of myocardial infarction: the Danish Diet, Cancer and Health Cohort. Am J Clin Nutr. 2016;103(4):999–1007.
Johnsen NF, Frederiksen K, Christensen J, Skeie G, Lund E, Landberg R, et al. Whole-grain products and whole-grain types are associated with lower all-cause and cause-specific mortality in the scandinavian HELGA cohort. Br J Nutr. 2015;114(4):608–23.
Juan J, Liu G, Willett WC, Hu FB, Rexrode KM, Sun Q. Whole grain consumption and risk of ischemic stroke: results from 2 prospective cohort studies. Stroke. 2017;48(12):3203–9.
van Dam RM, Hu FB, Rosenberg L, Krishnan S, Palmer JR. Dietary calcium and magnesium, major food sources, and risk of type 2 diabetes in U.S. black women. Diabetes Care. 2006;29(10):2238–43.
Li J, Glenn AJ, Yang Q, Ding D, Zheng L, Bao W, et al. Dietary protein sources, mediating biomarkers, and incidence of type 2 diabetes: findings from the women’s Health Initiative and the UK Biobank. Diabetes Care. 2022;45(8):1742–53.
Larsson SC, Giovannucci E, Bergkvist L, Wolk A. Whole grain consumption and risk of colorectal cancer: a population-based cohort of 60,000 women. Br J Cancer. 2005;92(9):1803–7.
Wirstrom T, Hilding A, Gu HF, Ostenson CG, Bjorklund A. Consumption of whole grain reduces risk of deteriorating glucose tolerance, including progression to prediabetes. Am J Clin Nutr. 2013;97(1):179–87.
Meyer KA, Kushi LH, Jacobs DR Jr., Slavin J, Sellers TA, Folsom AR. Carbohydrates, dietary fiber, and incident type 2 diabetes in older women. Am J Clin Nutr. 2000;71(4):921–30.
Montonen J, Knekt P, Jarvinen R, Aromaa A, Reunanen A. Whole-grain and fiber intake and the incidence of type 2 diabetes. Am J Clin Nutr. 2003;77(3):622–9.
Aune D, Chan DS, Lau R, Vieira R, Greenwood DC, Kampman E, et al. Dietary fibre, whole grains, and risk of colorectal cancer: systematic review and dose-response meta-analysis of prospective studies. BMJ. 2011;343:d6617.
Zheng P, Barber R, Sorensen RJD, Murray CJL, Aravkin AY. Trimmed constrained mixed effects models: formulations and algorithms. J Comput Graphical Stat. 2021;30(3):544–56.
Akbaraly TN, Sabia S, Shipley MJ, Batty GD, Kivimaki M. Adherence to healthy dietary guidelines and future depressive symptoms: evidence for sex differentials in the Whitehall II study. Am J Clin Nutr. 2013;97(2):419–27.
Maukonen M, Harald K, Kaartinen NE, Tapanainen H, Albanes D, Eriksson J, et al. Partial substitution of red or processed meat with plant-based foods and the risk of type 2 diabetes. Sci Rep. 2023;13(1):5874.
Hu Y, Willett WC, Manson JAE, Rosner B, Hu FB, Sun Q. Intake of whole grain foods and risk of coronary heart disease in US men and women. BMC Med. 2022;20(1):192.
Jacobs DR Jr., Andersen LF, Blomhoff R. Whole-grain consumption is associated with a reduced risk of noncardiovascular, noncancer death attributed to inflammatory diseases in the Iowa women’s Health Study. Am J Clin Nutr. 2007;85(6):1606–14.
Yang J, Du H, Guo Y, Bian Z, Yu C, Chen Y, et al. Coarse Grain Consumption and Risk of Cardiometabolic diseases: a prospective cohort study of Chinese adults. J Nutr. 2022;152(6):1476–86.
Steffen LM, Jacobs DR Jr., Stevens J, Shahar E, Carithers T, Folsom AR. Associations of whole-grain, refined-grain, and fruit and vegetable consumption with risks of all-cause mortality and incident coronary artery disease and ischemic stroke: the atherosclerosis risk in communities (ARIC) Study. Am J Clin Nutr. 2003;78(3):383–90.
Um CY, Campbell PT, Carter B, Wang Y, Gapstur SM, McCullough ML. Association between grains, gluten and the risk of colorectal cancer in the Cancer Prevention Study-II Nutrition Cohort. Eur J Nutr. 2020;59(4):1739–49.
Skeie G, Braaten T, Olsen A, Kyro C, Tjonneland A, Nilsson LM, et al. Whole grain intake and survival among scandinavian colorectal cancer patients. Nutr Cancer. 2014;66(1):6–13.
Egeberg R, Olsen A, Loft S, Christensen J, Johnsen NF, Overvad K, et al. Intake of wholegrain products and risk of colorectal cancers in the Diet, Cancer and Health cohort study. Br J Cancer. 2010;103(5):730–4.
Rautiainen S, Levitan EB, Orsini N, Åkesson A, Morgenstern R, Mittleman MA, et al. Total antioxidant capacity from diet and risk of myocardial infarction: a prospective cohort of women. Am J Med. 2012;125(10):974–80.
Wirström T, Hilding A, Gu HF, Östenson CG, Björklund A. Consumption of whole grain reduces risk of deteriorating glucose tolerance, including progression to prediabetes. Am J Clin Nutr. 2013;97(1):179–87.
Kyrø C, Tjønneland A, Overvad K, Olsen A, Landberg R. Higher whole-grain intake is Associated with Lower Risk of type 2 diabetes among Middle-aged men and women: the Danish Diet, Cancer, and Health Cohort. J Nutr. 2018;148(9):1434–44.
Swaminathan S, Dehghan M, Raj JM, Thomas T, Rangarajan S, Jenkins D, et al. Associations of cereal grains intake with cardiovascular disease and mortality across 21 countries in Prospective Urban and rural epidemiology study: prospective cohort study. BMJ. 2021;372:m4948.
Mizrahi A, Knekt P, Montonen J, Laaksonen MA, Heliovaara M, Jarvinen R. Plant foods and the risk of cerebrovascular diseases: a potential protection of fruit consumption. Br J Nutr. 2009;102(7):1075–83.
Kyro C, Tjonneland A, Overvad K, Olsen A, Landberg R. Higher whole-grain intake is Associated with Lower Risk of type 2 diabetes among Middle-aged men and women: the Danish Diet, Cancer, and Health Cohort. J Nutr. 2018;148(9):1434–44.
Vieira AR, Abar L, Chan DSM, Vingeliene S, Polemiti E, Stevens C, et al. Foods and beverages and colorectal cancer risk: a systematic review and meta-analysis of cohort studies, an update of the evidence of the WCRF-AICR continuous Update Project. Ann Oncol. 2017;28(8):1788–802.
Murray CJ, Ezzati M, Lopez AD, Rodgers A, Vander Hoorn S. Comparative quantification of health risks conceptual framework and methodological issues. Popul Health Metr. 2003;1(1):1.
Trepanowski JF, Ioannidis JPA, Perspective. Limiting dependence on Nonrandomized studies and improving randomized trials in Human Nutrition Research: why and how. Adv Nutr. 2018;9(4):367–77.
Ioannidis JPA. The challenge of reforming nutritional epidemiologic research. JAMA. 2018;320(10):969–70.
Yeh R, Nashed YSG, Peterka T, Tricoche X. Fast automatic knot Placement Method for Accurate B-spline curve fitting. Comput Aided Des. 2020;128.
San-Cristobal R, Navas-Carretero S, Martinez-Gonzalez MA, Ordovas JM, Martinez JA. Contribution of macronutrients to obesity: implications for precision nutrition. Nat Rev Endocrinol. 2020;16(6):305–20.
Thielecke F, Nugent AP. Contaminants in Grain-A Major Risk for Whole Grain Safety? Nutrients. 2018;10(9).
World Health Organization. Diet, nutrition and the prevention of chronic diseases: Report of a joint WHO/FAO expert consultation. 2018.
Collaborators GBD. Health effects of dietary risks in 195 countries, 1990–2017: a systematic analysis for the global burden of Disease Study 2017. Lancet. 2019;393(10184):1958–72.
Willett W, Rockstrom J, Loken B, Springmann M, Lang T, Vermeulen S, et al. Food in the Anthropocene: the EAT-Lancet Commission on healthy diets from sustainable food systems. Lancet. 2019;393(10170):447–92.
Wehrli F, Taneri PE, Bano A, Bally L, Blekkenhorst LC, Bussler W et al. Oat intake and risk of type 2 diabetes, Cardiovascular Disease and all-cause mortality: a systematic review and Meta-analysis. Nutrients. 2021;13(8).
Zhou BF, Stamler J, Dennis B, Moag-Stahlberg A, Okuda N, Robertson C, et al. Nutrient intakes of middle-aged men and women in China, Japan, United Kingdom, and United States in the late 1990s: the INTERMAP study. J Hum Hypertens. 2003;17(9):623–30.
Muthayya S, Sugimoto JD, Montgomery S, Maberly GF. An overview of global rice production, supply, trade, and consumption. Ann N Y Acad Sci. 2014;1324:7–14.
Download references
This study was supported by the Key Discipline of Zhejiang Province in Public Health and Preventative Medicine (First Class, Category A), Hangzhou Medical College.
Author information
Jing Wang and Yingjun Li contributed equally to this work.
Authors and Affiliations
Department of Epidemiology and Health Statistics, School of Public Health, Hangzhou Medical College, 481 Binwen Road, Hangzhou, 310053, China
Houpu Liu, Jiahao Zhu, Rui Gao, Lilu Ding, Ye Yang, Wenxia Zhao, Jing Wang & Yingjun Li
Department of Radiology, Key Laboratory of Cancer Prevention and Therapy, Tianjin Medical University Cancer Institute & Hospital, National Clinical Research Center for Cancer, Tianjin, China
Xiaonan Cui
Department of Epidemiology and Health Statistics, School of Public health, Tianjin Medical University, Tianjin, China
You can also search for this author in PubMed Google Scholar
Contributions
All authors contributed to the study’s conception and design. Liu Houpu, Wang, Jing, and Li, Yingjun conceived the study, searched the literature and performed data extraction. Liu, Houpu and Zhu, Jiahao had the idea for the article, performed the main analysis and written the first manuscript. Gao Rui, Yang Ye and Zhao Wenxia conducted the sensitivity analysis and inspected the analysis results. Ding Lilu, Cui, Xiaonan, and Lu, Wenli provided statistical expertise. Wang, Jing and Li, Yingjun reviewed the original manuscript and critically revised the work. All authors commented on previous versions of the manuscript. All authors read and approved the final manuscript.
Corresponding authors
Correspondence to Jing Wang or Yingjun Li .
Ethics declarations
Ethics approval and consent to participate.
Not applicable.
Consent for publication
Patient and public involvement, competing interests.
The authors declare no competing interests.
Additional information
Publisher’s note.
Springer Nature remains neutral with regard to jurisdictional claims in published maps and institutional affiliations.
Electronic supplementary material
Below is the link to the electronic supplementary material.
Supplementary Material 1
Rights and permissions.
Open Access This article is licensed under a Creative Commons Attribution 4.0 International License, which permits use, sharing, adaptation, distribution and reproduction in any medium or format, as long as you give appropriate credit to the original author(s) and the source, provide a link to the Creative Commons licence, and indicate if changes were made. The images or other third party material in this article are included in the article’s Creative Commons licence, unless indicated otherwise in a credit line to the material. If material is not included in the article’s Creative Commons licence and your intended use is not permitted by statutory regulation or exceeds the permitted use, you will need to obtain permission directly from the copyright holder. To view a copy of this licence, visit http://creativecommons.org/licenses/by/4.0/ . The Creative Commons Public Domain Dedication waiver ( http://creativecommons.org/publicdomain/zero/1.0/ ) applies to the data made available in this article, unless otherwise stated in a credit line to the data.
Reprints and permissions
About this article
Cite this article.
Liu, H., Zhu, J., Gao, R. et al. Estimating effects of whole grain consumption on type 2 diabetes, colorectal cancer and cardiovascular disease: a burden of proof study. Nutr J 23 , 49 (2024). https://doi.org/10.1186/s12937-024-00957-x
Download citation
Received : 13 December 2023
Accepted : 07 May 2024
Published : 14 May 2024
DOI : https://doi.org/10.1186/s12937-024-00957-x
Share this article
Anyone you share the following link with will be able to read this content:
Sorry, a shareable link is not currently available for this article.
Provided by the Springer Nature SharedIt content-sharing initiative
- Whole grains
- Bayes Theorem
- Cardiovascular diseases
- Diabetes Mellitus, type 2
- Colorectal neoplasms
Nutrition Journal
ISSN: 1475-2891
- General enquiries: [email protected]
- Open access
- Published: 14 May 2024
The relationship between diabetes and the dementia risk: a meta-analysis
- Fang Cao 1 ,
- Fushuang Yang 2 ,
- Jian Li 2 ,
- Wei Guo 2 ,
- Chongheng Zhang 2 ,
- Xinxin Sun 3 ,
- Yi Zhou 4 &
- Wenfeng Zhang 1
Diabetology & Metabolic Syndrome volume 16 , Article number: 101 ( 2024 ) Cite this article
191 Accesses
Metrics details
The link between diabetes and dementia risk is not well understood. This study evaluates the factors linking diabetes to dementia onset, providing guidance for preventing dementia in diabetic patients.
This analysis utilized databases such as PubMed, Embase, Web of Science, and the Cochrane Library to review literature from January 31, 2012, to March 5, 2023. Articles were rigorously assessed using specific inclusion and exclusion criteria. The Newcastle-Ottawa Scale (NOS) was used to evaluate the quality of the studies. Data analysis was performed with STATA 15.0.
The study analyzed 15 articles, covering 10,103,868 patients, with 8,821,516 diagnosed with diabetes. The meta-analysis reveals a substantial association between diabetes and an increased risk of dementia [RR: 1.59, 95%CI (1.40–1.80), P < 0.01, I² =96.4%]. A diabetes duration of less than five years is linked to a higher dementia risk [RR: 1.29, 95%CI (1.20–1.39), P < 0.01, I² =92.6%]. Additionally, hypoglycemia significantly raises dementia risk [RR: 1.56, 95%CI (1.13–2.16), P < 0.01, I² =51.5%]. Analyses of blood sugar control, glycated hemoglobin, and fasting blood sugar indicated no significant effects on the onset of dementia.
Diabetes notably increases dementia risk, particularly where diabetes duration is under five years or hypoglycemia is present.
Registration
The research protocol was registered with PROSPERO and assigned the registration number CRD42023394942.
Introduction
Diabetes, a prevalent chronic metabolic disorder, is marked by elevated blood sugar levels from hypoinsulinism or insulin resistance [ 1 ]. The International Diabetes Federation (IDF) highlights a significant increase in global diabetes cases—from 463 million in 2019 to nearly 537 million by 2021.The prevalence is projected to rise by 46% by 2045, especially in developing regions like India and China [ 2 , 3 ]. Chronic high blood sugar may degrade vital organs, including nerves, blood vessels, the heart, kidneys, and the retina, markedly lowering life quality and posing substantial health risks [ 4 ]. Experimental research indicates that high blood sugar can trigger reactive oxygen species, leading to neuroinflammation and significant neuronal losses in areas like the hippocampus, thereby impairing cognitive functions and potentially resulting in dementia [ 5 , 6 , 7 , 8 ]. Systematic reviews suggest that diabetes heightens the risk of progressing from cognitive impairments to dementia [ 9 ].
Dementia, a severe neurodegenerative condition, drastically affects the ability to conduct daily activities. According to the World Health Organization, over 550,000 people globally are diagnosed with dementia annually, with nearly 100,000 new cases each year; more than 60% of these cases occur in low and middle-income countries [ 10 ]. Age is a primary factor in the onset of dementia, and diabetes significantly exacerbates damage to nerves and blood vessels, thus increasing dementia risk. A 2013 meta-analysis by Kapil Gudala et al. revealed that diabetic patients have a 73% higher risk of dementia compared to non-diabetics, including a 56% increased risk of Alzheimer’s Disease (AD) [ 11 ]. Subsequent analyses confirm that diabetes doubles the risk of all-cause dementia, elevating the risks for both vascular dementia and AD [ 12 ]. Earlier studies have shown similar trends, with diabetic individuals facing up to twice the risk of developing all forms of dementia, including increases in AD and vascular dementia risks [ 13 ]. Reviews have consistently indicated that diabetes, along with hypertension, dyslipidemia, and obesity, is a significant predictor of dementia in those aged over 65 [ 14 ].
To examine if the risk relationship between diabetes and dementia has evolved recently, a comprehensive systematic review and meta-analysis were conducted. This research, spanning studies from 2013 to 2023, builds upon the 2013 meta-analysis by Kapil Gudala et al. Its purpose is to quantify the association between diabetes and dementia, evaluate factors that might influence this relationship, and compare current findings with the 2013 results to identify any changes in the risk associated with diabetes and dementia over the last decade.
Materials and methods” section
Literature search.
A literature search was conducted across PubMed, Embase, Web of Science, and the Cochrane Library from January 31, 2012, to March 5, 2023. The search focused on combining “Diabetes Mellitus” with “Dementia.” Details on search strategies are available in the supplementary files.
Inclusion and exclusion criteria
Inclusion criteria.
(1) Studies with adult participants. (2) Research examining the relationship between diabetes and dementia risk. (3) Studies employing cohort, cross-sectional, and other observational methodologies.
Exclusion criteria
(1) Papers discussing only dementia or diabetes without addressing the risk relationship between the two. (2) Animal studies or cellular research. (3) Reviews, conference articles, guidelines, and other non-original research. (4) Non-English publications. (5) Studies with fewer than 30 participants, due to limited generalizability.
Data extraction
Data extraction was independently performed by two authors (YFS and GW) who initially screened titles and abstracts to eliminate unsuitable studies. Full texts of potentially relevant studies were then thoroughly reviewed for final inclusion. Any disagreements were resolved by consulting a third author (LJ).
Both YFS and GW separately collected and verified data using a standardized format. Disputed articles were referred to the third author (LJ) for final adjudication. Extracted data included the author, publication years, study designs, locations, sample sizes, demographic details of participants (age, gender, race), follow-up periods, and diagnostic criteria for diabetes and dementia.
Quality assessment
The Newcastle-Ottawa Scale (NOS) was utilized to evaluate the quality of the included studies. This tool assesses three dimensions across eight criteria, with comparability scoring up to 2 points and the other criteria scoring 1 point each. Studies with scores from 7 to 9 were considered high quality, while those scoring 4 to 6 were deemed moderate quality. Two evaluators (YFS and GW) independently rated each study and cross-checked their assessments. Any discrepancies were resolved through consultation with a third researcher (LJ).
Data processing and statistical analysis
To assess the impact of diabetes on the risk of developing dementia, this study used diabetes as the primary outcome. Additional measures encompassed duration of diabetes, blood sugar control factors, hypoglycemia, glycated hemoglobin, and fasting blood glucose.
Statistical processing was performed using Stata 15.0 and R 4.2.1. Heterogeneity was assessed quantitatively with Cochran’s Q test and Higgins I² , employing a random-effects model for significant heterogeneity ( P < 0.10 or I² > 50%). Otherwise, a fixed-effects model was used. In cases of high heterogeneity, sensitivity and subgroup analyses were conducted to identify sources. Publication bias was visually inspected using funnel plots and statistically tested with Egger’s and Begg’s tests. The trim-and-fill method was applied to adjust for detected publication biases. A significance level of P < 0.05 was used to determine statistical relevance of the findings.
Literature search results
A systematic search across PubMed, Embase, Web of Science, and the Cochrane Library yielded 5,210 articles. After removing 827 duplicates, 4,282 articles were excluded during initial screening because their titles and abstracts did not meet the inclusion criteria. This process shortlisted 101 articles for detailed assessment. Upon full-text review, 86 articles were excluded for reasons including irrelevance to the study’s focus on diabetes and dementia, being review articles, or containing duplicate data. Ultimately, 15 articles qualified for inclusion in the analysis. The selection process is depicted in Fig. 1 .
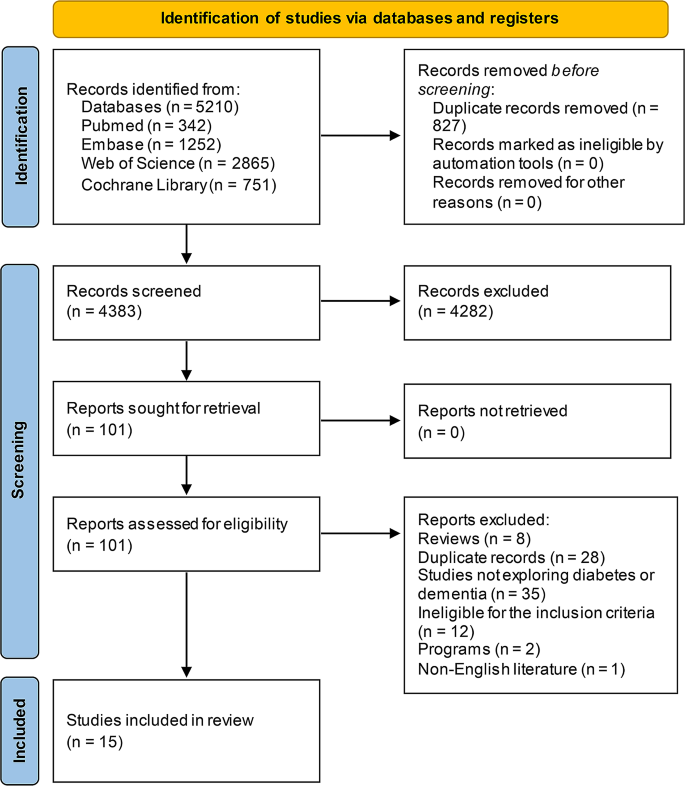
Literature search and selection procedure
Characteristics of the studies
This analysis included 15 articles [ 15 , 16 , 17 , 18 , 19 , 20 , 21 , 22 , 23 , 24 , 25 , 26 , 27 , 28 , 29 ], comprising 12 cohort and three cross-sectional studies. These articles, published between 2012 and 2023, investigated the relationship between diabetes and dementia risk. Most studies focused on Type 2 diabetes, although one specifically addressed Type 1 diabetes [ 17 ]. Six studies did not specify diabetes types [ 16 , 18 , 21 , 22 , 25 , 28 ]. Together, these studies accounted for 10,103,868 participants, with 8,821,516 diagnosed with diabetes. Of these, 29,619 cases of dementia were documented, though not all articles provided specific figures for statistical inclusion. Follow-up periods ranged from 0 to 32 years.
Diagnosis of dementia predominantly used International Disease Classification codes (ICD-9, ICD-10), with some studies [ 18 , 27 ] utilizing the Diagnostic and Statistical Manual of Mental Disorders (DSM-IV) or regional standards like the Korean Classification of Diseases (KCD-6) [ 20 ]. Diabetes diagnoses were primarily based on blood sugar levels, although some studies [ 24 ] also used patient registration and prescription records. Detailed demographic information and diagnostic criteria are presented in Table 1 .
A quality assessment using the NOS showed that the overall quality of the studies was high, with specific quality scores detailed in Table 1 .
Primary outcome measures and group analysis relationship between diabetes and dementia onset
Among the 15 studies analyzed, 9 [ 16 , 17 , 19 , 21 , 22 , 23 , 24 , 26 , 28 ] focused on the relationship between diabetes and dementia onset. These studies showed significant heterogeneity ( P < 0.01, I² = 96.4%), leading to the use of a random-effects model. Results demonstrated a significantly increased risk of dementia in diabetic patients [RR: 1.59, 95%CI (1.40–1.80), P < 0.01], as shown in Fig. 2 . A comparison with a 2013 meta-analysis, which reported a RR of 1.73 for All Types of Dementia (ATD) in diabetics [95%CI 1.65–1.82, P < 0.01], indicated consistent findings. The combined data from the current and 2013 studies reaffirmed diabetes as a significant dementia risk factor [RR: 1.62, 95%CI (1.43–1.83), P < 0.01], with persistent high heterogeneity ( P < 0.01, I² = 97.1%), depicted in Fig. 3 .
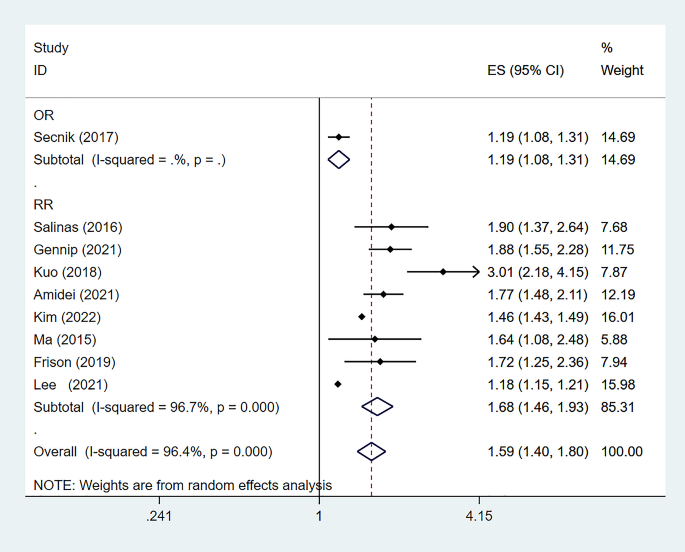
Risk of dementia in diabetes, CI (confidence interval)
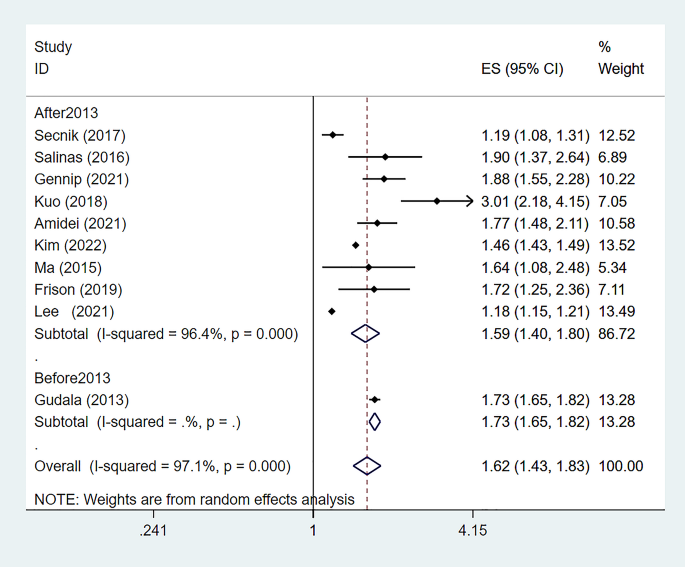
Risk of dementia in diabetes compared with 2013 study data, CI
Further analysis assessed the impact of various factors on dementia onset, including diabetes duration in three studies [ 16 , 26 , 28 ], diabetes control in two studies [ 23 , 27 ], hypoglycemic events in four studies [ 15 , 20 , 23 , 29 ], glycosylated hemoglobin in three studies [ 15 , 21 , 27 ], and fasting blood glucose in two studies [ 23 , 26 ], with details presented in Table 2 . Notable heterogeneity was observed in associations for diabetes duration of less than five years ( I² = 92.6%), hypoglycemic events ( I² = 51.5%), glycosylated hemoglobin ( I² = 69.5%), and fasting blood glucose ( I² = 70.7%), requiring the application of a random-effects model. Conversely, the analysis of diabetes control factors showed minimal heterogeneity ( P = 0.862, I² = 0.0%), allowing for a fixed-effects model.
Analysis from three articles indicated that shorter durations of diabetes are associated with an increased risk of dementia. Specifically, patients with diabetes for less than five years exhibited a 29% higher risk of dementia compared to their non-diabetic counterparts [RR: 1.29, 95%CI (1.20–1.39), P < 0.01], as shown in Supplementary Fig. 1 . Four studies highlighted a significant relationship between hypoglycemic events and the onset of dementia. Patients experiencing hypoglycemic events faced a 56% increased risk of dementia [RR: 1.56, 95%CI (1.13–2.16), P = 0.007], presented in Supplementary Fig. 2 .
The meta-analysis revealed a low correlation between dementia onset and diabetes management factors such as blood glucose control, glycosylated hemoglobin, and fasting blood glucose. No statistically significant differences were observed in dementia onset between patients with and without managed blood sugar levels [RR: 1.00, 95%CI (0.90–1.11), P = 0.988], outlined in Supplementary Fig. 3 . A minimal association was found between glycosylated hemoglobin levels and increased dementia risk [RR: 1.04, 95%CI (1.00-1.09), P = 0.052], as illustrated in Supplementary Fig. 4 . Similarly, fasting blood glucose levels suggested a 37% increase in dementia risk, although this was not statistically significant [RR: 1.37, 95%CI (0.78–2.41), P = 0.275], as detailed in Supplementary Fig. 5 .
Sensitivity analysis
Sensitivity analysis was conducted to assess the stability of the findings related to the link between diabetes and dementia onset. By sequentially excluding each study, the results consistently fell within the range of the overall effect value, indicating that the conclusions are robust and stable. These details are shown in Fig. 4 .
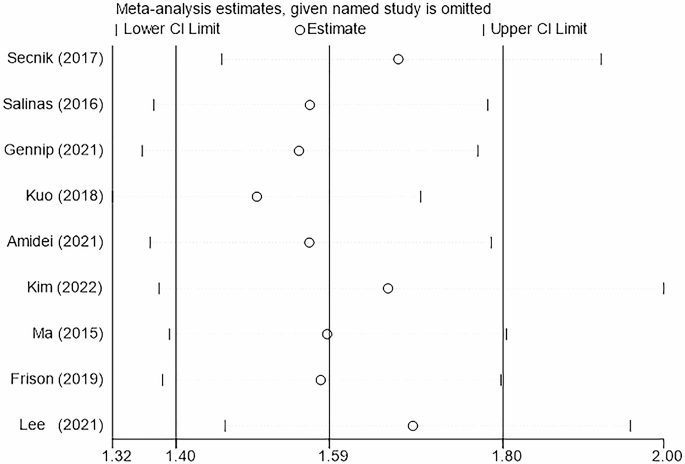
Publication Bias
Publication bias was assessed visually using a funnel plot and statistically through Begg’s and Egger’s tests. The results of these tests showed values of P Begg = 1.00 and P Egger = 0.429, both above the threshold of 0.05, suggesting no significant publication bias. This analysis is illustrated in Supplementary Fig. 6 .
This meta-analysis evaluated the results of 15 observational studies and discovered that diabetic patients have a 59% increased risk of developing dementia compared to non-diabetics. This finding is consistent with a 2013 meta-analysis (RR: 1.73, 95%CI: 1.65–1.82).
Research has investigated the connection between diabetes and dementia. Leibson and colleagues found in their meta-analysis that among 1,455 adult diabetic patients tracked for 15 years, 101 developed dementia and 77 developed AD, indicating a 66% increased risk [ 30 ]. Similarly, Salinas et al. observed that the risk of dementia in Mexican patients with type 2 diabetes was double that of non-diabetics [ 23 ]. This aligns with results from a large prospective cohort study in Rotterdam, the Netherlands, which noted that diabetes nearly doubles the risk of dementia and AD [ 31 ]. A comprehensive review of 243 prospective studies and 153 randomized control trials identified diabetes as a significant risk factor, increasing the risk of AD by 69% [ 32 ]. Additionally, another study indicated that diabetic patients have a 1.25 to 1.91 times higher risk of cognitive impairment and dementia, with increased risks manifesting even in the early stages of diabetes [ 33 ].
These studies collectively support the robust link between diabetes and the onset of dementia, highlighting that this association may begin as early as within the first five years of diabetes. The underlying mechanisms of this relationship, however, remain to be fully elucidated.
Biological studies have shown that diabetes-related complications such as insulin resistance, acute fluctuations in blood glucose, changes in blood flow rate, and chronic inflammation may increase the risk of dementia. Research involving diabetic animals has demonstrated that diminished granule cell proliferation in the hippocampal areas CA1 and CA3 results in neuronal apoptosis and, consequently, dementia. Diabetes is also associated with brain damage, manifesting as cerebral atrophy, altered brain vasculature, impaired synaptic plasticity, and neuroglial cell dysfunction, all of which compromise brain function [ 34 , 35 , 36 ].
Imaging and neuropathological studies indicate that the brains of diabetic patients may undergo changes akin to those seen in early-stage AD patients [ 12 , 37 ]. AD is characterized by the accumulation of amyloid-beta (Aβ) proteins, hyperphosphorylation of Tau proteins, and the formation of neurofibrillary tangles, all contributing to the onset of dementia [ 38 ].
Recent studies confirm that dementia and cognitive impairments are significant complications associated with Type 2 diabetes (T2D) [ 39 ]. With the increasing prevalence of T2D and challenges in self-management among patients, the incidence of dementia is expected to rise [ 40 ]. Although the effect of diabetes duration on dementia onset requires further study. The American ARIC study indicated that a longer duration of diabetes correlates with an increased risk of developing dementia [ 41 ]. Findings suggest that cognitive function declines sharply within the first few years after diabetes onset, and this decline is not significantly influenced by age [ 42 , 43 ]. Likely, our research reveals a 29% increased risk of dementia when the duration of diabetes is less than five years, indicating that the impact of diabetes on dementia may begin early in the disease process. This is in line with a UK prospective study, which has identified a clear linear relationship between the duration of diabetes, blood sugar control, and the onset of dementia, showing a 15% increase in dementia incidence for every five years of diabetes duration [ 44 ]. Similarly, a German prospective study found higher rates of dementia diagnosis in the early stages of diabetes, consistent with our findings, suggesting that cognitive impairment may start even before diabetes is clinically diagnosed due to prolonged abnormal blood sugar levels [ 45 ].
Our research indicates that hypoglycemic events significantly escalate the risk of dementia among diabetic patients. Hypoglycemia, a common acute complication of diabetes, can cause substantial vascular damage in the brain, leading to neuronal injury and contributing to cognitive impairments [ 20 , 46 , 47 ]. Cohort studies reveal that diabetic patients with severe hypoglycemia are at an increased risk of developing dementia. Notably, there is a linear relationship between the frequency of severe hypoglycemic episodes and dementia risk, especially among AD patients. Even a single severe episode can heighten the risk of mortality, independent of dementia status [ 48 ].
Further studies underscore that elderly T2D patients with a history of severe hypoglycemia face a higher dementia risk, suggesting a potential bidirectional relationship between hypoglycemia and dementia risk in this population [ 49 , 50 ]. Our findings corroborate that diabetic patients experiencing hypoglycemic events have a 56% increased risk of developing dementia compared to those without such episodes, confirming the detrimental impact of hypoglycemia on brain health and consequently dementia.
Additionally, a systematic review has quantified this relationship, noting that severe hypoglycemic events raise the risk of dementia [RR: 1.47, 95%CI (1.24–1.74)]. The risk escalates with the frequency of episodes: one event increases the risk by 29% [RR: 1.29, 95%CI (1.15–1.44)], two episodes by 68% [RR: 1.68, 95%CI (1.38–2.04)], and three or more episodes double the risk [RR: 1.99, 95%CI (1.48–2.68)] [ 51 ]. These findings highlight the significant role of hypoglycemic events as a factor in the development of dementia in diabetic patients.
Strengths and limitations
This meta-analysis encompasses studies from 2012 to 2023, providing an updated and comprehensive evaluation of the relationship between diabetes and dementia. It also examines critical factors such as early-stage diabetes, hypoglycemia, diabetes control, glycated hemoglobin, and fasting blood sugar, enhancing our understanding of the intricate link between diabetes and dementia.
Despite its strengths, this study has several limitations. The relatively small number of included studies may restrict the breadth and representativeness of the patient population. This limitation prevented in-depth subgroup analyses by different dementia types like vascular dementia or AD, or by diabetes types such as Type 1, Type 2, or gestational diabetes, which could influence the outcomes. Additionally, the analysis of diabetes-related factors was limited, and the absence of tiered analysis for these factors may affect the robustness of the results. Excluding studies published in non-English languages may also introduce selection bias.
Our findings reaffirm that diabetic patients have a higher risk of dementia, aligning with conclusions from a 2013 study. Future research should incorporate larger sample sizes and adopt a longitudinal approach to investigate various risk factors more accurately. Further studies are encouraged to include diverse types of dementia and diabetes, expand the number of studies reviewed, increase the data volume, and assess the impact of measurement errors on risk estimates.
Data availability
No datasets were generated or analysed during the current study.
Abbreviations
Ottawa Scale (NOS)
Alzheimer’s Disease
Incretin glucagon-like peptide1
Sodium-glucose cotransporter2
Diagnosis and classification of diabetes mellitus. Diabetes Care. 2013;36(Suppl 1):S67–74.
Google Scholar
Sun H, Saeedi P, Karuranga S, Pinkepank M, Ogurtsova K, Duncan BB, et al. IDF Diabetes Atlas: Global, regional and country-level diabetes prevalence estimates for 2021 and projections for 2045. Diabetes Res Clin Pract. 2022;183:109119.
Article PubMed Google Scholar
Saeedi P, Petersohn I, Salpea P, Malanda B, Karuranga S, Unwin N, et al. Global and regional diabetes prevalence estimates for 2019 and projections for 2030 and 2045: results from the International Diabetes Federation Diabetes Atlas, 9(th) edition. Diabetes Res Clin Pract. 2019;157:107843.
Reddy MA, Zhang E, Natarajan R. Epigenetic mechanisms in diabetic complications and metabolic memory. Diabetologia. 2015;58(3):443–55.
Article CAS PubMed Google Scholar
Franzon R, Chiarani F, Mendes RH, Belló-Klein A, Wyse AT. Dietary soy prevents brain Na+, K(+)-ATPase reduction in streptozotocin diabetic rats. Diabetes Res Clin Pract. 2005;69(2):107–12.
Lester-Coll N, Rivera EJ, Soscia SJ, Doiron K, Wands JR, de la Monte SM. Intracerebral Streptozotocin model of type 3 diabetes: relevance to sporadic Alzheimer’s disease. J Alzheimers Dis. 2006;9(1):13–33.
Zhang WJ, Tan YF, Yue JT, Vranic M, Wojtowicz JM. Impairment of hippocampal neurogenesis in streptozotocin-treated diabetic rats. Acta Neurol Scand. 2008;117(3):205–10.
Liu X, Mo Y, Gong J, Li Z, Peng H, Chen J, et al. Puerarin ameliorates cognitive deficits in streptozotocin-induced diabetic rats. Metab Brain Dis. 2016;31(2):417–23.
Cooper C, Sommerlad A, Lyketsos CG, Livingston G. Modifiable predictors of dementia in mild cognitive impairment: a systematic review and meta-analysis. Am J Psychiatry. 2015;172(4):323–34.
Chowdhary N, Barbui C, Anstey KJ, Kivipelto M, Barbera M, Peters R, et al. Reducing the risk of Cognitive decline and Dementia: WHO recommendations. Front Neurol. 2021;12:765584.
Gudala K, Bansal D, Schifano F, Bhansali A. Diabetes mellitus and risk of dementia: a meta-analysis of prospective observational studies. J Diabetes Investig. 2013;4(6):640–50.
Article PubMed PubMed Central Google Scholar
Ninomiya T. Diabetes mellitus and dementia. Curr Diab Rep. 2014;14(5):487.
Biessels GJ, Staekenborg S, Brunner E, Brayne C, Scheltens P. Risk of dementia in diabetes mellitus: a systematic review. Lancet Neurol. 2006;5(1):64–74.
Kloppenborg RP, van den Berg E, Kappelle LJ, Biessels GJ. Diabetes and other vascular risk factors for dementia: which factor matters most? A systematic review. Eur J Pharmacol. 2008;585(1):97–108.
Zheng B, Su B, Price G, Tzoulaki I, Ahmadi-Abhari S, Middleton L, Glycemic, Control. Diabetic complications, and risk of dementia in patients with diabetes: results from a large U.K. Cohort Study. Diabetes Care. 2021;44(7):1556–63.
Lee DY, Kim J, Park S, Park SY, Yu JH, Seo JA, et al. Fasting glucose variability and the risk of Dementia in individuals with diabetes: a Nationwide Cohort Study. Diabetes Metab J. 2022;46(6):923–35.
Kuo CL, Lu CL, Chang YH, Li CY. Population-based Cohort Study on Dementia Risk in patients with type 1 diabetes Mellitus. Neuroepidemiology. 2018;50(1–2):57–62.
Jia Y, Liu R, Tang S, Zhang D, Wang Y, Cong L, et al. Associations of the Glycaemic Control of Diabetes with dementia and physical function in Rural-Dwelling older Chinese adults: a Population-based study. Clin Interv Aging. 2021;16:1503–13.
van Gennip ACE, Stehouwer CDA, van Boxtel MPJ, Verhey FRJ, Koster A, Kroon AA, et al. Association of Type 2 diabetes, according to the number of risk factors within target range, with structural brain abnormalities, cognitive performance, and risk of Dementia. Diabetes Care. 2021;44(11):2493–502.
Chin SO, Rhee SY, Chon S, Baik SH, Park Y, Nam MS, et al. Hypoglycemia is associated with dementia in elderly patients with type 2 diabetes mellitus: an analysis based on the Korea National Diabetes Program Cohort. Diabetes Res Clin Pract. 2016;122:54–61.
Ma F, Wu T, Miao R, Xiao YY, Zhang W, Huang G. Conversion of mild cognitive impairment to dementia among subjects with diabetes: a population-based study of incidence and risk factors with five years of follow-up. J Alzheimers Dis. 2015;43(4):1441–9.
Frison E, Dufouil C, Helmer C, Berr C, Auriacombe S, Chêne G. Diabetes-Associated Dementia Risk and competing risk of death in the three-city study. J Alzheimers Dis. 2019;71(4):1339–50.
Salinas RM, Hiriart M, Acosta I, Sosa AL, Prince MJ. Type 2 diabetes mellitus as a risk factor for dementia in a Mexican population. J Diabetes Complications. 2016;30(7):1234–9.
Secnik J, Cermakova P, Fereshtehnejad SM, Dannberg P, Johnell K, Fastbom J, et al. Diabetes in a large dementia cohort: clinical characteristics and treatment from the Swedish Dementia Registry. Diabetes Care. 2017;40(9):1159–66.
Javanshiri K, Waldö ML, Friberg N, Sjövall F, Wickerström K, Haglund M, et al. Atherosclerosis, hypertension, and diabetes in Alzheimer’s Disease, Vascular Dementia, and mixed dementia: prevalence and presentation. J Alzheimers Dis. 2018;65(4):1247–58.
Barbiellini Amidei C, Fayosse A, Dumurgier J, Machado-Fragua MD, Tabak AG, van Sloten T, et al. Association between Age at Diabetes Onset and subsequent risk of Dementia. JAMA. 2021;325(16):1640–9.
Gong J, Harris K, Hackett M, Peters SAE, Brodaty H, Cooper M, et al. Sex differences in risk factors for cognitive decline and dementia, including death as a competing risk, in individuals with diabetes: results from the ADVANCE trial. Diabetes Obes Metab. 2021;23(8):1775–85.
Kim WJ, Lee SJ, Lee E, Lee EY, Han K. Risk of Incident Dementia according to Glycemic Status and comorbidities of Hyperglycemia: a Nationwide Population-based Cohort Study. Diabetes Care. 2022;45(1):134–41.
Alkabbani W, Maxwell CJ, Marrie RA, Tyas SL, Lega IC, Gamble JM. Hypoglycaemia and the risk of dementia: a population-based cohort study using exposure density sampling. Int J Epidemiol. 2023;52(3):908–20.
Leibson CL, Rocca WA, Hanson VA, Cha R, Kokmen E, O’Brien PC, et al. Risk of dementia among persons with diabetes mellitus: a population-based cohort study. Am J Epidemiol. 1997;145(4):301–8.
Ott A, Stolk RP, van Harskamp F, Pols HA, Hofman A, Breteler MM. Diabetes mellitus and the risk of dementia: the Rotterdam Study. Neurology. 1999;53(9):1937–42.
Yu JT, Xu W, Tan CC, Andrieu S, Suckling J, Evangelou E, et al. Evidence-based prevention of Alzheimer’s disease: systematic review and meta-analysis of 243 observational prospective studies and 153 randomised controlled trials. J Neurol Neurosurg Psychiatry. 2020;91(11):1201–9.
Xue M, Xu W, Ou YN, Cao XP, Tan MS, Tan L, et al. Diabetes mellitus and risks of cognitive impairment and dementia: a systematic review and meta-analysis of 144 prospective studies. Ageing Res Rev. 2019;55:100944.
Li ZG, Zhang W, Grunberger G, Sima AA. Hippocampal neuronal apoptosis in type 1 diabetes. Brain Res. 2002;946(2):221–31.
Faheem NM, El Askary A. Neuroprotective role of curcumin on the hippocampus against the structural and serological alterations of streptozotocin-induced diabetes in Sprague Dawely rats. Iran J Basic Med Sci. 2017;20(6):690–9.
PubMed PubMed Central Google Scholar
Chen R, Shi J, Yin Q, Li X, Sheng Y, Han J, et al. Morphological and pathological characteristics of Brain in Diabetic Encephalopathy. J Alzheimers Dis. 2018;65(1):15–28.
Mayeda ER, Whitmer RA, Yaffe K. Diabetes and cognition. Clin Geriatr Med. 2015;31(1):101–15. ix.
Baglietto-Vargas D, Shi J, Yaeger DM, Ager R, LaFerla FM. Diabetes and Alzheimer’s disease crosstalk. Neurosci Biobehav Rev. 2016;64:272–87.
Tomic D, Shaw JE, Magliano DJ. The burden and risks of emerging complications of diabetes mellitus. Nat Rev Endocrinol. 2022;18(9):525–39.
Ciudin A, Hernández C, Simó-Servat O, Simó R. The usefulness of the retina for identifying people with type 2 diabetes with prodromal stages of dementia. Neurosci Biobehav Rev. 2024;159:105592.
Rawlings AM, Sharrett AR, Albert MS, Coresh J, Windham BG, Power MC, et al. The Association of late-life diabetes status and hyperglycemia with incident mild cognitive impairment and dementia: the ARIC Study. Diabetes Care. 2019;42(7):1248–54.
Article CAS PubMed PubMed Central Google Scholar
Ji X, Gao H, Sun D, Zhuang J, Fang Y, Wang K, et al. Trajectories of Cognition and Daily Functioning before and after Incident Diabetes. Diabetes Care. 2023;46(1):75–82.
Chen Q, Zhu S, Shang J, Fang Q, Xue Q, Hua J. Trends in cognitive function before and after diabetes onset: the China Health and Retirement Longitudinal Study. Neurology. 2024;102(7):e209165.
Li FR, Yang HL, Zhou R, Zheng JZ, Chen GC, Wu XX, et al. Influence of diabetes duration and Glycemic Control on Dementia: a Cohort Study. J Gerontol Biol Sci Med Sci. 2021;76(11):2062–70.
Article CAS Google Scholar
Reinke C, Buchmann N, Fink A, Tegeler C, Demuth I, Doblhammer G. Diabetes duration and the risk of dementia: a cohort study based on German health claims data. Age Ageing. 2022;51(1).
Song J, Bai H, Xu H, Xing Y, Chen S. HbA1c variability and the risk of dementia in patients with diabetes: a Meta-analysis. Int J Clin Pract. 2022;2022:7706330.
Hendrie HC, Zheng M, Li W, Lane K, Ambuehl R, Purnell C, et al. Glucose level decline precedes dementia in elderly African americans with diabetes. Alzheimers Dement. 2017;13(2):111–8.
Han E, Han KD, Lee BW, Kang ES, Cha BS, Ko SH, et al. Severe hypoglycemia increases dementia risk and related mortality: a Nationwide, Population-based Cohort Study. J Clin Endocrinol Metab. 2022;107(5):e1976–86.
Whitmer RA, Karter AJ, Yaffe K, Quesenberry CP Jr., Selby JV. Hypoglycemic episodes and risk of dementia in older patients with type 2 diabetes mellitus. JAMA. 2009;301(15):1565–72.
Yaffe K, Falvey CM, Hamilton N, Harris TB, Simonsick EM, Strotmeyer ES, et al. Association between hypoglycemia and dementia in a biracial cohort of older adults with diabetes mellitus. JAMA Intern Med. 2013;173(14):1300–6.
Gómez-Guijarro MD, Álvarez-Bueno C, Saz-Lara A, Sequí-Domínguez I, Lucerón-Lucas-Torres M, Cavero-Redondo I. Association between severe hypoglycaemia and risk of dementia in patients with type 2 diabetes mellitus: a systematic review and meta-analysis. Diabetes Metab Res Rev. 2023;39(3):e3610.
Download references
Acknowledgements
An ethics statement is not applicable because this study is based exclusively on published literature.
This study was supported by Zhang Wenfeng Inheritance Workshop for Famous Elderly Chinese Medicine Experts (Project of Jilin Provincial Administration of Traditional Chinese Medicine). The fund was not involved in any study design, data collection, analysis and interpretation, report writing, and article submission for publication.
Author information
Authors and affiliations.
School of Basic Medical Sciences, Changchun University of Chinese Medicine, Changchun, 130117, China
Fang Cao & Wenfeng Zhang
College of Chinese Medicine, Changchun University of Chinese Medicine, Changchun, 130117, China
Fushuang Yang, Jian Li, Wei Guo, Chongheng Zhang & Fa Gao
Department of Nutrition, Chinese People’s Armed Police Force Medical Characteristic Center, Tianjin, 300162, China
Department of Geriatrics, Baotou Mengshi Hospital of Traditional Chinese Medicine, Baotou, 014000, China
You can also search for this author in PubMed Google Scholar
Contributions
Conceptualization: Fang Cao, Fushuang Yang, Wenfeng Zhang. Data curation: Fang Cao, Wei Guo. Formal analysis: Fang Cao, Chongheng Zhang. Funding acquisition: Fang Cao, Chongheng Zhang. Investigation: Fushuang Yang, Fa Gao. Methodology: Fushuang Yang, Fa Gao. Project administration: Fushuang Yang, Xinxin Sun. Resources: Wei Guo, Xinxin Sun. Software: Wei Guo, Yi Zhou. Supervision: Jian Li, Wenfeng Zhang. Validation: Jian Li, Wenfeng Zhang. Visualization: Fang Cao, Wenfeng Zhang. Writing -original draft: Fang Cao, Fushuang Yang, Wei Guo, Jian Li, Chongheng Zhang, Fa Gao, Xinxin Sun, Yi Zhou, Wenfeng Zhang. Writing-review & editing: Fang Cao, Fushuang Yang, Wei Guo, Jian Li, Chongheng Zhang, Fa Gao, Xinxin Sun, Yi Zhou,
Corresponding author
Correspondence to Wenfeng Zhang .
Ethics declarations
Ethics approval and consent to participate.
Not applicable.
Consent for publication
Conflict of interest.
The authors declare that they have no competing interests.
Additional information
Publisher’s note.
Springer Nature remains neutral with regard to jurisdictional claims in published maps and institutional affiliations.
Electronic supplementary material
Below is the link to the electronic supplementary material.
13098_2024_1346_MOESM1_ESM.docx
Supplementary Material 1: Search strategy. Figure S1: Association between diabetes duration (<5 years) and risk of dementia; CI, confidence interval. Figure S2: Association between hypoglycemic events and risk of dementia; CI, confidence interval. Figure S3: Association between diabetes control and risk of dementia; CI, confidence interval. Figure S4: Association between glycated hemoglobin and risk of dementia; CI, confidence interval. Figure S5: Association between fasting blood glucose and risk of dementia, CI, confidence interval. Figure S6: Publication bias.
Rights and permissions
Open Access This article is licensed under a Creative Commons Attribution 4.0 International License, which permits use, sharing, adaptation, distribution and reproduction in any medium or format, as long as you give appropriate credit to the original author(s) and the source, provide a link to the Creative Commons licence, and indicate if changes were made. The images or other third party material in this article are included in the article’s Creative Commons licence, unless indicated otherwise in a credit line to the material. If material is not included in the article’s Creative Commons licence and your intended use is not permitted by statutory regulation or exceeds the permitted use, you will need to obtain permission directly from the copyright holder. To view a copy of this licence, visit http://creativecommons.org/licenses/by/4.0/ . The Creative Commons Public Domain Dedication waiver ( http://creativecommons.org/publicdomain/zero/1.0/ ) applies to the data made available in this article, unless otherwise stated in a credit line to the data.
Reprints and permissions
About this article
Cite this article.
Cao, F., Yang, F., Li, J. et al. The relationship between diabetes and the dementia risk: a meta-analysis. Diabetol Metab Syndr 16 , 101 (2024). https://doi.org/10.1186/s13098-024-01346-4
Download citation
Received : 26 January 2024
Accepted : 04 May 2024
Published : 14 May 2024
DOI : https://doi.org/10.1186/s13098-024-01346-4
Share this article
Anyone you share the following link with will be able to read this content:
Sorry, a shareable link is not currently available for this article.
Provided by the Springer Nature SharedIt content-sharing initiative
Diabetology & Metabolic Syndrome
ISSN: 1758-5996
- Submission enquiries: [email protected]

Food & Function
Inhibitory effect of extracts from edible parts of nuts on α-amylase activity: a systematic review †.

* Corresponding authors
a Department of Nutrition, Dietetics and Food, Monash University, BASE Facility, Level 1, 264 Ferntree Gully Road, Notting Hill, VIC 3168, Australia E-mail: [email protected]
b Victorian Heart Institute, Monash University, 631 Blackburn Road, Clayton, VIC 3168, Australia
c School of Health Sciences, Swinburne University of Technology, John St, Hawthorn, VIC 3122, Australia
Elevated blood glucose concentration is a risk factor for developing metabolic dysfunction and insulin resistance, leading to type 2 diabetes and cardiovascular diseases. Nuts have the potential to inhibit α-amylase activity, and so lower postprandial glucose, due to their content of polyphenols and other bioactive compounds. We conducted a systematic literature review to assess the ability of extracts from commonly consumed edible parts of nuts to inhibit α-amylase. Among the 31 included papers, only four utilised human α-amylases. These papers indicated that polyphenol-rich chestnut skin extracts exhibited strong inhibition of both human salivary and pancreatic α-amylases, and that a polyphenol-rich almond skin extract was a potent inhibitor of human salivary α-amylase. The majority of the reviewed studies utilised porcine pancreatic α-amylase, which has ∼86% sequence homology with the corresponding human enzyme but with some key amino acid variations located within the active site. Polyphenol-rich extracts from chestnut, almond, kola nut, pecan and walnut, and peptides isolated from cashew, inhibited porcine pancreatic α-amylase. Some studies used α-amylases sourced from fungi or bacteria, outcomes from which are entirely irrelevant to human health, as they have no sequence homology with the human enzyme. Given the limited research involving human α-amylases, and the differences in inhibition compared to porcine enzymes and especially enzymes from microorganisms, it is recommended that future in vitro experiments place greater emphasis on utilising enzymes sourced from humans to facilitate a reliable prediction of effects in intervention studies.
- This article is part of the themed collection: Food & Function Review Articles 2024
Supplementary files
- Supplementary information PDF (128K)
Article information

Download Citation
Permissions.

Inhibitory effect of extracts from edible parts of nuts on α-amylase activity: a systematic review
M. Farazi, M. J. Houghton, B. R. Cardoso, M. Murray and G. Williamson, Food Funct. , 2024, 15 , 5209 DOI: 10.1039/D4FO00414K
This article is licensed under a Creative Commons Attribution-NonCommercial 3.0 Unported Licence . You can use material from this article in other publications, without requesting further permission from the RSC, provided that the correct acknowledgement is given and it is not used for commercial purposes.
To request permission to reproduce material from this article in a commercial publication , please go to the Copyright Clearance Center request page .
If you are an author contributing to an RSC publication, you do not need to request permission provided correct acknowledgement is given.
If you are the author of this article, you do not need to request permission to reproduce figures and diagrams provided correct acknowledgement is given. If you want to reproduce the whole article in a third-party commercial publication (excluding your thesis/dissertation for which permission is not required) please go to the Copyright Clearance Center request page .
Read more about how to correctly acknowledge RSC content .
Social activity
Search articles by author, advertisements.
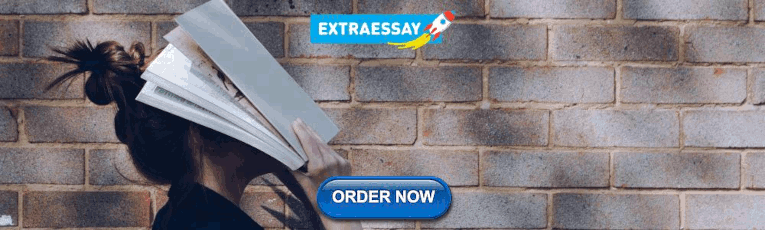
IMAGES
VIDEO
COMMENTS
In this paper, we present a systematic review of the literature on the association of these risk factors with the incidence/prevalence of type 2 diabetes. We give insights on the contribution of independent risk factors in the development of type 2 diabetes along with possible solutions towards a preventive approach.
Misra, Wagner et al. systematically review if strategies to subclassify type 2 diabetes (T2D) are associated with high quality evidence and patient outcomes. Cluster-based stratification yields ...
Objective To assess what proportions of studies reported increasing, stable, or declining trends in the incidence of diagnosed diabetes. Design Systematic review of studies reporting trends of diabetes incidence in adults from 1980 to 2017 according to PRISMA guidelines. Data sources Medline, Embase, CINAHL, and reference lists of relevant publications. Eligibility criteria Studies of open ...
This Review summarizes information from systematic reviews and major cohort studies regarding emerging complications of type 1 and type 2 diabetes mellitus to identify and quantify associations ...
The global burden of type 2 diabetes mellitus (T2DM) is rapidly increasing, affecting individuals of all ages. The global T2DM prevalence nearly doubled in the adult population over the past decade from 4.7% in 1980 to 8.5% in 2014 [].The global burden of T2DM in people 20-79 years is further projected to increase to 629 million in 2045 compared to 425 million in 2017 [].
Aims To reduce the burden of type 2 diabetes (T2DM), the disease decision model plays a vital role in supporting decision-making. Currently, there is no comprehensive summary and assessment of the existing decision models for T2DM. The objective of this review is to provide an overview of the characteristics and capabilities of published decision models for T2DM. We also discuss which models ...
There is limited evidence on whether screening for type 2 diabetes mellitus affects health outcomes. A recent systematic review of randomised clinical trials found only one trial that met their inclusion criteria; therefore, current guidelines for screening interventions for type 2 diabetes mellitus are based on expert opinions and best practice rather than synthesised evidence.
Dietary Patterns and Type 2 Diabetes: A Systematic Literature Review and Meta-Analysis of Prospective Studies 1 2. Author links open overlay panel Jannasch Franziska 4 5, Kröger Janine 4 5, ... cardiovascular disease, and type 2 diabetes: systematic review and meta-analysis of observational studies. BMJ, 351 (2015), p. 3978.
Background Cardiovascular disease (CVD) is a common comorbidity in type 2 diabetes (T2DM). CVD's prevalence has been growing over time. Purpose To estimate the current prevalence of CVD among adults with T2DM by reviewing literature published within the last 10 years (2007-March 2017). Methods We searched Medline, Embase, and proceedings of major scientific meetings for original research ...
Mikhael EM, Hassali MA, Hussain SA. Effectiveness of diabetes self-management educational programs for type 2 diabetes mellitus patients in middle east countries: A systematic review. Diabetes Metab Syndr Obes 2020;13:117.
The purpose of this literature review was to identify educational approaches addressing low health literacy for people with type 2 diabetes. Low health literacy can lead to poor management of diabetes, low engagement with health care providers, increased hospitalization rates, and higher health care costs.
Effectiveness of diabetes self-management education (DSME) in type 2 diabetes mellitus (T2DM) patients: Systematic literature review Ucik Ernawati, Titin Andri Wihastuti, Yulian Wiji Utami School of Nursing, Faculty of Medicine, Universitas Brawijaya, Malang, Indonesia Abstract Diabetes mellitus is a chronic disease characterized by high
The rising prevalence of type 2 diabetes (T2DM) and hypertension in older adults, and the deleterious effect of these conditions on cerebrovascular and brain health, is creating a growing discrepancy between the "typical" cognitive aging trajectory and a "healthy" cognitive aging trajectory. These changing health demographics make T2DM and hypertension important topics of study in ...
DNA methylation influences gene expression and function in the pathophysiology of type 2 diabetes mellitus (T2DM). Mapping of T2DM-associated DNA methylation could aid early detection and/or therapeutic treatment options for diabetics. A systematic literature search for associations between T2DM and DNA methylation was performed. Prospero registration ID: CRD42020140436.
The prevalence of diabetes-specific emotional distress in people with Type 2 diabetes: A systematic review and meta-analysis. Diabet. Med. 34 (11), 1508-1520 (2017).
The current umbrella review included 51 systematic reviews with meta-analysis articles with 270 associations. We re-calculated each association and found only one convincing evidence (Class I) for an association between blood group B and type 2 diabetes mellitus risk compared with the non-B blood group.
We conducted a literature search in the Medline and Web of Science up to June 12, 2023, to identify related cohort studies and systematic reviews. The mean RR (relative risk) curve and uncertainty intervals (UIs), BPRF function, risk-outcome score (ROS), and the theoretical minimum risk exposure level (TMREL) were estimated to evaluate the ...
The literature search returned a total of 776 articles. From these, 139 duplicates were removed. The titles and abstracts of the remaining 637 articles were reviewed ... Differential manifestation of type 2 diabetes in Black Africans and White Europeans with recently diagnosed type 2 diabetes: A systematic review Author: Administrator Created Date:
The link between diabetes and dementia risk is not well understood. This study evaluates the factors linking diabetes to dementia onset, providing guidance for preventing dementia in diabetic patients. This analysis utilized databases such as PubMed, Embase, Web of Science, and the Cochrane Library to review literature from January 31, 2012, to March 5, 2023.
Elevated blood glucose concentration is a risk factor for developing metabolic dysfunction and insulin resistance, leading to type 2 diabetes and cardiovascular diseases. Nuts have the potential to inhibit α-amylase activity, and so lower postprandial glucose, due to their content of polyphenols and other bioactive Food &; Function Review Articles 2024
This paper aims to identify risk factors and elements associated with type 2 diabetes. Both quantitative and qualitative approaches were used to collect data. Risk factors for type 2 diabetes are also presented from the systematic literature review to determine the variables and which variable seems to have the greatest impact on type 2 diabetes.