Root out friction in every digital experience, super-charge conversion rates, and optimize digital self-service
Uncover insights from any interaction, deliver AI-powered agent coaching, and reduce cost to serve
Increase revenue and loyalty with real-time insights and recommendations delivered to teams on the ground
Know how your people feel and empower managers to improve employee engagement, productivity, and retention
Take action in the moments that matter most along the employee journey and drive bottom line growth
Whatever they’re are saying, wherever they’re saying it, know exactly what’s going on with your people
Get faster, richer insights with qual and quant tools that make powerful market research available to everyone
Run concept tests, pricing studies, prototyping + more with fast, powerful studies designed by UX research experts
Track your brand performance 24/7 and act quickly to respond to opportunities and challenges in your market
Explore the platform powering Experience Management
- Free Account
- Product Demos
- For Digital
- For Customer Care
- For Human Resources
- For Researchers
- Financial Services
- All Industries
Popular Use Cases
- Customer Experience
- Employee Experience
- Net Promoter Score
- Voice of Customer
- Customer Success Hub
- Product Documentation
- Training & Certification
- XM Institute
- Popular Resources
- Customer Stories
- Artificial Intelligence
Market Research
- Partnerships
- Marketplace
The annual gathering of the experience leaders at the world’s iconic brands building breakthrough business results, live in Salt Lake City.
- English/AU & NZ
- Español/Europa
- Español/América Latina
- Português Brasileiro
- REQUEST DEMO
- Experience Management
- Causal Research
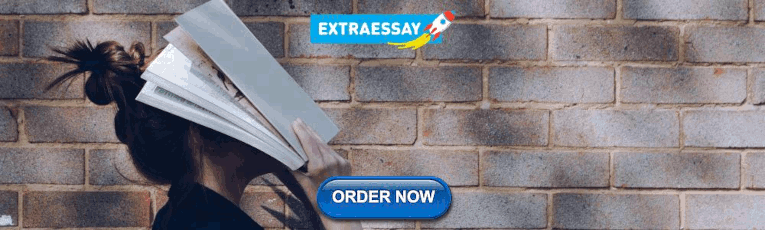
Try Qualtrics for free
Causal research: definition, examples and how to use it.
16 min read Causal research enables market researchers to predict hypothetical occurrences & outcomes while improving existing strategies. Discover how this research can decrease employee retention & increase customer success for your business.
What is causal research?
Causal research, also known as explanatory research or causal-comparative research, identifies the extent and nature of cause-and-effect relationships between two or more variables.
It’s often used by companies to determine the impact of changes in products, features, or services process on critical company metrics. Some examples:
- How does rebranding of a product influence intent to purchase?
- How would expansion to a new market segment affect projected sales?
- What would be the impact of a price increase or decrease on customer loyalty?
To maintain the accuracy of causal research, ‘confounding variables’ or influences — e.g. those that could distort the results — are controlled. This is done either by keeping them constant in the creation of data, or by using statistical methods. These variables are identified before the start of the research experiment.
As well as the above, research teams will outline several other variables and principles in causal research:
- Independent variables
The variables that may cause direct changes in another variable. For example, the effect of truancy on a student’s grade point average. The independent variable is therefore class attendance.
- Control variables
These are the components that remain unchanged during the experiment so researchers can better understand what conditions create a cause-and-effect relationship.
This describes the cause-and-effect relationship. When researchers find causation (or the cause), they’ve conducted all the processes necessary to prove it exists.
- Correlation
Any relationship between two variables in the experiment. It’s important to note that correlation doesn’t automatically mean causation. Researchers will typically establish correlation before proving cause-and-effect.
- Experimental design
Researchers use experimental design to define the parameters of the experiment — e.g. categorizing participants into different groups.
- Dependent variables
These are measurable variables that may change or are influenced by the independent variable. For example, in an experiment about whether or not terrain influences running speed, your dependent variable is the terrain.
Why is causal research useful?
It’s useful because it enables market researchers to predict hypothetical occurrences and outcomes while improving existing strategies. This allows businesses to create plans that benefit the company. It’s also a great research method because researchers can immediately see how variables affect each other and under what circumstances.
Also, once the first experiment has been completed, researchers can use the learnings from the analysis to repeat the experiment or apply the findings to other scenarios. Because of this, it’s widely used to help understand the impact of changes in internal or commercial strategy to the business bottom line.
Some examples include:
- Understanding how overall training levels are improved by introducing new courses
- Examining which variations in wording make potential customers more interested in buying a product
- Testing a market’s response to a brand-new line of products and/or services
So, how does causal research compare and differ from other research types?
Well, there are a few research types that are used to find answers to some of the examples above:
1. Exploratory research
As its name suggests, exploratory research involves assessing a situation (or situations) where the problem isn’t clear. Through this approach, researchers can test different avenues and ideas to establish facts and gain a better understanding.
Researchers can also use it to first navigate a topic and identify which variables are important. Because no area is off-limits, the research is flexible and adapts to the investigations as it progresses.
Finally, this approach is unstructured and often involves gathering qualitative data, giving the researcher freedom to progress the research according to their thoughts and assessment. However, this may make results susceptible to researcher bias and may limit the extent to which a topic is explored.
2. Descriptive research
Descriptive research is all about describing the characteristics of the population, phenomenon or scenario studied. It focuses more on the “what” of the research subject than the “why”.
For example, a clothing brand wants to understand the fashion purchasing trends amongst buyers in California — so they conduct a demographic survey of the region, gather population data and then run descriptive research. The study will help them to uncover purchasing patterns amongst fashion buyers in California, but not necessarily why those patterns exist.
As the research happens in a natural setting, variables can cross-contaminate other variables, making it harder to isolate cause and effect relationships. Therefore, further research will be required if more causal information is needed.
Get started on your market research journey with CoreXM
How is causal research different from the other two methods above?
Well, causal research looks at what variables are involved in a problem and ‘why’ they act a certain way. As the experiment takes place in a controlled setting (thanks to controlled variables) it’s easier to identify cause-and-effect amongst variables.
Furthermore, researchers can carry out causal research at any stage in the process, though it’s usually carried out in the later stages once more is known about a particular topic or situation.
Finally, compared to the other two methods, causal research is more structured, and researchers can combine it with exploratory and descriptive research to assist with research goals.
Summary of three research types
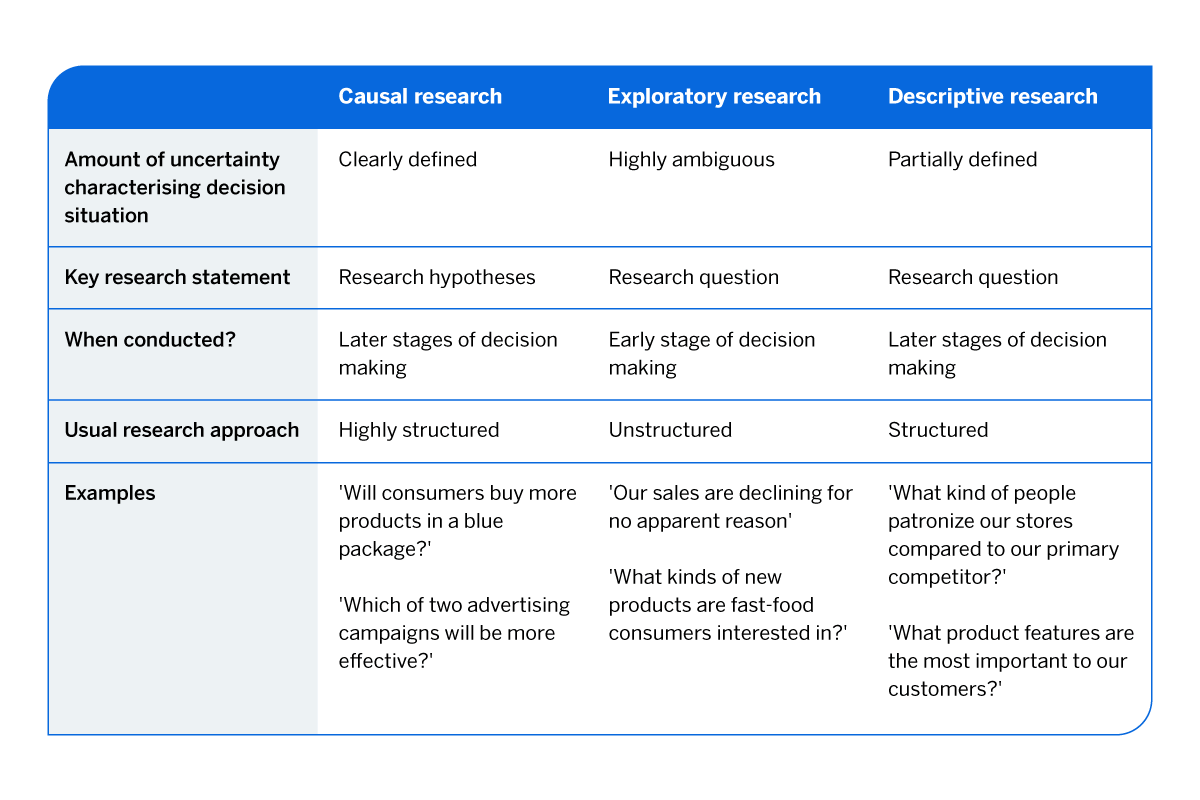
What are the advantages of causal research?
- Improve experiences
By understanding which variables have positive impacts on target variables (like sales revenue or customer loyalty), businesses can improve their processes, return on investment, and the experiences they offer customers and employees.
- Help companies improve internally
By conducting causal research, management can make informed decisions about improving their employee experience and internal operations. For example, understanding which variables led to an increase in staff turnover.
- Repeat experiments to enhance reliability and accuracy of results
When variables are identified, researchers can replicate cause-and-effect with ease, providing them with reliable data and results to draw insights from.
- Test out new theories or ideas
If causal research is able to pinpoint the exact outcome of mixing together different variables, research teams have the ability to test out ideas in the same way to create viable proof of concepts.
- Fix issues quickly
Once an undesirable effect’s cause is identified, researchers and management can take action to reduce the impact of it or remove it entirely, resulting in better outcomes.
What are the disadvantages of causal research?
- Provides information to competitors
If you plan to publish your research, it provides information about your plans to your competitors. For example, they might use your research outcomes to identify what you are up to and enter the market before you.
- Difficult to administer
Causal research is often difficult to administer because it’s not possible to control the effects of extraneous variables.
- Time and money constraints
Budgetary and time constraints can make this type of research expensive to conduct and repeat. Also, if an initial attempt doesn’t provide a cause and effect relationship, the ROI is wasted and could impact the appetite for future repeat experiments.
- Requires additional research to ensure validity
You can’t rely on just the outcomes of causal research as it’s inaccurate. It’s best to conduct other types of research alongside it to confirm its output.
- Trouble establishing cause and effect
Researchers might identify that two variables are connected, but struggle to determine which is the cause and which variable is the effect.
- Risk of contamination
There’s always the risk that people outside your market or area of study could affect the results of your research. For example, if you’re conducting a retail store study, shoppers outside your ‘test parameters’ shop at your store and skew the results.
How can you use causal research effectively?
To better highlight how you can use causal research across functions or markets, here are a few examples:
Market and advertising research
A company might want to know if their new advertising campaign or marketing campaign is having a positive impact. So, their research team can carry out a causal research project to see which variables cause a positive or negative effect on the campaign.
For example, a cold-weather apparel company in a winter ski-resort town may see an increase in sales generated after a targeted campaign to skiers. To see if one caused the other, the research team could set up a duplicate experiment to see if the same campaign would generate sales from non-skiers. If the results reduce or change, then it’s likely that the campaign had a direct effect on skiers to encourage them to purchase products.
Improving customer experiences and loyalty levels
Customers enjoy shopping with brands that align with their own values, and they’re more likely to buy and present the brand positively to other potential shoppers as a result. So, it’s in your best interest to deliver great experiences and retain your customers.
For example, the Harvard Business Review found that an increase in customer retention rates by 5% increased profits by 25% to 95%. But let’s say you want to increase your own, how can you identify which variables contribute to it?Using causal research, you can test hypotheses about which processes, strategies or changes influence customer retention. For example, is it the streamlined checkout? What about the personalized product suggestions? Or maybe it was a new solution that solved their problem? Causal research will help you find out.
Discover how to use analytics to improve customer retention.
Improving problematic employee turnover rates
If your company has a high attrition rate, causal research can help you narrow down the variables or reasons which have the greatest impact on people leaving. This allows you to prioritize your efforts on tackling the issues in the right order, for the best positive outcomes.
For example, through causal research, you might find that employee dissatisfaction due to a lack of communication and transparency from upper management leads to poor morale, which in turn influences employee retention.
To rectify the problem, you could implement a routine feedback loop or session that enables your people to talk to your company’s C-level executives so that they feel heard and understood.
How to conduct causal research first steps to getting started are:
1. Define the purpose of your research
What questions do you have? What do you expect to come out of your research? Think about which variables you need to test out the theory.
2. Pick a random sampling if participants are needed
Using a technology solution to support your sampling, like a database, can help you define who you want your target audience to be, and how random or representative they should be.
3. Set up the controlled experiment
Once you’ve defined which variables you’d like to measure to see if they interact, think about how best to set up the experiment. This could be in-person or in-house via interviews, or it could be done remotely using online surveys.
4. Carry out the experiment
Make sure to keep all irrelevant variables the same, and only change the causal variable (the one that causes the effect) to gather the correct data. Depending on your method, you could be collecting qualitative or quantitative data, so make sure you note your findings across each regularly.
5. Analyze your findings
Either manually or using technology, analyze your data to see if any trends, patterns or correlations emerge. By looking at the data, you’ll be able to see what changes you might need to do next time, or if there are questions that require further research.
6. Verify your findings
Your first attempt gives you the baseline figures to compare the new results to. You can then run another experiment to verify your findings.
7. Do follow-up or supplemental research
You can supplement your original findings by carrying out research that goes deeper into causes or explores the topic in more detail. One of the best ways to do this is to use a survey. See ‘Use surveys to help your experiment’.
Identifying causal relationships between variables
To verify if a causal relationship exists, you have to satisfy the following criteria:
- Nonspurious association
A clear correlation exists between one cause and the effect. In other words, no ‘third’ that relates to both (cause and effect) should exist.
- Temporal sequence
The cause occurs before the effect. For example, increased ad spend on product marketing would contribute to higher product sales.
- Concomitant variation
The variation between the two variables is systematic. For example, if a company doesn’t change its IT policies and technology stack, then changes in employee productivity were not caused by IT policies or technology.
How surveys help your causal research experiments?
There are some surveys that are perfect for assisting researchers with understanding cause and effect. These include:
- Employee Satisfaction Survey – An introductory employee satisfaction survey that provides you with an overview of your current employee experience.
- Manager Feedback Survey – An introductory manager feedback survey geared toward improving your skills as a leader with valuable feedback from your team.
- Net Promoter Score (NPS) Survey – Measure customer loyalty and understand how your customers feel about your product or service using one of the world’s best-recognized metrics.
- Employee Engagement Survey – An entry-level employee engagement survey that provides you with an overview of your current employee experience.
- Customer Satisfaction Survey – Evaluate how satisfied your customers are with your company, including the products and services you provide and how they are treated when they buy from you.
- Employee Exit Interview Survey – Understand why your employees are leaving and how they’ll speak about your company once they’re gone.
- Product Research Survey – Evaluate your consumers’ reaction to a new product or product feature across every stage of the product development journey.
- Brand Awareness Survey – Track the level of brand awareness in your target market, including current and potential future customers.
- Online Purchase Feedback Survey – Find out how well your online shopping experience performs against customer needs and expectations.
That covers the fundamentals of causal research and should give you a foundation for ongoing studies to assess opportunities, problems, and risks across your market, product, customer, and employee segments.
If you want to transform your research, empower your teams and get insights on tap to get ahead of the competition, maybe it’s time to leverage Qualtrics CoreXM.
Qualtrics CoreXM provides a single platform for data collection and analysis across every part of your business — from customer feedback to product concept testing. What’s more, you can integrate it with your existing tools and services thanks to a flexible API.
Qualtrics CoreXM offers you as much or as little power and complexity as you need, so whether you’re running simple surveys or more advanced forms of research, it can deliver every time.
Related resources
Market intelligence 10 min read, marketing insights 11 min read, ethnographic research 11 min read, qualitative vs quantitative research 13 min read, qualitative research questions 11 min read, qualitative research design 12 min read, primary vs secondary research 14 min read, request demo.
Ready to learn more about Qualtrics?
Causal Research: Definition, Design, Tips, Examples
Appinio Research · 21.02.2024 · 34min read
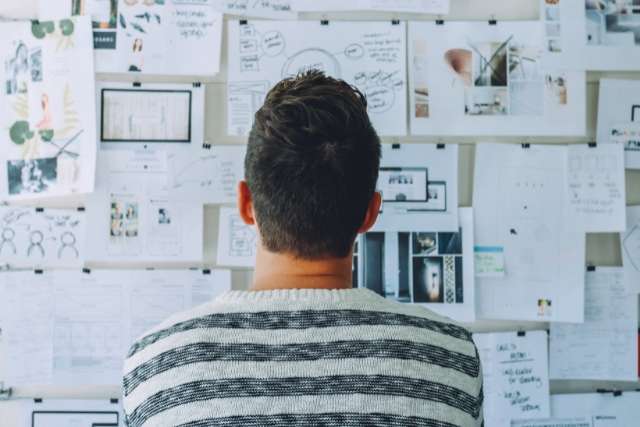
Ever wondered why certain events lead to specific outcomes? Understanding causality—the relationship between cause and effect—is crucial for unraveling the mysteries of the world around us. In this guide on causal research, we delve into the methods, techniques, and principles behind identifying and establishing cause-and-effect relationships between variables. Whether you're a seasoned researcher or new to the field, this guide will equip you with the knowledge and tools to conduct rigorous causal research and draw meaningful conclusions that can inform decision-making and drive positive change.
What is Causal Research?
Causal research is a methodological approach used in scientific inquiry to investigate cause-and-effect relationships between variables. Unlike correlational or descriptive research, which merely examine associations or describe phenomena, causal research aims to determine whether changes in one variable cause changes in another variable.
Importance of Causal Research
Understanding the importance of causal research is crucial for appreciating its role in advancing knowledge and informing decision-making across various fields. Here are key reasons why causal research is significant:
- Establishing Causality: Causal research enables researchers to determine whether changes in one variable directly cause changes in another variable. This helps identify effective interventions, predict outcomes, and inform evidence-based practices.
- Guiding Policy and Practice: By identifying causal relationships, causal research provides empirical evidence to support policy decisions, program interventions, and business strategies. Decision-makers can use causal findings to allocate resources effectively and address societal challenges.
- Informing Predictive Modeling : Causal research contributes to the development of predictive models by elucidating causal mechanisms underlying observed phenomena. Predictive models based on causal relationships can accurately forecast future outcomes and trends.
- Advancing Scientific Knowledge: Causal research contributes to the cumulative body of scientific knowledge by testing hypotheses, refining theories, and uncovering underlying mechanisms of phenomena. It fosters a deeper understanding of complex systems and phenomena.
- Mitigating Confounding Factors: Understanding causal relationships allows researchers to control for confounding variables and reduce bias in their studies. By isolating the effects of specific variables, researchers can draw more valid and reliable conclusions.
Causal Research Distinction from Other Research
Understanding the distinctions between causal research and other types of research methodologies is essential for researchers to choose the most appropriate approach for their study objectives. Let's explore the differences and similarities between causal research and descriptive, exploratory, and correlational research methodologies .
Descriptive vs. Causal Research
Descriptive research focuses on describing characteristics, behaviors, or phenomena without manipulating variables or establishing causal relationships. It provides a snapshot of the current state of affairs but does not attempt to explain why certain phenomena occur.
Causal research , on the other hand, seeks to identify cause-and-effect relationships between variables by systematically manipulating independent variables and observing their effects on dependent variables. Unlike descriptive research, causal research aims to determine whether changes in one variable directly cause changes in another variable.
Similarities:
- Both descriptive and causal research involve empirical observation and data collection.
- Both types of research contribute to the scientific understanding of phenomena, albeit through different approaches.
Differences:
- Descriptive research focuses on describing phenomena, while causal research aims to explain why phenomena occur by identifying causal relationships.
- Descriptive research typically uses observational methods, while causal research often involves experimental designs or causal inference techniques to establish causality.
Exploratory vs. Causal Research
Exploratory research aims to explore new topics, generate hypotheses, or gain initial insights into phenomena. It is often conducted when little is known about a subject and seeks to generate ideas for further investigation.
Causal research , on the other hand, is concerned with testing hypotheses and establishing cause-and-effect relationships between variables. It builds on existing knowledge and seeks to confirm or refute causal hypotheses through systematic investigation.
- Both exploratory and causal research contribute to the generation of knowledge and theory development.
- Both types of research involve systematic inquiry and data analysis to answer research questions.
- Exploratory research focuses on generating hypotheses and exploring new areas of inquiry, while causal research aims to test hypotheses and establish causal relationships.
- Exploratory research is more flexible and open-ended, while causal research follows a more structured and hypothesis-driven approach.
Correlational vs. Causal Research
Correlational research examines the relationship between variables without implying causation. It identifies patterns of association or co-occurrence between variables but does not establish the direction or causality of the relationship.
Causal research , on the other hand, seeks to establish cause-and-effect relationships between variables by systematically manipulating independent variables and observing their effects on dependent variables. It goes beyond mere association to determine whether changes in one variable directly cause changes in another variable.
- Both correlational and causal research involve analyzing relationships between variables.
- Both types of research contribute to understanding the nature of associations between variables.
- Correlational research focuses on identifying patterns of association, while causal research aims to establish causal relationships.
- Correlational research does not manipulate variables, while causal research involves systematically manipulating independent variables to observe their effects on dependent variables.
How to Formulate Causal Research Hypotheses?
Crafting research questions and hypotheses is the foundational step in any research endeavor. Defining your variables clearly and articulating the causal relationship you aim to investigate is essential. Let's explore this process further.
1. Identify Variables
Identifying variables involves recognizing the key factors you will manipulate or measure in your study. These variables can be classified into independent, dependent, and confounding variables.
- Independent Variable (IV): This is the variable you manipulate or control in your study. It is the presumed cause that you want to test.
- Dependent Variable (DV): The dependent variable is the outcome or response you measure. It is affected by changes in the independent variable.
- Confounding Variables: These are extraneous factors that may influence the relationship between the independent and dependent variables, leading to spurious correlations or erroneous causal inferences. Identifying and controlling for confounding variables is crucial for establishing valid causal relationships.
2. Establish Causality
Establishing causality requires meeting specific criteria outlined by scientific methodology. While correlation between variables may suggest a relationship, it does not imply causation. To establish causality, researchers must demonstrate the following:
- Temporal Precedence: The cause must precede the effect in time. In other words, changes in the independent variable must occur before changes in the dependent variable.
- Covariation of Cause and Effect: Changes in the independent variable should be accompanied by corresponding changes in the dependent variable. This demonstrates a consistent pattern of association between the two variables.
- Elimination of Alternative Explanations: Researchers must rule out other possible explanations for the observed relationship between variables. This involves controlling for confounding variables and conducting rigorous experimental designs to isolate the effects of the independent variable.
3. Write Clear and Testable Hypotheses
Hypotheses serve as tentative explanations for the relationship between variables and provide a framework for empirical testing. A well-formulated hypothesis should be:
- Specific: Clearly state the expected relationship between the independent and dependent variables.
- Testable: The hypothesis should be capable of being empirically tested through observation or experimentation.
- Falsifiable: There should be a possibility of proving the hypothesis false through empirical evidence.
For example, a hypothesis in a study examining the effect of exercise on weight loss could be: "Increasing levels of physical activity (IV) will lead to greater weight loss (DV) among participants (compared to those with lower levels of physical activity)."
By formulating clear hypotheses and operationalizing variables, researchers can systematically investigate causal relationships and contribute to the advancement of scientific knowledge.
Causal Research Design
Designing your research study involves making critical decisions about how you will collect and analyze data to investigate causal relationships.
Experimental vs. Observational Designs
One of the first decisions you'll make when designing a study is whether to employ an experimental or observational design. Each approach has its strengths and limitations, and the choice depends on factors such as the research question, feasibility , and ethical considerations.
- Experimental Design: In experimental designs, researchers manipulate the independent variable and observe its effects on the dependent variable while controlling for confounding variables. Random assignment to experimental conditions allows for causal inferences to be drawn. Example: A study testing the effectiveness of a new teaching method on student performance by randomly assigning students to either the experimental group (receiving the new teaching method) or the control group (receiving the traditional method).
- Observational Design: Observational designs involve observing and measuring variables without intervention. Researchers may still examine relationships between variables but cannot establish causality as definitively as in experimental designs. Example: A study observing the association between socioeconomic status and health outcomes by collecting data on income, education level, and health indicators from a sample of participants.
Control and Randomization
Control and randomization are crucial aspects of experimental design that help ensure the validity of causal inferences.
- Control: Controlling for extraneous variables involves holding constant factors that could influence the dependent variable, except for the independent variable under investigation. This helps isolate the effects of the independent variable. Example: In a medication trial, controlling for factors such as age, gender, and pre-existing health conditions ensures that any observed differences in outcomes can be attributed to the medication rather than other variables.
- Randomization: Random assignment of participants to experimental conditions helps distribute potential confounders evenly across groups, reducing the likelihood of systematic biases and allowing for causal conclusions. Example: Randomly assigning patients to treatment and control groups in a clinical trial ensures that both groups are comparable in terms of baseline characteristics, minimizing the influence of extraneous variables on treatment outcomes.
Internal and External Validity
Two key concepts in research design are internal validity and external validity, which relate to the credibility and generalizability of study findings, respectively.
- Internal Validity: Internal validity refers to the extent to which the observed effects can be attributed to the manipulation of the independent variable rather than confounding factors. Experimental designs typically have higher internal validity due to their control over extraneous variables. Example: A study examining the impact of a training program on employee productivity would have high internal validity if it could confidently attribute changes in productivity to the training intervention.
- External Validity: External validity concerns the extent to which study findings can be generalized to other populations, settings, or contexts. While experimental designs prioritize internal validity, they may sacrifice external validity by using highly controlled conditions that do not reflect real-world scenarios. Example: Findings from a laboratory study on memory retention may have limited external validity if the experimental tasks and conditions differ significantly from real-life learning environments.
Types of Experimental Designs
Several types of experimental designs are commonly used in causal research, each with its own strengths and applications.
- Randomized Control Trials (RCTs): RCTs are considered the gold standard for assessing causality in research. Participants are randomly assigned to experimental and control groups, allowing researchers to make causal inferences. Example: A pharmaceutical company testing a new drug's efficacy would use an RCT to compare outcomes between participants receiving the drug and those receiving a placebo.
- Quasi-Experimental Designs: Quasi-experimental designs lack random assignment but still attempt to establish causality by controlling for confounding variables through design or statistical analysis . Example: A study evaluating the effectiveness of a smoking cessation program might compare outcomes between participants who voluntarily enroll in the program and a matched control group of non-enrollees.
By carefully selecting an appropriate research design and addressing considerations such as control, randomization, and validity, researchers can conduct studies that yield credible evidence of causal relationships and contribute valuable insights to their field of inquiry.
Causal Research Data Collection
Collecting data is a critical step in any research study, and the quality of the data directly impacts the validity and reliability of your findings.
Choosing Measurement Instruments
Selecting appropriate measurement instruments is essential for accurately capturing the variables of interest in your study. The choice of measurement instrument depends on factors such as the nature of the variables, the target population , and the research objectives.
- Surveys : Surveys are commonly used to collect self-reported data on attitudes, opinions, behaviors, and demographics . They can be administered through various methods, including paper-and-pencil surveys, online surveys, and telephone interviews.
- Observations: Observational methods involve systematically recording behaviors, events, or phenomena as they occur in natural settings. Observations can be structured (following a predetermined checklist) or unstructured (allowing for flexible data collection).
- Psychological Tests: Psychological tests are standardized instruments designed to measure specific psychological constructs, such as intelligence, personality traits, or emotional functioning. These tests often have established reliability and validity.
- Physiological Measures: Physiological measures, such as heart rate, blood pressure, or brain activity, provide objective data on bodily processes. They are commonly used in health-related research but require specialized equipment and expertise.
- Existing Databases: Researchers may also utilize existing datasets, such as government surveys, public health records, or organizational databases, to answer research questions. Secondary data analysis can be cost-effective and time-saving but may be limited by the availability and quality of data.
Ensuring accurate data collection is the cornerstone of any successful research endeavor. With the right tools in place, you can unlock invaluable insights to drive your causal research forward. From surveys to tests, each instrument offers a unique lens through which to explore your variables of interest.
At Appinio , we understand the importance of robust data collection methods in informing impactful decisions. Let us empower your research journey with our intuitive platform, where you can effortlessly gather real-time consumer insights to fuel your next breakthrough. Ready to take your research to the next level? Book a demo today and see how Appinio can revolutionize your approach to data collection!
Book a Demo
Sampling Techniques
Sampling involves selecting a subset of individuals or units from a larger population to participate in the study. The goal of sampling is to obtain a representative sample that accurately reflects the characteristics of the population of interest.
- Probability Sampling: Probability sampling methods involve randomly selecting participants from the population, ensuring that each member of the population has an equal chance of being included in the sample. Common probability sampling techniques include simple random sampling , stratified sampling, and cluster sampling .
- Non-Probability Sampling: Non-probability sampling methods do not involve random selection and may introduce biases into the sample. Examples of non-probability sampling techniques include convenience sampling, purposive sampling, and snowball sampling.
The choice of sampling technique depends on factors such as the research objectives, population characteristics, resources available, and practical constraints. Researchers should strive to minimize sampling bias and maximize the representativeness of the sample to enhance the generalizability of their findings.
Ethical Considerations
Ethical considerations are paramount in research and involve ensuring the rights, dignity, and well-being of research participants. Researchers must adhere to ethical principles and guidelines established by professional associations and institutional review boards (IRBs).
- Informed Consent: Participants should be fully informed about the nature and purpose of the study, potential risks and benefits, their rights as participants, and any confidentiality measures in place. Informed consent should be obtained voluntarily and without coercion.
- Privacy and Confidentiality: Researchers should take steps to protect the privacy and confidentiality of participants' personal information. This may involve anonymizing data, securing data storage, and limiting access to identifiable information.
- Minimizing Harm: Researchers should mitigate any potential physical, psychological, or social harm to participants. This may involve conducting risk assessments, providing appropriate support services, and debriefing participants after the study.
- Respect for Participants: Researchers should respect participants' autonomy, diversity, and cultural values. They should seek to foster a trusting and respectful relationship with participants throughout the research process.
- Publication and Dissemination: Researchers have a responsibility to accurately report their findings and acknowledge contributions from participants and collaborators. They should adhere to principles of academic integrity and transparency in disseminating research results.
By addressing ethical considerations in research design and conduct, researchers can uphold the integrity of their work, maintain trust with participants and the broader community, and contribute to the responsible advancement of knowledge in their field.
Causal Research Data Analysis
Once data is collected, it must be analyzed to draw meaningful conclusions and assess causal relationships.
Causal Inference Methods
Causal inference methods are statistical techniques used to identify and quantify causal relationships between variables in observational data. While experimental designs provide the most robust evidence for causality, observational studies often require more sophisticated methods to account for confounding factors.
- Difference-in-Differences (DiD): DiD compares changes in outcomes before and after an intervention between a treatment group and a control group, controlling for pre-existing trends. It estimates the average treatment effect by differencing the changes in outcomes between the two groups over time.
- Instrumental Variables (IV): IV analysis relies on instrumental variables—variables that affect the treatment variable but not the outcome—to estimate causal effects in the presence of endogeneity. IVs should be correlated with the treatment but uncorrelated with the error term in the outcome equation.
- Regression Discontinuity (RD): RD designs exploit naturally occurring thresholds or cutoff points to estimate causal effects near the threshold. Participants just above and below the threshold are compared, assuming that they are similar except for their proximity to the threshold.
- Propensity Score Matching (PSM): PSM matches individuals or units based on their propensity scores—the likelihood of receiving the treatment—creating comparable groups with similar observed characteristics. Matching reduces selection bias and allows for causal inference in observational studies.
Assessing Causality Strength
Assessing the strength of causality involves determining the magnitude and direction of causal effects between variables. While statistical significance indicates whether an observed relationship is unlikely to occur by chance, it does not necessarily imply a strong or meaningful effect.
- Effect Size: Effect size measures the magnitude of the relationship between variables, providing information about the practical significance of the results. Standard effect size measures include Cohen's d for mean differences and odds ratios for categorical outcomes.
- Confidence Intervals: Confidence intervals provide a range of values within which the actual effect size is likely to lie with a certain degree of certainty. Narrow confidence intervals indicate greater precision in estimating the true effect size.
- Practical Significance: Practical significance considers whether the observed effect is meaningful or relevant in real-world terms. Researchers should interpret results in the context of their field and the implications for stakeholders.
Handling Confounding Variables
Confounding variables are extraneous factors that may distort the observed relationship between the independent and dependent variables, leading to spurious or biased conclusions. Addressing confounding variables is essential for establishing valid causal inferences.
- Statistical Control: Statistical control involves including confounding variables as covariates in regression models to partially out their effects on the outcome variable. Controlling for confounders reduces bias and strengthens the validity of causal inferences.
- Matching: Matching participants or units based on observed characteristics helps create comparable groups with similar distributions of confounding variables. Matching reduces selection bias and mimics the randomization process in experimental designs.
- Sensitivity Analysis: Sensitivity analysis assesses the robustness of study findings to changes in model specifications or assumptions. By varying analytical choices and examining their impact on results, researchers can identify potential sources of bias and evaluate the stability of causal estimates.
- Subgroup Analysis: Subgroup analysis explores whether the relationship between variables differs across subgroups defined by specific characteristics. Identifying effect modifiers helps understand the conditions under which causal effects may vary.
By employing rigorous causal inference methods, assessing the strength of causality, and addressing confounding variables, researchers can confidently draw valid conclusions about causal relationships in their studies, advancing scientific knowledge and informing evidence-based decision-making.
Causal Research Examples
Examples play a crucial role in understanding the application of causal research methods and their impact across various domains. Let's explore some detailed examples to illustrate how causal research is conducted and its real-world implications:
Example 1: Software as a Service (SaaS) User Retention Analysis
Suppose a SaaS company wants to understand the factors influencing user retention and engagement with their platform. The company conducts a longitudinal observational study, collecting data on user interactions, feature usage, and demographic information over several months.
- Design: The company employs an observational cohort study design, tracking cohorts of users over time to observe changes in retention and engagement metrics. They use analytics tools to collect data on user behavior , such as logins, feature usage, session duration, and customer support interactions.
- Data Collection: Data is collected from the company's platform logs, customer relationship management (CRM) system, and user surveys. Key metrics include user churn rates, active user counts, feature adoption rates, and Net Promoter Scores ( NPS ).
- Analysis: Using statistical techniques like survival analysis and regression modeling, the company identifies factors associated with user retention, such as feature usage patterns, onboarding experiences, customer support interactions, and subscription plan types.
- Findings: The analysis reveals that users who engage with specific features early in their lifecycle have higher retention rates, while those who encounter usability issues or lack personalized onboarding experiences are more likely to churn. The company uses these insights to optimize product features, improve onboarding processes, and enhance customer support strategies to increase user retention and satisfaction.
Example 2: Business Impact of Digital Marketing Campaign
Consider a technology startup launching a digital marketing campaign to promote its new product offering. The company conducts an experimental study to evaluate the effectiveness of different marketing channels in driving website traffic, lead generation, and sales conversions.
- Design: The company implements an A/B testing design, randomly assigning website visitors to different marketing treatment conditions, such as Google Ads, social media ads, email campaigns, or content marketing efforts. They track user interactions and conversion events using web analytics tools and marketing automation platforms.
- Data Collection: Data is collected on website traffic, click-through rates, conversion rates, lead generation, and sales revenue. The company also gathers demographic information and user feedback through surveys and customer interviews to understand the impact of marketing messages and campaign creatives .
- Analysis: Utilizing statistical methods like hypothesis testing and multivariate analysis, the company compares key performance metrics across different marketing channels to assess their effectiveness in driving user engagement and conversion outcomes. They calculate return on investment (ROI) metrics to evaluate the cost-effectiveness of each marketing channel.
- Findings: The analysis reveals that social media ads outperform other marketing channels in generating website traffic and lead conversions, while email campaigns are more effective in nurturing leads and driving sales conversions. Armed with these insights, the company allocates marketing budgets strategically, focusing on channels that yield the highest ROI and adjusting messaging and targeting strategies to optimize campaign performance.
These examples demonstrate the diverse applications of causal research methods in addressing important questions, informing policy decisions, and improving outcomes in various fields. By carefully designing studies, collecting relevant data, employing appropriate analysis techniques, and interpreting findings rigorously, researchers can generate valuable insights into causal relationships and contribute to positive social change.
How to Interpret Causal Research Results?
Interpreting and reporting research findings is a crucial step in the scientific process, ensuring that results are accurately communicated and understood by stakeholders.
Interpreting Statistical Significance
Statistical significance indicates whether the observed results are unlikely to occur by chance alone, but it does not necessarily imply practical or substantive importance. Interpreting statistical significance involves understanding the meaning of p-values and confidence intervals and considering their implications for the research findings.
- P-values: A p-value represents the probability of obtaining the observed results (or more extreme results) if the null hypothesis is true. A p-value below a predetermined threshold (typically 0.05) suggests that the observed results are statistically significant, indicating that the null hypothesis can be rejected in favor of the alternative hypothesis.
- Confidence Intervals: Confidence intervals provide a range of values within which the true population parameter is likely to lie with a certain degree of confidence (e.g., 95%). If the confidence interval does not include the null value, it suggests that the observed effect is statistically significant at the specified confidence level.
Interpreting statistical significance requires considering factors such as sample size, effect size, and the practical relevance of the results rather than relying solely on p-values to draw conclusions.
Discussing Practical Significance
While statistical significance indicates whether an effect exists, practical significance evaluates the magnitude and meaningfulness of the effect in real-world terms. Discussing practical significance involves considering the relevance of the results to stakeholders and assessing their impact on decision-making and practice.
- Effect Size: Effect size measures the magnitude of the observed effect, providing information about its practical importance. Researchers should interpret effect sizes in the context of their field and the scale of measurement (e.g., small, medium, or large effect sizes).
- Contextual Relevance: Consider the implications of the results for stakeholders, policymakers, and practitioners. Are the observed effects meaningful in the context of existing knowledge, theory, or practical applications? How do the findings contribute to addressing real-world problems or informing decision-making?
Discussing practical significance helps contextualize research findings and guide their interpretation and application in practice, beyond statistical significance alone.
Addressing Limitations and Assumptions
No study is without limitations, and researchers should transparently acknowledge and address potential biases, constraints, and uncertainties in their research design and findings.
- Methodological Limitations: Identify any limitations in study design, data collection, or analysis that may affect the validity or generalizability of the results. For example, sampling biases , measurement errors, or confounding variables.
- Assumptions: Discuss any assumptions made in the research process and their implications for the interpretation of results. Assumptions may relate to statistical models, causal inference methods, or theoretical frameworks underlying the study.
- Alternative Explanations: Consider alternative explanations for the observed results and discuss their potential impact on the validity of causal inferences. How robust are the findings to different interpretations or competing hypotheses?
Addressing limitations and assumptions demonstrates transparency and rigor in the research process, allowing readers to critically evaluate the validity and reliability of the findings.
Communicating Findings Clearly
Effectively communicating research findings is essential for disseminating knowledge, informing decision-making, and fostering collaboration and dialogue within the scientific community.
- Clarity and Accessibility: Present findings in a clear, concise, and accessible manner, using plain language and avoiding jargon or technical terminology. Organize information logically and use visual aids (e.g., tables, charts, graphs) to enhance understanding.
- Contextualization: Provide context for the results by summarizing key findings, highlighting their significance, and relating them to existing literature or theoretical frameworks. Discuss the implications of the findings for theory, practice, and future research directions.
- Transparency: Be transparent about the research process, including data collection procedures, analytical methods, and any limitations or uncertainties associated with the findings. Clearly state any conflicts of interest or funding sources that may influence interpretation.
By communicating findings clearly and transparently, researchers can facilitate knowledge exchange, foster trust and credibility, and contribute to evidence-based decision-making.
Causal Research Tips
When conducting causal research, it's essential to approach your study with careful planning, attention to detail, and methodological rigor. Here are some tips to help you navigate the complexities of causal research effectively:
- Define Clear Research Questions: Start by clearly defining your research questions and hypotheses. Articulate the causal relationship you aim to investigate and identify the variables involved.
- Consider Alternative Explanations: Be mindful of potential confounding variables and alternative explanations for the observed relationships. Take steps to control for confounders and address alternative hypotheses in your analysis.
- Prioritize Internal Validity: While external validity is important for generalizability, prioritize internal validity in your study design to ensure that observed effects can be attributed to the manipulation of the independent variable.
- Use Randomization When Possible: If feasible, employ randomization in experimental designs to distribute potential confounders evenly across experimental conditions and enhance the validity of causal inferences.
- Be Transparent About Methods: Provide detailed descriptions of your research methods, including data collection procedures, analytical techniques, and any assumptions or limitations associated with your study.
- Utilize Multiple Methods: Consider using a combination of experimental and observational methods to triangulate findings and strengthen the validity of causal inferences.
- Be Mindful of Sample Size: Ensure that your sample size is adequate to detect meaningful effects and minimize the risk of Type I and Type II errors. Conduct power analyses to determine the sample size needed to achieve sufficient statistical power.
- Validate Measurement Instruments: Validate your measurement instruments to ensure that they are reliable and valid for assessing the variables of interest in your study. Pilot test your instruments if necessary.
- Seek Feedback from Peers: Collaborate with colleagues or seek feedback from peer reviewers to solicit constructive criticism and improve the quality of your research design and analysis.
Conclusion for Causal Research
Mastering causal research empowers researchers to unlock the secrets of cause and effect, shedding light on the intricate relationships between variables in diverse fields. By employing rigorous methods such as experimental designs, causal inference techniques, and careful data analysis, you can uncover causal mechanisms, predict outcomes, and inform evidence-based practices. Through the lens of causal research, complex phenomena become more understandable, and interventions become more effective in addressing societal challenges and driving progress. In a world where understanding the reasons behind events is paramount, causal research serves as a beacon of clarity and insight. Armed with the knowledge and techniques outlined in this guide, you can navigate the complexities of causality with confidence, advancing scientific knowledge, guiding policy decisions, and ultimately making meaningful contributions to our understanding of the world.
How to Conduct Causal Research in Minutes?
Introducing Appinio , your gateway to lightning-fast causal research. As a real-time market research platform, we're revolutionizing how companies gain consumer insights to drive data-driven decisions. With Appinio, conducting your own market research is not only easy but also thrilling. Experience the excitement of market research with Appinio, where fast, intuitive, and impactful insights are just a click away.
Here's why you'll love Appinio:
- Instant Insights: Say goodbye to waiting days for research results. With our platform, you'll go from questions to insights in minutes, empowering you to make decisions at the speed of business.
- User-Friendly Interface: No need for a research degree here! Our intuitive platform is designed for anyone to use, making complex research tasks simple and accessible.
- Global Reach: Reach your target audience wherever they are. With access to over 90 countries and the ability to define precise target groups from 1200+ characteristics, you'll gather comprehensive data to inform your decisions.
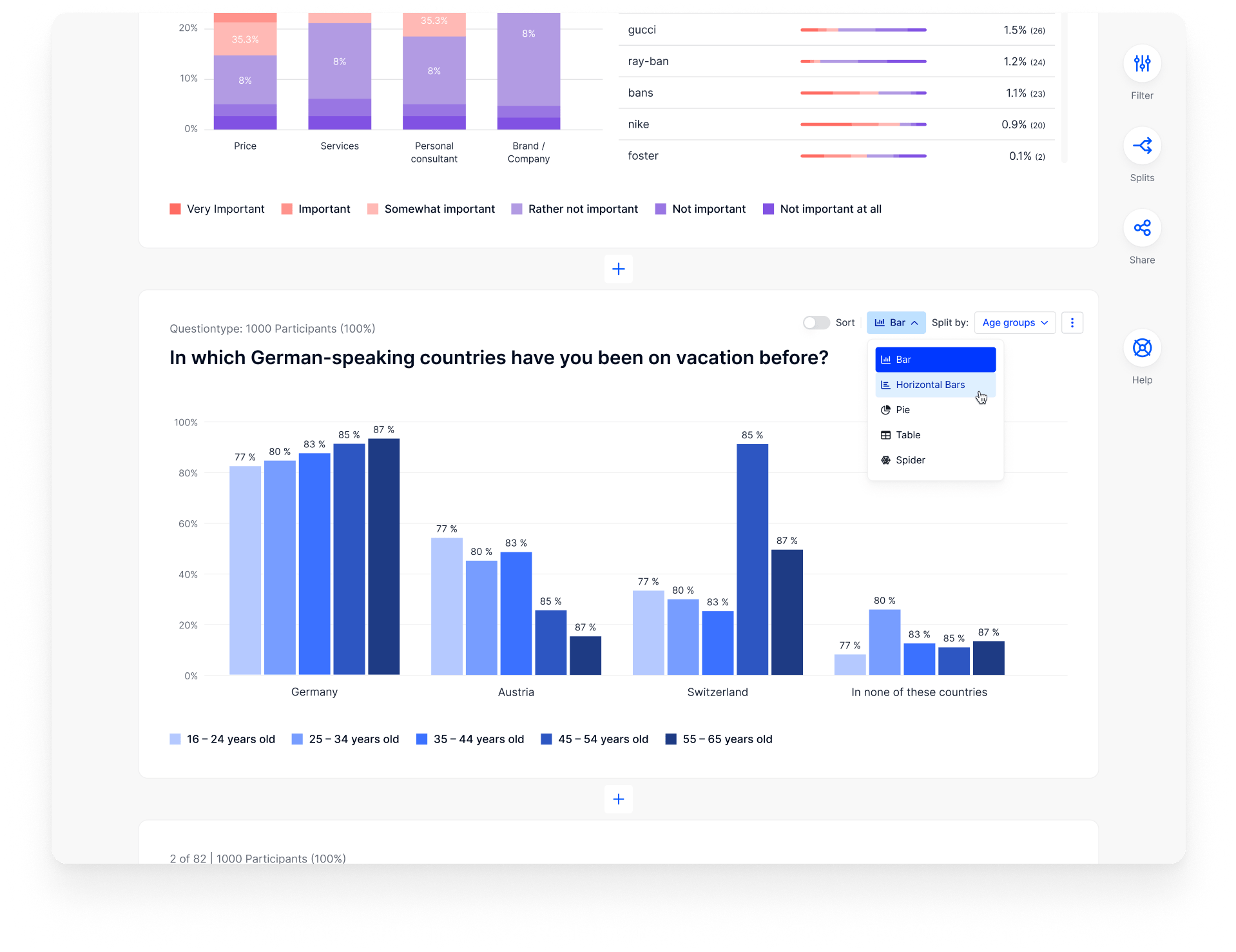
Get free access to the platform!
Join the loop 💌
Be the first to hear about new updates, product news, and data insights. We'll send it all straight to your inbox.
Get the latest market research news straight to your inbox! 💌
Wait, there's more
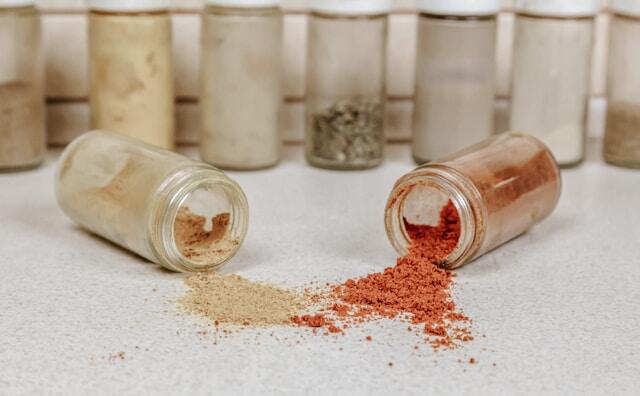
18.06.2024 | 6min read
Future Flavors: How Burger King nailed Concept Testing with Appinio's Predictive Insights
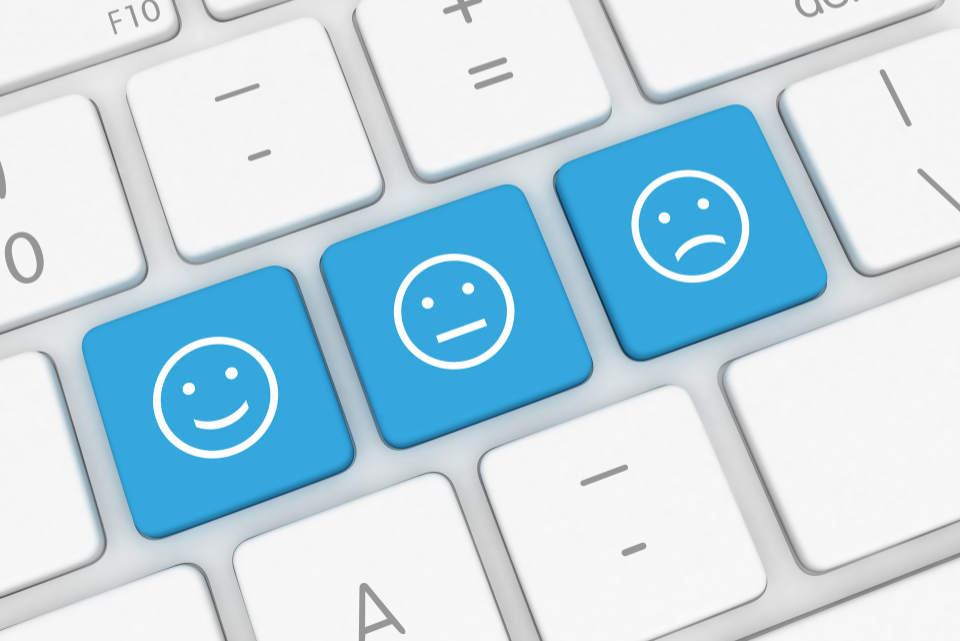
18.06.2024 | 32min read
What is a Pulse Survey? Definition, Types, Questions
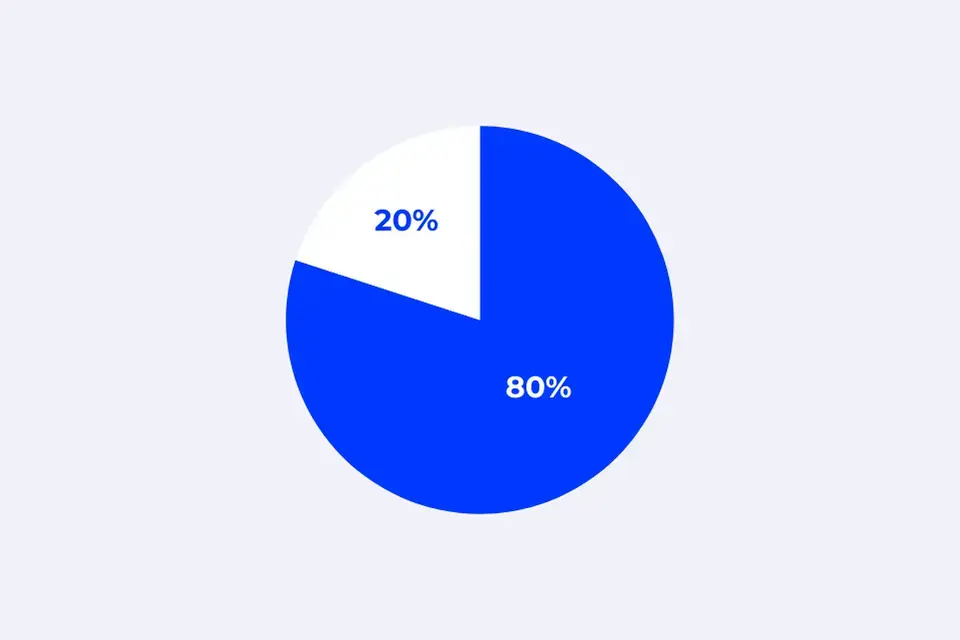
30.05.2024 | 29min read
Pareto Analysis: Definition, Pareto Chart, Examples
What is causal research design?
Last updated
14 May 2023
Reviewed by
Short on time? Get an AI generated summary of this article instead
Examining these relationships gives researchers valuable insights into the mechanisms that drive the phenomena they are investigating.
Organizations primarily use causal research design to identify, determine, and explore the impact of changes within an organization and the market. You can use a causal research design to evaluate the effects of certain changes on existing procedures, norms, and more.
This article explores causal research design, including its elements, advantages, and disadvantages.
Analyze your causal research
Dovetail streamlines causal research analysis to help you uncover and share actionable insights
- Components of causal research
You can demonstrate the existence of cause-and-effect relationships between two factors or variables using specific causal information, allowing you to produce more meaningful results and research implications.
These are the key inputs for causal research:
The timeline of events
Ideally, the cause must occur before the effect. You should review the timeline of two or more separate events to determine the independent variables (cause) from the dependent variables (effect) before developing a hypothesis.
If the cause occurs before the effect, you can link cause and effect and develop a hypothesis .
For instance, an organization may notice a sales increase. Determining the cause would help them reproduce these results.
Upon review, the business realizes that the sales boost occurred right after an advertising campaign. The business can leverage this time-based data to determine whether the advertising campaign is the independent variable that caused a change in sales.
Evaluation of confounding variables
In most cases, you need to pinpoint the variables that comprise a cause-and-effect relationship when using a causal research design. This uncovers a more accurate conclusion.
Co-variations between a cause and effect must be accurate, and a third factor shouldn’t relate to cause and effect.
Observing changes
Variation links between two variables must be clear. A quantitative change in effect must happen solely due to a quantitative change in the cause.
You can test whether the independent variable changes the dependent variable to evaluate the validity of a cause-and-effect relationship. A steady change between the two variables must occur to back up your hypothesis of a genuine causal effect.
- Why is causal research useful?
Causal research allows market researchers to predict hypothetical occurrences and outcomes while enhancing existing strategies. Organizations can use this concept to develop beneficial plans.
Causal research is also useful as market researchers can immediately deduce the effect of the variables on each other under real-world conditions.
Once researchers complete their first experiment, they can use their findings. Applying them to alternative scenarios or repeating the experiment to confirm its validity can produce further insights.
Businesses widely use causal research to identify and comprehend the effect of strategic changes on their profits.
- How does causal research compare and differ from other research types?
Other research types that identify relationships between variables include exploratory and descriptive research .
Here’s how they compare and differ from causal research designs:
Exploratory research
An exploratory research design evaluates situations where a problem or opportunity's boundaries are unclear. You can use this research type to test various hypotheses and assumptions to establish facts and understand a situation more clearly.
You can also use exploratory research design to navigate a topic and discover the relevant variables. This research type allows flexibility and adaptability as the experiment progresses, particularly since no area is off-limits.
It’s worth noting that exploratory research is unstructured and typically involves collecting qualitative data . This provides the freedom to tweak and amend the research approach according to your ongoing thoughts and assessments.
Unfortunately, this exposes the findings to the risk of bias and may limit the extent to which a researcher can explore a topic.
This table compares the key characteristics of causal and exploratory research:
|
|
|
Main research statement | Research hypotheses | Research question |
Amount of uncertainty characterizing decision situation | Clearly defined | Highly ambiguous |
Research approach | Highly structured | Unstructured |
When you conduct it | Later stages of decision-making | Early stages of decision-making |
Descriptive research
This research design involves capturing and describing the traits of a population, situation, or phenomenon. Descriptive research focuses more on the " what " of the research subject and less on the " why ."
Since descriptive research typically happens in a real-world setting, variables can cross-contaminate others. This increases the challenge of isolating cause-and-effect relationships.
You may require further research if you need more causal links.
This table compares the key characteristics of causal and descriptive research.
|
|
|
Main research statement | Research hypotheses | Research question |
Amount of uncertainty characterizing decision situation | Clearly defined | Partially defined |
Research approach | Highly structured | Structured |
When you conduct it | Later stages of decision-making | Later stages of decision-making |
Causal research examines a research question’s variables and how they interact. It’s easier to pinpoint cause and effect since the experiment often happens in a controlled setting.
Researchers can conduct causal research at any stage, but they typically use it once they know more about the topic.
In contrast, causal research tends to be more structured and can be combined with exploratory and descriptive research to help you attain your research goals.
- How can you use causal research effectively?
Here are common ways that market researchers leverage causal research effectively:
Market and advertising research
Do you want to know if your new marketing campaign is affecting your organization positively? You can use causal research to determine the variables causing negative or positive impacts on your campaign.
Improving customer experiences and loyalty levels
Consumers generally enjoy purchasing from brands aligned with their values. They’re more likely to purchase from such brands and positively represent them to others.
You can use causal research to identify the variables contributing to increased or reduced customer acquisition and retention rates.
Could the cause of increased customer retention rates be streamlined checkout?
Perhaps you introduced a new solution geared towards directly solving their immediate problem.
Whatever the reason, causal research can help you identify the cause-and-effect relationship. You can use this to enhance your customer experiences and loyalty levels.
Improving problematic employee turnover rates
Is your organization experiencing skyrocketing attrition rates?
You can leverage the features and benefits of causal research to narrow down the possible explanations or variables with significant effects on employees quitting.
This way, you can prioritize interventions, focusing on the highest priority causal influences, and begin to tackle high employee turnover rates.
- Advantages of causal research
The main benefits of causal research include the following:
Effectively test new ideas
If causal research can pinpoint the precise outcome through combinations of different variables, researchers can test ideas in the same manner to form viable proof of concepts.
Achieve more objective results
Market researchers typically use random sampling techniques to choose experiment participants or subjects in causal research. This reduces the possibility of exterior, sample, or demography-based influences, generating more objective results.
Improved business processes
Causal research helps businesses understand which variables positively impact target variables, such as customer loyalty or sales revenues. This helps them improve their processes, ROI, and customer and employee experiences.
Guarantee reliable and accurate results
Upon identifying the correct variables, researchers can replicate cause and effect effortlessly. This creates reliable data and results to draw insights from.
Internal organization improvements
Businesses that conduct causal research can make informed decisions about improving their internal operations and enhancing employee experiences.
- Disadvantages of causal research
Like any other research method, casual research has its set of drawbacks that include:
Extra research to ensure validity
Researchers can't simply rely on the outcomes of causal research since it isn't always accurate. There may be a need to conduct other research types alongside it to ensure accurate output.
Coincidence
Coincidence tends to be the most significant error in causal research. Researchers often misinterpret a coincidental link between a cause and effect as a direct causal link.
Administration challenges
Causal research can be challenging to administer since it's impossible to control the impact of extraneous variables .
Giving away your competitive advantage
If you intend to publish your research, it exposes your information to the competition.
Competitors may use your research outcomes to identify your plans and strategies to enter the market before you.
- Causal research examples
Multiple fields can use causal research, so it serves different purposes, such as.
Customer loyalty research
Organizations and employees can use causal research to determine the best customer attraction and retention approaches.
They monitor interactions between customers and employees to identify cause-and-effect patterns. That could be a product demonstration technique resulting in higher or lower sales from the same customers.
Example: Business X introduces a new individual marketing strategy for a small customer group and notices a measurable increase in monthly subscriptions.
Upon getting identical results from different groups, the business concludes that the individual marketing strategy resulted in the intended causal relationship.
Advertising research
Businesses can also use causal research to implement and assess advertising campaigns.
Example: Business X notices a 7% increase in sales revenue a few months after a business introduces a new advertisement in a certain region. The business can run the same ad in random regions to compare sales data over the same period.
This will help the company determine whether the ad caused the sales increase. If sales increase in these randomly selected regions, the business could conclude that advertising campaigns and sales share a cause-and-effect relationship.
Educational research
Academics, teachers, and learners can use causal research to explore the impact of politics on learners and pinpoint learner behavior trends.
Example: College X notices that more IT students drop out of their program in their second year, which is 8% higher than any other year.
The college administration can interview a random group of IT students to identify factors leading to this situation, including personal factors and influences.
With the help of in-depth statistical analysis, the institution's researchers can uncover the main factors causing dropout. They can create immediate solutions to address the problem.
Is a causal variable dependent or independent?
When two variables have a cause-and-effect relationship, the cause is often called the independent variable. As such, the effect variable is dependent, i.e., it depends on the independent causal variable. An independent variable is only causal under experimental conditions.
What are the three criteria for causality?
The three conditions for causality are:
Temporality/temporal precedence: The cause must precede the effect.
Rationality: One event predicts the other with an explanation, and the effect must vary in proportion to changes in the cause.
Control for extraneous variables: The covariables must not result from other variables.
Is causal research experimental?
Causal research is mostly explanatory. Causal studies focus on analyzing a situation to explore and explain the patterns of relationships between variables.
Further, experiments are the primary data collection methods in studies with causal research design. However, as a research design, causal research isn't entirely experimental.
What is the difference between experimental and causal research design?
One of the main differences between causal and experimental research is that in causal research, the research subjects are already in groups since the event has already happened.
On the other hand, researchers randomly choose subjects in experimental research before manipulating the variables.
Should you be using a customer insights hub?
Do you want to discover previous research faster?
Do you share your research findings with others?
Do you analyze research data?
Start for free today, add your research, and get to key insights faster
Editor’s picks
Last updated: 18 April 2023
Last updated: 27 February 2023
Last updated: 6 February 2023
Last updated: 15 January 2024
Last updated: 6 October 2023
Last updated: 5 February 2023
Last updated: 16 April 2023
Last updated: 7 March 2023
Last updated: 9 March 2023
Last updated: 12 December 2023
Last updated: 11 March 2024
Last updated: 13 May 2024
Latest articles
Related topics, .css-je19u9{-webkit-align-items:flex-end;-webkit-box-align:flex-end;-ms-flex-align:flex-end;align-items:flex-end;display:-webkit-box;display:-webkit-flex;display:-ms-flexbox;display:flex;-webkit-flex-direction:row;-ms-flex-direction:row;flex-direction:row;-webkit-box-flex-wrap:wrap;-webkit-flex-wrap:wrap;-ms-flex-wrap:wrap;flex-wrap:wrap;-webkit-box-pack:center;-ms-flex-pack:center;-webkit-justify-content:center;justify-content:center;row-gap:0;text-align:center;max-width:671px;}@media (max-width: 1079px){.css-je19u9{max-width:400px;}.css-je19u9>span{white-space:pre;}}@media (max-width: 799px){.css-je19u9{max-width:400px;}.css-je19u9>span{white-space:pre;}} decide what to .css-1kiodld{max-height:56px;display:-webkit-box;display:-webkit-flex;display:-ms-flexbox;display:flex;-webkit-align-items:center;-webkit-box-align:center;-ms-flex-align:center;align-items:center;}@media (max-width: 1079px){.css-1kiodld{display:none;}} build next, decide what to build next.

Users report unexpectedly high data usage, especially during streaming sessions.

Users find it hard to navigate from the home page to relevant playlists in the app.

It would be great to have a sleep timer feature, especially for bedtime listening.

I need better filters to find the songs or artists I’m looking for.
Log in or sign up
Get started for free
- What is Causal Research? Definition + Key Elements

Cause-and-effect relationships happen in all aspects of life, from business to medicine, to marketing, to education, and so much more. They are the invisible threads that connect both our actions and inactions to their outcomes.
Causal research is the type of research that investigates cause-and-effect relationships. It is more comprehensive than descriptive research, which just talks about how things affect each other.
Let’s take a closer look at how you can use informal research to gain insight into your research results and make more informed decisions.

Defining Causal Research
Causal research investigates why one variable (the independent variable) is causing things to change in another ( the dependent variable).
For example, a causal research study about the cause-and-effect relationship between smoking and the prevalence of lung cancer. Smoking prevalence would be the independent variable, while lung cancer prevalence would be the dependent variable.
You would establish that smoking causes lung cancer by modulating the independent variable (smoking) and observing the effects on the dependent variable (lung cancer).
What’s the Difference Between Correlation and Causation
Correlation simply means that two variables are related to each other. But it does not necessarily mean that one variable causes changes in the other.
For example, let’s say there is a correlation between high coffee sales and low ice cream sales. This does not mean that people are not buying ice cream because they prefer coffee.
Both of these variables correlate because they’re influenced by the same factor: cold weather.
The Need for Causal Research

The major reason for investigating causal relationships between variables is better decision-making , which leads to developing effective solutions to complex problems. Here’s a breakdown of how it works:
- Decision-Making
Causal research enables us to figure out how variables relate to each other and how a change in one variable affects another. This helps us make better decisions about resource allocation, problem-solving, and achieving our goals.
In business, for example, customer satisfaction (independent variable) directly impacts sales (dependent variable). If customers are happy with your product or service, they’re more likely to keep returning and recommending it to their friends, which translates into more sales.
- Developing Effective Solutions to Problems
Understanding the causes of a problem, allows you to develop more effective solutions to address it. For example, medical causal research enables you to understand symptoms better, create new prevention strategies, and provide more effective treatment for illnesses.

Examples of Where Causal Relationships Are Critical
Here are a couple of ways you can leverage causal research:
- Policy-making : Causal research informs policy decisions about issues such as education, healthcare, and the environment. Let’s say causal research shows that the availability of junk food in schools directly impacts the prevalence of obesity in teenagers. This would inform the decision to incorporate more healthy food options in schools.
- Marketing strategies : Causal research studies allow you to identify factors that influence customer behavior to develop effective marketing strategies. For example, you can use causal research to reach and attract your target audience with the right content.
- Product development : Causal research enables you to create successful products by understanding users’ pain points and providing products that meet these needs.

Key Elements of Causal Research
Let’s take a deep dive into what it takes to design and conduct a causal study:
- Control and Experimental Groups
In a controlled study, the researchers randomly put people into one of two groups: the control group, who don’t get the treatment, or the experimental group, who do.
Having a control group allows you to compare the effects of the treatment to the effects of no treatment. It enables you to rule out the possibility that any changes in the dependent variable are due to factors other than the treatment.
- Independent variable : The independent variable is the variable that affects the dependent variable. It is the variable that you alter to see the effect on the dependent variable.
- Dependent variable : The dependent variable is the variable that is affected by the independent variable. This is what you measure to see the impact of the independent variable.
An Illustration of How Independent vs Dependent Variable Works in Causal Research
Here’s an illustration to help you understand how to differentiate and use variables in causal research:
Let’s say you want to investigate “ the effect of dieting on weight loss ”, dieting would be the independent variable, and weight loss would be the dependent variable. Next, you would vary the independent variable (dieting) by assigning some participants to a restricted diet and others to a control group.
You will see the cause-and-effect relationship between dieting and weight loss by measuring the dependent variable (weight loss) in both groups.
Skip the setup hassle! Get a head start on your research with our ready-to-use Experimental Research Survey Template
Research Designs for Establishing Causality
There are several ways to investigate the relationship between variables, but here are the most common:
A. Experimental Design
Experimental designs are the gold standard for establishing causality. In an experimental design, the researcher randomly assigns participants to either a control group or an experimental group. The control group does not receive the treatment, while the experimental group does.
Pros of experimental designs :
- Highly rigorous
- Explicitly establishes causality
- Strictly controls for extraneous variables
- Time-consuming and expensive
- Difficult to implement in real-world settings
- Not always ethical
B. Quasi-Experimental Design
A quasi-experimental design attempts to determine the causal relationship without fully randomizing the participant distribution into groups. The primary reason for this is ethical or practical considerations.
Different types of quasi-experimental designs
- Time series design : This design involves collecting data over time on the same group of participants. You see the cause-and-effect relationship by identifying the changes in the dependent variable that coincide with changes in the independent variable.
- Nonequivalent control group design : This design involves comparing an experimental group to a control group that is not randomly assigned. The differences between the two groups explain the cause-and-effect relationship.
- Interrupted time series design : Unlike the time series that measures changes over time, this introduces treatment at a specific point in time. You figure out the relationship between treatment and the dependent variable by looking for any changes that occurred at the time the treatment was introduced.
Pros of quasi-experimental designs
- Cost-effective
- More feasible to implement in real-world settings
- More ethical than experimental designs
- Not as thorough as experimental designs
- May not accurately establish causality
- More susceptible to bias
Establishing Causality without Experiments
Using experiments to determine the cause-and-effect relationship between each dependent variable and the independent variable can be time-consuming and expensive. As a result, the following are cost-effective methods for establishing a causal relationship:
- Longitudinal Studies
Long-term studies are observational studies that follow the same participants or groups over a long period. This way, you can see changes in variables you’re studying over time, and establish a causal relationship between them.
For example, you can use a longitudinal study to determine the effect of a new education program on student performance. You then track students’ academic performance over the years to see if the program improved student performance.
Challenges of Longitudinal Studies
One of the biggest problems of longitudinal studies is confounding variables. These are factors that are related to both the independent variable and the dependent variable.
Confounding variables can make it hard to isolate the cause of an independent variable’s effect. Using the earlier example, if you’re looking at how new educational programs affect student success, you need to make sure you’re controlling for factors such as students’ socio-economic background and their prior academic performance.
- Instrumental Variables (IV) Analysis
Instrumental variable analysis (IV) is a statistical approach that enables you to estimate causal effects in observational studies. An instrumental variable is a variable that is correlated with the independent variable but is not correlated with the dependent variable except through the independent variable.
For example, in academic achievement research, an instrumental variable could be the distance to the nearest college. This variable is correlated with family income but doesn’t correlate with academic achievement except through family income.
Challenges of Instrumental Variables (IV) Analysis
A primary limitation of IV analysis is that it can be challenging to find a good instrumental variable. IV analysis can also be very sensitive to the assumptions of the model.
Challenges and Pitfalls

It is a powerful tool for solving problems, making better decisions, and advancing human knowledge. However, causal research is not without its challenges and pitfalls.
- Confounding Variables
A confounding variable is a variable that correlates with both the independent and dependent variables, and it can make it difficult to isolate the causal effect of the independent variable.
For example, let’s say you are interested in the causal effect of smoking on lung cancer. If you simply compare smokers to nonsmokers, you may find that smokers are more likely to get lung cancer.
However, the relationship between smoking and lung cancer may be confounded by other factors, such as age, socioeconomic status, or exposure to secondhand smoke. These other factors may be responsible for the increased risk of lung cancer in smokers, rather than smoking itself.
Unlock the research secrets that top professionals use: Get the facts you need about Desk Research here
Strategy to Control for Confounding Variables
Confounding variables can lead to misleading results and make it difficult to determine the cause-and-effect between variables. Here are some strategies that allow you to control for confounding variables and improve the reliability of causal research findings:
- Randomized Controlled Trial (RCT)
In an RCT, participants are randomly assigned to either the treatment group or the control group. This ensures that the two groups are comparable on all confounding variables, except for the treatment itself.
- Statistical Methods
Using statistical methods such as multivariate regression analysis allows you to control for multiple confounding variables simultaneously.
Reverse Causation
Reverse Causation is when the relationship between the cause and effect of variables is reversed.
For example, let’s say you want to find a correlation between education and income. You’d expect people with higher levels of education to earn more, right?
Well, what if it’s the other way around? What if people with higher income are only more college-educated because they can afford it and lower-income people can’t?
Strategy to Control for Reverse Causation
Here are some ways to prevent and mitigate the effect of reverse causation:
- Longitudinal study
A longitudinal study follows the same individuals or groups over time. This allows researchers to see how changes in one variable (e.g., education) are associated with changes in another variable (e.g., income) over time.
- Instrumental Variables Analysis
Instrumental variables analysis is a statistical technique that estimates the causal effect of a variable when there is reverse causation.
Real-World Applications
Causal research allows us to identify the root causes of problems and develop solutions that work. Here are some examples of the real-world applications of causal research:
- Healthcare Research:
Causal research enables healthcare professionals to figure out what causes diseases and how to treat them.
For example, medical researchers can use casual research to figure out if a drug or treatment is effective for a specific condition. It also helps determine what causes certain diseases.
Randomized controlled trials (RCTs) are widely regarded as the standard for determining causal relationships in healthcare research. They have been used to determine the effects of multiple medical interventions, such as the effectiveness of new drugs and vaccines, surgery, as well as lifestyle changes on health.
- Public Policy Impact
Causal research can also be used to inform public policy decisions. For example, a causal study showed that early childhood education for disadvantaged children improved their academic performance and reduced their likelihood of dropping out. This has been leveraged to support policies that increase early childhood education access.
You can also use causal research to see if existing policies are working. For example, a causal study proves that giving ex-offenders job training reduces their chances of reoffending. The governments would be motivated to set up, fund, and mandate ex-offenders to take training programs.
Understanding causal effects helps us make informed decisions across different fields such as health, business, lifestyle, public policy, and more. But, this research method has its challenges and limitations.
Using the best practices and strategies in this guide can help you mitigate the limitations of causal research. Start your journey to seamlessly collecting valid data for your research with Formplus .
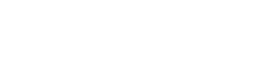
Connect to Formplus, Get Started Now - It's Free!
- casual research
- research design
- Moradeke Owa
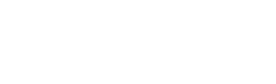
You may also like:
Projective Techniques In Surveys: Definition, Types & Pros & Cons
Introduction When you’re conducting a survey, you need to find out what people think about things. But how do you get an accurate and...

Desk Research: Definition, Types, Application, Pros & Cons
If you are looking for a way to conduct a research study while optimizing your resources, desk research is a great option. Desk research...
Writing Research Proposals: Tips, Examples & Mistakes
In this article, we’ll discover several tips for writing an effective research proposal and common pitfalls you should look out for.
43 Market Research Terminologies You Need To Know
Introduction Market research is a process of gathering information to determine the needs, wants, or behaviors of consumers or...
Formplus - For Seamless Data Collection
Collect data the right way with a versatile data collection tool. try formplus and transform your work productivity today..
Establishing Cause and Effect
A central goal of most research is the identification of causal relationships, or demonstrating that a particular independent variable (the cause) has an effect on the dependent variable of interest (the effect). The three criteria for establishing cause and effect – association, time ordering (or temporal precedence), and non-spuriousness – are familiar to most researchers from courses in research methods or statistics. While the classic examples used to illustrate these criteria may imply that establishing cause and effect is straightforward, it is often one of the most challenging aspects of designing research studies for implementation in real world conditions.
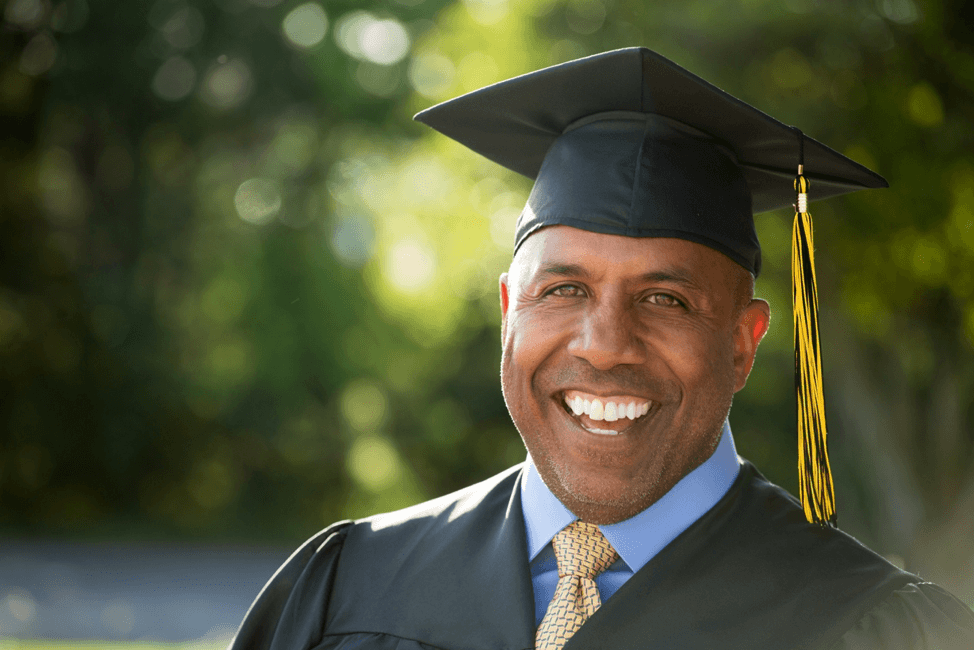
Discover How We Assist to Edit Your Dissertation Chapters
Aligning theoretical framework, gathering articles, synthesizing gaps, articulating a clear methodology and data plan, and writing about the theoretical and practical implications of your research are part of our comprehensive dissertation editing services.
- Bring dissertation editing expertise to chapters 1-5 in timely manner.
- Track all changes, then work with you to bring about scholarly writing.
- Ongoing support to address committee feedback, reducing revisions.
The first step in establishing causality is demonstrating association; simply put, is there a relationship between the independent variable and the dependent variable? If both variables are numeric, this can be established by looking at the correlation between the two to determine if they appear to convey. A common example is the relationship between education and income: in general, individuals with more years of education are also likely to earn higher incomes. Cross tabulation, which cross-classifies the distributions of two categorical variables, can also be used to examination association. For example, we may observe that 60% of Protestants support the death penalty while only 35% of Catholics do so, establishing an association between denomination and attitudes toward capital punishment. There is ongoing debate regarding just how closely associated variables must be to make a causal claim, but in general researchers are more concerned with the statistical significance of an association (whether it is likely to exist in the population) than with the actual strength of the association.
Once an association has been established, our attention turns to determining the time order of the variables of interest. In order for the independent variable to cause the dependent variable, logic dictates that the independent variable must occur first in time; in short, the cause must come before the effect. This time ordering is easy to ensure in an experimental design where the researcher carefully controls exposure to the treatment (which would be the independent variable) and then measures the outcome of interest (the dependent variable). In cross-sectional designs the time ordering can be much more difficult to determine, especially when the relationship between variables could reasonably go in the opposite direction. For example, although education usually precedes income, it is possible that individuals who are making a good living may finally have the money necessary to return to school. Determining time ordering thus may involve using logic, existing research, and common sense when a controlled experimental design is not possible. In any case, researchers must be very careful about specifying the hypothesized direction of the relationship between the variables and provide evidence (either theoretical or empirical) to support their claim.
The third criterion for causality is also the most troublesome, as it requires that alternative explanations for the observed relationship between two variables be ruled out. This is termed non-spuriousness, which simply means “not false.” A spurious or false relationship exists when what appears to be an association between the two variables is actually caused by a third extraneous variable. Classic examples of spuriousness include the relationship between children’s shoe sizes and their academic knowledge: as shoe size increases so does knowledge, but of course both are also strongly related to age. Another well-known example is the relationship between the number of fire fighters that respond to a fire and the amount of damage that results – clearly, the size of the fire determines both, so it is inaccurate to say that more fire fighters cause greater damage. Though these examples seem straightforward, researchers in the fields of psychology, education, and the social sciences often face much greater challenges in ruling out spurious relationships simply because there are so many other factors that might influence the relationship between two variables. Appropriate study design (using experimental procedures whenever possible), careful data collection and use of statistical controls, and triangulation of many data sources are all essential when seeking to establish non-spurious relationships between variables.
- Skip to main content
- Skip to primary sidebar
- Skip to footer
- QuestionPro

- Solutions Industries Gaming Automotive Sports and events Education Government Travel & Hospitality Financial Services Healthcare Cannabis Technology Use Case NPS+ Communities Audience Contactless surveys Mobile LivePolls Member Experience GDPR Positive People Science 360 Feedback Surveys
- Resources Blog eBooks Survey Templates Case Studies Training Help center

Home Market Research Research Tools and Apps
Causal Research: What it is, Tips & Examples
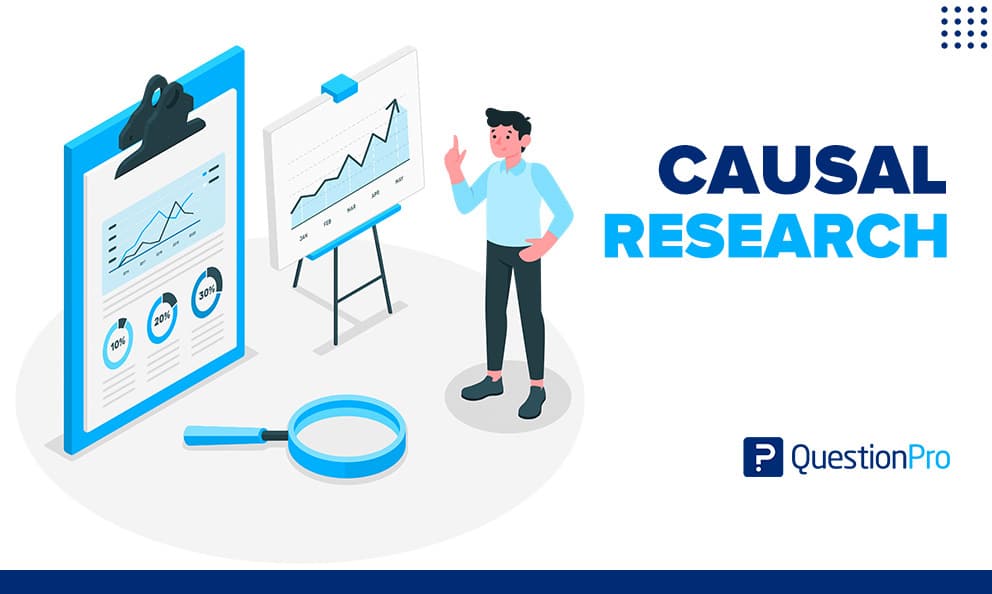
Causal research is classified as conclusive research since it attempts to build a cause-and-effect link between two variables. This research is mainly used to determine the cause of particular behavior. We can use this research to determine what changes occur in an independent variable due to a change in the dependent variable.
It can assist you in evaluating marketing activities, improving internal procedures, and developing more effective business plans. Understanding how one circumstance affects another may help you determine the most effective methods for satisfying your business needs.
LEARN ABOUT: Behavioral Research
This post will explain causal research, define its essential components, describe its benefits and limitations, and provide some important tips.
Content Index
What is causal research?
Temporal sequence, non-spurious association, concomitant variation, the advantages, the disadvantages, causal research examples, causal research tips.
Causal research is also known as explanatory research . It’s a type of research that examines if there’s a cause-and-effect relationship between two separate events. This would occur when there is a change in one of the independent variables, which is causing changes in the dependent variable.
You can use causal research to evaluate the effects of particular changes on existing norms, procedures, and so on. This type of research examines a condition or a research problem to explain the patterns of interactions between variables.
LEARN ABOUT: Research Process Steps
Components of causal research
Only specific causal information can demonstrate the existence of cause-and-effect linkages. The three key components of causal research are as follows:
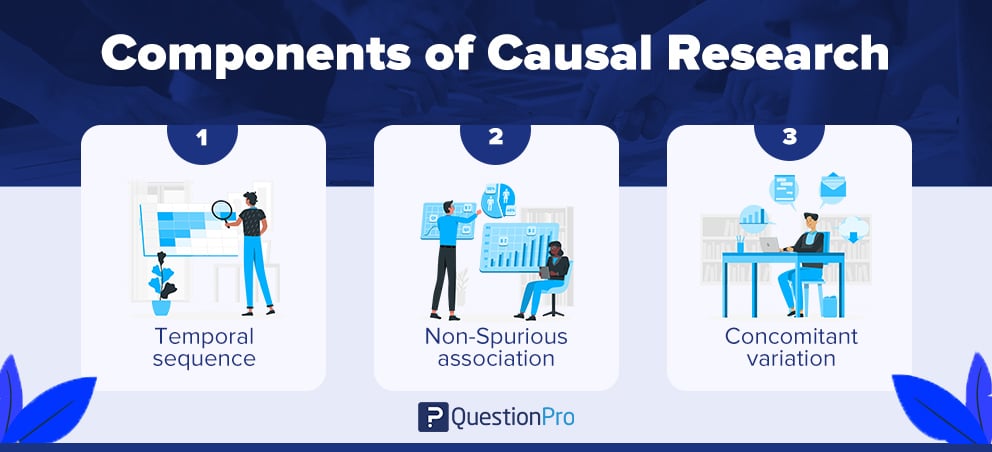
Prior to the effect, the cause must occur. If the cause occurs before the appearance of the effect, the cause and effect can only be linked. For example, if the profit increase occurred before the advertisement aired, it cannot be linked to an increase in advertising spending.
Linked fluctuations between two variables are only allowed if there is no other variable that is related to both cause and effect. For example, a notebook manufacturer has discovered a correlation between notebooks and the autumn season. They see that during this season, more people buy notebooks because students are buying them for the upcoming semester.
During the summer, the company launched an advertisement campaign for notebooks. To test their assumption, they can look up the campaign data to see if the increase in notebook sales was due to the student’s natural rhythm of buying notebooks or the advertisement.
Concomitant variation is defined as a quantitative change in effect that happens solely as a result of a quantitative change in the cause. This means that there must be a steady change between the two variables. You can examine the validity of a cause-and-effect connection by seeing if the independent variable causes a change in the dependent variable.
For example, if any company does not make an attempt to enhance sales by acquiring skilled employees or offering training to them, then the hire of experienced employees cannot be credited for an increase in sales. Other factors may have contributed to the increase in sales.
Causal Research Advantages and Disadvantages
Causal or explanatory research has various advantages for both academics and businesses. As with any other research method, it has a few disadvantages that researchers should be aware of. Let’s look at some of the advantages and disadvantages of this research design .
- Helps in the identification of the causes of system processes. This allows the researcher to take the required steps to resolve issues or improve outcomes.
- It provides replication if it is required.
- Causal research assists in determining the effects of changing procedures and methods.
- Subjects are chosen in a methodical manner. As a result, it is beneficial for improving internal validity .
- The ability to analyze the effects of changes on existing events, processes, phenomena, and so on.
- Finds the sources of variable correlations, bridging the gap in correlational research .
- It is not always possible to monitor the effects of all external factors, so causal research is challenging to do.
- It is time-consuming and might be costly to execute.
- The effect of a large range of factors and variables existing in a particular setting makes it difficult to draw results.
- The most major error in this research is a coincidence. A coincidence between a cause and an effect can sometimes be interpreted as a direction of causality.
- To corroborate the findings of the explanatory research , you must undertake additional types of research. You can’t just make conclusions based on the findings of a causal study.
- It is sometimes simple for a researcher to see that two variables are related, but it can be difficult for a researcher to determine which variable is the cause and which variable is the effect.
Since different industries and fields can carry out causal comparative research , it can serve many different purposes. Let’s discuss 3 examples of causal research:
Advertising Research
Companies can use causal research to enact and study advertising campaigns. For example, six months after a business debuts a new ad in a region. They see a 5% increase in sales revenue.
To assess whether the ad has caused the lift, they run the same ad in randomly selected regions so they can compare sales data across regions over another six months. When sales pick up again in these regions, they can conclude that the ad and sales have a valuable cause-and-effect relationship.
LEARN ABOUT: Ad Testing
Customer Loyalty Research
Businesses can use causal research to determine the best customer retention strategies. They monitor interactions between associates and customers to identify patterns of cause and effect, such as a product demonstration technique leading to increased or decreased sales from the same customers.
For example, a company implements a new individual marketing strategy for a small group of customers and sees a measurable increase in monthly subscriptions. After receiving identical results from several groups, they concluded that the one-to-one marketing strategy has the causal relationship they intended.
Educational Research
Learning specialists, academics, and teachers use causal research to learn more about how politics affects students and identify possible student behavior trends. For example, a university administration notices that more science students drop out of their program in their third year, which is 7% higher than in any other year.
They interview a random group of science students and discover many factors that could lead to these circumstances, including non-university components. Through the in-depth statistical analysis, researchers uncover the top three factors, and management creates a committee to address them in the future.
Causal research is frequently the last type of research done during the research process and is considered definitive. As a result, it is critical to plan the research with specific parameters and goals in mind. Here are some tips for conducting causal research successfully:
1. Understand the parameters of your research
Identify any design strategies that change the way you understand your data. Determine how you acquired data and whether your conclusions are more applicable in practice in some cases than others.
2. Pick a random sampling strategy
Choosing a technique that works best for you when you have participants or subjects is critical. You can use a database to generate a random list, select random selections from sorted categories, or conduct a survey.
3. Determine all possible relations
Examine the different relationships between your independent and dependent variables to build more sophisticated insights and conclusions.
To summarize, causal or explanatory research helps organizations understand how their current activities and behaviors will impact them in the future. This is incredibly useful in a wide range of business scenarios. This research can ensure the outcome of various marketing activities, campaigns, and collaterals. Using the findings of this research program, you will be able to design more successful business strategies that take advantage of every business opportunity.
At QuestionPro, we offer all kinds of necessary tools for researchers to carry out their projects. It can help you get the most out of your data by guiding you through the process.
MORE LIKE THIS
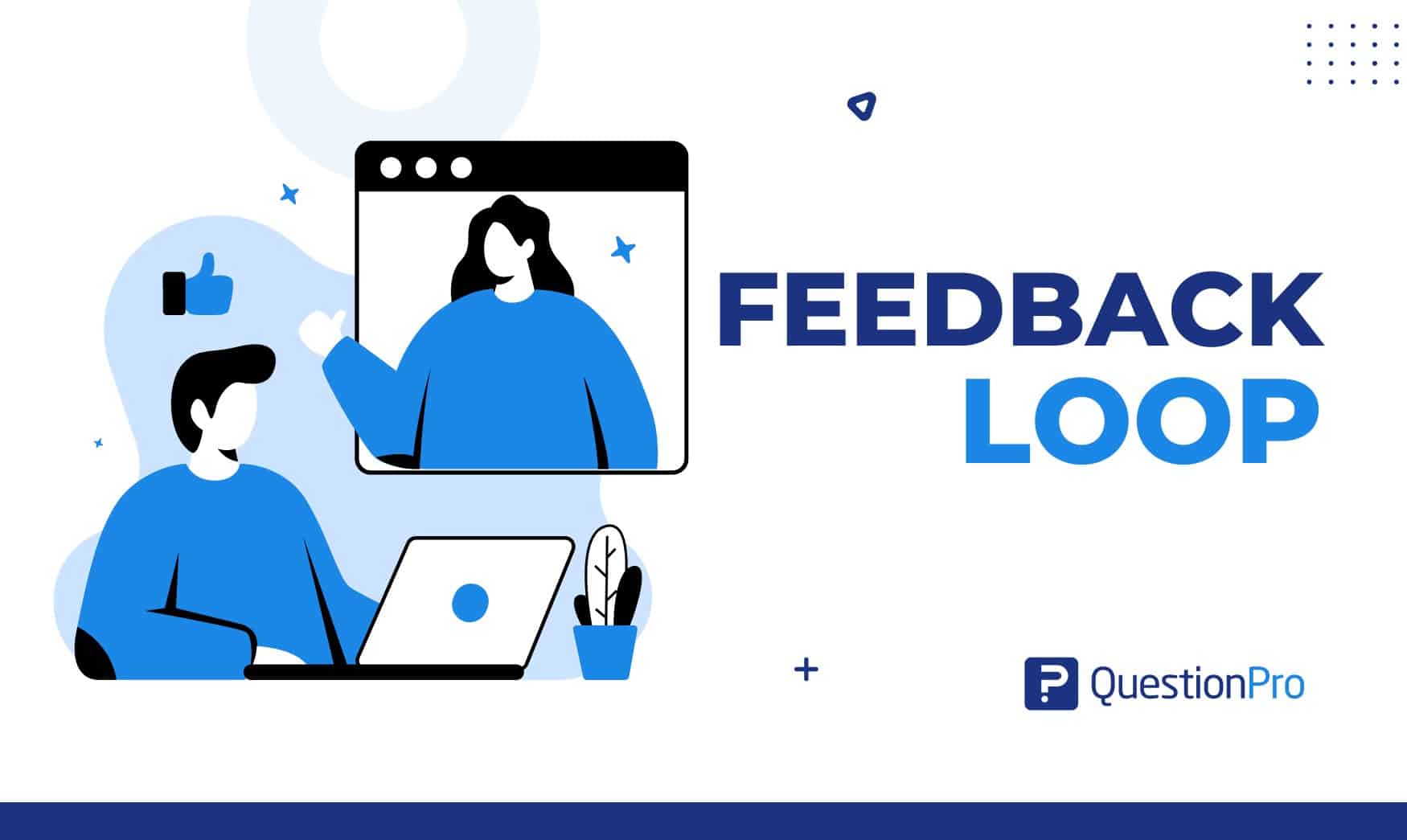
Feedback Loop: What It Is, Types & How It Works?
Jun 21, 2024
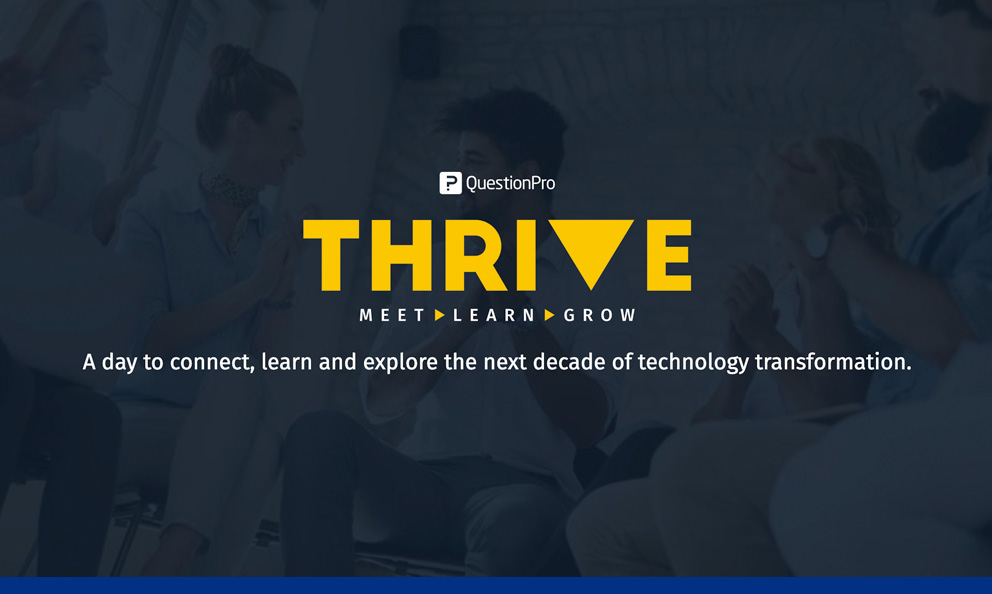
QuestionPro Thrive: A Space to Visualize & Share the Future of Technology
Jun 18, 2024
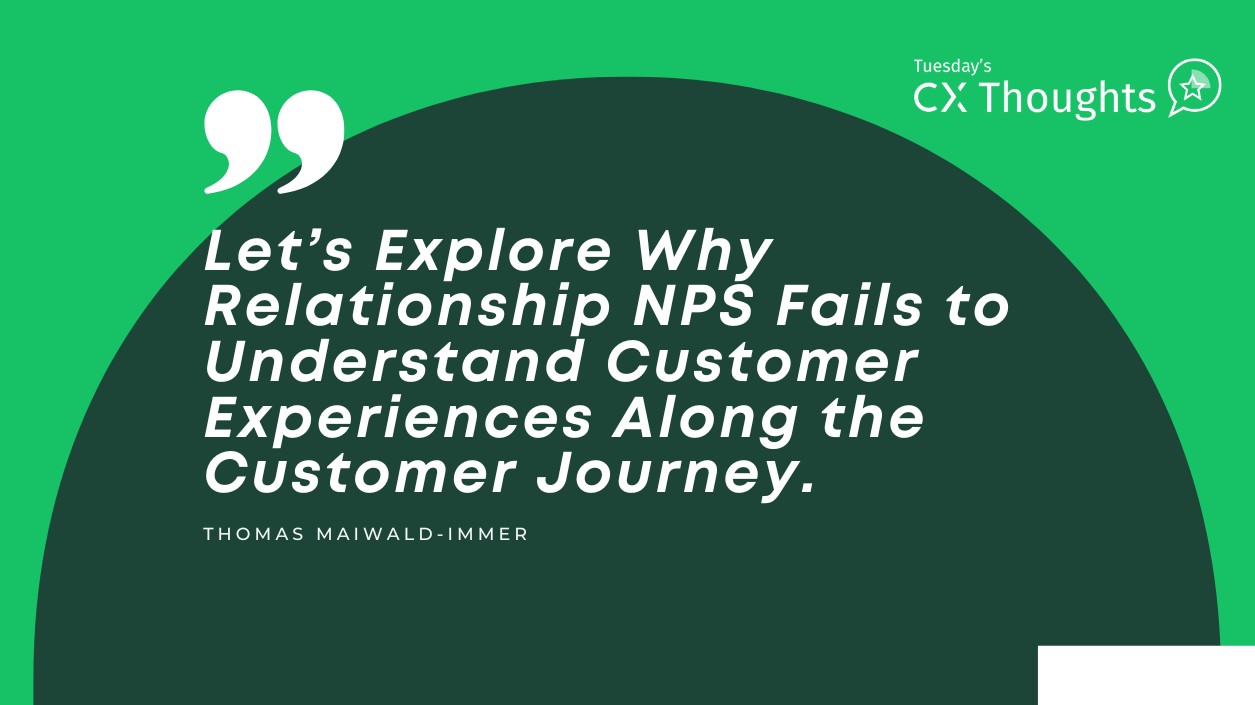
Relationship NPS Fails to Understand Customer Experiences — Tuesday CX
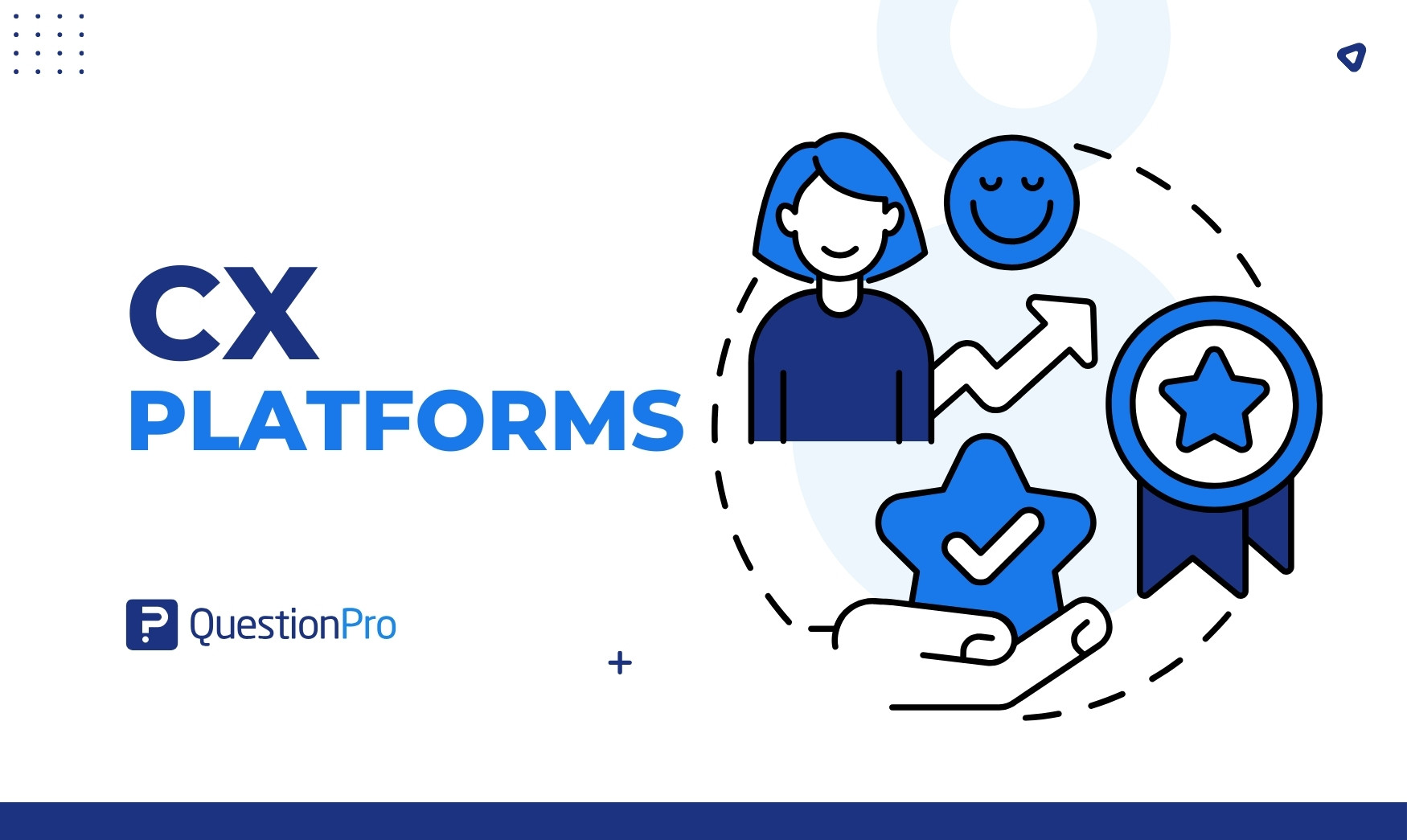
CX Platform: Top 13 CX Platforms to Drive Customer Success
Jun 17, 2024
Other categories
- Academic Research
- Artificial Intelligence
- Assessments
- Brand Awareness
- Case Studies
- Communities
- Consumer Insights
- Customer effort score
- Customer Engagement
- Customer Experience
- Customer Loyalty
- Customer Research
- Customer Satisfaction
- Employee Benefits
- Employee Engagement
- Employee Retention
- Friday Five
- General Data Protection Regulation
- Insights Hub
- Life@QuestionPro
- Market Research
- Mobile diaries
- Mobile Surveys
- New Features
- Online Communities
- Question Types
- Questionnaire
- QuestionPro Products
- Release Notes
- Research Tools and Apps
- Revenue at Risk
- Survey Templates
- Training Tips
- Tuesday CX Thoughts (TCXT)
- Uncategorized
- Video Learning Series
- What’s Coming Up
- Workforce Intelligence
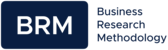
Causal Research (Explanatory research)
Causal research, also known as explanatory research is conducted in order to identify the extent and nature of cause-and-effect relationships. Causal research can be conducted in order to assess impacts of specific changes on existing norms, various processes etc.
Causal studies focus on an analysis of a situation or a specific problem to explain the patterns of relationships between variables. Experiments are the most popular primary data collection methods in studies with causal research design.
The presence of cause cause-and-effect relationships can be confirmed only if specific causal evidence exists. Causal evidence has three important components:
1. Temporal sequence . The cause must occur before the effect. For example, it would not be appropriate to credit the increase in sales to rebranding efforts if the increase had started before the rebranding.
2. Concomitant variation . The variation must be systematic between the two variables. For example, if a company doesn’t change its employee training and development practices, then changes in customer satisfaction cannot be caused by employee training and development.
3. Nonspurious association . Any covarioaton between a cause and an effect must be true and not simply due to other variable. In other words, there should be no a ‘third’ factor that relates to both, cause, as well as, effect.
The table below compares the main characteristics of causal research to exploratory and descriptive research designs: [1]
Amount of uncertainty characterising decision situation | Clearly defined | Highly ambiguous | Partially defined |
Key research statement | Research hypotheses | Research question | Research question |
When conducted? | Later stages of decision making | Early stage of decision making | Later stages of decision making |
Usual research approach | Highly structured | Unstructured | Structured |
Examples | ‘Will consumers buy more products in a blue package?’ ‘Which of two advertising campaigns will be more effective?’ | ‘Our sales are declining for no apparent reason’ ‘What kinds of new products are fast-food consumers interested in?’ | ‘What kind of people patronize our stores compared to our primary competitor?’ ‘What product features are the most important to our customers?’ |
Main characteristics of research designs
Examples of Causal Research (Explanatory Research)
The following are examples of research objectives for causal research design:
- To assess the impacts of foreign direct investment on the levels of economic growth in Taiwan
- To analyse the effects of re-branding initiatives on the levels of customer loyalty
- To identify the nature of impact of work process re-engineering on the levels of employee motivation
Advantages of Causal Research (Explanatory Research)
- Causal studies may play an instrumental role in terms of identifying reasons behind a wide range of processes, as well as, assessing the impacts of changes on existing norms, processes etc.
- Causal studies usually offer the advantages of replication if necessity arises
- This type of studies are associated with greater levels of internal validity due to systematic selection of subjects
Disadvantages of Causal Research (Explanatory Research)
- Coincidences in events may be perceived as cause-and-effect relationships. For example, Punxatawney Phil was able to forecast the duration of winter for five consecutive years, nevertheless, it is just a rodent without intellect and forecasting powers, i.e. it was a coincidence.
- It can be difficult to reach appropriate conclusions on the basis of causal research findings. This is due to the impact of a wide range of factors and variables in social environment. In other words, while casualty can be inferred, it cannot be proved with a high level of certainty.
- It certain cases, while correlation between two variables can be effectively established; identifying which variable is a cause and which one is the impact can be a difficult task to accomplish.
My e-book, The Ultimate Guide to Writing a Dissertation in Business Studies: a step by step assistance contains discussions of theory and application of research designs. The e-book also explains all stages of the research process starting from the selection of the research area to writing personal reflection. Important elements of dissertations such as research philosophy , research approach , methods of data collection , data analysis and sampling are explained in this e-book in simple words.
John Dudovskiy
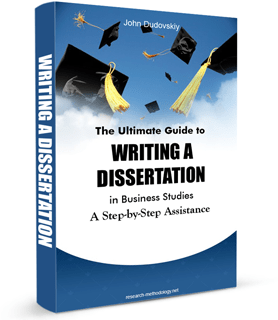
[1] Source: Zikmund, W.G., Babin, J., Carr, J. & Griffin, M. (2012) “Business Research Methods: with Qualtrics Printed Access Card” Cengage Learning
Child Care and Early Education Research Connections
Causal study design.
Researchers conduct experiments to study cause and effect relationships and to estimate the impact of child care and early childhood programs on children and their families. There are two basic types of experiments:
Randomized experiments
Quasi-experiments.
An experiment is a study in which the researcher manipulates the treatment, or intervention, and then measures the outcome. It addresses the question “if we change X (the treatment or intervention), what happens to Y (the outcome)?” Conducted both in the laboratory and in real life situations, experiments are powerful techniques for evaluating cause-and-effect relationships. The researcher may manipulate whether research subjects receive a treatment (e.g., attendance in a Head Start program: yes or no) or the level of treatment (e.g., hours per day in the program).
Suppose, for example, a group of researchers was interested in the effect of government-funded child care subsidies on maternal employment. They might hypothesize that the provision of government-subsidized child care would promote such employment. They could then design an experiment in which some mothers would be provided the option of government-funded child care subsidies and others would not. The researchers might also manipulate the value of the child care subsidies in order to determine if higher subsidy values might result in different levels of maternal employment.
The group of participants that receives the intervention or treatment is known as the "treatment group," and the group that does not is known as the “control group” in randomized experiments and “comparison group” in quasi-experiments.
The key distinction between randomized experiments and quasi-experiments lies in the fact that in a randomized experiment, participants are randomly assigned to either the treatment or the control group whereas participants are not in a quasi-experiment.
Randomized Experiments
Random assignment ensures that all participants have the same chance of being in a given experimental condition. Randomized experiments (also known as RCT or randomized control trials) are considered to be the most rigorous approach, or the “gold standard,” to identifying causal effects because they theoretically eliminate all preexisting differences between the treatment and control groups. However, some differences might occur due to chance. In practice, therefore, researchers often control for observed characteristics that might differ between individuals in the treatment and control groups when estimating treatment effects. The use of control variables improves the precision of treatment effect estimates.
Cluster-randomized experiments
Despite being the “gold standard” in causal study design, randomized experiments are not common in social science research because it is often impossible or unethical to randomize individuals to experimental conditions. Cluster-randomized experiments, in which groups (e.g., schools or classes) instead of individuals are randomized, often encounter less objections out of ethical concerns and therefore are more feasible in real life. They also prevent treatment spill over to the control group. For example, if students in the same class are randomly assigned to either the treatment or control group with the treatment being a new curriculum, teachers may introduce features of the treatment (i.e., new curriculum) when working with students in the control group in ways that might affect the outcomes.
One drawback of cluster-randomized experiments is a reduction in statistical power. That is, the likelihood that a true effect is detected is reduced with this design.
Quasi-Experiments
Quasi-experiments are characterized by the lack of randomized assignment. They may or may not have comparison groups. When there are both comparison and treatment groups in a quasi-experiment, the groups differ not only in terms of the experimental treatment they receive, but also in other, often unknown or unknowable, ways. As a result, there may be several "rival hypotheses" competing with the experimental manipulation as explanations for observed results.
There are a variety of quasi-experiments. Below are some of the most common types in social and policy research, arranged in the order of weak to strong in terms of their capabilities of addressing threats to a statement that the relationship between the treatment and the outcome of interest is causal.
One group only
One-group pretest-posttest A single group that receives the treatment is observed at two time points, one before the treatment and one after the treatment. Changes in the outcome of interest are presumed to be the effect of the treatment. For example, a new fourth grade math curriculum is introduced and students' math achievement is assessed in the fall and spring of the school year. Improved scores on the assessment are attributed to the curriculum. The biggest weakness of this design is that a number of events can happen around the time of the treatment and influence the outcome. There can be multiple plausible alternative explanations for the observed results.
Interrupted time series A single group that receives the treatment is observed at multiple time points both before and after the treatment. A change in the trend around the time of the treatment is presumed to be the treatment effect. For example, individuals participating in an exercise program might be weighed each week before and after a new exercise routine is introduced. A downward trend in their weight around the time the new routine was introduced would be seen as evidence of the effectiveness of the treatment. This design is stronger than one-group pretest-posttest because it shows the trend in the outcome variable both before and after the treatment instead of a simple two-point-in-time comparison. However, it still suffers the same weakness that other events can happen at the time of the treatment and be the alternative causes of the observed outcome.
Static-group comparison A group that has experienced some treatment is compared with one that has not. Observed differences between the two groups are assumed to be the result of the treatment. For example, fourth graders in some classrooms in a school district are introduced to a new math curriculum while fourth graders in other classrooms in the district are not. Differences in the math scores of the two groups assessed in the spring of the school year only are assumed to be the result of the new curriculum. The weakness of this design is that the treatment and comparison groups may not be truly comparable because participants are not randomly assigned to the groups and there may be important differences in the characteristics and experiences of the groups, only some of which may be known. If the two groups differ in ways that affect the outcome of interest, the causal claim cannot be presumed.
Difference-in-differences Both treatment and comparison groups are measured before and after the treatment. The difference between the two before-after differences is presumed to be the treatment effect. This design is an improvement of the static-group comparison because it compares outcomes that are measured both before and after the treatment is introduced instead of two post-treatment outcomes. For example, the fourth graders in the prior example are assessed in both the fall (pre-treatment) and spring (post-treatment). Differences in the fall-spring scores between the two fourth grade groups are seen as evidence of the effect of the curriculum. For this reason, the treatment and comparison groups in difference-in-differences do not have to be perfectly comparable. The biggest challenge for the researcher is to defend the parallel trend assumption, namely the change in the treatment group would be the same as the change in the comparison group in the absence of the treatment.
Regression discontinuity Participants are assigned to experimental conditions based on whether their scores are above or below a cut point for a quantitative variable. For example, students who score below 75 on a math test are assigned to the treatment group with the treatment being an intensive tutoring program. Those who score at or above 75 are assigned to the comparison group. The students who score just above or below the cut point are considered to be on average identical because their score differences are most likely due to chance. These students therefore act as if they were randomly assigned. The difference in the outcome of interest (e.g., math ability as measured by a different test after the treatment) between the students right around the cut point is presumed to be the treatment effect.
Regression discontinuity is an alternative to randomized experiments when the latter design is not possible. It is the only recognized quasi-experimental design that meets the Institute of Education Sciences standards for establishing causal effects. Although considered to be a strong quasi-experimental design, it needs to meet certain conditions.
See the following for additional information on randomized and quasi-experimental designs.
- The Core Analytics of Randomized Experiments for Social Research (PDF)
- Experimental and Quasi-Experimental Designs for Research (PDF)
- Experimental and Quasi-Experimental Designs for Generalized Causal Inference (PDF)
Instrumental Variables (IV) Approach
An instrumental variable is a variable that is correlated with the independent variable of interest and only affects the dependent variable through that independent variable. The IV approach can be used in both randomized experiments and quasi-experiments.
In randomized experiments, the IV approach is used to estimate the effect of treatment receipt, which is different from treatment offer. Many social programs can only offer participants the treatment, or intervention, but not mandate them to use it. For example, parents are randomly assigned by way of lottery to a school voucher program. Those in the treatment group are offered vouchers to help pay for private school, but ultimately it is up to the parents to decide whether or not they will use the vouchers. If the researcher is interested in estimating the impact of voucher usage, namely the effect of treatment receipt, the IV approach is one way to do so. In this case, the IV is the treatment assignment status (e.g., a dummy variable with 1 being in the treatment group and 0 being in the control group), which is used to predict the probability of a parent using the voucher, which is in turn used as the independent variable of interest to estimate the effect of voucher usage.
In quasi-experiments, the IV approach is used to address the issue of endogeneity, namely that the treatment status is determined by participants themselves (self-selection) or by criteria established by the program designer (treatment selection). Endogeneity is an issue that plagues quasi-experiments and often a source of threats to the causal claim. The IV approach can be used to tease out the causal impact of an endogenous variable on the outcome. For example, researchers used cigarette taxes as an instrumental variable to estimate the effect of maternal smoking on birth outcomes (Evans and Ringel, 1999). Cigarette taxes affect how much pregnant mothers smoke but not birth outcomes. They therefore meet the condition of being an IV, which correlates with the independent variable/treatment (i.e., maternal smoking habit) and only affects the dependent variable (i.e., birth outcomes) through that independent variable. The estimated effect is, strictly speaking, a local average treatment effect, namely the effect of treatment (maternal smoking) among those mothers affected by the IV (cigarette taxes). It does not include mothers whose smoking habit is not affected by the price of cigarettes (e.g., chain smokers who may be addicted to nicotine).
An instrumental variable needs to meet certain conditions to provide a consistent estimate of a causal effect.
See the following for additional information on instrumental variables.
- An introduction to instrumental variable assumptions, validation and estimation
- An Introduction to Instrumental Variables (PDF)
Validity of Results from Causal Designs
The two types of validity are internal and external. It is often difficult to achieve both in social science research experiments.
- Internal validity refers to the strength of evidence of a causal relationship between the treatment (e.g., child care subsidies) and the outcome (e.g., maternal employment).
- When subjects are randomly assigned to treatment or control groups, we can assume that the treatment caused the observed outcomes because the two groups should not have differed from one another at the start of the experiment.
- For example, take the child care subsidy example above. Since research subjects were randomly assigned to the treatment (child care subsidies available) and control (no child care subsidies available) groups, the two groups should not have differed at the outset of the study. If, after the intervention, mothers in the treatment group were more likely to be working, we can assume that the availability of child care subsidies promoted maternal employment.
One potential threat to internal validity in experiments occurs when participants either drop out of the study or refuse to participate in the study. If individuals with particular characteristics drop out or refuse to participate more often than individuals with other characteristics, this is called differential attrition. For example, suppose an experiment was conducted to assess the effects of a new reading curriculum on the reading achievement of 10th graders. Schools were randomly assigned to use the new curriculum in all classrooms (treatment schools) or to continue using their current curriculum (control schools). If many of the slowest readers in treatment schools left the study before it was completed (e.g., dropped out of school or transferred to a school in another state), schools with the new curriculum would experience an increase in the average reading scores. The reason they experienced an increase in reading scores, however, is because weaker readers left the school, not because the new curriculum improved students' reading skills. The effects of the curriculum on the achievement of 10th graders might be overestimated, if schools in the control schools did not experience the same type of attrition.
- External validity, or generalizability, is also of particular concern in social science experiments.
- It can be very difficult to generalize experimental results to groups that were not included in the study.
- Studies that randomly select participants from the most diverse and representative populations are more likely to have external validity.
For example, a study shows that a new curriculum improved reading comprehension of third-grade children in Iowa. To assess the study's external validity, the researcher would consider whether this new curriculum would also be effective with third graders in New York or with children in other elementary grades.
Advantages and Disadvantages of Experimental and Quasi-Experimental Designs
- Yield the most accurate assessment of cause and effect.
- Typically have strong internal validity.
- Ensure that the treatment and control groups are truly comparable and that treatment status is not determined by participant characteristics that might influence the outcome.
- In social policy research, it can be impractical or unethical to conduct randomized experiments.
- They typically have limited external validity due to the fact that they often rely on volunteers and are implemented in a somewhat artificial experimental setting with a small number of participants.
- Despite being the “gold standard” for identifying causal impacts, they can also be faced with threats to internal validity such as attrition, contamination, cross-overs, and Hawthorne effects.
- Often have stronger external validity than randomized experiments because they are typically implemented in real-world settings and on larger scale.
- May be more feasible than randomized experiments because they have fewer time and logistical constraints often associated with randomized experiments.
- Avoid the ethical concerns associated with random assignment.
- Are often less expensive than randomized experiments.
- They often have weaker internal validity than randomized experiments.
- The lack of randomized assignment means that the treatment and control groups may not be comparable and that treatment status may be driven by participant characteristics or other experiences that might influence the outcome.
- Conclusions about causality are less definitive than randomized experiments due to the lack of randomization and reduced internal validity.
- Despite having weaker internal validity, they are often the best option available when it is impractical or unethical to conduct randomized experiments.
Thank you for visiting nature.com. You are using a browser version with limited support for CSS. To obtain the best experience, we recommend you use a more up to date browser (or turn off compatibility mode in Internet Explorer). In the meantime, to ensure continued support, we are displaying the site without styles and JavaScript.
- View all journals
- Explore content
- About the journal
- Publish with us
- Sign up for alerts
- Published: April 2010
Cause and effect
Nature Methods volume 7 , page 243 ( 2010 ) Cite this article
14k Accesses
5 Citations
9 Altmetric
Metrics details
- Biological techniques
- Research management
The experimental tractability of biological systems makes it possible to explore the idea that causal relationships can be estimated from observational data.
“Happy is he who is able to know the causes of things.”— Virgil
The idea that one needs to do an experiment—a controlled perturbation of a single variable—to assign cause and effect is deeply embedded in traditional thinking about the way scientific knowledge is obtained, but it is largely absent from everyday life. One knows, without doing an experiment, that the street is wet on a rainy day because the rain has fallen. To be sure, this form of causal reasoning requires prior knowledge. One has seen the co-occurrence of rain and the wet street many times and been taught that rain causes wetness. And although such relationships are, in the strict sense, merely very good correlations, human beings routinely, and necessarily, use them to assign cause and effect.
As discussed on this page a year ago, this form of thinking, at least as a starting point for hypothesis-making, is in practice not uncommon in scientific research as well. Even before our data-driven age, a testable idea often began with an observation. When the structure of a voltage-gated potassium channel was first solved, for instance, the physical basis for potassium selectivity was suggested from observing the disposition of the residues known to allow potassium, but not sodium, ions to pass. In another example a century or so earlier, Ramón y Cajal famously predicted many features of the operation of the nervous system, including the directionality of neuronal signaling, based on his observations of the organization of neurons in the brain. Experiments had to be designed to test these ideas, but the hypotheses about cause and effect were generated at least in part by observation.
Many areas of contemporary biology seek to learn causal relationships from biological data. In systems biology, for instance, researchers use measurements of gene expression, cellular protein amounts or metabolite levels, among other types of data, to assign causal or regulatory relationships in models describing the cell. In the context of large-scale systems data, it is usually not possible to assign such relationships just by looking at the data by eye. Statistical and visualization tools are needed, when, for example, one is looking at lists of expression data of thousands of genes and trying to determine which genes regulate what other genes. The methods used to assign causal arrows typically involve perturbation experiments. When unperturbed data are used, additional information such as change over time or prior biological knowledge has been used to order the data.
A Correspondence by Maathuis and colleagues published in this issue (p. 247), in contrast, explores the notion that it might be possible to estimate causal relationships simply by observing random variation in unperturbed data, with no other information added. Making use of gene expression data obtained either from single gene knockouts in yeast—a classical perturbation experiment—or from parallel control measurements on wild-type yeast, an unperturbed system in which there is presumably only random variation, the authors report that, under some assumptions, statistical analysis can be used to predict the strongest causal effects from the control data alone.
The idea that such prediction is theoretically possible is not in itself new and has received some interest in, among others, the social scientific, economic and medical spheres. But it is an idea that is not easy to test in a real-world setting. In a sense, then, the study in this issue exploits the unique properties of biological systems—their complexity, the availability of good tools for precise and ethical system manipulation, and the well-developed technology for acquiring large-scale unbiased data—to test an idea that could have interest and value outside the biological realm as well.
It is worth noting that the assumptions made—in its current iteration, the approach by Maathuis and colleagues provides no allowance for feedback and does not incorporate change over time—could pose serious obstacles for understanding biological as well as other systems. What is more, statistical inference will clearly not replace perturbation experiments in systems that are amenable to manipulation.
Nonetheless, causal inference from purely observed data could have practical value in the prioritization and design of perturbation experiments. Perturbations can be impossible, for instance, if the tools available are not specific enough, unethical, for example in human studies, or simply unfeasible owing to cost or impracticality. Observational data could be used to identify candidate causal relationships, which could then be the basis for the design of targeted perturbations or for further analysis.
Rights and permissions
Reprints and permissions
About this article
Cite this article.
Cause and effect. Nat Methods 7 , 243 (2010). https://doi.org/10.1038/nmeth0410-243
Download citation
Issue Date : April 2010
DOI : https://doi.org/10.1038/nmeth0410-243
Share this article
Anyone you share the following link with will be able to read this content:
Sorry, a shareable link is not currently available for this article.
Provided by the Springer Nature SharedIt content-sharing initiative
Quick links
- Explore articles by subject
- Guide to authors
- Editorial policies
Sign up for the Nature Briefing newsletter — what matters in science, free to your inbox daily.

- Bipolar Disorder
- Therapy Center
- When To See a Therapist
- Types of Therapy
- Best Online Therapy
- Best Couples Therapy
- Best Family Therapy
- Managing Stress
- Sleep and Dreaming
- Understanding Emotions
- Self-Improvement
- Healthy Relationships
- Student Resources
- Personality Types
- Guided Meditations
- Verywell Mind Insights
- 2024 Verywell Mind 25
- Mental Health in the Classroom
- Editorial Process
- Meet Our Review Board
- Crisis Support
Introduction to Research Methods in Psychology
Kendra Cherry, MS, is a psychosocial rehabilitation specialist, psychology educator, and author of the "Everything Psychology Book."
:max_bytes(150000):strip_icc():format(webp)/IMG_9791-89504ab694d54b66bbd72cb84ffb860e.jpg)
Emily is a board-certified science editor who has worked with top digital publishing brands like Voices for Biodiversity, Study.com, GoodTherapy, Vox, and Verywell.
:max_bytes(150000):strip_icc():format(webp)/Emily-Swaim-1000-0f3197de18f74329aeffb690a177160c.jpg)
There are several different research methods in psychology , each of which can help researchers learn more about the way people think, feel, and behave. If you're a psychology student or just want to know the types of research in psychology, here are the main ones as well as how they work.
Three Main Types of Research in Psychology
stevecoleimages/Getty Images
Psychology research can usually be classified as one of three major types.
1. Causal or Experimental Research
When most people think of scientific experimentation, research on cause and effect is most often brought to mind. Experiments on causal relationships investigate the effect of one or more variables on one or more outcome variables. This type of research also determines if one variable causes another variable to occur or change.
An example of this type of research in psychology would be changing the length of a specific mental health treatment and measuring the effect on study participants.
2. Descriptive Research
Descriptive research seeks to depict what already exists in a group or population. Three types of psychology research utilizing this method are:
- Case studies
- Observational studies
An example of this psychology research method would be an opinion poll to determine which presidential candidate people plan to vote for in the next election. Descriptive studies don't try to measure the effect of a variable; they seek only to describe it.
3. Relational or Correlational Research
A study that investigates the connection between two or more variables is considered relational research. The variables compared are generally already present in the group or population.
For example, a study that looks at the proportion of males and females that would purchase either a classical CD or a jazz CD would be studying the relationship between gender and music preference.
Theory vs. Hypothesis in Psychology Research
People often confuse the terms theory and hypothesis or are not quite sure of the distinctions between the two concepts. If you're a psychology student, it's essential to understand what each term means, how they differ, and how they're used in psychology research.
A theory is a well-established principle that has been developed to explain some aspect of the natural world. A theory arises from repeated observation and testing and incorporates facts, laws, predictions, and tested hypotheses that are widely accepted.
A hypothesis is a specific, testable prediction about what you expect to happen in your study. For example, an experiment designed to look at the relationship between study habits and test anxiety might have a hypothesis that states, "We predict that students with better study habits will suffer less test anxiety." Unless your study is exploratory in nature, your hypothesis should always explain what you expect to happen during the course of your experiment or research.
While the terms are sometimes used interchangeably in everyday use, the difference between a theory and a hypothesis is important when studying experimental design.
Some other important distinctions to note include:
- A theory predicts events in general terms, while a hypothesis makes a specific prediction about a specified set of circumstances.
- A theory has been extensively tested and is generally accepted, while a hypothesis is a speculative guess that has yet to be tested.
The Effect of Time on Research Methods in Psychology
There are two types of time dimensions that can be used in designing a research study:
- Cross-sectional research takes place at a single point in time. All tests, measures, or variables are administered to participants on one occasion. This type of research seeks to gather data on present conditions instead of looking at the effects of a variable over a period of time.
- Longitudinal research is a study that takes place over a period of time. Data is first collected at the beginning of the study, and may then be gathered repeatedly throughout the length of the study. Some longitudinal studies may occur over a short period of time, such as a few days, while others may take place over a period of months, years, or even decades.
The effects of aging are often investigated using longitudinal research.
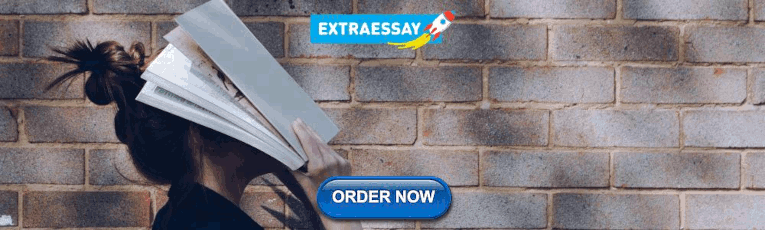
Causal Relationships Between Psychology Research Variables
What do we mean when we talk about a “relationship” between variables? In psychological research, we're referring to a connection between two or more factors that we can measure or systematically vary.
One of the most important distinctions to make when discussing the relationship between variables is the meaning of causation.
A causal relationship is when one variable causes a change in another variable. These types of relationships are investigated by experimental research to determine if changes in one variable actually result in changes in another variable.
Correlational Relationships Between Psychology Research Variables
A correlation is the measurement of the relationship between two variables. These variables already occur in the group or population and are not controlled by the experimenter.
- A positive correlation is a direct relationship where, as the amount of one variable increases, the amount of a second variable also increases.
- In a negative correlation , as the amount of one variable goes up, the levels of another variable go down.
In both types of correlation, there is no evidence or proof that changes in one variable cause changes in the other variable. A correlation simply indicates that there is a relationship between the two variables.
The most important concept is that correlation does not equal causation. Many popular media sources make the mistake of assuming that simply because two variables are related, a causal relationship exists.
Psychologists use descriptive, correlational, and experimental research designs to understand behavior . In: Introduction to Psychology . Minneapolis, MN: University of Minnesota Libraries Publishing; 2010.
Caruana EJ, Roman M, Herandez-Sanchez J, Solli P. Longitudinal studies . Journal of Thoracic Disease. 2015;7(11):E537-E540. doi:10.3978/j.issn.2072-1439.2015.10.63
University of Berkeley. Science at multiple levels . Understanding Science 101 . Published 2012.
By Kendra Cherry, MSEd Kendra Cherry, MS, is a psychosocial rehabilitation specialist, psychology educator, and author of the "Everything Psychology Book."
Experimental Method In Psychology
Saul Mcleod, PhD
Editor-in-Chief for Simply Psychology
BSc (Hons) Psychology, MRes, PhD, University of Manchester
Saul Mcleod, PhD., is a qualified psychology teacher with over 18 years of experience in further and higher education. He has been published in peer-reviewed journals, including the Journal of Clinical Psychology.
Learn about our Editorial Process
Olivia Guy-Evans, MSc
Associate Editor for Simply Psychology
BSc (Hons) Psychology, MSc Psychology of Education
Olivia Guy-Evans is a writer and associate editor for Simply Psychology. She has previously worked in healthcare and educational sectors.
On This Page:
The experimental method involves the manipulation of variables to establish cause-and-effect relationships. The key features are controlled methods and the random allocation of participants into controlled and experimental groups .
What is an Experiment?
An experiment is an investigation in which a hypothesis is scientifically tested. An independent variable (the cause) is manipulated in an experiment, and the dependent variable (the effect) is measured; any extraneous variables are controlled.
An advantage is that experiments should be objective. The researcher’s views and opinions should not affect a study’s results. This is good as it makes the data more valid and less biased.
There are three types of experiments you need to know:
1. Lab Experiment
A laboratory experiment in psychology is a research method in which the experimenter manipulates one or more independent variables and measures the effects on the dependent variable under controlled conditions.
A laboratory experiment is conducted under highly controlled conditions (not necessarily a laboratory) where accurate measurements are possible.
The researcher uses a standardized procedure to determine where the experiment will take place, at what time, with which participants, and in what circumstances.
Participants are randomly allocated to each independent variable group.
Examples are Milgram’s experiment on obedience and Loftus and Palmer’s car crash study .
- Strength : It is easier to replicate (i.e., copy) a laboratory experiment. This is because a standardized procedure is used.
- Strength : They allow for precise control of extraneous and independent variables. This allows a cause-and-effect relationship to be established.
- Limitation : The artificiality of the setting may produce unnatural behavior that does not reflect real life, i.e., low ecological validity. This means it would not be possible to generalize the findings to a real-life setting.
- Limitation : Demand characteristics or experimenter effects may bias the results and become confounding variables .
2. Field Experiment
A field experiment is a research method in psychology that takes place in a natural, real-world setting. It is similar to a laboratory experiment in that the experimenter manipulates one or more independent variables and measures the effects on the dependent variable.
However, in a field experiment, the participants are unaware they are being studied, and the experimenter has less control over the extraneous variables .
Field experiments are often used to study social phenomena, such as altruism, obedience, and persuasion. They are also used to test the effectiveness of interventions in real-world settings, such as educational programs and public health campaigns.
An example is Holfing’s hospital study on obedience .
- Strength : behavior in a field experiment is more likely to reflect real life because of its natural setting, i.e., higher ecological validity than a lab experiment.
- Strength : Demand characteristics are less likely to affect the results, as participants may not know they are being studied. This occurs when the study is covert.
- Limitation : There is less control over extraneous variables that might bias the results. This makes it difficult for another researcher to replicate the study in exactly the same way.
3. Natural Experiment
A natural experiment in psychology is a research method in which the experimenter observes the effects of a naturally occurring event or situation on the dependent variable without manipulating any variables.
Natural experiments are conducted in the day (i.e., real life) environment of the participants, but here, the experimenter has no control over the independent variable as it occurs naturally in real life.
Natural experiments are often used to study psychological phenomena that would be difficult or unethical to study in a laboratory setting, such as the effects of natural disasters, policy changes, or social movements.
For example, Hodges and Tizard’s attachment research (1989) compared the long-term development of children who have been adopted, fostered, or returned to their mothers with a control group of children who had spent all their lives in their biological families.
Here is a fictional example of a natural experiment in psychology:
Researchers might compare academic achievement rates among students born before and after a major policy change that increased funding for education.
In this case, the independent variable is the timing of the policy change, and the dependent variable is academic achievement. The researchers would not be able to manipulate the independent variable, but they could observe its effects on the dependent variable.
- Strength : behavior in a natural experiment is more likely to reflect real life because of its natural setting, i.e., very high ecological validity.
- Strength : Demand characteristics are less likely to affect the results, as participants may not know they are being studied.
- Strength : It can be used in situations in which it would be ethically unacceptable to manipulate the independent variable, e.g., researching stress .
- Limitation : They may be more expensive and time-consuming than lab experiments.
- Limitation : There is no control over extraneous variables that might bias the results. This makes it difficult for another researcher to replicate the study in exactly the same way.
Key Terminology
Ecological validity.
The degree to which an investigation represents real-life experiences.
Experimenter effects
These are the ways that the experimenter can accidentally influence the participant through their appearance or behavior.
Demand characteristics
The clues in an experiment lead the participants to think they know what the researcher is looking for (e.g., the experimenter’s body language).
Independent variable (IV)
The variable the experimenter manipulates (i.e., changes) is assumed to have a direct effect on the dependent variable.
Dependent variable (DV)
Variable the experimenter measures. This is the outcome (i.e., the result) of a study.
Extraneous variables (EV)
All variables which are not independent variables but could affect the results (DV) of the experiment. EVs should be controlled where possible.
Confounding variables
Variable(s) that have affected the results (DV), apart from the IV. A confounding variable could be an extraneous variable that has not been controlled.
Random Allocation
Randomly allocating participants to independent variable conditions means that all participants should have an equal chance of participating in each condition.
The principle of random allocation is to avoid bias in how the experiment is carried out and limit the effects of participant variables.
Order effects
Changes in participants’ performance due to their repeating the same or similar test more than once. Examples of order effects include:
(i) practice effect: an improvement in performance on a task due to repetition, for example, because of familiarity with the task;
(ii) fatigue effect: a decrease in performance of a task due to repetition, for example, because of boredom or tiredness.

Related Articles
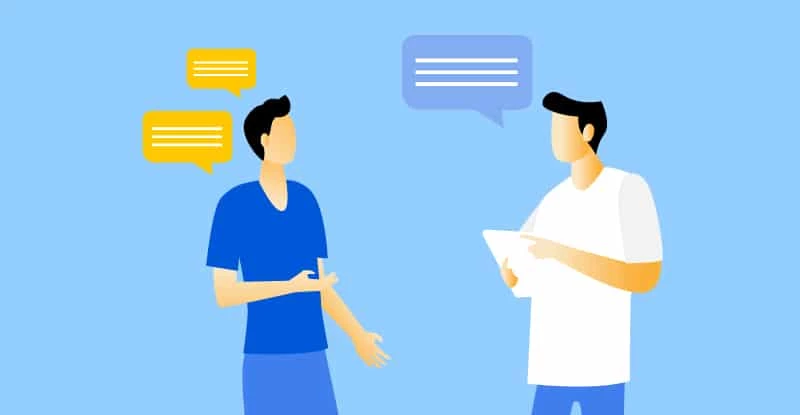
Research Methodology
Discourse Analysis
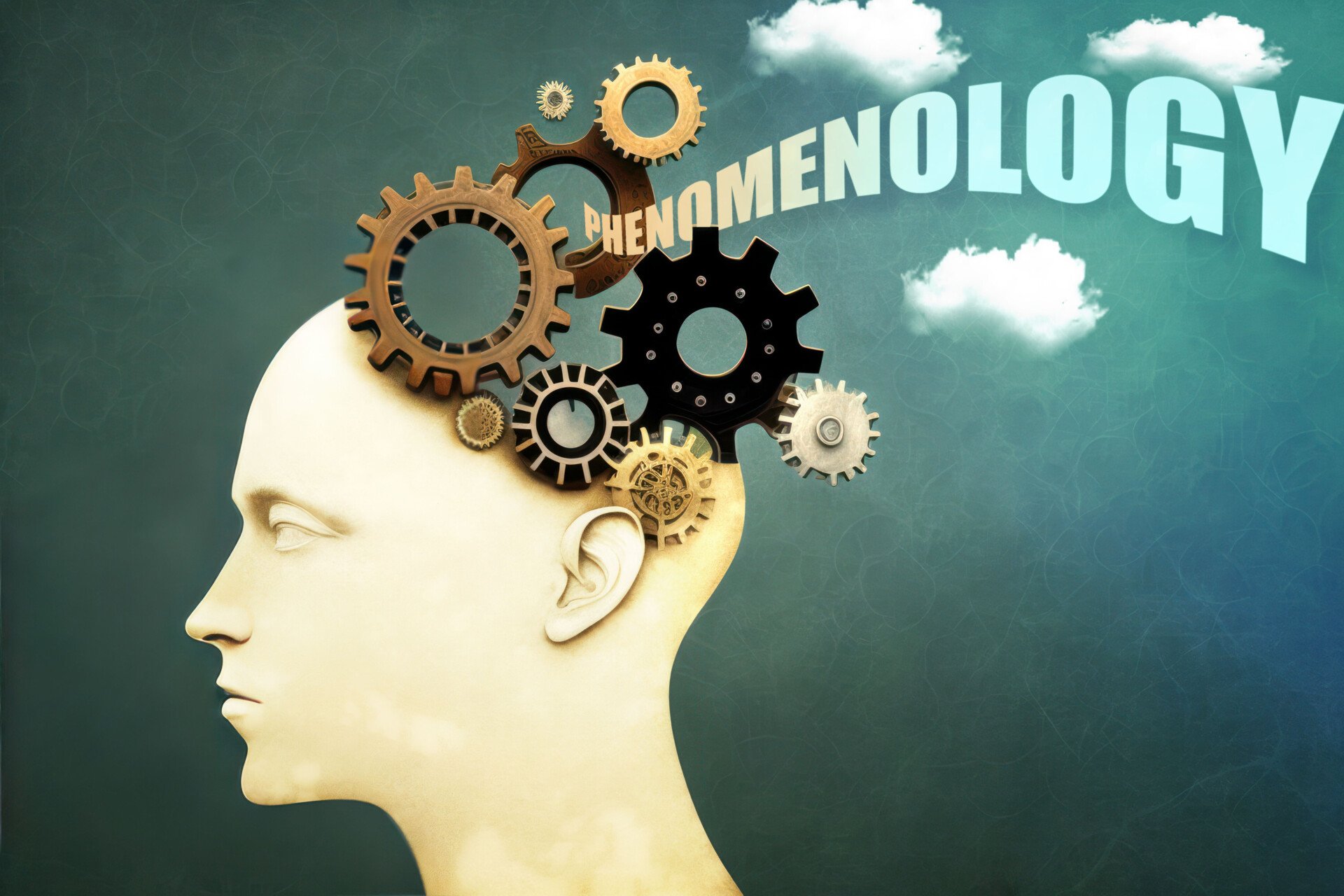
Phenomenology In Qualitative Research
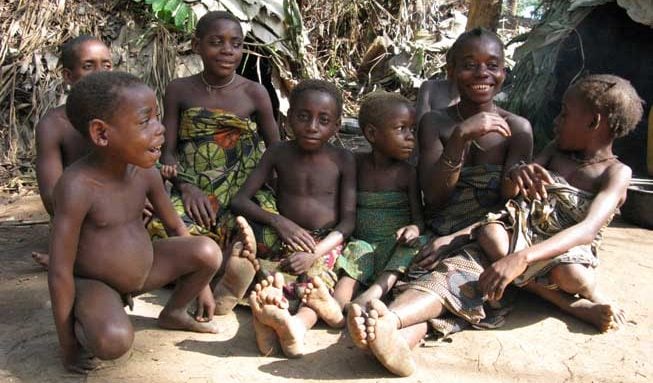
Ethnography In Qualitative Research
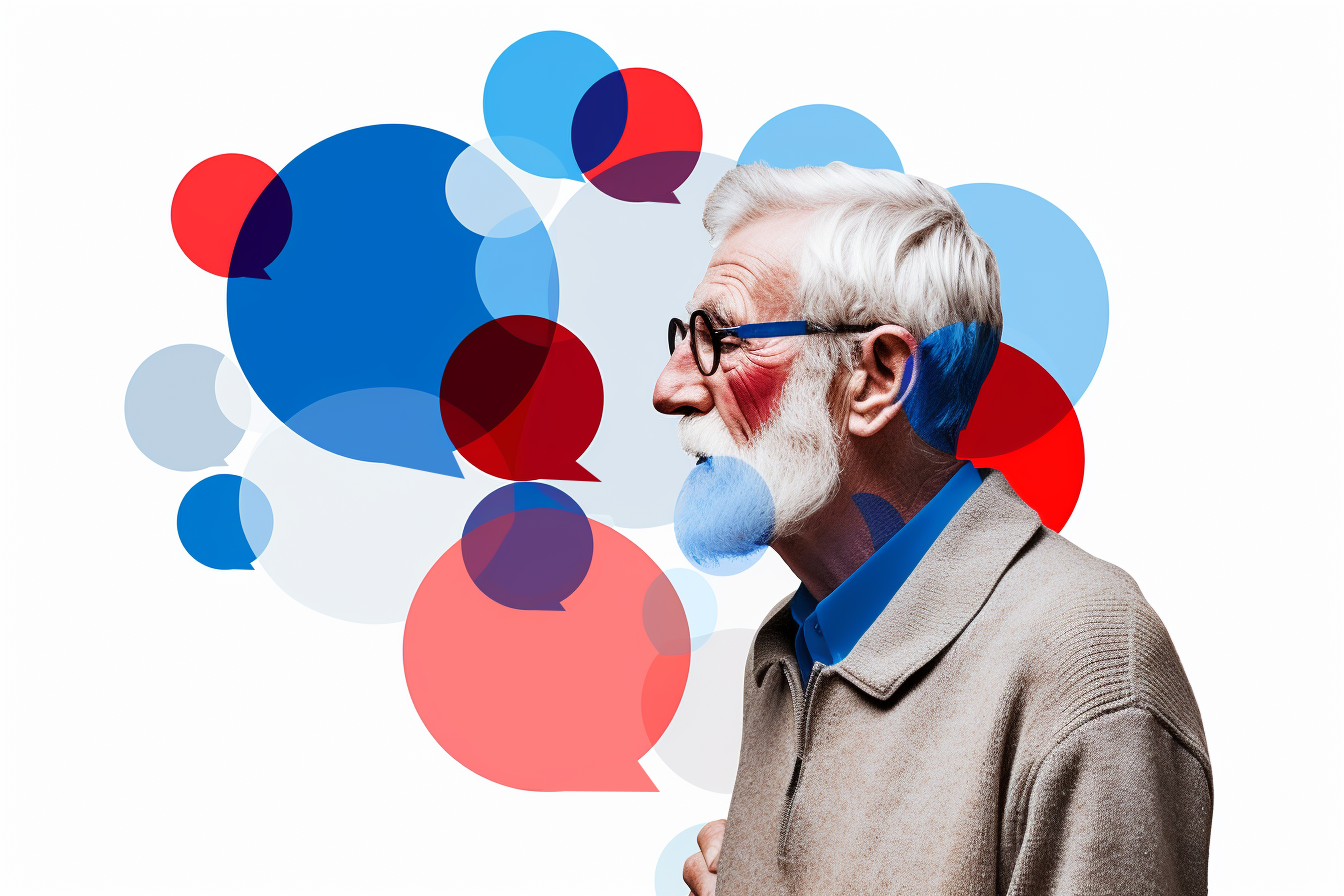
Narrative Analysis In Qualitative Research
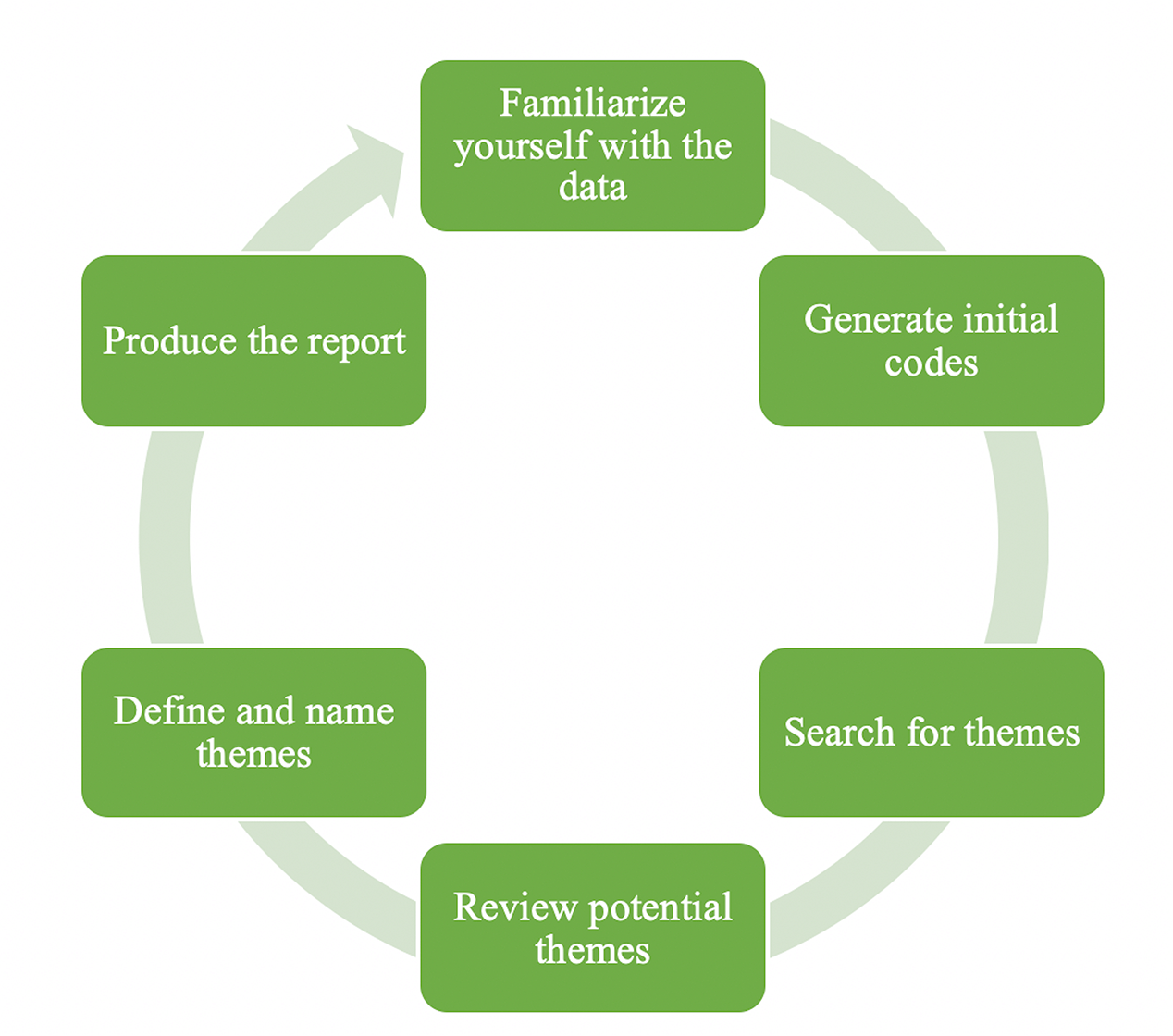
Thematic Analysis: A Step by Step Guide
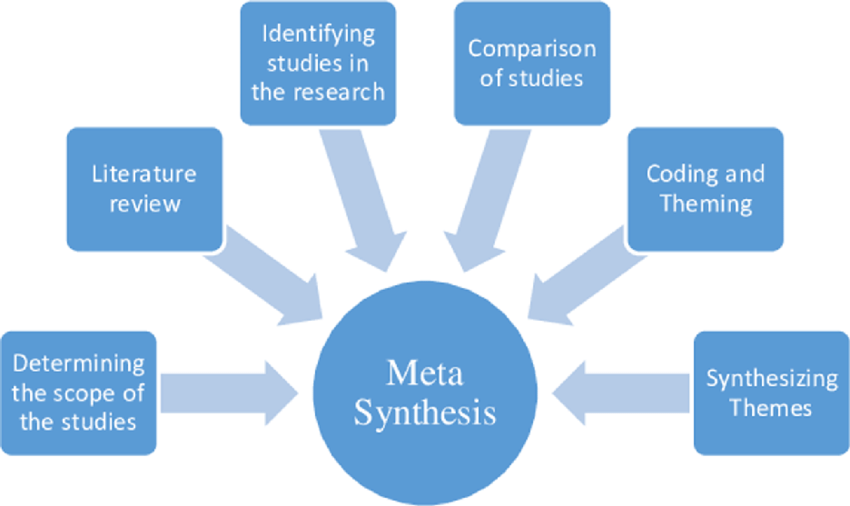
Metasynthesis Of Qualitative Research

An official website of the United States government
The .gov means it’s official. Federal government websites often end in .gov or .mil. Before sharing sensitive information, make sure you’re on a federal government site.
The site is secure. The https:// ensures that you are connecting to the official website and that any information you provide is encrypted and transmitted securely.
- Publications
- Account settings
Preview improvements coming to the PMC website in October 2024. Learn More or Try it out now .
- Advanced Search
- Journal List
- HHS Author Manuscripts

A Consumer’s Guide to Causal Modeling: Part I
RESEARCH IS the tool that scientists use to further their knowledge of the world. Scientists ask questions about phenomena and design research studies to answer those questions. In many instances, scientists are interested in what causes certain outcomes to occur. The most powerful and direct way to establish cause and effect is to design an experiment. In order to be considered an experiment, the study must have a control (or comparison) group and at least one experimental (or treatment) group; subjects must be assigned to groups randomly, not by choice of the subject or the investigator; and the researcher must manipulate the independent variable so that the experimental group gets the treatment and the control group does not ( Polit & Hungler, 1991 ).
When random assignment to group is not feasible, a quasi-experimental design that requires a comparison group and manipulation of the independent variable may be used; however, in some circumstances, manipulation of the independent variable is not possible, forcing the researcher to use naturally occurring groups. For example, suppose the researcher is interested in the effects of a mother’s employment on her child’s development. One way to study this would be to compare children of employed mothers (“treatment group”) with children of nonemployed mothers (“comparison group”). Although there are two groups, random assignment of mothers to employment or nonemployment would raise both practical and ethical concerns. Manipulating either the mother’s employment status or the number of hours the mother is employed (the independent variable) also raises these concerns. Thus, the researcher must use naturally occurring groups, a design that provides very weak evidence of causality. Causal modeling, a methodological and analytical technique, may provide stronger evidence about the direction and strength of cause-and-effect relationships hypothesized by the researcher. The purpose of this two-part series is to discuss the conceptual basis for causal modeling and to provide the consumer of nursing research with guidelines for evaluating published reports of research that used causal modeling techniques.
Nursing phenomena are often complex with many variables in the causal chain. One shortcoming of the experimental design study is that it fails to provide information about the effects of other variables on the independent or dependent variables because random assignment of subjects to groups breaks any relationships between these other variables and the independent variable. Removal of these relationships strengthens the evidence for causality, but it does not represent the complexity of the process by which an outcome occurs in the real world. Some statistical techniques allow the researcher to analyze the effect of independent variables on a dependent variable while controlling for the effects of other variables on the process. These techniques tell us how much influence a variable exerts directly on the dependent variable (direct effect). However, sometimes a variable’s influence is transmitted through another variable (indirect effect). In that case, the total amount of influence the variable has on the dependent variable is underestimated by these techniques because the indirect effect is not considered. Causal modeling can be used to represent very complex relationships among a set of variables, including direct and indirect effects, which increases the model’s correspondence with reality.
CONSTRUCTING A CAUSAL MODEL
Causal modeling requires the researcher to construct a model to explain the relationships among concepts related to a specific phenomenon ( Asher, 1983 ). A causal model is a diagram of the relationships between independent, control, and dependent variables. A path model is a less complex type of causal model. Causal models are also known as “structural equation models,” “covariance structure models,” or “LISREL models.” Each of these terms has a slightly different meaning based on the specific analysis technique used but are often used interchangeably in the literature.
Construction of a causal model can be accomplished in two basic ways. The preferred method is to build the model before the study begins based on theoretical expectations. In this method, the researcher considers the relationship between the independent and dependent variables of interest. Then variables that could affect either the independent or the dependent variable or the relationship between them are added to the model, and the direction of their effects is specified. Each linkage must be supported by expectations from other theories (theoretical evidence), by results of research (empirical evidence), or by experiential knowledge. An example from the author’s research is provided to illustrate how support for the proposed linkages is described.
The causal model in Figure 1 is a simplified version* of the expected system of relationships between maternal employment status and child development that is currently being used to guide a study of this phenomenon with preschool children, some born prematurely and some born at term, and their families. Each of the linkages can be supported theoretically or empirically. Research suggests that employment has a negative effect on mother-child interaction, at least in infancy ( Barglow, Vaughn, & Molitor, 1987 ; Belsky & Rovine, 1988 ), although other studies find no effect or a positive effect ( Schubert, Bradley-Johnson, & Nuttal, 1980 ). Because of these conflicting findings, we are positing a relationship between employment and mother-child interaction, but we are not specifying whether this will be a positive or a negative relationship. Consistency between employment attitudes and employment status repeatedly has been found to have a positive effect on mother-child interaction ( Benn, 1986 ; Bronfenbrenner, Alvarez, & Henderson, 1984 ; Hock, 1980 ).

Causal model diagram
Family functioning is defined as the quality of relationships among family members. Again, research findings are conflicting but support the importance of investigating the effect of employment on family functioning. Some researchers find no adverse effects on marital satisfaction ( Locksley, 1980 ), and others find negative effects ( Hardesty & Betz, 1980 ). In a series of studies, Youngblut, Loveland-Cherry, and Horan ( 1991 , 1993 , in press ) found that parents in nonemployed-mother families were more satisfied with their family’s relationships than parents in employed-mother families when the family’s preterm infant was 18 months old (in press), but not at 3 months (1991) or at 9 and 12 months (1993). The path from consistency to family functioning is supported by a positive relationship between degree of choice in the employment decision and parents’ perceptions of family relationships and family cohesion ( Youngblut et al., in press ).
The link between mother-child relationship and child development is strongly supported by the work of many researchers (see Sameroff & Chandler, 1975 , for review of the full-term literature; or Meisels & Plunkett, 1988 , for review of the preterm literature). Because research on the effects of family functioning on preterm infant development is sparse, this link is supported with theoretical literature. Family systems theory ( Friedman, 1986 ) suggests that quality of family relationships will affect the individual members of the family. Therefore, if the mother-child relationship has an effect on child development, it is likely that family relationships will also have an effect on the child’s development. In addition, both Bronfenbrenner (1985) and Hoffman (1989) have advocated broadening inquiry beyond the mother-child relationship to include the family. Finally, the effect of mother’s education level on child outcomes is included as a control variable with empirical support by many researchers (Heynes, 1982; Hoffman, 1989 ). When constructed theoretically, the causal model will serve as a guide for study design and implementation and for data analysis.
Causal models can also be built empirically. In this case, construction of the model is based on the correlations among the variables that were actually obtained in the study. Although this may be more appealing and perhaps simpler, there are several issues that need to be considered. First is the issue of relying on simple (bivariate) correlations to indicate relationships. A correlation between two variables indicates the total amount of overlap between the two variables. This overlap represents the combination of direct effects, indirect effects, and other effects. Direct effects are those that are due to one variable when the effects of other variables are controlled. Indirect effects are transmitted through intervening, or mediating, variables. Other effects could be due to sampling or measurement error or to the effect of another variable on both of the variables being correlated ( Pedhazur, 1982 ).
For example, if the correlation between employment and child development is high, one might consider putting a direct path between the two variables ( Figure 2 , solid line, top diagram). However, it may be that the quality of the mother-child relationship mediates the effect of employment on child development ( Figure 2 , broken line, top diagram). If so, controlling for quality of mother-child interaction would show that there is really no correlation between employment and development. Or it may be that the correlation between employment and development ( Figure 2 , curved broken line, bottom diagram) is due to the effect of neonatal health status on both employment and development ( Figure 2 , solid lines, bottom diagram); in other words, the correlation is a coincidence (known as a spurious correlation) that is due to the impact of a third variable on the other two. Thus, relying on the bivariate relationships would be misleading and would obscure the “true” path of the effect.

Diagram of direct, indirect, and spurious effects.
Importantly, using the correlations obtained with the study’s data changes the analysis from hypothesis testing to a fishing expedition. Causal models represent a system of hypotheses about the interrelationships of variables when the model is constructed theoretically before the data are collected. Testing the model then represents testing each of the implied hypotheses. However, if the model is constructed from the data, it can no longer be considered to represent a system of hypotheses and the analysis becomes post hoc exploration of the data. This practice of data snooping often yields results that are not theoretically defensible, do not make sense conceptually, and are less likely to be replicated in future research.
In summary, causal modeling is an important tool for knowledge development in nursing, and its use is increasing. Therefore, consumers of nursing research must have some familiarity with the conceptual underpinnings of causal modeling and an appreciation of how the technique may be used. In addition, the readers’ ability to critique published reports of model testing is crucial so that they can evaluate the results of these studies. The first part of this two-part series was aimed at increasing the reader’s level of sophistication regarding causal modeling techniques. The second part will provide guidelines for evaluating published reports of research.
Acknowledgments
Supported in part by a grant from the National Institute for Nursing Research (No. ROI-NR02707) and by an administrative supplement from the Office of Research on Women’s Health through the National Institute for Nursing Research.
Address reprint requests to JoAnne M. Youngblut. PhD. RN. Frances Payne Bolton School of Nursing. Case Western Reserve University, 10900 Euclid Ave, Cleveland, OH 44106-4904.
- Asher HB. Causal modeling. 2nd ed Sage; Beverly Hills, CA: 1983. [ Google Scholar ]
- Barglow P, Vaughn BE, Molitor N. Effects of maternal absence due to employment on the quality of infant-mother attachment in a low-risk sample. Child Development. 1987; 58 :945–954. [ PubMed ] [ Google Scholar ]
- Belsky J, Rovine MJ. Nonmaternal care in the first year of life and the security of infant-parent attachment. Child Development. 1988; 59 :157–167. [ PubMed ] [ Google Scholar ]
- Benn R. Factors promoting secure attachment relationships between employed mothers and their sons. Child Development. 1986; 57 :1224–1231. [ Google Scholar ]
- Bronfenbrenner U. Organism-environment interaction from an ecological perspective. Paper presented at the meeting of the Society for Research in Child Development; Toronto, Ontario. Apr, 1985. [ Google Scholar ]
- Bronfenbrenner U, Alvarez WF, Henderson CR., Jr. Working and watching: Maternal employment status and parents’ perceptions of their three-year-old children. Child Development. 1984; 55 :1362–1378. [ Google Scholar ]
- Friedman M. Family nursing: Theory and assessment. Appleton-Century-Crofts; Norwalk, CT: 1986. [ Google Scholar ]
- Hardesty SA, Betz NE. The relationships of career salience, attitudes toward women, and demographic and family characteristics to marital adjustment in dual-career couples. Journal of Vocational Behavior. 1980; 17 :242–250. [ PubMed ] [ Google Scholar ]
- Heyns B. The influence of parents’ work on children’s school achievement. In: Kamerman SB, Hayes CD, editors. Families that work: Children in a changing world. National Academy Press; Washington, DC: 1982. pp. 229–267. [ Google Scholar ]
- Hock E. Working and nonworking mothers and their infants: A comparative study of maternal caregiving characteristics and infant social behavior. Merrill-Palmer Quarterly. 1980; 26 :79–101. [ Google Scholar ]
- Hoffman LW. Effects of maternal employment in the two-parent family. American Psychologist. 1989; 44 :283–292. [ Google Scholar ]
- Locksley A. On the effects of wives’ employment on marital adjustment and companionship. Journal of Marriage and the Family. 1980; 42 :337–346. [ Google Scholar ]
- Meisels SJ, Plunkett JW. Developmental consequences of preterm birth: Are there long-term effects? In: Baltes PB, Featherman DL, Lerner RM, editors. Life-span development and behavior. Laurence, Erlbaum Associates; Hillsdale, NJ: 1988. [ Google Scholar ]
- Pedhazur EJ. Multiple regression in behavioral research. 2nd ed Holt, Rinehart, and Winston; New York, NY: 1982. [ Google Scholar ]
- Polit DF, Hungler BP. Nursing research: Principles and methods. 4th ed Lippincott; Philadelphia, PA: 1991. [ Google Scholar ]
- Sameroff AJ, Chandler MJ. Reproductive risk and the continuum of caretaking casualty. In: Horowitz F, Hetherington M, Scarr-Salapatek S, Siegel G, editors. Review of child development research. vol. 4. University of Chicago Press; Chicago, IL: 1975. pp. 187–244. [ Google Scholar ]
- Schubert JB, Bradley-Johnson S, Nuttal J. Mother-infant communication and maternal employment. Child Development. 1980; 51 :246–249. [ PubMed ] [ Google Scholar ]
- Youngblut JM, Loveland-Cherry CJ, Horan M. Maternal employment effects on family and preterm infants at three months. Nursing Research. 1991; 40 :272–275. [ PMC free article ] [ PubMed ] [ Google Scholar ]
- Youngblut JM, Loveland-Cherry CJ, Horan M. Maternal employment, family functioning, and preterm infant development at 9 and 12 months. Research in Nursing and Health. 1993; 16 :33–43. [ PMC free article ] [ PubMed ] [ Google Scholar ]
- Youngblut JM, Loveland-Cherry CJ, Horan M. Maternal employment effects on families and preterm infants at 18 months. Nursing Research. (in press) [ PMC free article ] [ PubMed ] [ Google Scholar ]
- Search Menu
Sign in through your institution
- Browse content in Arts and Humanities
- Browse content in Archaeology
- Historical Archaeology
- Browse content in Architecture
- History of Architecture
- Browse content in Art
- History of Art
- Browse content in Classical Studies
- Classical Literature
- Religion in the Ancient World
- Browse content in History
- Colonialism and Imperialism
- History by Period
- Intellectual History
- Military History
- Political History
- Regional and National History
- Social and Cultural History
- Theory, Methods, and Historiography
- Browse content in Literature
- Literary Studies (Romanticism)
- Literary Studies (European)
- Literary Studies - World
- Literary Studies (19th Century)
- Literary Studies (African American Literature)
- Literary Studies (Early and Medieval)
- Literary Studies (Poetry and Poets)
- Literary Studies (Women's Writing)
- Literary Theory and Cultural Studies
- Mythology and Folklore
- Shakespeare Studies and Criticism
- Media Studies
- Browse content in Music
- Musical Structures, Styles, and Techniques
- Musicology and Music History
- Browse content in Philosophy
- Aesthetics and Philosophy of Art
- History of Western Philosophy
- Metaphysics
- Moral Philosophy
- Philosophy of Language
- Philosophy of Science
- Philosophy of Religion
- Social and Political Philosophy
- Browse content in Religion
- Biblical Studies
- Christianity
- History of Religion
- Judaism and Jewish Studies
- Religious Studies
- Society and Culture
- Browse content in Law
- Company and Commercial Law
- Comparative Law
- Constitutional and Administrative Law
- Criminal Law
- History of Law
- Browse content in Science and Mathematics
- Browse content in Biological Sciences
- Aquatic Biology
- Biochemistry
- Ecology and Conservation
- Evolutionary Biology
- Genetics and Genomics
- Molecular and Cell Biology
- Zoology and Animal Sciences
- Browse content in Computer Science
- Programming Languages
- Environmental Science
- History of Science and Technology
- Browse content in Mathematics
- Applied Mathematics
- History of Mathematics
- Mathematical Education
- Mathematical Finance
- Mathematical Analysis
- Numerical and Computational Mathematics
- Probability and Statistics
- Pure Mathematics
- Browse content in Neuroscience
- Cognition and Behavioural Neuroscience
- Development of the Nervous System
- Browse content in Physics
- Astronomy and Astrophysics
- Biological and Medical Physics
- Computational Physics
- Condensed Matter Physics
- History of Physics
- Mathematical and Statistical Physics
- Browse content in Psychology
- Cognitive Neuroscience
- Social Psychology
- Browse content in Social Sciences
- Browse content in Anthropology
- Anthropology of Religion
- Regional Anthropology
- Social and Cultural Anthropology
- Browse content in Business and Management
- Business History
- Industry Studies
- International Business
- Knowledge Management
- Public and Nonprofit Management
- Criminology and Criminal Justice
- Browse content in Economics
- Asian Economics
- Behavioural Economics and Neuroeconomics
- Econometrics and Mathematical Economics
- Economic History
- Economic Development and Growth
- Financial Markets
- Financial Institutions and Services
- History of Economic Thought
- International Economics
- Macroeconomics and Monetary Economics
- Microeconomics
- Browse content in Education
- Higher and Further Education
- Browse content in Politics
- Asian Politics
- Comparative Politics
- Conflict Politics
- Environmental Politics
- International Relations
- Political Economy
- Political Sociology
- Political Theory
- Public Policy
- Security Studies
- UK Politics
- US Politics
- Browse content in Regional and Area Studies
- Middle Eastern Studies
- Native American Studies
- Browse content in Social Work
- Social Work and Crime and Justice
- Browse content in Sociology
- Comparative and Historical Sociology
- Economic Sociology
- Gender and Sexuality
- Health, Illness, and Medicine
- Migration Studies
- Occupations, Professions, and Work
- Population and Demography
- Race and Ethnicity
- Social Theory
- Social Movements and Social Change
- Social Research and Statistics
- Social Stratification, Inequality, and Mobility
- Sociology of Religion
- Sociology of Education
- Urban and Rural Studies
- Reviews and Awards
- Journals on Oxford Academic
- Books on Oxford Academic

- < Previous chapter
- Next chapter >
3 Causes-of-Effects versus Effects-of-Causes
- Published: September 2012
- Cite Icon Cite
- Permissions Icon Permissions
This chapter examines two approaches used in social science research: the “causes-of-effects” approach and the “effects-of-causes” approach. The quantitative and qualitative cultures differ in the extent to which and the ways in which they address causes-of-effects and effects-of-causes questions. Quantitative scholars, who favor the effects-of-causes approach, focus on estimating the average effects of particular variables within populations or samples. By contrast, qualitative scholars employ individual case analysis to explain outcomes as well as the effects of particular causal factors. The chapter first considers the type of research question addressed by both quantitative and qualitative researchers before discussing the use of within-case analysis by the latter to investigate individual cases versus cross-case analysis by the former to elucidate central tendencies in populations. It also describes the complementarities between qualitative and quantitative research that make mixed-method research possible.
Personal account
- Sign in with email/username & password
- Get email alerts
- Save searches
- Purchase content
- Activate your purchase/trial code
- Add your ORCID iD
Institutional access
Sign in with a library card.
- Sign in with username/password
- Recommend to your librarian
- Institutional account management
- Get help with access
Access to content on Oxford Academic is often provided through institutional subscriptions and purchases. If you are a member of an institution with an active account, you may be able to access content in one of the following ways:
IP based access
Typically, access is provided across an institutional network to a range of IP addresses. This authentication occurs automatically, and it is not possible to sign out of an IP authenticated account.
Choose this option to get remote access when outside your institution. Shibboleth/Open Athens technology is used to provide single sign-on between your institution’s website and Oxford Academic.
- Click Sign in through your institution.
- Select your institution from the list provided, which will take you to your institution's website to sign in.
- When on the institution site, please use the credentials provided by your institution. Do not use an Oxford Academic personal account.
- Following successful sign in, you will be returned to Oxford Academic.
If your institution is not listed or you cannot sign in to your institution’s website, please contact your librarian or administrator.
Enter your library card number to sign in. If you cannot sign in, please contact your librarian.
Society Members
Society member access to a journal is achieved in one of the following ways:
Sign in through society site
Many societies offer single sign-on between the society website and Oxford Academic. If you see ‘Sign in through society site’ in the sign in pane within a journal:
- Click Sign in through society site.
- When on the society site, please use the credentials provided by that society. Do not use an Oxford Academic personal account.
If you do not have a society account or have forgotten your username or password, please contact your society.
Sign in using a personal account
Some societies use Oxford Academic personal accounts to provide access to their members. See below.
A personal account can be used to get email alerts, save searches, purchase content, and activate subscriptions.
Some societies use Oxford Academic personal accounts to provide access to their members.
Viewing your signed in accounts
Click the account icon in the top right to:
- View your signed in personal account and access account management features.
- View the institutional accounts that are providing access.
Signed in but can't access content
Oxford Academic is home to a wide variety of products. The institutional subscription may not cover the content that you are trying to access. If you believe you should have access to that content, please contact your librarian.
For librarians and administrators, your personal account also provides access to institutional account management. Here you will find options to view and activate subscriptions, manage institutional settings and access options, access usage statistics, and more.
Our books are available by subscription or purchase to libraries and institutions.
Month: | Total Views: |
---|---|
October 2022 | 8 |
November 2022 | 2 |
December 2022 | 2 |
January 2023 | 5 |
February 2023 | 5 |
March 2023 | 4 |
April 2023 | 5 |
May 2023 | 4 |
June 2023 | 11 |
July 2023 | 2 |
August 2023 | 13 |
September 2023 | 8 |
October 2023 | 5 |
November 2023 | 4 |
December 2023 | 5 |
January 2024 | 15 |
February 2024 | 7 |
March 2024 | 4 |
April 2024 | 8 |
May 2024 | 6 |
June 2024 | 3 |
- About Oxford Academic
- Publish journals with us
- University press partners
- What we publish
- New features
- Open access
- Rights and permissions
- Accessibility
- Advertising
- Media enquiries
- Oxford University Press
- Oxford Languages
- University of Oxford
Oxford University Press is a department of the University of Oxford. It furthers the University's objective of excellence in research, scholarship, and education by publishing worldwide
- Copyright © 2024 Oxford University Press
- Cookie settings
- Cookie policy
- Privacy policy
- Legal notice
This Feature Is Available To Subscribers Only
Sign In or Create an Account
This PDF is available to Subscribers Only
For full access to this pdf, sign in to an existing account, or purchase an annual subscription.
- Login to Survey Tool Review Center
How to Use Surveys in Cause-Effect Research
Summary: to understand cause and effect, we need to conduct experiments. experiments may include surveys as a data collection method, but surveys in themselves can’t provide the answer..
3 minutes to read. By author Michaela Mora on July 29, 2019 Topics: Analysis Techniques , Market Research , Survey Design
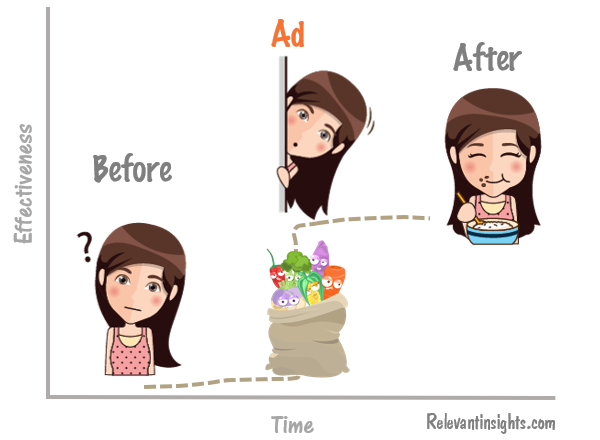
Cause-effect research requires special research designs. However, many assume that surveys can uncover cause and effect links without much consideration.
Not long ago I got a call from a potential client asking for research to determine why a recent marketing campaign failed to increase sales, despite a significant increase in awareness.
He had conducted an advertising awareness survey, and the results showed that many in the target audience had noted the advertising and gave it high ratings, but didn’t make a purchase.
All possible explanations were mere speculations. He couldn’t pinpoint any particular cause for this.
The main problem was that he looked for evidence of a cause-effect link, but the research design was not appropriate for that.
Cause-Effect Research=Experiment
The main method for cause-effect research is experimentation . In experimental-based research, we manipulate the causal or independent variables in a relatively controlled environment. This means that we control and monitor other variables affecting the dependent variable (e.g. sales) as much as possible.
In this case, the client had conducted the survey and analyzed the data without taking into account the effectiveness of different marketing collaterals, market penetration, competitor activity, and some characteristics of the purchase decision-makers.
After doing some digging around, we uncovered that in some markets, competitors had launched high-frequency advertising campaigns. This helped the client indirectly by increasing category awareness, but not his sales.
Moreover, the program targeted recent buyers who probably didn’t have a need for his products at that particular moment.
Marketing Experiments
Surveys that are not part of an experimental approach may show correlations, but not causality .
To really connect the dots between cause and effect, we needed to create an experiment. This would include different renditions of the marketing collaterals, different markets, customers at different stages in the purchase cycle, and actions taken by competitors.
Experimentation in marketing has traditionally taken the form of standard test markets. In this approach, you launch controlled advertising in designated markets and sell the product through regular distribution channels.
However, these tests can be time-consuming, are often expensive, and may be difficult to administer.
Market Simulations
Simulated test markets are a more affordable solution. In this approach, we expose individuals to the product or concept (e.g. via actual marketing collaterals), and give them the opportunity to buy it. If they buy it, we ask them to evaluate the product and state their repeat purchase intent.
We can then combine trial and repeat estimates with data about promotions, distribution levels, competitor activity, and other relevant pieces of information.
User Research (UX)
Another experimentation channel is the popular freemium model , which mimics this process to some extent. The basic principle is to let people try it and observe what decision they make.
After this, we can follow up with research to understand what drove their decision while controlling for other variables that may affect the outcome. This approach goes deeper into user research .
Experiments are the Answer
In short, if you want to understand cause and effect, you need to conduct experiments.
Experiments may include surveys as a data collection method, but surveys in themselves can’t provide the answer. It is the experimental design that will lead you to it.
(An earlier version of this article was originally published on January 18, 2012. The article was last updated and revised on July 29, 2019.)
Related Articles
- What To Consider in Survey Design
- Is It Right to Include a Neutral Point in Rating Questions?
- Multi-Response or Dichotomous Grid Questions?
- Avoid These Survey Design Mistakes
- How To Minimize Memory Errors in Surveys
- The Accuracy of Election Polls
- How Bad Surveys Can Turn Respondents Off
- 10 Cognitive Biases You Shouldn’t Ignore In Research
- Which Rating Scales Should I Use?
- Survey Gamification? It’s About Good Survey Design
- How Survey Tools May Improve User Experience
- Guidelines to Write Attitudinal Questions in Surveys
- Why We Need to Avoid Long Surveys
- An Alternative To Multiple-Choice Questions
- Validity and Reliability in Surveys
- Making The Case For MaxDiff
- How To Improve Online Survey Response Rates
- Why Conjoint Analysis Is Best for Price Research
- Making the Case Against the Van Westendorp Price Sensitivity Meter
- Comparing Willingness To Pay Measurements To Real Purchase
- 6 Decisions To Make When Designing Product Concept Tests
- What Is The Right Sample Size For A Survey?
- 12 Research Techniques to Solve Choice Overload
- UX Research Methods For User-Centric Design
- 10 Key Pieces of Advice On How to Do And Use Market Research
Subscribe to our newsletter to get notified about future articles
Subscribe and don’t miss anything!
Recent Articles
- How AI Can Further Remove Researchers in Search of Productivity and Lower Costs
- Re: Design/Growth Podcast – Researching User Experiences for Business Growth
- Why You Need Positioning Concept Testing in New Product Development
- The Rise of UX
- How to Future-Proof Experience Management and Your Business
- When Using Focus Groups Makes Sense
- How to Make Segmentation Research Actionable
- How To Integrate Market Research and UX Research for Desired Business Outcomes
Popular Articles
- Step by Step Guide to the Market Research Process
- Write Winning Product Concepts To Get Accurate Results In Concept Tests
- What Is Market Research?
- How to Use Qualitative and Quantitative Research in Product Development
- The Opportunity of UX Research Webinar
- Myths & Misunderstandings About UX – MR Realities Podcast
- Concept Testing for UX Researchers
- UX Research Geeks Podcast – Using Market Research for Better Context in UX
- A Researcher’s Path – Data Stories Leaders At Work Podcast
- How to Leverage UX and Market Research To Understand Your Customers
- How To Improve Racial and Gender Inclusion in Survey Design
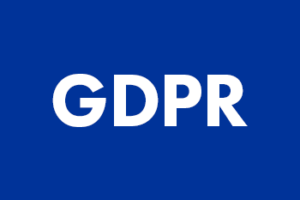
- Privacy Overview
- Strictly Necessary Cookies
This website uses cookies so that we can provide you with the best user experience possible. Cookie information is stored in your browser and performs functions such as recognising you when you return to our website and helping our team to understand which sections of the website you find most interesting and useful.
Strictly Necessary Cookie should be enabled at all times so that we can save your preferences for cookie settings.
If you disable this cookie, we will not be able to save your preferences. This means that every time you visit this website you will need to enable or disable cookies again.
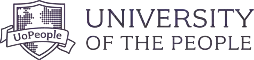
Higher Education News , Tips for Online Students , Tips for Students
A Comprehensive Guide to Different Types of Research

Updated: June 19, 2024
Published: June 15, 2024
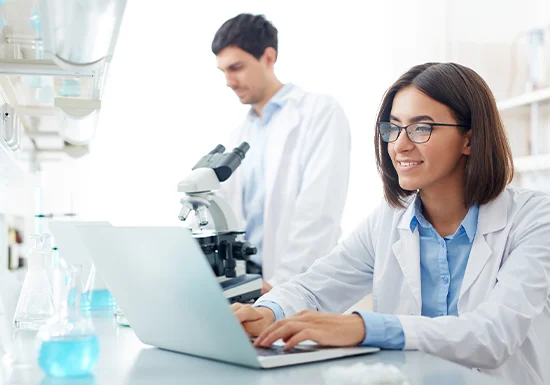
When embarking on a research project, selecting the right methodology can be the difference between success and failure. With various methods available, each suited to different types of research, it’s essential you make an informed choice. This blog post will provide tips on how to choose a research methodology that best fits your research goals .
We’ll start with definitions: Research is the systematic process of exploring, investigating, and discovering new information or validating existing knowledge. It involves defining questions, collecting data, analyzing results, and drawing conclusions.
Meanwhile, a research methodology is a structured plan that outlines how your research is to be conducted. A complete methodology should detail the strategies, processes, and techniques you plan to use for your data collection and analysis.
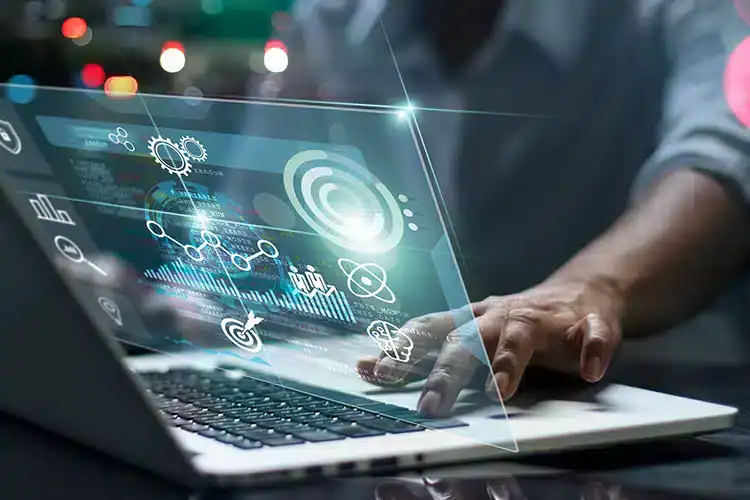
Research Methods
The first step of a research methodology is to identify a focused research topic, which is the question you seek to answer. By setting clear boundaries on the scope of your research, you can concentrate on specific aspects of a problem without being overwhelmed by information. This will produce more accurate findings.
Along with clarifying your research topic, your methodology should also address your research methods. Let’s look at the four main types of research: descriptive, correlational, experimental, and diagnostic.
Descriptive Research
Descriptive research is an approach designed to describe the characteristics of a population systematically and accurately. This method focuses on answering “what” questions by providing detailed observations about the subject. Descriptive research employs surveys, observational studies , and case studies to gather qualitative or quantitative data.
A real-world example of descriptive research is a survey investigating consumer behavior toward a competitor’s product. By analyzing the survey results, the company can gather detailed insights into how consumers perceive a competitor’s product, which can inform their marketing strategies and product development.
Correlational Research
Correlational research examines the statistical relationship between two or more variables to determine whether a relationship exists. Correlational research is particularly useful when ethical or practical constraints prevent experimental manipulation. It is often employed in fields such as psychology, education, and health sciences to provide insights into complex real-world interactions, helping to develop theories and inform further experimental research.
An example of correlational research is the study of the relationship between smoking and lung cancer. Researchers observe and collect data on individuals’ smoking habits and the incidence of lung cancer to determine if there is a correlation between the two variables. This type of research helps identify patterns and relationships, indicating whether increased smoking is associated with higher rates of lung cancer.
Experimental Research
Experimental research is a scientific approach where researchers manipulate one or more independent variables to observe their effect on a dependent variable. This method is designed to establish cause-and-effect relationships. Fields like psychology , medicine, and social sciences frequently employ experimental research to test hypotheses and theories under controlled conditions.
A real-world example of experimental research is Pavlov’s Dog experiment. In this experiment, Ivan Pavlov demonstrated classical conditioning by ringing a bell each time he fed his dogs. After repeating this process multiple times, the dogs began to salivate just by hearing the bell, even when no food was presented. This experiment helped to illustrate how certain stimuli can elicit specific responses through associative learning.
Diagnostic Research
Diagnostic research tries to accurately diagnose a problem by identifying its underlying causes. This type of research is crucial for understanding complex situations where a precise diagnosis is necessary for formulating effective solutions. It involves methods such as case studies and data analysis and often integrates both qualitative and quantitative data to provide a comprehensive view of the issue at hand.
An example of diagnostic research is studying the causes of a specific illness outbreak. During an outbreak of a respiratory virus, researchers might conduct diagnostic research to determine the factors contributing to the spread of the virus. This could involve analyzing patient data, testing environmental samples, and evaluating potential sources of infection. The goal is to identify the root causes and contributing factors to develop effective containment and prevention strategies.
Using an established research method is imperative, no matter if you are researching for marketing , technology , healthcare , engineering, or social science. A methodology lends legitimacy to your research by ensuring your data is both consistent and credible. A well-defined methodology also enhances the reliability and validity of the research findings, which is crucial for drawing accurate and meaningful conclusions.
Additionally, methodologies help researchers stay focused and on track, limiting the scope of the study to relevant questions and objectives. This not only improves the quality of the research but also ensures that the study can be replicated and verified by other researchers, further solidifying its scientific value.
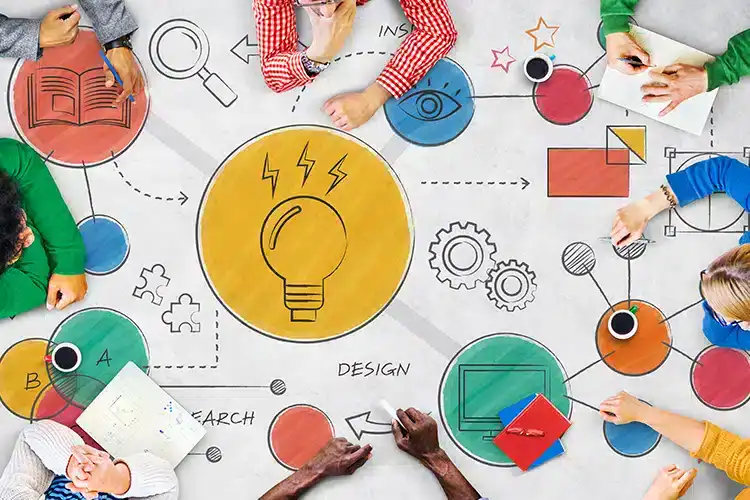
How to Choose a Research Methodology
Choosing the best research methodology for your project involves several key steps to ensure that your approach aligns with your research goals and questions. Here’s a simplified guide to help you make the best choice.
Understand Your Goals
Clearly define the objectives of your research. What do you aim to discover, prove, or understand? Understanding your goals helps in selecting a methodology that aligns with your research purpose.
Consider the Nature of Your Data
Determine whether your research will involve numerical data, textual data, or both. Quantitative methods are best for numerical data, while qualitative methods are suitable for textual or thematic data.
Understand the Purpose of Each Methodology
Becoming familiar with the four types of research – descriptive, correlational, experimental, and diagnostic – will enable you to select the most appropriate method for your research. Many times, you will want to use a combination of methods to gather meaningful data.
Evaluate Resources and Constraints
Consider the resources available to you, including time, budget, and access to data. Some methodologies may require more resources or longer timeframes to implement effectively.
Review Similar Studies
Look at previous research in your field to see which methodologies were successful. This can provide insights and help you choose a proven approach.
By following these steps, you can select a research methodology that best fits your project’s requirements and ensures robust, credible results.
Completing Your Research Project
Upon completing your research, the next critical step is to analyze and interpret the data you’ve collected. This involves summarizing the key findings, identifying patterns, and determining how these results address your initial research questions. By thoroughly examining the data, you can draw meaningful conclusions that contribute to the body of knowledge in your field.
It’s essential that you present these findings clearly and concisely, using charts, graphs, and tables to enhance comprehension. Furthermore, discuss the implications of your results, any limitations encountered during the study, and how your findings align with or challenge existing theories.
Your research project should conclude with a strong statement that encapsulates the essence of your research and its broader impact. This final section should leave readers with a clear understanding of the value of your work and inspire continued exploration and discussion in the field.
Now that you know how to perform quality research , it’s time to get started! Applying the right research methodologies can make a significant difference in the accuracy and reliability of your findings. Remember, the key to successful research is not just in collecting data, but in analyzing it thoughtfully and systematically to draw meaningful conclusions. So, dive in, explore, and contribute to the ever-growing body of knowledge with confidence. Happy researching!
At UoPeople, our blog writers are thinkers, researchers, and experts dedicated to curating articles relevant to our mission: making higher education accessible to everyone.
Related Articles

Search the United Nations
- What Is Climate Change
- Myth Busters
- Renewable Energy
- Finance & Justice
- Initiatives
- Sustainable Development Goals
- Paris Agreement
- Climate Ambition Summit 2023
- Climate Conferences
- Press Material
- Communications Tips
Causes and Effects of Climate Change
Fossil fuels – coal, oil and gas – are by far the largest contributor to global climate change, accounting for over 75 per cent of global greenhouse gas emissions and nearly 90 per cent of all carbon dioxide emissions.
As greenhouse gas emissions blanket the Earth, they trap the sun’s heat. This leads to global warming and climate change. The world is now warming faster than at any point in recorded history. Warmer temperatures over time are changing weather patterns and disrupting the usual balance of nature. This poses many risks to human beings and all other forms of life on Earth.
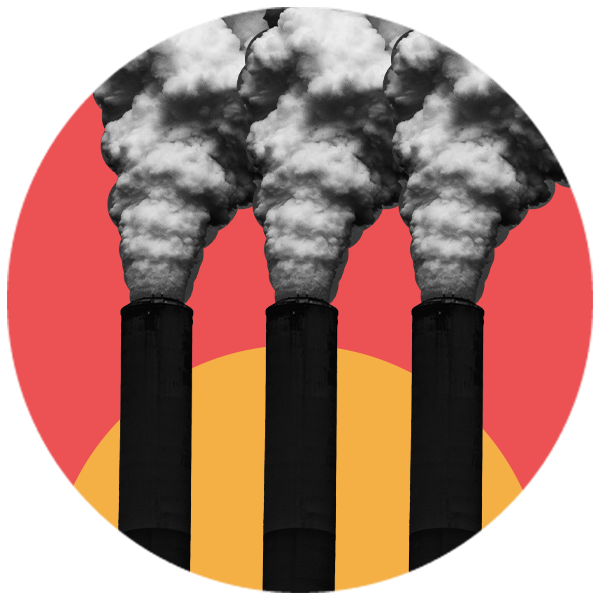
Causes of Climate Change
Generating power
Generating electricity and heat by burning fossil fuels causes a large chunk of global emissions. Most electricity is still generated by burning coal, oil, or gas, which produces carbon dioxide and nitrous oxide – powerful greenhouse gases that blanket the Earth and trap the sun’s heat. Globally, a bit more than a quarter of electricity comes from wind, solar and other renewable sources which, as opposed to fossil fuels, emit little to no greenhouse gases or pollutants into the air.
Manufacturing goods
Manufacturing and industry produce emissions, mostly from burning fossil fuels to produce energy for making things like cement, iron, steel, electronics, plastics, clothes, and other goods. Mining and other industrial processes also release gases, as does the construction industry. Machines used in the manufacturing process often run on coal, oil, or gas; and some materials, like plastics, are made from chemicals sourced from fossil fuels. The manufacturing industry is one of the largest contributors to greenhouse gas emissions worldwide.
Cutting down forests
Cutting down forests to create farms or pastures, or for other reasons, causes emissions, since trees, when they are cut, release the carbon they have been storing. Each year approximately 12 million hectares of forest are destroyed. Since forests absorb carbon dioxide, destroying them also limits nature’s ability to keep emissions out of the atmosphere. Deforestation, together with agriculture and other land use changes, is responsible for roughly a quarter of global greenhouse gas emissions.
Using transportation
Most cars, trucks, ships, and planes run on fossil fuels. That makes transportation a major contributor of greenhouse gases, especially carbon-dioxide emissions. Road vehicles account for the largest part, due to the combustion of petroleum-based products, like gasoline, in internal combustion engines. But emissions from ships and planes continue to grow. Transport accounts for nearly one quarter of global energy-related carbon-dioxide emissions. And trends point to a significant increase in energy use for transport over the coming years.
Producing food
Producing food causes emissions of carbon dioxide, methane, and other greenhouse gases in various ways, including through deforestation and clearing of land for agriculture and grazing, digestion by cows and sheep, the production and use of fertilizers and manure for growing crops, and the use of energy to run farm equipment or fishing boats, usually with fossil fuels. All this makes food production a major contributor to climate change. And greenhouse gas emissions also come from packaging and distributing food.
Powering buildings
Globally, residential and commercial buildings consume over half of all electricity. As they continue to draw on coal, oil, and natural gas for heating and cooling, they emit significant quantities of greenhouse gas emissions. Growing energy demand for heating and cooling, with rising air-conditioner ownership, as well as increased electricity consumption for lighting, appliances, and connected devices, has contributed to a rise in energy-related carbon-dioxide emissions from buildings in recent years.
Consuming too much
Your home and use of power, how you move around, what you eat and how much you throw away all contribute to greenhouse gas emissions. So does the consumption of goods such as clothing, electronics, and plastics. A large chunk of global greenhouse gas emissions are linked to private households. Our lifestyles have a profound impact on our planet. The wealthiest bear the greatest responsibility: the richest 1 per cent of the global population combined account for more greenhouse gas emissions than the poorest 50 per cent.
Based on various UN sources
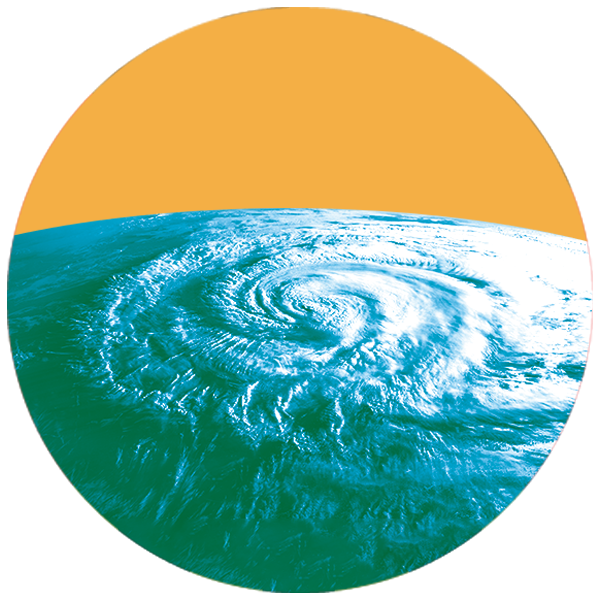
Effects of Climate Change
Hotter temperatures
As greenhouse gas concentrations rise, so does the global surface temperature. The last decade, 2011-2020, is the warmest on record. Since the 1980s, each decade has been warmer than the previous one. Nearly all land areas are seeing more hot days and heat waves. Higher temperatures increase heat-related illnesses and make working outdoors more difficult. Wildfires start more easily and spread more rapidly when conditions are hotter. Temperatures in the Arctic have warmed at least twice as fast as the global average.
More severe storms
Destructive storms have become more intense and more frequent in many regions. As temperatures rise, more moisture evaporates, which exacerbates extreme rainfall and flooding, causing more destructive storms. The frequency and extent of tropical storms is also affected by the warming ocean. Cyclones, hurricanes, and typhoons feed on warm waters at the ocean surface. Such storms often destroy homes and communities, causing deaths and huge economic losses.
Increased drought
Climate change is changing water availability, making it scarcer in more regions. Global warming exacerbates water shortages in already water-stressed regions and is leading to an increased risk of agricultural droughts affecting crops, and ecological droughts increasing the vulnerability of ecosystems. Droughts can also stir destructive sand and dust storms that can move billions of tons of sand across continents. Deserts are expanding, reducing land for growing food. Many people now face the threat of not having enough water on a regular basis.
A warming, rising ocean
The ocean soaks up most of the heat from global warming. The rate at which the ocean is warming strongly increased over the past two decades, across all depths of the ocean. As the ocean warms, its volume increases since water expands as it gets warmer. Melting ice sheets also cause sea levels to rise, threatening coastal and island communities. In addition, the ocean absorbs carbon dioxide, keeping it from the atmosphere. But more carbon dioxide makes the ocean more acidic, which endangers marine life and coral reefs.
Loss of species
Climate change poses risks to the survival of species on land and in the ocean. These risks increase as temperatures climb. Exacerbated by climate change, the world is losing species at a rate 1,000 times greater than at any other time in recorded human history. One million species are at risk of becoming extinct within the next few decades. Forest fires, extreme weather, and invasive pests and diseases are among many threats related to climate change. Some species will be able to relocate and survive, but others will not.
Not enough food
Changes in the climate and increases in extreme weather events are among the reasons behind a global rise in hunger and poor nutrition. Fisheries, crops, and livestock may be destroyed or become less productive. With the ocean becoming more acidic, marine resources that feed billions of people are at risk. Changes in snow and ice cover in many Arctic regions have disrupted food supplies from herding, hunting, and fishing. Heat stress can diminish water and grasslands for grazing, causing declining crop yields and affecting livestock.
More health risks
Climate change is the single biggest health threat facing humanity. Climate impacts are already harming health, through air pollution, disease, extreme weather events, forced displacement, pressures on mental health, and increased hunger and poor nutrition in places where people cannot grow or find sufficient food. Every year, environmental factors take the lives of around 13 million people. Changing weather patterns are expanding diseases, and extreme weather events increase deaths and make it difficult for health care systems to keep up.
Poverty and displacement
Climate change increases the factors that put and keep people in poverty. Floods may sweep away urban slums, destroying homes and livelihoods. Heat can make it difficult to work in outdoor jobs. Water scarcity may affect crops. Over the past decade (2010–2019), weather-related events displaced an estimated 23.1 million people on average each year, leaving many more vulnerable to poverty. Most refugees come from countries that are most vulnerable and least ready to adapt to the impacts of climate change.
Learn more about...
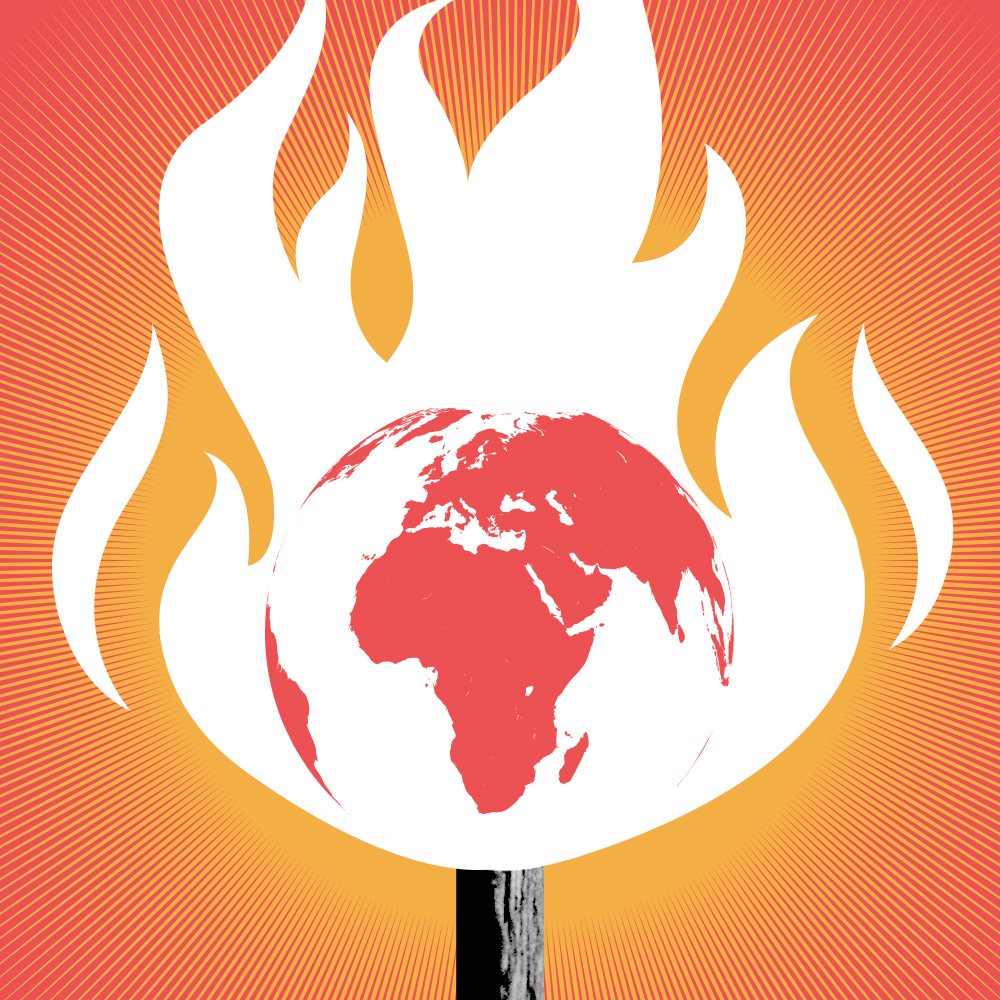
- What is climate change?
Our climate 101 offers a quick take on the how and why of climate change.
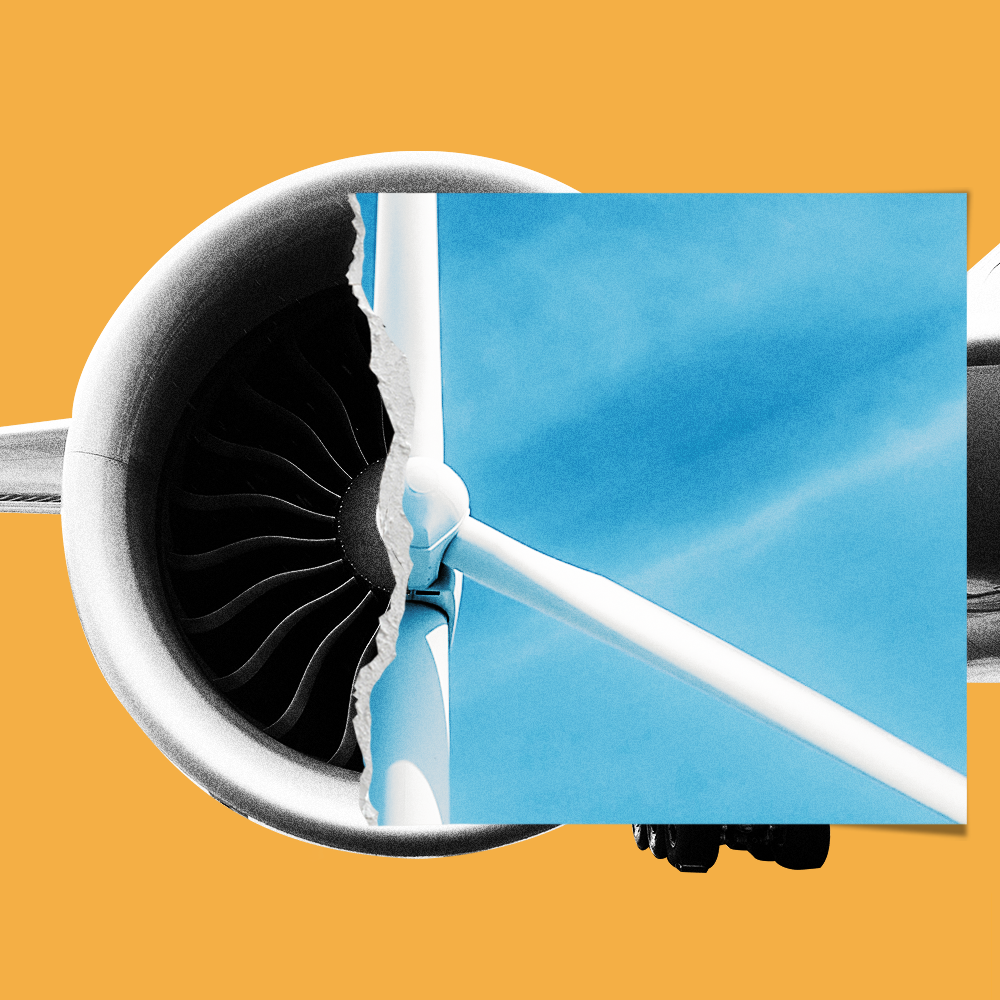
What is “net zero”, why is it important, and is the world on track to reach it?
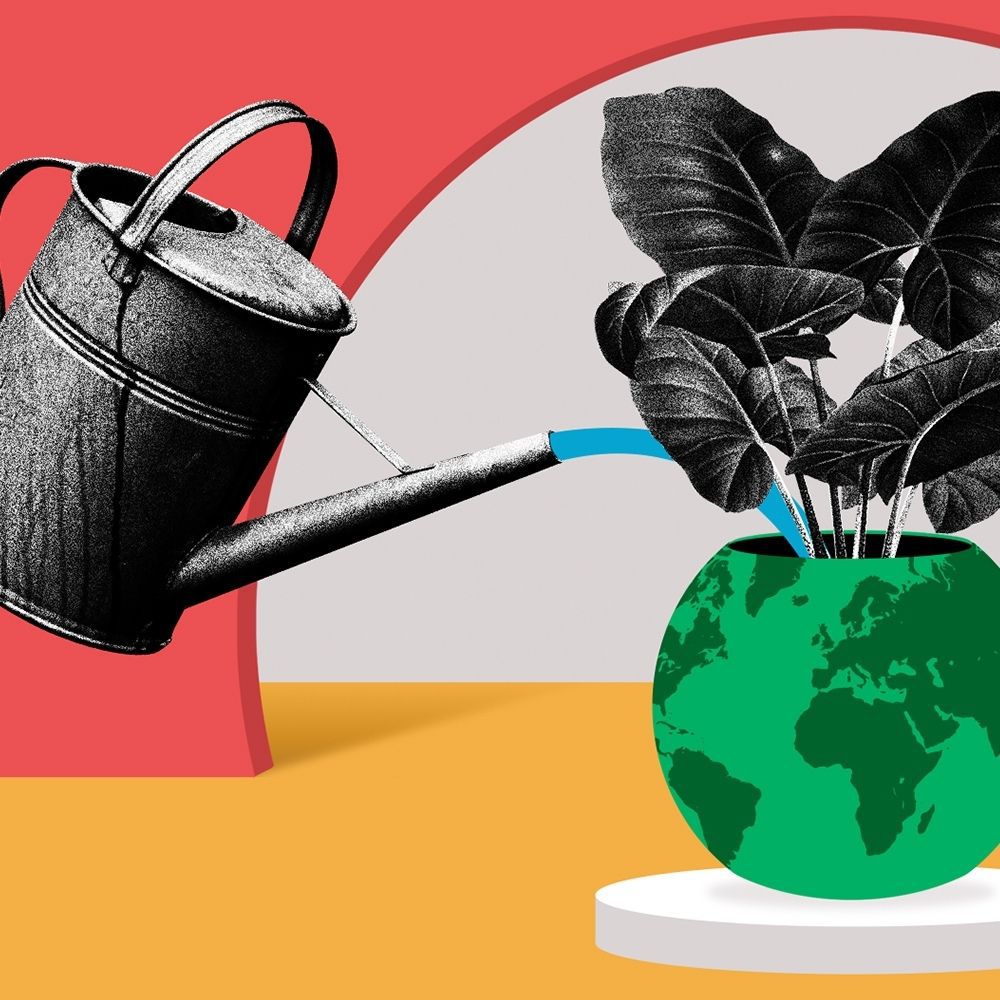
Initiatives for action
Read about global initiatives aimed at speeding up the pace of climate action.
Facts and figures
- Causes and effects
- Myth busters
Cutting emissions
- Explaining net zero
- High-level expert group on net zero
- Checklists for credibility of net-zero pledges
- Greenwashing
- What you can do
Clean energy
- Renewable energy – key to a safer future
- What is renewable energy
- Five ways to speed up the energy transition
- Why invest in renewable energy
- Clean energy stories
- A just transition
Adapting to climate change
- Climate adaptation
- Early warnings for all
- Youth voices
Financing climate action
- Finance and justice
- Loss and damage
- $100 billion commitment
- Why finance climate action
- Biodiversity
- Human Security
International cooperation
- What are Nationally Determined Contributions
- Acceleration Agenda
- Climate Ambition Summit
- Climate conferences (COPs)
- Youth Advisory Group
- Action initiatives
- Secretary-General’s speeches
- Press material
- Fact sheets
- Communications tips
Here’s how you know
- U.S. Department of Health and Human Services
- National Institutes of Health
Probiotics: What You Need To Know
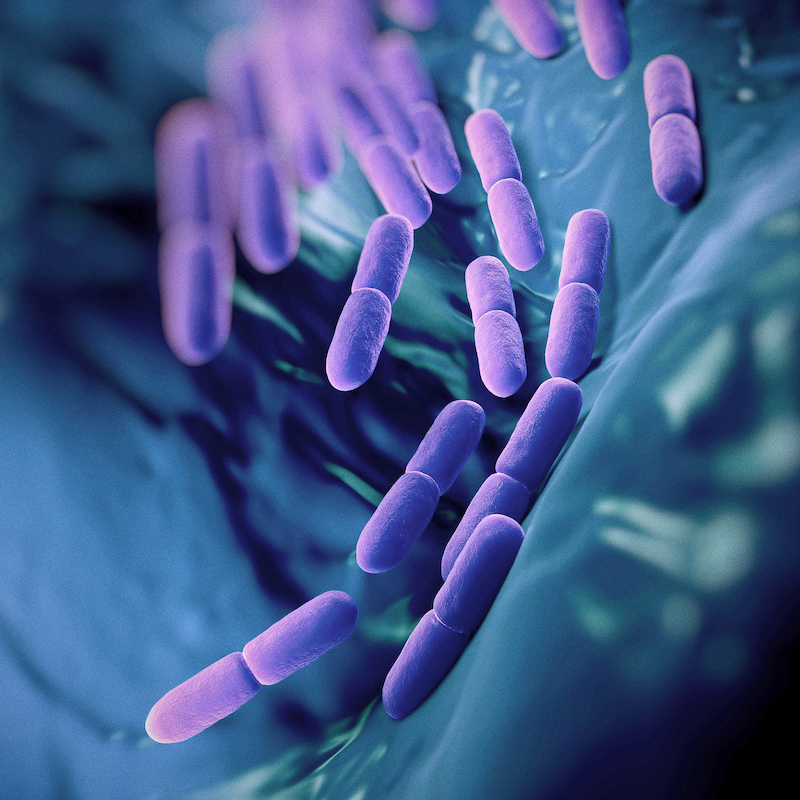
.header_greentext{color:green!important;font-size:24px!important;font-weight:500!important;}.header_bluetext{color:blue!important;font-size:18px!important;font-weight:500!important;}.header_redtext{color:red!important;font-size:28px!important;font-weight:500!important;}.header_darkred{color:#803d2f!important;font-size:28px!important;font-weight:500!important;}.header_purpletext{color:purple!important;font-size:31px!important;font-weight:500!important;}.header_yellowtext{color:yellow!important;font-size:20px!important;font-weight:500!important;}.header_blacktext{color:black!important;font-size:22px!important;font-weight:500!important;}.header_whitetext{color:white!important;font-size:22px!important;font-weight:500!important;}.header_darkred{color:#803d2f!important;}.Green_Header{color:green!important;font-size:24px!important;font-weight:500!important;}.Blue_Header{color:blue!important;font-size:18px!important;font-weight:500!important;}.Red_Header{color:red!important;font-size:28px!important;font-weight:500!important;}.Purple_Header{color:purple!important;font-size:31px!important;font-weight:500!important;}.Yellow_Header{color:yellow!important;font-size:20px!important;font-weight:500!important;}.Black_Header{color:black!important;font-size:22px!important;font-weight:500!important;}.White_Header{color:white!important;font-size:22px!important;font-weight:500!important;} What are probiotics?
Probiotics are live microorganisms that are intended to have health benefits when consumed or applied to the body. They can be found in yogurt and other fermented foods, dietary supplements , and beauty products. Cases of severe or fatal infections have been reported in premature infants who were given probiotics, and the U.S. Food and Drug Administration (FDA) has warned health care providers about this risk.
Although people often think of bacteria and other microorganisms as harmful “germs,” many are actually helpful. Some bacteria help digest food, destroy disease-causing cells, or produce vitamins. Many of the microorganisms in probiotic products are the same as or similar to microorganisms that naturally live in our bodies.
What types of bacteria are in probiotics?
Probiotics may contain a variety of microorganisms. The most common are bacteria that belong to groups called Lactobacillus and Bifidobacterium . Other bacteria may also be used as probiotics, and so may yeasts such as Saccharomyces boulardii .
Different types of probiotics may have different effects. For example, if a specific kind of Lactobacillus helps prevent an illness, that doesn’t necessarily mean that another kind of Lactobacillus or any of the Bifidobacterium probiotics would do the same thing.
Are prebiotics the same as probiotics?
No, prebiotics aren’t the same as probiotics. Prebiotics are nondigestible food components that selectively stimulate the growth or activity of desirable microorganisms.
What are synbiotics?
Synbiotics are products that combine probiotics and prebiotics.
How popular are probiotics?
The 2012 National Health Interview Survey (NHIS) showed that about 4 million (1.6 percent) U.S. adults had used probiotics or prebiotics in the past 30 days. Among adults, probiotics or prebiotics were the third most commonly used dietary supplement other than vitamins and minerals. The use of probiotics by adults quadrupled between 2007 and 2012. The 2012 NHIS also showed that 300,000 children age 4 to 17 (0.5 percent) had used probiotics or prebiotics in the 30 days before the survey.
How might probiotics work?
Probiotics may have a variety of effects in the body, and different probiotics may act in different ways.
Probiotics might:
- Help your body maintain a healthy community of microorganisms or help your body’s community of microorganisms return to a healthy condition after being disturbed
- Produce substances that have desirable effects
- Influence your body’s immune response.
How are probiotics regulated in the United States?
Government regulation of probiotics in the United States is complex. Depending on a probiotic product’s intended use, the FDA might regulate it as a dietary supplement, a food ingredient, or a drug.
Many probiotics are sold as dietary supplements, which don’t require FDA approval before they are marketed. Dietary supplement labels may make claims about how the product affects the structure or function of the body without FDA approval, but they aren’t allowed to make health claims, such as saying the supplement lowers your risk of getting a disease, without the FDA’s consent.
If a probiotic is going to be marketed as a drug for treatment of a disease or disorder, it has to meet stricter requirements. It must be proven safe and effective for its intended use through clinical trials and be approved by the FDA before it can be sold.
.header_greentext{color:green!important;font-size:24px!important;font-weight:500!important;}.header_bluetext{color:blue!important;font-size:18px!important;font-weight:500!important;}.header_redtext{color:red!important;font-size:28px!important;font-weight:500!important;}.header_darkred{color:#803d2f!important;font-size:28px!important;font-weight:500!important;}.header_purpletext{color:purple!important;font-size:31px!important;font-weight:500!important;}.header_yellowtext{color:yellow!important;font-size:20px!important;font-weight:500!important;}.header_blacktext{color:black!important;font-size:22px!important;font-weight:500!important;}.header_whitetext{color:white!important;font-size:22px!important;font-weight:500!important;}.header_darkred{color:#803d2f!important;}.Green_Header{color:green!important;font-size:24px!important;font-weight:500!important;}.Blue_Header{color:blue!important;font-size:18px!important;font-weight:500!important;}.Red_Header{color:red!important;font-size:28px!important;font-weight:500!important;}.Purple_Header{color:purple!important;font-size:31px!important;font-weight:500!important;}.Yellow_Header{color:yellow!important;font-size:20px!important;font-weight:500!important;}.Black_Header{color:black!important;font-size:22px!important;font-weight:500!important;}.White_Header{color:white!important;font-size:22px!important;font-weight:500!important;} Learning About the Microbiome
The community of microorganisms that lives on us and in us is called the “microbiome,” and it’s a hot topic for research. The Human Microbiome Project, supported by the National Institutes of Health (NIH) from 2007 to 2016, played a key role in this research by mapping the normal bacteria that live in and on the healthy human body. With this understanding of a normal microbiome as the basis, researchers around the world, including many supported by NIH, are now exploring the links between changes in the microbiome and various diseases. They’re also developing new therapeutic approaches designed to modify the microbiome to treat disease and support health.
The National Center for Complementary and Integrative Health (NCCIH) is among the many agencies funding research on the microbiome. Researchers supported by NCCIH are studying the interactions between components of food and microorganisms in the digestive tract. The focus is on the ways in which diet-microbiome interactions may lead to the production of substances with beneficial health effects.
.header_greentext{color:green!important;font-size:24px!important;font-weight:500!important;}.header_bluetext{color:blue!important;font-size:18px!important;font-weight:500!important;}.header_redtext{color:red!important;font-size:28px!important;font-weight:500!important;}.header_darkred{color:#803d2f!important;font-size:28px!important;font-weight:500!important;}.header_purpletext{color:purple!important;font-size:31px!important;font-weight:500!important;}.header_yellowtext{color:yellow!important;font-size:20px!important;font-weight:500!important;}.header_blacktext{color:black!important;font-size:22px!important;font-weight:500!important;}.header_whitetext{color:white!important;font-size:22px!important;font-weight:500!important;}.header_darkred{color:#803d2f!important;}.Green_Header{color:green!important;font-size:24px!important;font-weight:500!important;}.Blue_Header{color:blue!important;font-size:18px!important;font-weight:500!important;}.Red_Header{color:red!important;font-size:28px!important;font-weight:500!important;}.Purple_Header{color:purple!important;font-size:31px!important;font-weight:500!important;}.Yellow_Header{color:yellow!important;font-size:20px!important;font-weight:500!important;}.Black_Header{color:black!important;font-size:22px!important;font-weight:500!important;}.White_Header{color:white!important;font-size:22px!important;font-weight:500!important;} What has science shown about the effectiveness of probiotics for health conditions?
A great deal of research has been done on probiotics, but much remains to be learned about whether they’re helpful and safe for various health conditions.
Probiotics have shown promise for a variety of health purposes, including prevention of antibiotic-associated diarrhea (including diarrhea caused by Clostridium difficile ), prevention of necrotizing enterocolitis and sepsis in premature infants, treatment of infant colic , treatment of periodontal disease , and induction or maintenance of remission in ulcerative colitis .
However, in most instances, we still don’t know which probiotics are helpful and which are not. We also don’t know how much of the probiotic people would have to take or who would be most likely to benefit. Even for the conditions that have been studied the most, researchers are still working toward finding the answers to these questions.
The following sections summarize the research on probiotics for some of the conditions for which they’ve been studied.
Gastrointestinal Conditions
.header_greentext{color:greenimportant;font-size:24pximportant;font-weight:500important;}.header_bluetext{color:blueimportant;font-size:18pximportant;font-weight:500important;}.header_redtext{color:redimportant;font-size:28pximportant;font-weight:500important;}.header_darkred{color:#803d2fimportant;font-size:28pximportant;font-weight:500important;}.header_purpletext{color:purpleimportant;font-size:31pximportant;font-weight:500important;}.header_yellowtext{color:yellowimportant;font-size:20pximportant;font-weight:500important;}.header_blacktext{color:blackimportant;font-size:22pximportant;font-weight:500important;}.header_whitetext{color:whiteimportant;font-size:22pximportant;font-weight:500important;}.header_darkred{color:#803d2fimportant;}.green_header{color:greenimportant;font-size:24pximportant;font-weight:500important;}.blue_header{color:blueimportant;font-size:18pximportant;font-weight:500important;}.red_header{color:redimportant;font-size:28pximportant;font-weight:500important;}.purple_header{color:purpleimportant;font-size:31pximportant;font-weight:500important;}.yellow_header{color:yellowimportant;font-size:20pximportant;font-weight:500important;}.black_header{color:blackimportant;font-size:22pximportant;font-weight:500important;}.white_header{color:whiteimportant;font-size:22pximportant;font-weight:500important;} antibiotic-associated diarrhea.
- Probiotics have been studied for antibiotic-associated diarrhea in general, as well as for antibiotic-associated diarrhea caused by one specific bacterium, Clostridium difficile . This section discusses the research on antibiotic-associated diarrhea in general. C. difficile is discussed in a separate section below.
- A 2017 review of 17 studies (3,631 total participants) in people who were not hospitalized indicated that giving probiotics to patients along with antibiotics was associated with a decrease of about half in the likelihood of antibiotic-associated diarrhea. However, this conclusion was considered tentative because the quality of the studies was only moderate. Patients who were given probiotics had no more side effects than patients who didn’t receive them.
- Probiotics may be helpful for antibiotic-associated diarrhea in young and middle-aged people, but a benefit has not been demonstrated in elderly people, according to a 2016 review of 30 studies (7,260 participants), 5 of which focused on people age 65 or older. It’s uncertain whether probiotics actually don’t work in elderly people or whether no effect was seen because there were only a few studies of people in this age group.
- A review of 23 studies (with 3,938 participants) of probiotics to prevent antibiotic-associated diarrhea in children provided moderate quality evidence that probiotics had a protective effect. No serious side effects were observed in children who were otherwise healthy, except for the infection for which they were being treated.
.header_greentext{color:green!important;font-size:24px!important;font-weight:500!important;}.header_bluetext{color:blue!important;font-size:18px!important;font-weight:500!important;}.header_redtext{color:red!important;font-size:28px!important;font-weight:500!important;}.header_darkred{color:#803d2f!important;font-size:28px!important;font-weight:500!important;}.header_purpletext{color:purple!important;font-size:31px!important;font-weight:500!important;}.header_yellowtext{color:yellow!important;font-size:20px!important;font-weight:500!important;}.header_blacktext{color:black!important;font-size:22px!important;font-weight:500!important;}.header_whitetext{color:white!important;font-size:22px!important;font-weight:500!important;}.header_darkred{color:#803d2f!important;}.Green_Header{color:green!important;font-size:24px!important;font-weight:500!important;}.Blue_Header{color:blue!important;font-size:18px!important;font-weight:500!important;}.Red_Header{color:red!important;font-size:28px!important;font-weight:500!important;}.Purple_Header{color:purple!important;font-size:31px!important;font-weight:500!important;}.Yellow_Header{color:yellow!important;font-size:20px!important;font-weight:500!important;}.Black_Header{color:black!important;font-size:22px!important;font-weight:500!important;}.White_Header{color:white!important;font-size:22px!important;font-weight:500!important;} Clostridium difficile Infection
- The bacterium Clostridium difficile can infect the colon (large intestine) of patients who have received antibiotics, causing diarrhea that can range from mild to severe. C. difficile infection is difficult to treat and sometimes comes back after treatment. It’s more common in people who take antibiotics long-term and in elderly people, and it can spread in hospitals and nursing homes. C. difficile infection affects about half a million people a year in the United States and causes about 15,000 deaths.
- A 2017 analysis of 31 studies (8,672 total patients) concluded that it is moderately certain that probiotics can reduce the risk of C. difficile diarrhea in adults and children who are receiving antibiotics. Most of these studies involved hospital patients. The analysis also concluded that the use of probiotics along with antibiotics appears to be safe, except for patients who are very weak or have poorly functioning immune systems.
- The types of probiotics that would be most useful in reducing the risk of C. difficile diarrhea, the length of time for which they should be taken, and the most appropriate doses are uncertain.
.header_greentext{color:green!important;font-size:24px!important;font-weight:500!important;}.header_bluetext{color:blue!important;font-size:18px!important;font-weight:500!important;}.header_redtext{color:red!important;font-size:28px!important;font-weight:500!important;}.header_darkred{color:#803d2f!important;font-size:28px!important;font-weight:500!important;}.header_purpletext{color:purple!important;font-size:31px!important;font-weight:500!important;}.header_yellowtext{color:yellow!important;font-size:20px!important;font-weight:500!important;}.header_blacktext{color:black!important;font-size:22px!important;font-weight:500!important;}.header_whitetext{color:white!important;font-size:22px!important;font-weight:500!important;}.header_darkred{color:#803d2f!important;}.Green_Header{color:green!important;font-size:24px!important;font-weight:500!important;}.Blue_Header{color:blue!important;font-size:18px!important;font-weight:500!important;}.Red_Header{color:red!important;font-size:28px!important;font-weight:500!important;}.Purple_Header{color:purple!important;font-size:31px!important;font-weight:500!important;}.Yellow_Header{color:yellow!important;font-size:20px!important;font-weight:500!important;}.Black_Header{color:black!important;font-size:22px!important;font-weight:500!important;}.White_Header{color:white!important;font-size:22px!important;font-weight:500!important;} Constipation
- A 2014 review of 14 studies (1,182 participants) of probiotics for constipation in adults showed some evidence of benefit, especially for Bifidobacterium lactis .
- A 2017 evaluation of 9 studies (778 participants) of probiotics for constipation in elderly people indicated that probiotics produced a small but meaningful benefit. The type of bacteria most often tested was Bifidobacterium longum . The researchers who performed the evaluation suggested that probiotics might be helpful for chronic constipation in older people as an addition to the usual forms of treatment.
- A 2017 review looked at 7 studies of probiotics for constipation in children (515 participants). The studies were hard to compare because of differences in the groups of children studied, the types of probiotics used, and other factors. The reviewers did not find evidence that any of the probiotics tested in the children were helpful. A second 2017 review, which included 4 of the same studies and 2 others (498 total participants in the 6 studies examined), took a more optimistic view of the evidence, noting that overall, probiotics did increase stool frequency, and that the effect was more noticeable in Asian than European children.
.header_greentext{color:green!important;font-size:24px!important;font-weight:500!important;}.header_bluetext{color:blue!important;font-size:18px!important;font-weight:500!important;}.header_redtext{color:red!important;font-size:28px!important;font-weight:500!important;}.header_darkred{color:#803d2f!important;font-size:28px!important;font-weight:500!important;}.header_purpletext{color:purple!important;font-size:31px!important;font-weight:500!important;}.header_yellowtext{color:yellow!important;font-size:20px!important;font-weight:500!important;}.header_blacktext{color:black!important;font-size:22px!important;font-weight:500!important;}.header_whitetext{color:white!important;font-size:22px!important;font-weight:500!important;}.header_darkred{color:#803d2f!important;}.Green_Header{color:green!important;font-size:24px!important;font-weight:500!important;}.Blue_Header{color:blue!important;font-size:18px!important;font-weight:500!important;}.Red_Header{color:red!important;font-size:28px!important;font-weight:500!important;}.Purple_Header{color:purple!important;font-size:31px!important;font-weight:500!important;}.Yellow_Header{color:yellow!important;font-size:20px!important;font-weight:500!important;}.Black_Header{color:black!important;font-size:22px!important;font-weight:500!important;}.White_Header{color:white!important;font-size:22px!important;font-weight:500!important;} Diarrhea Caused by Cancer Treatment
- Diarrhea is a common side effect of chemotherapy or radiotherapy for cancer. It’s been suggested that probiotics might help prevent or treat this type of diarrhea. However, a 2018 review of 12 studies (1,554 participants) found that the evidence for a beneficial effect of probiotics was inconclusive.
.header_greentext{color:green!important;font-size:24px!important;font-weight:500!important;}.header_bluetext{color:blue!important;font-size:18px!important;font-weight:500!important;}.header_redtext{color:red!important;font-size:28px!important;font-weight:500!important;}.header_darkred{color:#803d2f!important;font-size:28px!important;font-weight:500!important;}.header_purpletext{color:purple!important;font-size:31px!important;font-weight:500!important;}.header_yellowtext{color:yellow!important;font-size:20px!important;font-weight:500!important;}.header_blacktext{color:black!important;font-size:22px!important;font-weight:500!important;}.header_whitetext{color:white!important;font-size:22px!important;font-weight:500!important;}.header_darkred{color:#803d2f!important;}.Green_Header{color:green!important;font-size:24px!important;font-weight:500!important;}.Blue_Header{color:blue!important;font-size:18px!important;font-weight:500!important;}.Red_Header{color:red!important;font-size:28px!important;font-weight:500!important;}.Purple_Header{color:purple!important;font-size:31px!important;font-weight:500!important;}.Yellow_Header{color:yellow!important;font-size:20px!important;font-weight:500!important;}.Black_Header{color:black!important;font-size:22px!important;font-weight:500!important;}.White_Header{color:white!important;font-size:22px!important;font-weight:500!important;} Diverticular Disease
- In diverticulosis, small pouches develop at weak spots in the wall of the colon (large intestine). In most cases, this does not cause any symptoms. If symptoms (such as bloating, constipation, diarrhea, or cramping) do occur, the condition is called diverticular disease. If any of the pouches become inflamed, the condition is called diverticulitis. Patients with diverticulitis can have severe abdominal pain and may develop serious complications.
- A 2016 review of 11 studies (764 participants) of probiotics for diverticular disease was unable to reach conclusions on whether the probiotics were helpful because of the poor quality of the studies.
.header_greentext{color:green!important;font-size:24px!important;font-weight:500!important;}.header_bluetext{color:blue!important;font-size:18px!important;font-weight:500!important;}.header_redtext{color:red!important;font-size:28px!important;font-weight:500!important;}.header_darkred{color:#803d2f!important;font-size:28px!important;font-weight:500!important;}.header_purpletext{color:purple!important;font-size:31px!important;font-weight:500!important;}.header_yellowtext{color:yellow!important;font-size:20px!important;font-weight:500!important;}.header_blacktext{color:black!important;font-size:22px!important;font-weight:500!important;}.header_whitetext{color:white!important;font-size:22px!important;font-weight:500!important;}.header_darkred{color:#803d2f!important;}.Green_Header{color:green!important;font-size:24px!important;font-weight:500!important;}.Blue_Header{color:blue!important;font-size:18px!important;font-weight:500!important;}.Red_Header{color:red!important;font-size:28px!important;font-weight:500!important;}.Purple_Header{color:purple!important;font-size:31px!important;font-weight:500!important;}.Yellow_Header{color:yellow!important;font-size:20px!important;font-weight:500!important;}.Black_Header{color:black!important;font-size:22px!important;font-weight:500!important;}.White_Header{color:white!important;font-size:22px!important;font-weight:500!important;} Inflammatory Bowel Disease
- Inflammatory bowel disease is a term for a group of conditions that cause a portion of the digestive system to become inflamed; the most common types are ulcerative colitis and Crohn’s disease. Symptoms may include abdominal pain, diarrhea (which may be bloody), loss of appetite, weight loss, and fever. The symptoms can range from mild to severe, and they may come and go. Treatment includes medicines and in some cases, surgery.
- A 2014 review of 21 studies in patients with ulcerative colitis (1,700 participants) indicated that adding probiotics, prebiotics, or synbiotics to conventional treatment could be helpful in inducing or maintaining remission of the disease. The same review also looked at 14 studies (746 participants) of probiotics, prebiotics, or synbiotics for Crohn’s disease and did not find evidence that they were beneficial.
.header_greentext{color:green!important;font-size:24px!important;font-weight:500!important;}.header_bluetext{color:blue!important;font-size:18px!important;font-weight:500!important;}.header_redtext{color:red!important;font-size:28px!important;font-weight:500!important;}.header_darkred{color:#803d2f!important;font-size:28px!important;font-weight:500!important;}.header_purpletext{color:purple!important;font-size:31px!important;font-weight:500!important;}.header_yellowtext{color:yellow!important;font-size:20px!important;font-weight:500!important;}.header_blacktext{color:black!important;font-size:22px!important;font-weight:500!important;}.header_whitetext{color:white!important;font-size:22px!important;font-weight:500!important;}.header_darkred{color:#803d2f!important;}.Green_Header{color:green!important;font-size:24px!important;font-weight:500!important;}.Blue_Header{color:blue!important;font-size:18px!important;font-weight:500!important;}.Red_Header{color:red!important;font-size:28px!important;font-weight:500!important;}.Purple_Header{color:purple!important;font-size:31px!important;font-weight:500!important;}.Yellow_Header{color:yellow!important;font-size:20px!important;font-weight:500!important;}.Black_Header{color:black!important;font-size:22px!important;font-weight:500!important;}.White_Header{color:white!important;font-size:22px!important;font-weight:500!important;} Irritable Bowel Syndrome
- A 2018 review of 53 studies (5,545 total participants) of probiotics for irritable bowel syndrome (IBS) concluded that probiotics may have beneficial effects on global IBS symptoms and abdominal pain, but it was not possible to draw definite conclusions about their effectiveness or to identify which species, strains, or combinations of probiotics are most likely to be helpful.
For more information, see the NCCIH fact sheet on irritable bowel syndrome .
.header_greentext{color:green!important;font-size:24px!important;font-weight:500!important;}.header_bluetext{color:blue!important;font-size:18px!important;font-weight:500!important;}.header_redtext{color:red!important;font-size:28px!important;font-weight:500!important;}.header_darkred{color:#803d2f!important;font-size:28px!important;font-weight:500!important;}.header_purpletext{color:purple!important;font-size:31px!important;font-weight:500!important;}.header_yellowtext{color:yellow!important;font-size:20px!important;font-weight:500!important;}.header_blacktext{color:black!important;font-size:22px!important;font-weight:500!important;}.header_whitetext{color:white!important;font-size:22px!important;font-weight:500!important;}.header_darkred{color:#803d2f!important;}.Green_Header{color:green!important;font-size:24px!important;font-weight:500!important;}.Blue_Header{color:blue!important;font-size:18px!important;font-weight:500!important;}.Red_Header{color:red!important;font-size:28px!important;font-weight:500!important;}.Purple_Header{color:purple!important;font-size:31px!important;font-weight:500!important;}.Yellow_Header{color:yellow!important;font-size:20px!important;font-weight:500!important;}.Black_Header{color:black!important;font-size:22px!important;font-weight:500!important;}.White_Header{color:white!important;font-size:22px!important;font-weight:500!important;} Traveler’s Diarrhea
- A 2018 review evaluated 11 studies (5,143 participants) of probiotics or prebiotics for prevention of traveler’s diarrhea and found evidence that they may be helpful. However, the review didn’t assess the quality of the studies and didn’t include data on side effects.
- A 2017 clinical practice guideline by the International Society of Travel Medicine stated that there’s insufficient evidence to recommend probiotics or prebiotics to prevent or treat traveler’s diarrhea. The guidelines acknowledged that there’s evidence suggesting a small benefit but pointed out that studies vary greatly in terms of factors such as the probiotic strains used, the causes of the diarrhea, and geographic locations. Also, some studies had weaknesses in their design.
.header_greentext{color:green!important;font-size:24px!important;font-weight:500!important;}.header_bluetext{color:blue!important;font-size:18px!important;font-weight:500!important;}.header_redtext{color:red!important;font-size:28px!important;font-weight:500!important;}.header_darkred{color:#803d2f!important;font-size:28px!important;font-weight:500!important;}.header_purpletext{color:purple!important;font-size:31px!important;font-weight:500!important;}.header_yellowtext{color:yellow!important;font-size:20px!important;font-weight:500!important;}.header_blacktext{color:black!important;font-size:22px!important;font-weight:500!important;}.header_whitetext{color:white!important;font-size:22px!important;font-weight:500!important;}.header_darkred{color:#803d2f!important;}.Green_Header{color:green!important;font-size:24px!important;font-weight:500!important;}.Blue_Header{color:blue!important;font-size:18px!important;font-weight:500!important;}.Red_Header{color:red!important;font-size:28px!important;font-weight:500!important;}.Purple_Header{color:purple!important;font-size:31px!important;font-weight:500!important;}.Yellow_Header{color:yellow!important;font-size:20px!important;font-weight:500!important;}.Black_Header{color:black!important;font-size:22px!important;font-weight:500!important;}.White_Header{color:white!important;font-size:22px!important;font-weight:500!important;} Conditions in Infants
.header_greentext{color:greenimportant;font-size:24pximportant;font-weight:500important;}.header_bluetext{color:blueimportant;font-size:18pximportant;font-weight:500important;}.header_redtext{color:redimportant;font-size:28pximportant;font-weight:500important;}.header_darkred{color:#803d2fimportant;font-size:28pximportant;font-weight:500important;}.header_purpletext{color:purpleimportant;font-size:31pximportant;font-weight:500important;}.header_yellowtext{color:yellowimportant;font-size:20pximportant;font-weight:500important;}.header_blacktext{color:blackimportant;font-size:22pximportant;font-weight:500important;}.header_whitetext{color:whiteimportant;font-size:22pximportant;font-weight:500important;}.header_darkred{color:#803d2fimportant;}.green_header{color:greenimportant;font-size:24pximportant;font-weight:500important;}.blue_header{color:blueimportant;font-size:18pximportant;font-weight:500important;}.red_header{color:redimportant;font-size:28pximportant;font-weight:500important;}.purple_header{color:purpleimportant;font-size:31pximportant;font-weight:500important;}.yellow_header{color:yellowimportant;font-size:20pximportant;font-weight:500important;}.black_header{color:blackimportant;font-size:22pximportant;font-weight:500important;}.white_header{color:whiteimportant;font-size:22pximportant;font-weight:500important;} infant colic.
- Colic is excessive, unexplained crying in young infants. Babies with colic may cry for 3 hours a day or more, but they eat well and grow normally. The cause of colic is not well understood, but studies have shown differences in the microbial community in the digestive tract between infants who have colic and those who don’t, which suggests that microorganisms may be involved.
- A 2018 review of 7 studies (471 participants) of probiotics for colic, 5 of which involved the probiotic Lactobacillus reuteri DSM 17938, found that this probiotic was associated with successful treatment (defined as a reduction of more than half in daily crying time). However, the effect was mainly seen in exclusively breastfed infants.
- No harmful effects were seen in a review of 4 studies (345 participants) of L. reuteri DSM 17938 for colic or in a small NCCIH-funded study that included repeated physical examinations and blood tests in infants with colic who were given this probiotic, as well as parents’ reports of symptoms.
.header_greentext{color:green!important;font-size:24px!important;font-weight:500!important;}.header_bluetext{color:blue!important;font-size:18px!important;font-weight:500!important;}.header_redtext{color:red!important;font-size:28px!important;font-weight:500!important;}.header_darkred{color:#803d2f!important;font-size:28px!important;font-weight:500!important;}.header_purpletext{color:purple!important;font-size:31px!important;font-weight:500!important;}.header_yellowtext{color:yellow!important;font-size:20px!important;font-weight:500!important;}.header_blacktext{color:black!important;font-size:22px!important;font-weight:500!important;}.header_whitetext{color:white!important;font-size:22px!important;font-weight:500!important;}.header_darkred{color:#803d2f!important;}.Green_Header{color:green!important;font-size:24px!important;font-weight:500!important;}.Blue_Header{color:blue!important;font-size:18px!important;font-weight:500!important;}.Red_Header{color:red!important;font-size:28px!important;font-weight:500!important;}.Purple_Header{color:purple!important;font-size:31px!important;font-weight:500!important;}.Yellow_Header{color:yellow!important;font-size:20px!important;font-weight:500!important;}.Black_Header{color:black!important;font-size:22px!important;font-weight:500!important;}.White_Header{color:white!important;font-size:22px!important;font-weight:500!important;} Necrotizing Enterocolitis
- Necrotizing enterocolitis is a serious, sometimes fatal disease that occurs in premature infants. It involves injury or damage to the intestinal tract, causing death of intestinal tissue. Its exact cause is unknown, but an abnormal reaction to food components and the microorganisms that live in a premature baby’s digestive tract may play a role.
- A 2017 review of 23 studies (7,325 infants) showed that probiotics helped to prevent necrotizing enterocolitis in very-low-birth-weight infants. However, the results of individual studies varied; not all showed a benefit. Probiotics that included both Lactobacillus and Bifidobacterium seemed to produce the best results, but it was not possible to identify the most beneficial strains within these large groups of bacteria.
- None of the infants in the studies described above developed harmful short-term side effects from the probiotics. However, the long-term effects of receiving probiotics at such a young age are uncertain. Outside of these studies, there have been instances when probiotics did have harmful effects in newborns. In 2023, the FDA warned health care providers that premature infants who are given probiotics are at risk of severe, potentially fatal infections caused by the microorganisms in the products.
.header_greentext{color:green!important;font-size:24px!important;font-weight:500!important;}.header_bluetext{color:blue!important;font-size:18px!important;font-weight:500!important;}.header_redtext{color:red!important;font-size:28px!important;font-weight:500!important;}.header_darkred{color:#803d2f!important;font-size:28px!important;font-weight:500!important;}.header_purpletext{color:purple!important;font-size:31px!important;font-weight:500!important;}.header_yellowtext{color:yellow!important;font-size:20px!important;font-weight:500!important;}.header_blacktext{color:black!important;font-size:22px!important;font-weight:500!important;}.header_whitetext{color:white!important;font-size:22px!important;font-weight:500!important;}.header_darkred{color:#803d2f!important;}.Green_Header{color:green!important;font-size:24px!important;font-weight:500!important;}.Blue_Header{color:blue!important;font-size:18px!important;font-weight:500!important;}.Red_Header{color:red!important;font-size:28px!important;font-weight:500!important;}.Purple_Header{color:purple!important;font-size:31px!important;font-weight:500!important;}.Yellow_Header{color:yellow!important;font-size:20px!important;font-weight:500!important;}.Black_Header{color:black!important;font-size:22px!important;font-weight:500!important;}.White_Header{color:white!important;font-size:22px!important;font-weight:500!important;} Sepsis in Infants
- Sepsis is a serious illness in which the body has a harmful, overwhelming response to an infection. It can cause major organs and body systems to stop working properly and can be life threatening. The risk of sepsis is highest in infants, children, the elderly, and people with serious medical problems. One group particularly at risk for sepsis is premature infants.
- A review of 37 studies (9,416 participants) found that probiotics were helpful in reducing the risk of sepsis in premature infants.
.header_greentext{color:green!important;font-size:24px!important;font-weight:500!important;}.header_bluetext{color:blue!important;font-size:18px!important;font-weight:500!important;}.header_redtext{color:red!important;font-size:28px!important;font-weight:500!important;}.header_darkred{color:#803d2f!important;font-size:28px!important;font-weight:500!important;}.header_purpletext{color:purple!important;font-size:31px!important;font-weight:500!important;}.header_yellowtext{color:yellow!important;font-size:20px!important;font-weight:500!important;}.header_blacktext{color:black!important;font-size:22px!important;font-weight:500!important;}.header_whitetext{color:white!important;font-size:22px!important;font-weight:500!important;}.header_darkred{color:#803d2f!important;}.Green_Header{color:green!important;font-size:24px!important;font-weight:500!important;}.Blue_Header{color:blue!important;font-size:18px!important;font-weight:500!important;}.Red_Header{color:red!important;font-size:28px!important;font-weight:500!important;}.Purple_Header{color:purple!important;font-size:31px!important;font-weight:500!important;}.Yellow_Header{color:yellow!important;font-size:20px!important;font-weight:500!important;}.Black_Header{color:black!important;font-size:22px!important;font-weight:500!important;}.White_Header{color:white!important;font-size:22px!important;font-weight:500!important;} Dental Disorders
.header_greentext{color:greenimportant;font-size:24pximportant;font-weight:500important;}.header_bluetext{color:blueimportant;font-size:18pximportant;font-weight:500important;}.header_redtext{color:redimportant;font-size:28pximportant;font-weight:500important;}.header_darkred{color:#803d2fimportant;font-size:28pximportant;font-weight:500important;}.header_purpletext{color:purpleimportant;font-size:31pximportant;font-weight:500important;}.header_yellowtext{color:yellowimportant;font-size:20pximportant;font-weight:500important;}.header_blacktext{color:blackimportant;font-size:22pximportant;font-weight:500important;}.header_whitetext{color:whiteimportant;font-size:22pximportant;font-weight:500important;}.header_darkred{color:#803d2fimportant;}.green_header{color:greenimportant;font-size:24pximportant;font-weight:500important;}.blue_header{color:blueimportant;font-size:18pximportant;font-weight:500important;}.red_header{color:redimportant;font-size:28pximportant;font-weight:500important;}.purple_header{color:purpleimportant;font-size:31pximportant;font-weight:500important;}.yellow_header{color:yellowimportant;font-size:20pximportant;font-weight:500important;}.black_header{color:blackimportant;font-size:22pximportant;font-weight:500important;}.white_header{color:whiteimportant;font-size:22pximportant;font-weight:500important;} dental caries (tooth decay).
- A small amount of research, all in infants and young children, has examined the possibility that probiotics might be helpful in preventing dental caries (also called cavities or tooth decay). A review of 7 studies (1,715 total participants) found that the use of probiotics was associated with fewer cavities in 4 of the 7 studies, but the quality of the evidence was low, and no definite conclusions about the effectiveness of probiotics could be reached.
.header_greentext{color:green!important;font-size:24px!important;font-weight:500!important;}.header_bluetext{color:blue!important;font-size:18px!important;font-weight:500!important;}.header_redtext{color:red!important;font-size:28px!important;font-weight:500!important;}.header_darkred{color:#803d2f!important;font-size:28px!important;font-weight:500!important;}.header_purpletext{color:purple!important;font-size:31px!important;font-weight:500!important;}.header_yellowtext{color:yellow!important;font-size:20px!important;font-weight:500!important;}.header_blacktext{color:black!important;font-size:22px!important;font-weight:500!important;}.header_whitetext{color:white!important;font-size:22px!important;font-weight:500!important;}.header_darkred{color:#803d2f!important;}.Green_Header{color:green!important;font-size:24px!important;font-weight:500!important;}.Blue_Header{color:blue!important;font-size:18px!important;font-weight:500!important;}.Red_Header{color:red!important;font-size:28px!important;font-weight:500!important;}.Purple_Header{color:purple!important;font-size:31px!important;font-weight:500!important;}.Yellow_Header{color:yellow!important;font-size:20px!important;font-weight:500!important;}.Black_Header{color:black!important;font-size:22px!important;font-weight:500!important;}.White_Header{color:white!important;font-size:22px!important;font-weight:500!important;} Periodontal Diseases (Gum Disease)
- Periodontal diseases result from infections and inflammation of the gums and bone that surround and support the teeth. If the disease is severe, the gums can pull away from the teeth, bone can be lost, and teeth may loosen or fall out.
- A 2016 review of 12 studies (452 participants) that evaluated probiotics for periodontal disease found evidence that they could be a helpful addition to treatment by reducing disease-causing bacteria and improving clinical signs of the disease. However, effects may differ for different probiotics.
.header_greentext{color:green!important;font-size:24px!important;font-weight:500!important;}.header_bluetext{color:blue!important;font-size:18px!important;font-weight:500!important;}.header_redtext{color:red!important;font-size:28px!important;font-weight:500!important;}.header_darkred{color:#803d2f!important;font-size:28px!important;font-weight:500!important;}.header_purpletext{color:purple!important;font-size:31px!important;font-weight:500!important;}.header_yellowtext{color:yellow!important;font-size:20px!important;font-weight:500!important;}.header_blacktext{color:black!important;font-size:22px!important;font-weight:500!important;}.header_whitetext{color:white!important;font-size:22px!important;font-weight:500!important;}.header_darkred{color:#803d2f!important;}.Green_Header{color:green!important;font-size:24px!important;font-weight:500!important;}.Blue_Header{color:blue!important;font-size:18px!important;font-weight:500!important;}.Red_Header{color:red!important;font-size:28px!important;font-weight:500!important;}.Purple_Header{color:purple!important;font-size:31px!important;font-weight:500!important;}.Yellow_Header{color:yellow!important;font-size:20px!important;font-weight:500!important;}.Black_Header{color:black!important;font-size:22px!important;font-weight:500!important;}.White_Header{color:white!important;font-size:22px!important;font-weight:500!important;} Conditions Related to Allergy
.header_greentext{color:greenimportant;font-size:24pximportant;font-weight:500important;}.header_bluetext{color:blueimportant;font-size:18pximportant;font-weight:500important;}.header_redtext{color:redimportant;font-size:28pximportant;font-weight:500important;}.header_darkred{color:#803d2fimportant;font-size:28pximportant;font-weight:500important;}.header_purpletext{color:purpleimportant;font-size:31pximportant;font-weight:500important;}.header_yellowtext{color:yellowimportant;font-size:20pximportant;font-weight:500important;}.header_blacktext{color:blackimportant;font-size:22pximportant;font-weight:500important;}.header_whitetext{color:whiteimportant;font-size:22pximportant;font-weight:500important;}.header_darkred{color:#803d2fimportant;}.green_header{color:greenimportant;font-size:24pximportant;font-weight:500important;}.blue_header{color:blueimportant;font-size:18pximportant;font-weight:500important;}.red_header{color:redimportant;font-size:28pximportant;font-weight:500important;}.purple_header{color:purpleimportant;font-size:31pximportant;font-weight:500important;}.yellow_header{color:yellowimportant;font-size:20pximportant;font-weight:500important;}.black_header{color:blackimportant;font-size:22pximportant;font-weight:500important;}.white_header{color:whiteimportant;font-size:22pximportant;font-weight:500important;} allergic rhinitis (hay fever).
- A review of 23 studies (1,919 participants) in which probiotics were tested for treating allergic rhinitis found some evidence that they may be helpful for improving symptoms and quality of life. However, because the studies tested different probiotics and measured different effects, no recommendations about the use of probiotics could be made. Few side effects of probiotics were reported in these studies.
.header_greentext{color:green!important;font-size:24px!important;font-weight:500!important;}.header_bluetext{color:blue!important;font-size:18px!important;font-weight:500!important;}.header_redtext{color:red!important;font-size:28px!important;font-weight:500!important;}.header_darkred{color:#803d2f!important;font-size:28px!important;font-weight:500!important;}.header_purpletext{color:purple!important;font-size:31px!important;font-weight:500!important;}.header_yellowtext{color:yellow!important;font-size:20px!important;font-weight:500!important;}.header_blacktext{color:black!important;font-size:22px!important;font-weight:500!important;}.header_whitetext{color:white!important;font-size:22px!important;font-weight:500!important;}.header_darkred{color:#803d2f!important;}.Green_Header{color:green!important;font-size:24px!important;font-weight:500!important;}.Blue_Header{color:blue!important;font-size:18px!important;font-weight:500!important;}.Red_Header{color:red!important;font-size:28px!important;font-weight:500!important;}.Purple_Header{color:purple!important;font-size:31px!important;font-weight:500!important;}.Yellow_Header{color:yellow!important;font-size:20px!important;font-weight:500!important;}.Black_Header{color:black!important;font-size:22px!important;font-weight:500!important;}.White_Header{color:white!important;font-size:22px!important;font-weight:500!important;} Asthma
- A review of 11 studies (910 participants) of probiotics for asthma in children had inconclusive results.
.header_greentext{color:green!important;font-size:24px!important;font-weight:500!important;}.header_bluetext{color:blue!important;font-size:18px!important;font-weight:500!important;}.header_redtext{color:red!important;font-size:28px!important;font-weight:500!important;}.header_darkred{color:#803d2f!important;font-size:28px!important;font-weight:500!important;}.header_purpletext{color:purple!important;font-size:31px!important;font-weight:500!important;}.header_yellowtext{color:yellow!important;font-size:20px!important;font-weight:500!important;}.header_blacktext{color:black!important;font-size:22px!important;font-weight:500!important;}.header_whitetext{color:white!important;font-size:22px!important;font-weight:500!important;}.header_darkred{color:#803d2f!important;}.Green_Header{color:green!important;font-size:24px!important;font-weight:500!important;}.Blue_Header{color:blue!important;font-size:18px!important;font-weight:500!important;}.Red_Header{color:red!important;font-size:28px!important;font-weight:500!important;}.Purple_Header{color:purple!important;font-size:31px!important;font-weight:500!important;}.Yellow_Header{color:yellow!important;font-size:20px!important;font-weight:500!important;}.Black_Header{color:black!important;font-size:22px!important;font-weight:500!important;}.White_Header{color:white!important;font-size:22px!important;font-weight:500!important;} Atopic Dermatitis
- Atopic dermatitis is an itchy chronic skin disorder that’s associated with allergies but not caused by them. It’s most common in infants and may start as early as age 2 to 6 months. Many people outgrow it by early adulthood. Atopic dermatitis is one of several types of eczema.
- A 2017 review of 13 studies (1,271 participants) of probiotics for the treatment of atopic dermatitis in infants and children did not find consistent evidence of a beneficial effect. A review of 9 studies (269 participants) in adults provided preliminary evidence that some strains of probiotics might be beneficial for symptoms of atopic dermatitis.
.header_greentext{color:green!important;font-size:24px!important;font-weight:500!important;}.header_bluetext{color:blue!important;font-size:18px!important;font-weight:500!important;}.header_redtext{color:red!important;font-size:28px!important;font-weight:500!important;}.header_darkred{color:#803d2f!important;font-size:28px!important;font-weight:500!important;}.header_purpletext{color:purple!important;font-size:31px!important;font-weight:500!important;}.header_yellowtext{color:yellow!important;font-size:20px!important;font-weight:500!important;}.header_blacktext{color:black!important;font-size:22px!important;font-weight:500!important;}.header_whitetext{color:white!important;font-size:22px!important;font-weight:500!important;}.header_darkred{color:#803d2f!important;}.Green_Header{color:green!important;font-size:24px!important;font-weight:500!important;}.Blue_Header{color:blue!important;font-size:18px!important;font-weight:500!important;}.Red_Header{color:red!important;font-size:28px!important;font-weight:500!important;}.Purple_Header{color:purple!important;font-size:31px!important;font-weight:500!important;}.Yellow_Header{color:yellow!important;font-size:20px!important;font-weight:500!important;}.Black_Header{color:black!important;font-size:22px!important;font-weight:500!important;}.White_Header{color:white!important;font-size:22px!important;font-weight:500!important;} Prevention of Allergies
- It’s been suggested that changes in people’s lifestyles and environment may have led to reduced contact with microorganisms early in life, and that this decrease may have contributed to an increase in allergies. This is sometimes called the “hygiene hypothesis,” although factors unrelated to hygiene, such as smaller family size and the use of antibiotics, may also play a role. Studies have been done in which probiotics were given to pregnant women and/or young infants in the hope of preventing the development of allergies.
- A 2015 review of 17 studies (4,755 participants) that evaluated the use of probiotics during pregnancy or early infancy found that infants exposed to probiotics had a lower risk of developing atopic dermatitis, especially if they were exposed to a mixture of probiotics. However, probiotics did not have an effect on the risks of asthma, wheezing, or hay fever (allergic rhinitis).
.header_greentext{color:green!important;font-size:24px!important;font-weight:500!important;}.header_bluetext{color:blue!important;font-size:18px!important;font-weight:500!important;}.header_redtext{color:red!important;font-size:28px!important;font-weight:500!important;}.header_darkred{color:#803d2f!important;font-size:28px!important;font-weight:500!important;}.header_purpletext{color:purple!important;font-size:31px!important;font-weight:500!important;}.header_yellowtext{color:yellow!important;font-size:20px!important;font-weight:500!important;}.header_blacktext{color:black!important;font-size:22px!important;font-weight:500!important;}.header_whitetext{color:white!important;font-size:22px!important;font-weight:500!important;}.header_darkred{color:#803d2f!important;}.Green_Header{color:green!important;font-size:24px!important;font-weight:500!important;}.Blue_Header{color:blue!important;font-size:18px!important;font-weight:500!important;}.Red_Header{color:red!important;font-size:28px!important;font-weight:500!important;}.Purple_Header{color:purple!important;font-size:31px!important;font-weight:500!important;}.Yellow_Header{color:yellow!important;font-size:20px!important;font-weight:500!important;}.Black_Header{color:black!important;font-size:22px!important;font-weight:500!important;}.White_Header{color:white!important;font-size:22px!important;font-weight:500!important;} Other Conditions
.header_greentext{color:greenimportant;font-size:24pximportant;font-weight:500important;}.header_bluetext{color:blueimportant;font-size:18pximportant;font-weight:500important;}.header_redtext{color:redimportant;font-size:28pximportant;font-weight:500important;}.header_darkred{color:#803d2fimportant;font-size:28pximportant;font-weight:500important;}.header_purpletext{color:purpleimportant;font-size:31pximportant;font-weight:500important;}.header_yellowtext{color:yellowimportant;font-size:20pximportant;font-weight:500important;}.header_blacktext{color:blackimportant;font-size:22pximportant;font-weight:500important;}.header_whitetext{color:whiteimportant;font-size:22pximportant;font-weight:500important;}.header_darkred{color:#803d2fimportant;}.green_header{color:greenimportant;font-size:24pximportant;font-weight:500important;}.blue_header{color:blueimportant;font-size:18pximportant;font-weight:500important;}.red_header{color:redimportant;font-size:28pximportant;font-weight:500important;}.purple_header{color:purpleimportant;font-size:31pximportant;font-weight:500important;}.yellow_header{color:yellowimportant;font-size:20pximportant;font-weight:500important;}.black_header{color:blackimportant;font-size:22pximportant;font-weight:500important;}.white_header{color:whiteimportant;font-size:22pximportant;font-weight:500important;} acne.
- Research has identified mechanisms by which probiotics, either taken orally or used topically (applied to the skin), might influence acne. However, there has been very little research in people on probiotics for acne, and the American Academy of Dermatology’s 2016 guidelines for managing acne state that the existing evidence isn’t strong enough to justify any recommendations about the use of probiotics.
.header_greentext{color:green!important;font-size:24px!important;font-weight:500!important;}.header_bluetext{color:blue!important;font-size:18px!important;font-weight:500!important;}.header_redtext{color:red!important;font-size:28px!important;font-weight:500!important;}.header_darkred{color:#803d2f!important;font-size:28px!important;font-weight:500!important;}.header_purpletext{color:purple!important;font-size:31px!important;font-weight:500!important;}.header_yellowtext{color:yellow!important;font-size:20px!important;font-weight:500!important;}.header_blacktext{color:black!important;font-size:22px!important;font-weight:500!important;}.header_whitetext{color:white!important;font-size:22px!important;font-weight:500!important;}.header_darkred{color:#803d2f!important;}.Green_Header{color:green!important;font-size:24px!important;font-weight:500!important;}.Blue_Header{color:blue!important;font-size:18px!important;font-weight:500!important;}.Red_Header{color:red!important;font-size:28px!important;font-weight:500!important;}.Purple_Header{color:purple!important;font-size:31px!important;font-weight:500!important;}.Yellow_Header{color:yellow!important;font-size:20px!important;font-weight:500!important;}.Black_Header{color:black!important;font-size:22px!important;font-weight:500!important;}.White_Header{color:white!important;font-size:22px!important;font-weight:500!important;} Hepatic Encephalopathy
- When the liver is damaged and unable to remove toxic substances from the blood, the toxins can build up in the bloodstream and affect the nervous system. This may lead to impairments of brain function called hepatic encephalopathy.
- A 2017 review looked at 21 studies (1,420 participants) of probiotics for hepatic encephalopathy and concluded that they were generally of low quality. There was evidence that compared with a placebo (an inactive substance) or no treatment, probiotics probably had beneficial effects on hepatic encephalopathy, but it was uncertain whether probiotics were better than lactulose, a conventional treatment for liver disease.
.header_greentext{color:green!important;font-size:24px!important;font-weight:500!important;}.header_bluetext{color:blue!important;font-size:18px!important;font-weight:500!important;}.header_redtext{color:red!important;font-size:28px!important;font-weight:500!important;}.header_darkred{color:#803d2f!important;font-size:28px!important;font-weight:500!important;}.header_purpletext{color:purple!important;font-size:31px!important;font-weight:500!important;}.header_yellowtext{color:yellow!important;font-size:20px!important;font-weight:500!important;}.header_blacktext{color:black!important;font-size:22px!important;font-weight:500!important;}.header_whitetext{color:white!important;font-size:22px!important;font-weight:500!important;}.header_darkred{color:#803d2f!important;}.Green_Header{color:green!important;font-size:24px!important;font-weight:500!important;}.Blue_Header{color:blue!important;font-size:18px!important;font-weight:500!important;}.Red_Header{color:red!important;font-size:28px!important;font-weight:500!important;}.Purple_Header{color:purple!important;font-size:31px!important;font-weight:500!important;}.Yellow_Header{color:yellow!important;font-size:20px!important;font-weight:500!important;}.Black_Header{color:black!important;font-size:22px!important;font-weight:500!important;}.White_Header{color:white!important;font-size:22px!important;font-weight:500!important;} Upper Respiratory Infections
- Probiotics have been tested for their effects against upper respiratory infections (a group that includes the common cold, middle ear infections, sinusitis, and various throat infections). A 2015 evaluation of 12 studies with 3,720 total participants indicated that people taking probiotics may have fewer and shorter upper respiratory infections. However, the quality of the evidence was low because some of the studies were poorly conducted.
.header_greentext{color:green!important;font-size:24px!important;font-weight:500!important;}.header_bluetext{color:blue!important;font-size:18px!important;font-weight:500!important;}.header_redtext{color:red!important;font-size:28px!important;font-weight:500!important;}.header_darkred{color:#803d2f!important;font-size:28px!important;font-weight:500!important;}.header_purpletext{color:purple!important;font-size:31px!important;font-weight:500!important;}.header_yellowtext{color:yellow!important;font-size:20px!important;font-weight:500!important;}.header_blacktext{color:black!important;font-size:22px!important;font-weight:500!important;}.header_whitetext{color:white!important;font-size:22px!important;font-weight:500!important;}.header_darkred{color:#803d2f!important;}.Green_Header{color:green!important;font-size:24px!important;font-weight:500!important;}.Blue_Header{color:blue!important;font-size:18px!important;font-weight:500!important;}.Red_Header{color:red!important;font-size:28px!important;font-weight:500!important;}.Purple_Header{color:purple!important;font-size:31px!important;font-weight:500!important;}.Yellow_Header{color:yellow!important;font-size:20px!important;font-weight:500!important;}.Black_Header{color:black!important;font-size:22px!important;font-weight:500!important;}.White_Header{color:white!important;font-size:22px!important;font-weight:500!important;} Urinary Tract Infections
- A 2015 review of 9 studies (735 participants) of probiotics for the prevention of urinary tract infection did not find evidence of a beneficial effect.
.header_greentext{color:green!important;font-size:24px!important;font-weight:500!important;}.header_bluetext{color:blue!important;font-size:18px!important;font-weight:500!important;}.header_redtext{color:red!important;font-size:28px!important;font-weight:500!important;}.header_darkred{color:#803d2f!important;font-size:28px!important;font-weight:500!important;}.header_purpletext{color:purple!important;font-size:31px!important;font-weight:500!important;}.header_yellowtext{color:yellow!important;font-size:20px!important;font-weight:500!important;}.header_blacktext{color:black!important;font-size:22px!important;font-weight:500!important;}.header_whitetext{color:white!important;font-size:22px!important;font-weight:500!important;}.header_darkred{color:#803d2f!important;}.Green_Header{color:green!important;font-size:24px!important;font-weight:500!important;}.Blue_Header{color:blue!important;font-size:18px!important;font-weight:500!important;}.Red_Header{color:red!important;font-size:28px!important;font-weight:500!important;}.Purple_Header{color:purple!important;font-size:31px!important;font-weight:500!important;}.Yellow_Header{color:yellow!important;font-size:20px!important;font-weight:500!important;}.Black_Header{color:black!important;font-size:22px!important;font-weight:500!important;}.White_Header{color:white!important;font-size:22px!important;font-weight:500!important;} Can probiotics be harmful?
- Probiotics have an extensive history of apparently safe use, particularly in healthy people. However, few studies have looked at the safety of probiotics in detail, so there’s a lack of solid information on the frequency and severity of side effects.
- The risk of harmful effects from probiotics is greater in people with severe illnesses or compromised immune systems. When probiotics are being considered for high-risk individuals, such as premature infants or seriously ill hospital patients, the potential risks of probiotics should be carefully weighed against their benefits. Cases of severe or fatal infections have been reported in premature infants who were given probiotics, and the U.S. Food and Drug Administration (FDA) has warned health care providers about this risk.
- Possible harmful effects of probiotics include infections, production of harmful substances by the probiotic microorganisms, and transfer of antibiotic resistance genes from probiotic microorganisms to other microorganisms in the digestive tract.
- Some probiotic products have been reported to contain microorganisms other than those listed on the label. In some instances, these contaminants may pose serious health risks.
NCCIH-Funded Research
NCCIH sponsors a variety of research projects related to probiotics or the microbiome. In addition to the previously mentioned studies on diet-microbiome interactions in the digestive tract, recent topics include:
- The mechanisms by which probiotics may help to reduce postmenopausal bone loss
- Engineering probiotics to synthesize natural substances for microbiome-brain research
- The mechanisms by which certain probiotics may relieve chronic pelvic pain
- The effects of a specific Bifidobacterium strain on changes in short-chain fatty acid production in the gut that may play a role in antibiotic-associated diarrhea.
More To Consider
- Don’t use probiotics as a reason to postpone seeing your health care provider about any health problem.
- If you’re considering a probiotic dietary supplement, consult your health care provider first. This is especially important if you have health problems. Anyone with a serious underlying health condition should be monitored closely while taking probiotics.
- Take charge of your health—talk with your health care providers about any complementary health approaches you use. Together, you can make shared, well-informed decisions.
For More Information
Nccih clearinghouse.
The NCCIH Clearinghouse provides information on NCCIH and complementary and integrative health approaches, including publications and searches of Federal databases of scientific and medical literature. The Clearinghouse does not provide medical advice, treatment recommendations, or referrals to practitioners.
Toll-free in the U.S.: 1-888-644-6226
Telecommunications relay service (TRS): 7-1-1
Website: https://www.nccih.nih.gov
Email: [email protected] (link sends email)
Know the Science
NCCIH and the National Institutes of Health (NIH) provide tools to help you understand the basics and terminology of scientific research so you can make well-informed decisions about your health. Know the Science features a variety of materials, including interactive modules, quizzes, and videos, as well as links to informative content from Federal resources designed to help consumers make sense of health information.
Explaining How Research Works (NIH)
Know the Science: How To Make Sense of a Scientific Journal Article
Understanding Clinical Studies (NIH)
A service of the National Library of Medicine, PubMed® contains publication information and (in most cases) brief summaries of articles from scientific and medical journals. For guidance from NCCIH on using PubMed, see How To Find Information About Complementary Health Approaches on PubMed .
Website: https://pubmed.ncbi.nlm.nih.gov/
MedlinePlus
To provide resources that help answer health questions, MedlinePlus (a service of the National Library of Medicine) brings together authoritative information from the National Institutes of Health as well as other Government agencies and health-related organizations.
Website: https://www.medlineplus.gov
.header_greentext{color:green!important;font-size:24px!important;font-weight:500!important;}.header_bluetext{color:blue!important;font-size:18px!important;font-weight:500!important;}.header_redtext{color:red!important;font-size:28px!important;font-weight:500!important;}.header_darkred{color:#803d2f!important;font-size:28px!important;font-weight:500!important;}.header_purpletext{color:purple!important;font-size:31px!important;font-weight:500!important;}.header_yellowtext{color:yellow!important;font-size:20px!important;font-weight:500!important;}.header_blacktext{color:black!important;font-size:22px!important;font-weight:500!important;}.header_whitetext{color:white!important;font-size:22px!important;font-weight:500!important;}.header_darkred{color:#803d2f!important;}.Green_Header{color:green!important;font-size:24px!important;font-weight:500!important;}.Blue_Header{color:blue!important;font-size:18px!important;font-weight:500!important;}.Red_Header{color:red!important;font-size:28px!important;font-weight:500!important;}.Purple_Header{color:purple!important;font-size:31px!important;font-weight:500!important;}.Yellow_Header{color:yellow!important;font-size:20px!important;font-weight:500!important;}.Black_Header{color:black!important;font-size:22px!important;font-weight:500!important;}.White_Header{color:white!important;font-size:22px!important;font-weight:500!important;} Key References
- Bafeta A, Koh M, Riveros C, et al. Harms reporting in randomized controlled trials of interventions aimed at modifying microbiota: a systematic review. Annals of Internal Medicine . 2018;169(4):240-247.
- Blaabjerg S, Artzi DM, Aabenhus R. Probiotics for the prevention of antibiotic-associated diarrhea in outpatients—a systematic review and meta-analysis. Antibiotics . 2017;6(4).pii:E21.
- Butel M-J. Probiotics, gut microbiota and health. Médecine et Maladies Infectieuses . 2014;44(1):1-8.
- Cohen PA. Probiotic safety—no guarantees. JAMA Internal Medicine . 2018;178(12):1577-1578.
- Degnan FH. The US Food and Drug Administration and probiotics: regulatory categorization. Clinical Infectious Diseases. 2008;46(Suppl 2):S133–S136.
- Didari T, Solki S, Mozaffari S, et al. A systematic review of the safety of probiotics. Expert Opinion on Drug Safety . 2014;13(2):227–239.
- Dryl R, Szajewska H. Probiotics for management of infantile colic: a systematic review of randomized controlled trials. Archives of Medical Science. 2018;14(5):1137-1143.
- Fijan S. Microorganisms with claimed probiotic properties: an overview of recent literature. International Journal of Environmental Research and Public Health. 2014;11(5):4745-4767.
- Ford AC, Harris LA, Lacy BE, et al. Systematic review with meta-analysis: the efficacy of prebiotics, probiotics, synbiotics and antibiotics in irritable bowel syndrome. Alimentary Pharmacology & Therapeutics . 2018;48(10):1044-1060.
- Goldenberg JZ, Yap C, Lytvyn L, et al. Probiotics for the prevention of Clostridium difficile -associated diarrhea in adults and children. Cochrane Database of Systematic Reviews. 2017;(12):CD006095. Accessed at www.cochranelibrary.com on January 23, 2018.
- Guarner F, Khan AG, Garisch J, et al. World Gastroenterology Organisation Global Guidelines. Probiotics and Prebiotics. October 2011. Journal of Clinical Gastroenterology . 2012;46(6):468–481.
- Hempel S, Newberry SJ, Maher AR, et al. Probiotics for the prevention and treatment of antibiotic-associated diarrhea: a systematic review and meta-analysis. JAMA . 2012;307(18):1959–1969.
- Hempel S, Newberry S, Ruelaz A, et al. Safety of Probiotics to Reduce Risk and Prevent or Treat Disease. Evidence Report/Technology Assessment no. 200. Rockville, MD: Agency for Healthcare Research and Quality; 2011. AHRQ publication no. 11-E007.
- Rao SC, Athalye-Jape GK, Deshpande GC, et al. Probiotic supplementation and late-onset sepsis in preterm infants: a meta-analysis. Pediatrics. 2016;137(3):e20153684.
- Sanders ME, Akkermans LM, Haller D, et al. Safety assessment of probiotics for human use. Gut Microbes . 2010;1(3):164-185.
- Thomas JP, Raine T, Reddy S, et al. Probiotics for the prevention of necrotizing enterocolitis in very low-birth-weight infants: a meta-analysis and systematic review. Acta Paediatrica . 2017;106(11):1729-1741.
- U.S. Food and Drug Administration. Warning Regarding Use of Probiotics in Preterm Infants. Issued September 29, 2023. Accessed at https://www.fda.gov/media/172606 on October 2, 2023.
- Zuccotti G, Meneghin F, Aceti A, et al. Probiotics for prevention of atopic diseases in infants: systematic review and meta-analysis. Allergy. 2015;70(11):1356-13
.header_greentext{color:green!important;font-size:24px!important;font-weight:500!important;}.header_bluetext{color:blue!important;font-size:18px!important;font-weight:500!important;}.header_redtext{color:red!important;font-size:28px!important;font-weight:500!important;}.header_darkred{color:#803d2f!important;font-size:28px!important;font-weight:500!important;}.header_purpletext{color:purple!important;font-size:31px!important;font-weight:500!important;}.header_yellowtext{color:yellow!important;font-size:20px!important;font-weight:500!important;}.header_blacktext{color:black!important;font-size:22px!important;font-weight:500!important;}.header_whitetext{color:white!important;font-size:22px!important;font-weight:500!important;}.header_darkred{color:#803d2f!important;}.Green_Header{color:green!important;font-size:24px!important;font-weight:500!important;}.Blue_Header{color:blue!important;font-size:18px!important;font-weight:500!important;}.Red_Header{color:red!important;font-size:28px!important;font-weight:500!important;}.Purple_Header{color:purple!important;font-size:31px!important;font-weight:500!important;}.Yellow_Header{color:yellow!important;font-size:20px!important;font-weight:500!important;}.Black_Header{color:black!important;font-size:22px!important;font-weight:500!important;}.White_Header{color:white!important;font-size:22px!important;font-weight:500!important;} Additional References
- Bae J-M. Prophylactic efficacy of probiotics on travelers’ diarrhea: an adaptive meta-analysis of randomized controlled trials. Epidemiology and Health . 2018;40:e2018043.
- Black LI, Clarke TC, Barnes PM, Stussman BJ, Nahin RL. Use of complementary health approaches among children aged 4-17 years in the United States: National Health Interview Survey, 2007-2012. National health statistics reports; no 78. Hyattsville, MD: National Center for Health Statistics. 2015.
- Cao L, Wang L, Yang L, et al. Long-term effect of early-life supplementation with probiotics on preventing atopic dermatitis: a meta-analysis. Journal of Dermatological Treatment . 2015;26(6):537-540.
- Clarke TC, Black LI, Stussman BJ, Barnes PM, Nahin RL. Trends in the use of complementary health approaches among adults: United States, 2002–2012. National health statistics reports; no 79. Hyattsville, MD: National Center for Health Statistics. 2015.
- Dalal R, McGee RG, Riordan SM, et al. Probiotics for people with hepatic encephalopathy. Cochrane Database of Systematic Reviews . 2017;(2):CD008716. Accessed at www.cochranelibrary.com on November 15, 2018.
- Dimidi E, Christodoulides S, Fragkos KC, et al. The effect of probiotics on functional constipation in adults: a systematic review and meta-analysis of randomized controlled trials. American Journal of Clinical Nutrition . 2014;100(4):1075-1084.
- Doron S, Snydman DR. Risk and safety of probiotics. Clinical Infectious Diseases . 2015;60(Suppl 2):S129-S134.
- Fatheree NY, Liu Y, Taylor CM, et al. Lactobacillus reuteri for infants with colic: a double-blind, placebo-controlled, randomized clinical trial. Journal of Pediatrics . 2017;191:170-178.
- Ghouri YA, Richards DM, Rahimi EF, et al. Systematic review of randomized controlled trials of probiotics, prebiotics, and synbiotics in inflammatory bowel disease. Clinical and Experimental Gastroenterology . 2014;7:473-487.
- Gibson GR, Hutkins R, Sanders ME, et al. Expert consensus document: The International Scientific Association for Probiotics and Prebiotics (ISAPP) consensus statement on the definition and scope of prebiotics. Nature Reviews. Gastroenterology & Hepatology . 2017;14(8):491-502.
- Goldenberg JZ, Lytvyn L, Steurich J, et al. Probiotics for the prevention of pediatric antibiotic-associated diarrhea. Cochrane Database of Systematic Reviews . 2015;(12):CD004827. Accessed at www.cochranelibrary.com on November 2, 2018.
- Hao Q, Dong BR, Wu T. Probiotics for preventing acute upper respiratory tract infections. Cochrane Database of Systematic Reviews . 2015;(2):CD006895. Accessed at www.cochranelibrary.com on March 6, 2018.
- Hoffmann DE, Fraser CM, Palumbo FB, et al. Probiotics: finding the right regulatory balance. Science . 2013;342(6156):314-315.
- Huang R, Hu J. Positive effect of probiotics on constipation in children: a systematic review and meta-analysis of six randomized controlled trials. Frontiers in Cellular and Infection Microbiology. 2017;7:153.
- Huang R, Ning H, Shen M, et al. Probiotics for the treatment of atopic dermatitis in children: a systematic review and meta-analysis of randomized controlled trials. Frontiers in Cellular and Infection Microbiology. 2017;7:392.
- Jafarnejad S, Shab-Bidar S, Speakman JR, et al. Probiotics reduce the risk of antibiotic-associated diarrhea in adults (18-64 years) but not the elderly (>65 years): a meta-analysis. Nutrition in Clinical Practice . 2016;31(4):502-513.
- Jørgensen MR, Castiblanco G, Twetman S, et al. Prevention of caries with probiotic bacteria during early childhood. Promising but inconsistent findings. American Journal of Dentistry . 2016;29(3):127-131.
- Kechagia M, Basoulis D, Konstantopoulou S, et al. Health benefits of probiotics: a review. ISRN Nutrition . 2013;2013:48165.
- Kelesidis T, Pothoulakis C. Efficacy and safety of the probiotic Saccharomyces boulardii for the prevention and therapy of gastrointestinal disorders. Therapeutic Advances in Gastroenterology . 2012;5(2):111-125.
- Kober M-M, Bowe WP. The effect of probiotics on immune regulation, acne, and photoaging. International Journal of Women’s Dermatology . 2015;1(2):85-89.
- Lahner E, Bellisario C, Hassan C, et al. Probiotics in the treatment of diverticular disease. A systematic review. Journal of Gastrointestinal and Liver Diseases . 2016;25(1):79-86.
- Lin J, Zhang Y, He C, et al. Probiotics supplementation in children with asthma: a systematic review and meta-analysis. Journal of Paediatrics and Child Health. 2018;54(9):953-961.
- Marcason W. Probiotics: where do we stand? Journal of the Academy of Nutrition and Dietetics. 2013;113(10):1424.
- Martínez-Martínez MI, Calabuig-Tolsá R, Cauli O. The effect of probiotics as a treatment for constipation in elderly people: a systematic review. Archives of Gerontology and Geriatrics. 2017;71:142-149.
- Matsubara VH, Bandara HM, Ishikawa KH, et al. The role of probiotic bacteria in managing periodontal disease: a systematic review. Expert Review of Anti-infective Therapy . 2016;14(7):643-655.
- Notay M, Foolad N, Vaughn AR, et al. Probiotics, prebiotics, and synbiotics for the treatment and prevention of adult dermatological diseases. American Journal of Clinical Dermatology . 2017;18(6):721-732.
- Oelschlaeger TA. Mechanisms of probiotic actions – A review. International Journal of Medical Microbiology . 2010;300(1):57-62.
- Osborn DA, Sinn JKH. Prebiotics in infants for prevention of allergy. Cochrane Database of Systematic Reviews . 2013(3):CD006474. Accessed at www.cochranelibrary.com on March 6, 2018.
- Petschow B, Doré J, Hibberd P, et al. Probiotics, prebiotics, and the host microbiome: the science of translation. Annals of the New York Academy of Sciences . 2013;1306:1–17.
- Reid G. Probiotics: definition, scope and mechanisms of action. Best Practice & Research: Clinical Gastroenterology. 2016;30(1):17-25.
- Riddle MS, Connor BA, Beeching NJ, et al. Guidelines for the prevention and treatment of travelers’ diarrhea: a graded expert panel report. Journal of Travel Medicine . 2017;24(Suppl 1):S63-S80.
- Sanders ME, Guarner F, Guerrant R, et al. An update on the use and investigation of probiotics in health and disease. Gut. 2013;62(5):787-796.
- Schwenger EM, Tejani AM, Loewen PS. Probiotics for preventing urinary tract infections in adults and children. Cochrane Database of Systematic Reviews . 2015;(12):CD008772. Accessed at www.cochranelibrary.com on November 9, 2018.
- Shanahan F. A commentary on the safety of probiotics. Gastroenterology Clinics of North America . 2012;41(4):869–876.
- Steele SR, McCormick J, Melton GB, et al. Practice parameters for the management of Clostridium difficile infection. Diseases of the Colon and Rectum . 2015;58(1):10-24.
- Sung V, D’Amico F, Cabana MD, et al. Lactobacillus reuteri to treat infant colic: a meta-analysis. Pediatrics . 2018;141(1):e20171811.
- Vallabhaneni S, Walker TA, Lockhart SR, et al. Fatal gastrointestinal mucormycosis in a premature infant associated with a contaminated dietary supplement—Connecticut, 2014. Morbidity and Mortality Weekly Report. 2015;64(6):155-156.
- Venugopalan V, Shriner KA, Wong-Beringer A. Regulatory oversight and safety of probiotic use. Emerging Infectious Diseases. 2010;16(11):1661-1665.
- Wei D, Heus P, van de Wetering FT, et al. Probiotics for the prevention or treatment of chemotherapy- or radiotherapy-related diarrhoea in people with cancer. Cochrane Database of Systematic Reviews. 2018;(8):CD008831. Accessed at www.cochranelibrary.com on November 9, 2018.
- Wojtyniak K, Szajewska H. Systematic review: probiotics for functional constipation in children. European Journal of Pediatrics . 2017;176(9):1155-1162.
- Zaenglein AL, Pathy AL, Schlosser BJ, et al. Guidelines of care for the management of acne vulgaris. Journal of the American Academy of Dermatology . 2016;74(5):945-973.
- Zajac AE, Adams AS, Turner JH. A systematic review and meta-analysis of probiotics for the treatment of allergic rhinitis. International Forum of Allergy and Rhinology . 2015;5(6):524-532.
Acknowledgments
NCCIH thanks Yisong Wang, Ph.D., and David Shurtleff, Ph.D., for their review of the 2019 update of this publication.
This publication is not copyrighted and is in the public domain. Duplication is encouraged.
NCCIH has provided this material for your information. It is not intended to substitute for the medical expertise and advice of your health care provider(s). We encourage you to discuss any decisions about treatment or care with your health care provider. The mention of any product, service, or therapy is not an endorsement by NCCIH.
For Consumers
5 Things To Know About Probiotics
For Health Care Providers
Risk of Invasive Disease in Preterm Infants Given Probiotics Formulated To Contain Live Bacteria or Yeast
Irritable Bowel Syndrome and Complementary Health Approaches
Probiotics - Systematic Reviews/Reviews/Meta-analyses (PubMed®)
Probiotics - Randomized Controlled Trials (PubMed®)
Research Results
A Probiotic/Prebiotic Combination Reduces Behavioral Symptoms Associated With Stress
Related Fact Sheets
Irritable Bowel Syndrome: What You Need To Know
Spinal Cord Injury
According to the National Spinal Cord Injury Association, as many as 450,000 people in the United States are living with a spinal cord injury (SCI). Other organizations conservatively estimate this figure to be about 250,000.
Every year, an estimated 17,000 new SCIs occur in the U.S. Most of these are caused by trauma to the vertebral column , thereby affecting the spinal cord’s ability to send and receive messages from the brain to the body’s systems that control sensory, motor and autonomic function below the level of injury.
According to the Centers for Diseases Control and Prevention (CDC) , SCI costs the nation an estimated $9.7 billion each year.
Motor vehicle accidents are the leading cause of SCI in the U. S. in younger individuals, while falls are the leading cause for SCI for people over 65. Acts of violence and sports/recreation activities are other common causes for these injuries.Just over half of all SCI occurs in persons age 16-30, most of whom are male (80%). Males also represent nearly all (90%) of sports-related SCIs.
SYMPTOMS + TYPES
A complete SCI produces total loss of all motor and sensory function below the level of injury. Nearly 50% of all SCIs are complete. Both sides of the body are equally affected. Even with a complete SCI, the spinal cord is rarely cut or transected. More commonly, loss of function is caused by a contusion or bruise to the spinal cord or by compromise of blood flow to the injured part of the spinal cord.
In an incomplete SCI , some function remains below the primary level of the injury. A person with an incomplete injury may be able to move one arm or leg more than the other or may have more functioning on one side of the body than the other.
SCIs are graded according to the American Spinal Injury Association (ASIA) grading scale, which describes the severity of the injury. The scale is graded with letters:
- ASIA A : injury is complete spinal cord injury with no sensory or motor function preserved.
- ASIA B : a sensory incomplete injury with complete motor function loss.
- ASIA C : a motor incomplete injury, where there is some movement, but less than half the muscle groups are anti-gravity (can lift up against the force of gravity with a full range of motion).
- ASIA D : a motor incomplete injury with more than half of the muscle groups are anti-gravity.
- ASIA E : normal.
The more severe the injury, the less likely a recovery will occur.
Spinal concussions can also occur. These can be complete or incomplete, but spinal cord dysfunction is transient, generally resolving within one or two days. Football players are especially susceptible to spinal concussions and spinal cord contusions. The latter may produce neurological symptoms, including numbness, tingling, electric shock-like sensations and burning in the extremities.
Open or penetrating injuries to the spine and spinal cord, especially those caused by firearms, may present somewhat different challenges. Most gunshot wounds to the spine are stable; i.e., they do not carry as much risk of excessive and potentially dangerous motion of the injured parts of the spine. Depending upon the anatomy of the injury, the patient may need to be immobilized with a collar or brace for several weeks or months so that the parts of the spine fractured by the bullet heals. In most cases, surgery to remove the bullet does not yield much benefit and may create additional risks, including infection, cerebrospinal fluid leak and bleeding. However, occasional cases of gunshot wounds to the spine may require surgical decompression and/or fusion in an attempt to optimize outcome.
When & How to Seek Medical Care
Following trauma, seek immediate medical attention if you experience any of the following:
- Extreme pain or pressure in the neck, head or back
- Tingling or loss of sensation in the hand, fingers, feet or toes
- Partial or complete loss of control over any part of the body
- Urinary or bowel urgency, incontinence or retention
- Abnormal band-like sensations in the thorax (pain, pressure)
- Impaired breathing after injury
- Unusual lumps on the head or spine
Testing & Diagnosis
In the trauma situation, the doctor will check first to make sure the patient has a working airway, is breathing and has a pulse. The next step in the evaluation is to assess an individual’s neurologic function. The doctor will do this by testing the patient’s strength and sensation in his/her arms and legs. If there is obvious weakness or the patient is not fully awake, the patient is kept in a rigid cervical collar and on a spine board until a full imaging assessment can be complete.
Radiological Evaluation
Historically, the radiological diagnosis of SCI started with x-rays. However, with the technological advancements and availability at most hospitals, the entire spine may be imaged with computerized tomography (CT or CAT scan) as an initial screen to identify fractures and other bony abnormalities. For patients with known or suspected injuries, MRI is helpful for looking at the actual spinal cord itself as well as for detecting any blood clots, herniated discs or other masses that may be compressing the spinal cord.
Treatment of SCI begins before the patient is admitted to the hospital. Paramedics or other emergency medical services personnel carefully immobilize the entire spine at the scene of the accident. In the emergency department, this immobilization is continued while more immediate life-threatening problems are identified and addressed. If the patient must undergo emergency surgery because of trauma to the abdomen, chest or another area, immobilization and alignment of the spine are maintained during the operation.
Non-Surgical Treatments
If a patient has a SCI, he or she will usually be admitted to an intensive care unit (ICU). For many injuries of the cervical spine, traction may be indicated to help bring the spine into proper alignment. Standard ICU care, including maintaining a stable blood pressure, monitoring cardiovascular function, ensuring adequate ventilation and lung function and preventing and promptly treating infection and other complications, is essential so that SCI patients can achieve the best possible outcome.
Occasionally, a surgeon may wish to take a patient to the operating room immediately if the spinal cord appears to be compressed by a herniated disc, blood clot or other lesion. This is most commonly done for patients with an incomplete SCI or with progressive neurological deterioration. Even if surgery cannot reverse damage to the spinal cord, surgery may be needed to stabilize the spine to prevent future pain or deformity. The surgeon will decide which procedure will provide the greatest benefit to the patient.
Persons with neurologically complete tetraplegia are at high risk for secondary medical complications, including pneumonia, pressure ulcers and deep vein thrombosis. Pressure ulcers are the most frequently observed complications, beginning at 15% during the first year post-injury and steadily increasing thereafter.
Recovery of function depends upon the severity of the initial injury . Unfortunately, those who sustain a complete SCI are unlikely to regain function below the level of injury. However, if there is some degree of improvement, it usually evidences itself within the first few days after the accident.
Incomplete injuries usually show some degree of improvement over time, but this varies with the type of injury. Although full recovery may be unlikely in most cases, some patients may be able to improve at least enough to ambulate and to control bowel and bladder function.
Once a patient is stabilized, care and treatment focuses on supportive care and rehabilitation. Family members, nurses or specially trained aides all may provide supportive care. This care might include helping the patient bathe, dress, change positions to prevent bedsores and other assistance.
Rehabilitation often includes physical therapy, occupational therapy and counseling for emotional support. The services may initially be provided while the patient is hospitalized. Following hospitalization, some patients are admitted to a rehabilitation facility. Other patients can continue rehab on an outpatient basis and/or at home.
Latest Research
- Nature.com. (2019). Spinal Cord Injury
- NIH. (2018). Spinal Cord Injury: Hope Through Research
- Masterson, K. (2018). A New Spinal Cord Injury Treatment is Getting Patients Back on Their Feet. Retrieved from
Resources for More Information
Spinal Cord Injury Facts and Figures:
- American Spinal Injury Association
- National Spinal Cord Injury Statistical Center. (2018). Spinal Cord Injury Facts and Figures at a Glance.
- World Health Organization
Other resources:
Christopher Reeve Paralysis Foundation Foundation for SCI Prevention, Care & Cure The National SCI Association (NSCIA) The Travis Roy Foundation
Note from AANS
The AANS does not endorse any treatments, procedures, products or physicians referenced in these patient fact sheets. This information is provided as an educational service and is not intended to serve as medical advice. Anyone seeking specific neurosurgical advice or assistance should consult his or her neurosurgeon, or locate one in your area through the AANS’ Find a Board-certified Neurosurgeon online tool.
We value your privacy
Privacy overview.
Cookie | Duration | Description |
---|---|---|
__cf_bm | 1 hour | This cookie, set by Cloudflare, is used to support Cloudflare Bot Management. |
ASPSESSIONIDACQATATC | session | ASP Session cookie |
ASPSESSIONIDACQBSDQD | session | ASP Session cookie |
ASPSESSIONIDSARBSARA | session | ASP Session cookie |
ASPSESSIONIDSCDBCDAB | session | ASP Session cookie |
AWSALBCORS | 7 days | Amazon Web Services set this cookie for load balancing. |
cookielawinfo-checkbox-advertisement | 1 year | Set by the GDPR Cookie Consent plugin, this cookie records the user consent for the cookies in the "Advertisement" category. |
cookielawinfo-checkbox-analytics | 11 months | This cookie is set by GDPR Cookie Consent plugin. The cookie is used to store the user consent for the cookies in the category "Analytics". |
cookielawinfo-checkbox-functional | 11 months | The cookie is set by GDPR cookie consent to record the user consent for the cookies in the category "Functional". |
cookielawinfo-checkbox-necessary | 11 months | This cookie is set by GDPR Cookie Consent plugin. The cookies is used to store the user consent for the cookies in the category "Necessary". |
cookielawinfo-checkbox-others | 11 months | This cookie is set by GDPR Cookie Consent plugin. The cookie is used to store the user consent for the cookies in the category "Other. |
cookielawinfo-checkbox-performance | 11 months | This cookie is set by GDPR Cookie Consent plugin. The cookie is used to store the user consent for the cookies in the category "Performance". |
CookieLawInfoConsent | 1 year | CookieYes sets this cookie to record the default button state of the corresponding category and the status of CCPA. It works only in coordination with the primary cookie. |
viewed_cookie_policy | 11 months | The cookie is set by the GDPR Cookie Consent plugin and is used to store whether or not user has consented to the use of cookies. It does not store any personal data. |
wpEmojiSettingsSupports | session | WordPress sets this cookie when a user interacts with emojis on a WordPress site. It helps determine if the user's browser can display emojis properly. |
Cookie | Duration | Description |
---|---|---|
yt-player-headers-readable | never | The yt-player-headers-readable cookie is used by YouTube to store user preferences related to video playback and interface, enhancing the user's viewing experience. |
yt-remote-cast-available | session | The yt-remote-cast-available cookie is used to store the user's preferences regarding whether casting is available on their YouTube video player. |
yt-remote-cast-installed | session | The yt-remote-cast-installed cookie is used to store the user's video player preferences using embedded YouTube video. |
yt-remote-connected-devices | never | YouTube sets this cookie to store the user's video preferences using embedded YouTube videos. |
yt-remote-device-id | never | YouTube sets this cookie to store the user's video preferences using embedded YouTube videos. |
yt-remote-fast-check-period | session | The yt-remote-fast-check-period cookie is used by YouTube to store the user's video player preferences for embedded YouTube videos. |
yt-remote-session-app | session | The yt-remote-session-app cookie is used by YouTube to store user preferences and information about the interface of the embedded YouTube video player. |
yt-remote-session-name | session | The yt-remote-session-name cookie is used by YouTube to store the user's video player preferences using embedded YouTube video. |
ytidb::LAST_RESULT_ENTRY_KEY | never | The cookie ytidb::LAST_RESULT_ENTRY_KEY is used by YouTube to store the last search result entry that was clicked by the user. This information is used to improve the user experience by providing more relevant search results in the future. |
Cookie | Duration | Description |
---|---|---|
AWSALB | 7 days | AWSALB is an application load balancer cookie set by Amazon Web Services to map the session to the target. |
Cookie | Duration | Description |
---|---|---|
_ga | 1 year 1 month 4 days | Google Analytics sets this cookie to calculate visitor, session and campaign data and track site usage for the site's analytics report. The cookie stores information anonymously and assigns a randomly generated number to recognise unique visitors. |
_ga_* | 1 year 1 month 4 days | Google Analytics sets this cookie to store and count page views. |
_gcl_au | 3 months | Google Tag Manager sets the cookie to experiment advertisement efficiency of websites using their services. |
_sp_id.* | 1 year 1 month 4 days | Snowplow sets this cookie to store user information that is created when a user first visits a site and is updated on subsequent visits. |
_sp_ses.* | 1 hour | Snowplow sets this cookie to store user information that is created when a user first visits a site and is updated on subsequent visits. |
feathr_session_id | 1 hour | This cookie is set by Feathr. This cookie is used to keep track of user activity. |
Cookie | Duration | Description |
---|---|---|
f_id | 1 year | This cookie is used by feathr to track pages. The cookie establishes a unique identification per browser which helps in advertising. |
IDE | 1 year 24 days | Google DoubleClick IDE cookies store information about how the user uses the website to present them with relevant ads according to the user profile. |
test_cookie | 15 minutes | doubleclick.net sets this cookie to determine if the user's browser supports cookies. |
VISITOR_INFO1_LIVE | 6 months | YouTube sets this cookie to measure bandwidth, determining whether the user gets the new or old player interface. |
VISITOR_PRIVACY_METADATA | 6 months | YouTube sets this cookie to store the user's cookie consent state for the current domain. |
YSC | session | Youtube sets this cookie to track the views of embedded videos on Youtube pages. |
yt.innertube::nextId | never | YouTube sets this cookie to register a unique ID to store data on what videos from YouTube the user has seen. |
yt.innertube::requests | never | YouTube sets this cookie to register a unique ID to store data on what videos from YouTube the user has seen. |
Cookie | Duration | Description |
---|---|---|
_cfuvid | session | Description is currently not available. |
ASPSESSIONIDCAQARCSD | session | Description is currently not available. |
ASPSESSIONIDQCBCDABA | session | Description is currently not available. |
ASPSESSIONIDQCDCABBB | session | Description is currently not available. |
AWSALBTG | 7 days | No description available. |
AWSALBTGCORS | 7 days | No description available. |
cf_clearance | 1 year | Description is currently not available. |
OasisLMS | session | Description is currently not available. |

An official website of the United States government
Here’s how you know
Official websites use .gov A .gov website belongs to an official government organization in the United States.
Secure .gov websites use HTTPS A lock ( Lock Locked padlock icon ) or https:// means you’ve safely connected to the .gov website. Share sensitive information only on official, secure websites.

Alcohol's Effects on Health
Research-based information on drinking and its impact.
National Institute on Alcohol Abuse and Alcoholism (NIAAA)
Alcohol facts and statistics.

Updated: 2024
Learn up-to-date facts and statistics on alcohol consumption and its impact in the United States and globally. Explore topics related to alcohol misuse and treatment, underage drinking, the effects of alcohol on the human body, and more.
Find up-to-date statistics on lifetime drinking, past-year drinking, past-month drinking, binge drinking, heavy alcohol use, and high-intensity drinking.
Learn how many people ages 12 to 20 engage in underage alcohol misuse in the United States and the impact it has.
Explore how many people ages 18 to 25 engage in alcohol misuse in the United States and the impact it has.
Find out how many people have alcohol use disorder in the United States across age groups and demographics.
Discover how many people with alcohol use disorder in the United States receive treatment across age groups and demographics.
Discover the effects that alcohol consumption has on the human body such as increased risk for diseases and injuries.
Learn statistics about alcohol use during pregnancy.
Discover the impact alcohol has on children living with a parent or caregiver with alcohol use disorder.
Explore statistics on alcohol-related deaths and emergency visits in the United States.
Learn more about the financial impact of alcohol misuse in the United States.
Learn more about impacts of alcohol misuse globally.
niaaa.nih.gov
An official website of the National Institutes of Health and the National Institute on Alcohol Abuse and Alcoholism
Have a language expert improve your writing
Run a free plagiarism check in 10 minutes, generate accurate citations for free.
- Knowledge Base
Methodology
- Quasi-Experimental Design | Definition, Types & Examples
Quasi-Experimental Design | Definition, Types & Examples
Published on July 31, 2020 by Lauren Thomas . Revised on January 22, 2024.
Like a true experiment , a quasi-experimental design aims to establish a cause-and-effect relationship between an independent and dependent variable .
However, unlike a true experiment, a quasi-experiment does not rely on random assignment . Instead, subjects are assigned to groups based on non-random criteria.
Quasi-experimental design is a useful tool in situations where true experiments cannot be used for ethical or practical reasons.
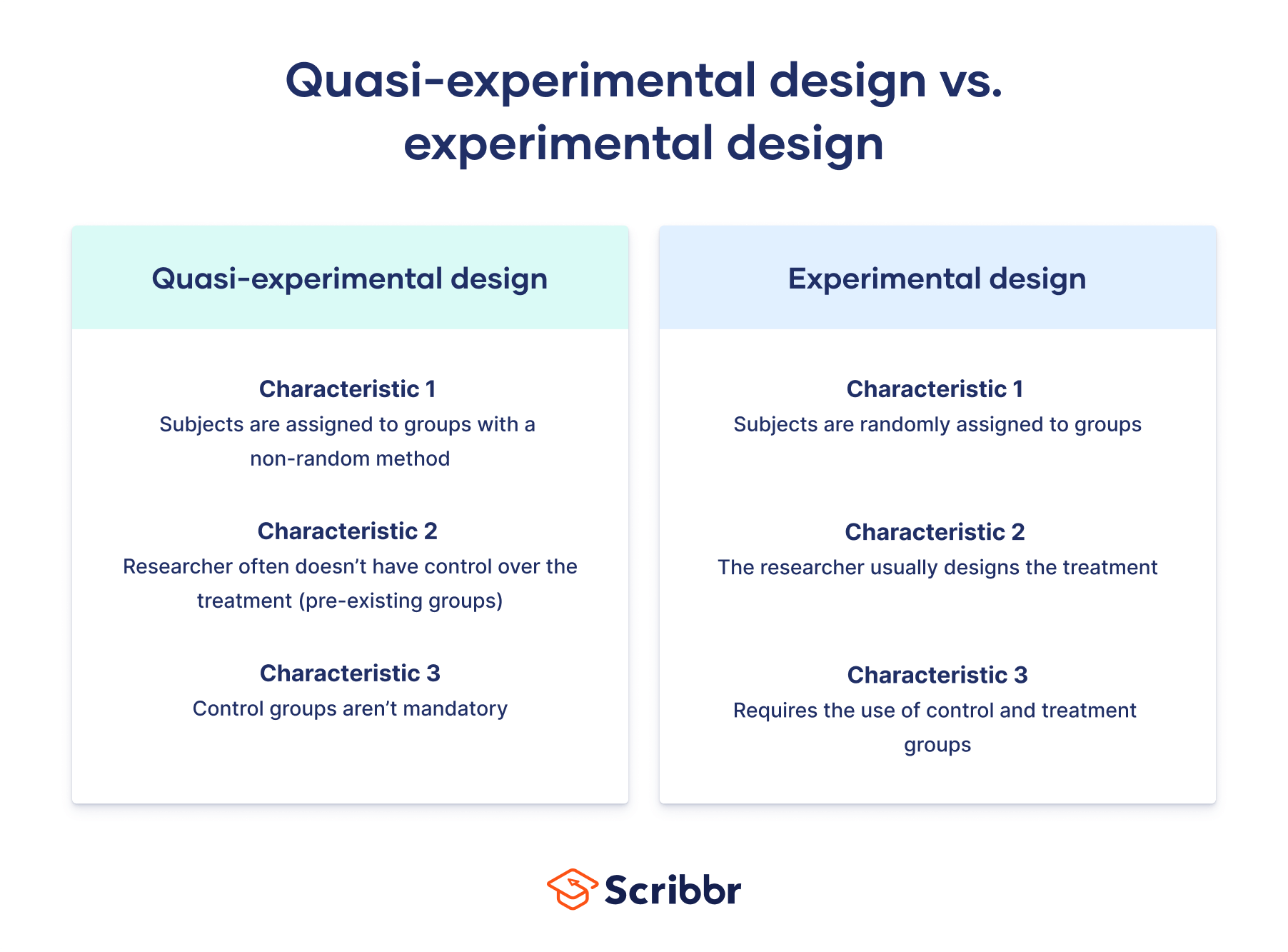
Table of contents
Differences between quasi-experiments and true experiments, types of quasi-experimental designs, when to use quasi-experimental design, advantages and disadvantages, other interesting articles, frequently asked questions about quasi-experimental designs.
There are several common differences between true and quasi-experimental designs.
True experimental design | Quasi-experimental design | |
---|---|---|
Assignment to treatment | The researcher subjects to control and treatment groups. | Some other, method is used to assign subjects to groups. |
Control over treatment | The researcher usually . | The researcher often , but instead studies pre-existing groups that received different treatments after the fact. |
Use of | Requires the use of . | Control groups are not required (although they are commonly used). |
Example of a true experiment vs a quasi-experiment
However, for ethical reasons, the directors of the mental health clinic may not give you permission to randomly assign their patients to treatments. In this case, you cannot run a true experiment.
Instead, you can use a quasi-experimental design.
You can use these pre-existing groups to study the symptom progression of the patients treated with the new therapy versus those receiving the standard course of treatment.
Here's why students love Scribbr's proofreading services
Discover proofreading & editing
Many types of quasi-experimental designs exist. Here we explain three of the most common types: nonequivalent groups design, regression discontinuity, and natural experiments.
Nonequivalent groups design
In nonequivalent group design, the researcher chooses existing groups that appear similar, but where only one of the groups experiences the treatment.
In a true experiment with random assignment , the control and treatment groups are considered equivalent in every way other than the treatment. But in a quasi-experiment where the groups are not random, they may differ in other ways—they are nonequivalent groups .
When using this kind of design, researchers try to account for any confounding variables by controlling for them in their analysis or by choosing groups that are as similar as possible.
This is the most common type of quasi-experimental design.
Regression discontinuity
Many potential treatments that researchers wish to study are designed around an essentially arbitrary cutoff, where those above the threshold receive the treatment and those below it do not.
Near this threshold, the differences between the two groups are often so minimal as to be nearly nonexistent. Therefore, researchers can use individuals just below the threshold as a control group and those just above as a treatment group.
However, since the exact cutoff score is arbitrary, the students near the threshold—those who just barely pass the exam and those who fail by a very small margin—tend to be very similar, with the small differences in their scores mostly due to random chance. You can therefore conclude that any outcome differences must come from the school they attended.
Natural experiments
In both laboratory and field experiments, researchers normally control which group the subjects are assigned to. In a natural experiment, an external event or situation (“nature”) results in the random or random-like assignment of subjects to the treatment group.
Even though some use random assignments, natural experiments are not considered to be true experiments because they are observational in nature.
Although the researchers have no control over the independent variable , they can exploit this event after the fact to study the effect of the treatment.
However, as they could not afford to cover everyone who they deemed eligible for the program, they instead allocated spots in the program based on a random lottery.
Although true experiments have higher internal validity , you might choose to use a quasi-experimental design for ethical or practical reasons.
Sometimes it would be unethical to provide or withhold a treatment on a random basis, so a true experiment is not feasible. In this case, a quasi-experiment can allow you to study the same causal relationship without the ethical issues.
The Oregon Health Study is a good example. It would be unethical to randomly provide some people with health insurance but purposely prevent others from receiving it solely for the purposes of research.
However, since the Oregon government faced financial constraints and decided to provide health insurance via lottery, studying this event after the fact is a much more ethical approach to studying the same problem.
True experimental design may be infeasible to implement or simply too expensive, particularly for researchers without access to large funding streams.
At other times, too much work is involved in recruiting and properly designing an experimental intervention for an adequate number of subjects to justify a true experiment.
In either case, quasi-experimental designs allow you to study the question by taking advantage of data that has previously been paid for or collected by others (often the government).
Quasi-experimental designs have various pros and cons compared to other types of studies.
- Higher external validity than most true experiments, because they often involve real-world interventions instead of artificial laboratory settings.
- Higher internal validity than other non-experimental types of research, because they allow you to better control for confounding variables than other types of studies do.
- Lower internal validity than true experiments—without randomization, it can be difficult to verify that all confounding variables have been accounted for.
- The use of retrospective data that has already been collected for other purposes can be inaccurate, incomplete or difficult to access.
Prevent plagiarism. Run a free check.
If you want to know more about statistics , methodology , or research bias , make sure to check out some of our other articles with explanations and examples.
- Normal distribution
- Degrees of freedom
- Null hypothesis
- Discourse analysis
- Control groups
- Mixed methods research
- Non-probability sampling
- Quantitative research
- Ecological validity
Research bias
- Rosenthal effect
- Implicit bias
- Cognitive bias
- Selection bias
- Negativity bias
- Status quo bias
A quasi-experiment is a type of research design that attempts to establish a cause-and-effect relationship. The main difference with a true experiment is that the groups are not randomly assigned.
In experimental research, random assignment is a way of placing participants from your sample into different groups using randomization. With this method, every member of the sample has a known or equal chance of being placed in a control group or an experimental group.
Quasi-experimental design is most useful in situations where it would be unethical or impractical to run a true experiment .
Quasi-experiments have lower internal validity than true experiments, but they often have higher external validity as they can use real-world interventions instead of artificial laboratory settings.
Cite this Scribbr article
If you want to cite this source, you can copy and paste the citation or click the “Cite this Scribbr article” button to automatically add the citation to our free Citation Generator.
Thomas, L. (2024, January 22). Quasi-Experimental Design | Definition, Types & Examples. Scribbr. Retrieved June 18, 2024, from https://www.scribbr.com/methodology/quasi-experimental-design/
Is this article helpful?
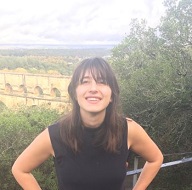
Lauren Thomas
Other students also liked, guide to experimental design | overview, steps, & examples, random assignment in experiments | introduction & examples, control variables | what are they & why do they matter, "i thought ai proofreading was useless but..".
I've been using Scribbr for years now and I know it's a service that won't disappoint. It does a good job spotting mistakes”
- Patient Care & Health Information
- Diseases & Conditions
Hair loss (alopecia) can affect just your scalp or your entire body, and it can be temporary or permanent. It can be the result of heredity, hormonal changes, medical conditions or a normal part of aging. Anyone can lose hair on their head, but it's more common in men.
Baldness typically refers to excessive hair loss from your scalp. Hereditary hair loss with age is the most common cause of baldness. Some people prefer to let their hair loss run its course untreated and unhidden. Others may cover it up with hairstyles, makeup, hats or scarves. And still others choose one of the treatments available to prevent further hair loss or restore growth.
Before pursuing hair loss treatment, talk with your doctor about the cause of your hair loss and treatment options.
Products & Services
- A Book: Mayo Clinic Family Health Book
- Newsletter: Mayo Clinic Health Letter — Digital Edition
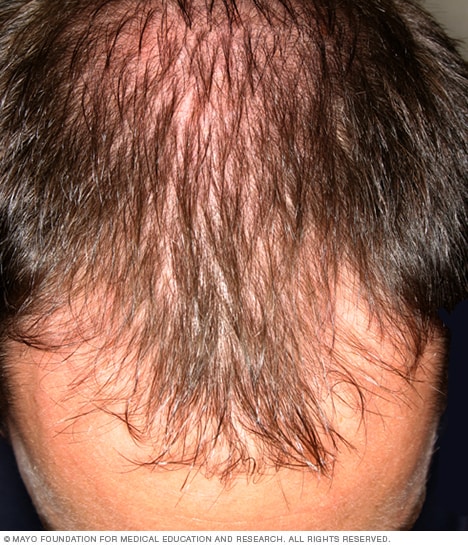
- Male-pattern baldness
Male-pattern baldness typically appears first at the hairline or top of the head. It can progress to partial or complete baldness.
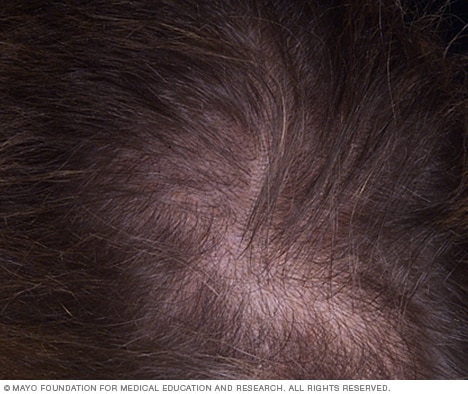
- Female-pattern baldness
Female-pattern baldness typically starts with scalp hairs becoming progressively less dense. Many women first experience hair thinning and hair loss where they part their hair and on the top-central portion of the head.
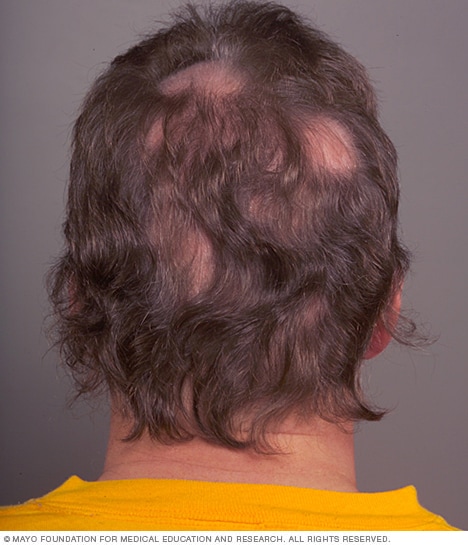
- Patchy hair loss (alopecia areata)
In the type of patchy hair loss known as alopecia areata, hair loss occurs suddenly and usually starts with one or more circular bald patches that may overlap.
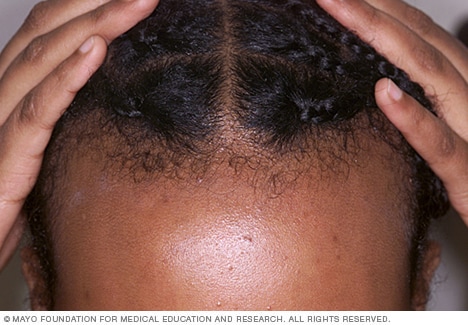
- Traction alopecia
Hair loss can occur if you wear pigtails, braids or cornrows, or use tight hair rollers. This is called traction alopecia.
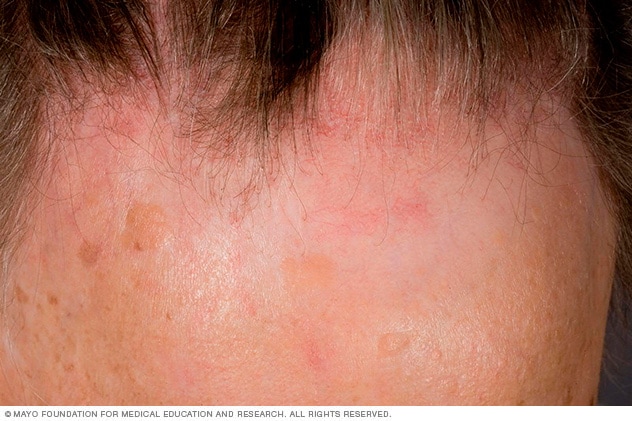
- Frontal fibrosing alopecia
Early treatment of a receding hairline (frontal fibrosing alopecia) might help avoid significant permanent baldness. The cause of this condition is unknown, but it primarily affects older women.
Hair loss can appear in many different ways, depending on what's causing it. It can come on suddenly or gradually and affect just your scalp or your whole body.
Signs and symptoms of hair loss may include:
- Gradual thinning on top of head. This is the most common type of hair loss, affecting people as they age. In men, hair often begins to recede at the hairline on the forehead. Women typically have a broadening of the part in their hair. An increasingly common hair loss pattern in older women is a receding hairline (frontal fibrosing alopecia).
- Circular or patchy bald spots. Some people lose hair in circular or patchy bald spots on the scalp, beard or eyebrows. Your skin may become itchy or painful before the hair falls out.
- Sudden loosening of hair. A physical or emotional shock can cause hair to loosen. Handfuls of hair may come out when combing or washing your hair or even after gentle tugging. This type of hair loss usually causes overall hair thinning but is temporary.
- Full-body hair loss. Some conditions and medical treatments, such as chemotherapy for cancer, can result in the loss of hair all over your body. The hair usually grows back.
- Patches of scaling that spread over the scalp. This is a sign of ringworm. It may be accompanied by broken hair, redness, swelling and, at times, oozing.
When to see a doctor
See your doctor if you are distressed by persistent hair loss in you or your child and want to pursue treatment. For women who are experiencing a receding hairline (frontal fibrosing alopecia), talk with your doctor about early treatment to avoid significant permanent baldness.
Also talk to your doctor if you notice sudden or patchy hair loss or more than usual hair loss when combing or washing your or your child's hair. Sudden hair loss can signal an underlying medical condition that requires treatment.
There is a problem with information submitted for this request. Review/update the information highlighted below and resubmit the form.
From Mayo Clinic to your inbox
Sign up for free and stay up to date on research advancements, health tips, current health topics, and expertise on managing health. Click here for an email preview.
Error Email field is required
Error Include a valid email address
To provide you with the most relevant and helpful information, and understand which information is beneficial, we may combine your email and website usage information with other information we have about you. If you are a Mayo Clinic patient, this could include protected health information. If we combine this information with your protected health information, we will treat all of that information as protected health information and will only use or disclose that information as set forth in our notice of privacy practices. You may opt-out of email communications at any time by clicking on the unsubscribe link in the e-mail.
Thank you for subscribing!
You'll soon start receiving the latest Mayo Clinic health information you requested in your inbox.
Sorry something went wrong with your subscription
Please, try again in a couple of minutes
People typically lose 50 to 100 hairs a day. This usually isn't noticeable because new hair is growing in at the same time. Hair loss occurs when new hair doesn't replace the hair that has fallen out.
Hair loss is typically related to one or more of the following factors:
- Family history (heredity). The most common cause of hair loss is a hereditary condition that happens with aging. This condition is called androgenic alopecia, male-pattern baldness and female-pattern baldness. It usually occurs gradually and in predictable patterns — a receding hairline and bald spots in men and thinning hair along the crown of the scalp in women.
- Hormonal changes and medical conditions. A variety of conditions can cause permanent or temporary hair loss, including hormonal changes due to pregnancy, childbirth, menopause and thyroid problems. Medical conditions include alopecia areata (al-o-PEE-she-uh ar-e-A-tuh), which is immune system related and causes patchy hair loss, scalp infections such as ringworm, and a hair-pulling disorder called trichotillomania (trik-o-til-o-MAY-nee-uh).
- Medications and supplements. Hair loss can be a side effect of certain drugs, such as those used for cancer, arthritis, depression, heart problems, gout and high blood pressure.
- Radiation therapy to the head. The hair may not grow back the same as it was before.
- A very stressful event. Many people experience a general thinning of hair several months after a physical or emotional shock. This type of hair loss is temporary.
- Hairstyles and treatments. Excessive hairstyling or hairstyles that pull your hair tight, such as pigtails or cornrows, can cause a type of hair loss called traction alopecia. Hot-oil hair treatments and permanents also can cause hair to fall out. If scarring occurs, hair loss could be permanent.
More Information
Hair loss care at Mayo Clinic
Risk factors
A number of factors can increase your risk of hair loss, including:
- A family history of balding on your mother's or father's side
- Significant weight loss
- Certain medical conditions, such as diabetes and lupus
- Poor nutrition
Most baldness is caused by genetics (male-pattern baldness and female-pattern baldness). This type of hair loss is not preventable.
These tips may help you avoid preventable types of hair loss:
- Be gentle with your hair. Use a detangler and avoid tugging when brushing and combing, especially when your hair is wet. A wide-toothed comb might help prevent pulling out hair. Avoid harsh treatments such as hot rollers, curling irons, hot-oil treatments and permanents. Limit the tension on hair from styles that use rubber bands, barrettes and braids.
- Ask your doctor about medications and supplements you take that might cause hair loss.
- Protect your hair from sunlight and other sources of ultraviolet light.
- Stop smoking. Some studies show an association between smoking and baldness in men.
- If you're being treated with chemotherapy, ask your doctor about a cooling cap. This cap can reduce your risk of losing hair during chemotherapy.
Living with hair loss?
Connect with others like you for support and answers to your questions in the Cancer: Managing Symptoms support group on Mayo Clinic Connect, a patient community.
Cancer: Managing Symptoms Discussions

108 Replies Wed, Jun 12, 2024
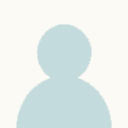
186 Replies Mon, Jun 10, 2024
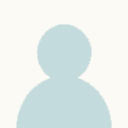
70 Replies Tue, Jun 11, 2024
- AskMayoExpert. Alopecia. Mayo Clinic; 2019.
- Malki L, et al. Variant PAD13 in central centrifugal cicatricial alopecia. New England Journal of Medicine. 2019; doi:10.1056/NEJMoa1816614.
- Briggs JK. Hair loss. Triage Protocols for Aging Adults. Waloters Kluwer; 2019.
- Warner KJ. Allscripts EPSi. Mayo Clinic, Nov. 25, 2019.
- Office of Patient Education. Hair replacement surgery. Mayo Clinic; 2017.
- Hair loss. American Academy of Dermatology. https://www.aad.org/public/diseases/hair-loss/treatment/causes/fall-out. Accessed Jan. 31, 2020.
- Hair loss. American Cancer Society. https://www.cancer.org/treatment/treatments-and-side-effects/physical-side-effects/hair-loss.html. Accessed Jan. 2, 2020.
- Van Zuuren EJ, et al. Interventions for female pattern hair loss. JAMA Dermatology. 2017; doi:10.1001/jamadermatol.2016.5790.
- Shapiro J, et al. Evaluation and diagnosis of hair loss. https://www.uptodate.com/contents/search. Accessed Jan. 2, 2020.
- Shapiro J, et al. Alopecia areata: Clinical manifestations and diagnosis. https://www.uptodate.com/contents/search. Accessed Jan. 2, 2020.
- Hereditary hair loss. Mayo Clinic Health Letter. 2016;4:1.
- Cold caps and scalp cooling systems. https://www.breastcancer.org/tips/hair_skin_nails/cold-caps. Accessed Jan. 31, 2020.
- Mirmirati P. Age-related hair changes in men: Mechanisms and management of alopecia and graying. Maturitas. 2015; doi.10.1016/j.maturitas.2014.10.008.
- Chemotherapy and you: Support for people with cancer. National Cancer Institute. https://www.cancer.gov/publications/patient-education/chemotherapy-and-you.pdf. Accessed Feb. 3, 2020.
- Shapiro J. Lichen planopilaris. https://www.uptodate.com/contents/search. Accessed March 26, 2020.
- Gibson LE (expert opinion). Mayo Clinic. March 26, 2020.
- Symptoms & causes
- Diagnosis & treatment
- Doctors & departments
- Care at Mayo Clinic
Mayo Clinic does not endorse companies or products. Advertising revenue supports our not-for-profit mission.
- Opportunities
Mayo Clinic Press
Check out these best-sellers and special offers on books and newsletters from Mayo Clinic Press .
- Mayo Clinic on Incontinence - Mayo Clinic Press Mayo Clinic on Incontinence
- The Essential Diabetes Book - Mayo Clinic Press The Essential Diabetes Book
- Mayo Clinic on Hearing and Balance - Mayo Clinic Press Mayo Clinic on Hearing and Balance
- FREE Mayo Clinic Diet Assessment - Mayo Clinic Press FREE Mayo Clinic Diet Assessment
- Mayo Clinic Health Letter - FREE book - Mayo Clinic Press Mayo Clinic Health Letter - FREE book
Your gift holds great power – donate today!
Make your tax-deductible gift and be part of the cutting-edge research and care that's changing medicine.
An official website of the United States government
Here’s how you know
Official websites use .gov A .gov website belongs to an official government organization in the United States.
Secure .gov websites use HTTPS A lock ( Lock Locked padlock icon ) or https:// means you’ve safely connected to the .gov website. Share sensitive information only on official, secure websites.
- Entire Site
- Research & Funding
- Health Information
- About NIDDK
- Endocrine Diseases
- Adrenal Insufficiency & Addison's Disease
- Symptoms & Causes
- Español
Symptoms & Causes of Adrenal Insufficiency & Addison's Disease
What are the symptoms of adrenal insufficiency.
The most common symptoms of adrenal insufficiency are
- chronic, or long-lasting, fatigue
- muscle weakness
- loss of appetite
- weight loss
- abdominal pain
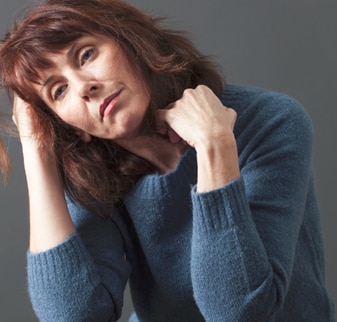
Other symptoms of adrenal insufficiency can include
- low blood pressure that drops further when you stand up, causing dizziness or fainting
- irritability and depression
- craving salty foods
- hypoglycemia , or low blood glucose
- irregular or no menstrual periods
- loss of interest in sex
People with Addison’s disease may also have darkening of their skin. This darkening is most visible on scars; skin folds; pressure points such as the elbows, knees, knuckles, and toes; lips; and mucous membranes such as the lining of the cheek.
Because symptoms of adrenal insufficiency come on slowly over time, they may be overlooked or confused with other illnesses. Sometimes symptoms appear for the first time during adrenal crisis. If you always feel tired, weak, or are losing weight, ask your health care professional if you might have adrenal insufficiency. Early treatment can help avoid an adrenal crisis.
What causes adrenal insufficiency?
Different types of adrenal insufficiency have different causes. The most common cause of adrenal insufficiency overall is suddenly stopping corticosteroids after taking them for a long time.
Addison’s disease
Damage to the adrenal glands in Addison’s disease is usually caused by autoimmune disease—when your immune system attacks your body’s own cells and organs. In developed countries, autoimmune disease causes 8 or 9 of every 10 cases of Addison’s disease. 4
Certain infections can also cause Addison’s disease. Tuberculosis (TB) can damage the adrenal glands and used to be the most common cause of Addison’s disease. As treatment improved over the years, TB became a much less common cause. People with HIV/AIDS , whose weakened immune systems can’t fight off infections that could cause Addison’s disease, are also at risk.
Less common causes of Addison’s disease are
- cancer cells in the adrenal glands
- surgical removal of the adrenal glands to treat other conditions
- bleeding into the adrenal glands
- genetic disorders that affect the way the adrenal glands develop or function
- certain medicines, such as antifungal medicines or etomidate, a type of general anesthesia
Secondary adrenal insufficiency
Anything that affects the pituitary’s ability to make ACTH can cause secondary adrenal insufficiency. The pituitary makes many different hormones, so ACTH may not be the only hormone that’s lacking.
Causes of secondary adrenal insufficiency include
- autoimmune disease
- pituitary tumors or infection
- bleeding in the pituitary
- genetic diseases that affect the way the pituitary gland develops or functions
- surgical removal of the pituitary to treat other conditions
- traumatic brain injury
Tertiary adrenal insufficiency
The most common cause of tertiary adrenal insufficiency is suddenly stopping corticosteroids after taking them for a long time. Prescription doses of corticosteroids can cause higher levels of cortisol in your blood than your body normally makes. High levels in your blood for a long time cause the hypothalamus to make less CRH. Less CRH means less ACTH, which in turn causes the adrenal glands to stop making cortisol.
Once you stop taking corticosteriods, your adrenal glands may be slow to start working again. To give them time to start making cortisol again, your doctor will gradually reduce your dose over a period of weeks or even months. Even so, your adrenal glands might not begin to work normally for many months. Your doctor should watch you carefully for symptoms of adrenal insufficiency.
Tertiary adrenal insufficiency can also occur after Cushing’s syndrome is cured. Cushing’s syndrome is a hormonal disorder caused by high levels of cortisol in the blood for a long time. Sometimes Cushing’s syndrome is caused by tumors, usually noncancerous, in the pituitary or adrenal glands that make too much ACTH or cortisol. Once the tumors are surgically removed, the source of excess ACTH or cortisol is suddenly gone. Your adrenal glands may be slow to start working again.
This content is provided as a service of the National Institute of Diabetes and Digestive and Kidney Diseases (NIDDK), part of the National Institutes of Health. NIDDK translates and disseminates research findings to increase knowledge and understanding about health and disease among patients, health professionals, and the public. Content produced by NIDDK is carefully reviewed by NIDDK scientists and other experts.
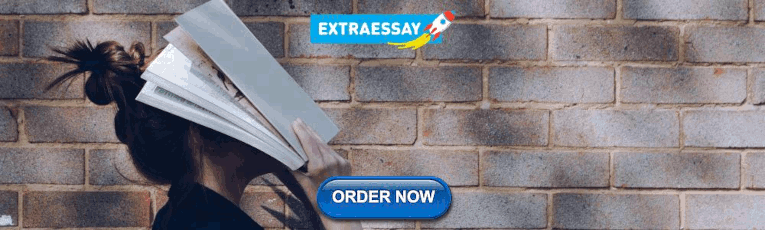
COMMENTS
You can't rely on just the outcomes of causal research as it's inaccurate. It's best to conduct other types of research alongside it to confirm its output. Trouble establishing cause and effect; Researchers might identify that two variables are connected, but struggle to determine which is the cause and which variable is the effect.
Causal research, on the other hand, seeks to identify cause-and-effect relationships between variables by systematically manipulating independent variables and observing their effects on dependent variables. Unlike descriptive research, causal research aims to determine whether changes in one variable directly cause changes in another variable.
You can also create a mixed methods research design that has elements of both. Descriptive research vs experimental research. Descriptive research gathers data without controlling any variables, while experimental research manipulates and controls variables to determine cause and effect.
Causal research is sometimes called an explanatory or analytical study. It delves into the fundamental cause-and-effect connections between two or more variables. Researchers typically observe how changes in one variable affect another related variable. Examining these relationships gives researchers valuable insights into the mechanisms that ...
Step 2: Choose a type of research design. Within both qualitative and quantitative approaches, there are several types of research design to choose from. Each type provides a framework for the overall shape of your research. ... Experiments are the strongest way to test cause-and-effect relationships without the risk of other variables ...
Cause-and-effect relationships happen in all aspects of life, from business to medicine, to marketing, to education, and so much more. They are the invisible threads that connect both our actions and inactions to their outcomes. Causal research is the type of research that investigates cause-and-effect relationships.
That is, randomized experiments allow researchers to be confident about a cause-effect relationship with only very few additional assumptions, but many more assumptions might be needed to convincingly argue that this cause-effect relationship is actually the one of interest. ... Designing Studies and Evaluating Research Results: Type M and Type ...
Establishing Cause and Effect. A central goal of most research is the identification of causal relationships, or demonstrating that a particular independent variable (the cause) has an effect on the dependent variable of interest (the effect). The three criteria for establishing cause and effect - association, time ordering (or temporal precedence), and non-spuriousness - are familiar to ...
Causal research is also known as explanatory research. It's a type of research that examines if there's a cause-and-effect relationship between two separate events. This would occur when there is a change in one of the independent variables, which is causing changes in the dependent variable. You can use causal research to evaluate the ...
Causal research, also known as explanatory research is conducted in order to identify the extent and nature of cause-and-effect relationships. Causal research can be conducted in order to assess impacts of specific changes on existing norms, various processes etc. Causal studies focus on an analysis of a situation or a specific problem to ...
Causal Study Design. Researchers conduct experiments to study cause and effect relationships and to estimate the impact of child care and early childhood programs on children and their families. There are two basic types of experiments: Randomized experiments. Quasi-experiments.
Nature Methods 7 , 243 ( 2010) Cite this article. The experimental tractability of biological systems makes it possible to explore the idea that causal relationships can be estimated from ...
Psychology research can usually be classified as one of three major types. 1. Causal or Experimental Research. When most people think of scientific experimentation, research on cause and effect is most often brought to mind. Experiments on causal relationships investigate the effect of one or more variables on one or more outcome variables.
Regression-discontinuity methods have been little used in medical research to date, but they can be helpful in the study of cause-and-effect relationships from observational data . Regression-discontinuity design is a quasi-experimental approach ( box 3 ) that was developed in educational psychology in the 1960s ( 18 ).
Cause and effect understanding is the highest achievement (the jewel in the crown) of scientific knowledge, including epidemiology. ... an ethical responsibility to apply knowledge even when, from a scientific point of view, further research is advised. Yet, this ethical imperative may be perilous. Early application of knowledge sometimes has ...
1. Lab Experiment. A laboratory experiment in psychology is a research method in which the experimenter manipulates one or more independent variables and measures the effects on the dependent variable under controlled conditions. A laboratory experiment is conducted under highly controlled conditions (not necessarily a laboratory) where ...
Causal research, sometimes referred to as explanatory research, is a type of study that evaluates whether two different situations have a cause-and-effect relationship. Since many alternative factors can contribute to cause-and-effect, researchers design experiments to collect statistical evidence of the connection between the situations.
Causal modeling requires the researcher to construct a model to explain the relationships among concepts related to a specific phenomenon ( Asher, 1983 ). A causal model is a diagram of the relationships between independent, control, and dependent variables. A path model is a less complex type of causal model.
Revised on June 22, 2023. Correlation means there is a statistical association between variables. Causation means that a change in one variable causes a change in another variable. In research, you might have come across the phrase "correlation doesn't imply causation.". Correlation and causation are two related ideas, but understanding ...
Let us distinguish two different ways to ask and address causal questions. One can begin with an outcome, i.e., Y, and then work backward to the causes, i.e., X s. The second option works in the other direction; one starts with a potential cause and then asks about its impact on Y.The former procedure is often called the "causes-of-effects" approach, whereas the latter is known as the ...
This type of research will recognize trends and patterns in data, but it does not go so far in its analysis to prove causes for these observed patterns. Cause and effect is not the basis of this type of observational research. The data, relationships, and distributions of variables are studied only. Variables are not manipulated; they are only ...
The main method for cause-effect research is experimentation. In experimental-based research, we manipulate the causal or independent variables in a relatively controlled environment. This means that we control and monitor other variables affecting the dependent variable (e.g. sales) as much as possible.
This method is designed to establish cause-and-effect relationships. Fields like psychology, medicine, and social sciences frequently employ experimental research to test hypotheses and theories under controlled conditions. ... Diagnostic research tries to accurately diagnose a problem by identifying its underlying causes. This type of research ...
Generating power. Generating electricity and heat by burning fossil fuels causes a large chunk of global emissions. Most electricity is still generated by burning coal, oil, or gas, which produces ...
Probiotics are live microorganisms that are intended to have health benefits when consumed or applied to the body. They can be found in yogurt and other fermented foods, dietary supplements, and beauty products. Cases of severe or fatal infections have been reported in premature infants who were given probiotics, and the U.S. Food and Drug Administration (FDA) has warned health care providers ...
Causes. Motor vehicle accidents are the leading cause of SCI in the U. S. in younger individuals, while falls are the leading cause for SCI for people over 65. Acts of violence and sports/recreation activities are other common causes for these injuries.Just over half of all SCI occurs in persons age 16-30, most of whom are male (80%).
Learn up-to-date facts and statistics on alcohol consumption and its impact in the United States and globally. Explore topics related to alcohol misuse and treatment, underage drinking, the effects of alcohol on the human body, and more.
Revised on January 22, 2024. Like a true experiment, a quasi-experimental design aims to establish a cause-and-effect relationship between an independent and dependent variable. However, unlike a true experiment, a quasi-experiment does not rely on random assignment. Instead, subjects are assigned to groups based on non-random criteria.
This type of hair loss is temporary. Hairstyles and treatments. Excessive hairstyling or hairstyles that pull your hair tight, such as pigtails or cornrows, can cause a type of hair loss called traction alopecia. Hot-oil hair treatments and permanents also can cause hair to fall out. If scarring occurs, hair loss could be permanent.
In developed countries, autoimmune disease causes 8 or 9 of every 10 cases of Addison's disease. 4. Certain infections can also cause Addison's disease. Tuberculosis (TB) can damage the adrenal glands and used to be the most common cause of Addison's disease. As treatment improved over the years, TB became a much less common cause.