
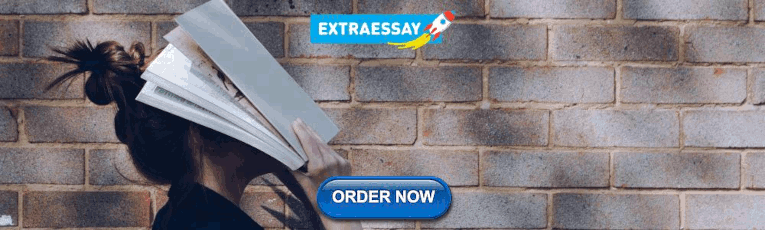
Cornell Research & Innovation
Extreme Talent. Radical Collaboration. Results That Matter.
From the laboratory bench to the hospital bedside and from cultural evolution to concepts in sustainability, our researchers and scholars are translating discovery into meaningful, measurable impact that is changing the world for the better.
Research Around the University
Study confirms frequency of undetected responsiveness in severe brain injury
Online crowds wield power, for good and bad
Sleep resets neurons for new memories the next day
Breakthrough addresses sex-related weight gain and disease
Summer program gives undergraduates a taste of research life
Smart food choices at family level can ease chronic illness
Timely responses – even from a car – drive babies’ learning
Manager visits heighten workers’ motivation, productivity
What went wrong in 2022 baby formula crisis?
Augustine was ‘wrong about slavery’: Book reexamines key figure

“I think none of us anticipated that this would turn into anything more than a class paper, but we all felt that there was something there.”

“Knowing that what I'm doing will actually help decrease carbon emissions and contribute to mitigating the climate change impacts is very rewarding.”

“Plastics like polyethylene and polypropylene are great materials; we use them for everything. But the problem is their strong properties, which make them so great for so many applications, also mean they don’t degrade…. We have to do something about that.”
Radical Collaboration
Multidisciplinary partnerships.
The challenges of the world and the big picture of what’s possible rarely fit neatly into a single academic discipline. This is why we’ve made collaboration and communication a way of life at Cornell. We foster a community of renowned scholars that spans disciplines, locations, and industries in order to convert promising ideas and innovations into results that truly matter.

Extreme Talent
The best & brightest faculty.
Talented researchers and top scholars know that teaching and learning are intrinsic to one another. That's why they come to Cornell. They know they can push the boundaries of discovery by engaging with students who, in turn, engage with and challenge them.

Michael Goldstein

Lisa Kaltenegger

Rick Johnson

Martha Field

Research Examples
Research support and funding.

Support for everything from proposal development to grant management—and all the administrative issues in between.
Centers and Institutes

More than 100 interdisciplinary research organizations bring students and faculty together to pursue research, teaching, and outreach.
Labs and Facilities
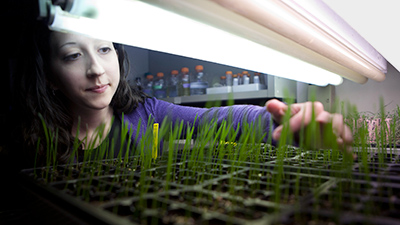
Millions of dollars of funding go into our labs every year. Hundreds of inventions, patents, licenses, copyrights, and plants emerge every year. Now we just need you.
Cornell Global Research Stories
Cornell research delivers results that matter all around the world.

Male lyrebirds snare mates with ‘acoustic illusion’
Researchers discover that Australia’s superb lyrebird males imitate the panicked alarm calls of a mixed-species flock of birds while they are courting and even while mating with a female.
Historic Alaskan Tlingit 1804 battle fort site found
Cornell and National Park Service researchers have pinpointed the exact location of a Tlingit fort in Sitka, Alaska used in 1804 to defend against Russian colonization forces.
Hold still, big cat: Vaccination could save Siberian tigers
New research out of the College of Veterinary Medicine has revealed that vaccination of endangered Siberian tigers is the only practical strategy to protect these big cats from potentially deadly canine distemper virus.
Study: European unions’ support varies for precarious workers
In many cases, unions in Europe have helped nonunionized workers whose jobs are precarious, according to new Cornell research.
Research Links
Research gateways.
- Research Centers, Institutes, Labs and Programs
- Undergraduate Research
- Graduate Study
Corporate Gateways
- Center for Technology Licensing at Cornell (CTL)
- Vice President for Research and Innovation
- Leadership and Contacts
- Office of Sponsored Programs
- Center for Animal Resources and Education
- Office of Research Integrity and Assurance
- Research Administration Information Services
- Affiliated Centers
- Publications and Data
Publications
- Cornell Chronicle
- Cornellians
Continue Exploring …
- Plan a Visit
- About Cornell
- Student Life
Stack Exchange Network
Stack Exchange network consists of 183 Q&A communities including Stack Overflow , the largest, most trusted online community for developers to learn, share their knowledge, and build their careers.
Q&A for work
Connect and share knowledge within a single location that is structured and easy to search.
Binomial Distribution about ties
A research team at Cornell University conducted a study showing that approximately 10% of all businessmen who wear ties wear them so tight that they actually reduce blood flow to the brain, diminishing cerebral functions. At a board meeting of 20 businessmen, all of whom wear ties, what is the probability that a)at least one tie is too tight b)no more that two ties are too tight c)no ties are too tight d)at least 18 ties are too tight
- binomial-theorem

- $\begingroup$ en.wikipedia.org/wiki/Binomial_distribution $\endgroup$ – vadim123 Commented Apr 14, 2014 at 16:31
- $\begingroup$ Upvoted for making me smile. $\endgroup$ – André Nicolas Commented Apr 14, 2014 at 16:39
You must log in to answer this question.
Browse other questions tagged binomial-theorem ..
- Featured on Meta
- We've made changes to our Terms of Service & Privacy Policy - July 2024
- Bringing clarity to status tag usage on meta sites
- 2024 Community Moderator Election
- 2024 Election Results: Congratulations to our new moderator!
Hot Network Questions
- How would Earth's plants change in this speculated Earth-like planet?
- Postdoc supervisor has stopped helping
- What is the reason for 29.9kW PV inverters, when there are 30kW rated ones?
- What sort of impact did the discovery that water could be broken down (via electrolysis) into gas?
- Does the overall mAh of the battery add up when batteries are parallel?
- How does a 0-conf signature bond work?
- How to find intersecting linear equations between two lists?
- How to display 3 horizontal nested tabs on mobile device
- Can objective morality be derived as a corollary from the assumption of God's existence?
- How is Nationality Principle applied in practice?
- How to raise a vector to powers contained in a vector, change the list into a product, and do this for all the lines of a matrix, efficiently?
- Is sudoku only one puzzle?
- Uniqueness of units in group objects
- How do closed cycle liquid engines actually work?
- What prevents applications from misusing private keys?
- Do linguists have a noun for referring to pieces of commendatory language, as a sort of antonym of 'pejoratives'?
- What might cause these striations in this solder joint?
- The Reforger NPC is stuck underground. How do I get him to move?
- Why did Worf respond when Picard ordered the Enterprise out of the asteroid in TNG: The Pegasus?
- The McDonald's Option
- Consistency strength of HoTT
- C - mini string lib
- Drawing an arc on a rectangle
- Why are swimming goggles typically made from a different material than diving masks?
Research & Innovation
Office of the vice president for research & innovation (ovpri), generative ai in academic research: perspectives and cultural norms.
Download the full report as a PDF: Generative AI in Academic Research: Perspectives and Cultural Norms ( PDF)
- Executive Summary
- Introduction
- Framework for Using Generative AI in Research
- GenAI for Literature Review
- GenAI for Research Infrastructure
- GenAI for Data Collection and Generation
- GenAI for Ideation & Hypothesis Generation
B. Research Dissemination Stage
C. research translation stage, d. research funding & funding agreement compliance stage, considerations for the cornell research community.
- Considerations for Cornell Leadership
- A. Research Ideation and Execution Stage
D. Research Funding and Funding Agreement Compliance Stage
- Appendix 1. Existing Community Publication Policies
- Appendix 2. References Consulted or Cited
- Appendix 3. Task Force Charge
E xecutive Summary
This report offers perspectives and practical guidelines to the Cornell community, specifically on the use of Generative Artificial Intelligence (GenAI) in the practice and dissemination of academic research. As emphasized in the charge to a Cornell task force representing input across all campuses, the report aims to establish the initial set of perspectives and cultural norms for Cornell researchers, research team leaders, and research administration staff. It is meant as internal advice rather than a set of binding rules. As GenAI policies and guardrails are rapidly evolving, we stress the importance of staying current with the latest developments, and updating procedures and rules governing the use of GenAI tools in research thoughtfully over time. This report was developed within the same 12-month period that GenAI became available to a much wider number of researchers (and citizens) than AI specialists who help create such tools. While the Cornell community is the intended audience, this report is publicly available as a resource for other research communities to use or adapt. No endorsement of specific tools is implied, but specific examples are referenced to illustrate concepts.
Recognizing many potential benefits and risks of GenAI tools, we address the use of GenAI at four stages of the research process: (i) research conception and execution stage, (ii) research dissemination stage, (iii) research translation stage, and (iv) research funding and funding agreement compliance stage. We further outline coordinating duties by researchers that apply across these stages: duty of discretion, duty of verification, and duty of disclosure; identify categories of GenAI use in research; and illustrate how these duties apply to specific categories and situations in the research process. We emphasize the importance of clearly defining individual and collective/communal responsibilities for meeting these duties throughout the research process. We conclude by offering a set of guidelines for Cornell researchers in varied faculty, staff, and student roles, as well as considerations for Cornell leadership. It is important that Cornell offers its research community access to appropriate GenAI tools and resources, particularly to improve our “AI literacy” regarding the limits of the appropriate use of specific public and commercial GenAI tools and the risks involved in their use for academic research. It is equally important that researchers have Cornell-facilitated access to licensed GenAI tools with privacy/confidentiality provisions, and thus important that Cornell researchers from varied communities understand the value, limitations, and trade-offs of using such tools in research.
The report also contains responses to anticipated questions about best practices and use cases for each of the four stages of research (Appendix 0) that may serve as discussion starters for research communities. Finally, we offer a summary of existing community publication policies regarding the use of GenAI in research from funders, journals, professional societies, and peers, which we surveyed as part of the preparation of this report (Appendix 1); references consulted and cited that include a list of recommended resources (Appendix 2); and task force charge (Appendix 3). Notably, the task force included Cornell faculty and staff quite familiar with GenAI tools and uses, and the task force elected to not use GenAI in drafting the structure, text, or figures of this report.
I ntroduction
Generative Artificial Intelligence (GenAI) offers transformative capabilities, but we must strike a balance between exploring the potential of these tools and ensuring that research meets standards of veracity, validity, originality, and reproducibility. Briefly, GenAI has the capacity to generate new content (new text or images or audio), typically by computer-generated pattern recognition gleaned from access to large volumes of prior examples. These prior examples, data collectively called training sets or training data, can be provided by the GenAI user or provided by others, with or without their explicit awareness or consent.
This exciting capability to spark new ideas from prior knowledge, perhaps now connected in unexpected new ways, can now be accessed by the masses via online tools and for-fee apps. These users include the masses of academic researchers with a shared sense of research integrity, but with widely varied experience in computer programming and in cultural norms for creation, authorship, and invention. Many of these tools, whether “free to the user” or fee-based, have been released by for-profit companies that maintain the model details as proprietary (i.e., do not disclose details of the trained models or the training data sets that serve as the basis for the GenAI output). Open-source approaches for GenAI development can be a counterpoint that provides a more transparent toolset, but are not an automatic panacea to responsible development or use of such tools in academic research. Thus, we need to develop common ground and guardrails that prioritize research integrity, accelerate innovation, address obvious issues like data privacy and security, and reflect on non-obvious issues like how practices and expertise of research communities will evolve for better and worse. No one policy can cover the range of research carried out at a university, from archives to surveys to experimental labs to pure math and the visual arts. GenAI capabilities and affordances are also changing from month to month, only a year into publicly available and initially free versions, but for the cost of providing your own email address or mobile phone number to a for-profit company. External to Cornell, GenAI policies and guardrails are rapidly evolving, and procedures and rules governing the use of GenAI tools in the research enterprise should be regularly updated to stay current with the latest developments. Internal to Cornell, aligning practices for GenAI use with existing policies on research data and with our institutional values will also remain a work in progress for years to come.
GenAI has many potential benefits for researchers at all stages of their research career, for administrative staff who provide key support to but do not participate in research and translation activities directly, and for other users in the course of conducting and administering research. These include:
- Abstractions. Many systems for data analysis and document retrieval have been available only to those with substantial programming experience. Likewise, systems for creative audiovisual generation have been limited to specialists or those with years of content production experience. GenAI tools can provide powerful results with interfaces accessible to anyone.
- Efficiency. Even when researchers, administrative staff, and other users have the capability to perform a task, GenAI may be able to produce comparable output in dramatically less time, allowing users to focus on more difficult, human interaction-intensive, and/or rewarding tasks.
- Scale . GenAI may allow users to perform a task such as coding/annotating documents or generating infographic images on a larger scale than would be possible with manual effort. This ability may allow users to explore larger or broader data sets that were previously limited.
At the same time, we have identified several concerns that apply across the wide range of research disciplines at Cornell and represented internationally. These include:
- Blind spots and potential bias. All current generative language models are entirely defined by their training data, and thus perpetuate the omissions and biases of that training data. The model behind ChatGPT has no access to information that was not presented during training, and can only access that information through learned combinations of parameters. There is no explicit and verifiable representation of data or text encoded in the model. Other GenAI systems, such as GPT4, may increasingly have access to web searches and databases to retrieve verifiable sources, and the underlying language model may be able to interact with text returned from those searches (in the same way it interacts with user queries), but the model itself still has no “knowledge” beyond the statistical regularities of its training data.
- Validation and responsibility. There is a risk that systems are good enough that users will become trusting and complacent, but bad enough that there are serious problems that have profound consequences. A system that produces seemingly plausible answers, yet is prone to false and biased information, can cause researchers to lower their guard. Therefore, we emphasize the crucial role of researcher validation of research outputs produced with the help of GenAI tools. Responsibility is an area that is particularly sensitive to discipline-specific variation. Most fields have tacit understandings of roles and responsibilities (e.g., principal investigator, Ph.D. student, corresponding author), which may differ substantially from those in even closely related fields.
- Transparency and documentation. Guidelines for the use of GenAI in research vary greatly across journals, funding agencies, and professional associations, from blanket bans to restrictions on certain outputs to permissions with disclosure (most with the emphasis that AI-generated outputs should be original, i.e., reflecting the authors’ actual work). Laws and regulations for patents, copyright and data use are evolving and vary among countries. As the policies regarding the use of GenAI continue to evolve, maintaining documentation and reporting transparency will remain critical to ensure the reproducibility and replicability of research findings produced with the help of GenAI tools.
- Data privacy and protection. GenAI tools should not be assumed a priori to be private or secure. Users must understand the potential risks associated with inputting sensitive, private, confidential, or proprietary data into these tools, and that doing so may violate legal or contractual requirements, or expectations for privacy.
- Resource utilization tradeoffs. Because GenAI users perceive the output of such tools to be a “free good” or at least generated “in the cloud” even when user fee-based, as with research travel impacts the resource utilization of this computational output can be out of sight and out of mind. However, the magnitude of computational resources operating on Earth to create models based on large volumes of training data, and the electricity use and potential cooling water use associated with such computational processing, can be in tension with values associated with sustainability. A recent study posted on arXiv and currently under peer review attempts to quantify this resource tradeoff in terms of carbon dioxide emissions, equating the carbon cost for you to generate a single image using a specific energy-intensive GenAI model to that required to fully charge your mobile phone (Luccioni et al., 2023 preprint). (CITE: https://arxiv.org/pdf/2311.16863.pdf ). Certainly, other research-related activities may contribute more significantly on a per-instance basis (e.g., a research group flying to present at an overseas conference). However, Cornell’s public commitment to climate action and our individual sense of responsibility for our own resource use choices benefit from our shared awareness that the use phase (or inference phase) of GenAI can be estimated, is non-zero and will remain so without concerted effort, and naturally scales with access to computational resources.
F ramework for Using Generative AI in Research
The epochal developments of the past five years in GenAI have enabled systems to generate complex, recognizable outputs such as text, programming code, images, and voices. AI as a field has been around for decades, but the output of systems has often been narrow, binary predictions: whether an email is spam, or whether a transaction is likely to be fraudulent. GenAI offers dramatic
new capabilities, generating output in response to prompts (i.e., questions, requests, instructions) from the user. See inset: What are generative uses of AI?
GenAI provides the user a sense of power in its apparent intellectual assistance on demand, which unsurprisingly also vests the user with a need to take responsibility. Academic research groups and projects often include multiple users with different stages of contribution, different degrees of experience and leadership, and different responsibilities to research integrity and translation of research results to societal impact. Thus, we begin with the following general framework describing categories of uses of GenAI in research and categories of duties that researchers may have.
There are many levels of potential uses in research, ranging from surface level adjustments to applications that blur the boundary of authorship. At one extreme, we might consider systems that simulate a copyeditor, correcting spelling or grammar, which are already integrated in many word processing systems. At the other extreme might be a system that acts as a ghost writer, converting vague descriptions and specifications into polished final presentations. In between, systems might act more like research assistants, collecting and collating information, writing short computer programs, or filling in paragraph bodies from thesis statements. Other uses might be more like reviewers or editors, enabling researchers to “bounce ideas” or summarize a passage of text to ensure that it reads correctly.
These uses imply corresponding duties by researchers. Most high-performance GenAI systems are currently available as third-party (i.e., company product, not university-managed resource) cloud (i.e., using remotely located computers) applications, so there is a researcher duty of discretion in what data should be uploaded. GenAI, while usually convincing and fluent (at least in English), is often factually incorrect or lacking in attribution, so verification is another key duty to ensure accuracy and validity of research outputs. Researchers may also have a duty to provide transparency and disclosure to identify how and where GenAI contributed. Finally, we need clear lines of individual and collective responsibility to ensure that the other duties are actually executed.
For the remainder of this report we will identify specific situations in the research process, and describe how they relate to these categories of use and what duties we believe apply in academic research – and are consistent with Cornell shared values. In all of these research stages, we consider GenAI to be a useful research tool that can and should be explored and used to great scholarly advantage. As with all tools, the user is responsible for understanding how to use such tools wisely. As with all academic research, the responsibilities are shared, but the research leader – called principal investigator in some fields and contexts, and lead author, corresponding author, or lead inventor in others – is considered responsible for communicating expectations to their research colleagues and students, and ultimately bearing consequences of intentional or incidental errors in tool use.
G enerative AI Use across Research Stages
We considered four stages of research, each of which may receive different emphasis among Cornell’s impressive breadth of research and scholarship areas. Figure 1 illustrates these four stages where GenAI can be used to great advantage, with appropriate sense of duty by the researcher(s).
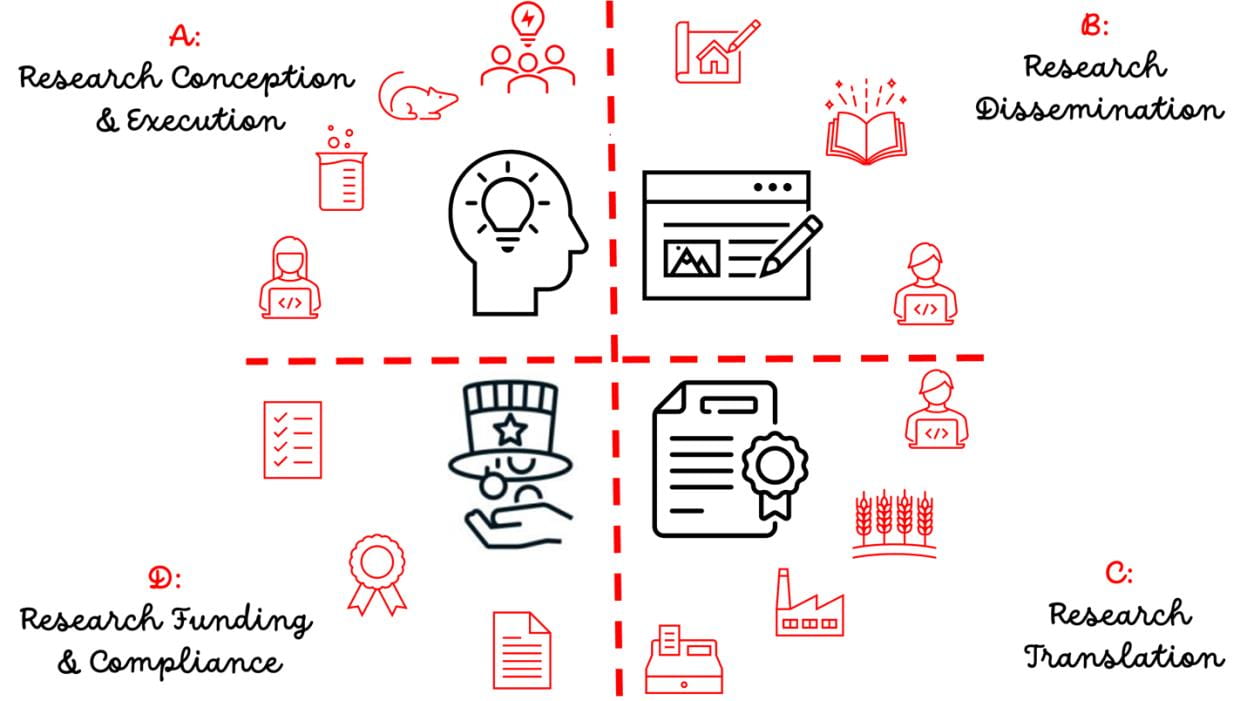
These four stages that can be considered in the life cycle of research include:
- Research Conception and Execution Stage: Includes ideation by the individual and research team, prior to any public dissemination of ideas or research results.
- Research Dissemination Stage: Includes public sharing of research ideas and results, including peer-reviewed journal publications, manuscripts and books, and other creative works.
- Research Translation Stage: Includes reducing research findings or results to practice, which may be in the form of patented inventions or copyrights, for products or processes or policies.
- Research Funding and Funding Agreement Compliance Stage: Includes proposals seeking funding of research plans, as well as compliance with expectations of sponsors or the US government policies relevant to Cornell as an institution of higher education and a research university.
A. Research Conception and Execution Stage
In this section we discuss uses of GenAI for the “internal” research process prior to the preparation of public documents. Research conception and execution includes literature review, research infrastructure, research ideation, and hypothesis generation.
G enAI for Literature Review
The volume of published research literature and data have been expanding exponentially, accelerating with technology advances such as movable type and publishing changes such as electronic journal proliferation and public databases. It is widely assumed that there are pockets of information in distinct fields that, if combined, could lead to breakthroughs. But despite the volume of published information, those serendipitous connections are infrequent because fields are mutually inaccessible due to technical language, and no one from either field knows to look for the other (e.g., epidemiologists and aerosol physicists). In fact, interdisciplinary research often espouses the mixing of existing information in new ways, implying that GenAI systems which can keep track of vastly more information than any person may find connections that might be missed entirely by humans. Acting as “state-of-the-art information retrieval” systems (Extance, 2018), they go beyond conventional databases, such as Google Scholar and PubMed, by being able to retrieve, synthesize, visualize, and summarize massive amounts of existing knowledge (e.g., Semantic Scholar, Scopus AI, Microsoft Academic, Iris.ai, Scite, Consensus). As such, they have the potential to help overcome the problem of “undiscovered public knowledge” (Swanson, 1986; Davies, 1989), which may exist within the published literature, and break through disciplinary silos, facilitating the discovery of relevant research across diverse academic disciplines.
In this context, suggested practices for using GenAI in the literature review phase of research conception are that:
- GenAI can be used to triage, organize, summarize, and quickly get directionally oriented, in the context of an exponentially growing base of reported claims and established knowledge.
- GenAI can be used to assist with drafting literature reviews, although researchers should fact-check and be aware of incomplete, biased, or even false GenAI outputs. In some cases, it can help to provide GenAI with explicit prompt text to try to guard against the use of fake references (e.g., Dowling & Lucey, 2023), although it still does not guarantee accurate results.
- Subject to authorship, citation, and fact-checking considerations, it can be helpful to use GenAI to ideate and iterate on the quality of a literature review. Examples include (a) refining the review to include both prior research and its connection to the new research idea, (b) rewriting the style of the literature review, and (c) refining the literature review to emphasize the contribution of the new research, such as is relevant to other gaps in literature, uncertainties, or even market sentiments.
Duty of verification . The reliability and quality of AI-powered literature review tools are limited by the databases they search, which can affect the comprehensiveness and accuracy of the results. Therefore, it is advisable to use these tools in conjunction with other methods. Another major concern when using these tools is plagiarism, as they can produce verbatim copies of existing work without proper attribution or introduce ideas and results from actual published work but provide incorrect or missing citations. To minimize the risk of unintentional plagiarism, it is best to start with original text and then use GenAI assistance to refine it, in line with the distinction between AI-assisted and AI-written text (van Dis, Bollen, Zuidema et al., 2023). This will help ensure that AI-generated text is original and reflects the authors’ own work, as also emphasized in journals and professional societies that permit the use of GenAI tools (but note that some journals prohibit the use of GenAI for any part of the research process entirely; see Appendix 1 for a summary of existing community publication policies, noting that such policies are subject to change by those communities and publishers). Finally, depending on the extent of GenAI assistance with information search and literature review production (specifically, when it is used beyond grammatical polishing of authorwritten text), researchers may have a duty of disclosure for this research stage.
G enAI for Research Infrastructure
One of the more benign possible uses of AI is in improving workflows and research processes. Collecting and processing data often involve custom software, using complicated APIs (application programming interface, or software with a specific function) that may be poorly documented. Code generation tools such as Copilot have become powerful and successful, leading to significant improvements in users’ ability to create software to collate and analyze data. Other ways might involve using GenAI to help construct or critique survey questions or interview templates. In each case, the AI is not involved in producing or recording data, but in building the infrastructure that is itself used to produce data. A second category of infrastructure might include code or language generation for presentation of research results. APIs for generating figures, such as matplotlib or ggplot2, are notoriously complicated, with innumerable options for modifying the appearance and layout of graphics. Code generation may help in producing programs to generate graphics from data sets, without being directly involved in the construction of data sets themselves. Similarly, language models might assist in generating alt-text for image accessibility. Duty of verification. As with any other use of GenAI, infrastructure-building uses require careful checking to ensure that outputs are correct. There should be clear responsibility for who will do this verification. We see less need to disclose the use of GenAI in these “back office” contexts relative to other uses, though still with care for potential implications at later research stages.
G enAI for Data Collection and Generation
A subtle but important distinction is when we move from using GenAI to help develop tools that we use in research to using GenAI as a tool for research, specifically for data collection and generation. In principle, the potential for data collection is enormous. GenAI can be used to help construct data sets from unstructured data, such as descriptions of patents, job vacancies, SEC (US Security and Exchange Commission) filings, banker speeches, etc. GenAI tools can also be used to synthesize information coming from text, or images. They can be employed to self-assess (predict accuracy) and augment which coding tasks are conducted by human iteration. Advantages for data collection and generation using GenAI as a tool for research include:
- Collecting and organizing data. Consider the Cornell Lab of Ornithology’s example of eBird as one data-rich source: Through this global application platform, birdwatchers have submitted a large amount of bird observations that have already informed development of species distribution models (Sullivan et al., 2014).
- Generating data out of unstructured information.
- Summarizing data coming from various sources. Data related to human clinical trials or patient outcomes hold different and important data privacy concerns, but the collection and organization/cleaning of such data is a key step in inference for patient-centered health outcomes (Waitman et al., 2023).
- Scaling up data collection with GenAI by conducting faster and less resource-intensive experiments.
The challenges of using GenAI tools for data collection and generation primarily relate to the duties of verification and disclosure :
- Issues with performance and accuracy: Large language models like ChatGPT are currently not fundamentally trained to speak accurately or stay faithful to some ground truth.
- The reliance on large amounts of data may be challenging, and the needed data may not always be available.
- Bias (King and Zenil, 2023): AI is traditionally trained on data that has been processed by humans. Example: In using ML to categorize different types of astronomical images, humans might need to feed the system with a series of images they have already categorized and labeled. This would allow the system to learn the differences between the images. However, those doing the labeling might have different levels of competence, make mistakes and so on. GenAI could be used to detect and to some extent redress such biases.
- Attribution: Data sources may not always be tracked. There is a need for ensuring correct attribution of data sources.
Given these challenges, the use of GenAI tools for data generation and collection must be carefully documented and disclosed to facilitate research assessment, transparency, and reproducibility.
G enAI for Ideation & Hypothesis Generation
While the use of GenAI for idea generation is under early consideration by most academic researchers, it is important to weigh its strengths and weaknesses in the early phases of research. If we think of the idea generation process as a creative process (as opposed to fact-checking or verification), then complementing ideation with GenAI can potentially offset human weaknesses, such as comparatively poorer memory recall versus recognition processes and narrower breadth of knowledge bases. In this sense, GenAI can complement individual researchers during the idea generation process and democratize access to research assistants. On the other hand, scientific knowledge relies on the ability to reason rationally, do abstract modeling and make logical inferences. However, these abilities are handled poorly by statistical machine learning (ML). Humans do not need a large amount of data or observations to generate a hypothesis, while statistical ML relies on vast amounts of data. As a consequence, computers are still unable to formulate impactful research questions, design proper experiments, and understand and describe their limitations.
Furthermore, assessing the scientific value of a hypothesis requires in-depth, domain-specific, and subject-matter expertise. An example is the potential of “Language Based Discovery” (LBD) as the possibility to create entirely new, plausible and scientifically non-trivial hypotheses by combining findings or assertions across multiple documents. If one article asserts that “A affects B” and another that “B affects C,” then “A affects C” is a natural hypothesis. The challenge is for LBD to identify which assertions of the type “A affects C” are novel, scientifically plausible, non-trivial and sufficiently interesting that a scientist would find them worthy of study (Smalheiser et al. 2023). Whereas GenAI does well in identifying and retrieving potential data constructs, researcher domain expertise remains critical for determining the quality of output (Dowling and Lucey, 2023).
While considering the possibilities of GenAI-human collaboration for research ideation, it is essential to emphasize the duty of discretion to prevent the leakage of proprietary, sensitive, and confidential information into public information space. Furthermore, since using GenAI tools is an evolving space, academics should learn more about GenAI technologies and stay abreast of potentially useful ways for hypothesis generation. For example, as food for thought, one of the GenAI prompts used by Dowling and Lucey (2023) for idea generation: “You [the GenAI tool] created this research idea, and I’d like you to improve it. Could you see if there is an additional article that can be added, to improve the research idea. Can you also talk about the novel contribution of the idea. Please keep it to about 100 words.” Moreover, as we humans gain experience and familiarity with new tools, we do well to be observant to the expectation that they can also change how we conduct research and interact with researchers at this ideation and hypothesis generation stage – in ways that are not always easy to identify a priori.
Finally, we note that research execution includes expectations of responsible conduct of research, which for some studies and disciplines includes prior approval of data use and management, animal welfare and procedures, and human subjects. Use of GenAI in research will likely augment considerations of these approvals per expectations of sponsors or federal agencies through research integrity review processes of the university. Those considerations related to research compliance are expanded in Section D. Next, we consider the stage where research of any type is disseminated through public disclosure including peer-reviewed publications.
GenAI offers new affordances that support both positive and negative outcomes for research dissemination (Nordling, 2003). On the positive side there is the potential to level the playing field for non-native speakers of English; to provide writing assistance resulting in improved clarity; and for new tools that aid in more equitable discovery of related work (improving on common practices of searching for well-known authors, for example). On the negative side there are serious and reasonable concerns such as erroneous information being disseminated because of inadequate verification; easier plagiarism (either intentional or accidental); lack of appropriate attribution because current LLM-based tools are unable to indicate the source of information; bias and ideological influence; and inappropriate use of GenAI as a lazy peer review tool. Additionally, we must be aware that careless use of GenAI may entrench biases in scholarly communication and dissemination, such as reinforcing the positions of prominent scholars and preferring sources in English as the dominant language of the initial trained models available to the general public. However, future GenAI tools may also provide new interventions to oppose existing biases that are entrenched in current practice. As such, the following is less focused on specific GenAI tools available today, but more on general recommendations for the responsible use of GenAI tools in research dissemination that upholds research integrity as a principal value at Cornell.
In this section and research stage, we do not discuss questions of copyright, confidentiality or intellectual property (see Section C), but instead focus on the conceptual impact that GenAI can have on producing research output. Following from the definition of GenAI from above, a key distinction is whether the tool produces output for dissemination that contains concepts and interpretations that the author did not supply . From this perspective, GenAI tools that fill in concepts or interpretations for the authors can fundamentally change the research methodology, they can provide authors with “shortcuts” that lead to a breakdown of rigor, and they can introduce bias. This makes it imperative that users of GenAI tools are well aware of the limitations of these tools, and that it is clear who is responsible for the integrity of the research and the output that is produced.
Below we discuss these issues in more detail and provide a minimal set of norms that we recommend across all disciplines. However, we recognize that the methodology and standards are differentially impacted by GenAI across disciplines (e.g., humanities as well as engineering), and that community norms around the use of GenAI may be stricter than what is outlined below.
Authorship : We posit that GenAI tools do not deserve author credit and cannot take on author responsibility. This means that authors of research outputs, not any GenAI tools used in the process, carry the responsibility for checking the correctness of any statements. Authors must be aware that GenAI tools can and do produce erroneous results including “hallucinated” citations. The content will be viewed as statements made by the authors. Indeed, there are emerging concerns on impact to scientific publishing with which publishers and AI ethicists are now grappling (Conroy 2023), but the responsibility of authentic authorship is a component of research integrity that will continue to rest with the human authors.
Impact on Concepts and Interpretations : Researchers need to be aware that GenAI tools can have a substantial impact on the research output depending on how they are used to fill in concepts and add interpretations. If the impact is substantial, we recommend that the use of GenAI is disclosed and detailed so that readers are aware of its potential impact. What constitutes substantial impact depends on the type of publication (e.g., journal articles, books, talks, reports, reviews, research proposals) and community norms in the respective discipline. An example that is probably considered to have a substantial impact in any discipline is the use of GenAI to draft a related work section.
Impact on Methodology : Writing and other dissemination activities typically cannot be separated from conducting the research, and the act of writing is explicitly part of the research methodology in some disciplines. A key concern is that the use of GenAI as a “shortcut” can lead to a degradation of methodological rigor. If the use of GenAI tools can be viewed as part of the research methodology, then we recommend disclosure so that readers can assess the rigor of the methodology. Indeed, there may be collective impact on methodology at the scale of the research community’s practices. Whether GenAI becomes a tool that sharpens our minds or a blunt instrument that dulls them is a question that Cornell (and other communities of research scholars) must address actively over time. Historically, human imagination sees most tools as helpful implements to move on to harder problems and more creative discovery and analysis, if one masters the tool instead of the other way around. But we can also recognize from past experiences that zeal for rapid development of exciting new research-enabling capabilities – especially when these provide competitive advantage over peers that can relate to economic competition or even national security – can shift even the best intentioned individuals to start to behave collectively as a group that focuses sharply on the benefits without openly discussing the costs and trade-offs.
Potential for Bias : Just as authors need to be aware of human biases in their sources, authors using GenAI tools need to be aware that these tools have biases and may reflect specific ideologies. It is the authors’ responsibility to counteract or avoid these biases, and authors cannot offload responsibility for bias in their work on the AI system. For example, use of a GenAI tool to create a hospital scene might result in an image in which the nurses are female and the doctors are male. Changing the prompt could address this bias. Another issue is that GenAI tools may reflect a particular ideology, or they may perpetuate historical biases because GenAI tools are trained on historical data. This may be compounded by particular algorithms such as citation analysis which has an inherent time lag, and might further bias recommendations back in time or towards a dominant group or language.
Acceptable Use : There are many different forms and venues of research dissemination: journal articles, books, talks, reports, reviews, research proposals, etc. What is acceptable use of GenAI in one form of communication is not necessarily acceptable in other forms, and authors must adhere to community standards. To take an extreme example, having a GenAI tool draft a peer review from scratch runs counter to the idea of peer review and has an extremely high impact on the review, even if the author checks and edits the review. This is likely unacceptable in most communities. Even within communities, different publication venues (e.g., journal, conference) may have different policies, and authors must check and follow these if more stringent than what is outlined here.
AI Literacy to support Research Integrity : Rigorous and ambitious use of GenAI tools requires a good understanding of the strengths and weaknesses of these tools. Furthermore, as GenAI has become part of the integrity of research and its dissemination, then research leaders such as principal investigators and faculty supervising student research should now make the appropriate use of GenAI part of their mentoring. In particular, part of their mentoring is to communicate the standards and the norms in their specific fields to the researchers and students they lead – just as they mentor on other topics of research conduct (e.g., plagiarism, co-authorship, privacy regulations).
Regulations : Any use of GenAI tools needs to be compliant with regulations (e.g., copyright law, privacy regulations such as HIPAA and FERPA, confidentiality agreements, and intellectual property). In particular, users must be aware that use of GenAI tools may disclose sensitive information to a third party, which may be in violation of regulations and confidentiality norms (Lauer et al. 2023; Conroy 2023). This extension to implications for subsequent research translation to policies, processes, and products of all types is discussed further in Section C.
C . Research Translation Stage
The use of GenAI in any stage of the research process may impact the translation, protection and licensing of intellectual property (IP), commercialization of technology, open-source release of software and other uses of the research output downstream. Interpretations of laws and new regulations regarding GenAI are major topics for governments in many countries including the US. There may be new government agencies and international organizations created for AI regulation and coordination in the near future. In fact, while the European Union recently announced new regulations on artificial intelligence, current understanding is that most of this EU policy effort to create these “first rules” preceded widespread use of GenAI (European Union Commission 2023).
We can draw no immediate conclusions on how the EU’s risk-based approach may impact GenAI development and uses specifically. The nature of the impact of GenAI is still evolving and may change in coming years, with legislation and guidelines expected to lag the use of GenAI and its shorter term implications, which may be inadequately addressed under current laws and regulations. The following are important areas for researchers to consider for translation when they use GenAI in their research process:
Inventorship and Patentability: Recent US case law has held that inventors of patents must be human beings under the US Patent Act. Documentation of human contribution and disclosure of the nature of GenAI utilization are essential for patent eligibility. Key information needs to be carefully documented, such as:
- Specific GenAI tools used and rationale for their use;
- Detailed input into and output of the GenAI tool;
- Whether the outputs lead to any aspects of the conception of the invention;
- Contributions of individual inventors in the inventive idea, and how they directed and refined the GenAI output; and
- For research done in teams, delineate the role of GenAI for each inventor.
Copyright and Authorship : Under current US copyright law, copyright can protect only material that is the product of human creativity and authors of copyrighted materials must be human beings. When incorporating GenAI-generated content, the authors should:
- Clearly document the boundary between human-created and GenAI-created content with clear annotations.
- According to guidance published by US Copyright Office, if copyright registration is sought, the nature and extent of the use of GenAI, if containing more than de minimis AI-generated material, must be disclosed with clarifications of what part of the work was created by the researchers and what part of the work was created by the GenAI.
- Specifically for computational algorithms and code, where research code can be further translated to wider use through copyright and various licensing types including open-source licensing, considerations attach at a time of active discussion. We note emerging considerations of copyright infringement, not only for creative works such as songs but also for computational code. For example, it is possible that code generated by a LLM reflects code reproduced verbatim from the LLM training set unbeknownst to the user. When such code is part of a research outcome that may be made available to licensees (even open-source licensees), it is possible but not yet well understood how use of such code, even when unintentionally plagiarized from other original sources, may violate copyright or invalidate licenses.
Commercialization and Fair Use : For research that leads to commercialization and publications with financial benefits, to mitigate risks of potential infringement claims, the inventors and authors should:
- Prioritize the use of GenAI tools that are trained using only public domain works, which is a small but growing area of development. For example, the recently announced AI Alliance coalition that includes Cornell as a founding member and anchored by two for-profit companies, IBM and Meta, advocates for development of open-source software tools including those enabling GenAI ( https://thealliance.ai/ ; Lin, B., 2023).
- Understand that the commercial intent can significantly impact fair use considerations. Consult with relevant university offices, such as the Center for Technology Licensing or General Counsel’s office, when there are questions.
- Stay informed of ongoing litigation that may influence the use of copyrighted materials in GenAI training data set. There are pending class action copyright suits by authors against entities owning GenAI tools for training without compensation to the authors.
Data Privacy and Protection : For data that researchers enter into GenAI themselves, it is important that researchers follow Cornell Policy 4.21 on Research Data Retention. Note that this is an existing policy and practice, simply extended to GenAI. Private, confidential, or proprietary data owned or controlled by Cornell may have certain contractual or legal restrictions or limitations, such as those to sponsors or collaborators, that would preclude their use in GenAI research projects, and it is a researcher’s responsibility to verify/determine whether any such data sets have restrictions on such use before inputting them into public-facing GenAI and ensuring compliance with any restrictions mandated by contract, law, or governing body (e.g., IRB, IAUCC). Any use of patient or human derived data should be disclosed to such governing body during the approval process and any such data set should only be used in research projects upon the explicit approval of the relevant governing body on campus.
Training : Specific to this stage of research translation, it is recommended that the university provide ongoing workshops on campus or through online platforms, and offer training materials through websites and other distribution channels, on topics related to the use of GenAI and its impact on patent rights, copyrighted materials, commercialization, open-source release and other uses to aid the researchers in understanding their rights, obligations, best practices and landscapes of relevant laws and regulations. Indeed, Cornell includes faculty and staff experts that can facilitate and co-develop such resources as part of their scholarly practice.
During the research funding and funding-agreement compliance stage, there are many potential applications of GenAI. For example, these tools can be leveraged to assist in the writing of technical, science-related information for a proposal to a sponsor or a donor, such as the technical scope and anticipated impact. On the non-technical side, they can also be used to draw appropriate data from multiple data sources to develop information for a biosketch, a report of Current and Pending Support, and other documentation relevant at this stage of the research process.
Work conducted during the Research Funding and Funding Agreement Compliance stage is poised to benefit from the use of GenAI tools, for example, due to efficiency improvements and reductions in the time taken to produce previously time-consuming work. However, the use of these tools also comes with risks. GenAI may produce outputs that include incorrect or incomplete information. These tools also may lack sufficient security and privacy protections, both in the tools themselves, and in any plug-ins and add-ons to the tools.
Note that we and many federal agency sponsors refer to the person of primary responsibility in research as the PI , or principal investigator, and pronounced pee-I, for shorthand. We acknowledge that this term is common for research in the sciences and engineering with cultures of team-based research and that other fields have a tradition of independent scholarship and authorship even when enrolled as graduate students.
Responsibility: As with the earlier stages of research, users of GenAI hold some burden of responsibility (or duty ) in the Research Funding and Funding Agreement Compliance stage. In this stage, however, it is common to attach the primary responsibility of compliance to the leader of the research effort. For example, the accuracy of any information contained in a proposal for funding is ultimately the responsibility of the PI, and so if the PI uses GenAI in the development of materials for that proposal, they must review the information in those materials and correct any omissions, errors, or otherwise inaccurate information. The PI must also understand that although resources (for example, research administration staff professionals in Cornell departments, colleges/schools, or research & innovation office units) are available to help them during this stage of the research process, these resources cannot certify to the accuracy of much of the information provided to them, and therefore cannot be expected to identify mistakes in that information, such as those generated by GenAI. The PI must also understand that they are responsible for the activities of students and research personnel working on funded projects under their supervision or mentorship, and for ensuring the appropriate use of GenAI tools by those individuals. See Appendix 0 , Prompts on Gen AI in Research for suggested discussion starters.
During this stage, individuals may desire to input information into GenAI to assist in the production of their research proposals, reports to sponsors, or even the public dissemination and translation stage documents that may have specific restrictions placed by the sponsors. Because some of this information will be highly sensitive, such as unpublished technical information or private funding data, users of GenAI tools must understand their responsibility for protecting the privacy and security of any information they input into these tools, and must seek approval to do so from the owner (such as the PI) of any such information. In fact, even in the peer review of sponsored research proposals (e.g., faculty serving on review panels for NSF or study sections for NIH), the use of GenAI may not be allowable by the sponsor (NSF Notice to Research Community, Dec 2023).
In this stage of the research process, it is also important for those who are responsible for making decisions regarding the use of sponsored funds to consider whether, and under what circumstances, it is appropriate to charge the use of GenAI tools to a research account, and to ensure their awareness with each sponsor’s requirements. Although some sponsors are clear on whether and how funds may be applied to the use of GenAI, others are not.
Guidance and Training: The nature of these tools, their potential applications, and the associated benefits and pitfalls will continue to develop and change over time, and thus, so will the appropriate guidance on how to use them. Although information and guidance should be shared with users about the risks of the use of GenAI and about which tools to avoid, it is also important to share information and training on how users can make use of these tools, how to navigate security and privacy concerns with confidence, and to provide access to tools that have been vetted and found to be aligned with the university’s expectations for security and privacy.
In this context, we suggest the following considerations as resources developed by and for the research community, including staff professionals experienced in research integrity, information systems, and user experience.
- Broad communications and outreach about GenAI in responsible conduct of research. These communications should include guidance and resources on the use of GenAI, as well as information about training, what tools to use or avoid, and references to offices and units that are available to provide support. When appropriate, this outreach should be shared by central offices and posted to central web pages – such as the recently developed Artificial Intelligence website hosted by Cornell Information Technology (CIT) that links to Cornell’s GenAI in Education report and other resources – rather than from individual units or departments, to create consistent understanding and information access across campus. Providing this type of outreach from central offices can help ensure that the university as a whole is looking to the same resources; that inquiries and concerns come to the appropriate offices; that approaches, advice, and guidance given are consistent across units; and that gaps in accessibility of information and learning are kept to a minimum.As with training on the use of, for example, animals, human participants, or biological agents in research, centralized training should be provided on the use of GenAI. This training should not only focus on risks and concerns, but on how to get the most out of these types of tools, and how to use them better. “Hackathons as Training” should make it enjoyable for researchers to gain new skills, while also contributing to the safe and responsible use of GenAI.
- Guidance on navigating mistakes made and security breaches should be communicated university-wide. It is important to acknowledge that with these new tools comes some anxiety about making mistakes in using them appropriately or even safely. To an extent, inadvertent mistakes present opportunities for education and training. However, it is also important that any mistakes that lead to security, privacy, or other concerns are handled correctly and in a timely manner. Information should be shared university-wide about Cornell’s expectations and processes with regard to what to do in the case of a potential security or other risk related to the use of GenAI, and which responsible offices should be notified.
Additional tools and resources should be developed to provide guidance. It would be beneficial to researchers and administrative staff alike to develop a GenAI-enabled tool (e.g., a form of a chatbot) that would respond to common inquiries about the use of GenAI in research. For example, “Can I use GenAI to edit my scope of work?” This tool could be populated with responses to common questions, so that consistent answers could be communicated broadly – even while appreciating that perspectives and cultural norms and even sponsor requirements and expectations may be changing fluidly in the coming years. Because such a tool would be automated and would provide immediate access to answers to these types of common questions, it would both reduce wait times and delays associated with other means of gathering this information, and reduce administrative workload in responding to these types of common requests. Similarly, resources that facilitate awareness of resource use (e.g., estimated carbon dioxide emissions associated with tool use; see Section A) can be made available at the Artificial Intelligence website and/or developed by Cornellians whose research and translation focus includes sustainability practices (e.g., Cornell Atkinson Center for Sustainability).
P erspectives and Cultural Norms
Having framed the use of GenAI in research across the stages of research above, we here summarize the perspectives that can inform our cultural norms. The widespread availability of GenAI tools offers new opportunities of creativity and efficiency, and as with any new tool depends on humans for responsible and ethical deployment in research and society. Thus, it is important that Cornell anticipates that researchers can and should use such tools appropriately, facilitates researcher access to appropriate GenAI tools and to resources to improve researchers’ “AI literacy.” It is also important that we develop shared understanding of the limits of appropriate use of specific publicly available and commercial GenAI tools, as well as the tradeoffs or risks involved in their use.
While these perspectives and cultural norms will vary reasonably among different research communities, and likely vary over time in the coming years, we offer the following summary considerations. These are considerations of both opportunity (ambitious use that may create new knowledge, insights, and impact for the world) and accountability or responsibility (duty grounded in research integrity of individuals, research teams, and institutions including Cornell). We consider these to be peer-to-peer guidelines, not a suggestion of any formalized university policy. However, we remind our fellow Cornellians that two existing policies naturally extend to use of GenAI tools in research:
- As noted in the University Privacy Statement , Cornell strives to honor the Privacy Principles : Notice, Choice, Accountability for Onward Transfer, Security, Data Integrity and Purpose Limitation, Access, and Recourse. This is noted on Cornell’s Artificial Intelligence website, along with preliminary guidelines of accountability that are discussed in this report in the context of researcher duties and research integrity.
- Cornell Policy 4.21 on Research Data Retention . Private, confidential, or proprietary data owned or controlled by Cornell may have certain contractual or legal restrictions or limitations, such as those to sponsors or collaborators, that would preclude their use in GenAI research projects. It is the researcher’s responsibility to verify/determine whether any such data sets have restrictions on such use before inputting them into public-facing GenAI and ensuring governing body compliance (e.g., IRB, IAUCC). Relatedly, any use of patient or human derived data should be disclosed to such governing body during the approval process, and any such data set should only be used in research projects upon the explicit approval of the relevant governing body on campus.
We as colleagues encourage faculty, research and administrative staff, and students to help develop the norms, technology, and public reflection on GenAI use in research, to both shape and stay current on these uses and scholarly practices. These five areas of consideration for the Cornell research community are summarized below, as part of responsible experimentation.
HELP DEVELOP THE NORMS, TECHNOLOGY, and PUBLIC LITERACY around GenAI.
- Actively develop the norms and best practices around the use of GenAI in their disciplines.
- Develop GenAI technology that is particularly suited for research (e.g., improved attribution). GenAI development for academic use should not be left solely to for-profit companies.
- Engage in GenAI public literacy efforts to foster responsible and ethical use of GenAI tools. Using at least one of these tools is enormously helpful to being part of that conversation and process, and many are freely and publicly available with associated caveats on risks of use. Table 1 provides examples of currently available GenAI tools that can be accessed (denoted as “free” to indicate no financial charge to the user). We emphasize user awareness and appropriate caution: only publicly available data should be included, and the user should assume that any entry of information by the user can be absorbed into that tool’s training set and potentially exposed to others.
STAY UP-TO-DATE with GenAI Uses and Practices
- Each research subcommunity (whether a faculty member’s research group, a department, interdisciplinary research center or institute, or college/school as those researchers see fit) gather relevant information on relevant policies by professional associations, journals and funding institutions to stay up-to-date with evolving policies, practices, and requirements in your field . Appendix 0 may serve as a discussion starter.
- Train in how to use GenAI tools in a safe, effective, and productive manner in research and innovation. Develop expertise in the potential limitations and risks of GenAI tools.
| Go to in any browser | Generates text. The free version of ChatGPT uses the OpenAI GPT-3.5 model. |
| Go to . In the Microsoft Edge browser, you can open up Bing Chat in a sidebar that gives you additional functionality, by clicking on the icon in the top right corner. For help installing Edge you may need to reach out to your IT support. | Generates text and images. With the sidebar version in Edge, allows you to ask questions about specific web pages or PDFs in the browser window. The free version of Bing Chat that is available now uses the latest OpenAI models, GPT-4 for text and DALL-E 3 for images. |
| Go to in any browswr. | Generates text. It now uses a more powerful model called Gemini. |
| Go to in any browser. | Generates images using the open Stable Diffusion XL model. |
| Go to in any browser. | Generates video from text or still images using the Runway Gen-2 model. |
Further, when acting as well-informed academic researchers with access to this research tool among others, consider the individual and shared duties of verification, disclosure, and discretion across the stages of research ideation and execution, public disclosure, translation, and funding expectations:
Duty OF VERIFICATION
- DO verify the accuracy and validity of GenAI outputs. The responsibility for research accuracy remains with researchers.
- DO check for unintentional plagiarism. GenAI can produce verbatim copies of existing work, or more subtly, introduce ideas and results from other sources but provide incorrect or missing citations.
Duty OF DISCLOSURE
- DO keep documentation and provide disclosure of GenAI use in all aspects of a research process, in accordance with the principles of research reproducibility, research transparency, authorship and inventorship.
Duty of DISCRETION
X DO NOT assume that GenAI is private. GenAI systems run on training examples, and user input and behavior are a prime source. Even if organizations that provide GenAI tools do not currently claim to use data in this way, there is no guarantee that they may not in the future.
X DO NOT share confidential, sensitive, proprietary, and export-controlled information with publicly available GenAI tools.
X DO NOT assume that GenAI output is already considered part of the public domain (e.g., not legally encumbered by copyright). GenAI tools can “memorize” their training data and repeat it with a level of verbatim accuracy that violates copyright. Even material that is not copyrighted may produce liability for corporate partners in sponsored research, if it is derived from data generated by a competitor.
C onsiderations for Cornell Leadership
We also provide considerations for Cornell leadership, particularly for aspects of GenAI preparedness and facilitated use in research and innovation that can be implemented collectively across Cornell’s colleges, schools, and campuses.
- Develop a knowledge base module, perhaps as part of responsible research conduct training resources, for rigorous, ethical and responsible use of GenAI in research and related activities. Users of GenAI tools need to understand their strengths and weaknesses, as well as regulations around privacy, special data considerations such as personally identifiable, human subject, or proprietary commercial data, and confidentiality and commercialization.
- Consider procurement of Cornell-licensed GenAI tools with data and privacy protection as facilities for research, as well as for administrative and teaching uses. Text generation and chat, program code generation, streamlined processes, and image generation would likely all be of value.
- Consider development or co-development of GenAI tools that are particularly suited for academic research use cases, including use cases in research administration services.
- Identify relevant central offices responsible for providing university-wide communications, guidance, outreach, and training to all GenAI users on various aspects of uses. To the extent that it is possible and relevant, information on the use of GenAI should be shared from central locations to encourage consistent access and understanding across the university, and to avoid siloed, inconsistent, or incorrect information.
- In support of Cornell’s public engagement mission, recognize Cornell efforts that improve GenAI public literacy beyond the university-affiliated community.
- Consider periodic updates to Cornell guidance, through a task force or other appropriate mechanisms, given the rapidly changing landscape of generative AI tools, uses, and considerations in academic research and translation of research outcomes.
A ppendix 0. Prompts on GenAI in Research (Discussion Starters or Frequently Asked Questions)
We further consider best practices and use cases in response to questions (prompts) for each of the research stages. During the task force, we simply used these prompts to stimulate early conversations and perspectives among Cornell colleagues from different fields of research and at different stages of research. The responses to such questions provided below are not prescriptive or complete, but share our initial, collective responses to such prompts as a diverse group of faculty and staff began shared discussion of this topic.
These same questions could be used within Cornell research group discussions or at department faculty meetings. For researchers to generate familiarity or insights into how these tools work to generate text or images or audio, these same prompts could be entered into multiple GenAI-enabled programs, or entered multiple times in the same GenAI-enabled program, or variations on these prompts.
A. Re search Ideation and Execution Stage
When using a tool such as ChatGPT to generate research ideas for a research project sponsored by NSF, how do the researcher and principal investigator decide on which information and ideas to enter and “share” with ChatGPT?
Any information entered into public versions of ChatGPT involves sending that data to a third-party that is under limited confidentiality and privacy restrictions (if any) with end users and not party to agreements with the NSF via data entry by other users, and the information that is entered can eventually become public. As such, where the use of ChatGPT for research idea generation does not currently violate any known general NSF policies, users should also be sure not to violate any other agreements that may exist relative to their funding sponsorship agreements, such as confidentiality, intellectual property, and entity identification clauses (e.g., mentioning NSF in any input data may be discouraged to the extent that it is in conflict with an agreement).
When using a tool such as ChatGPT to brainstorm solutions for research sponsored by a company (e.g., Samsung, Boeing, Johnson and Johnson, Google), how do the researcher and principal investigator decide what information about the project can be entered and shared?
Again, any information entered into public versions of ChatGPT involves sending that data to a third-party that is under limited confidentiality and privacy restrictions (if any) with end users and not party to agreements with corporate sponsors via data entry by other users. Furthermore, any information that is entered can eventually become public. As such, where the use of ChatGPT for brainstorming solutions does not currently violate any known general policies, users should also be sure not to violate any other agreements that may exist relative to their funding sponsorship agreements, especially including but not limited to, confidentiality and intellectual property clauses.
When using generative AI tools to summarize the literature for the introduction or discussion of a peer-reviewed article, how should the researcher and corresponding author attribute or disclose this section of a manuscript or thesis?
In general, authors and/or principal investigators have ultimate responsibility for works (including their accuracy), and furthermore, summaries should not violate plagiarism rules and regulations. Citation style guides and support websites (e.g., for APA, Chicago, Harvard) are being updated to reflect proper citations for verbatim output from generative AI and other uses. As a general practice, authors should be transparent and fully disclose uses of generative AI technologies, consistent with publication outlet, department, or area policies.
What are the conditions, if any, that a researcher should not use GenAI to generate research ideas? Examples may vary among research fields, sources of information included in a prompt including FERPA or HIPAA data, and collaborating or sponsoring organizations.
There are no general rules that prohibit the user of generative AI to generate research ideas. However, because inputs into public generative AI platforms are not confidential and data can also become public, sensitive information and individual data should never be entered for any phase of a research project, pursuant to personal data identification, re-identification, and/or chain of custody FERPA and HIPAA requirements.
In the process of research publication development, can tools such as Bard or ChatGPT be used: To summarize responses to online surveys of income level of state residents? To summarize preclinical research animal model histology? To summarize patients’ blood oxygen levels in a registered NIH study? How can the differences among these use cases be distinguished in the responsible conduct of research?
The summary of online survey data and/or other data sets can be assisted by Bard or ChatGPT to the extent that the researcher 1) does not enter confidential or data protected by other laws (e.g., HIPAA), 2) does not violate a broader agreement (e.g., between researcher, institution, host, and/or funder), and 3) has responsibility for the accuracy of the summary.
When a figure for a publication or presentation or patent disclosure is generated by AI tools (e.g., Midjourney; DeepAI), how should the principal investigator (who is typically the corresponding author or communicating inventor) verify the accuracy and intellectual ownership over the data or content of that image?
We see a distinction between cases where the author supplies all semantics and uses a tool for layout and rendering, and when a tool is used to introduce semantics such as organization of ideas or the generation of structure. Where the author supplies all semantics the use is akin to PowerPoint style suggestions or an automated layout tool, and acknowledgement is generally unnecessary unless publication policies require it. However, when GenAI introduces new semantics then we advise acknowledging its use, and checking the output carefully for accuracy. Norms around intellectual ownership are in flux at this time, and we caution authors that this poses a substantial risk.
Can images used in publications and theses be created wholly by generative AI? How does this expectation change if the GenAI-drafted images are edited by the authors? How does that vary among research disciplines? 24
Uses include generation of a cover image for a book or presentation, or images similar to clipart. We advise that authors should generally acknowledge the use of GenAI in this case, and they should carefully check images for bias and accuracy. Authors should be aware that, in the US and many other jurisdictions, it is not possible to claim copyright in non-human created works even if any human additions/edits may be copyrightable. There are unresolved legal questions regarding possible copyright infringement both as a result of the training of GenAI programs on works under copyright, and as a result of output that might closely resemble works under copyright (see, for example, the US Congressional Research Service, “Generative Artificial Intelligence and Copyright Law”, Updated September 29, 2023, https://crsreports.congress.gov/product/pdf/LSB/LSB10922 ). There is also significant variability in the acceptability of GenAI-generated images based on the publication venue.
When and how should the corresponding author inform a journal of manuscript elements created by GenAI, if not explicitly required to disclose by the publisher and when not obviously using or studying GenAI? Examples may be a proposed cover image, a graphical depiction of a new method, a graph containing research-generated data, a set of test data, generation of derivative data, etc.
GenAI technology is increasingly being built into services that provide grammar checks, polish language, and proofreading. General purpose tools such as ChapGPT are also effective for these tasks. It is not usual to acknowledge the use of checking and suggestion tools. GenAI tools, like human proofreaders who may not understand the subject matter in detail, can suggest changes that change the intended meaning so authors must still verify suggestions with care. Commercial checking and suggestion tools are being extended with GenAI features to draft entire sections or articles, or summarize texts, so authors should consider when their use crosses the line into generative use as defined above.
When and how should research group leaders (e.g., faculty) communicate these expectations of appropriate/ethical/responsible use of GenAI in research to researchers who are undergraduate students? Graduate students? Postdoctoral researchers? Other research staff?
Educating about the responsible use of GenAI should become part of the regular training on research methodology and norms of the respective discipline. This includes training that research leaders provide, but it also is a responsibility of Cornell to educate faculty and students on the affordances and pitfalls of GenAI tools.
If an invention is reduced to practice in part by use of generative AI, how should the inventors document and inform others when considering a disclosure of invention or copyright?
Any use of GenAI in the conception and reduction to practice of an invention or generation of copyrighted materials should be carefully documented and disclosed to the Center For Technology Licensing by the inventors/authors.
For example, as to the conception and reduction to practice:
- What was the GenAI tool used?
- What were the inputs to the GenAI? Do you have rights to the data used for input?
- What were the outputs of the GenAI?
- How did the outputs of the GenAI, if at all, lead to the conception of any aspect of the invention?
- Were there any difficulties encountered in using the GenAI to yield the outputs desired and, if so, which researchers updated use of the GenAI, model and/or data to yield the desired outputs?
- Which researchers substantively contributed to/controlled the development of the input and output corresponding to the invention?
If the research outcome is open-source licensable and/or posted on an open-source repository (e.g., code or algorithm or app), should and how should the researcher disclose use of GenAI in creation of the “open source” item?
Disclosure of the use of a specific GenAI tool and possibly even the origins of, and the rights to use, the input data used will likely be viewed as the standard for ethical behavior over time. Currently there are no hard and fast rules.
If the research outcome is a creative work (e.g., book, play, sculpture, musical score, multimedia exhibit) that used GenAI in the creation of that work, how should the researcher disclose that contribution in discussions of copyright?
According to Guidance published by USCO, if copyright registration is sought, the nature and extent of the use of GenAI, if containing more than de minimis AI-generated material, must be disclosed, and what part of the work was created by the researchers and what part of the work was created by the GenAI.
How should the researcher inform themselves of the uncompensated contributions of others to the GenAI output used in their own creative and/or copyrighted work or invention? How does this responsibility depend on whether the researcher derives personal financial benefit (e.g., royalties on published book) from the research outcome?
There are pending class action copyright suits by authors against entities owning GenAI tools. In those suits, GenAI tools are alleged to utilize existing copyrighted works for training without compensation to the authors. Commercial purpose is an important factor in the determination of fair use. For research that leads to commercialization and publications with financial benefits, it will be safer to use GenAI tools that are trained using only public domain works. For data that the researchers put into GenAI themselves, it’s important that they make sure they have the rights to do so regardless of whether they expect financial benefits from the output of GenAI.
If grant proposal information related to science (technical scope) and non-science (biosketch, current & pending funding) components are generated by generative AI, who is responsible for editing them before submission to a potential sponsor? Who is responsible if there are omissions or errors in those work products?
The PI is responsible for the accuracy of information related to the science, as well as for omissions and errors in that information. On the non-science side, the PI is, again, primarily responsible for the information contained in their proposal. There are resources available to help them (such as research administration staff), but those resources cannot certify the accuracy of the information provided to them, or identify mistakes in information provided to them that occurred as a result of the use of GenAI.
It is also important for the PI and their less experienced collaborators (mentees, supervised students) to discuss concerns about inputting information into GenAI tools. This information can be highly sensitive (unpublished technical information, for example) or personal to an individual (Current & Pending funding that must be disclosed to employers and sponsors but not to peers or the general public). To an extent, whether information is considered sensitive may depend on the context of the use of GenAI or of the research field itself. Consensus of this task force was that the PI is responsible for the security of his or her research information, but that anyone who intended to input information into GenAI would need to seek approval to do so from the owner of that information (such as the PI).
Should the costs of Generative AI be charged to a research account, assuming this is not disallowed by the corresponding funding agreement (i.e., not disallowed by a research sponsor)?
The appropriate source of funds for GenAI in research may depend on how the GenAI is being used. If such use is categorized in such a way that other things falling under the same type of use could be charged to a research account (e.g., software services), then it is plausible that the use of GenAI may be acceptable. In some cases, sponsors note definitively whether such charges to sponsored project accounts are allowed, but this is not always the case.
If a principal investigator becomes aware that her graduate student queried a generative AI tool (e.g., ChatGPT) with proprietary data obtained appropriately from a company when summarizing research team meeting notes, what should her next steps be? Who is responsible for notifying the company? Who is responsible for remedying the action if the company has grounds to sue for breach of the data use agreement?
The PI is responsible for what their students do in the course of their Cornell work, and is therefore responsible for ensuring that these individuals use GenAI resources appropriately. That said, mistakes are bound to happen, and they present great opportunities for education and training of both the faculty and of the students.
Further, in these situations when proprietary information is input into GenAI inappropriately, it is reasonable that the PI may feel compelled to directly report this issue to their technical contact at the company, but doing so may not align with Cornell’s processes for resolution. Therefore, we should educate faculty about the appropriate way to resolve something like this, which Cornell resources are available to them, and what offices – such as OSP or Counsel’s Office – are available to help.
What tools or approaches might Cornell researchers find useful for shared awareness of responsible GenAI use?
The use of GenAI comes with significant privacy and security concerns, and it may be important for the university to gain an understanding of the privacy policies of GenAI companies in order to determine whether they are safe to use. Also of concern are plug‑ins to GenAI programs, which may come with their own privacy and security issues.
Although Cornell should provide guidance on risks and tools to avoid, it would also be very useful to provide researchers with information about what tools and resources they can or should use, as well as access to those tools, and confirmation that they’ve been vetted and found to be secure.
The university could also provide information about the use of GenAI through other means:
- Creation of a tool – “Asking for a Friend” – which could be used to answer questions researchers may have (ex.: “Can I use GenAI to edit my scope of work?”).
- Training should not only focus on risks and concerns, we should also provide training on how to get the most out of these types of tools, and how to use them better. “Hackathons as Training” – could make it fun for researchers to gain new skills, while also contributing to the safe and responsible use of GenAI.
- The IT@Cornell web page is a centralized location that can be used to post preliminary guidelines, general information about GenAI, and what researchers need to know about it.
In order to educate researchers on the use of GenAI, communication and outreach are key. We should educate researchers about the central offices that issue training, guidance, etc. that can help them, rather than leaving them to rely on potentially siloed offices in the units that may not provide consistent advice. If the university as a whole is looking to the same resources, and inquiries consistently come to the same/appropriate offices, approaches/advice/guidance given is more likely to be consistent university-wide.
Finally, much like training on the use of other things in research (animals, human participants, biological agents, etc.), education and training should be provided on how to use GenAI safely.
A ppendix 1. Existing Community Publication Policies
We surveyed current policies regarding the use of GenAI in research from funders, journals, professional societies, and peers. We found that most of these examples were stated by journals, professional societies, and research funders, and centered around the research dissemination phase. These include the authorship and review of publications. As of fall 2023, we found relatively little policy about the “private” phases of research, such as ideation or data analysis in what Fig. 1 of our report describes as research stage A, the ideation and execution phase. In these policies, institutions tend to be cautious rather than eager to embrace the possibilities of AI.
Current policies on AI use are often cited in the context of publication, through journals, funding agencies, or professional societies that run peer-reviewed conferences. Many express an openness to the use of GenAI as a tool for writing and editing, especially in so far as it “levels the playing field” for researchers who are not native English speakers. But many also express serious concerns about generation of text beyond grammatical polishing of author-written text. Potential harms usually fall into two categories. First, AI can produce plausible-sounding information that is not, in fact, correct. The published record could become increasingly polluted by unfounded information that is extremely difficult to detect. Second, AI can produce verbatim copies of existing work, possibly causing unintentional plagiarism. More subtly, AI could introduce ideas and findings from actual published work but omit or provide inaccurate citations.
There is significant concern about responsibility. We can find no example of a journal that allows non-human authors, and several that explicitly ban such a practice, as it cannot meet the authorship criteria of accountability for the work. But there are also more subtle questions of duty. Given that the use of generative AI provides substantial risk of inappropriate output (either false or inadequately cited) and may require substantial work to fact-check, who should carry out that work, and who should be punished if it is not done adequately? There is unlikely to be a single policy throughout academia as there are many distinct cultures of collaboration and responsibility. Some fields make strong distinctions between a principal investigator (PI)/advisor’s work and PhD student work. In this case a PI may have comparatively little responsibility to check an advisee’s use of GenAI. In other fields PIs and students work collaboratively on multi-author publications, where a senior or last author may be expected to have a more supervisory (and therefore responsible) role.
Some agencies also raise the issue of sensitive data. The best current language models are beyond the capabilities of typical laptop hardware, so they are often available as a cloud-based service. While there have been differing statements about what OpenAI or Google might do with information uploaded to the systems, the bottom line is that using such tools exposes potentially sensitive information to third parties. Therefore, institutions explicitly ban entering confidential, sensitive, proprietary, and export-controlled information into publicly available GenAI tools. Similarly, grant agencies and publications prohibit using AI tools in the peer review process to avoid the confidentiality breach.
When AI tools are permitted, there is a consensus about documenting their use in research conception and execution for reporting transparency and reproducibility/replicability purposes. Most publications require disclosure of GenAI use in the Materials and Methods section of a submitted manuscript as well as in a disclosure to editors, except when AI is used as an editorial assistant for author-written text.
Living guidelines for generative AI published in Nature, https://www.nature.com/articles/d41586-023-03266-1 :
“For Researchers, reviewers and editors of scientific journals
- Interpretation of data analysis;
- Writing of manuscripts;
- Evaluating manuscripts (journal editors);
- Peer review;
- Identifying research gaps;
- Formulating research aims;
- Developing hypotheses.
- Researchers should always acknowledge and specify for which tasks they have used generative AI in (scientific) research publications or presentations.
- Researchers should acknowledge which generative AI tools (including which versions) they used in their work.
- To adhere to open-science principles, researchers should preregister the use of generative AI in scientific research (such as which prompts they will use) and make the input and output of generative AI tools available with the publication.
- Researchers who have extensively used a generative AI tool in their work are recommended to replicate their findings with a different generative AI tool (if applicable).
- Scientific journals should acknowledge their use of generative AI for peer review or selection purposes.
- Scientific journals should ask reviewers to what extent they used generative AI for their review.”
A ppendix 2. References Consulted or Cited
Conroy, G. (2023). How ChatGPT and other AI tools could disrupt scientific publishing, Nature 622 , 234-236.
Current Cornell guidance on Gen AI for use in research, education, and administration (2023): https://it.cornell.edu/ai
Current Cornell guidance on Gen AI in teaching (Nov 2023): Available for download at https://teaching.cornell.edu/sites/default/files/2023-08/Cornell-GenerativeAIForEducation-Report_2.pdf and accessible online at https://teaching.cornell.edu/generative-artificial-intelligence/cu-committee-report-generative-artificial-intelligence-education .
Bockting, C. L., van Dis, E. A. M., van Rooij, R., Zuidema, W., & Bollen, J. (2023). Living guidelines for generative AI—why scientists must oversee its use. Nature , 622 (7984), 693-696.
Davies, R. (1989). The creation of new knowledge by information retrieval and classification. Journal of documentation , 45 (4), 273-301.
Dwivedi, Y. K., Kshetri, N., Hughes, L., Slade, E. L., Jeyaraj, A., Kar, A. K., … & Wright, R. (2023). “So what if ChatGPT wrote it?” Multidisciplinary perspectives on opportunities, challenges and implications of generative conversational AI for research, practice and policy. International Journal of Information Management , 71 , 102642.
Dowling, M., & Lucey, B. (2023). ChatGPT for (finance) research: The Bananarama conjecture. Finance Research Letters , 53 , 103662. 30
European Union Commission (2023). Press release, Dec 9, 2023: Commission welcomes political agreement on Artificial Intelligence Act. https://ec.europa.eu/commission/presscorner/detail/%20en/ip_23_6473
Figure 1: Subfigure component sources were obtained from three sources: Microsoft Powerpoint; Freepix free license (patent certificate icon): https://www.flaticon.com/free-icons/certificate” title=”certificate icons . Certificate icons created by Freepik – Flaticon; and iStock (istockphoto.com) standard license with subscription (top hat icon).
Free GenAI to write your research paper with/for you: https://jenni.ai
Free GenAI to assist with literature review: https://www.semanticscholar.org/ (among many available AI-powered tools for literature review, such as Iris.ai, Microsoft Academic, Scopus AI, Elicit, Scite, and Consensus).
Glossary of GenAI-related terms. Steven Rosenbush, Isabelle Bousquette and Belle Lin, “Learn these AI basics,” the Wall Street Journal https://www.wsj.com/story/learn-these-ai-basics-39247aaf
Institutional Review Board considerations: Some research centers consider these implications as part of the scholarly effort of the research practice, such as the Center for Precision Nutrition and Health: https://www.cpnh.cornell.edu/bond-kids-1 .
King, R., & Zenil, H. (2023). Artificial Intelligence in Science: Artificial intelligence in scientific discovery: Challenges and opportunities. OECD Publishing, Paris, https://doi.org/10.1787/a8d820bd-en .
Lauer, M., Constant, S., & Wernimont, A. (2023). Using AI in peer review is a breach of confidentiality. National Institutes of Health , 23 .
Lin, B. (2023). Meta and IBM Launch AI Alliance. Dec 5, 2023, Wall Street Journal .
Luccioni, A.S., Jernite, Y., & Strubell, E. (28 Nov 2023). Power Hungry Processing: Watts Driving the Cost of AI Deployment? https://arxiv.org/pdf/2311.16863.pdf
National Science Foundation (NSF) Notice to research community: Use of generative artificial intelligence technology in the NSF merit review process (Dec 14, 2023): https://new.nsf.gov/news/notice-to-the-research-community-on-ai?utm_medium=email&utm_source=govdelivery
Nordling, L. (2023). How ChatGPT is transforming the postdoc experience. Nature , 622 (7983), 655-657.
Smalheiser, N. R., Hahn-Powell, G., Hristovski, D., & Sebastian, Y. (2023). From knowledge discovery to knowledge creation: How can literature-based discovery accelerate progress in science?. In Artificial Intelligence in Science: Challenges, Opportunities and the Future of Research . OECD Publishing. 31
Swanson, D. R. (1986). Undiscovered public knowledge. The Library Quarterly , 56 (2), 103-118.
Table 1: Content summarized in tabular form by N. Bazarova as Associate Vice Provost, Research & Innovation; and Z. Jacques as Director, Research Administration Information Services for Cornell’s Ithaca and Cornell Tech campuses, with format suggested by B. Maddox, Chief Information Officer at Cornell’s Ithaca campus.
Van Dis, E. A., Bollen, J., Zuidema, W., van Rooij, R., & Bockting, C. L. (2023). ChatGPT: five priorities for research. Nature , 614 (7947), 224-226.
Van Noorden, R., & Perkel, J. M. (2023). AI and science: what 1,600 researchers think. Nature , 621 (7980), 672-675.
Verma, P. and Ormeus, W. These lawyers used ChatGPT to save time. They got fired and fined. Washington Post , published 11/16/2023.
Waitman, L.R., Bailey, L.C., Becich, M.J., Chung-Bridges, K., Dusetzina, S.B., Espino, J.U., Hogan, R., Kaushal, R., McClay, J.C., Merritt, J.G., Rothman, R.L., Shenkman, E.A., Song, X., & Nauman, E. (2023). Avenues for strengthening PCORnet’s capacity to advance patient-centered economic outcomes in patient-centered outcomes research (PCOR). Medical Care 61(12), S153-S160.
Zewe, A. Explained: What is Generative AI? MIT News, published 11/9/2023.
A ppendix 3. Task Force Charge
The following charge was provided to the task force by Cornell’s vice president for research & innovation, Krystyn J. Van Vliet, who worked with the task force comprising membership across Cornell’s several campuses of research communities in New York state (Ithaca and Geneva, Cornell Tech, and Weill Cornell Medicine) to finalize the report prior to public release. The task force engaged a wider cross-section of the research community’s departments and disciplines through discussions during the report development, and a Cornell-internal comment period on a draft version of the report in fall 2023 engaged faculty and staff from additional departments, colleges, interdisciplinary research centers, and offices.
Charge on Generative AI in Academic Research: Perspectives and Cultural Norms
Cornell’s leadership recognizes the opportunity and challenge of generative artificial intelligence (GenAI) on academic research, as well as the communication and translation of research outcomes to research peers and broader society. The Vice President for Research & Innovation charges this task force to discuss and offer guidelines and practices for GenAI in the practice and dissemination of research. The outcome of this ad hoc task force provides clarity in establishing perspectives and cultural norms for Cornell researchers and research team leaders, as internal advice, and is not meant to be a set of binding rules.
Charge to Task Force
Generative artificial intelligence is a tool that is now widely available to the research community. Such capabilities can provide new efficiencies and insights in research, and can also introduce new quandaries for the responsible conduct of research. Faculty and senior research scientists (also called principal investigators of externally funded research) are leaders of research projects, and are thus ultimately responsible for setting and adhering to such norms – particularly when formal guidelines are nascent or disparate. Cornell now has the opportunity to discuss and establish these cultural and professional norms, consistent with our wider institutional values in responsible research across many fields.
This group of staff and faculty is charged to consider any guidelines and best practices on appropriate use and attribution of generative AI that should be shared with the Cornell research community of students, staff and faculty. This task force should identify the range of cultural norms consistent with Cornell values when using this class of tools for research. These recommendations should be communicated in a brief (<10 pages written), internal advisory report by Monday 6 November 2023.
The task force should not include extensions to Cornell education or admissions or hiring practices or institutional communications; those use cases are under consideration elsewhere.
Task Force Roster (listed alphabetically by family name)
- Natalie Bazarova Department of Communication, College of Agriculture and Life Sciences
- Michèle Belot Department of Economics, School of Industrial and Labor Relations 33
- Olivier Elemento Department of Physiology and Biophysics, Weill Cornell Medicine
- Thorsten Joachims Departments of Computer Science and Information Science, Cornell Bowers CIS
- Alice Li Cornell Center for Technology Licensing, OVPRI
- Bridget MacRae Office of Research Integrity Assurance, OVPRI
- David Mimno Information Science, Cornell Bowers CIS
- Lisa Placanica Cornell Center for Technology Licensing, OVPRI and Weill Cornell Medicine
- Alexander (Sasha) M. Rush Cornell Tech and Department of Computer Science, Cornell Bowers CIS
- Stephen Shu Dyson School, SC Johnson College of Business
- Simeon Warner Cornell University Library
- Fengqi You Smith School of Chemical and Biomolecular Engineering, College of Engineering

Wayfinder menu
- Clinical Trials
- Translational Research
Ethical Conduct of Research
Introduction, policies and forms, announcements.
The RIO oversees research integrity, compliance, misconduct, conflict management, and all elements of conducting research in an ethical and appropriate manner as per federal, state and institutional regulations.
WCM Research Integrity Policy
Research integrity is defined as:
- The use of honest and verifiable methods in proposing, performing, and evaluating research;
- Reporting research results with particular attention to adherence to rules, regulations, and guidelines; and,
- Following commonly accepted professional codes or norms.
The WCM Research Misconduct Policy can be found below.
Research Misconduct
Under WCM's Research Integrity Policy, research misconduct is defined as scientific misconduct and other conduct that seriously deviates from acceptable research practices, as explained below.
Scientific misconduct is generally defined as any act that violates the standards of integrity in proposing, performing or reviewing research or in reporting research results. Such acts include, but are not limited to:
- Fabrication means the making up of data or results and recording or reporting them.
- Plagiarism means the appropriation of another person’s ideas, processes, results or words without giving appropriate credit.
- Falsification means the manipulation of research materials, equipment or processes or changing or omitting data or results such that the research is not accurately represented in the research record.
Other conduct that seriously deviates from acceptable research practices. Examples of conduct that seriously deviates from acceptable research practices include:
- Abuse of Confidentiality means misuses of confidential information or failure to maintain the confidentiality of such information, e.g., “stealing” of information obtained through review of research proposals, manuscripts, etc.
- Violation of pertinent federal or institutional regulations, ethical codes, policies or procedures , e.g. those involving the protection of human subjects and the welfare of laboratory animals or the retention of records.
- Aiding or Facilitating acts of academic dishonesty by others.
- Breaches of research integrity other than those enumerated above that seriously deviate from those that are commonly accepted in the research community for proposing, conducting, reviewing or reporting research.
Research misconduct may also occur if an investigator is aware or recklessly disregards that members of his/her lab or in another lab are engaging in prohibited activities and does not take action to report such activities. To that end, Laboratory directors and scientific leaders must accept special responsibility for the appropriate supervision and teaching of other staff and students, and ultimately must assume responsibility for the validity of all research communications emanating from their laboratories. Carefully recorded experimental protocols and methods are strong deterrents to research misconduct. It is the responsibility of the researcher to ensure that records are maintained to adequately document the work performed.
Honest error or honest differences in interpretation or judgment of data are not regarded as research misconduct.
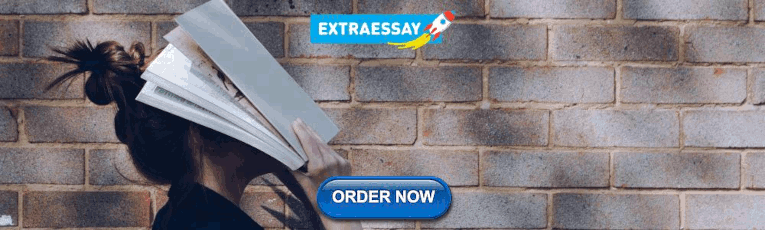
Reporting Misconduct
To have an anonymous confidential conversation regrading questions concerning research misconduct you can contact the RIO or you may contact Cornell University’s Ethics point hotline or website:
EthicsPoint hotline: (866) 293-3077 EthicsPoint website: www.hotline.cornell.edu Office email: [email protected]
General Inquiries
If you have any questions, you may contact the Research Integrity Office at [email protected] .
Ethical Conduct of Research Course
All WCM Academic staff must take the Ethical Conduct of Research (ECR) training course within 60 days of joining WCM. The training must be renewed every five years.
Click here to download i nstructions on finding the ECR course in the Learning Management System.
Responsible Conduct of Research Course for Post Doctoral Trainees and Graduate Students
The purpose of this course is to heighten the awareness of trainees to ethical considerations relevant to the conduct of research; Inform trainees of federal, state, and institutional policies, regulations and procedures applicable to the ethical conduct of research; and provide trainees with the opportunity to discuss, in a relatively informal setting, with senior faculty and among their peers, the implications of these policies and procedures for their own behavior in a research environment.
Undue Foreign Influence On Research Integrity
OSRA has developed resources to help walk you through the intricacies of foreign collaborations.
Click here to go to WCM’s Foreign Influence Tools and Resources.

July 5, 2022: On July 11, 2022, two updated University-wide policies will come into effect for all WCM faculty, staff, and students. The policies are Policy 4.21, Research Data Retention, and Policy 4.22, Export and Import Control Compliance. Click here for full memo.
Office Contacts
You are using an outdated browser. Please upgrade your browser to improve your experience.
Skip to content

- About Research
- Why Perform Research at Cornell?
Undergraduate research is an enriching process by which you meet people, gain skills and become an active member of the university community.
Undergraduate research teaches you about a field you are interested in and helps you define your interests. There is no one reason for doing research: if asked, students will tell you a myriad of answers.
Involvement in undergraduate research has many benefits:
- Learn in a unique way. You will gain practical hands-on knowledge as you develop a good research question, experimental or research design and interpretation and presentation of results. You will develop critical and creative thinking skills and develop ownership of your research project.
- Work closely with a faculty member and other research team members. Along the way, you will find mentors, friends, and perhaps even future professional colleagues. You will discover that teamwork often makes for successful research. Some undergraduate researchers even have opportunities to meet alumni, or other industry professionals, who support their work and can offer practical advice and assistance as students search for a job after graduation. Many Cornell students eventually become authors on research publications that include their work.
- Access to state of the art lab equipment and technology not usually available in classes.
- Become familiar with techniques and knowledge that will transfer beyond Cornell, and perfect your effective reading and quantitative analysis skills.
- Be surrounded by people who are passionate about their interests and learning.
- Learn teamwork in a world-class research group.
- Undergraduate research may confirm your career decisions , or may lead you to an entirely new life plan !
- Defining Research
- Student Research by the Numbers
- Is Research for You?
- In the Beginning: What to Consider
- Research Skills

- Find My GCO
- IACUC applications (Cayuse Animal Management System)
- IBC Applications (eMUA)
- IRB Applications (RASS-IRB) External
- Institutional Profile & DUNS
- Rates and budgets
- Report external interests (COI)
- Join List Servs
- Ask EHS External
- Research Development Services
- Cornell Data Services External
- Find Your Next Funding Opportunity
- Travel Registry External
- RASS (Formerly Form 10 and NFA) External
- International research activities External
- Register for Federal and Non-Federal Systems
- Disclose Foreign Collaborations and Support
- Web Financials (WebFin2) External
- PI Dashboard External
- Research metrics & executive dashboards
- Research Financials (formerly RA Dashboard) External
- Subawards in a Proposal
- Proposal Development, Review, and Submission
- Planning for Animals, Human Participants, r/sNA, Hazardous Materials, Radiation
- Budgets, Costs, and Rates
- Collaborate with Weill Cornell Medicine
- Award Negotiation and Finalization
- Travel and International Activities
- Project Finances
- Project Modifications
- Research Project Staffing
- Get Confidential Info, Data, Equipment, or Materials
- Managing Subawards
- Animals, Human Participants, r/sNA, Hazardous Materials, Radiation
- Project Closeout Financials
- Project Closeout
- End a Project Early
- Protecting an Invention, Creation, Discovery
- Entrepreneurial and Startup Company Resources
- Gateway to Partnership Program
- Engaging with Industry
Responsible Conduct of Research (RCR)
- Export Controls
- Research with Human Participants
- Research Security
- Work with Live Vertebrate Animals
- Research Safety
- Regulated Biological Materials in Research
- Financial Management
- Conflicts of Interest
- Search
Research Data Retention
Accurate and detailed research records are an essential component of any research project whether supported by University resources or by external funders. Cornell researchers (faculty, staff, and students who are involved in the design, conduct, or reporting of research at, or under the auspices of, Cornell University, including Weill Cornell Medicine), and the University share in this responsibility as outlined in the University Policy 4.21 . Each research team member needs to understand their role in data retention and their specific responsibilities.
Definition of Research Data:
Research data is defined as the recorded factual material commonly accepted in the scientific community as necessary to validate research findings, but not any of the following: preliminary analyses, drafts of scientific papers, plans for future research, peer reviews, or communications with colleagues, as defined in 2 CFR 200.315 (e) (3) .
How to comply with the Research Data Retention Policy?
To ensure researchers understand their research data retention responsibilities, Cornell researchers are required to complete a CU Learn Course “ RSRCH-OVPRI-101 Research Data Retention Policy 4.21 .” The course includes the following:
- An overview of the policy, describing its principles, to whom it applies, and the need for such a policy; and
- The required attestation that the researcher understands and will comply with the terms of the policy.
The required course will take about 5 minutes. Once completed, you will have fulfilled the attestation requirement and only be asked to complete the course in the future if there are policy changes.
Who to Contact to Review My Research Program and Data Retention Requirements?
The following resources provide support for research data retention requirements:
- The Office of Research Integrity and Assurance (ORIA) provides support regarding compliance with University Policy 4.21 .
- Cornell Data Services provides expertise in all facets of data retention, from developing data management plans to providing guidance on data disposition.
- The Office of Sponsored Programs (OSP) handles and signs incoming data use agreements .
- Center for Technology Licensing provides expertise on technology transfer agreements and intellectual property protection.
- Cornell Center for Social Sciences provides services supporting computing, secure data, research methods, and outreach.
Research Integrity
The research integrity council, responsible conduct of research committee (rcrc), responsible conduct of research (rcr) topics and case studies, rcr requirements for research awards, nih training grant requirement: rcr symposium summary, responsible conduct of research (rcr) symposium and workshop.

Snapsolve any problem by taking a picture. Try it in the Numerade app?
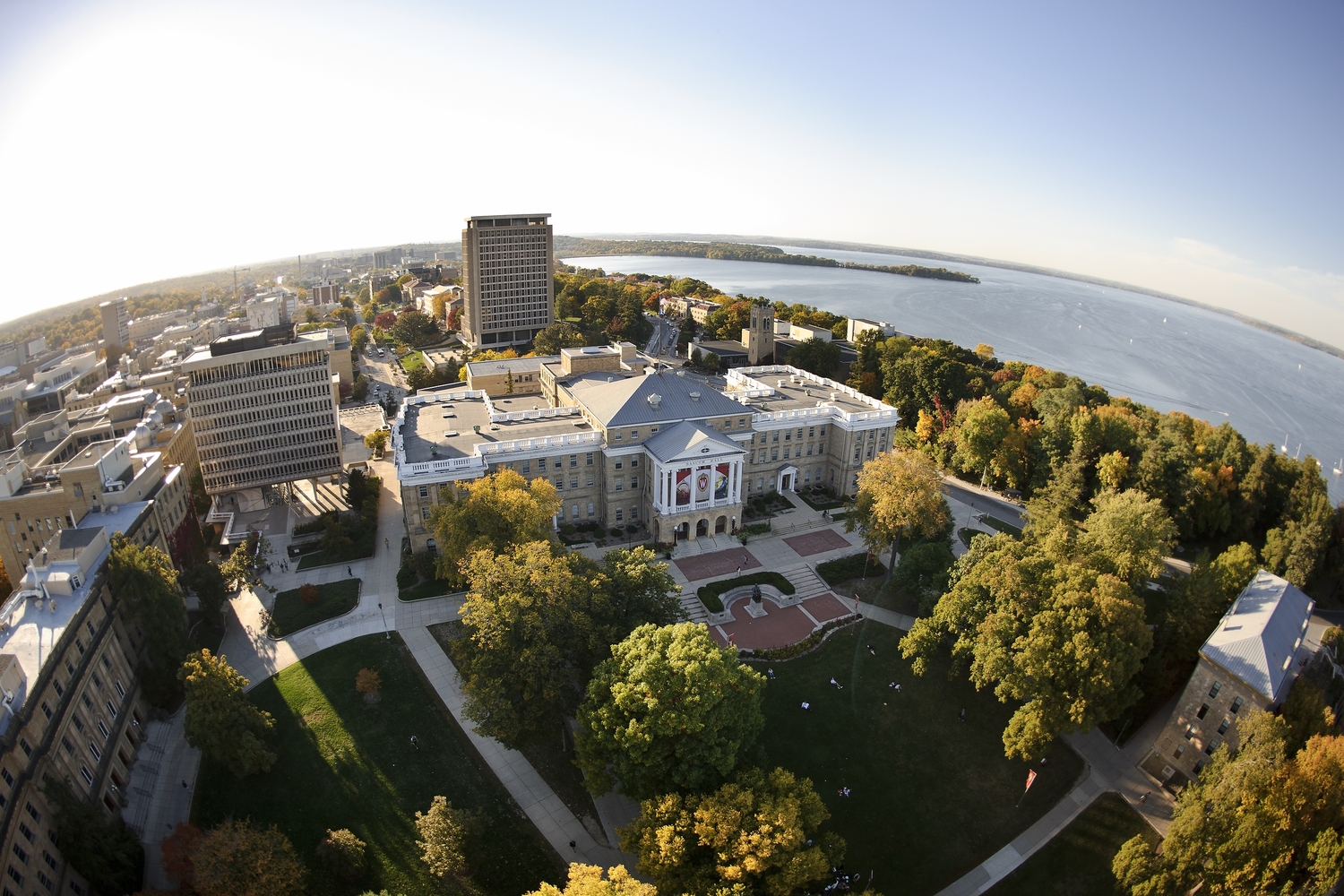
Research Specialist - Baum Lab
- Madison, Wisconsin
- VC FOR RESEARCH AND GRADUATE EDUCATION/WISCONSIN INSTITUTE FOR DISCOVERY
- Staff-Full Time
- Opening at: Aug 22 2024 at 14:00 CDT
- Closing at: Sep 15 2024 at 23:55 CDT
Job Summary:
Research Specialist to assist in chemical studies of the origins of life and astrobiology. About the research project: This NSF-funded project is entitled "From ecological dynamics to adaptive evolution during the origin of life." The overall aim is to use theoretical and laboratory studies to better understand how chemical systems on the early earth transitioned from ecological to evolutionary dynamics and how this can explain the origin of life. The Research Specialist hired would focus primarily on conducting laboratory experiments and providing technical support for other experiments conducted by other members of the team. The bulk of the work will involve assembling chemical mixtures pertinent to origins of life, conducting serial transfer experiments, and tracking changes using diverse chemical analysis methods, but especially liquid chromatography - mass-spectrometry. This research is broadly interdisciplinary and addresses one of the most significant unanswered scientific questions: How does life emerge? About Wisconsin Institute for Discovery (WID): Our mission is to discover and inspire through interdisciplinary research conducted in a dynamic, collaborative community. WID advances frontiers of knowledge, accelerates development of solutions, and engages the State of Wisconsin through interdisciplinary research. The Baum lab uses a combination of theoretical and computational research bridging chemistry, physics, ecology, and evolutionary biology with laboratory research on the emergence of ecological and evolutionary dynamics in simple chemical systems.
Responsibilities:
- 50% Conducts research experiments according to established research protocols with moderate impact to the project(s). Collects data and monitors test results
- 25% Operates, cleans, and maintains organization of research equipment and research area. Tracks inventory levels and places replenishment orders
- 10% Reviews, analyzes, and interprets data and/or documents results for presentations and/or reporting to internal and external audiences
- 5% Participates in the development, interpretation, and implementation of research methodology and materials
- 5% Provides operational guidance on day-to-day activities of unit or program staff and/or student workers
- 5% Performs literature reviews and writes reports
Institutional Statement on Diversity:
Diversity is a source of strength, creativity, and innovation for UW-Madison. We value the contributions of each person and respect the profound ways their identity, culture, background, experience, status, abilities, and opinion enrich the university community. We commit ourselves to the pursuit of excellence in teaching, research, outreach, and diversity as inextricably linked goals. The University of Wisconsin-Madison fulfills its public mission by creating a welcoming and inclusive community for people from every background - people who as students, faculty, and staff serve Wisconsin and the world. For more information on diversity and inclusion on campus, please visit: Diversity and Inclusion
Preferred Bachelor's Degree in science, preferably in the chemical or biological sciences
Qualifications:
Required: 1. At least one year of experience working in a wet-lab environment. 2. Experience with at least one kind of relevant analytical chemistry method e.g., (LC-MS, NMR). 3. Coursework or research experience in either analytical chemistry, evolutionary biology, or both. Preferred: 1. Experience in metabolomic or proteomic analysis and/or exposure to origins of life or astrobiology research.
Full Time: 100% It is anticipated this position requires work be performed in-person, onsite, at a designated campus work location.
Appointment Type, Duration:
Ongoing/Renewable
Minimum $46,000 ANNUAL (12 months) Depending on Qualifications The typical starting salary for this position is $46,000 to $63,697 depending on qualifications and experience. The full salary range for this position is $46,000 to $82,851. Employees in this position can expect to receive benefits such as generous vacation, holidays and paid time off; competitive insurances and saving accounts; and retirement benefits.
Additional Information:
It may be possible to work remotely (20 or 40%) if you have sufficient expertise with mass-spectrometry analysis and can do that work without being on site.
How to Apply:
To apply for this position, please click on the "Apply Now" button. You will be asked to upload a current resume/CV and a cover letter briefly describing how you meet the minimum and preferred qualifications for the position. You will also be asked to provide contact information for three (3) references, including your current/most recent supervisor during the application process. References will not be contacted without prior notice.
Johnny Wertz [email protected] 608-316-4496 Relay Access (WTRS): 7-1-1. See RELAY_SERVICE for further information.
Official Title:
Research Specialist(RE047)
Department(s):
A34-OVCR/WID/WI INST DISC
Employment Class:
Academic Staff-Renewable
Job Number:
The university of wisconsin-madison is an equal opportunity and affirmative action employer..
You will be redirected to the application to launch your career momentarily. Thank you!
Frequently Asked Questions
Applicant Tutorial
Disability Accommodations
Pay Transparency Policy Statement
Refer a Friend
You've sent this job to a friend!
Website feedback, questions or accessibility issues: [email protected] .
Learn more about accessibility at UW–Madison .
© 2016–2024 Board of Regents of the University of Wisconsin System • Privacy Statement
Before You Go..
Would you like to sign-up for job alerts.
Thank you for subscribing to UW–Madison job alerts!
- Open access
- Published: 19 August 2024
The impact of study habits and personal factors on the academic achievement performances of medical students
- Mohammed A. Aljaffer 1 ,
- Ahmad H. Almadani 1 ,
- Abdullah S. AlDughaither 2 ,
- Ali A. Basfar 2 ,
- Saad M. AlGhadir 2 ,
- Yahya A. AlGhamdi 2 ,
- Bassam N. AlHubaysh 2 ,
- Osamah A. AlMayouf 2 ,
- Saleh A. AlGhamdi 3 ,
- Tauseef Ahmad 4 &
- Hamza M. Abdulghani 5
BMC Medical Education volume 24 , Article number: 888 ( 2024 ) Cite this article
205 Accesses
2 Altmetric
Metrics details
Academic achievement is essential for all students seeking a successful career. Studying habits and routines is crucial in achieving such an ultimate goal.
This study investigates the association between study habits, personal factors, and academic achievement, aiming to identify factors that distinguish academically successful medical students.
A cross-sectional study was conducted at the College of Medicine, King Saud University, Riyadh, Saudi Arabia. The participants consisted of 1st through 5th-year medical students, with a sample size of 336. The research team collected study data using an electronic questionnaire containing three sections: socio-demographic data, personal characteristics, and study habits.
The study results indicated a statistically significant association between self-fulfillment as a motivation toward studying and academic achievement ( p = 0.04). The results also showed a statistically significant correlation between recalling recently memorized information and academic achievement ( p = 0.05). Furthermore, a statistically significant association between preferring the information to be presented in a graphical form rather than a written one and academic achievement was also found ( p = 0.03). Students who were satisfied with their academic performance had 1.6 times greater chances of having a high-grade point average (OR = 1.6, p = 0.08).
The results of this study support the available literature, indicating a correlation between study habits and high academic performance. Further multicenter studies are warranted to differentiate between high-achieving students and their peers using qualitative, semi-structured interviews. Educating the students about healthy study habits and enhancing their learning skills would also be of value.
Peer Review reports
Introduction
Academic performance is a common indicator used to measure student achievement [ 1 , 2 ]. It is a compound process influenced by many factors, among which is study habits [ 2 , 3 ]. Study habit is defined as different individual behavior in relation to studying, and is a combination of study methods and skills [ 2 , 3 , 4 ]. Put differently, study habits involve various techniques that would increase motivation and transform the study process into an effective one, thus enhancing learning [ 5 ]. Students’ perspectives and approaches toward studying were found to be the key factors in predicting their academic success [ 6 , 7 ]. However, these learning processes vary from one student to another due to variations in the students’ cognitive processing [ 8 ].
The study habits of students are the regular practices and habits they exhibit during the learning process [ 9 , 10 ]. Over time, several study habits have been developed, such as time management, setting appropriate goals, choosing a comfortable study environment, taking notes effectively, choosing main ideas, and being organized [ 11 ]. Global research shows that study habits impact academic performance and are the most important predictor of it [ 12 ]. It is difficult for medical students to organize and learn a lot of information, and they need to employ study skills to succeed [ 1 , 2 , 5 , 13 ].
Different lifestyle and social factors could affect students’ academic performance. For instance, Jafari et al. found that native students had better study habits compared to dormitory students [ 1 ]. This discrepancy between native and dormitory students was also indicated by Jouhari et al. who illustrated that dormitory students scored lower in attitude, test strategies, choosing main ideas, and concentration [ 10 ]. Regarding sleeping habits, Curcio G et al. found that students with a regular and adequate sleeping pattern had higher Grade Point Average (GPA) scores [ 14 ]. Lifestyle factors, such as watching television and listening to music, were shown to be unremarkable in affecting students’ grades [ 15 , 16 ]. Social media applications, including WhatsApp, Facebook, and Twitter, distract students during learning [ 16 , 17 ].
Motivation was found to be a major factor in students’ academic success. Bonsaksen et al. found that students who chose “to seek meaning” when studying were associated with high GPA scores [ 18 ]. In addition, low scores on “fear of failure” and high scores on “achieving” correlated with a higher GPA [ 8 , 18 ].
Resource-wise, Alzahrani et al. found that 82.7% of students relied on textbooks assigned by the department, while 46.6% mainly relied on the department’s lecture slides [ 19 ]. The study also indicated that 78.8% perceived that the scientific contents of the lectures were adequate [ 19 ]. Another study found that most students relied on the lecture slides (> 83%) along with their notes, followed by educational videos (76.1%), and reference textbooks (46.1%) [ 20 ]. Striking evidence in that study, as well as in another study, indicated that most students tended to avoid textbooks and opted for lecture slides, especially when preparing for exams [ 20 , 21 ].
Several researchers studied the association between different factors and academic performance; however, more is needed to know about this association in the process of education among medical students [ 15 , 20 , 22 ], with some limitations to the conducted studies. Such limitations include the study sample and using self-reported questionnaires, which may generate inaccurate results. Moreover, in Saudi Arabia in particular, the literature concerning the topic remains limited. Since many students are unsatisfied with their performance and seek improvement [ 10 ], the present study was designed and conducted.
Unlike other studies in the region, this study aims to investigate the relationship between study habits and personal factors and measure their influence on academic achievement. The results of this study could raise awareness regarding the effect of study habits and personal factors on students’ performance and would also guide them toward achieving academic success. The study also seeks to identify the factors that distinguish academically successful students from their peers.
Study design, setting, and participants
This observational cross-sectional study, which took place between June and December 2022, was conducted among students attending the College of Medicine at King Saud University (KSU), Riyadh, Saudi Arabia. Its targeted population included all male and female medical students (first to fifth years) attending KSU during the academic year 2021/2022. Whereas, students at other colleges and universities, those who failed to complete the questionnaire, interns (the students who already graduated), and those who were enrolled in the university’s preparatory year, were all excluded from the current study. The sample size was calculated based on a study conducted in 2015 by Lana Al Shawwa [ 15 ]. Using the sample size formula for a single proportion (0.79), the required sample size was 255 using a confidence interval of 95% and a margin of error of 5%. After adding a 20% margin to accommodate non-responses and incomplete responses, the calculated sample size required for this study was 306. However, our research team collected a total of 336 participants for this study to ensure complete representation.
Study instrument
The research team developed and used an electronic questionnaire. The rationale is that no standardized questionnaire measuring the study objectives was found in the literature. However, the questionnaire was tested on a pilot of 15 students to test its clarity and address any possible misconceptions and ambiguity. The study questionnaire was distributed randomly to this cohort, who were asked to fill out the questionnaire. The students reported a complete understanding of the questionnaire’s contents, so the same questionnaire was used without any modifications. The questionnaire, written in English, consisted of three parts. The first part included eleven questions about the socio-demographic status of the participants. The second part contained twenty-one questions examining personal factors such as sleep and caffeine consumption. The last part included twenty-one questions regarding students’ study habits. The questionnaire was constructed based on an ordinal Likert scale which had: strongly agree, agree, neutral, disagree, and strongly disagree as possible answers. The questionnaire was sent to participants through email and social media applications like Twitter and WhatsApp to increase the study response. An informed consent that clearly states the study’s purpose was taken from all participants at the beginning of the questionnaire. In addition, all participants were assured that the collected data would be anonymous and confidential. Each participant was represented by a code for the sole purpose of analyzing the data. Furthermore, no incentives or rewards were given to the participants for their participation.
Study variables
Socio-demographic information (such as age, gender, and academic year), and personal factors (such as motivation, sleeping status, caffeine consumption, and self-management) were the independent variables. Study habits such as attendance, individual versus group study, memorization techniques, revision, learning style, and strategies were also independent variables.
Academic achievement refers to a student’s success in gaining knowledge and understanding in various subjects, as well as the ability to apply that knowledge effectively [ 23 ]. It is a measure of the student’s progress throughout the educational journey, encompassing both academic achievements and personal growth [ 3 , 24 ]. Academic achievement is judged based on the student’s GPA or performance score. In this study, students’ GPA scores, awareness, and satisfaction regarding their academic performance were the dependent variables.
We divided the study sample into two groups based on the GPA. We considered students with high GPAs to be exposed (i.e. exposed to the study habits we are investigating), and students with low GPAs to be the control group. The purpose of this study was to determine why an exposed group of students gets high grades and what study factors they adopt. Based on this exposure (high achieving students), we concluded what methods they used to achieve higher grades. Those in the first group had a GPA greater or equal to 4.5 (out of 5), while those in the second group had a GPA less than 4.5. The students’ data were kept confidential and never used for any other purpose.
Data analysis
The data collected were analyzed by using IBM SPSS Statistical software for Windows version 24.0. Descriptive statistics such as frequency and percentage were used to describe the socio-demographic data in a tabular form. Furthermore, data for categorical variables, including different study habits, motivation factors, memorizing and revising factors, and lifestyle factors, were tabulated and analyzed using the odds ratio test. Finally, we calculated the odds ratio statistic and a p-value of 0.05 to report the statistical significance of our results.
Ethical approval and consent to Participate
Before conducting the study, the research team obtained the Ethics Committee Approval from the Institutional Review Board of the College of Medicine, KSU, Riyadh, Saudi Arabia (project No. E-22-7044). Participants’ agreement/consent to participate was guaranteed by choosing “agree” after reading the consent form at the beginning of the questionnaire. Participation was voluntary, and consent was obtained from all participants. The research team carried out all methods following relevant guidelines and regulations.
The total 336 medical students participated in the study. All participants completed the study questionnaire, and there were no missing or incomplete data, with all of them being able to participate. As shown in Table 1 9.3% of participants were between 18 and 20, 44.9% were between the ages of 21 and 22, and 35.8% were 23–28 years old. In the current study, 62.5% of the participants were males and 37.5% were females. The proportion of first-year students was 21.4%, 20.8% of second-year students, 20.8% of third-year students, 18.2% of fourth-year students, and 18.8% of fifth-year students, according to academic year levels. Regarding GPA scores, 36.9% scored 4.75-5 and 32.4% scored 4.5–4.74. 23.8% achieved 4-4.49, 6.5% achieved 3-3.99, and only 0.4% achieved 2.99 or less. Participants lived with their families in 94.6% of cases, with friends in 1.2% of cases, and alone in 4.2% of cases. For smoking habits, 86.3% did not smoke, 11% reported using vapes, 2.1% used cigarettes, and 0.6% used Shisha. 91.4% of the participants did not report any chronic illnesses; however, 8.6% did. In addition, 83% had no mental illness, 8.9% had anxiety, 6% had depression, and 2.1% reported other mental illnesses.
Table 2 shows motivational factors associated with academic performance. There was a clear difference in motivation factors between students with high and low achievement in the current study. Students with high GPAs were 1.67 times more motivated toward their careers (OR = 1.67, p = 0.09) than those with low GPAs. Furthermore, significant differences were found between those students who had self-fulfillment or ambitions in life they had ~ 2 times higher (OR = 1.93, p = 0.04) GPA scores than low GPA students. Exam results did not motivate exposed or high GPA students (46%) or control students with low GPA students (41%), but the current study showed test results had little impact on low achiever students (OR = 1.03, p = 0.88). Furthermore, 72.6% of high achievers were satisfied with their academic performance, while only 41% of low achiever students were satisfied. Therefore, students who were satisfied with their academic performance had 1.6 times greater chances of a higher GPA (OR = 1.6, p = 0.08). Students who get support and help from those around them are more likely to get high GPAs (OR = 1.1, p = 0.73) than those who do not receive any support. When students reported feeling a sense of family responsibility, the odds (odds ratio) of their receiving higher grades were 1.15 times higher (OR = 1.15, p = 0.6) compared to those who did not feel a sense of family responsibility. The p-value, which indicates the level of statistical significance, was 0.6.
Table 3 shows the study habits of higher achiever students and low achiever students. Most of the high-achieving students (79.0%) attended most of the lectures and had 1.6 times higher chances of getting higher grades (OR = 1.6, p = 0.2) than those who did not attend regular lectures. The current study found that studying alone had no significant impact on academic achievement in either group. However, those students who had studied alone had lower GPAs (OR = 1.07, p = 0.81). The current study findings reported 29.8% of students walk or stand while studying rather than sit, and they had 1.57 times higher GPA chances compared to students with lower GPAs (OR = 0.73, p = 0.27). High achievers (54.0%) preferred studying early in the morning, and these students had higher chances of achieving good GPAs (OR = 1.3, p = 0.28) than low achiever groups of students. The number of students with high achievement (39.5%) went through the lecture before the lesson was taught. These students had 1.08 times higher chances of achieving than low achiever groups of students. Furthermore, students who made a weekly study schedule had 1.3 times higher chances of being good academic achievers than those who did not (OR = 1.3, p = 0.37). Additionally, high-achieving students paid closer attention to the lecturer (1.2 times higher). In addition, students with high GPAs spent more time studying when exam dates approached (OR = 1.3, p = 0.58).
Table 4 demonstrates the relationship between memorizing and revising with high and low GPA students. It was found that high achiever students (58.9%) studied lectures daily and had 1.4 times higher chances of achieving high grades (OR = 1.4, p = 0.16) than the other group. It was found that most of the high achievers (62.1%) skim the lecture beforehand before memorizing it, which led to 1.8 times higher chances of getting good grades in this exam (OR = 1.8, p = 0.06). One regular activity reported by high GPA students (82.3%) was recalling what had just been memorized. For this recalling technique, we found a significant difference between low-achieving students (OR = 0.8, p = 0.63) and high-achieving students (OR = 1.83, p = 0.05). A high achiever student writes notes before speaking out for the memorizing method, which gives 1.2 times greater chances of getting high grades (OR = 1.2, p = 0.55) than a student who does not write notes. A major difference in the current study was that high GPA achievers (70.2%) revise lectures more frequently than low GPA achievers (57.1%). They had 1.5 times more chances of getting high grades if they practiced and revised this method (OR = 1.5, p = 0.13).
Table 5 illustrates the relationship between negative lifestyle factors and students’ academic performance. The current study found that students are less likely to get high exam grades when they smoke. Students who smoke cigarettes and those who vape are 1.14 and 1.07 times respectively more likely to have a decrease in GPA than those who do not smoke. Those students with chronic illnesses had 1.22 times higher chances of a downgrade in the exam (OR = 1.22, p = 0.49). Additionally, students with high GPAs had higher mental pressures (Anxiety = 1.2, Depression = 1.18, and other mental pressures = 1.57) than those with low GPAs.
Learning is a multifaceted process that evolves throughout our lifetimes. The leading indicator that sets students apart is their academic achievement. Hence, it is crucial to investigate the factors that influence it. The present study examined the relationship between different study habits, personal characteristics, and academic achievement among medical students. In medical education, and more so in Saudi Arabia, there needs to be more understanding regarding such vital aspects.
Regarding motivational factors, the present study found some differences between high and low achievers. Students with high GPA scores were more motivated toward their future careers (OR = 1.67, p = 0.09). The study also indicated that students who had ambitions and sought self-fulfillment were more likely to have high GPA scores, which were statistically significant (OR = 1.93, p = 0.04). This was consistent with Bin Abdulrahman et al. [ 20 ], who indicated that the highest motivation was self-fulfillment and satisfying family dreams, followed by a high educational level, aspirations to join a high-quality residency program, and high income. Their study also found that few students were motivated by the desire to be regarded as unique students. We hypothesize that this probably goes back to human nature, where a highly rewarding incentive becomes the driving force of our work. Hence, schools should utilize this finding in exploring ways to enhance students’ motivation toward learning.
The present study did not find a significant effect of previous exam results on academic performance (OR = 1.03, p = 0.88). However, some studies reported that more than half of the high-achieving students admitted that high scores acquired on previous assessments are an important motivational factor [ 15 , 25 , 26 ]. We hypothesize that as students score higher marks, they become pleased and feel confident with their study approach. This finding shows how positive measurable results influence the students’ mentality.
The present study also explored the social environment surrounding medical students. The results indicated that those who were supported by their friends or family were slightly more likely to score higher GPAs (OR = 1.1, p = 0.73); however, the results did not reach a statistical significance. We hypothesize that a supportive and understanding environment would push the students to be patient and look for a brighter future. Our study results were consistent with previous published studies, which showed an association [ 3 , 27 , 28 , 29 , 30 ]. We hypothesize that students who spend most of their time with their families had less time to study, which made their study time more valuable. The findings of this study will hopefully raise awareness concerning the precious time that students have each day.
The association of different study habits among medical students with high and low GPAs was also studied in our study. It was noted that the high-achieving students try to attend their lectures compared to the lower achievers. This was in line with the previous published studies, which showed that significant differences were observed between the two groups regarding the attendance of lectures, tutorials, practical sessions, and clinical teachings [ 31 , 32 ]. The present study found that most students prefer to study alone, regardless of their level of academic achievement (82.1%). This finding is consistent with the study by Khalid A Bin Abdulrahman et al., which also showed that most students, regardless of their GPA, favored studying alone [ 20 ].
The present study findings suggest that a small number of students (29.8%) prefer to walk or stand while studying rather than sit, with most being high achievers (OR = 1.57, P = 0.15). A study reported that 40.3% of students with high GPAs seemed to favor a certain posture or body position, such as sitting or lying on the floor [ 15 ]. These contradictory findings might indicate that which position to adopt while studying should come down to personal preference and what feels most comfortable to each student. The present study also found that high achievers are more likely to prefer studying early in the morning (OR = 1.3, P = 0.28). The authors did not find similar studies investigating this same association in the literature. However, mornings might allow for more focused studying with fewer distractions, which has been shown to be associated with higher achievement in medical students [ 3 , 15 , 33 ].
Our study also found that 39.5% of the academically successful students reviewed pre-work or went through the material before they were taught it (OR = 1.08, p = 0.75), and 25% were neutral. Similar findings were reported in other studies, showing that academically successful students prepared themselves by doing their pre-work, watching videos, and revising slides [ 3 , 9 , 34 ]. Our study showed that 75% of high-achieving students tend to listen attentively to the lecturer (OR = 1.2, p = 0.48). Al Shawa et al. found no significant differences between the high achievers and low achievers when talking about attending lectures [ 15 ]. This could be due to the quality of teachers and the environment of the college or university.
Regarding the relationship between memorizing and revising with high and low GPA students, the present study found that students who study lectures daily are more likely to score higher than those who do not (OR = 1.4, p = 0.16). This finding is consistent with other studies [ 3 , 19 , 35 ]. For skimming lectures beforehand, an appreciable agreement was noted by high GPA students (62.1%), while only (42%) of low GPA students agreed to it. Similarly, previous published studies also found that highlighting and reading the content before memorization were both common among high-achieving students [ 15 , 36 ]. Furthermore, the present study has found recalling what has just been memorized to be statistically significantly associated with high GPA students (OR = 1.83, p = 0.05). Interestingly, we could not find any study that investigated this as an important factor, which could be justified by the high specificity of this question. Besides, when it comes to writing down/speaking out what has just been memorized, our study has found no recognizable differences between high-achieving students (75%) and low-achieving students (69%), as both categories had remarkably high percentages of reading and writing while studying.
The present study has found no statistical significance between regularly revising the lectures and high GPA ( p > 0.05), unlike the study conducted by Deborah A. Sleight et al. [ 37 ]. The difference in findings between our study and Deborah A. Sleight et al. might be due to a limitation of our study, namely the similar backgrounds of our participants. Another explanation could be related to curricular differences between the institutions where the two studies were conducted. Moreover, a statistically significant correlation between not preferring the data being presented in a written form instead of a graphical form and high GPA scores have been found in their study ( p < 0.05). However, a study conducted by Deborah A. Sleight et al. indicated that 66% of high achievers used notes prepared by other classmates compared to 84% of low achievers. Moreover, their study showed that only 59% of high achievers used tables and graphs prepared by others compared to 92% of low achievers. About 63% and 61% of the students in their study reported using self-made study aids for revision and memory aids, respectively [ 37 ].
The present study also examined the effects of smoking and chronic and mental illness, but found no statistical significance; the majority of both groups responded by denying these factors’ presence in their life. A similar finding by Al Shawwa et al. showed no statistical significance of smoking and caffeine consumption between low GPA and high GPA students [ 15 ]. We hypothesize that our findings occurred due to the study’s broad approach to examining such factors rather than delving deeper into them.
High-achieving students’ habits and factors contributing to their academic achievement were explored in the present study. High-achieving students were found to be more motivated and socially supported than their peers. Moreover, students who attended lectures, concentrated during lectures, studied early in the morning, prepared their weekly schedule, and studied more when exams approached were more likely to have high GPA scores. Studying techniques, including skimming before memorizing, writing what was memorized, active recall, and consistent revision, were adopted by high-achievers. To gain deeper insight into students’ strategies, it is recommended that qualitative semi-structured interviews be conducted to understand what distinguishes high-achieving students from their peers. Future studies should also explore differences between public and private university students. Additionally, further research is needed to confirm this study’s findings and provide guidance to all students. Future studies should collect a larger sample size from a variety of universities in order to increase generalizability.
Limitations and recommendations
The present study has some limitations. All the study’s findings indicated possible associations rather than causation; hence, the reader should approach the results of this study with caution. We recommend in-depth longitudinal studies to provide more insight into the different study habits and their impact on academic performance. Another limitation is that the research team created a self-reported questionnaire to address the study objectives, which carries a potential risk of bias. Hence, we recommend conducting interviews and having personal encounters with the study’s participants to reduce the risk of bias and better understand how different factors affect their academic achievement. A third limitation is that the research team only used the GPA scores as indicators of academic achievement. We recommend conducting other studies and investigating factors that cannot be solely reflected by the GPA, such as the student’s clinical performance and skills. Lastly, all participants included in the study share one background and live in the same environment. Therefore, the study’s findings do not necessarily apply to students who do not belong to such a geographic area and point in time. We recommend that future studies consider the sociodemographic and socioeconomic variations that exist among the universities in Saudi Arabia.
Availability of data materials
The datasets used and/or analyzed during the current study are available from the corresponding author on reasonable request.
Abbreviations
Grade Point Average
King Saud University
Institutional review board
Statistical package for the social sciences
Jafari H, Aghaei A, Khatony A. Relationship between study habits and academic achievement in students of medical sciences in Kermanshah-Iran. Adv Med Educ Pract. 2019;10:637–43.
Article Google Scholar
Abid N, Aslam S, Alghamdi AA, Kumar T. Relationships among students’ reading habits, study skills, and academic achievement in English at the secondary level. Front Psychol. 2023;14:1020269.
Abdulghani HM, Al-Drees AA, Khalil MS, Ahmad F, Ponnamperuma GG, Amin Z. What factors determine academic achievement in high achieving undergraduate medical students? A qualitative study. Med Teach. 2014;36(Suppl 1):S43–48.
Muntean LM, Nireștean A, Sima-Comaniciu A, Mărușteri M, Zăgan CA, Lukacs E. The relationship between personality, motivation and academic performance at Medical students from Romania. Int J Environ Res Public Health 2022, 19(15).
Reza HM, Alireza HJIJME. Investigating study Habits of Library and Information Sciences Students of Isfahan University and Isfahan University of Medical Sciences. 2014, 14:751–757.
Kurtz SM, Silverman JD. The Calgary-Cambridge Referenced Observation guides: an aid to defining the curriculum and organizing the teaching in communication training programmes. Med Educ. 1996;30(2):83–9.
Pun J, Kong B. An exploratory study of communication training for Chinese medicine practitioners in Hong Kong to integrate patients’ conventional medical history. BMC Complement Med Ther. 2023;23(1):10.
İlçin N, Tomruk M, Yeşilyaprak SS, Karadibak D, Savcı S. The relationship between learning styles and academic performance in TURKISH physiotherapy students. BMC Med Educ. 2018;18(1):291.
McKeirnan KC, Colorafi K, Kim AP, Stewart AS, Remsberg CM, Vu M, Bray BS. Study behaviors Associated with Student pharmacists’ academic success in an active Classroom Pharmacy Curriculum. Am J Pharm Educ. 2020;84(7):ajpe7695.
Jouhari Z, Haghani F, Changiz T. Assessment of medical students’ learning and study strategies in self-regulated learning. J Adv Med Educ Professionalism. 2016;4(2):72–9.
Google Scholar
Proctor BE, Prevatt FF, Adams KSS, Reaser A, Petscher Y. Study skills profiles of normal-achieving and academically-struggling College students. J Coll Student Dev. 2006;47(1):37–51.
Kyauta AMASY, Garba HS. The role of guidance and counseling service on academic performance among students of umar suleiman college of education, Gashua, Yobe State, Nigeria. KIU J Humanit. 2017;2(2):59–66.
Eva KW, Bordage G, Campbell C, Galbraith R, Ginsburg S, Holmboe E, Regehr G. Towards a program of assessment for health professionals: from training into practice. Adv Health Sci Education: Theory Pract. 2016;21(4):897–913.
Curcio G, Ferrara M, De Gennaro L. Sleep loss, learning capacity and academic performance. Sleep Med Rev. 2006;10(5):323–37.
Al Shawwa L, Abulaban AA, Abulaban AA, Merdad A, Baghlaf S, Algethami A, Abu-Shanab J, Balkhoyor A. Factors potentially influencing academic performance among medical students. Adv Med Educ Pract. 2015;6:65–75.
Ibrahim NK, Baharoon BS, Banjar WF, Jar AA, Ashor RM, Aman AA, Al-Ahmadi JR. Mobile Phone Addiction and its relationship to Sleep Quality and Academic Achievement of Medical students at King Abdulaziz University, Jeddah, Saudi Arabia. J Res Health Sci. 2018;18(3):e00420.
Alkhalaf AM, Tekian A, Park YS. The impact of WhatsApp use on academic achievement among Saudi medical students. Med Teach. 2018;40(sup1):S10–4.
Bonsaksen T, Brown T, Lim HB, Fong K. Approaches to studying predict academic performance in undergraduate occupational therapy students: a cross-cultural study. BMC Med Educ. 2017;17(1):76.
Alzahrani HA, Alzahrani OH. Learning strategies of medical students in the surgery department, Jeddah, Saudi Arabia. Adv Med Educ Pract. 2012;3:79–87.
Bin Abdulrahman KA, Khalaf AM, Bin Abbas FB, Alanazi OT. Study habits of highly effective medical students. Adv Med Educ Pract. 2021;12:627–33.
Jameel T, Gazzaz ZJ, Baig M, Tashkandi JM, Alharenth NS, Butt NS, Shafique A, Iftikhar R. Medical students’ preferences towards learning resources and their study habits at King Abdulaziz University, Jeddah, Saudi Arabia. BMC Res Notes. 2019;12(1):30.
Abdulghani HM, Alrowais NA, Bin-Saad NS, Al-Subaie NM, Haji AM, Alhaqwi AI. Sleep disorder among medical students: relationship to their academic performance. Med Teach. 2012;34(Suppl 1):S37–41.
Hwang G-J, Wang S-Y, Lai C-L. Effects of a social regulation-based online learning framework on students’ learning achievements and behaviors in mathematics. Comput Educ. 2021;160:104031.
Gamage KAA, Dehideniya D, Ekanayake SY. The role of personal values in learning approaches and student achievements. Behav Sci (Basel Switzerland) 2021, 11(7).
Linn Z, Tashiro Y, Morio K, Hori H. Peer evaluations of group work in different years of medical school and academic achievement: how are they related? BMC Med Educ. 2022;22(1):102.
Avonts M, Michels NR, Bombeke K, Hens N, Coenen S, Vanderveken OM, De Winter BY. Does peer teaching improve academic results and competencies during medical school? A mixed methods study. BMC Med Educ. 2022;22(1):431.
Topor DR, Keane SP, Shelton TL, Calkins SD. Parent involvement and student academic performance: a multiple mediational analysis. J Prev Interv Community. 2010;38(3):183–97.
Veas A, Castejón JL, Miñano P, Gilar-Corbí R. Relationship between parent involvement and academic achievement through metacognitive strategies: a multiple multilevel mediation analysis. Br J Educ Psychol. 2019;89(2):393–411.
Núñez JC, Regueiro B, Suárez N, Piñeiro I, Rodicio ML, Valle A. Student Perception of teacher and parent involvement in Homework and Student Engagement: the mediating role of motivation. Front Psychol. 2019;10:1384.
Abdulghani AH, Ahmad T, Abdulghani HM. The impact of COVID-19 pandemic on anxiety and depression among physical therapists in Saudi Arabia: a cross-sectional study. BMC Med Educ. 2022;22(1):751.
Park KH, Park JH, Kim S, Rhee JA, Kim JH, Ahn YJ, Han JJ, Suh DJ. Students’ perception of the educational environment of medical schools in Korea: findings from a nationwide survey. Korean J Med Educ. 2015;27(2):117–30.
Ahrberg K, Dresler M, Niedermaier S, Steiger A, Genzel L. The interaction between sleep quality and academic performance. J Psychiatr Res. 2012;46(12):1618–22.
Dikker S, Haegens S, Bevilacqua D, Davidesco I, Wan L, Kaggen L, McClintock J, Chaloner K, Ding M, West T, et al. Morning brain: real-world neural evidence that high school class times matter. Soc Cognit Affect Neurosci. 2020;15(11):1193–202.
Pittenger AL, Dimitropoulos E, Foag J, Bishop D, Panizza S, Bishop JR. Closing the Classroom Theory to practice gap by simulating a Psychiatric Pharmacy Practice Experience. Am J Pharm Educ. 2019;83(10):7276.
Walck-Shannon EM, Rowell SF, Frey RF. To what extent do Study habits relate to performance? CBE Life Sci Educ. 2021;20(1):ar6.
Abdulghani HM, Alanazi K, Alotaibi R, Alsubeeh NA, Ahmad T, Haque S. Prevalence of potential dropout thoughts and their influential factors among Saudi Medical Students. 2023, 13(1):21582440221146966.
Sleight DA, Mavis BE. Study skills and academic performance among second-Year Medical students in Problem-based learning. Med Educ Online. 2006;11(1):4599.
Download references
Acknowledgements
The authors are grateful to the Deanship of Scientific Research, King Saud University, for.
support through the Vice Deanship of Scientific Research Chairs.
Author information
Authors and affiliations.
Department of Psychiatry, College of Medicine, King Saud University, Riyadh, Saudi Arabia
Mohammed A. Aljaffer & Ahmad H. Almadani
College of Medicine, King Saud University, Riyadh, Saudi Arabia
Abdullah S. AlDughaither, Ali A. Basfar, Saad M. AlGhadir, Yahya A. AlGhamdi, Bassam N. AlHubaysh & Osamah A. AlMayouf
Department of Psychiatry, College of Medicine, Imam Mohammad Ibn Saud Islamic University, Riyadh, Saudi Arabia
Saleh A. AlGhamdi
Department of Medical Education, College of Medicine, King Saud University, P.O. Box: 230155, Riyadh, 11321, Saudi Arabia
Tauseef Ahmad
Department of Medical Education and Family Medicine, College of Medicine, King Saud University, Riyadh, Saudi Arabia
Hamza M. Abdulghani
You can also search for this author in PubMed Google Scholar
Contributions
Conception or design: AHA, MAA, and HMA. Acquisition, analysis, or interpretation of data: AAB, SMA, ASA, YAA, BNA, OAA and SAA. Drafting the work or revising: TA, AHA, ASA AAB. Final approval of the manuscript: MAA, HMA., AHA, and TA. The author(s) read and approved the final manuscript.
Corresponding author
Correspondence to Tauseef Ahmad .
Ethics declarations
Conflict of interest.
The Authors declare that they have no conflict of interest.
Consent for publication
Not applicable.
Competing interests
The authors declare no competing interests.
Human ethics and consent to participate declarations
Additional information, publisher’s note.
Springer Nature remains neutral with regard to jurisdictional claims in published maps and institutional affiliations.
Rights and permissions
Open Access This article is licensed under a Creative Commons Attribution-NonCommercial-NoDerivatives 4.0 International License, which permits any non-commercial use, sharing, distribution and reproduction in any medium or format, as long as you give appropriate credit to the original author(s) and the source, provide a link to the Creative Commons licence, and indicate if you modified the licensed material. You do not have permission under this licence to share adapted material derived from this article or parts of it. The images or other third party material in this article are included in the article’s Creative Commons licence, unless indicated otherwise in a credit line to the material. If material is not included in the article’s Creative Commons licence and your intended use is not permitted by statutory regulation or exceeds the permitted use, you will need to obtain permission directly from the copyright holder. To view a copy of this licence, visit http://creativecommons.org/licenses/by-nc-nd/4.0/ .
Reprints and permissions
About this article
Cite this article.
Aljaffer, M.A., Almadani, A.H., AlDughaither, A.S. et al. The impact of study habits and personal factors on the academic achievement performances of medical students. BMC Med Educ 24 , 888 (2024). https://doi.org/10.1186/s12909-024-05889-y
Download citation
Received : 26 September 2023
Accepted : 12 August 2024
Published : 19 August 2024
DOI : https://doi.org/10.1186/s12909-024-05889-y
Share this article
Anyone you share the following link with will be able to read this content:
Sorry, a shareable link is not currently available for this article.
Provided by the Springer Nature SharedIt content-sharing initiative
- Medical students
- Study habits
- Academic achievement
- Saudi Arabia
BMC Medical Education
ISSN: 1472-6920
- General enquiries: [email protected]
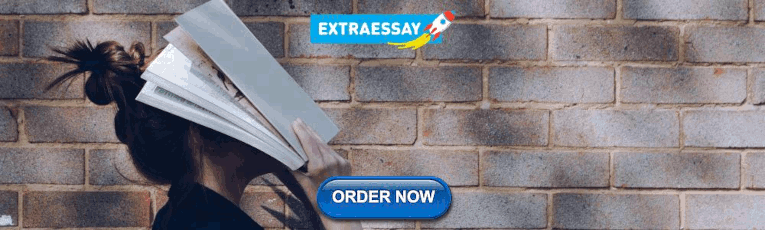
IMAGES
COMMENTS
Cornell University is a private research university that provides an exceptional education for undergraduates and graduate and professional students. Cornell's colleges and schools encompass more than 100 fields of study, with locations in Ithaca, New York, New York City and Doha, Qatar.
The integrity of research conducted at Cornell University is of the utmost importance to the institution, as well as to the public and our research sponsors. Our training and education program in Responsible Conduct of Research (RCR) is designed to meet the university's commitment to promoting and supporting the ethical and responsible conduct of research across all disciplines.
1. A research team at Cornell University conducted a study showing that approximately 10% of all businessmen who wear ties wear them so tight that they actually reduce blood flow to the brain, diminishing cerebral functions. At a board meeting of 20 businessmen, all of whom wear ties, what is the probability that a)at least one tie is too tight ...
A research team at Cornell University conducted a study showing that approximately 10% of all businessmen who wear ties wear them so tightly that they actually reduce blood flow to the brain, diminishing cerebral functions. At a board meeting of 20 businessmen, all of whom wear ties, what is the probability that at least 18 ties are not too tight?
The outcome of this ad hoc task force provides clarity in establishing perspectives and cultural norms for Cornell researchers and research team leaders, as internal advice, and is not meant to be a set of binding rules. Charge to Task Force. Generative artificial intelligence is a tool that is now widely available to the research community.
Responsible Conduct of Research is a crucial component of the education provided to students and trainees involved in Cornell research. The following topics are covered as a part of Cornell's RCR educational offerings. ... Cornell University: 2018 RCR Summer Workshop Case Studies; The Office of Research Integrity (ORI) RCR Casebook: Risky ...
Here's the best way to solve it. Ans: Use Binomial distribution with n=20 and p=0.1 P (x=k)=20Ck*0.1k* (1-0.1)20 …. 20) A research team at Cornell University conducted a study showing that approximately 10% of all businessmen who wear ties wear them so tightly that they actually reduce blood flow to the brain, diminishing cerebral functions ...
Early Career Faculty Research Funding. A majority of the research conducted at Cornell University is funded by sponsors that support research, training, and services through a variety of agreements. To maximize your likelihood of success in obtaining funding, choose a funding source and mechanism that best matches the topic, scope, and budget ...
To have an anonymous confidential conversation regrading questions concerning research misconduct you can contact the RIO or you may contact Cornell University's Ethics point hotline or website: EthicsPoint hotline: (866) 293-3077. EthicsPoint website: www.hotline.cornell.edu Office email: [email protected].
Undergraduate research is an enriching process by which you meet people, gain skills and become an active member of the university community. Undergraduate research teaches you about a field you are interested in and helps you define your interests. There is no one reason for doing research: if asked, students will tell you a myriad of answers.
A research team at Cornell University conducted a study showing that approximately $10 \%$ of all businessmen who wear ties wear them so tightly that they actually reduce blood flow to the brain, diminishing cerebral functions (Source: Chances: Risk and Odds in Everyday Life, by James Burke).
A research team at Cornell University conducted a study showing that approximately $10 \%$ of all businessmen who wear ties wear them so tightly that they actually reduce blood flow to the brain, diminishing cerebral functions (Source: Chances: Risk and Odds in Everyday Life, by James Burke). At a board meeting of 20 businessmen, all of whom ...
Question. A research team at Cornell University conducted a study showing that approximately 10% of all businessmen who wear ties wear them so tightly that they actually reduce blood flow to the brain, diminishing cerebral functions. At a board meeting of 20 businessmen, all of whom wear ties, what is the probability that no tie is too tight?
Cornell researchers (faculty, staff, and students who are involved in the design, conduct, or reporting of research at, or under the auspices of, Cornell University, including Weill Cornell Medicine), and the University share in this responsibility as outlined in the University Policy 4.21. Each research team member needs to understand their ...
Question: 4) A research team at Cornell University conducted a study showing that approximately 10% of all businessmen who wear ties wear them so tightly that they actually reduce blood flow to the brain, diminishing cerebral functions (Source: Chances: Risk and Odds in Everyday Life, by James Burke). At a board meeting of 20 businessmen, all ...
A research team at Cornell University conducted a study showing that approximately 10% of all businessmen who wear ties wear them so tightly that they actually reduce blood flow to the brain, diminishing cerebral functions (Source: Chances: Risk and Odds in Everyday Life, by James Burke).
A research team at Cornell University conducted a study showing that approximately $10 \%$ of all businessmen who wear ties wear them so tightly that they actually reduce blood flow to the brain, diminishing cerebral functions (Source: Chances: Risk and Odds in Everyday Life, by James Burke). At a board meeting of 20 businessmen, all of whom ...
Job Summary: Research Specialist to assist in chemical studies of the origins of life and astrobiology.About the research project: This NSF-funded project is entitled "From ecological dynamics to adaptive evolution during the origin of life." The overall aim is to use theoretical and laboratory studies to better understand how chemical systems on the early earth transitioned from ecological to ...
Table 3 shows the study habits of higher achiever students and low achiever students. Most of the high-achieving students (79.0%) attended most of the lectures and had 1.6 times higher chances of getting higher grades (OR = 1.6, p = 0.2) than those who did not attend regular lectures.The current study found that studying alone had no significant impact on academic achievement in either group.
See Answer. Question: 20.1 Sociology: Dress Habits A research team at Cornell University conducted a study showing that approximately 10% of all businessmen who wear ties wear them so tightly that they actually reduce blood flow to the brain, dimin- ishing cerebral functions (Source: Chances: Risk and Odds in Everyday Life, by James Burke).
The program has a 9% acceptance rate out of 67,380 applicants. 58% of students receive financial aid. This program provides a rigorous education in computer science for those interested in pursuing a career in the field. With a competitive acceptance rate, Cornell University's Computer Science program is a top choice for aspiring students in ...
Question: Check Your Understanding 5.2.20: A research team at Cornell University conducted a study showing that approximately 10% of all businessmen who wear ties wear them so tightly that they actually reduce blood flow to the brain, diminishing cerebral functions (Source: Chances: Risk and Odds in Everyday Life by James Burke).
Question: Question 15 (6 points) A research team at Cornell University conducted a study showing that approximately 10% of all businessmen who wear ties wear them so tight that they actually reduce blood flow to the brain, diminishing cerebral functions. At a board meeting of 11 businessmen, all of whom wear ties, what is the probability that ...